Pixels to pyrometrics: UAS-derived infrared imagery to evaluate and monitor prescribed fire behaviour and effects
Leo O’Neill
A
B
C
D
E
Abstract
Prescribed fire is vital for fuel reduction and ecological restoration, but the effectiveness and fine-scale interactions are poorly understood.
We developed methods for processing uncrewed aircraft systems (UAS) imagery into spatially explicit pyrometrics, including measurements of fuel consumption, rate of spread, and residence time to quantitatively measure three prescribed fires.
We collected infrared (IR) imagery continuously (0.2 Hz) over prescribed burns and one experimental calibration burn, capturing fire progression and combustion for multiple hours.
Pyrometrics were successfully extracted from UAS-IR imagery with sufficient spatiotemporal resolution to effectively measure and differentiate between fires. UAS-IR fuel consumption correlated with weight-based measurements of 10 1-m2 experimental burn plots, validating our approach to estimating consumption with a cost-effective UAS-IR sensor (R2 = 0.99; RMSE = 0.38 kg m−2).
Our findings demonstrate UAS-IR pyrometrics are an accurate approach to monitoring fire behaviour and effects, such as measurements of consumption. Prescribed fire is a fine-scale process; a ground sampling distance of <2.3 m2 is recommended. Additional research is needed to validate other derived measurements.
Refined fire monitoring coupled with refined objectives will be pivotal in informing fire management of best practices, justifying the use of prescribed fire and providing quantitative feedback in an uncertain environment.
Keywords: fire effects, fire radiative energy (FRE), fire radiative power (FRP), fire rate of spread, fuel consumption, image stabilisation, thermal imagery, unmanned aerial vehicles (UAV), uncrewed aircraft systems (UAS).
Introduction
Prescribed fires differ from wildfires in three aspects: (1) they are intentional and planned; (2) they burn with relatively lower intensities; and (3) are designed to achieve specific outcomes. During the planning phase, managers can manipulate the burn conditions (seasonality, firing pattern, weather) to achieve specific prescription objectives, such as reducing dead and down fuel loadings and understorey vegetation, retaining dominant trees and snags, and modifying forest structure and composition to the natural range of variability (Fernandes and Botelho 2003; Fulé et al. 2012; Reynolds et al. 2013; Ryan et al. 2013; Waring et al. 2016). The planning and outcome of prescribed fires has much more potential to be informed by research than full suppression wildfire operations, due to the fact that burns are conducted with the intention of achieving a desired prescription (Hiers et al. 2020). There is a growing need for research using spatially explicit tools to understand prescribed fire processes and quantitatively inform land management on treatment effectiveness and best practices (Hiers et al. 2020).
Fire behaviour is a complex interaction of many exogenous (weather, fuel loading and moisture, topography, etc.) and endogenous (fire line patterns, convective heating, fire-weather feedbacks) factors (Parsons et al. 2017; O’Brien et al. 2018; Hudak et al. 2020). The spatial pattern of fuel is a multiscale property, heterogeneous at fine (<1 m2) and coarse (>1 m2) scales (Loudermilk et al. 2012; Rowell et al. 2020). Consequently, fire is also a multiscale process, interacting with environmental factors at many scales depending on the intensity of the fire (Cruz et al. 2022). At low intensities, submeter fuel variability is linked to submeter fire variability (Loudermilk et al. 2012; Moran 2019; Cruz et al. 2022). While this spatial scale is critical for understanding ecological fire effects and processes (O’Brien et al. 2018), fire monitoring is unable to capture the detail needed to characterise these complex fire and fuel patterns (Hiers et al. 2020). Fire behaviour is often assessed by observing fire, such as estimating rate of spread by timing the fire front’s progression between points of known distances (Rothermel and Deeming 1980). Ocular estimation comes with ocular bias, restricting our understanding of fire behaviour with respect to fuels and effects.
Uncrewed aircraft systems (UAS) and new digital tools may serve to support prescribed fire management and research, as seen in other ecological applications (de Almeida et al. 2020; Jain et al. 2020; Boroujeni et al. 2024). Pyrometrics, which is the application of statistical, mathematical, and computational methods to characterise fire and fire effects, has undergone a transformation with the adoption of UAS and other sub-orbital remote sensing platforms (Nitoslawski et al. 2021). Pyrometric research using UAS and piloted aircrafts draws on theoretical and lab-tested work to produce spatially explicit measurements of fine-scale fire patterns and processes (O’Brien et al. 2015; Hudak et al. 2016; Moran et al. 2019, 2022; Bright et al. 2022; Schumacher et al. 2022). Pyrometrics derived specifically from UAS-borne imagery include energy release (Moran et al. 2022), rate of spread (Moran et al. 2019; Gowravaram et al. 2022; Schumacher et al. 2022), tree crown scorch (Moran et al. 2022), burn severity (Hillman et al. 2021), and fire behaviour interactions (Filkov et al. 2021). UAS-derived data bridges the spatiotemporal gap between satellite sensors and field data, potentially providing a cost-effective method of monitoring and studying fine-scale prescribed fire patterns.
Using infrared (IR) imagery to measure fire energy release, rate of spread, and other fire behaviour characteristics is common in fire ecology research (Wooster et al. 2005; Kremens et al. 2012; Smith et al. 2013; Mathews et al. 2016; Johnston et al. 2018; Sagel et al. 2021). However, most studies are limited to the laboratory setting or experimental burns where ample time is provided to set up equipment in a semi-controlled environment and cameras are mounted in fixed positions. To our knowledge, no research has tested the accuracy of UAS-borne IR imagery in estimating consumption of surface fuel, especially with a consumer-grade sensor. IR imagery can be used to estimate fuel consumption with multiple approaches appearing in literature (Wooster 2002; Wooster et al. 2005; Freeborn et al. 2008; Smith et al. 2013; Hudak et al. 2016; Klauberg et al. 2018). These approaches use a radiometrically calibrated IR image stack of the entire combustion process and then integrate power over time to calculate energy release. Radiative energy is then converted to fuel consumed using either the energy density or the moisture of the fuel. With the onset of UAS, using infrared imagery to measure fire behaviour has become much more attractive to research and local agencies. Consumer-grade UAS are cost-effective, accessible, and can be easily deployed for fire monitoring use. Conversely, UAS-borne (and similar crewed platforms) IR sensors often have a low saturation threshold, so maximum fire temperatures may not be measured (Moran et al. 2019). The imagery is unstable and requires colocation and registration pre-processing (Valero et al. 2021). Additionally, UAS often are limited in flight time, so post-frontal combustion may be omitted (Moran et al. 2022). Despite all these challenges, UAS may be able to deliver the missing piece of information to connect post-fire effects with pre-fire fuel, informing our understanding of fire as a mechanistic process.
We initiated this study to evaluate the accuracy of UAS to quantitatively monitor and measure prescribed fire. We collected UAS-derived radiometric, IR imagery of a calibration experimental burn and three prescribed fires to: (1) validate the accuracy of UAS-based radiative energy surface fuel consumption estimates; and (2) use extracted pyrometrics (consumption, rate of spread, and residence time) to characterise prescribed fire behaviour and effects. We expected UAS-derived IR imagery would be an accurate approach to estimating surface fuel consumption and that extracted pyrometrics would characterise the variability between and within each prescribed fire.
Materials and methods
UAS platform and imagery collection
Prior to ignition, we launched the UAS to collect images of the fire behaviour. We positioned the UAS directly overhead the centre of the plots (described in section 'Prescribed fire imagery aquisition and analysis'), facing north and at 122 m above ground, and collected thermal imagery at 0.2 Hz (5 s interval) to capture ignitions and post-frontal fuel consumption. All UAS imagery was collected with an Autel Evo II 640t UAS, featuring a 48-megapixel colour camera and a 0.32-megapixel IR camera. The thermal camera is an Infiniray Micro III Lite 640 (Yantai, Shandong, China) uncooled radiometric sensor that samples at the longwave IR wavelengths, 8–14 μm with a temperature range of 0–650°C. At 122 m, the colour and infrared sensors ground sampling distance are 1.2 and 14 cm pixel−1, respectively. With a realistic flight time of 30 min, five batteries, and field charging array, we could perpetually hover the UAS over the fire. About every 30 min, we paused thermal image sampling to swap the UAS battery, returning to the same altitude and location to resume monitoring. It is important to note we used the low gain setting to capture infrared images, which is less sensitive than the high gain but can sense at the full operational range of the camera. Saturation refers to the highest temperature at which a sensor can accurately measure. Above this point, the sensor cannot differentiate between temperatures and may provide inaccurate readings. Sensor saturation can lead to underestimation of the energy produced, which is critical to precisely estimating energy release of combusting biomass via IR sensing. The IR sensor used in this project is certified by the manufacturer to 650°C, below the maximum potential combustion temperature (Paugam et al. 2013; Moran et al. 2019) and is subject to the dependency of flame emissivity on flame depth (Johnston et al. 2014), but we selected this camera because of its affordability and simple use. While the camera limitation should be acknowledged, most prescribed fire typically involves low intensity fire, which is well below the maximum combustion temperature and IR saturation temperature (Iverson et al. 2004; Kennard et al. 2005).
Thermal imagery preprocessing
The thermal imagery acquired from the UAS in raw form was a 16-bit 1-band raster, with no geolocation information, requiring appropriate processing for quantitative spatial analysis. The three steps used to process the imagery are: (1) conversion to temperature (see Supplementary material Eqn S1); (2) stabilisation; and (3) georectification. All pyrometrics were derived from IR imagery preprocessed using the methods described, similar to Moran et al. (2019, 2022) and using equations developed and applied by O’Brien et al. (2015) and Hudak et al. (2016). After testing four stabilisation algorithms and the use of a mask to remove burning pixels, we determined an Enhanced Correlation Coefficient algorithm without a fire-mask was the best stabilising model for this study, used for all imagery hereafter (Fig. S2). The end result of the pre-processing were georeferenced, temperature-calibrated image stacks, where each pixel represents fire radiative power (FRP, W m−2) at a specified location. Each image stack can be thought of as a time-lapse of fire behaviour. FRP is then converted to fire radiative energy (FRE, MJ m2) by taking the integral of FRP over time. For more details on thermal imagery preprocessing, see Supplementary material S1.
Experimental calibration burn
We conducted an experimental burn to validate the estimates of surface fuel consumption derived from a UAS-mounted IR sensor. To do this, we burned known quantities of ponderosa fuel, measured the consumption directly by weighing fuel before and after the burn (hereafter weight-based), and compared it to consumption estimates derived from the UAS-IR imagery (hereafter UAS-based). Images were processed as described above to generate FRE of each plot. We then calculated fuel consumption using two published estimators (Eqns 1 and 2) and compared it with consumption calculated by weighing fuel before and after. This calibration burn compared the UAS-IR method of estimating consumption with a validated, ground-truth counterpart in a variety of fuel conditions.
We precisely weighed and homogeneously arranged variable amounts of dry ponderosa litter and cut logs (<15 cm in diameter) in 10 1-m2 plots. We selected coarse woody debris (CWD) and litter quantities that are representative of fuel conditions encountered in ponderosa forests, (Table 1). Roccaforte et al. (2012) reported a maximum 10 and 0.8 kg m−2 of CWD and litter loadings, given the bulk density of litter is ~4 Mg ha−1 cm−1 (Stephens et al. 2004). We doubled each of these quantities to account for the spatial heterogeneity typical of surface fuels. The design of the 10 plots was to burn across a range of fuel loads at different ratios of litter and wood. We first stratified our plots by wood weight (including moisture-related weight), arranging wood from 2 to 20 kg at 2-kg intervals at each plot. To ensure we sampled variable litter and wood ratios that were representative of the sites, we then added ponderosa needle cast collected nearby the calibration plot at weights of 0.5 kg, 1 kg, and 2 kg (Table 1). Fuel moisture was sampled immediately prior to ignitions from three wood and three litter samples and calculated as the relative loss of water content after drying in an oven at 70°C for 24-h intervals until the dry weight was stable (Keane 2015).
Plot | Dry wood weight (kg) | Dry litter weight (kg) | Wood:litter ratio | Fuel moisture (%) | Weight-based consumption (kg) | UAS-based consumption (kg) | Percent consumption (weight-based) (%) | Percent consumption (UAS-based) (%) | |
---|---|---|---|---|---|---|---|---|---|
1 | 1.83 | 0.43 | 4.3 | 11 | 2.14 | 2.44 | 95 | 108 | |
2 | 3.48 | 0.89 | 3.9 | 11 | 4.30 | 4.29 | 98 | 98 | |
3 | 5.36 | 1.78 | 3.0 | 11 | 7.00 | 7.19 | 98 | 101 | |
4 | 7.18 | 0.45 | 16 | 11 | 7.49 | 8.22 | 98 | 107 | |
5 | 8.93 | 0.90 | 9.9 | 11 | 9.64 | 10.64 | 98 | 108 | |
6 | 10.74 | 1.78 | 6.0 | 11 | 12.26 | 12.15 | 98 | 97 | |
7 | 12.53 | 0.45 | 28 | 11 | 12.70 | 13.51 | 98 | 104 | |
8 | 14.26 | 0.89 | 16 | 11 | 14.67 | 15.95 | 97 | 105 | |
9 | 16.06 | 1.78 | 9.0 | 11 | 17.50 | 18.19 | 98 | 102 | |
10 | 17.79 | 1.78 | 10 | 11 | 19.07 | 21.54 | 97 | 110 |
Plots were ignited with a flare to avoid adding fuel and energy to the plot during ignition. We collected IR imagery at 0.2 Hz, 61 m above ground for 2 h and 15 min. Consumption was immediately stopped by spraying all the plots with water and extinguishing any smouldering. After the burn, all remaining ash and charred wood was collected, dried until the weight stabilised, and weighed to calculate the total consumption following eqn 3 in Smith et al. (2005). This approach does not account for incombustible material in the fuel, the proportional combustion completeness, and potential loss of ash from convective heat and wind. A solution is to use equation 12 from Smith et al. (2005) that accounts for the entire combustion budget by calculating the loss on ignition of pre- and post-fire fuel components. Nonetheless, the simple subtraction method used here calculates fuel consumption to ~10% accuracy of the more time-consuming approach (Smith et al. 2005).
We compared methods for fuel consumption (FC) estimation using two different FRE-based approaches (Smith et al. 2013; Hudak et al. 2016):
where FC is consumption (kg m−2), hc is heat content (MJ kg−1), rf is radiative fraction, and Wc is water content (%). Eqn 1 is an energy density-based approach that accounts for the heat content of the fuel and the proportion of energy released radiatively (Hudak et al. 2016). We used an rf of 17%, the mean radiative fraction of the 12 Quercus litter and woody debris experimental burns carried out by Kremens et al. (2012). The average heat content for all ponderosa fuel components was 20.86 MJ kg−1 (Van Wagtendonk et al. 1998). Equation 2 comes from Smith et al. (2013). This moisture-based approach does not account for the effect of variable heat contents of fuel types and instead addresses the strong effect moisture has on radiative fraction and consumption (Smith et al. 2013).
Prescribed fire imagery acquisition and analysis
We collected data at three operational prescribed fires: two in Arizona; and one in Florida (Table 2). The prescribed fire sites were all composed of a fire tolerant Pinus species but displayed substantial variability in fuel loading, fire seasonality, and target fuel load reductions (Table 2). To collect data on prescribed fires in Arizona, we obtained permission from the USDA Regional Fire and Aviation Office to operate UAS and coordinated with local US Forest Service districts to attend approved prescribed fires. In Florida, the prescribed fire was on private land, so we coordinated with landowners to attend the fire. We adhered to the Federal Aviation Administration Part 107 rules and the safety regulations written in the US Forest Service research contract (when attending agency-administered prescribed fires). Sites were selected based on availability of prescribed fires during the 2022–2023 season. Prescribed fires were ignited in the Fall, Winter, and Spring and were within the target weather window described in the prescribed burn plans (see Supplementary material).
Site, state | Location (elevation, m) | Date | Forest type | Est. fuel load (kg m−2) | Avg. fuel moisture (%) | No. of plots | No. of images (IR/RGB) | IR image location error (RMSE, m) | Avg. thermal sampling time (min) | Avg. plot size (m2) | |
---|---|---|---|---|---|---|---|---|---|---|---|
Calibration Burn, AZ | 35.173°N, 111.653°W (2100) | September 2023 | Experimental | 2.5–22 | 11 | 10 | 1500 | 0.10 | 142 | 1 | |
Hanna Hammock Rx, FL | 30.660°N, 84.250°W (60) | February 2023 | Longleaf pine | 0.57 A | 15 | 2 | 800/400 | 1.93 | 38 | 4500 | |
Hundred Rx, AZ | 34.148°N, 110.813°W (2000) | April 2023 | Ponderosa pine | 3.4–4.5 B | 4.2 | 2 | 1600/800 | 1.01 | 71 | 3900 | |
Wild Bill Rx, AZ | 35.322°N, 111.783°W (2400) | May & June 2023 | Ponderosa pine | 5.9 | 4 | 5500/1400 | 1.24 | 152 | 4100 |
Prior to ignitions, we located plots that were representative of the fuel conditions of the prescribed fire sites. We placed plots near the perimeter of the fire, typically on opposite sides of the burn unit to capture multiple plots during one ignition operation. Plot size of the thermal imagery was variable, dependent on the UAS altitude, drift, and stability. Generally, plots were about 0.4 ha (Table 2). We placed four 40-cm diameter aluminium pans in each plot, one in the centre and three in the north, south, and west directions about 15–20 m from the centre pan (prioritising canopy openings to avoid occlusion). The pans acted as ground control points (GCPs), allowing us to continually fly the UAS over the same location, georectify images, and validate the georectification. Shiny aluminium contrasted the forest floor well and had a low emissivity, meaning the plates were clearly visible in the infrared and colour imagery. We used the pans to georectify the thermal image stacks and verify that the stabilisation and coregistration workflow produced acceptable results, as described below. At the Hanna Hammock Rx, we geolocated each pan using a real-time kinematics GPS with centimeter-level accuracy. At the Hundred Rx and Wild Bill Rx, we measured the distance between each GCP and inferred their coordinate position by orthorectifying pre-burn images of the plot via photogrammetry (Agisoft 2023). This method was centimetre-level accurate as well, but the geolocation was subject to the precision of the UAS GPS. We recorded the average daily fuel moisture of 10-h fuels from the nearest Remote Automated Weather Station, available from the Western Regional Climate Center (http://www.raws.dri.edu).
We used the best performing method from the calibration burn and the moisture-based approach (Eqn 2), to convert FRE to consumption for the prescribed fire plots. One challenge with monitoring with UAS is capturing post-frontal combustion (Moran et al. 2022), which may continue for hours or days after the burn. We balanced collecting as many plots as possible with capturing all possible post-frontal consumption by sampling about two plots per operational shift, each plot being sampled until radiant energy release had substantially subsided. To estimate post-frontal combustion, we fit a linear regression with a logarithmic transformation of the plot FRP at each infrared imagei in the image stack. Linear regressions were calculated for each burn:
where t is time. We fit the linear regression to the energy release from the peak energy release to the end of the image capture, which visually exhibited a logarithmic relationship. We then used this model to predict unmeasured energy release of the plots from when data collection stopped to when the theoretical energy release ≤1 W m−2. Comparing the total energy (FRE) measured with the predicted unmeasured energy release gave us a corrective factor, which we applied to each consumption raster to account for any unmeasured energy. For example, if the regression predicted 20% of energy was not captured during collection, then each pixel would be multiplied by 1.2.
To estimate rate of spread, we used the masked FRP raster stack to generate an arrival time, or progression, raster of the fire when each pixel reached flaming power, 1070 W m−2 (Hudak et al. 2016). The rate of spread was then derived as the rate of change or slope (radians) of the progression raster (Moore et al. 2020) using the equation:
where ROS is measured in m min−1. The slope raster was then converted to points and interpolated for all progression pixels using inverse distance weighing. Residence time was calculated as the total time (in seconds) that each pixel was greater than or equal to 300°C (Wotton et al. 2012).
Statistical analysis
To evaluate the effectiveness of UAS-IR imagery at capturing the ‘signal’ of prescribed fires, we assessed whether the spatial resolution could adequately characterise fire behaviour variability. In signal processing, the Nyquist criterion states the sampling interval (or resolution) should be twice that of the frequency in the sample (Shannon 1949; Hengl 2006). Therefore, we evaluated the resolution of the ‘signal’ to determine the resolution needed of the sensor. We constructed a semi-variogram of each plot’s FRE raster to determine the spatial scale where autocorrelation was no longer significant. Then, we calculated the median semi-variogram range of all the plots and resampled each pyrometric raster to this value. We confirmed that autocorrelation was reduced with a Moran's I-test. These spatially-independent samples of all three pyrometrics were then compared to assess differences among prescribed burns with a non-parametric Kruskal–Wallis test. If the Kruskal–Wallis test indicated significant differences, we used the Dunn test, a post hoc test for non-parametric data, to determine specifically which burns were different from one another.
Results
We successfully collected 16 h of imagery of fire behaviour, stabilising and processing it into spatially explicit pyrometrics from three prescribed fires and one calibration burn. The average plot size of the thermal imagery was 0.41 ha (approximately square with 64-m sides) and the average duration of fire captured was 87 min (Table 2). The longest duration of thermal imagery was 3.5 h, captured at plot 3 of Wild Bill prescribed fire.
UAS-derived consumption
Observed weight-based consumption of the 10 1-m2 plots ranged from 2.1 to 19 kg m−2 with all plots consuming 95% or more of dry weight fuel (Table 1). We measured average litter and wood fuel moisture as 11%. UAS-based consumption estimates were in high agreement with observations across all plots (Fig. 1). Converting radiative energy using either Eqns 1 or 2 to consumption was highly correlated with observed consumption. However, using equation 1 from Hudak et al. (2016) consistently underpredicted consumption. The linear regression of observation as the response had a slope of 0.77 (R2 = 0.99). Converting FRE using the fuel moisture approach from Smith et al. (2013), Eqn 2 was closer to a 1:1 relationship with a linear regression slope of 1.1 (R2 = 0.99). Therefore, all estimates of consumption for the prescribed fires (Table 2) were generated with the Smith et al. (2013) equation (Eqn 2).
Calibration burn results from weight-based measurements of consumption and UAS-based consumption (left plot). Brown points (triangles) use Eqn 1 from Hudak et al. (2016) and green points use Eqn 2 from Smith et al. (2013). Linear regressions of observed as a function of predicted (observed ~ predicted consumption, solid lines) reveal a slight overprediction when applying Eqn 2 and an underprediction when applying Eqn 1. The density plot (right plot) highlights the variability of fire radiative energy (FRE) pixels of plot 1 (left image) and 10 (right image), with remaining plots represented as lines.
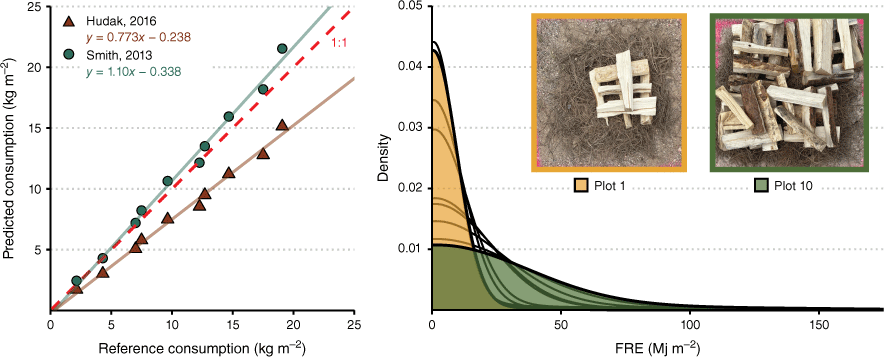
UAS-IR fire behaviour
Extracted pyrometrics from the UAS-derived IR imagery agreed with qualitative ocular observations of the burns: Hanna Hammock spread the fastest; and Hundred and Wild Bill prescribed fires consumed more fuel with longer residence times (Fig. 2). Predicted FRE using Eqn 3 to account for unmeasured FRP performed well, with strong model fits with the captured energy (Fig. 3). Plots where the UAS imagery collection captured enough post-frontal combustion that a decay curve was evident had the strongest model performance. At the Hanna Hammock plots, majority of the energy was released within 10-min, resulting in most energy being directly measured and only a minor fraction modelled with Eqn 3. The prescribed burns in Arizona continued to release significant energy for hours after the peak FRP and therefore the regression-based estimates of energy release were critical for estimating total energy released and consumption. Average total consumption for Hanna Hammock, Hundred, and Wild Bill were 0.3, 1.4, and 4.9 kg m−2, respectively. The consumption data was heavily positively skewed (Fig. 4). Maximum within-plot consumption was 65 kg m−2, sampled at plot 1 of the Wild Bill Rx. Median rate of spread was 2.5, 0.7, and 0.7 m min−1 for Hanna Hammock, Hundred, and Wild Bill prescribed fires, respectively. Rate of spread reported by the UAS imagery ranged from 0.002 to 4.0 m min−1, the maximum recorded at Plot 1 of Hanna Hammock and the minimum recorded in plot 4 of Wild Bill prescribed fire. Median residence time was 40, 96, and 170 s for Hanna Hammock, Hundred, and Wild Bill prescribed fires, respectively.
Location and images of fire behaviour of the prescribed fires monitored (a), selected IR images and derived rate of spread raster of the Hanna Hammock plot 1 (b), and selected IR images and derived consumption raster of the Wild Bill plot 3 (c). Hanna Hammock Rx had the highest visually observed rate of spread and lowest consumption. Wild Bill Rx had a large amount of coarse woody debris present in plots and had the highest visually observed estimate of consumption and residence time.
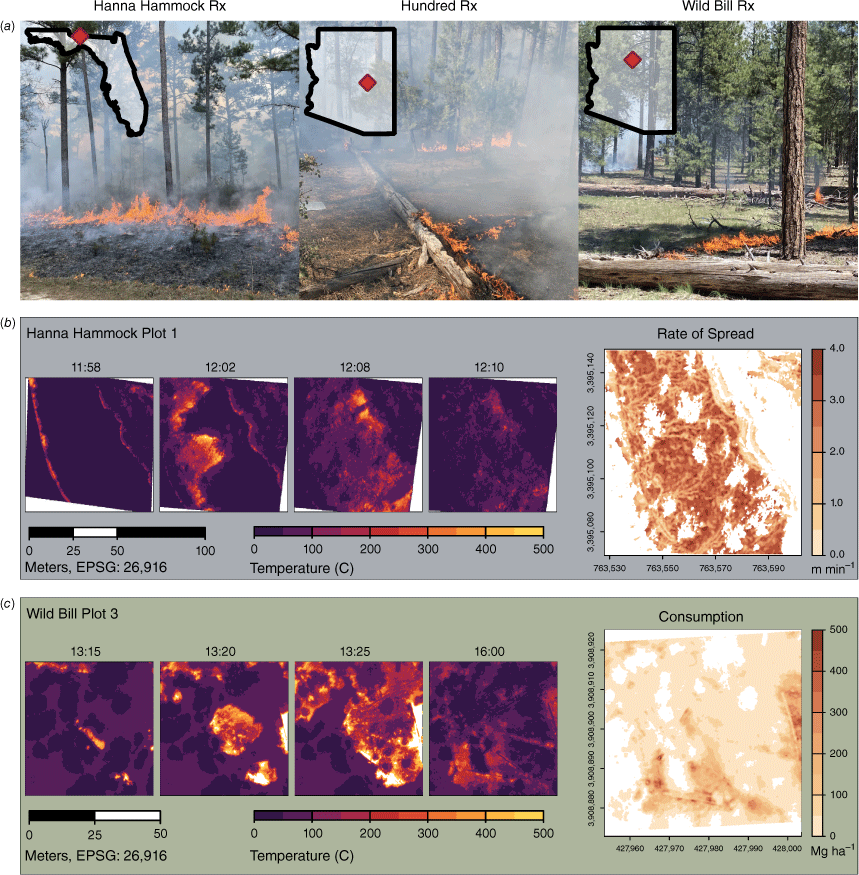
Fire radiative power (W m−2) over time of each of the burn plots. Red dashed line is the logarithmic transformed linear regression and respective R2 values denote model performance evaluated with measured energy data. Dark shaded area is the energy measured by the UAS-IR imagery, lightly shaded area is unmeasured energy predicted using the regression. Measured and predicted energy values were summed in the final consumption estimates. The green fine-dashed line in Wild Bill 4 plot represents a long pause (~1 h) in imagery collection during minimal, static fire activity. This particular plot was burned in early morning when conditions were relatively cool and wet, so fire intensity was particularly low. Ignition operations then made a second pass at 150 min, corresponding to the spike in fire radiative power.
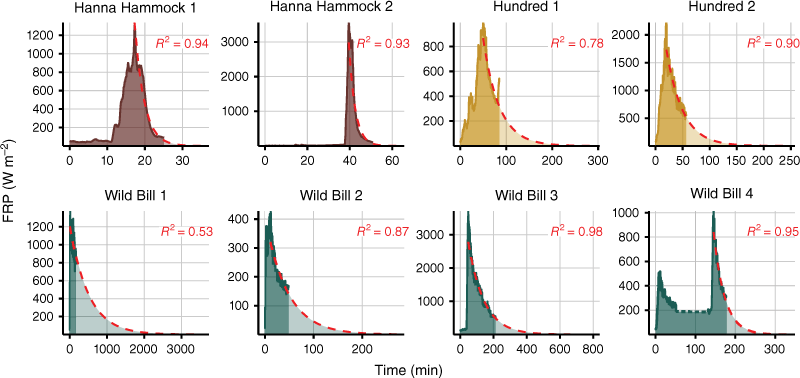
Violin distributions and boxplots of UAS-derived pyrometrics from each prescribed fire: Hanna Hammock Rx, Hundred Rx; and Wild Bill Rx. A significant Kruskal–Wallis rank sum test that two or more of the distributions are different is marked as an asterisk on the x-axis label. Post hoc Dunn tests to determine pairs of significantly different distributions are marked as asterisks on the y-axis label.
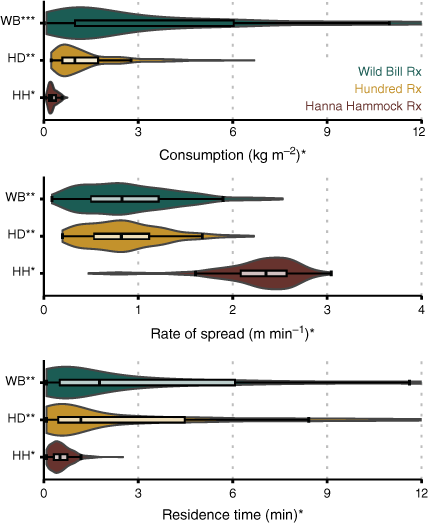
Using a semi-variogram to identify autocorrelation for each plot’s radiative energy (FRE) raster, we found the median range to be 4.6 m. Before resampling, FRE rasters of each plot had a median resolution of 0.13 m (Table 2) and Moran’s I-value of 0.96, indicating a very strong positive autocorrelation. 35 pixels were resampled to 4.6 m resolution to minimise autocorrelation. Therefore, the Nyquist sampling theorem would suggest a sampling resolution of 2.3 m or less is needed to characterise the fire behaviour of prescribed fires. After spatial resampling (to 4.6 m), median Moran’s I-value was reduced to 0.29: while spatial autocorrelation was still present, it was markedly reduced. A Kruskal–Wallis test of the resampled pyrometric data showed that each pyrometric had significantly different groups (Fig. 4). The post hoc Dunn tests showed that Wild Bill and Hundred groups were similar in terms of rate of spread or residence time distributions (Fig. 4), though all other Dunn tests were significant (P < 0.025).
Discussion
The preprocessing workflow developed to calibrate, stabilise, and georeference UAS-IR imagery was effective for deriving pyrometrics. We converted IR emittance to temperature using a bench-tested algorithm (Eqn S1) that performed well (R2 = 0.99). Then, we selected the ECC-affine transformation model to stabilise image stacks with sub-meter drift. Finally, we georeferenced image stacks using GCPs with 1 m-level precision (average RMSE = 1.1 m).
Our calibration experiment demonstrated that estimating fuel consumption using UAS-IR imagery was accurate in a range of fuel loading conditions and could be applied to the prescribed fire IR imagery. The fuel moisture-based equation from Smith et al. (2013) (Eqn 2) was the best approach for our purposes. While reliable and often time consuming, traditional sampling methods for estimating consumption are not spatially explicit and are less accurate (Lutes et al. 2006; Sikkink and Keane 2008). This research suggested that UAS-IR imagery is an alternative option to measuring fuel consumption. While the calibration experiment was representative of the prescribed fire fuel and monitoring conditions, additional research is needed to validate in-situ consumption estimates in a range of fuel types, moisture contents, and arrangements.
Caution should be exercised when selecting a method to converting fire radiative energy to consumption. Previous research by Hudak et al. (2016) found the energy approach (Eqn 1) underestimated consumption, aligning with our results (Fig. 1). One possible source for the underestimated consumption was the fuel moisture present. In the context of thermal signal saturation, these results are also limited to the sensor tested because of the relatively low thermal sensor saturation temperature. While it is possible that the plots may have burned at temperatures greater than the saturation point, the high precision of Fig. 1 results suggest the sensor did not reach saturation or saturation was equal for each plot.
Pyrometrics derived from UAS imagery proved to be useful for prescribed fire evaluation and monitoring. Three prescribed fires were examined using thermal infrared imagery, yielding spatially explicit measurements of consumption, rate of spread, and residence time. From a qualitative standpoint, these measurements were consistent with ocular estimates of fire behaviour and effects, highlighting discernible variability among the burns (Fig. 4). The semivariograms illustrated that prescribed fire behaviour measured at each plot occurs at moderate scales (<4.6 m). The Nyquist Criterion states that to accurately sample a continuous signal and reconstruct it without aliasing, you must sample at least twice the frequency (or resolution) of the highest frequency component in the signal, suggesting sensor resolutions of less than 2.3 m are ideal. As prescribed fire is often affected by fine-scale processes, sampling at high temporal (>0.1 Hz) and spatial (<1 m2) resolutions is imperative to capture the heterogeneity of pyrometrics (Moran et al. 2019). The sampling resolution will need to be considered for each fire and fuel type. For example, sampling at lower resolutions for moderate intensity, slow spreading fires in forested ecosystems will likely still capture the heterogeneity of fire behaviour. In grass-dominant fuel types though, high temporal sampling is critical to capture the brief peak in radiative power release when the grass fuel is combusting. We sampled fire behaviour at sub-meter resolutions, ensuring detailed spatial variability was captured. The Kruskal–Wallis and post hoc tests of the aggregated pyrometrics illustrated the variability of fire captured and also corroborated that our approach can effectively detect this variability and differentiate between prescribed burn pyrometrics (consumption, rate of spread, and residence time). While the intensities of the prescribed fires sampled were variable, fire effects were characteristic of low severity fire in their respective fire regimes (Table 2) (Varner et al. 2005; Fulé et al. 2012). These sites captured a variety of prescribed fire dynamics in fire tolerant Pinus forests, representative of the many scenarios encountered in the US prescribed fire program. Future research could build off this work, testing this process in higher fuel loads and in other forest types.
We found that two out of the three evaluated prescribed fires likely achieved their objectives as far as can be determined from the 2–4 study plots in each fire. However, some of the burns lacked specific objectives related to fuel consumption. Hanna Hammock showcased the fastest rate of spread (peak and average spread) and lowest consumption (Fig. 4). Given a pre-fire fuel loading of 0.57 kg m−2 (Table 2) and the consumption objective of 95% fuel reduction (see Supplementary material for details on burn objectives), desired consumption was 0.54 kg m−2. We estimated consumption averaged 0.3 kg m−2, 56% the desired objective. At this particular burn, adjacent research sampled pre- and post-burn fuel loading data of the site using 25 0.25 m2 clip-plots (S. W. Bigelow, pers. comm.). Field fuel sampling inferred consumption to be 0.38 kg m−2, or 66% of pre-burn fuel mass. This adjacent study corroborates our consumption estimate and presents solid evidence that the desired outcome of the burn, while stringent, was not achieved. At the Hundred Rx, 50–85% reduction in fuel loading was desired, approximately equal to 1–2 kg m−2. UAS-IR consumption at the two plots sampled was estimated to be 1.1 and 1.6 kg m−2, so consumption objectives were likely achieved. The Wild Bill Rx burn had significantly higher consumption than the other burns, estimated at 4.9 kg m−2. Unfortunately, the burn plan only specified post-fire fuel loading (1.1–1.6 kg m−2) and not pre-fire fuel conditions or consumption, so direct comparisons were challenging. Given our consumption estimate and the desired post-fire fuel loading specified in the objectives, estimated consumption would equate to 75–81%. In similar forest types, desired consumption was specified to be 50–85% (Hundred Rx) and 40–80% (Waring et al. 2016) reduction in fuel loading. So, it is plausible that the Wild Bill Rx burn achieved consumption objectives, although there remains a degree of uncertainty.
A limitation of our study and the use of aerial infrared sensors was the occlusion of the thermal signal from tree canopy. Canopy foliage intercepted the thermal radiation of the fire, so we corrected for occluded radiative energy by masking pixels that did not exceed 1070 W m−2 (see Supplementary material for more details). While the effect of canopy cover on radiative energy can be accounted for (Hudak et al. 2016; Mathews et al. 2016), caution should be exercised when estimating FRE in densely forested areas, especially when surface fuels are heterogeneous. Without the addition of other sources of sub-canopy fuel characteristics (lidar or ground measurements), our approach to monitoring prescribed fire requires unobstructed view of the surface fuels. We suggest our approach only be applied in areas with less than 75% canopy cover (which we adhered to in our study) unless sub-canopy fuels are accounted for. Additionally, caution should be exercised when interpreting our results of rate of spread and residence times since these measurements were only compared to ocular estimates of fire behaviour as a means of validation at the eight prescribed fire plots. Future research quantitatively validating UAS-IR measurements of rate of spread and residence time and accounting for sub-canopy fire behaviour is recommended.
Researching prescribed fire during active incidents presented significant challenges. Often, burns were delayed, relocated, and difficult to access. Attending prescribed fires with UAS required extensive authorisation from the responsible agency, and Red Card qualified and FAA Part 107 certified researchers to operate a UAS over an active incident. The prescribed fires attended had a narrow window of favourable weather conditions and 1–3 day’s notice of location and ignition time was typical. Collecting ground measurements was not possible with the limited time and resources available to us, though these scenarios represent the situations in which UAS may be implemented to monitor fire effects. Increasing our knowledge of prescribed fire and application of innovative technologies will require more research on active incidents and active collaboration between practitioners and researchers. Research-led development of software to automatically process UAS-IR imagery could provide faster results of prescribed burn effectiveness. Deriving and evaluating initial or rapidly-estimated pyrometrics directly from raw thermal images without extensive post-processing is a necessary research thread for achieving real-time pyrometrics and instantaneous feedback to managers.
Operationally, our findings support the efficacy of UAS-derived pyrometrics for monitoring and evaluating prescribed fires, specifically consumption, rate of spread, and residence time. We noted that the burn plans of the fires we attended contained broad objectives with wide ranges of desired outcomes. Refined fire monitoring coupled with refined objectives will be pivotal in informing fire management of best practices, justifying the use of prescribed fire, and providing quantitative feedback in an environment saturated with uncertainty. The application of prescribed fire is often subject to constraints due to concerns of disciplinary experts within agencies about the effect of fire on natural and cultural resources. Interdisciplinary cooperation would help prescribed fire to achieve objectives beyond fuel reduction. For example, detailed UAS-derived pyrometrics could be translated into estimates of belowground heat penetration (heat per unit area and residence time), affecting seed banks and root tissues, as well as aboveground energy release affecting canopy scorch and bole char (O’Brien et al. 2018). Wildlife habitat (Mason and Lashley 2021) and hydrological impacts (Flerchinger et al. 2016; Williams et al. 2020) can also be linked to fine-scale fire effects. The effect of prescribed burns on cultural resources such as fire-sensitive archaeological sites could be monitored in detail, allowing managers to assess whether pre-fire protection efforts such as clearing adjacent fuels were successful (Ryan 2010).
Data availability
Data used to generate results of this study are available by request from the authors and through an online open-access portal (Hopkins B, O’Neill L, Marinaccio M, Rowell E, Parsons R, Flanary S, Nazim I, Afghah F, in prep.). Additionally, the code is available publicly on github (https://github.com/leo-oneill/Pyrometrics).
Conflicts of interest
Co-author Peter Fulé is an Associate Editor and did not take part in any editorial decisions about this manuscript to mitigate any conflict of interest. This has not affected our research or the peer-review process and the authors have no other conflicts of interest.
Declaration of funding
This research was funded by National Science Foundation (CPS-2039026) and Salt River Project (Award #8200007407).
Acknowledgements
This project would not have been possible without the help of multiple organisations and individuals including Preston Mercer and True Brown from Coconino National Forest; Jeremiah Boyd from Interagency Aviation Management Office; Karen Cummins, Morgan Varner, Seth Bigelow, and Vanessa Niemczyk from Tall Timbers Research Station; Andrew Nordquist from Tonto National Forest; and Floyd Hardin from Salt River Project.
References
Agisoft (2023) Metashape Professional. Available at https://www.agisoft.com
Boroujeni SPH, Razi A, Khoshdel S, Afghah F, Coen JL, ONeill L, Fule PZ, Watts A, Kokolakis N-MT, Vamvoudakis KG (2024) A comprehensive survey of research towards AI-enabled unmanned aerial systems in pre-, active-, and post-wildfire management. ArXiv Available at https://arxiv.org/abs/2401.02456.
| Google Scholar |
Bright BC, Hudak AT, McCarley TR, Spannuth A, Sánchez-López N, Ottmar RD, Soja AJ (2022) Multitemporal lidar captures heterogeneity in fuel loads and consumption on the Kaibab Plateau. Fire Ecology 18, 18.
| Crossref | Google Scholar | PubMed |
Cruz MG, Alexander ME, Fernandes PM (2022) Evidence for lack of a fuel effect on forest and shrubland fire rates of spread under elevated fire danger conditions: implications for modelling and management. International Journal of Wildland Fire 31, 471-479.
| Crossref | Google Scholar |
de Almeida DRA, Stark SC, Valbuena R, Broadbent EN, Silva TSF, de Resende AF, Ferreira MP, Cardil A, Silva CA, Amazonas N, Zambrano AMA, Brancalion PHS (2020) A new era in forest restoration monitoring. Restoration Ecology 28, 8-11.
| Crossref | Google Scholar |
Fernandes PM, Botelho HS (2003) A review of prescribed burning effectiveness in fire hazard reduction. International Journal of Wildland Fire 12, 117-128.
| Crossref | Google Scholar |
Filkov A, Cirulis B, Penman T (2021) Quantifying merging fire behaviour phenomena using unmanned aerial vehicle technology. International Journal of Wildland Fire 30, 197-214.
| Crossref | Google Scholar |
Flerchinger GN, Seyfried MS, Hardegree SP (2016) Hydrologic response and recovery to prescribed fire and vegetation removal in a small rangeland catchment. Ecohydrology 9, 1604-1619.
| Crossref | Google Scholar |
Freeborn PH, Wooster MJ, Hao WM, Ryan CA, Nordgren BL, Baker SP, Ichoku C (2008) Relationships between energy release, fuel mass loss, and trace gas and aerosol emissions during laboratory biomass fires. Journal of Geophysical Research: Atmospheres 113, D01301.
| Crossref | Google Scholar |
Fulé PZ, Crouse JE, Roccaforte JP, Kalies EL (2012) Do thinning and/or burning treatments in western USA ponderosa or Jeffrey pine-dominated forests help restore natural fire behavior? Forest Ecology and Management 269, 68-81.
| Crossref | Google Scholar |
Gowravaram S, Chao H, Zhao T, Parsons S, Hu X, Xin M, Flanagan H, Tian P (2022) Prescribed grass fire evolution mapping and rate of spread measurement using orthorectified thermal imagery from a fixed-wing UAS. International Journal of Remote Sensing 43, 2357-2376.
| Crossref | Google Scholar |
Hengl T (2006) Finding the right pixel size. Computers & Geosciences 32, 1283-1298.
| Crossref | Google Scholar |
Hiers JK, O’Brien JJ, Varner JM, Butler BW, Dickinson M, Furman J, Gallagher M, Godwin D, Goodrick SL, Hood SM, Hudak A, Kobziar LN, Linn R, Loudermilk EL, McCaffrey S, Robertson K, Rowell EM, Skowronski N, Watts AC, Yedinak KM (2020) Prescribed fire science: the case for a refined research agenda. Fire Ecology 16, 11.
| Crossref | Google Scholar |
Hillman S, Hally B, Wallace L, Turner D, Lucieer A, Reinke K, Jones S (2021) High-resolution estimates of fire severity—an evaluation of uas image and lidar mapping approaches on a sedgeland forest boundary in Tasmania, Australia. Fire 4, 14.
| Crossref | Google Scholar |
Hudak AT, Dickinson MB, Bright BC, Kremens RL, Loudermilk EL, O’brien JJ, Hornsby BS, Ottmar RD (2016) Measurements relating fire radiative energy density and surface fuel consumption - RxCADRE 2011 and 2012. International Journal of Wildland Fire 25, 25-37.
| Crossref | Google Scholar |
Hudak AT, Kato A, Bright BC, Loudermilk EL, Hawley C, Restaino JC, Ottmar RD, Prata GA, Cabo C, Prichard SJ, Rowell EM, Weise DR (2020) Towards spatially explicit quantification of pre- and postfire fuels and fuel consumption from traditional and point cloud measurements. Forest Science 66, 428-442.
| Crossref | Google Scholar |
Iverson LR, Yaussy DA, Rebbeck J, Hutchinson TF, Long RP, Prasad AM (2004) A comparison of thermocouples and temperature paints to monitor spatial and temporal characteristics of landscape-scale prescribed fires. International Journal of Wildland Fire 13, 311-322.
| Crossref | Google Scholar |
Jain P, Coogan SC, Ganapathi Subramanian S, Crowley M, Taylor S, Flannigan MD, Coogan S, Subramanian S, Crowley M, Taylor S (2020) A review of machine learning applications in wildfire science and management. Environmental Reviews 28, 478-505.
| Crossref | Google Scholar |
Johnston JM, Wooster MJ, Lynham TJ (2014) Experimental confirmation of the MWIR and LWIR grey body assumption for vegetation fire flame emissivity. International Journal of Wildland Fire 23, 463-479.
| Crossref | Google Scholar |
Johnston JM, Wheatley MJ, Wooster MJ, Paugam R, Matt Davies G, Deboer KA (2018) Flame-front rate of spread estimates for moderate scale experimental fires are strongly influenced by measurement approach. Fire 1, 16.
| Crossref | Google Scholar |
Keane RE (2015) ‘Wildland fuel fundamentals and applications.’ (Springer International Publishing) 10.1007/978-3-319-09015-3/COVER
Kennard DK, Outcalt KW, Jones D, O’Brien JJ (2005) Comparing techniques for estimating flame temperature of prescribed fires. Fire Ecology 1, 75-84.
| Crossref | Google Scholar |
Klauberg C, Hudak AT, Bright BC, Boschetti L, Dickinson MB, Kremens RL, Silva CA (2018) Use of ordinary kriging and Gaussian conditional simulation to interpolate airborne fire radiative energy density estimates. International Journal of Wildland Fire 27, 228-240.
| Crossref | Google Scholar |
Kremens RL, Dickinson MB, Bova AS (2012) Radiant flux density, energy density and fuel consumption in mixed-oak forest surface fires. International Journal of Wildland Fire 21, 722-730.
| Crossref | Google Scholar |
Loudermilk EL, O’Brien JJ, Mitchell RJ, Cropper WP, Hiers JK, Grunwald S, Grego J, Fernandez-Diaz JC (2012) Linking complex forest fuel structure and fire behaviour at fine scales. International Journal of Wildland Fire 21, 882.
| Crossref | Google Scholar |
Lutes DC, Keane RE, Caratti JF, Key CH, Benson NC, Sutherland S, Gangi LJ (2006) FIREMON: Fire effects monitoring and inventory system. General Technical Report RMRS-GTR-164. (U.S. Department of Agriculture, Forest Service, Rocky Mountain Research Station) 10.2737/RMRS-GTR-164
Mason DS, Lashley MA (2021) Spatial scale in prescribed fire regimes: an understudied aspect in conservation with examples from the southeastern United States. Fire Ecology 17, 3.
| Crossref | Google Scholar |
Mathews BJ, Strand EK, Smith AMS, Hudak AT, Dickinson B, Kremens RL (2016) Laboratory experiments to estimate interception of infrared radiation by tree canopies. International Journal of Wildland Fire 25, 1009-1014.
| Crossref | Google Scholar |
Moore B, Thompson DK, Schroeder D, Johnston JM, Hvenegaard S (2020) Using infrared imagery to assess fire behaviour in a mulched fuel bed in black spruce forests. Fire 3, 1-11.
| Crossref | Google Scholar |
Moran CJ (2019) New, multi-scale approaches to characterize patterns in vegetation, fuels, and wildfire. PhD Thesis, University of Montana, Missoula, MT, United States. Available at https://scholarworks.umt.edu/etd/11464
Moran CJ, Seielstad CA, Cunningham MR, Hoff V, Parsons RA, Queen L, Sauerbrey K, Wallace T (2019) Deriving fire behavior metrics from UAS imagery. Fire 2, 36.
| Crossref | Google Scholar |
Moran CJ, Hoff V, Parsons RA, Queen LP, Seielstad CA (2022) Mapping fine-scale crown scorch in 3D with remotely piloted aircraft systems. Fire 5, 59.
| Crossref | Google Scholar |
Nitoslawski SA, Wong‐Stevens K, Steenberg JWN, Witherspoon K, Nesbitt L, Konijnendijk van den Bosch CC (2021) The digital forest: mapping a decade of knowledge on technological applications for forest ecosystems. Earth’s Future 9, e2021EF002123.
| Crossref | Google Scholar |
O’Brien JJ, Loudermilk EL, Hornsby B, Hudak AT, Bright BC, Dickinson MB, Hiers JK, Teske C, Ottmar RD (2015) High-resolution infrared thermography for capturing wildland fire behaviour: RxCADRE 2012. International Journal of Wildland Fire 25, 62-75.
| Crossref | Google Scholar |
O’Brien JJ, Hiers JK, Varner JM, Hoffman CM, Dickinson MB, Michaletz ST, Loudermilk EL, Butler BW (2018) Advances in mechanistic approaches to quantifying biophysical fire effects. Current Forestry Reports 4, 161-177.
| Crossref | Google Scholar |
Parsons RA, Linn RR, Pimont F, Hoffman C, Sauer J, Winterkamp J, Sieg CH, Jolly WM (2017) Numerical investigation of aggregated fuel spatial pattern impacts on fire behavior. Land 6, 43.
| Crossref | Google Scholar |
Paugam R, Wooster MJ, Roberts G (2013) Use of handheld thermal imager data for airborne mapping of fire radiative power and energy and flame front rate of spread. IEEE Transactions on Geoscience and Remote Sensing 51, 3385-3399.
| Crossref | Google Scholar |
Reynolds RT, Sánchez Meador AJ, Youtz JA, Nicolet T, Matonis MS, Jackson PL, DeLorenzo DG, Graves AD (2013) ‘Restoring composition and structure in southwestern firequent-fire forests: A science-based framework for improving ecosystem resiliency.’ (USDA: Fort Collins, CO) Available at https://www.fs.usda.gov/rm/pubs/rmrs_gtr310.pdf
Roccaforte JP, Fulé PZ, Chancellor WW, Laughlin DC (2012) Woody debris and tree regeneration dynamics following severe wildfires in Arizona ponderosa pine forests. Canadian Journal of Forest Research 42, 593-604.
| Crossref | Google Scholar |
Rowell E, Loudermilk EL, Hawley C, Pokswinski S, Seielstad C, Queen Ll, O’Brien JJ, Hudak AT, Goodrick S, Hiers JK (2020) Coupling terrestrial laser scanning with 3D fuel biomass sampling for advancing wildland fuels characterization. Forest Ecology and Management 462, 117945.
| Crossref | Google Scholar |
Ryan KC (2010) Effects of fire on cultural resources. In ‘VI International Conference on Forest Fire Research’. (Ed. D Viegas) (U.S. Department of Agriculture, Forest Service, Rocky Mountain Research Station) Available at https://www.fs.usda.gov/rm/pubs_other/rmrs_2010_ryan_k004.pdf
Ryan KC, Knapp EE, Varner JM (2013) Prescribed fire in North American forests and woodlands: history, current practice, and challenges. Frontiers in Ecology and the Environment 11, e15-e24.
| Crossref | Google Scholar |
Sagel D, Speer K, Pokswinski S, Quaife B (2021) Fine-scale fire spread in pine straw. Fire 4, 69.
| Crossref | Google Scholar |
Schumacher B, Melnik KO, Katurji M, Zhang J, Clifford V, Pearce HG (2022) Rate of spread and flaming zone velocities of surface fires from visible and thermal image processing. International Journal of Wildland Fire 31, 759-773.
| Crossref | Google Scholar |
Shannon CE (1949) Communication in the presence of noise. Proceedings of the IRE 37, 10-21.
| Crossref | Google Scholar |
Sikkink PG, Keane RE (2008) A comparison of five sampling techniques to estimate surface fuel loading in montane forests. International Journal of Wildland Fire 17, 363-379.
| Crossref | Google Scholar |
Smith AMS, Wooster MJ, Drake NA, Dipotso FM, Perry GLW (2005) Fire in African savanna: testing the impact of incomplete combustion on pyrogenic emissions estimates. Ecological Applications 15, 1074-1082.
| Crossref | Google Scholar |
Smith AMS, Tinkham WT, Roy DP, Boschetti L, Kremens RL, Kumar SS, Sparks AM, Falkowski MJ (2013) Quantification of fuel moisture effects on biomass consumed derived from fire radiative energy retrievals. Geophysical Research Letters 40, 6298-6302.
| Crossref | Google Scholar |
Stephens SL, Finney MA, Schantz H (2004) Bulk density and fuel loads of ponderosa pine and white fir forest floors: impacts of leaf morphology. Northwest Science 78, 93-100.
| Google Scholar |
Valero MM, Verstockt S, Butler B, Jimenez D, Rios O, Mata C, Queen Ll, Pastor E, Planas E (2021) Thermal infrared video stabilization for aerial monitoring of active wildfires. IEEE Journal of Selected Topics in Applied Earth Observations and Remote Sensing 14, 2817-2832.
| Crossref | Google Scholar |
Van Wagtendonk JW, Sydoriak WM, Benedict JM (1998) Heat content variation of Sierra Nevada conifers. International Journal of Wildland Fire 8, 147-158.
| Crossref | Google Scholar |
Varner JM, Gordon DR, Putz FE, Kevin Hiers J (2005) Restoring fire to long-unburned Pinus palustris ecosystems: novel fire effects and consequences for long-unburned ecosystems. Restoration Ecology 13, 536-544.
| Crossref | Google Scholar |
Waring KM, Hansen KJ, Flatley W (2016) Evaluating prescribed fire effectiveness using permanent monitoring plot data: a case study. Fire Ecology 12, 2-25.
| Crossref | Google Scholar |
Williams CJ, Pierson FB, Nouwakpo SK, Al-Hamdan OZ, Kormos PR, Weltz MA (2020) Effectiveness of prescribed fire to re-establish sagebrush steppe vegetation and ecohydrologic function on woodland-encroached sagebrush rangelands, Great Basin, USA: Part I: Vegetation, hydrology, and erosion responses. Catena 185, 103477.
| Crossref | Google Scholar |
Wooster MJ (2002) Small-scale experimental testing of fire radiative energy for quantifying mass combusted in natural vegetation fires. Geophysical Research Letters 29, 23-1.
| Crossref | Google Scholar |
Wooster MJ, Roberts G, Perry GLW, Kaufman YJ (2005) Retrieval of biomass combustion rates and totals from fire radiative power observations: FRP derivation and calibration relationships between biomass consumption and fire radiative energy release. Journal of Geophysical Research: Atmospheres 110, D24311.
| Crossref | Google Scholar |
Wotton BM, Gould JS, McCaw WL, Cheney NP, Taylor SW (2012) Flame temperature and residence time of fires in dry eucalypt forest. International Journal of Wildland Fire 21, 270-281.
| Crossref | Google Scholar |