A comment on the use of visually assessed fuel hazard ratings and scores for Australian fire management and research
Miguel G. Cruz
A
Abstract
Assessment of fuel hazard has become the dominant method of describing Australian forest fuel complexes, despite a lack of evidence supporting the veracity of its underpinning assumptions.
To analyse and discuss the merits of fuel hazard ratings and scores in representing measurable fuel characteristics, such as fuel load and fire behaviour potential.
Published findings were reviewed, and available data analysed to investigate the validity of the Australian fuel hazard assessment concepts.
Multiple published studies showed the Australian fuel hazard assessment methods to be subjective and non-replicable. All available evidence shows no relationship between fuel hazard ratings and fuel quantity. No relationship between the ratings and fire behaviour potential was found.
The principles underpinning the use of fuel hazard ratings for fuel assessment were shown to be unfounded. The ratings cannot be converted into physical fuel characteristics or fire behaviour potential, and its application in Australian fire management is unwarranted.
Keywords: Bushfire fuels, Bushfire simulation, Eucalyptus forest, fire behaviour potential, forest fuels, fuel assessment, fuel structure, overall fuel hazard rating (OFHR), visual fuel assessment.
Introduction
In the context of understanding and predicting wildland fire behaviour and its impacts, fuel is, as simply put by Hal Anderson, the source of the matter (Anderson 1973). Fuel physical and chemical characteristics such as mass per area, compactness, and size distribution, determine how much fuel is available for combustion, combustion efficiency, energy release and emissions (Pyne et al. 1996).
In the last two decades in Australia, fuel assessment and inventory shifted from a focus on direct measurement of fuel physical characteristics, such as mass per unit area, cover, height (e.g. Peet 1971a, 1971b; Walker 1981; Marsden-Smedley and Catchpole 1995; McCaw 2011; Watson 2012) to the assessment of fuel hazard (Wilson 1992a, 1992b, McCarthy et al. 1998, 1999). Fuel hazard rating or scoring, henceforth FHR, is a concept that incorporates different fuel characteristics, such as mass, cover, height, continuity (vertical and horizontal), density and perceived flammability, to qualify fire behaviour potential and difficulty of control associated with the fuel complex. FHR assessment is predominantly based on the visual1 appraisal of attributes. FHR is assessed independently for different fuel layers (e.g. surface litter, near-surface, elevated and bark) and rated (McCarthy et al. 1998; Hines et al. 2010), or scored (Gould et al. 2007a), into five classes (Low to Extreme or 0–4; Gould et al. 2007b added a sixth class). The individual fuel layer ratings can be integrated to produce an overall fuel hazard rating (OFHR) (McCarthy et al. 1998; Hines et al. 2010).
Originally, the fuel hazard rating system was developed as a simple field assessment method to categorise fuels into fuel hazard classes with several applications, namely (but not restricted to) to support field decisions regarding ‘fuel management’, ‘identifying fuel hazards’ during fire suppression operations, and ‘to conduct prescribed burning’ (McCarthy et al. (1998). A variation of this rating system was proposed by Gould et al. (2007a, 2011), with two main changes: instead of the class rating, a continuous numerical score directly applicable for modelling fire behaviour (Cheney et al. 2012), and the separation of the surface fuel layer into a surface (essentially litter) and near-surface (grasses, low shrubs, creepers and collapsed dead material) layers.
Associated with the FHR assessment guides are tables that allow a direct conversion of hazard into fuel loads (McCarthy et al. 1998, 1999; Gould et al. 2007b; Hines et al. 2010)2. McCarthy et al. (1998, 1999) explicitly states that the fuel loads have use as an input to the McArthur (1967, 1973) Forest Fire Danger Meter. Since the introduction of these FHR assessment guides their application to fire management and research purposes, uses the system was not originally designed for, has significantly expanded across Australia, namely using the Hines et al. (2010) version. This was partially because of the expeditious nature of the assessments and the perceived relevance of the results. In particular, after Phoenix Rapidfire (Tolhurst et al. 2008), a computer-based fire behaviour simulator used operationally and in research applications in Australia (e.g. Duff and Penman 2021; Penman et al. 2022), implemented a fuel modelling approach based on FHR. In this system, FHRs are assumed to evolve after fire through a negative exponential relationship which can then be converted into an associated fuel quantity (Tolhurst 2005; McColl-Gausden et al. 2020), which is a required input for a number of the simulator fire behaviour models (Chong et al. 2012).
As an example of the amount of effort expended in FHR assessments across Australia over the years, McColl-Gausden et al. (2020) used a database with 21,176 FHR assessments3 conducted by Victorian Department of Environment, Land, Water and Planning (DELWP), now the Department of Energy, Environment and Climate Action (DEECA), between 1995 and 2017. Duff and Tumino (2019) report on other Victorian datasets in addition to the DELWP set, totalling more than 7,000 fuel complex assessments. In New South Wales, the National Parks and Wildlife Service (NPWS) has a database of 13,800 FHR assessments conducted between 2012 and 2022. Other agencies in Australia have comparable albeit probably smaller in scope FHR assessment programs. It is noted that many of assessments are not recorded into a centralised system, making it difficult to estimate the actual size of existing datasets. From conversations with agency members, the author estimates that in excess of 3,000 FHR assessments have been conducted per year across Australia in the last decade.
The use of FHR for research purposes is quite extensive covering a broad range of applications, such as studying of the effect of thinning operations on fire potential (e.g. Proctor and McCarthy 2015; Volkova and Weston 2019), examining climate, anthropogenic and environmental factors on fuel dynamics (Duff et al. 2013; Wilson et al. 2018; Jenkins et al. 2020; McColl-Gausden et al. 2020), quantifying landscape fire risk (Jenkins et al. 2019), and testing remote sensing sensors to map fuel characteristics (e.g. Price and Gordon 2016; Hillman et al. 2021; Wallace et al. 2022).
At the core of FHR assessment methods and applications are three assumptions: (1) the assessment method is objective and replicable (McCarthy et al. 1998; Gould et al. 2011; McColl-Gausden and Penman 2017); (2) there is a relationship between the assessed rating and physical fuel properties driving fire behaviour, namely fine fuel mass per unit area (as used in a number of Australian fire behaviour modelling systems); and (3) that the assessed overall rating is directly and proportionally associated with fire behaviour potential. The author contends none of these three assumptions are valid and that the use of FHR assessments in Australia is not only unwarranted, but also detrimental to both effective fire management and research applications. Findings from various published research were reviewed and available data analysed to test this assertion. Alternative methods for assessing fuels that should guide sound application of fuel data on fire management and science are then explored.
Subjectivity and non-replicable nature of fuel hazard assessment methods
Visual-based assessment methods of fuel structure and flammability are inherently subjective. When considering the FHR approach, there is a noticeable absence of objectivity due to a lack of clarity in the guide’s instructional material (Duff and Tumino 2019; Pickering et al. 2023). For example, although the method is designed for the assessment of a number of key fuel attributes, its multidimensional nature requires the assessor to make personal judgements when the attributes ranking is spread over a range of classes (e.g. Moderate horizontal continuity but Very High dead fuel percentage). Furthermore, there is considerable uncertainty in the differentiation of the near-surface and the elevated fuel layers (Watson et al. 2012a, 2012b; Duff and Tumino 2019; Pickering et al. 2023). Fuel strata cover, one of the key fuel attributes in defining FHR class, has been shown to be not amenable to accurate visual estimation (see Watson et al. 2012a), particularly at ground level where parallax viewing error is difficult to identify. Visual assessment results are also known to be dependent on the expertise and experience of the assessor (Keane 2013), namely the range of fuel types known to individual assessors.
To overcome the issue of subjectivity, some studies have relied on a single observer (e.g. McColl-Gausden and Penman 2017) or a close quality control of fuel assessors (Gould et al. 2011). But it should be noticed that although the use of a single observer reduces subjectivity, it can introduce a consistent bias. In contrast, in the real world of FHR assessment, studies showed that the subjectivity of the methods leads to large variability in the results and questionable replicability of the methods. Watson et al. (2012a) conducted a carefully controlled evaluation of trained fuel assessors and found significant differences between assessor groups. The differences were highest for the fuel layers that the FHR method specifically aimed to describe: near-surface, elevated and bark. A broader study of operational FHR assessments by Volkova et al. (2016) found the largest uncertainty lies in the ranking of the surface (litter) fuel layer. Training is known to play an important role in the reliability of visual assessment methods (Gould et al. 2011; Volkova et al. 2016). Nonetheless, outside of the research environment, where assessors are often carefully instructed in the use of visual rating methods, minimal or complete absence of effective training seems to be common. Teams of FHR field assessors the author contacted received only informal training and on-the-job instruction from more experienced colleagues, who, suprisingly, had also learned on the job from other colleagues. This lack of formalised training perpetuates biases and partially explains why the analyses of available FHR datasets fail to find an effect of time since fire on fuel hazard.
Duff and Tumino (2019) attempted to model FHR dynamics with time since fire for the State of Victoria’s main forest fuel types with a large FHR database (>40,000 FHR assessments). Despite the large amount of data, the authors found limited signals from which FHR dynamic models for specific fuel types could be developed. Only 16% of the possible fuel type/fuel layer combinations present were amenable to modelling as a function of time, and of these the prediction intervals were too broad to be of value. Similarly, Newnham et al. (2020) tested the effect of burn severity on FHR in Victorian forest fuel types subjected to prescribed burning, and found no significant differences between burned and unburned areas, or between different burn severity classes within burned areas, even when the FHR assessment was conducted only 3–12 months after a burn. In other words, the FHR assessment failed to identify changes in fuel bed structure after a prescribed burn, regardless of the intensity and severity of the burn.
A number of authors, e.g. Watson et al. (2012a), Volkova et al. (2016), and Pickering et al. (2023), provided suggestions about how to improve the FHR assessment method, namely by reducing the ambiguity in the rules and training to reduce observer bias and uncertainty. Nonetheless, as a protocol largely based on visual assessment of fuel structure (of the 17 attributes assessed, one is measured and 16 are visually estimated), a significant level of uncertainty is likely to always be present.
The subjectivity of these visually-based assessment methods contributes to substantial uncertainty on fire behaviour and danger predictions, either when (1) using the Cheney et al. (2012) fire spread model that relies on surface and near-surface fuel layer hazard score as a direct input, or (2) when converting ratings into fuel load metrics for input to other fire behaviour models (next section).
Nonexistence of a relationship between visually assessed ratings and fuel load
Fuel hazard rating is an abstraction (Duff and Tumino 2019) with one of its main applications, outside of its original aim of field assessment of fuels, being the conversion of ratings into fuel load values (e.g. Tolhurst 2005; Penman et al. 2022), that are then used as input to fire behaviour models such as McArthur (1967) Forest Fire Danger Meter and Phoenix Rapidfire (Tolhurst et al. 2008). Kenny et al. (2024) mentioned the use of a similar approach in the Australian Fire Danger Rating System prototype (Hollis et al. 2024) when no known fuel information was available. McCarthy et al. (1999) and Hines et al. (2010) provide tables that allow estimating a fuel load range from a rating. Tolhurst (2005; see also McColl-Gausden et al. 2022) give equations for this fuel load estimation. The bases of these tables and equations are unknown.
Two main issues arise from this conversion of FHR to fuel load. Firstly, the subjective nature of the FHR assessment causes a large uncertainty in the estimated fuel loads. Watson et al. (2012a) noted that when converting the FHR assessments of different trained observers into fuel loads, the results varied by more than a threefold. Secondly and most importantly, there is no evidence that the relationships derived between FHR and fuel load hold true. Volkova et al. (2016) conducted an extensive and authoritative evaluation of these relationships with paired destructive fuel mass sampling and FHR data from different sources across eastern and southern Australia, finding no relationship between assigned FHR and measured biomass for surface, near-surface or elevated fuel components. In particular, they found that the visual assessment of surface fuels — the layer containing the bulk of the mass of fuel consumed by a fire in an Australian dry sclerophyll eucalypt forest — was not statistically different from a random allocation of hazard level! They also found that (1) on average less than half of the measured fuel loads were within the broad FHR classes, and (2) for High, Very High and Extreme classes there was not only a typical over-prediction of fuel load, but also there were no significant differences in fuel load between the classes.
The lack of a true relationship between FHR and fuel load is well visualised by the Tasmanian dataset (Fig. 1) used by Volkova et al. (2016) and detailed by Marsden-Smedley et al. (2022). Analysis of this subset of data, that relies only on fuels sampled in the steady-state stage of the fuel accumulation process, produces models of fuel load from FHR with very poor explanatory power (R2 between 0.01 and 0.22), i.e. at most only 22% of the variation in the fuel load is explained by FHR. The figure also shows the discrepancy of the Tolhurst (2005) FHR – fuel load equations relative to the sampled data. A multiple comparison test (Tukey Honestly Significance Difference (HSD)) showed no significant differences in fuel load between FHR ratings for 9 of the 10 surface fuel load comparisons (the only statistically significant difference was between the Moderate and Very High rating), and no significant differences in any of the near-surface and elevated rating comparisons. In a smaller scale study, relying on a sole assessor and focusing on surface fuels only, McColl-Gausden and Penman (2017) found comparable results to Volkova et al. (2016). Sampled surface fuel loads in the lower FHR rating classes were within the expected broad fuel load classes ranges, and no differences existed between the fuel loads sampled in the High and above classes. These results, coupled with the findings by Watson et al. (2012b) and Marsden-Smedley et al. (2022) (that fuel layer ratings of Low and Moderate are not common for fuel ages older than 5 years) further suggests that the FHR assessment method mostly fails when representative fuel information is most needed.
Relationship between observed fuel load and fuel hazard rating (as per Hines et al. 2010) for (a) surface, (b) near-surface and (c) elevated fuel layers in the Tasmanian data from Marsden-Smedley et al. (2022). Data shown is constrained to time since the last fire beingmore than 9 years to ensure the fuel complexes approached or are within the steady state phase of fuel accumulation in the Eucalyptus forests. Tolhurst (2005) curves are based on fuel layer-specific equations that convert FHR into fuel load. Near-surface fuel was not explicitly quantified at the time of Tolhurst (2005). In McCarthy et al. (1999) the near-surface fuel effect was allocated to the surface fuel layer.
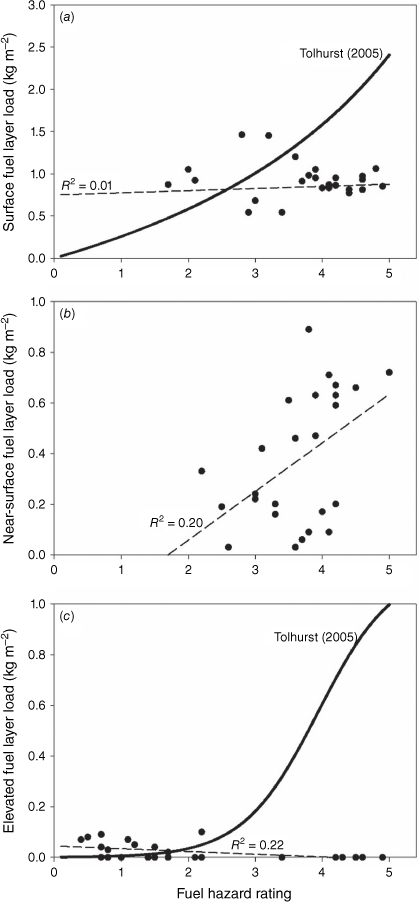
It is noted that the study by Gould et al. (2011) found a relationship between Fuel Hazard Scores of each layer and its respective fuel load. But these results were achieved in a unique set of circumstances, namely the fuel assessment/sampling was conducted on a sole forest type in two locations with variations in fuel structure being driven mostly by known time since fire. The assessors knew precisely the fuel ages (five different fuel ages/time since fire) and during the course of the study they attended multiple audits and recalibration sessions to ensure consistency. The results were also only applicable to the forest type in which the study was conducted (Jim Gould, pers. comm.).
Are visually assessed ratings related to fire behaviour potential?
The rationale behind the definition and assessment of FHR is to better capture the effect of fuels other than surface fine fuels on fire behaviour potential, with the overall fuel hazard rating (OFHR) representing ‘the effect that the fuel arrangement is likely to have on fire behaviour’ and ‘the ability of suppression forces to control a fire in these fuels’ under a defined set of fire weather conditions equivalent to a Forest Fire Danger Index (McArthur 1967) of 25 (McCarthy et al. 1998). The rules defining fuel layer hazard lack a physical basis that would explain transition between classes. No documentation for the threshold values defining classes seem to exist. Similarly, the way the various layer FHR are integrated to estimate the OFHR lacks a physical reasoning and ignores the fact that changes in the status of different fuel layers with time are often autocorrelated (e.g. Gould et al. 2011). Despite the widespread use of the OFHR concept in Australia, the author is aware of only one attempt to evaluate ratings from a point of view of fire behaviour potential or the difficulty of controling it. McCarthy and Tolhurst (1998) found that predicted fireline intensity was a better predictor of the first attack success, extended initial attack and the first attack failure, than FHR variables. The lack of scrutiny of the veracity of the ratings contrasts with the high value Australian fire management agencies place on the fuel hazard assessments as evidenced by the investment in conducting thousands of such assessments every year.
The data provided by Marsden-Smedley et al. (2022) with FHR assessed using Hines et al. (2010) methods and physical descriptors of the understorey fuel layers sampled/measured offer an opportunity to evaluate the overall fuel hazard ratings against predictions of fire behaviour models, namely answering the question: what is the potential fire behaviour associated with an observed OFHR under the environmental conditions used in their definition? The Vesta Mk 2 rate of fire spread model (Cruz et al. 2022) takes three (surface, near-surface and elevated) of the four fuel layers used for determining overall fuel hazard rating as direct inputs. Bark fuel is not used as an input in this, or in any other Australian rate of fire spread model, although it is used in models describing spotting dynamics (e.g. Gould et al. 2007b; above mentioned Phoenix Rapidfire).
Fig. 2 illustrates the distribution of predicted fireline intensities (as per Byram 1959; Alexander 1982) calculated for Marsden-Smedley et al. (2022) data for fuel ages >9 years4 using the Vesta Mk 2 model for predicting the rate of fire spread. This was undertaken using the weather scenario used in the definition of the OFHR (Hines et al. 2010), namely: air temperature 33°C; relative humidity 25%; wind speed 20 km h−1; drought factor 10. Slope is considered to be flat. Fireline intensity was calculated from the product of the predicted rate of spread, the total mass of fine fuels in the three considered fuel layers (assuming total consumption) and the fuel low heat content of 18,600 kJ kg−1. Model simulations did not show an effect of OFHR on fireline intensity (Fig. 2). Variation within OFHR classes was larger than between classes with a multiple comparison test (Tukey HSD) showing no significant differences in fireline intensity between classes.
Distribution of predicted fireline intensity by overall fuel hazard rating score for the data in Marsden-Smedley et al. (2022) (subset with fuel age >9 years). Fuel hazard rating was calculated as per Hines et al. (2010). Fireline intensity is calculated using the rate of fire spread predicted by Cruz et al. (2022) model and the assumptions that all and only fine fuel is consumed in flaming combustion, and that fuel has a heat content of 18,600 kJ kg-1 (no further corrections for mineral and fuel moisture content effects added). The weather scenario used is: air temperature 33°C; relative humidity 25%; wind speed 20 km h-1; drought factor 10. Slope steepness is considered to be 0° (Hines et al. 2010). The box plot depicts the interquartile range, with whiskers being the 10th and 90th percentiles. The thick horizontal line is the mean, whereas the finer line within the box plot is the median. Black dots represent outliers.
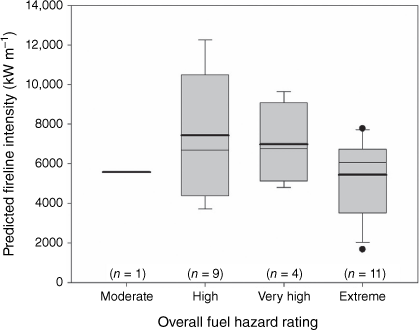
This lack of a relationship between OFHR and fireline intensity has several possible explanations. Most likely it arises from the differences between the rules defining FHR and the model sensitivity to fuel variation, as defined by the model functional forms. Bark is not an input to the Vesta Mk 2 fire spread rate model. But bark hazard rating has a strong influence on the OFHR, with a bark rating of Very High or Extreme resulting in a Very High or above OFHR for most of the possible surface – near-surface – elevated – bark FHR combinations. It should be noted that the effect of bark hazard on the OFHR is arbitrary as it does not have a physical or scientific basis. In the particular case of the data in Fig. 2, the sites rated as Extreme OFHR were largely determined by the bark FHR, explaining why the increase in rating did not result in an increase in simulated fire behaviour potential. It can be also expected that the previously mentioned subjectivity in assessing each fuel layer FHR also has an effect on these results.
The results in Fig. 2 question the validity of the overall fuel hazard rating in capturing the effect of fuel arrangement on fire behaviour variables, as envisioned by its developers (McCarthy et al. 1998; Hines et al. 2010) and accepted by research and operational users of the method.
Back to basics – on the need for measurement and inventory of fuel physical characteristics
The last two decades have witnessed a significant investment by Australian fire authorities and land management agencies in conducting visual assessments of fuel structure in the form of FHR for fuel attribute estimation. The evidence presented above highlights the futility of such investment, with FHR being continually shown to be unrelated to key fuel structure variables and fire behaviour potential. The lack of evidence for these relationships is not surprising given that the rules defining the fuel hazard ratings lack a physical reasoning. Through this time period, the number of scientific studies focusing on fuel attribute metrics, such as load, height and cover have been limited (e.g. Gould et al. 2011; Fontaine et al. 2012; Cruz et al. 2018; Tangney et al. 2022). It is the opinion of the author that had the effort invested into visual assessments of FHR been instead applied to the measurement of physical fuel characteristics, the information collected would have provided a more accurate and meaningful measure of the temporal and spatial variation in fuel properties. Although more onerous to carry out than visual-based FHR assessments, fuel measurement methods based on well-established, destructive, double sampling and other sampling approaches (e.g. Catchpole and Wheeler 1992), provide accurate and meaningful data that can then be used in an array of applications, such as supporting estimations of fire danger, risk and behaviour assessments, modelling temporal variation in fuels and implications of different fuel management activities, and fire risk mitigation operations. In contrast, the benefit of FHR data is unclear, as the overwhelming evidence clearly shows that the data do not describe what its authors envisioned and its users imagined. The conformity of users in this space is typical of the collective illusion phenomena (Rose 2022).
The absence of national guidelines or accepted methodologies (e.g. as per Brown 1974; Brown et al. 1982) for measuring the physical attributes of fuels as inputs to the various fire behaviour models is a limitation to consistency, precision and accuracy of fuel assessments in Australia (Duff et al. 2017). Work commissioned by the Australian Fire and Emergency Service Authorities Council (AFAC) resulted in guidance for fuel assessment in Australian vegetation types (Gould and Cruz 2012), although this work lacked consultation with agencies and had restricted circulation. Within a number of Australian fire and land management agencies there is a renewed interest to define more effective fuel appraisal methods (e.g. Duff et al. 2017; JJ Hollis, S Samson, MG Cruz, WL McCaw, JS Gould, unpublished) that are widely accepted and that overcome the limitations of current assessment methods based on visual estimation of fuel structure (e.g. Volkova et al. 2016; Pickering et al. 2023). A focus on efficiently combining different methods is the key to user uptake, allowing for tailoring different approaches for different needs, e.g. scientific study vs pre-burn fuel assessment (Duff et al. 2017), while maintaining consistency. Such guidelines, upon agreement by the various agencies, are necessary before replicable fuel inventory schemes are applied across Australia.
Concluding remarks
The published evidence of the merits of the visually assessed fuel hazard rating approach as utilised in Australia has been reviewed here. The evidence based on the analysis of hundreds of fuel load/FHR pairs and tens of thousands of FHR assessments, points to the fact that none of the underlying assumptions are supported by the evidence explored. Namely, the subjectivity of the methodology results in large uncertainty in the assessed ratings (Watson et al. 2012a) and precludes its uses in other applications, such as the development of predictive equations (e.g. Duff and Tumino 2019). No reliable relationship was found between hazard rating of fuel layers and their physical properties, namely fuel load (e.g. Volkova et al. 2016; McColl-Gausden and Penman 2017), although an exception occurred on a tightly controlled scientific study (Gould et al. 2011) for a very specific vegetation complex. Finally, fire behaviour simulations using data with paired overall fuel hazard rating/fuel characteristics showed no relationship between the overall rating and predicted fireline intensity, a metric related to suppression difficulty.
These findings have a range of implications within the broad spectrum of the use of fuel information in fire management applications. From an operational fire management perspective, it can be argued that the FHR methodology is mildly misleading at best, but also has potential to introduce significant error and uncertainty in critical fire management and suppression decision making, as well as in public safety matters. In instances where FHR (or FHR equations utilising time since the last fire) is used to estimate fuel quantity for fire behaviour simulations, errors introduced by these assumptions are likely corrected throughout the process of adjusting predictions to previously observed and expected fire behaviour. Over-estimation of fuel quantity will not result in critical under-prediction of fire spread or behaviour, but continued over-prediction bias has issues of its own (e.g. cry-wolf syndrome, distrust on released public warnings). Over-prediction of fireline intensity, smoke production or emissions will likely be associated with the use of these FHR – fuel quantity relationships (Volkova et al. 2016). It is unclear to the author how the uncertainty and over-estimation of fuel load will result in unwarranted changes in fire management in different situations, but this is certainly possible (Watson et al. 2012a). Similarly, the application of FHR – fuel load relationships in the Australian Fire Danger Rating System (Kenny et al. 2024) has potential to introduce uncertainty in fire danger ratings, unwarranted inflated ratings and public mistrust in the system.
Volkova et al. (2016) also point out some more mundane implications of the fuel quantity over estimation: in the procedure to develop building applications used in rural areas of Australia (Standards Australia 2018), the FHR-based fuel quantity estimation will artificially inflate building costs.
It should be noted that the method still has relevance for fuel appraisal in the field as a systematic way for non-technical experts, such as the public, to integrate different fuel structure variables into a cohesive measure of the forest fuel complex ‘flammability’. In particular, the Hines et al. (2010) guide is still a valuable tool for Australian fire practitioners to bring together the complexity of understorey forest fuel arrangements into simple concepts. That said, the author believes no decision making should be based on fuel hazard ratings due to limitations pointed out in this comment paper.
Australian rural fire authorities and land management agencies have supported the FHR assessment approach and have conducted extensive inventories of fuels using it in the belief that they were collecting useful data. In the past, there has been an element of disregarding negative evidence of its value, likely due to the convenience and low costs of the assessment process and because it is easy to believe in what you want to believe.
Data availability
Data sharing is not applicable as no new data were generated or analysed during this study.
Declaration of funding
This research was funded by the Commonwealth Scientific and Industrial Research Organisation.
Acknowledgements
The author appreciates the comments received by Matt Plucinski, Andrew Sullivan and two anonymous reviewers on earlier versions of this paper.
References
Adams MA, Neumann M (2024) Perspective: Flawed assumptions behind analysis of litter decomposition, steady state and fire risks in Australia. Forest Ecology and Management 556, 121741.
| Crossref | Google Scholar |
Alexander ME (1982) Calculating and interpreting forest fire intensities. Canadian Journal of Botany 60, 349-357.
| Crossref | Google Scholar |
Catchpole WR, Wheeler CJ (1992) Estimating plant biomass: a review of techniques. Australian Journal of Ecology 17, 121-131.
| Crossref | Google Scholar |
Cheney NP, Gould JS, McCaw WL, Anderson WR (2012) Predicting fire behaviour in dry eucalypt forest in southern Australia. Forest Ecology and Management 280, 120-131.
| Crossref | Google Scholar |
Chong D, Tolhurst K, Duff T (2012) ‘PHOENIX RapidFire 4.0 Convection and Ember Dispersal Model.’ (Bushfire CRC: Melbourne) Available at http://www.bushfirecrc.com/sites/default/files/phoenix_4_convection_and_spotting.pdf
Cruz MG, Sullivan AL, Gould JS, Hurley RJ, Plucinski MP (2018) Got to burn to learn: the effect of fuel load on grassland fire behaviour and its management implications. International Journal of Wildland Fire 27, 727-741.
| Crossref | Google Scholar |
Cruz MG, Cheney NP, Gould JS, McCaw WL, Kilinc M, Sullivan AL (2022) An empirical-based model for predicting the forward spread rate of wildfires in eucalypt forests. International Journal of Wildland Fire 31, 81-95.
| Crossref | Google Scholar |
Duff TJ, Penman TD (2021) Determining the likelihood of asset destruction during wildfires: modelling house destruction with fire simulator outputs and local-scale landscape properties. Safety Science 139, 105196.
| Crossref | Google Scholar |
Duff TJ, Bell TL, York A (2013) Predicting continuous variation in forest fuel load using biophysical models: a case study in south-eastern Australia. International Journal of Wildland Fire 22, 318-332.
| Crossref | Google Scholar |
Duff T, Keane R, Penman T, Tolhurst K (2017) Revisiting wildland fire fuel quantification methods: the challenge of understanding a dynamic, biotic entity. Forests 8, 351.
| Crossref | Google Scholar |
Fontaine JB, Westcott VC, Enright NJ, Lade JC, Miller BP (2012) Fire behaviour in south-western Australian shrublands: evaluating the influence of fuel age and fire weather. International Journal of Wildland Fire 21, 385-395.
| Crossref | Google Scholar |
Gould JS, Cruz MG (2012) Australian fuel classification: Stage 2. In ‘A Report for the Australasian Fire and Emergency Service Authorities Council. National Burning Project Sub-Project No. 5. CSIRO Epublish ID: EP126505’. 98 pp. (CSIRO Ecosystem Sciences and Climate Adaptation Flagship, Bushfire Dynamics and Applications: Canberra, ACT)
Gould JS, McCaw WL, Cheney NP (2011) Quantifying fine fuel dynamics and structure in dry eucalypt forest (Eucalyptus marginata) in Western Australia for fire management. Forest Ecology and Management 262, 531-546.
| Crossref | Google Scholar |
Hillman S, Wallace L, Lucieer A, Reinke K, Turner D, Jones S (2021) A comparison of terrestrial and UAS sensors for measuring fuel hazard in a dry sclerophyll forest. International Journal of Applied Earth Observation and Geoinformation 95, 102261.
| Crossref | Google Scholar |
Hollis JJ, Matthews S, Fox-Hughes P, Grootemaat S, Heemstra S, Kenny BJ, Sauvage S (2024) Introduction to the Australian Fire Danger Rating System. International Journal of Wildland Fire 33, WF23140.
| Crossref | Google Scholar |
Jenkins M, Price O, Collins L, Penman T, Bradstock R (2019) The influence of planting size and configuration on landscape fire risk. Journal of Environmental Management 248, 109338.
| Crossref | Google Scholar | PubMed |
Jenkins ME, Bedward M, Price O, Bradstock RA (2020) Modelling bushfire fuel hazard using biophysical parameters. Forests 11, 925.
| Crossref | Google Scholar |
Keane RE (2013) Describing wildland surface fuel loading for fire management: a review of approaches, methods and systems. International Journal of Wildland Fire 22, 51-62.
| Crossref | Google Scholar |
Kenny BJ, Matthews S, Sauvage S, Grootemaat S, Hollis JJ, Fox-Hughes P (2024) Australian Fire Danger Rating System: implementing fire behaviour calculations to forecast fire danger in a research prototype. International Journal of Wildland Fire 33, WF23142.
| Crossref | Google Scholar |
Marsden-Smedley JB, Catchpole WR (1995) Fire modelling in Tasmanian buttongrass moorlands I. Fuel characteristics. International Journal of Wildland Fire 5, 203-214.
| Google Scholar |
Marsden-Smedley JB, Anderson WR, Pyrke AF (2022) Fuel in Tasmanian dry eucalypt forests: prediction of fuel load and fuel hazard rating from fuel age. Fire 5, 103.
| Crossref | Google Scholar |
McCaw WL (2011) Characteristics of jarrah (Eucalyptus marginata) forest at FORESTCHECK monitoring sites in south-west Western Australia: stand structure, litter, woody debris, soil and foliar nutrients. Australian Forestry 74, 254-265.
| Crossref | Google Scholar |
McColl-Gausden SC, Penman TD (2017) Visual assessment of surface fuel loads does not align with destructively sampled surface fuels. Forests 8, 408.
| Crossref | Google Scholar |
McColl-Gausden SC, Bennett LT, Duff TJ, Cawson JG, Penman TD (2020) Climatic and edaphic gradients predict variation in wildland fuel hazard in south-eastern Australia. Ecography 43, 443-455.
| Crossref | Google Scholar |
McColl-Gausden SC, Bennett LT, Clarke HG, Ababei DA, Penman TD (2022) The fuel–climate–fire conundrum: how will fire regimes change in temperate eucalypt forests under climate change? Global Change Biology 28, 5211-5226.
| Crossref | Google Scholar | PubMed |
Peet GB (1971b) Litter accumulation in jarrah and karri forests. Australian Forestry 35, 258-262.
| Crossref | Google Scholar |
Penman TD, McColl-Gausden SC, Cirulis BA, Kultaev D, Ababei DA, Bennett LT (2022) Improved accuracy of wildfire simulations using fuel hazard estimates based on environmental data. Journal of Environmental Management 301, 113789.
| Crossref | Google Scholar | PubMed |
Pickering B, Bennett LT, Cawson JG (2023) Extending methods for assessing fuel hazard in temperate Australia to enhance data quality and consistency. International Journal of Wildland Fire 32, 1422-1437.
| Crossref | Google Scholar |
Price OF, Gordon CE (2016) The potential for LiDAR technology to map fire fuel hazard over large areas of Australian forest. Journal of Environmental Management 181, 663-673.
| Crossref | Google Scholar | PubMed |
Proctor E, McCarthy G (2015) Changes in fuel hazard following thinning operations in mixed-species forests in East Gippsland, Victoria. Australian Forestry 78, 195-206.
| Crossref | Google Scholar |
Tangney R, Miller RG, Fontaine JB, Veber WP, Ruthrof KX, Miller BP (2022) Vegetation structure and fuel dynamics in fire-prone, Mediterranean-type Banksia woodlands. Forest Ecology and Management 505, 119891.
| Crossref | Google Scholar |
Tolhurst K, Shields B, Chong D (2008) Phoenix: development and application of a bushfire risk management tool. The Australian Journal of Emergency Management 23, 47-54.
| Google Scholar |
Volkova L, Weston CJ (2019) Effect of thinning and burning fuel reduction treatments on forest carbon and bushfire fuel hazard in Eucalyptus sieberi forests of South-Eastern Australia. Science of The Total Environment 694, 133708.
| Crossref | Google Scholar | PubMed |
Volkova L, Sullivan AL, Roxburgh SH, Weston CJ (2016) Visual assessments of fuel loads are poorly related to destructively sampled fuel loads in eucalypt forests. International Journal of Wildland Fire 25, 1193-1201.
| Crossref | Google Scholar |
Wallace L, Hillman S, Hally B, Taneja R, White A, McGlade J (2022) Terrestrial laser scanning: an operational tool for fuel hazard mapping? Fire 5, 85.
| Crossref | Google Scholar |
Watson PJ, Penman SH, Bradstock RA (2012a) A comparison of bushfire fuel hazard assessors and assessment methods in dry sclerophyll forest near Sydney, Australia. International Journal of Wildland Fire 21, 755-763.
| Crossref | Google Scholar |
Wilson N, Car GJ, Gibbons P (2018) Relationships between mature trees and fire fuel hazard in Australian forest. International Journal of Wildland Fire 27, 353-362.
| Crossref | Google Scholar |
Footnotes
1 At the request of one of the anonymous reviewers, it is noted that in the original FHR assessment (McCarthy et al. 1998) the surface (i.e. litter) fuel layer was accessed not visually but from measurement of litter bed height. In the current system (Hines et al. 2010), the surface fuel hazard assessment relies on this height measurement plus the visual evaluation of two other attributes. Of the overall 17 attributes used in the overall fuel hazard rating, one consists of a measurement (litter bed height), with the remaining 16 being visual observations (Hines et al. 2010). The system is by and large a visual assessment method.
2 Given the approximate nature of the tabled fuel load values, these fuel loads have been called equivalent (McCarthy et al. 1998), indicative (Hines et al. 2010), or available (Gould et al. 2007b) fuel loads.
3 An assessment consists of the application of the method to an area of interest. An assessment can comprise several plots where FHR is estimated (Hines et al. 2010).
4 Simulations only included fuel ages above 9 years since fire because in a number of the sites inventoried a few years separated the structural fuel sampling from the hazard assessments. To overcome the bias that this could cause in the analysis, we restricted the analysis to the sites where the first sampling date was close or within the assumed steady-state phase of fuel dynamics (Adams and Neumann 2024). The arbitrary use of a 9 year cut off value came from the analysis of Marsden-Smedley et al. (2022) fuel dynamics curves.