Incorporating burn heterogeneity with fuel load estimates may improve fire behaviour predictions in south-east Australian eucalypt forest
Rachael H. Nolan

A
B
C
D
E
F
G
Abstract
Simulations of fire spread are vital for operational fire management and strategic risk planning.
To quantify burn heterogeneity effects on post-fire fuel loads, and test whether modifying fuel load estimates based on the fire severity and patchiness of the last fire improves the accuracy of simulations of subsequent fires.
We (1) measured fine fuels in eucalypt forests in south-eastern Australia following fires of differing severity; (2) modified post-fire fuel accumulation estimates based on our results; and (3) ran different fire simulations for a case-study area which was subject to a planned hazard reduction burn followed by a wildfire shortly thereafter.
Increasing fire severity resulted in increased reduction in bark fuels. In contrast, surface and elevated fuels were reduced by similar amounts following both low-moderate and high-extreme fire severity. Accounting for burn heterogeneity, and fire severity effects on bark, improved the accuracy of fire spread for a case study fire.
Integration of burn heterogeneity into post-burn fuel load estimates may substantially improve fire behaviour predictions.
Without accounting for burn heterogeneity, patchy burns of low severity may mean that risk estimations are incorrect. This has implications for evaluating the cost-effectiveness of planned burn programmes.
Keywords: burn severity, Eucalyptus, fire behaviour, fire history, fire management, modelling, prescribed burn, trees.
Introduction
Climate modified changes to fire regimes globally (Duane et al. 2021) are increasing the need for accurate predictions of wildfire behaviour. Fire behaviour models that predict fire intensity and rate of spread are vital tools for assessing the threat posed by wildfires and developing management responses (e.g. McArthur 1967; Van Wagner 1987). Simulations of fire sprefig.ad are used for operational purposes; e.g. allocating resources during wildfires, planning suppression tactics and evacuating communities (Calkin et al. 2011; Plucinski et al. 2017). These fire simulations are also used for strategic risk modelling, which can inform long-term management strategies such as fuel management (Florec et al. 2020; Penman and Cirulis 2020). Fire behaviour simulations require inputs of weather, topography and fuel load, with fuel load estimates having the largest uncertainty (Penman et al. 2022).
Fuel is aboveground biomass and varies spatially and temporally. In Australia, fuel is typically delineated into dead and live and coarse and fine fuel (Gould et al. 2011). Both the amount of fuel (fuel load, t ha−1), and the structure and arrangement of fuel are important drivers of fire behaviour (Stephens and Moghaddas 2005; McCaw et al. 2012). We note that the importance of fuel properties on fire behaviour, in particular fire spread, is a contentious issue within the Australian fire behaviour modelling community (Cruz et al. 2022b). Early research indicated that surface fine fuel load has a directly proportional effect on fire spread (McArthur 1967; Noble et al. 1980). More recent research has expanded to examine other fuel strata (such as elevated fuels), and has found that fuel properties (such as load and structure) have a diminishing effect on rate of spread under extreme fire weather conditions, particularly for wind-driven fires (Burrows 1999; Cruz et al. 2022b). Further, fuel structure may be more important than fuel load in driving fire spread under some circumstances (Zylstra et al. 2016; Cruz et al. 2022b). However, as noted by Storey et al. (2021) and Duff et al. (2017), the effect of fuel load on fire rate of spread may be difficult to assess due to limitations in fuel load data. Additionally, as argued by Cruz et al. (2022a), rate of spread is one component of fire behaviour. Fireline intensity and flame length (which are related) are directly proportional to fuel consumption, and are thus dependent on fuel quantity; i.e. fuel load (Alexander 1982; Cruz et al. 2022a).
Spatial variation in fuel loads is driven by biophysical factors that control the rate of fuel production, primarily biomass growth, and the rate of decomposition (Thomas et al. 2014; McColl-Gausden and Penman 2019). Temporal variation in fuels is driven by a range of factors such as rainfall (Pook et al. 1997; Archibald et al. 2010) and herbivory, particularly in rangelands (Starns et al. 2019). In forests, a large driver of temporal variation of fuel loads is time since fire. Following fire, fuel loads are heavily reduced, and then accumulate through time at a rate determined by site productivity and other environmental conditions (Fox et al. 1979; Raison et al. 1986; Penman and York 2010).
In Australia, fine fuels are defined as dead plant material <6 mm diameter, and live plant material <3 mm diameter, and are those fuels that burn in the continuous flaming zone (Hines et al. 2010), and thus contribute most to fire rate of spread (McCaw et al. 2012). Fine fuels are delineated into different strata. These strata are defined as litter, near surface (<0.5 m height, not including litter, e.g. herbaceous fuel), elevated (>0.5 m, primarily shrubs and tree saplings, but not overstorey trees), canopy (overstorey trees) and bark fuels (Hines et al. 2010; Price et al. 2022). In Australia, fuel loads, particularly for dead fuels, are commonly modelled as a function of time since fire using a negative exponential model adapted from Olson (1963). The Olson (1963) model assumes fuels reduce to zero after fire, and then slowly recover until a steady-state is achieved. In reality, fuels often are not completely consumed by fire (Price et al. 2022), and thus the Olson (1963) model has been adapted to reflect this, through the addition of an initial post-fire fuel load term (‘c’ in Eqn 1) (Fensham 1992; Morrison et al. 1996). The modified Olson (1963) fuel accumulation curve takes the form:
where Wt is fuel load (in t ha−1), c is the post-fire fuel load, r + c is the fuel load under steady-state (i.e. long unburnt) conditions, k is a constant related to decomposition, and t is years since fire.
Fuel accumulation curves are typically derived for different vegetation types for operational fire models in Australia and elsewhere (Watson 2012; Zazali et al. 2021). These curves are largely developed from observations of fuel loads over time since fire (Watson 2012; Penman et al. 2022). For litter these curves can also be derived from observations of litterfall and litter decomposition rates (Watson 2012). However, there is little empirical data on initial post-fire fuel loads, and they are often assumed to be zero, or close to it, for many vegetation types (Watson 2012; Zazali et al. 2021). Quantifying initial fuel loads is important since this will impact the rate of fuel accumulation and subsequent fuel loads, particularly in the early post-fire years. Quantifying fuel dynamics in these early post-fire years is important since planned burns are often conducted to reduce the risk posed by wildfire (Duff et al. 2019), and these burns are thought to be most effective in the immediate post-fire years. For example, the probability of high severity wildfire is often reduced within the first 5 years following fire, depending on fire weather (Hislop et al. 2020; Nolan et al. 2021). Further, rates of house loss during wildfires are often lower when the surrounding landscape was burnt in the previous 5 years (Gibbons et al. 2012; Nolan et al. 2021).
Fuel loads immediately post-fire will depend on fuel consumption. Fuel consumption, in turn, is dependent on many factors including pre-fire fuel load, fuel chemistry, fuel geometry, fuel moisture content, fuel density and fire weather and terrain (Ottmar 2014). Fire severity, by definition, describes the biotic impact of fire (Keeley 2009), and in Australia is typically measured as the relative fraction of foliage scorched or consumed by fire. In the weeks to months immediately following fire, fire severity can also affect the rate of post-fire fuel accumulation through effects on fuel inputs. For example, a fire that completely scorches the canopy will result in a large influx of dead fine fuels to the forest floor, which would not occur following a low severity fire that does not affect the canopy (Price et al. 2022). Thus, in the years following fire, fuel loads may vary as a function of fire severity. However, to date, fuel accumulation curves used operationally in Australia do not account for fire severity.
Most fires do not burn homogenously, and contain unburnt patches within the fire extent (Duff et al. 2019). For example, in smaller burns in dry sclerophyll forest in south-eastern Australia, burn coverage ranged from 6 to 90% (Penman et al. 2007), while burn coverage in larger burns ranged from 5 to 68% (McCarthy et al. 2017). For wildfires, a study on the 2019/20 Australian Black Summer fires found that 9% of the mapped burned area was unburnt (Collins et al. 2021). The extent of burn heterogeneity is driven in part by fuel properties, as well as fire weather conditions (Duff et al. 2019). Unburnt patches within a fire extent do not reduce fuel loads of the area within the fire perimeter. Therefore, improved burned area maps have the potential to improve fuel load estimates, and thus models of fire behaviour.
In this study, we (1) quantified changes in fuel loads with fire severity in temperate eucalypt forests; (2), adjusted fuel accumulation curves to account for fire severity where appropriate, as informed by (1); and (3) tested whether accounting for burn heterogeneity effects on fuel loads improved predictions of rates of spread. We did this by first measuring fine fuels in eucalypt forests in south-eastern Australia following fires of differing severity, including unburnt patches. We then adjusted existing fuel accumulation curves based on our observations of initial fuel loads. Finally, we examined effects of our adjusted fuel accumulation curves on predicted rates of fire spread for a case-study area which was subject to a planned hazard reduction burn followed by a wildfire less than 2 years later. We hypothesised that accounting for burn heterogeneity would improve predictions of rates of fire spread in future fire events.
Materials and methods
Study area
The study was undertaken in eucalypt forests within the state of New South Wales (NSW), in south-eastern Australia (Fig. 1). The climate is temperate, with warm summers and cold winters, with no dry season (Bureau of Meteorology 2022b). Mean annual rainfall ranges from 600 to 1500 mm, increasing towards the coast, and declining inland of the Great Dividing Range (a series of mountain ranges running approximately parallel with the coast; Bureau of Meteorology 2022b). Average minimum winter temperatures range from 0 to 9°C and average maximum summer temperatures range from 21 to 30°C (Bureau of Meteorology 2022b). Temperatures are coolest in the high elevation areas along the Great Dividing Range, and inland of the Range. Eucalypt forests dominate the native vegetation of the study area, and range from wet to dry sclerophyll forest types (Keith 2004). Ecologically sustainable fire intervals for these forests are estimated at 5–50 years for the dry sclerophyll forests and 25–60 years for the wet sclerophyll forests, with crown fires to be avoided at the lower range of the interval (Kenny et al. 2004).
(a) Location of study plots for fuel load observations and the case study 695 wildfire. (b) Fire severity of the 695 wildfire and mapped perimeter of the preceding hazard reduction burn. (c) Fire severity of the hazard reduction burn. Fire severity mapped following Gibson et al. (2020). Refer Fig. 2 to clearly see the intersection of the 695 wildfire and prior hazard reduction burn.
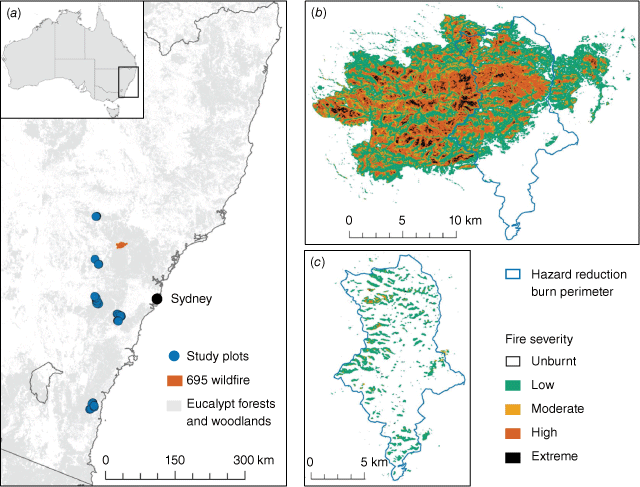
Changes in fuel loads with increasing fire severity
Post-fire fuel loads (t ha−1) were measured approximately 1 year following the 2019–20 Black Summer wildfires. Sampling at 1 year post-fire allowed for the influx of scorched foliage and branches from the canopy to the surface fuel layer, which can occur over the weeks and months following fire (Price et al. 2022). We measured loadings within four forest types: (1) western slopes dry sclerophyll; (2) Sydney hinterland dry sclerophyll; (3) south-east dry sclerophyll; and (4) Southern Tableland wet sclerophyll forests. Forest type classifications were based on vegetation classes described in Keith (2004). These forest types were selected because they span a relatively broad climatic gradient (670–1088 mm mean annual rainfall; Bureau of Meteorology 2022a), exhibit varying surface fine fuel loads (Watson 2012), and they were subject to a range of fire severities during the 2019–20 fires, but some unburnt forest remained in close proximity to burnt forest. The study design is described in greater detail in Nolan et al. (2022), which presents observations of post-fire tree mortality and above-ground carbon stocks
We measured 15 plots in each forest type spanning the range of fire severity classes. Fire severity was classified in the field into one of five categories following the severity class definitions of Gibson et al. (2020), and based on the proportion of leaf scorch and consumption in understorey and overstorey strata. Fire severity was assessed from satellite images, and confirmed in the field. Severity classes were: unburnt, low (<10% canopy scorch), moderate (20–90% canopy scorch), high (>90% canopy scorch but <50% canopy consumption) and extreme (>50% canopy consumption). We measured three unburnt plots for each forest type. We similarly aimed to measure three plots in each of the other fire severity classes, but this was not possible due to the nature of the wildfires where few locations burnt at both low and extreme severity. Due to the low replication in each fire severity category for some forest types (n = 2), we aggregated our plots into the following two fire severity categories: (1) ‘low-moderate’ fire severity, which represents predominantly understorey fire; (2) and ‘high-extreme’ fire severity, which represents homogenous canopy fire, n = 4–8 for these aggregated categories within each forest type.
We estimated fuel loads and assessed fuel hazard scores following the standard fuel classification used in eastern Australian forests (Hines et al. 2010). Fuel hazard scores are visual assessments of fuel that characterise the amount and connectivity of fine fuels (Hines et al. 2010). Fine fuels are defined as dead plant material <6 mm diameter, and live plant material <3 mm diameter Fine fuels are delineated into litter, near surface (<0.5 m height, not including litter, e.g. herbaceous fuel), elevated (>0.5 m, primarily shrubs and tree saplings, but not overstorey trees), canopy (overstorey trees) and bark fuels (Hines et al. 2010; Price et al. 2022). Canopy fuels are not included in most fire behaviour models in Australian forests, and so are not included in this study.
Fine fuels were assessed in circular plots of 45 m diameter, following Price et al. (2022). Within each plot, two 45-m transects were established, one along a north-south axis and one along an east-west axis. Litter and near-surface fuel loads were measured in three 1-m2 quadrats located approximately 12.5 and 32.5 m along the north and south transects and 7.5 m along the E transect. These fuels were destructively collected. For litter fuels, we weighed fresh biomass in the field and sub-sampled the fuel to estimate moisture content in the laboratory. The sub-sampled fuel was representative of the fuel across the fuel profile. For near-surface fuels, all fine fuel biomass was collected and transported to the laboratory. Fuel samples were stored in sealed plastic bags in a cooler for transport to the laboratory. Fuel samples were dried for 48 h at 105°C and dry mass measured. The moisture content of the litter fuels was then used to convert the fresh field-weights to dry weights.
Elevated fine fuels were measured in three circular sub-plots of 5 m diameter, unless sites exhibited very high stem density, then plots of 3 m diameter were used. These sub-plots were located at the same position as the quadrats. Within each sub-plot we measured the diameter at 10 cm height of all shrubs and recorded whether the plant was dead (i.e. lacking foliage) or alive. In burnt plots, we additionally assessed the fire impact on fine fuel biomass for each shrub by assessing the amount of foliage retained on the plant (live and dead) and the diameter of the shrub tips if all foliage had been consumed by fire. These stem diameters and shrub impact assessments were then used to estimate the total mass of fine fuels on the plant using allometric equations derived by Nolan et al. (2022) in the same study area. These equations were developed with five shrub and two tree seedling species, and performed similarly well to species-specific models Nolan et al. (2022).
For plants with live foliage, we estimated fine fuels as all biomass <3 mm diameter, but for plants with no live foliage, we assumed the biomass was dead and estimated fine fuels as all biomass <6 mm diameter. For non-woody elevated fuels, e.g. sedges and bracken fern (Pteridium esculentum), we counted the leaves on each plant and sub-sampled a section of leaves to measure oven-dry biomass in the laboratory. We then estimated plant-level biomass by multiplying the number of leaves by the average dry biomass per leaf. For burnt plots, we additionally identified whether elevated fine fuels were post-fire regrowth or retained from prior to the fire. Post-fire regrowth was easily identifiable as new seedlings and/or resprouting foliage from burnt plants.
We assessed bark fuels using visual fuel hazard assessments (Hines et al. 2010). Fuel hazard assessments were also undertaken for litter, near-surface and elevated fuels. Bark fuel assessments were based on bark type and the extent of charring on the trees, which provides an indicator of how tightly held the bark is and thus, its propensity for spotting and transport of fire to the crown. Litter fuel hazard assessments are based on the depth and coverage of the litter bed. Near-surface fuel hazard assessments are based on the percentage of plant cover and the percentage of dead fuels. Elevated fuels are assessed on the percentage plant cover and the percentage of dead fuels, and an additional assessment of fuel continuity. All visual hazard assessments classified fuels into five hazard scores ranging from ‘low’ to ‘extreme’ fuel hazard. We converted bark hazard scores into fine fuel loads using a look-up table in Hines et al. (2010). We note that these conversion tables often provide low accuracy (Watson et al. 2012; Volkova et al. 2016). However these conversions are routinely used by fire agencies in Australia to estimate fuel loads for predicting fire behaviour.
For each forest type, we assessed whether fine fuel loads and fuel hazard scores varied with fire severity, with a one-way ANOVA, and where this was significant, we undertook Tukey adjusted multiple comparisons. We confirmed that the data met ANOVA assumptions of homogeneity of variance and normality, assessed through visual inspection of diagnostic plots and Levene’s test. Many fuel strata data violated these assumptions, and so we used a Kruskal–Wallis test followed by a Dunn post hoc test. We undertook analyses on litter, near surface, elevated and bark fuel strata. For elevated fuels, we assessed all fine fuels present at the time of the study (1 year post-fire), in addition to fine fuels excluding post-fire regrowth. We additionally examined litter and near surface fuels combined (hereafter referred to as ‘surface’ fuels, since this is an input into the fire behaviour model used in this study. For the fuel hazard score data, we converted the hazard score categories into numerical values on an ordinal scale from 1 to 5.
We modified existing fuel accumulation coefficients (Eqn 1) for each vegetation type based on our observations of fine fuel loads following fire and in long unburnt forest. That is, we modified the parameters r (steady-state fuel load) and c (initial post-fire fuel load) in Eqn 1, based on our observations. Fuel accumulation curves were modified for surface (i.e. litter and near surface combined), elevated, and bark fuels. We tested to see whether c varied with fire severity, and if so, developed modified curves for both low and high severity fire.
Burn heterogeneity effects on subsequent fire spread
Our case-study wildfire was the ‘695’ fire, which burnt 19,090 ha in February 2018 (Fig. 1b). The 695 fire progressed over multiple days. We selected this fire as our case-study because a prior hazard reduction burn (conducted in June 2016; Figs 1c, 2), was expected to slow or limit the spread of the 695 wildfire, but this did not occur. While the area of the mapped hazard reduction burn was 10,172 ha, our analysis of fire severity found over 80% of the mapped burn area remained unburnt (see below for details on fire severity mapping). Where it did burn, the hazard reduction burn was primarily an understorey fire of low to moderate severity (Fig. 1c).
Fire history within the study area. (a) Fire history using current burned area mapping, and (b) fire history modified by fire severity mapping to account for unburnt patches within fire perimeters.
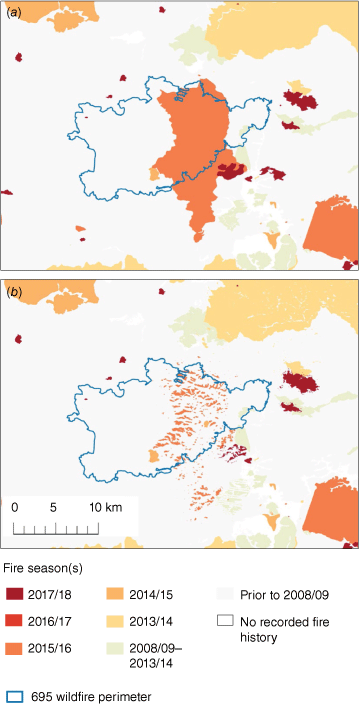
The 695 fire burnt predominately within Wollemi National Park, which is a 501,000 ha park located 130 km north-west of Sydney. Mean annual rainfall is estimated at 812 mm, and mean monthly minimum temperatures range from 3 to 14°C and mean monthly maximum temperatures range from 13 to 28°C. Climate data extracted from the SILO Australian Climate Dataset (http://www.longpaddock.qld.gov.au/silo; Jeffrey et al. 2001). Vegetation reflects that found in the wider study area, i.e. includes wet and dry sclerophyll forest types.
Fire history mapping was obtained from the New South Wales Rural Fire Service. Fire severity of the wildfire and hazard reduction fire, as well as all observed fire dating to the 1989–1990 fire year, was mapped with satellite imagery following Gibson et al. (2020), which uses an extensively trained and tested Random Forest supervised classification algorithm applied to satellite imagery from before and after the fire (Gibson et al. 2020; Department of Planning Industry and Environment 2021). The fire severity mapping approach in Gibson et al. (2020) classifies unburnt and extreme fire severity with very high accuracy (mean balanced accuracy statistic >0.95), low and high fire severity with high accuracy (mean >0.80) and moderate fire severity with relatively lower accuracy (mean >0.70).
The hazard reduction fire was mapped using Landsat satellite imagery (30 m resolution), whereas the wildfire was mapped using the higher resolution sensor Sentinel 2 (10 m) which was launched between the two fire events. The combined use of Landsat and Sentinel 2 sensors in remote sensing applications for fire extent and severity mapping has demonstrated substantial agreement between the outputs from the different sensors (White and Gibson 2022).
We used PHOENIX Rapidfire ver. 4.0.0.721 (PHOENIX) to model rates of fire spread for the case-study wildfire. For forests, the fire behaviour model underpinning PHOENIX is the McArthur Mk5 forest fire behaviour model (McArthur 1967; Noble et al. 1980). In the McArthur fire behaviour model fuel load has a directly proportional effect of fire rate of spread. PHOENIX dynamically simulates two-dimensional fire growth using Huygen’s algorithm (Knight and Coleman 1993). PHOENIX utilises 30-m gridded datasets of topography, fuel type and disruptions to fuel such as roads and waterbodies. Surface, elevated and bark fuel loads are estimated from fuel accumulation curves based on time-since-fire and fuel type. We used fuel accumulation coefficients specific to the vegetation types in the study area. For further details see Tolhurst et al. (2008), Saeedian et al. (2010) and Penman et al. (2014).
We ran PHOENIX simulations for the date of 14 February, from 11:00 hours to 23:00 hours, which represented the day with the greatest fire spread. This was also the day that the fire intersected the perimeter of the 2016 hazard reduction burn. We ran our simulations using the fire perimeter from the previous day. Fire progression in the preceding days to the 14th was minimal, so we do not present simulations for these dates. Weather data used for the PHOENIX simulations was sourced from the Australian Digital Forecast Database (The Bureau of Meteorology 2023).
We ran three PHOENIX simulations:
A simulation based on current operational models and fire history (‘current operational model’).
A simulation where fire history was modified by fire severity mapping to account for unburnt patches within historical fire perimeters (Fig. 2, ‘burn heterogeneity model’). This simulation addresses whether fire patchiness is important.
This simulation is the same as the previous simulation, with fuel accumulation curves modified based on our analyses of changes in fuel loads with fire severity. As detailed in the results, we found that only bark fuels differed significantly in response to fire severity. Hence, this simulation is termed ‘burn heterogeneity and bark model’.
For simulations (2) and (3), fire history was modified by severity mapping for all fires that occurred within the study area, dating to the 1989–1990 fire year (Fig. 2). This modified fire history incorporates all mapped historical fires which may affect modelled fuel accumulation, with fuel accumulation curves generally obtaining steady-state fuel loads within 25–30 years. For further details, see Supplementary Tables S3 and S4.
PHOENIX outputs a number of fire behaviour metrics, these include area burnt (ha), flame height (m), fireline intensity (kW m−1), rate of spread (m h−1), and spotting distance (m). We present violin plots of these metrics for each of the simulations. Note that it is not appropriate to conduct statistical tests of differences in these metrics, since each simulation represents one fire event (i.e. n = 1). Thus, the grid cells are not independent data points. To assess how each of the three PHOENIX simulations compared to the observed extent of the wildfire, we calculated the Jaccard similarity index. The Jaccard index is defined by the intersection of two binary maps, divided by their union.
Results
Changes in fuel loads with fire severity
Fire reduced fuel loads across all strata examined (Fig. 3); however, there was generally no change in fuel loads with increasing fire severity, with the exception of bark fuel loads (Fig. 3, Table S1). At 1 year post-fire, surface fuel loads ranged from 12.6 to 25.2 t ha−1 in unburnt forest, with fire reducing these fuel loads to 2.6–5.6 t ha−1. The surface fuels were primarily comprised of litter fuels, which ranged from 10.5 to 25.1 t ha−1 in unburnt forest, with near surface fuels only ranging from 0.1 to 2.4 t ha−1 (Fig. S1). The wet sclerophyll forest was the only forest type where higher fire severity was associated with significantly lower fuel loads at 1 year post-fire. For this forest type surface fuel loads following low-moderate severity fire were 5.3 t ha−1 and following high-extreme severity fire was 2.6 t ha−1.
Variation in fine fuel loads as a function of fire severity. Fuel strata represented are (a–d) surface fuels (i.e. litter and near surface fuel strata combined), (e–h) elevated fuels and (i–l) bark fuels. Data illustrated are averages ±1 s.e. Differing letters above bars indicate significant differences among fire severity classes (P < 0.05). For elevated fuels, lower case letters represent differences among fire severity categories at 1 year post-fire, while upper cases letters represent differences among fire severity classes excluding post-fire regrowth (i.e. the approximate fuel load immediately post-fire).
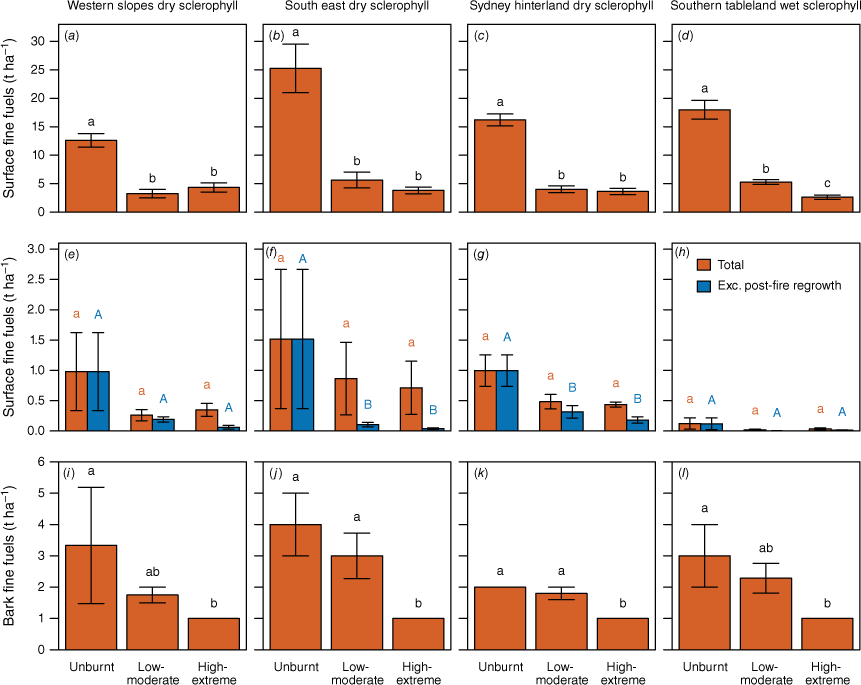
Elevated fine fuel loads ranged from 0.1 to 1.5 t ha−1 in unburnt forest (Fig. 3). Notably, there was large variation in elevated fuel loads within unburnt forest, as evidenced by high standard errors, which ranged from 0.1 to 1.1 t ha−1. At 1 year post-fire elevated fuels did not differ significantly between burnt and unburnt forest for any of the forest types. However, when the post-fire re-growth was excluded, which provides an approximation of immediate post-fire fuel loads, we did observe significantly lower fuel loads in burnt forest for two of the four forest types. Again, there was no change with fire severity.
Bark fine fuels were estimated to range widely (2–4 t ha−1) in unburnt forest (Fig. 3). Forest burnt at high-extreme fire severity had significantly lower bark fuel loads compared to unburnt forest, estimated at 1 t ha−1 for all four forest types. Forest burnt at low-moderate fire severity had intermediate fuel loads (1.8–3 t ha−1), which did not differ significantly from unburnt forest.
Fuel hazard scores showed similar trends to the fuel load data (Fig. S2, Table S2). That is, litter hazard scores were significantly lower in burnt compared to unburnt forest, but there was no effect of the severity of the fire. There was no significant effect of fire for near surface hazard scores for any of the forest types, with the exception of the Southern tableland wet sclerophyll forest, where the near surface hazard scores were significantly lower in forest burnt at high-extreme fire severity compared to unburnt forest. For elevated hazard scores, despite a trend of lower scores for burnt compared to unburnt forest, there was no significant differences observed, except for the Western slopes dry sclerophyll forest. For this forest type, forest burnt at low-moderate fire severity had significantly lower hazard scores compared to unburnt forest. Finally, for bark hazard scores, there were significantly lower values for forest burnt at high-extreme fire severity, but no difference between unburnt forest, and forest burnt at low-moderate severity.
Adjusted fuel accumulation curves
Modified fuel accumulation curves for surface fuels mostly aligned with existing curves (Fig. 4a–d), indicating that the steady-state and initial fuel load parameters in the current curves were similar to our field-based observations. The exception was the South-east dry sclerophyll forest type, where there were large differences between the current and modified curves, due to differences in the estimates of steady-state fuels. The current curves assume steady-state surface fuels are 12.0 t ha−1, while we observed fuel loads of 25.2 t ha−1 (Table S3).
Fuel accumulation curves for surface (a–d), elevated (e–h) and bark (i–l) fine fuels. Represented are the current fuel accumulation curves used operationally (‘Original model’), in addition to modified curves based on our observations of fuel loads in long unburnt forest and at 1 year post-fire. Note, where we did not observe a significant effect of fire severity on post-fire fuel loads, we pooled observations across fire severity classes to estimate the initial fuel load. Where we observed no significant effect of fire on fuel loads, we did not model fuel accumulation, instead assuming fuels remained at steady-state conditions.
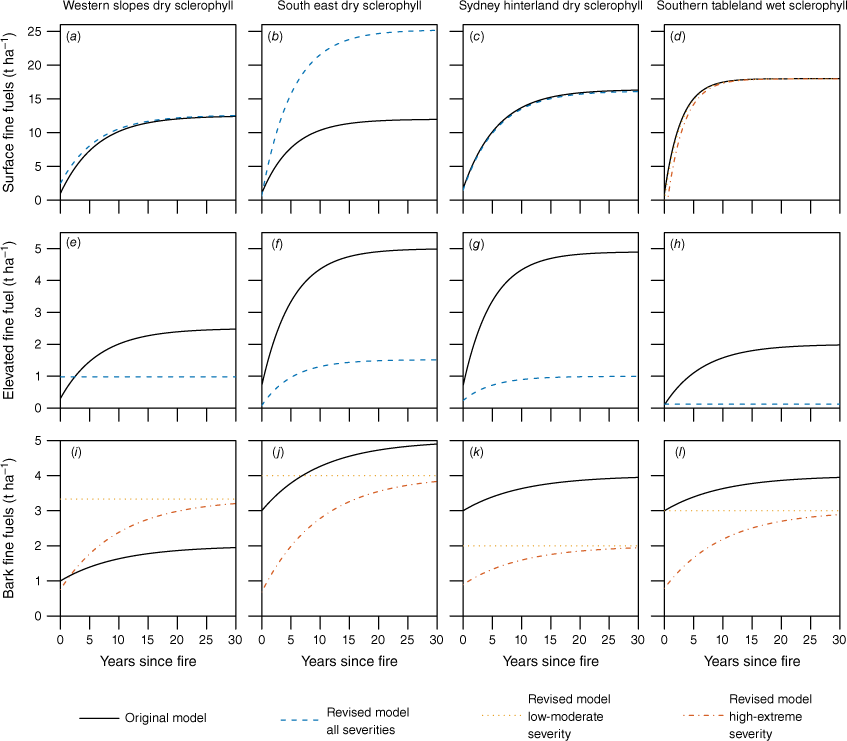
In contrast to surface fuels, there were considerable variations between current and modified curves for elevated fuels (Fig. 4e–h). This was primarily due to our estimates of steady-state fuels (0.1–1.5 t ha−1) being much lower than those in current curves (2.0–5.0 t ha−1). Our estimates of elevated fuel loads over time-since-fire also differed for two of the four forest types, where we did not observe significant differences in initial post-fire fuel loads in elevated fuels, and thus did not model fuel accumulation over time since fire.
For bark fuels, our modified fuel accumulation curves for high-extreme fire severity were either lower or higher than current operational curves (Fig. 4e–f). The differences between our curves and the operational curves reflect our differing observations of steady-state fuel loads. For example, steady-state bark fuel load estimates were considerably higher for the Western slopes dry sclerophyll forest in our modified curves (3.3 t ha−1), compared to current curves (2 t ha−1), resulting in much higher estimated fuel loads over time since fire. For forests burnt at low-moderate fire severity, where we did not observe a significant difference between burnt and unburnt forest, we found no change in fuel loads over time since fire, and thus our estimates of bark fuel loads were much higher in the early post-fire years compared with the current curves.
Burn heterogeneity effects on subsequent fire spread
The first PHOENIX simulation (‘current operational’ simulation), predicted that the spread of the case study 695 wildfire would be limited by the hazard reduction burn conducted in the preceding 2 years (Fig. 5a). In contrast, the PHOENIX simulation that accounted for the unburnt patches within historical fire perimeters (the ‘burn heterogeneity’ simulation, Fig. 5b) resulted in a much larger predicted burned area. For this simulation, the prior hazard reduction burn was predicted to have no effect on the spread of the wildfire (Fig. 5b). For the third simulation (‘Burn heterogeneity and bark’ simulation, Fig. 5c), we additionally modified bark fuel accumulation curves such that bark fine fuels were not modelled to decline following understorey fire, based on our results (Fig. 4). We additionally modified bark fuel accumulation curves based on our observations of steady state fuel loads. This third simulation predicted a burned area that was most similar to the observed burned area of the 695 wildfire (Fig. 5d).
PHOENIX simulations: (a) current operational; (b) ‘burn heterogeneity’ – fire history modified by fire severity mapping to account for unburnt patches within fire perimeters; and (c) ‘Burn heterogeneity and bark’, same as (b), in addition to bark fuel accumulation curves being modified, based on field survey data.
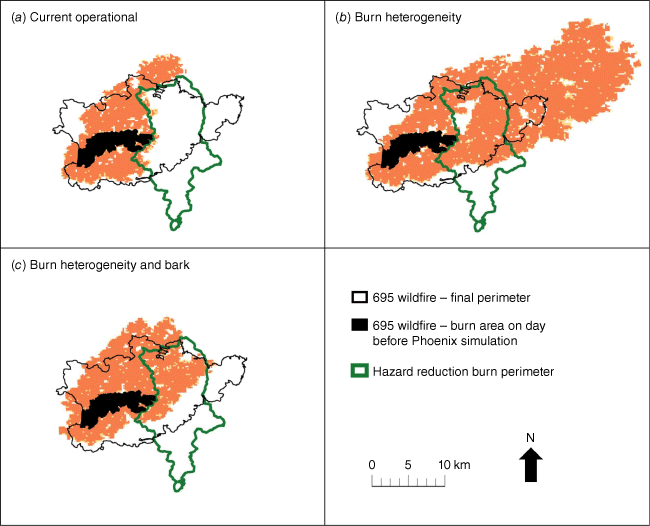
For all three PHOENIX simulations, predicted flame height, fireline intensity and rate of spread were all similar (Fig. 6). However, the predicted spotting distance for the third simulation (median value of 469 m) was lower compared to the second simulation (median value of 674 m), reflecting the lower predicted bark fuel loads used as inputs in this simulation. This lower spotting distance resulted in a smaller burned area compared to the second simulation (15,348 ha compared to 32,441 ha). The simulation that was most similar to the actual burn area was the third simulation (Jaccard index of 0.51, compared to 0.30–0.38; Table 1).
Boxplot summaries of fire behaviour from PHOENIX simulations, including (a) area burnt, (b) flame height, (c) fireline intensity, (d) rate of spread, and (e) spotting distance. The simulations are: (1) current operational; (2) ‘burn heterogeneity’ – fire history modified by fire severity mapping to account for unburnt patches within fire perimeters; and (3) ‘Burn heterogeneity and bark’, same as (b), in addition to bark fuel accumulation curves being modified, based on field survey data.
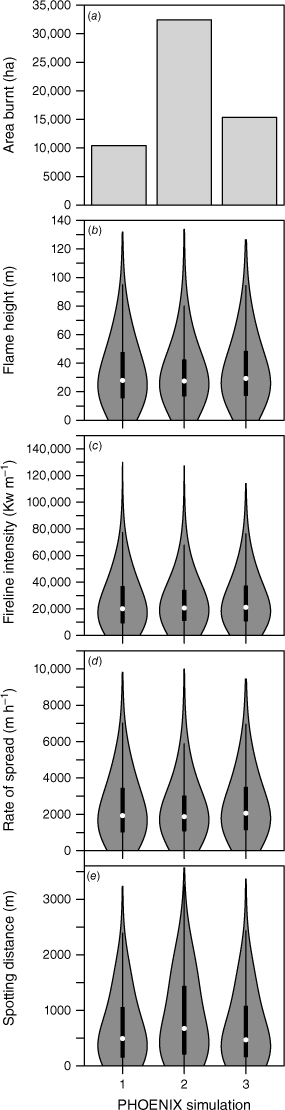
Discussion
Both wildfire and planned burns are known to be highly heterogeneous in burn area and fire severity (Penman et al. 2007; McCarthy et al. 2017; Collins et al. 2021). Our results demonstrate that accounting for burn heterogeneity has the potential to improve predictions of fire spread (Fig. 5). Here, we discuss how fire severity mapping can potentially improve model predictions of fuel loads, and subsequently, fire behaviour predictions.
Changes in fuel loads with fire severity
Similar to this study, Price et al. (2022) found post-fire surface fuel loads did not differ due to fire severity. Price et al. (2022) attributed this to post-fire litter deposits increasing with fire severity, offsetting the increased consumption of surface fuels. Surprisingly, we also found no significant effect of differing fire severity on elevated fuels at 1 year post-fire, as both understorey and canopy fire similarly reduce elevated fuel loads. This similarity in elevated fuel loads may reflect the smaller impacts of fire on the understorey in forest burnt at low to moderate fire severity, and rapid recovery of understorey vegetation in forest burnt at high to extreme severity in the year after the fire. In forest burnt at high to extreme severity, the high-light environment facilitates prolific post-fire recruitment in eucalypt forests (Bennett et al. 2016). We also found no effect of fire severity on the shrub density (Table S5). Other studies found elevated fuel loads, or shrub density and cover, are affected by fire severity (e.g. Landesmann et al. 2021; Barker et al. 2022). These studies generally examine elevated fuel trajectories over time or at a later time period than our study. These studies argue that increasing fire severity creates an environment with high light and reduced competition in the understorey while the canopy recovers (Bennett et al. 2016; Gordon et al. 2017; Etchells et al. 2020) and in turn this promotes increased shrub growth, and hence increased elevated fuel loads.
We observed spatial variation in fuel loads, particularly for elevated fuels. For example, in south-east dry sclerophyll forest, elevated fine fuel loads in unburnt forest ranged from 0.1 to 3.8 t ha−1 (Fig. 3f). Large spatial variability in elevated fuels has similarly been observed in south-east Australian eucalypt forests (McColl-Gausden et al. 2020; Nolan et al. 2022). There was less spatial variation in surface fine fuel loads when compared to elevated fuels (Fig. 3). The exception was the south-east dry sclerophyll forest type where our surface fuel load observations in unburnt forest were much higher than those modelled in current operational fuel curves (Fig. 4b). This may have been because the study plots were transitional between the dry sclerophyll forest and the nearby wet sclerophyll forest, which has much higher fuel loads. Spatial variation in fuels within vegetation communities is partially due to historical variation in fire frequency, severity and seasonality, which all affect plant recruitment (Enright et al. 2015; Miller et al. 2019). Additionally, factors that affect species distributions, such as fine-scale variation in soil attributes and topography, likely affect fuel loads. Thus, fuel load models may be improved by modelling fuels as a function of biophysical parameters that vary at a finer spatial resolution than vegetation communities. Indeed, recent biophysical models of fuel hazard scores have found that climatic and edaphic factors are good predictors of fuel hazard scores (Jenkins et al. 2020; McColl-Gausden et al. 2020).
Adjusted fuel accumulation curves
Our modified bark fuel accumulation curves following canopy fire were either lower or higher than current operational curves, reflecting differences in steady-state fuel loads. For the western slopes dry sclerophyll forest, we observed higher bark fine fuel loads in unburnt forest than predicted by current fuel curves. This variation from the fuel curve was due to the presence of species which produce higher bark fuels than the ironbark species which typically characterise this vegetation type (Hines et al. 2010; Horsey and Watson 2012). For the other three forest types, we observed lower bark fine fuel loads in both unburnt and burnt forest than predicted by current fuel curves. These results also reflect differences in the presence of species that produce high bark fuel loads, e.g. stringybark species, which produce the highest fine fuel loads (Hines et al. 2010).
Burn heterogeneity effects on subsequent fire spread
Accounting for burn heterogeneity in fuel load predictions was found to improve fire spread simulations from PHOENIX for our case study 695 wildfire (Fig. 5, Table 1). A previous hazard reduction burn had been predicted to limit the spread of the 695 wildfire. However, when the large area of unburnt patches within prior fire perimeters was incorporated into fire history mapping, the previous hazard reduction burn was no longer predicted to limit the spread of the wildfire. Thus, fine-scale resolution burned area mapping has the potential to improve fire behaviour simulations. As demonstrated here, utilising fire severity maps to improve fire history mapping substantially improves fuel load estimates, and thus predicted fire behaviour. Further improvements in predicted fire spread were gained by modifying bark fuel accumulation curves based on field observations (Fig. 5c). Eucalypt bark produces firebrands that can travel large distances, igniting new fires (McArthur 1967; Penman et al. 2017). Thus, improved models of fine fuel loads, particularly bark fuels that affect spotting distance, can further improve predictions of fire spread. However, we note that improved fuel load mapping may not necessarily lead to improved fire behaviour simulations under all weather conditions, with fuel exerting less influence on fire behaviour under more extreme fire weather conditions (Clarke et al. 2022).
While our results suggest that patchy hazard reduction burns may not be as effective as those with a homogenous burned area in reducing fire spread under wildfire conditions, it is important to note that fire refugia are important for supporting biodiversity and maintaining ecosystem processes (Cawson et al. 2013; Krawchuk et al. 2020). Indeed, many planned burns, including hazard reduction burns, have the aim of creating a mosaic of burnt and unburnt areas (Duncan et al. 2015). Our results also suggest that high severity fire is most effective at reducing bark fuel loads; however, it is important to note that our study does not consider longer-term vegetation dynamics that may affect fuel load and structure, and subsequent fire behaviour, that may operationally influence the ability to contain a fire or result in fires escaping. For example, a study by Barker et al. (2022) in the same region found that at 5 years post-fire, areas burnt at high severity had greater elevated fuel loads and fuel connectivity compared to areas burnt at low severity. In a related study, Barker and Price (2018) found that crown fire was more than twice as likely following a previous crown fire, compared to a previous understory fire. Similarly, in a study on mixed conifer forest in the USA, Coppoletta et al. (2016) found that the effects of high severity fire on standing deadwood and shrubs promoted high severity fire in subsequent fires. We also note that Barker and Price (2018) found that the lowest likelihood of high severity fire occurred in the longest unburnt forest, which indicates that the shape of fuel accumulation curves may need to be modified, particularly for elevated fuels (Zazali et al. 2021).
Conclusions
Fuel attributes are key inputs into fire behaviour models. Spatial fuel load predictions are improved by incorporating fire severity mapping into fuel models. Thus, improving fuel load estimates has the potential to substantially improve the accuracy of fire behaviour predictions. However, further research is required to determine whether the results from our case study are more widely applicable. Such studies should include wildfires that burn at a range of times following prior fire, and under a range of weather conditions. Any improvements to fire behaviour models are vitally important for informing operational decision making around suppression and providing early warning to the community during wildfire events. Further, improved fuel estimates will improve fire simulations for strategic risk modelling. Without accounting for burn heterogeneity, patchy burns may mean that risk estimations are low. This has implications for evaluating the cost-effectiveness of planned burn programmes.
We suggest that improved spatial resolution of burn area mapping (e.g. through fire severity mapping) is a relatively simple step with the potential to substantially improve fire behaviour predictions. Currently in Australia, government fire management agencies in NSW and Victoria routinely map fire severity following compatible methodologies, while agencies in other states are in the process of developing compatible severity mapping systems. Further improvements may also be gained from improved models of fuel dynamics, particularly bark fuel loads.
Declaration of funding
This research was supported by funding from the New South Wales Department of Planning, and Environment (DPE), via the NSW Bushfire Risk Management Research Hub, and through the Hermon Slade Foundation. The NSW Fire Extent and Severity Mapping (FESM) program has been supported by funding from DPE and the NSW Rural Fire Service since 2018.
Acknowledgements
We thank Katherine Rolls, Kirsty Milner, Ross Bradstock, Eli Bendall, Georgia Watson and Belinda Kenny for fieldwork assistance. The NSW National Parks and Wildlife Service facilitated site access and provided information on study sites. We acknowledge the traditional custodians and knowledge holders of the Country where we conduct our research, walk and live, in particular the Wiradjuri, Yuin, Wonnarua/Wanaruah, Dharawal and Gundungurra traditional owners of the areas where this study was located.
References
Alexander ME (1982) Calculating and interpreting forest fire intensities. Canadian Journal of Botany 60, 349-357.
| Crossref | Google Scholar |
Archibald S, Nickless A, Scholes RJ, Schulze R (2010) Methods to determine the impact of rainfall on fuels and burned area in southern African savannas. International Journal of Wildland Fire 19, 774-782.
| Crossref | Google Scholar |
Barker JW, Price OF (2018) Positive severity feedback between consecutive fires in dry eucalypt forests of southern Australia. Ecosphere 9, e02110.
| Crossref | Google Scholar |
Barker JW, Price OF, Jenkins ME (2022) High severity fire promotes a more flammable eucalypt forest structure. Austral Ecology 47, 519-529.
| Crossref | Google Scholar |
Bennett LT, Bruce MJ, MacHunter J, Kohout M, Tanase MA, Aponte C (2016) Mortality and recruitment of fire-tolerant eucalypts as influenced by wildfire severity and recent prescribed fire. Forest Ecology and Management 380, 107-117.
| Crossref | Google Scholar |
Bureau of Meteorology (2022a) Available at http://www.bom.gov.au/ [accessed 17 January 2022]
Bureau of Meteorology (2022b) Long-range weather and climate. Available at http://www.bom.gov.au/climate/ [accessed 17 January 2022]
Burrows ND (1999) Fire behaviour in Jarrah forest fuels: 1. Laboratory experiments. CALMScience 3, 31-56.
| Google Scholar |
Calkin DE, Thompson MP, Finney MA, Hyde KD (2011) A real-time risk assessment tool supporting wildland fire decisionmaking. Journal of Forestry 109, 274-280.
| Crossref | Google Scholar |
Cawson JG, Sheridan GJ, Smith HG, Lane PNJ (2013) Effects of fire severity and burn patchiness on hillslope-scale surface runoff, erosion and hydrologic connectivity in a prescribed burn. Forest Ecology and Management 310, 219-233.
| Crossref | Google Scholar |
Clarke H, Cirulis B, Penman T, Price O, Boer MM, Bradstock R (2022) The 2019–2020 Australian forest fires are a harbinger of decreased prescribed burning effectiveness under rising extreme conditions. Scientific Reports 12, 11871.
| Crossref | Google Scholar | PubMed |
Collins L, Bradstock RA, Clarke H, Clarke MF, Nolan RH, Penman TD (2021) The 2019/2020 mega-fires exposed Australian ecosystems to an unprecedented extent of high-severity fire. Environmental Research Letters 16, 044029.
| Crossref | Google Scholar |
Coppoletta M, Merriam KE, Collins BM (2016) Post-fire vegetation and fuel development influences fire severity patterns in reburns. Ecological Applications 26, 686-699.
| Crossref | Google Scholar | PubMed |
Cruz MG, Alexander ME, Fernandes PM (2022a) Evidence for lack of a fuel effect on forest and shrubland fire rates of spread under elevated fire danger conditions: implications for modelling and management. International Journal of Wildland Fire 31, 471-479.
| Crossref | Google Scholar |
Cruz MG, Cheney NP, Gould JS, McCaw WL, Kilinc M, Sullivan AL (2022b) An empirical-based model for predicting the forward spread rate of wildfires in eucalypt forests. International Journal of Wildland Fire 31, 81-95.
| Crossref | Google Scholar |
Department of Planning Industry and Environment (2021) Fire extent and severity mapping. Annual report for the 2019–20, 2018–19 and 2017–18 fire years NSW Government. https://www.environment.nsw.gov.au/research‐and‐publications/publications‐search/fire‐extent‐and‐severity‐mapping‐annual‐report‐2019‐20
Duane A, Castellnou M, Brotons L (2021) Towards a comprehensive look at global drivers of novel extreme wildfire events. Climatic Change 165, 43.
| Crossref | Google Scholar |
Duff TJ, Keane RE, Penman TD, Tolhurst KG (2017) Revisiting wildland fire fuel quantification methods: the challenge of understanding a dynamic, biotic entity. Forests 8, 351.
| Crossref | Google Scholar |
Duff TJ, Cawson JG, Penman TD (2019) Determining burnability: Predicting completion rates and coverage of prescribed burns for fuel management. Forest Ecology and Management 433, 431-440.
| Crossref | Google Scholar |
Duncan BW, Schmalzer PA, Breininger DR, Stolen ED (2015) Comparing fuels reduction and patch mosaic fire regimes for reducing fire spread potential: A spatial modeling approach. Ecological Modelling 314, 90-99.
| Crossref | Google Scholar |
Enright NJ, Fontaine JB, Bowman DM, Bradstock RA, Williams RJ (2015) Interval squeeze: altered fire regimes and demographic responses interact to threaten woody species persistence as climate changes. Frontiers in Ecology and the Environment 13, 265-272.
| Crossref | Google Scholar |
Etchells H, O’Donnell AJ, McCaw WL, Grierson PF (2020) Fire severity impacts on tree mortality and post-fire recruitment in tall eucalypt forests of southwest Australia. Forest Ecology and Management 459, 117850.
| Crossref | Google Scholar |
Fensham RJ (1992) The management implications of fine fuel dynamics in bushlands surrounding Hobart, Tasmania. Journal of Environmental Management 36, 301-320.
| Crossref | Google Scholar |
Florec V, Burton M, Pannell D, Kelso J, Milne G (2020) Where to prescribe burn: the costs and benefits of prescribed burning close to houses. International Journal of Wildland Fire 29, 440-458.
| Crossref | Google Scholar |
Fox BJ, Fox MD, McKay GM (1979) Litter accumulation after fire in a eucalypt forest. Australian Journal of Botany 27, 157-165.
| Crossref | Google Scholar |
Gibbons P, van Bommel L, Gill AM, Cary GJ, Driscoll DA, Bradstock RA, Knight E, Moritz MA, Stephens SL, Lindenmayer DB (2012) Land management practices associated with house loss in wildfires. PLoS One 7, e29212.
| Crossref | Google Scholar | PubMed |
Gibson R, Danaher T, Hehir W, Collins L (2020) A remote sensing approach to mapping fire severity in south-eastern Australia using sentinel 2 and random forest. Remote Sensing of Environment 240, 111702.
| Crossref | Google Scholar |
Gordon CE, Price OF, Tasker EM, Denham AJ (2017) Acacia shrubs respond positively to high severity wildfire: Implications for conservation and fuel hazard management. Science of the Total Environment 575, 858-868.
| Crossref | Google Scholar | PubMed |
Gould JS, McCaw WL, Phillip Cheney N (2011) Quantifying fine fuel dynamics and structure in dry eucalypt forest (Eucalyptus marginata) in Western Australia for fire management. Forest Ecology and Management 262, 531-546.
| Crossref | Google Scholar |
Hines F, Tolhurst KG, Wilson AAG, McCarthy GJ (2010) ‘Overall Fuel Hazard Assessment Guide, 4th edn. Fire and Adaptive Management Report no. 82. (East Melbourne). https://www.ffm.vic.gov.au/__data/assets/pdf_file/0005/21110/Report‐82‐overall‐fuel‐assess‐guide‐4th‐ed.pdf
Hislop S, Stone C, Haywood A, Skidmore A (2020) The effectiveness of fuel reduction burning for wildfire mitigation in sclerophyll forests. Australian Forestry 83, 255-264.
| Crossref | Google Scholar |
Jeffrey SJ, Carter JO, Moodie KB, Beswick AR (2001) Using spatial interpolation to construct a comprehensive archive of Australian climate data. Environmental Modelling & Software 16, 309-330.
| Crossref | Google Scholar |
Jenkins ME, Bedward M, Price O, Bradstock RA (2020) Modelling bushfire fuel hazard using biophysical parameters. Forests 11, 925.
| Crossref | Google Scholar |
Keeley JE (2009) Fire intensity, fire severity and burn severity: a brief review and suggested usage. International Journal of Wildland Fire 18, 116-126.
| Crossref | Google Scholar |
Knight I, Coleman J (1993) A fire perimeter expansion algorithm-based on Huygens wavelet propagation. International Journal of Wildland Fire 3, 73-84.
| Crossref | Google Scholar |
Krawchuk MA, Meigs GW, Cartwright JM, Coop JD, Davis R, Holz A, Kolden C, Meddens AJH (2020) Disturbance refugia within mosaics of forest fire, drought, and insect outbreaks. Frontiers in Ecology and the Environment 18, 235-244.
| Crossref | Google Scholar |
Landesmann JB, Tiribelli F, Paritsis J, Veblen TT, Kitzberger T (2021) Increased fire severity triggers positive feedbacks of greater vegetation flammability and favors plant community-type conversions. Journal of Vegetation Science 32, e12936.
| Crossref | Google Scholar |
McCarthy G, Moon K, Smith L (2017) Mapping fire severity and fire extent in forest in Victoria for ecological and fuel outcomes. Ecological Management & Restoration 18, 54-65.
| Crossref | Google Scholar |
McCaw WL, Gould JS, Phillip Cheney N, Ellis PFM, Anderson WR (2012) Changes in behaviour of fire in dry eucalypt forest as fuel increases with age. Forest Ecology and Management 271, 170-181.
| Crossref | Google Scholar |
McColl-Gausden SC, Penman TD (2019) Pathways of change: Predicting the effects of fire on flammability. Journal of Environmental Management 232, 243-253.
| Crossref | Google Scholar | PubMed |
McColl-Gausden SC, Bennett LT, Duff TJ, Cawson JG, Penman TD (2020) Climatic and edaphic gradients predict variation in wildland fuel hazard in south-eastern Australia. Ecography 43, 443-455.
| Crossref | Google Scholar |
Miller RG, Tangney R, Enright NJ, Fontaine JB, Merritt DJ, Ooi MKJ, Ruthrof KX, Miller BP (2019) Mechanisms of fire seasonality effects on plant populations. Trends in Ecology & Evolution 34, 1104-1117.
| Crossref | Google Scholar | PubMed |
Morrison DA, Buckney RT, Bewick BJ, Cary GJ (1996) Conservation conflicts over burning bush in south-eastern Australia. Biological Conservation 76, 167-175.
| Crossref | Google Scholar |
Noble IR, Gill AM, Bary GAV (1980) McArthur’s fire‐danger meters expressed as equations. Australian Journal of Ecology 5, 201-203.
| Crossref | Google Scholar |
Nolan RH, Bowman DMJS, Clarke H, Haynes K, Ooi MKJ, Price OF, Williamson GJ, Whittaker J, Bedward M, Boer MM, Cavanagh VI, Collins L, Gibson RK, Griebel A, Jenkins ME, Keith DA, Mcilwee AP, Penman TD, Samson SA, Tozer MG, Bradstock RA (2021) What do the Australian Black Summer fires signify for the global fire crisis? Fire 4, 97.
| Crossref | Google Scholar |
Nolan RH, Price OF, Samson SA, Jenkins ME, Rahmani S, Boer MM (2022) Framework for assessing live fine fuel loads and biomass consumption during fire. Forest Ecology and Management 504, 119830.
| Crossref | Google Scholar |
Olson JS (1963) Energy storage and the balance of producers and decomposers in ecological systems. Ecology 44, 322-331.
| Crossref | Google Scholar |
Ottmar RD (2014) Wildland fire emissions, carbon, and climate: Modeling fuel consumption. Forest Ecology and Management 317, 41-50.
| Crossref | Google Scholar |
Penman TD, Cirulis BA (2020) Cost effectiveness of fire management strategies in southern Australia. International Journal of Wildland Fire 29, 427-439.
| Crossref | Google Scholar |
Penman TD, York A (2010) Climate and recent fire history affect fuel loads in Eucalyptus forests: Implications for fire management in a changing climate. Forest Ecology and Management 260, 1791-1797.
| Crossref | Google Scholar |
Penman TD, Kavanagh RP, Binns DL, Melick DR (2007) Patchiness of prescribed burns in dry sclerophyll eucalypt forests in South-eastern Australia. Forest Ecology and Management 252, 24-32.
| Crossref | Google Scholar |
Penman TD, Bradstock RA, Price OF (2014) Reducing wildfire risk to urban developments: Simulation of cost-effective fuel treatment solutions in south eastern Australia. Environmental Modelling & Software 52, 166-175.
| Crossref | Google Scholar |
Penman TE, Cawson JG, Murphy S, Duff TJ (2017) Messmate stringybark: bark ignitability and burning sustainability in relation to fragment dimensions, hazard score and time since. International Journal of Wildland Fire 26, 866-876.
| Crossref | Google Scholar |
Penman TD, McColl-Gausden SC, Cirulis BA, Kultaev D, Ababei DA, Bennett LT (2022) Improved accuracy of wildfire simulations using fuel hazard estimates based on environmental data. Journal of Environmental Management 301, 113789.
| Crossref | Google Scholar | PubMed |
Plucinski MP, Sullivan AL, Rucinski CJ, Prakash M (2017) Improving the reliability and utility of operational bushfire behaviour predictions in Australian vegetation. Environmental Modelling & Software 91, 1-12.
| Crossref | Google Scholar |
Pook EW, Gill AM, Moore PHR (1997) Long-term variation of litter fall, canopy leaf area and flowering in a Eucalyptus maculata forest on the south coast of New South Wales. Australian Journal of Botany 45, 737-755.
| Crossref | Google Scholar |
Price OH, Nolan RH, Samson SA (2022) Fuel consumption rates in resprouting eucalypt forest during hazard reduction burns, cultural burns and wildfires. Forest Ecology and Management 505, 119894.
| Crossref | Google Scholar |
Raison RJ, Woods PV, Khanna PK (1986) Decomposition and accumulation of litter after fire in sub-alpine eucalypt forests. Australian Journal of Ecology 11, 9-19.
| Crossref | Google Scholar |
Starns HD, Fuhlendorf SD, Elmore RD, Twidwell D, Thacker ET, Hovick TJ, Luttbeg B (2019) Recoupling fire and grazing reduces wildland fuel loads on rangelands. Ecosphere 10, e02578.
| Crossref | Google Scholar |
Stephens SL, Moghaddas JJ (2005) Experimental fuel treatment impacts on forest structure, potential fire behavior, and predicted tree mortality in a California mixed conifer forest. Forest Ecology and Management 215, 21-36.
| Crossref | Google Scholar |
Storey MA, Bedward M, Price OF, Bradstock RA, Sharples JJ (2021) Derivation of a Bayesian fire spread model using large-scale wildfire observations. Environmental Modelling & Software 144, 105127.
| Crossref | Google Scholar |
The Bureau of Meteorology (2023) Australian Digital Forecast Database (ADFD), Version 21. http://www.bom.gov.au/catalogue/adfdUserGuide.pdf
Thomas PB, Watson PJ, Bradstock RA, Penman TD, Price OF (2014) Modelling surface fine fuel dynamics across climate gradients in eucalypt forests of south-eastern Australia. Ecography 37, 827-837.
| Crossref | Google Scholar |
Tolhurst KG, Shields B, Chong D (2008) Phoenix: development and application of a bushfire risk management tool. Australian Journal of Emergency Management 23, 47-54.
| Crossref | Google Scholar |
Volkova L, Sullivan AL, Roxburgh SH, Weston CJ (2016) Visual assessments of fuel loads are poorly related to destructively sampled fuel loads in eucalypt forests. International Journal of Wildland Fire 25, 1193-1201.
| Crossref | Google Scholar |
Watson PJ, Penman SH, Bradstock RA (2012) A comparison of bushfire fuel hazard assessors and assessment methods in dry sclerophyll forest near Sydney, Australia. International Journal of Wildland Fire 21, 755-763.
| Crossref | Google Scholar |
White LA, Gibson RK (2022) Comparing Fire Extent and Severity Mapping between Sentinel 2 and Landsat 8 Satellite Sensors. Remote Sensing 14, 1661.
| Crossref | Google Scholar |
Zazali HH, Towers IN, Sharples JJ (2021) A critical review of fuel accumulation models used in Australian fire management. International Journal of Wildland Fire 30, 42-56.
| Crossref | Google Scholar |
Zylstra P, Bradstock RA, Bedward M, Penman TD, Doherty MD, Weber RO, Gill AM, Cary GJ (2016) Biophysical mechanistic modelling quantifies the effects of plant traits on fire severity: species, not surface fuel loads, determine flame dimensions in eucalypt forests. PLoS One 11, e160715.
| Crossref | Google Scholar | PubMed |