Determining the sensitivity of grassland area burned to climate variation in Xilingol, China, with an autoregressive distributed lag approach
Ali Hassan Shabbir A B C , Jiquan Zhang A B C G , Xingpeng Liu A B C , James A. Lutz D , Carlos Valencia E and James D. Johnston FA Institute of Natural Disaster Research, School of Environment, Northeast Normal University, Changchun, 130024, China.
B State Environmental Protection Key Laboratory of Wetland Ecology and Vegetation Restoration, Northeast Normal University, Changchun, 130024, China.
C Key Laboratory for Vegetation Ecology, Ministry of Education, Changchun, 130024, China.
D Wildland Resources Department, Utah State University, 5230 Old Main Hill, Logan, UT 84322-5230, USA.
E Industrial Engineering Department, University of los Andes, Carrera 1 Este No. 19 A 40, Bogota, Colombia.
F College of Forestry, Oregon State University, 140 Peavy Hall, 3100 SW Jefferson Way, Corvallis, OR 97333, USA.
G Corresponding author. Email: zhangjq022@nenu.edu.cn
International Journal of Wildland Fire 28(8) 628-639 https://doi.org/10.1071/WF18171
Submitted: 2 May 2018 Accepted: 8 May 2019 Published: 1 July 2019
Journal Compilation © IAWF 2019 Open Access CC BY-NC-ND
Abstract
We examined the relationship between climate variables and grassland area burned in Xilingol, China, from 2001 to 2014 using an autoregressive distributed lag (ARDL) model, and describe the application of this econometric method to studies of climate influences on wildland fire. We show that there is a stationary linear combination of non-stationary climate time series (cointegration) that can be used to reliably estimate the influence of different climate signals on area burned. Our model shows a strong relationship between maximum temperature and grassland area burned. Mean monthly wind speed and monthly hours of sunlight were also strongly associated with area burned, whereas minimum temperature and precipitation were not. Some climate variables like wind speed had significant immediate effects on area burned, the strength of which varied over the 2001–14 observation period (in econometrics terms, a ‘short-run’ effect). The relationship between temperature and area burned exhibited a steady-state or ‘long-run’ relationship. We analysed three different periods (2001–05, 2006–10 and 2011–14) to illustrate how the effects of climate on area burned vary over time. These results should be helpful in estimating the potential impact of changing climate on the eastern Eurasian Steppe.
Additional keywords: ARDL model, climate change, climate sensitivity, grassland fire.
Introduction
Changing climate and land-use practices have had a significant impact on the productivity, community composition, structure and function of many terrestrial ecosystems (Mooney et al. 2009; Kipling et al. 2016; Zhang et al. 2016). Changes to ecosystem structure and function as a result of disturbance are more difficult to predict than directional climate change. An understanding of the sensitivity of grasslands – lands dominated by grasses as opposed to trees or shrubs – to climate-mediated changes such as fire is especially important because grasslands are one of the most spatially extensive vegetation types in the world, accounting for ~25% of the global land surface. Changes to grasslands could have global implications for resource cycling and carbon sequestration (Yang et al. 2016).
Anthropogenically derived activities are important drivers of grassland degradation, especially in developing countries (Yang et al. 2005; Verón et al. 2006; Bliege Bird et al. 2016; Gowlett 2016). Some studies have attributed grassland degradation to temperature increases and changes in precipitation seasonality (Ravi et al. 2010; Yeganeh et al. 2014), whereas other studies report that it may be difficult to differentiate between causal factors (Wessels et al. 2007; Gang et al. 2014). Less attention has been focused on fire-mediated change, and few studies have quantified the relationship between climate variability and fire extent in grasslands at large spatial scales (Zheng et al. 2006; Wessels et al. 2008).
Fire regimes of grasslands and forests have been analysed at multiple temporal and spatial scales (Abatzoglou and Williams 2016; Francos et al. 2016; Garbolino et al. 2016; Gaudreau et al. 2016; Harris et al. 2016; Kukavskaya et al. 2016; Liu and Wimberly 2016; Tian et al. 2016; Liu et al. 2017). Previous research suggests that extreme weather is an important determinant of fire severity and extent. Increased temperatures and wind speeds and lower relative humidity are correlated with increases in fire size, intensity and severity (Argañaraz et al. 2015; Holsinger et al. 2016). In many regions, wildfire activity has fluctuated dramatically during the last century, and fire regimes have been altered in most geographic regions (Ikemori et al. 2015; Semeraro et al. 2016). Analysis of the eastern Eurasian Steppe has only recently become possible with the availability of national fire datasets (Eugenio et al. 2016).
The objective of the present study was to examine changes in area burned in northern China given intra- and interannual climate trends. To accomplish this objective, we used an econometrics method to study the relationship between area burned and climate time series that are non-stationary, that is, the mean and the variance of the series do not remain constant during the observation period. In econometrics, it is common to estimate the effect on a response of interest, e.g. stock performance, given non-stationary trends in time series predictor variables, e.g. inflation and market capitalisation. In this financial market example, investigators are interested in relating past and present observations of predictor and response variables to future observations of the response. These effects can be characterised either as ‘short-run’, or immediate fluctuations in the response given a change in a predictor variable, or ‘long-run’ relationships from which variables deviate but always return to over the entire period of observation. In the case of grassland area burned in Xilingol over time, we used an autoregressive distributed lag (ARDL) approach to determine the sensitivity of grassland area burned to climate trends. We present a detailed account of the application of this methodology and its potential usefulness to the fire ecology community.
Data description and methodology
Study area and climate data
The Xilingol League (a league is an administrative unit of the autonomous region of Inner Mongolia within China) is located on the eastern Eurasian steppe in northern China (Fig. 1). The total area of Xilingol is 2.03 × 107 ha, of which 70% is grasslands. Xilingol grasslands are among the most productive prairies in China (Kobayashi et al. 1994; Chen et al. 2008), and have been shown to be highly sensitive both to climate change and human activity, including human-caused fire (Hardy 2005; Kaloudis et al. 2005; Zhijun et al. 2009).
Between 2001 and 2014, 564 fires within Xilingol burned 29 092 ha. Most of the fires (94.6%) occurred between March and October. Fire activity was somewhat bimodal, with large areas burned in April–May and also in September–October (Fig. 2). Area burned in Xilingol increased from 2001 to 2014, although the interannual and spatial pattern of fire varied during this time period (Fig. 3). This variability suggests potentially complex interactions between different climate drivers of fire.
![]() |
We used six climate variables to predict area burned: monthly average maximum temperature (Tmax), monthly average minimum temperature (Tmin), monthly precipitation (Precip), monthly average wind speed, monthly average sunlight, and monthly average relative humidity (percentage of saturation humidity). Two of these variables were weakly correlated (sunlight hours and relative humidity) and two variables were strongly correlated (Tmin and Tmax). Following the practice of most econometric analyses, we ignored multicollinearity of variables in implementing the ARDL model because the degree of differencing of data using this method tends to decompose model residuals and eliminate multicollinearity. Also, in theory, multicollinearity only inflates the errors of regression coefficients but coefficients remain unbiased (Farrar and Glauber 1967; Goldberger 1991). Climate data from 2001 to 2014 were taken from the China Meteorological Data Sharing Service Centre (https://data.cma.cn/en, last accessed 20 May 2019). Data for grassland area burned were acquired from the Monitoring Centre of the Ministry of Agriculture (http://www.moa.gov.cn/, last accessed 20 May 2019). The basic meteorological and burned area data used in the present paper are included in the online supplemental material.
ARDL model
The available climate data that are potentially explanatory of grassland area burned in Xilingol League are time series. A critical assumption of ordinary least-squares analysis methods that could be used to evaluate the relationship between area burned and climate is stationarity, i.e. the means and variances of the time series are constants that are independent of time. Failure to account for non-stationarity in time series risks spurious regression results in which t-statistics and measures of model fit are misleadingly significant (Granger and Newbold 1974; Phillips 1986).
This problem can be avoided by determining if there is a stationary linear combination of non-stationary climate variables, or cointegration of time series. Analysing multiple time series that are cointegrated can provide important information about system behaviour over long time periods. We used the ARDL cointegration model, which allowed us to test for the existence of long-term climate–fire relationships, irrespective of whether the underlying regressors require no differencing to obtain a stationary series (I(0)), require one order of differencing (I(l)), or are mutually cointegrated (Pesaran et al. 2001; Oteng-Abayie and Frimpong 2006). Model fitting was performed using Microfit 5.5 (Pesaran and Pesaran 2010; Pesaran 2015).
The ARDL model was originally proposed by Pesaran et al. (1999, 2001). The ARDL methodology is graphically illustrated in Fig. 4. According to several authors (e.g. Pesaran et al. 2001; Haug 2002; Oteng-Abayie and Frimpong 2006), the ARDL methodology is preferable to test cointegration when there is a single long-run relationship among the response and covariates and there are no long-run relations in the conditional error correction form of the covariates in terms of the response. In other words, there is a reduced form on which all underlying series are weakly exogenous and taken as explanatory variables. Among these explanatory variables, there can be a mix of I(0) and I(1) series, and one or more cointegrated relations. It is important to note that in a case where one of the time series in question is integrated of order ≥2, the ARDL method cannot be applied.
![]() |
In the case of our study, some variables were demonstrated to be stationary, whereas some of them required one order of differencing. In addition, we were interested in the impact of the climate variables on grassland burned area through a single equation, and therefore, the ARDL cointegration approach was the most pertinent econometric model to use in order to estimate long-run and short-run dynamics from the error correction model (ECM) specification. We also selected this methodology because ARDL modelling performs better with a smaller sample size than other cointegration techniques (Haug 2002; Abdullah Yusof and Duasa 2010). For instance, the ARDL methodology was shown to achieve good performance in measurement of environmental quality related indicators (such as sandy desertification and deforestation) (Ahmed et al. 2015; Ge et al. 2016).
As a first step (see Fig. 4), we used augmented Dickey–Fuller (ADF) (Dickey and Fuller 1979) and Phillips–Perron (PP) (Phillips 1987) unit root tests to test the order of integration for each variable. ARDL bounds testing requires that all variables should be integrated at purely order I(0), purely order I(1) or mutually cointegrated (Oteng-Abayie and Frimpong 2006). Therefore, it is necessary to test the integrating order of all variables before applying ARDL bounds testing; otherwise, the calculation of the F statistic of ARDL becomes invalid (Baum 2004).
The general formulation of an ARDL (n,m) model with a lag m for exogenous variables, , and lag n for response variable Y is:

where are the effects of the level and lagged exogenous variables on the response Y at time t. Coefficients
represent the autoregressive effect of past realisations of Y,
is the intercept term and
is the white noise error. This model can be reformulated as an error correction model (ECM) considering the marginal changes with the lag − 1 difference operator (with
, and
, as:

where, in the case of cointegration, δ represents the speed of adjustment to long-run equilibrium deviations. That is, the error correction term (ECMt) specifies the magnitude of the observed divergence from the equilibrium equation between Yt and Xt that should be corrected in each following period, and δ determines how much time it will take to come again to the long-run equilibrium position. In order to estimate the parameters and
that correspond to the short-run dynamics, the unobservable variables ECMt are estimated from the long-run parameters, and for a significant ECM, the parameter δ should be negative.
We natural-log-transformed response and predictor variables, which allowed us to report model coefficients as ‘elasticities’, or measures of how a variable changes in response to another variable. Unlike partial slope coefficients, which represent the unit change in the response variable for a unit change in the predictor variable, coefficients in the log–log model represent the estimated percentage change in the dependent variable for a percentage change in the independent variable. If cointegration exists, the long-run relation of the response with the covariables, in the particular case of our study, can be written as:

where νt is a zero mean stationary process and grassland area burned is in units of hectares, Tmin, and Tmax in degrees Celsius, Precip in millimetres, Humidity in percentage, Wind in metres per second, and Sunlight is in hours.
For testing cointegration, the conditional error correction model in Eqn 2 is expressed as:
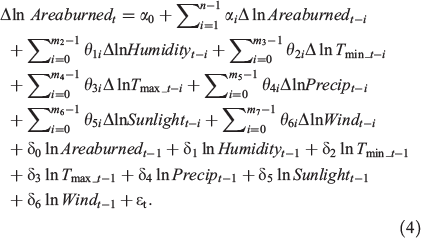
The parameters α and θ represent the short-run dynamics of the model. For each of the covariables, the long-run parameter βj is can be estimated from Eqn 4 as , for j = 1,2,…6. Parametrisation of the model in Eqn 4 is preferred in order to implement the bound test for cointegration Pesaran et al. (2001). Therefore, the null hypothesis of no long-run relationship between the variables is H0: δ0 = δ1 = δ2 = δ3 = δ4 = δ5 = δ6 = 0 and is checked against the alternative hypothesis H1: at least one δj ≠ 0, j = 0,1…6. Note that this test allows for degenerate long-run relations in the model, as stated in the alternative hypothesis.
Parameters in Eqn 4 can be estimated by ordinary least-squares (OLS) and the test statistics correspond to the partial F statistics for simultaneous significance of the lagged level of the variables. Narayan (2005) provides critical boundaries for the asymptotic distribution of the F statistic appropriate for small sample sizes. If the computed F statistic is greater than Narayan’s upper critical boundary, then we conclude there is cointegration between the variables. If the computed F statistic is less than the lower critical boundary, then there is no cointegration among the variables. We used the Akaike information criterion, Schwarz Bayesian criterion and Hannan–Quinn information criterion to determine the optimal lag selection (Schwarz 1971; Hannan and Quinn 1978; Akaike 1981).
After confirming long-run relationship among the variables, we applied the cumulative sum (CUSUM) and cumulative sum of squares (CUSUMSQ) tests developed by Brown et al. (1975). These tests are used to check the goodness of fit for ARDL as suggested by Pesaran et al. (2001). These tests are incorporated on the residuals of the error correction model and display results in graphical form. Plots of CUSUM and CUSUMSQ that fall within the 5% critical band demonstrate model stability.
Area burned increased over time and the spatial pattern of fire within Xilingol varied over time (Fig. 3). The ARDL model ultimately demonstrated both long-run and short-run effects of climate on area burned. To provide an easy to interpret demonstration of the effect of climate signals on area burned at different temporal scales, we calculated correlation coefficients of climate parameters and area burned for three different time periods: 2001 to 2005, 2006 to 2010, and 2010 to 2014.
Results
Unit root tests
The results of the unit root test are shown in Table 1. In the ADF test, ln Tmin and ln Wind were stationary at a 5% significance level, whereas in the PP test, ln Tmax and ln Precip were stationary at a 10% significance level. However, all variables became stationary after considering the first difference, which is confirmed by most of our unit root test approaches. Thus, they are indicated at order 1. The absence of a I(2) variable corroborates the application of the ARDL bounds-testing technique.
Bound testing for cointegration analysis
Before testing for cointegration, it is important to select the correct number of lags in y(n) considered in the ARDL model in order to avoid misspecification problems Pesaran et al. (2001). The use of one lag in the model was indicated by most selection criteria (Table 2).
The results of the ARDL bounds testing and critical values are presented in Table 3. The calculated value of the F statistic (5.11) was greater than the upper bound values (FU) at 5 and 10% levels of significance respectively, which demonstrates the existence of long-run relationships among climate variables irrespective of whether the covariables are I(0), I(1) or mutually cointegrated. From this result, it is possible to find long-run coefficients and short-run dynamics from the conditional vector ARDL equation with error correction term.
![]() |
Long-run coefficients
Once cointegration is proved, there is a linear combination of all variables, including predictors and response, that is stationary. This long-run relationship is scaled such that the coefficient of the grassland area burned is 1 (Table 4). Results are estimated from the selected ARDL model according to the Schwarz Bayesian criterion. Significant long-run effects calculated at a 5% significance level are wind, maximum average temperature and sunlight expressed in logarithmic scale. From these long-run elasticities, it is estimated that a 1% change in wind is associated with a 1.05% change in area burned. This long-run elasticity is not expressed instantaneously, but represents the long-term recovery to the stationary equilibrium. Similarly, a 1% change in average maximum temperature and sunlight are expected to produce a 1.98% change in area burned.
The reliability of the long-run results depends on an accurate specification of the ARDL model that meets all assumptions of this method. We performed several tests to validate the model. First, we checked the functional form of the model by applying Ramsey’s RESET test (Ramsey 1969). The results demonstrate the appropriateness of the functional form of the model both in the linear model (LM) version and F-version of diagnostic checks. We also performed Durbin–Watson tests, which indicated no first-order serial correlation (P = 0.154). Diagnostics also revealed no heteroscedasticity (P = 0.987). In addition, model residuals were shown to follow a normal distribution. Tests results are presented in the lower part of Table 4.
ARDL error correction model
Short-run coefficients from the ECM are displayed in Table 5. A 1% increase in sunlight (which can be interpreted as fires occurring closer to 21 June, the period of maximum sunlight) was associated with a 0.27% increase in area burned. A 1% increase in wind speed was associated with a 0.13% increase in area burned. A 1% change in relative humidity was associated with a 0.18% change in area burned. The lagged ECM term is negative as expected and statistically significant, implying that 60% of changes in grassland area burned were explained by short-run deviations from the long-run equilibrium. The relationship between area burned and different climate variables is shown in Fig. 5.
![]() |
To check the stability of the ECM, we incorporated CUSUM and CUSUMSQ tests. The results of the tests are presented in graphical form in Fig. 6. These plots demonstrate that the CUSUM and CUSUMSQ lines are within the critical band of 5% significance level over time, which confirms the stability of the model.
![]() |
Correlation analysis (shown in Table 6) demonstrates that simple models of grassland burned area produced more significant results when dividing the study period (2010–14) into three parts, 2001 to 2005, 2006 to 2010 and 2011 to 2014. Interestingly, we found that significance of some climate variables changed over these three periods. For example, during 2011 to 2014 sunlight was highly correlated with the area burned, but over the remaining periods, it was not. The significance of precipitation also varied during different time periods.
Discussion
Time series climate data with different temporal properties present several challenges for researchers. In the case of the present study, some climate time series data used to explain grassland area burned were stationary, whereas other data were stationary after differencing. ADRL bound testing methodology is well suited for these mixed time series orders. In addition, given that no variable had integration order larger than 1, and that cointegration in a single equation including the response grassland burned area was proved, it was possible to reparametrise the model as an ECM. With this type of model, relevant parameters can be estimated in a unique methodological framework. In particular, the elasticities (long-run coefficients), the short-run dynamics and the tendency of the series to recover the long-run stationary level explain the relevant information about the relations between the climate covariables and the response.
Absent cointegration among the grassland area burned and climate variables, regression analysis would have resulted in spurious relations with no reliable interpretation. Regression analysis of climate–fire relationships is only appropriate with integrated time series when there exists a linear combination of the variables at a level (same time) that behaves as a stationary process. Cointegration matches the order of non-stationarity of different variables in a form that combined with a specific linear equation produces a new predictive series. In addition, the same coefficients of this combination represent the long-run equilibrium relations that give important information about the effects on the response variable.
ARDL analysis revealed that maximum temperature is the most important variable affecting grassland fires in Xilingol (Table 4), a finding consistent with other studies in northern China and worldwide that show a relationship between higher temperatures and increased fire activity (Kobayashi et al. 1994; Su and Liu 2004; Hardy 2005; Kaloudis et al. 2005; Zhijun et al. 2009; Chen et al. 2008). Guo et al. (2016) found that as the temperature increased, the amount of boreal forest burned in China increased, even when increased temperatures were accompanied by higher than normal precipitation. Wind and sunlight also explain significant long-term changes in area burned.
Climate influences on grassland area burned in the eastern Eurasian steppe may vary given the temporal scale examined. Liu et al. (2017) analysed climate influences on daily fire progression in Hulun Buir, a region of the Inner Mongolia Autonomous Area north-east of Xilingol. They found positive correlations between grassland area burned and higher temperature, wind speed and sunlight, and negative correlations with precipitation and relative humidity. At the monthly and annual time steps analysed in the present study, we found precipitation to be less influential on fire extent. But our study and the findings of Liu et al. suggest that temperature exerts a powerful influence on fire behaviour at every spatial and temporal scale examined to date.
Directional temperature increases are likely to significantly increase winter temperatures relative to summer temperatures, and warmer winter and spring temperatures are likely to extend fire seasons (Westerling et al. 2006; Deser et al. 2012). Increases in area burned in Xilingol in May through October were associated with higher temperatures, but there were weak correlations between climate signals and area burned in February, March and April, suggesting that a threshold for increased winter and early spring fire activity in the eastern Eurasian steppe has not yet been reached.
Previous research in China has not incorporated wind into fire models (Yang et al. 2008; Zhang et al. 2010; Li et al. 2017). Wind played a key role in increasing grassland area burned in Xilingol, similarly to results from other regions (Moritz et al. 2010; Rolinski et al. 2016; Keeley and Syphard 2017). Higher wind speeds in the early season (e.g. February, R2 = 0.018, P > 0.012) can outweigh the effect of low temperatures. From 2001 to 2014, there were four monthly peaks in wind speed (May, August, September and October), with the highest peak in May. We found that the number of extreme events that occurred in 2003, 2004 and 2014 was associated with higher area burned in the northern regions (R2 = 0.810, P < 0.002).
Climate change and human factors are likely to continue to transform grasslands in northern China. Climate projections for north-eastern China predict higher temperatures along with lower precipitation and relative humidity Niu and Zhai (2012). Liu et al. (2012) also indicated that the expected change in population density is correlated with temperature as well as the change in precipitation. However, the effects of altered human ignitions cannot be easily predicted (Guo et al. 2016). Given the natural range of variation in this region, neither precipitation, relative humidity nor minimum temperature affected grassland area burned. But wind speed was a key factor in grassland area burned in northern areas, and the ARDL methods identified both long-term and short-term sensitivities.
Conclusion
Both climate change and human factors are likely to continue to transform grasslands in northern China. Climate projections for north-eastern China include higher temperatures along with lower precipitation and relative humidity (Niu and Zhai 2012). Projected changes in the wind regime are as yet unknown. Increases in population density are likely to occur in conjunction with increasing temperature as well as changes in precipitation patterns (Liu et al. 2012). However, the effects of altered human ignitions cannot be easily predicted (Guo et al. 2016).
The ARDL method identified both long-term and short-term sensitivities, showing that it can be a valuable tool in investigating trends in area burned, even in the presence of high variability and short periods of climatological data. In particular, the present study found that prevailing winds were an important determinant of area burned and also suggests that the wind regime may not be stationary.
Conflicts of interest
The authors declare they have no conflicts of interest.
Acknowledgements
This research was funded by the National Natural Science Foundation of China under grant no. 41301584, the National Key Technology R&D Program of China under grant nos 2013BAK05B01 and 2013BAK05B02, and the Youth Foundation of Jilin province (no. 20140520156JH), and the Program for Introducing Talents to Universities (B16011). We are grateful to the associate editor and two anonymous reviewers for extremely helpful comments and suggestions that significantly improved the manuscript.
References
Abatzoglou JT, Williams AP (2016) Impact of anthropogenic climate change on wildfire across western US forests. Proceedings of the National Academy of Sciences of the United States of America 113, 11770–11775.| Impact of anthropogenic climate change on wildfire across western US forests.Crossref | GoogleScholarGoogle Scholar | 27791053PubMed |
Abdullah Yusof S, Duasa J (2010) Household decision-making and expenditure patterns of married men and women in Malaysia. Journal of Family and Economic Issues 31, 371–381.
| Household decision-making and expenditure patterns of married men and women in Malaysia.Crossref | GoogleScholarGoogle Scholar |
Ahmed K, Shahbaz M, Qasim A, Long W (2015) The linkages between deforestation, energy and growth for environmental degradation in Pakistan. Ecological Indicators 49, 95–103.
| The linkages between deforestation, energy and growth for environmental degradation in Pakistan.Crossref | GoogleScholarGoogle Scholar |
Akaike H (1981) Likelihood of a model and information criteria. Journal of Econometrics 16, 3–14.
| Likelihood of a model and information criteria.Crossref | GoogleScholarGoogle Scholar |
Argañaraz JP, Pizarro GG, Zak M, Landi MA, Bellis LM (2015) Human and biophysical drivers of fires in semiarid Chaco mountains of central Argentina. The Science of the Total Environment 520, 1–12.
| Human and biophysical drivers of fires in semiarid Chaco mountains of central Argentina.Crossref | GoogleScholarGoogle Scholar | 25782079PubMed |
Baum CF (2004) A review of Stata 8.1 and its time series capabilities. International Journal of Forecasting 20, 151–161.
| A review of Stata 8.1 and its time series capabilities.Crossref | GoogleScholarGoogle Scholar |
Bliege Bird R, Bird DW, Codding BF (2016) People, El Niño Southern Oscillation and fire in Australia: fire regimes and climate controls in hummock grasslands. Philosophical Transactions of the Royal Society B 371, 20150343
| People, El Niño Southern Oscillation and fire in Australia: fire regimes and climate controls in hummock grasslands.Crossref | GoogleScholarGoogle Scholar |
Brown RL, Durbin J, Evans JM (1975) Techniques for testing the constancy of regression relationships over time. Journal of the Royal Statistical Society: Series B Methodological 37, 149–163.
| Techniques for testing the constancy of regression relationships over time.Crossref | GoogleScholarGoogle Scholar |
Chen J, Hori Y, Yamamura Y, Shiyomi M, Huang DM (2008) Spatial heterogeneity and diversity analysis of macrovegetation in the Xilingol region, Inner Mongolia, China, using the beta distribution. Journal of Arid Environments 72, 1110–1119.
| Spatial heterogeneity and diversity analysis of macrovegetation in the Xilingol region, Inner Mongolia, China, using the beta distribution.Crossref | GoogleScholarGoogle Scholar |
Deser C, Phillips A, Bourdette V, Teng H (2012) Uncertainty in climate change projections: the role of internal variability. Climate Dynamics 38, 527–546.
| Uncertainty in climate change projections: the role of internal variability.Crossref | GoogleScholarGoogle Scholar |
Dickey DA, Fuller WA (1979) Distribution of the estimators for autoregressive time series with a unit root. Journal of the American Statistical Association 74, 427–431.
Eugenio FC, dos Santos AR, Fiedler NC, Ribeiro GA, da Silva AG, Juvanhol RS, Pedra BD (2016) GIS applied to location of fires detection towers in domain area of tropical forest. The Science of the Total Environment 562, 542–549.
| GIS applied to location of fires detection towers in domain area of tropical forest.Crossref | GoogleScholarGoogle Scholar | 27110968PubMed |
Farrar DE, Glauber RR (1967) Multicollinearity in regression analysis: the problem revisited. The Review of Economics and Statistics 49, 92–107.
| Multicollinearity in regression analysis: the problem revisited.Crossref | GoogleScholarGoogle Scholar |
Francos M, Úbeda X, Tort J, Panareda JM, Cerdà A (2016) The role of forest fire severity on vegetation recovery after 18 years. Implications for forest management of Quercus suber L. in Iberian Peninsula. Global and Planetary Change 145, 11–16.
| The role of forest fire severity on vegetation recovery after 18 years. Implications for forest management of Quercus suber L. in Iberian Peninsula.Crossref | GoogleScholarGoogle Scholar |
Gang C, Zhou W, Chen Y, Wang Z, Sun Z, Li J, Qi J, Odeh I (2014) Quantitative assessment of the contributions of climate change and human activities on global grassland degradation. Environmental Earth Sciences 72, 4273–4282.
| Quantitative assessment of the contributions of climate change and human activities on global grassland degradation.Crossref | GoogleScholarGoogle Scholar |
Garbolino E, Sanseverino-Godfrin V, Hinojos-Mendoza G (2016) Describing and predicting of the vegetation development of Corsica due to expected climate change and its impact on forest fire risk evolution. Safety Science 88, 180–186.
| Describing and predicting of the vegetation development of Corsica due to expected climate change and its impact on forest fire risk evolution.Crossref | GoogleScholarGoogle Scholar |
Gaudreau J, Perez L, Drapeau P (2016) BorealFireSim: a GIS-based cellular automata model of wildfires for the boreal forest of Quebec in a climate change paradigm. Ecological Informatics 32, 12–27.
| BorealFireSim: a GIS-based cellular automata model of wildfires for the boreal forest of Quebec in a climate change paradigm.Crossref | GoogleScholarGoogle Scholar |
Ge X, Dong K, Luloff AE, Wang L, Xiao J (2016) Impact of land use intensity on sandy desertification: an evidence from Horqin Sandy Land, China. Ecological Indicators 61, 346–358.
| Impact of land use intensity on sandy desertification: an evidence from Horqin Sandy Land, China.Crossref | GoogleScholarGoogle Scholar |
Goldberger AS (1991) ‘A course in econometrics.’ (Harvard University Press: Cambridge, MA, USA)
Gowlett JAJ (2016) The discovery of fire by humans: a long and convoluted process. Philosophical Transactions of the Royal Society B 371, 20150164
| The discovery of fire by humans: a long and convoluted process.Crossref | GoogleScholarGoogle Scholar |
Granger CWJ, Newbold P (1974) Spurious regressions in econometrics. Journal of Econometrics 2, 111–120.
| Spurious regressions in econometrics.Crossref | GoogleScholarGoogle Scholar |
Guo F, Zhang L, Jin S, Tigabu M, Su Z, Wang W (2016) Modeling anthropogenic fire occurrence in the boreal forest of China using logistic regression and random forests. Forests 7, 250
| Modeling anthropogenic fire occurrence in the boreal forest of China using logistic regression and random forests.Crossref | GoogleScholarGoogle Scholar |
Hannan EJ, Quinn BG (1978) The determination of the lag length of an autoregression. Journal of the Royal Statistical Society. Series A (General) 41, 190–195.
Hardy CC (2005) Wildland fire hazard and risk: problems, definitions, and context. Forest Ecology and Management 211, 73–82.
| Wildland fire hazard and risk: problems, definitions, and context.Crossref | GoogleScholarGoogle Scholar |
Harris R, Remenyi TA, Williamson GJ, Bindoff NL, Bowman DM (2016) Climate vegetation fire interactions and feedbacks: trivial detail or major barrier to projecting the future of the Earth system? Wiley Interdisciplinary Reviews: Climate Change 7, 910–931.
| Climate vegetation fire interactions and feedbacks: trivial detail or major barrier to projecting the future of the Earth system?Crossref | GoogleScholarGoogle Scholar |
Haug AA (2002) Temporal aggregation and the power of cointegration tests: a Monte Carlo study. Oxford Bulletin of Economics and Statistics 64, 399–412.
| Temporal aggregation and the power of cointegration tests: a Monte Carlo study.Crossref | GoogleScholarGoogle Scholar |
Holsinger Lisa, Parks Sean A, Miller Carol (2016) Weather, fuels, and topography impede wildland fire spread in western US landscapes. Forest ecology and management 380, 59–69.
| Weather, fuels, and topography impede wildland fire spread in western US landscapes.Crossref | GoogleScholarGoogle Scholar |
Ikemori F, Honjyo K, Yamagami M, Nakamura T (2015) Influence of contemporary carbon originating from the 2003 Siberian forest fire on organic carbon in PM2.5 in Nagoya, Japan. The Science of the Total Environment 530–531, 403–410.
| Influence of contemporary carbon originating from the 2003 Siberian forest fire on organic carbon in PM2.5 in Nagoya, Japan.Crossref | GoogleScholarGoogle Scholar | 26066482PubMed |
Kaloudis S, Tocatlidou A, Lorentzos NA, Sideridis AB, Karteris M (2005) Assessing wildfire destruction danger: a decision support system incorporating uncertainty. Ecological Modelling 181, 25–38.
| Assessing wildfire destruction danger: a decision support system incorporating uncertainty.Crossref | GoogleScholarGoogle Scholar |
Keeley JE, Syphard AD (2017) Different historical fire–climate patterns in California. International Journal of Wildland Fire 26, 253–268.
| Different historical fire–climate patterns in California.Crossref | GoogleScholarGoogle Scholar |
Kipling RP, Virkajärvi P, Breitsameter L, Curnel Y, De Swaef T, Gustavsson AM, Nendel C (2016) Key challenges and priorities for modelling European grasslands under climate change. The Science of the Total Environment 566–567, 851–864.
| Key challenges and priorities for modelling European grasslands under climate change.Crossref | GoogleScholarGoogle Scholar | 27259038PubMed |
Kobayashi H, Sugiura T, Uto M, Suzuki S (1994) International study on the pasture improvement in the Inner Mongolia desert-steppe, China. In ‘Proceedings of the international symposium on grassland resources’. (Ed. B Li) pp. 1007–1009. (Agricultural Sciencetech Press: Beijing, China).
Kukavskaya EA, Buryak LV, Shvetsov EG, Conard SG, Kalenskaya OP (2016) The impact of increasing fire frequency on forest transformations in southern Siberia. Forest Ecology and Management 382, 225–235.
| The impact of increasing fire frequency on forest transformations in southern Siberia.Crossref | GoogleScholarGoogle Scholar |
Li Y, Zhao J, Guo X, Zhang Z, Tan G, Yang J (2017) The influence of land use on the grassland fire occurrence in the north-eastern Inner Mongolia Autonomous Region, China. Sensors 17, 437
| The influence of land use on the grassland fire occurrence in the north-eastern Inner Mongolia Autonomous Region, China.Crossref | GoogleScholarGoogle Scholar |
Liu Z, Wimberly MC (2016) Direct and indirect effects of climate change on projected future fire regimes in the western United States. The Science of the Total Environment 542, 65–75.
| Direct and indirect effects of climate change on projected future fire regimes in the western United States.Crossref | GoogleScholarGoogle Scholar | 26519568PubMed |
Liu Z, Yang J, Chang Y, Weisberg PJ, He HS (2012) Spatial patterns and drivers of fire occurrence and its future trend under climate change in a boreal forest of north-east China. Global Change Biology 18, 2041–2056.
| Spatial patterns and drivers of fire occurrence and its future trend under climate change in a boreal forest of north-east China.Crossref | GoogleScholarGoogle Scholar |
Liu M, Zhao J, Guo X, Zhang Z, Tan G, Yang J (2017) Study on climate and grassland fire in HulunBuir, Inner Mongolia Autonomous Region, China. Sensors 17, 616
| Study on climate and grassland fire in HulunBuir, Inner Mongolia Autonomous Region, China.Crossref | GoogleScholarGoogle Scholar |
Mooney H, Larigauderie A, Cesario M, Elmquist T, Hoegh-Guldberg O, Lavorel S, Mace GM, Palmer M, Scholes R, Yahara T (2009) Biodiversity, climate change, and ecosystem services. Current Opinion in Environmental Sustainability 1, 46–54.
| Biodiversity, climate change, and ecosystem services.Crossref | GoogleScholarGoogle Scholar |
Moritz MA, Moody TJ, Krawchuk MA, Hughes M, Hall A (2010) Spatial variation in extreme winds predicts large wildfire locations in chaparral ecosystems. Geophysical Research Letters 37, L04801
| Spatial variation in extreme winds predicts large wildfire locations in chaparral ecosystems.Crossref | GoogleScholarGoogle Scholar |
Narayan PK (2005) The saving and investment nexus for China: evidence from cointegration tests. Applied Economics 37, 1979–1990.
| The saving and investment nexus for China: evidence from cointegration tests.Crossref | GoogleScholarGoogle Scholar |
Niu R, Zhai P (2012) Study on forest fire danger over northern China during the recent 50 years. Climatic Change 111, 723–736.
| Study on forest fire danger over northern China during the recent 50 years.Crossref | GoogleScholarGoogle Scholar |
Oteng-Abayie EF, Frimpong JM (2006) Bounds testing approach: an examination of foreign direct investment, trade, and growth relationships. American Journal of Applied Sciences 3, 2079–2085.
Pesaran B, Pesaran MH (2010) ‘Time series econometrics using Microfit 5.0: a user’s manual.’ (Oxford University Press, Inc.: Oxford, UK)
Pesaran MH (2015) ‘Time series and panel data econometrics.’ (Oxford University Press: Oxford, UK)
Pesaran MH, Shin Y, Smith RP (1999) Pooled mean group estimation of dynamic heterogeneous panels. Journal of the American Statistical Association 94, 621–634.
| Pooled mean group estimation of dynamic heterogeneous panels.Crossref | GoogleScholarGoogle Scholar |
Pesaran MH, Shin Y, Smith RJ (2001) Bounds testing approaches to the analysis of level relationships. Journal of Applied Econometrics 16, 289–326.
| Bounds testing approaches to the analysis of level relationships.Crossref | GoogleScholarGoogle Scholar |
Phillips PCB (1986) Understanding spurious regressions in econometrics. Journal of Econometrics 33, 311–340.
| Understanding spurious regressions in econometrics.Crossref | GoogleScholarGoogle Scholar |
Phillips PCB (1987) Time series regression with a unit root. Econometrica 55, 277–301.
| Time series regression with a unit root.Crossref | GoogleScholarGoogle Scholar |
Ramsey J (1969) Tests for specification errors in classical linear least-squares regression analysis. Journal of the Royal Statistical Society: Series B (Methodological) 31, 350–371.
| Tests for specification errors in classical linear least-squares regression analysis.Crossref | GoogleScholarGoogle Scholar |
Ravi S, Breshears DD, Huxman TE, D’Odorico P (2010) Land degradation in drylands: interactions among hydrologiceaeolian erosion and vegetation dynamics. Geomorphology 116, 236–245.
| Land degradation in drylands: interactions among hydrologiceaeolian erosion and vegetation dynamics.Crossref | GoogleScholarGoogle Scholar |
Rolinski T, Capps SB, Fovell RG, Cao Y, D’Agostino BJ, Vanderburg S (2016) The Santa Ana wildfire threat index: methodology and operational implementation. Weather and Forecasting 31, 1881–1897.
| The Santa Ana wildfire threat index: methodology and operational implementation.Crossref | GoogleScholarGoogle Scholar |
Schwarz G (1971) A sequential Student test. Annals of Mathematical Statistics 42, 1003–1009.
Semeraro T, Mastroleo G, Aretano R, Facchinetti G, Zurlini G, Petrosillo I (2016) GIS fuzzy expert system for the assessment of ecosystems vulnerability to fire in managing Mediterranean natural protected areas. Journal of Environmental Management 168, 94–103.
| GIS fuzzy expert system for the assessment of ecosystems vulnerability to fire in managing Mediterranean natural protected areas.Crossref | GoogleScholarGoogle Scholar | 26696610PubMed |
Su H, Liu GX (2004) Elementary analyses on the progress of grassland fire disaster information management technique. Grassland of China 26, 69–71.
Tian X, Sohngen B, Kim JB, Ohrel S, Cole J (2016) Global climate change impacts on forests and markets. Environmental Research Letters 11, 035011
| Global climate change impacts on forests and markets.Crossref | GoogleScholarGoogle Scholar |
Verón SR, Paruelo JM, Oesterheld M (2006) Assessing desertification. Journal of Arid Environments 66, 751–763.
| Assessing desertification.Crossref | GoogleScholarGoogle Scholar |
Wessels KJ, Prince SD, Malherbe J, Small J, Frost PE, VanZyl D (2007) Can human-induced land degradation be distinguished from the effects of rainfall variability? A case study in South Africa. Journal of Arid Environments 68, 271–297.
| Can human-induced land degradation be distinguished from the effects of rainfall variability? A case study in South Africa.Crossref | GoogleScholarGoogle Scholar |
Wessels KJ, Prince SD, Reshef I (2008) Mapping land degradation by comparison of vegetation production to spatially derived estimates of potential production. Journal of Arid Environments 72, 1940–1949.
| Mapping land degradation by comparison of vegetation production to spatially derived estimates of potential production.Crossref | GoogleScholarGoogle Scholar |
Westerling AL, Hidalgo HG, Cayan DR, Swetnam TW (2006) Warming and earlier spring increase western US forest wildfire activity. Science 313, 940–943.
| Warming and earlier spring increase western US forest wildfire activity.Crossref | GoogleScholarGoogle Scholar | 16825536PubMed |
Yang H, Zhao C, Liu Y (2008) GIS-based Inner Mongolia grassland fire spread simulation system. In ‘Computer science and software engineering, 2008 international conference on Computer Science and Software Engineering’, Wuhan, China. Vol. 3, pp. 923–925. (IEEE: Piscataway, NJ, USA)
Yang Y, Wang Z, Li J, Gang C, Zhang Y, Zhang Y, Qi J (2016) Comparative assessment of grassland degradation dynamics in response to climate variation and human activities in China, Mongolia, Pakistan and Uzbekistan from 2000 to 2013. Journal of Arid Environments 135, 164–172.
| Comparative assessment of grassland degradation dynamics in response to climate variation and human activities in China, Mongolia, Pakistan and Uzbekistan from 2000 to 2013.Crossref | GoogleScholarGoogle Scholar |
Yang X, Zhang K, Jia B, Ci L (2005) Desertification assessment in China: an overview. Journal of Arid Environments 63, 517–531.
| Desertification assessment in China: an overview.Crossref | GoogleScholarGoogle Scholar |
Yeganeh H, Khajedein SJ, Amiri F, Shariff ARBM (2014) Monitoring rangeland ground cover vegetation using multitemporal MODIS data. Arabian Journal of Geosciences 7, 287–298.
| Monitoring rangeland ground cover vegetation using multitemporal MODIS data.Crossref | GoogleScholarGoogle Scholar |
Zhang T, Zhang Y, Xu M, Xi Y, Zhu J, Zhang X, Sun X (2016) Ecosystem response more than climate variability drives the inter-annual variability of carbon fluxes in three Chinese grasslands. Agricultural and Forest Meteorology 225, 48–56.
| Ecosystem response more than climate variability drives the inter-annual variability of carbon fluxes in three Chinese grasslands.Crossref | GoogleScholarGoogle Scholar |
Zhang ZX, Zhang HY, Zhou DW (2010) Using GIS spatial analysis and logistic regression to predict the probabilities of human-caused grassland fires. Journal of Arid Environments 74, 386–393.
| Using GIS spatial analysis and logistic regression to predict the probabilities of human-caused grassland fires.Crossref | GoogleScholarGoogle Scholar |
Zheng YR, Xie ZX, Robert C, Jiang LH, Shimizu H (2006) Did climate drive ecosystem change and induce desertification in Otindag sandy land, China, over the past 40 years? Journal of Arid Environments 64, 523–541.
| Did climate drive ecosystem change and induce desertification in Otindag sandy land, China, over the past 40 years?Crossref | GoogleScholarGoogle Scholar |
Zhijun T, Jiquan Z, Xingpeng L (2009) GIS-based risk assessment of grassland fire disaster in western Jilin province, China. Stochastic Environmental Research and Risk Assessment 23, 463–471.
| GIS-based risk assessment of grassland fire disaster in western Jilin province, China.Crossref | GoogleScholarGoogle Scholar |