Simplifying emissions modelling from wildland fires: laboratory-scale emission factors are independent of fine woody debris fuel load
Élise-Andrée Guérette




A
B
C
D
E
F
Abstract
Air quality modelling of smoke from wildfires requires knowledge of emission factors and how these vary.
Experimental fires were used to test the variation of emission factors with fuel load to improve a smoke forecasting model.
Gas and particle-phase chemical composition of smoke plumes from laboratory-scale fires was measured with different fuel loads and at different stages of fire progression.
Different fine woody debris loads had no significant effect on the emission factors. In contrast, different stages of the fire produced significant differences in emission factors. The lowest emission factors for most species (except carbon dioxide) were observed during the flaming fire front, which accounted for half the total emissions. Importantly, we found that emission ratios relative to carbon monoxide were consistent across different fuel loads and throughout the fire’s progression.
By modelling the modified combustion efficiency, it is possible to simultaneously model the emissions of carbon monoxide and the emissions of nearly all other pollutants throughout the progression of a fire.
The emissions of carbon monoxide, fine particulate matter and other key pollutants all scale with the intensity of the fire, simplifying the task of modelling these emitted pollutants downwind of prescribed fires.
Keywords: Biomass Burning, Emission Factors, Emission Ratios, Experimental fires, Fine woody debris, Fire behaviour, Fire emissions, Laboratory-scale fires, Modified Combustion Efficiency, Pyrotron.
Introduction
Wildland (forest, bush or landscape vegetation) fires are a large and variable emission source of many gas and aerosol phase pollutants to the atmosphere, with major implications for local air quality and for atmospheric chemistry on a global scale (e.g. Andreae and Merlet 2001; Akagi et al. 2011; Rea et al. 2016). In Australia, mean annual gross emissions of carbon from landscape fires (127 Tg C year−1) have been estimated to be greater than the emissions from fossil fuels (95 Tg C year−1) (Haverd et al. 2013). It has been assumed that rapid regrowth ensures that net emissions from such fires are much lower (only 26 Tg C year−1) (Haverd et al. 2013). However, there is emerging evidence that climate change is altering fire regimes and decreasing inter-fire intervals below the recovery time of forests, leading to permanent carbon loss in some ecosystems (Boer et al. 2016; Byrne et al. 2021). Either way, the emissions from these fires represent a significant perturbation to atmospheric composition, especially just downwind of the location of the fires (e.g. Rea et al. 2016; Price et al. 2023). Accurate estimates of the emissions of different atmospheric pollutants are required to predict the resultant impact on air quality in the region of such fires.
Fuel load, combustion completeness, fuel elemental carbon and nitrogen content, and molecular emission factors are necessary in algorithms used to estimate the emissions from fires (e.g. Andreae and Merlet 2001; Storey and Price 2022). The simplest versions, such as IPCC (Intergovernmental Panel on Climate Change) Tier 1 (i.e. default) methodologies use aggregated parameter estimates (Change 2006). However, more nuanced methodologies such as IPCC Tier 2 (Change 2006) usually stratify the algorithms into relevant vegetation type (e.g. forest vs grassland), fire type (wild vs planned) and fuel type classes (fine, coarse, heavy) to better account for the differences observed in individual fire events (Volkova et al. 2019). This rapidly expands the task of experimentally evaluating the factors that impact all parameters in all strata of the models, and therefore simplifications are made about combustion properties assumed to be common between strata. It is wise, however, to confirm that such assumptions are valid. Fuel bed structure and depth, for example, appear to influence emission factors (Meyer and Cook 2015). The fuel element size is another factor that may also influence fire intensity (a measure of how much energy is released from the fire based on fire behaviour characteristics such as flame length and rate of spread) and, hence, the proportions of emissions of different gases may not be constant with different fuel loads (Urbanski 2013). The combustion and subsequent emissions from logs, for example, are distinctly different from the combustion rates and emissions from litter composed of leaves and fine twigs (Sullivan 2017a). Fine fuel, commonly termed litter, comprises leaves, bark and twigs less than 6 mm in diameter. Fine fuel tends to burn to completion once ignited, progressing through a sequence of pyrolysis, flaming combustion and smouldering combustion to extinction, by which time the fuel is mostly consumed (Sullivan 2017a). Combustion of fallen logs progresses differently, logs often smouldering and self-extinguishing long before they are completely consumed (Sullivan 2017b). Intermediate between these two classes are the fuels comprising branches larger than 6 mm and less than 50 mm, which we term here ‘fine woody debris’. It has been assumed that fuel elements up to ~50 mm in diameter will burn with emission rates and composition like that of the fine fuels (Cope et al. 2019). This assumption is untested and is the primary focus of the research that we report here.
The fact that emissions change with the stage of the fire has been described since Crutzen and Andreae (1990) distinguished between an initial flaming stage that produces more complete combustion (dominated by CO2), followed by a smouldering stage that produces less complete combustion (dominated by CO). Several studies have pointed out that this is a simplification: a high-intensity heading fire can produce thick dark smoke containing large amounts of partially oxidised gases (Cheney and Sullivan 2008), whereas glowing combustion in the latter stages can be highly efficient (Sullivan and Ball 2012). Looking at the physio-chemical properties of fire chemistry shows that flaming combustion consists of gas phase oxidation reactions, whereas solid phase oxidation reactions produces smouldering or glowing combustion (Sullivan 2017a).
The transfer of heat from burning material to adjacent fuel is the fundamental process by which a fire will spread and there are a large number of factors that control this process (Sullivan 2017b). The direction in which the fire propagates, with or against the wind, is also expected to affect the intensity of the fire, and thus combustion efficiency and emission factors (Wooster et al. 2011; Sullivan and Ball 2012). A fire that propagates with the wind (a heading fire) is more intense. That is why hazard reduction burns and back burns are often set up to spread against a low wind, so that the fire remains under control. A fire that propagates in the opposite direction to the wind is called a backing fire. Surawski et al. (2015) presented the effect of fire spreading mode on emission factors and found that heading fires consume more of the fuel (leaving less residue) and therefore emit more CO2 and CO than backing fires.
Recent advances in air quality forecasting models have included the coupling of fire spread models with air quality models so that the impact of smoke downwind of the fires may be better assessed (Cope et al. 2019). Therefore, it is now possible to incorporate knowledge of how emissions may change depending on different fire behaviour factors including: the type, amount and arrangement (e.g. at ground level vs elevated) of the fuel, the mode with which a fire propagates (heading, backing) and the stage of the fire (from the moving fire front to the smouldering remnants of the fire). Fires emit many different trace gases of interest including volatile organic pollutants that are involved in the chemical production of PM2.5 (particulate matter less than 2.5 µm in diameter) and ozone and other gases that are either toxic or act as greenhouse gases.
In this study, we report the results of laboratory-scale fires conducted in a combustion wind tunnel facility aimed at verifying that fuel elements up to ~50 mm in diameter (termed fine woody debris) burn with similar emission factors and smoke composition as that of fine fuels. We used a fixed amount of fine fuels (10 Mg ha−1) and varied the amount of fine woody debris (0, 2, 6 or 12 Mg ha−1) and the direction of fire propagation (with varying levels of replication). A subset of emitted pollutants was measured in the smoke with high temporal resolution, allowing assessment of how emission factors varied for different stages of the fire as well as what fraction of the total emissions occurred during different stages of combustion. This information was used to refine the smoke emission and transport model, known as AQFx, used for air quality modelling in Australia (Cope et al. 2019).
Materials and methods
The CSIRO Pyrotron facility
The CSIRO Pyrotron is a facility built for the study of the combustion and propagation of flames through naturally occurring bushfire fuels under a range of controlled fuel and environmental conditions (Sullivan et al. 2013). At the time of experimentation, it consisted of a purpose-built 25.6 m-long open-circuit fire-proof horizontal wind tunnel (see Fig. 1). The working section had a 2 × 2 m cross-section and was 4.8 m long, accommodating fuel beds up to 1.5 m wide and 4.0 m long. Glass windows allowed fire progression and behaviour to be observed. Air flow was created by a centrifugal fan, and wind speeds up to 5.5 m s−1 could be achieved. The fire was ignited along one of the edges of the fuel bed using a 1.5 m channel filled with ethanol, lit using a butane lighter. Varying fire spread modes (heading or backing) could be created by placing the ignition bar in different positions in the fuel bed relative to the airflow. The temperature, pressure and relative humidity inside the tunnel were logged using Vaisala devices. Wind speed and direction in two dimensions were also measured with a sonic anemometer.
Picture of the CSIRO Pyrotron Facility at the time of experimentation (Sullivan et al. 2013) and a close-up view of the working section during a burn.
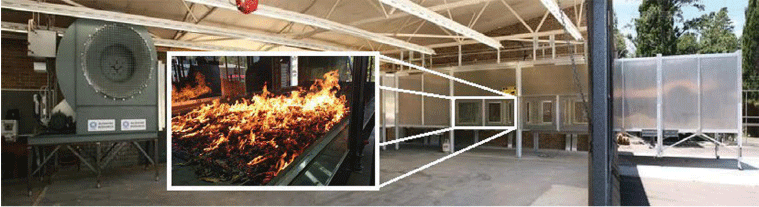
Smoke sampling inlets were located in the exit section, 2 m downwind from the end of the working section, at a height of 840 mm above the floor of the tunnel and positioned away from the walls. Smoke could also be characterised as it exited the tunnel (see Fig. 1). A detailed description of the CSIRO Pyrotron design and its thermofluid performance at the time of experimentation can be found in Sullivan et al. (2013), including an assessment of spatial uniformity across the entire cross-section.
Eucalypt litter fires in the CSIRO Pyrotron
Fuels were collected from 28 to 30 January 2014 in the Wombat State Forest, near Daylesford, Victoria, in areas earmarked for prescribed fires later in the year (Cope et al. 2019). The dominant overstorey species were Eucalyptus obliqua (L’Hér.), Eucalyptus dives (Schauer) and Eucalyptus radiata (Sieber ex DC.) (Sullivan et al. 2018). Fallen litter composed of dried leaves, bark, twigs and small branches was collected from the forest floor and sorted into categories: fine fuels, comprising senescent leaves, bark and twigs less than 6 mm in diameter, sieved to remove fragmented and inorganic material; and two classes of coarser fuels (fine woody debris): sticks larger than 6 mm in diameter but smaller than 25 mm, and logs larger than 25 mm in diameter but smaller than 50 mm. The fuels were stored and left to dry to the typical level of fuel moisture required for hazard reduction burns (i.e. ~12%; Sullivan et al. 2012). Samples of the fuel were taken and weighed (~100 g), dried for 24 h at 105°C and then re-weighed to determine the moisture content. The carbon and nitrogen contents of the different fuel elements (leaves, bark, twigs, sticks and logs) were determined using isotope ratio mass spectrometry (Muccio and Jackson 2009).
Four different fuel loads on a dry matter basis were chosen for the experiment with a common fine fuel load (10 Mg ha−1) and an increasing load of fine woody debris (see Table 1). Moisture content of the fuel was accounted for at the time of fuel bed preparation.
Load | Fine fuel loading (Mg ha−1) | Fine woody debris loading (Mg ha−1) | Total biomass – heading/backing fires (kg) | No. of replicates – heading/backing fires | Carbon content (Fc) (%) | Nitrogen content (%) | N/C ratio | |
---|---|---|---|---|---|---|---|---|
Load 1 | 10 | 0 | 6.0/3.0 | 3/1 | 50.4 | 0.37 | 0.007 | |
Load 2 | 10 | 2 | 7.2/3.6 | 5/1 | 50.2 | 0.33 | 0.007 | |
Load 3 | 10 | 6 | 9.6/4.8 | 5/1 | 49.9 | 0.29 | 0.006 | |
Load 4 | 10 | 12 | 13.2/6.6 | 5/1 | 49.6 | 0.25 | 0.005 |
A burn plan was prepared that ensured the scheduled backing and heading fires, with different loads, were spread over the 2 weeks of measurements, and approximately evenly spread across different times of day, in order to avoid any unintended systematic influence on the results (such as diurnal changes in atmospheric humidity). All fuel beds were prepared in a similar way: the fine fuels (10 Mg ha−1) were arranged evenly on the floor of the working section (depth: 12–39 mm, median 21 mm) and the fine woody debris was then laid on top using a randomised location across the fuel bed and a randomised orientation at the location (see Sullivan et al. 2018). The fuel bed was laid over an area 1.5 m width by 4 m length for the heading fires, and 1.5 m by 2 m for the backing fires. This decision was made to reduce the time taken for the backing fires and allow a more complete sampling of the emissions from the fires by the various instrumental techniques. This also meant half the quantity of fuel was used for backing fires.
A total of 18 heading fires (flames propagating with the wind) and 4 backing fires (flames propagating against the wind) were conducted during February and March 2014. The wind speed was set at 1.0 m s−1 for all fires. The moisture content of fine fuels was determined prior to each fire as explained in Surawski et al. (2015), and that of fine woody debris every few days (Sullivan et al. 2018). Moisture by weight varied between 10.0 and 12.7% in the fine fuels and from 9.2 to 11.6% in the fine woody debris. Analysis of the carbon and nitrogen content of fine fuels and fine woody debris was performed by a stable isotope mass spectrometric technique (with an analytical uncertainty of ±2%) to look for any differences between the fuel loads (see Sullivan et al. 2018 for details). Minimal differences were seen in the carbon content, but the woody debris contained less nitrogen than the fine fuels, so as more woody debris was added to the fuel mixture, the overall nitrogen content of the fuel decreased (see Table 1).
Fire observations (ignition time, rate of spread, flame characteristics, end of flame spread, extinction of fine fuels and extinction of fine woody debris) were recorded for each fire (Sullivan et al. 2018).
Atmospheric sampling techniques during the fires
A Fourier-transform infrared (FTIR) spectrometer (Bomen MB-100 series, with a resolution of 1 cm−1) was operated in open-path mode using an external beam splitter and mirrors to direct the modulated infrared beam into a 305 mm (12″) telescope (Meade LX300). The infrared beam was reflected to the telescope by an array of gold-plated corner cubes and directed to a liquid nitrogen-cooled mercury cadmium telluride detector. The experimental set-up is described in detail in Paton-Walsh et al. (2014). The spectrometer and telescope were placed on top of a tripod, itself placed on a trolley (for ease of positioning). The array of reflectors was placed on top of a 2.5 m high step ladder. The beam crossed the section outside the tunnel with an angle of approximately 30°, allowing sampling of most of the vertical profile of the exit of the wind tunnel (see Fig. 2). Three scans were co-added, producing one spectrum approximately every 20 s. Fires took between 10 and 30 min from ignition to extinction, depending on the fuel load and the direction of fire spread. This meant between 30 and 90 spectra were obtained for each fire.
Configuration of open-path FTIR system at the CSIRO Pyrotron showing line of sight diagonally across the open end of the tunnel shown as the dotted red line. Typical path length was ~10 m. The sampling line for the VOC samples is shown as the solid red line and is the rightmost sampling line visible in the zoomed-in photograph on the right. The leftmost sampling line with a filter holder on top is the bypass line from which the integrated-cavity-output spectroscopy (ICOS) greenhouse gas analysers were sampling, and in the middle is the sampling line for the DustTrak PM2.5 measurements.
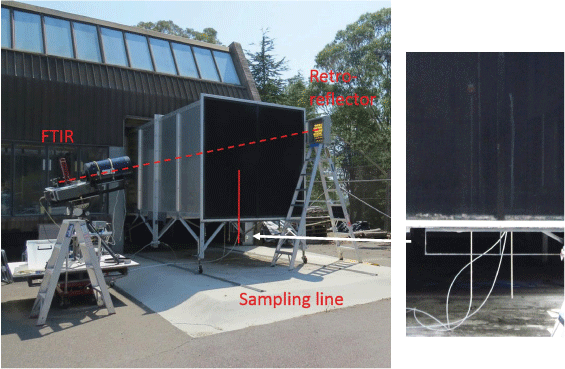
A least-square fitting of observed to calculated spectra was used to analyse the data (Griffith 1996; Griffith et al. 2012), using the spectral windows described in Paton-Walsh et al. (2014) to derive concentrations of carbon dioxide (CO2), carbon monoxide (CO), formaldehyde (CH2O), ammonia (NH3), acetylene (C2H4), methanol (CH3OH), formic acid (HCOOH) and acetic acid (CH3COOH) in the smoke plume every 20 s throughout the fire. The availability of these high-temporal-resolution measurements allows the determination of changes in emission factors during different stages of the fire. The open-path FTIR also measured methane (CH4) and nitrous oxide (N2O) but these two gases are measured with lower uncertainties by the integrated cavity output spectrometers (ICOS) described below.
Two off-axis integrated-cavity-output spectroscopy (off-axis ICOS) instruments from Los Gatos Research were used to sample CO2 and CH4 (Greenhouse Gas Analyser GGA-24r-EP) and CO and N2O (N2O/CO Analyser 907-0015). A dilution with zero air is necessary to avoid difficulties with the N2O retrieval due to the broadening of the CO spectral line when the CO mixing ratio exceeds 10 ppm (Surawski et al. 2015). One or several dilution ratios (ranging from 4 to 15) were used on the inlet sample line serving both instruments for each fire. The instruments were sampling from a bypass line, which itself was sampling at a rate of 4 L min−1 from a sampling tube placed in the exit section of the Pyrotron, centred on a cross-section 1 m from the end of the wind tunnel.
Measurements of particulate matter less than 2.5 µm in diameter (PM2.5) were made with a DustTrak™ II Aerosol Monitor (Model 8530, TSI Inc.) operated with a PM2.5 impactor and averaged to 1-min resolution. The DustTrak uses a light-scattering laser photometer to measure particulate concentrations up to 400 mg m−3. Air was drawn into the sensing chamber continuously using a diaphragm pump at a flow rate of 3.0 L min−1. Zero checks using the provided zero filter and flow checks of the DustTrak were performed before and after each burn. The continuous PM2.5 measurements were calibrated using gravimetric total particulate matter concentrations.
Pre-weighed 47-mm Fluoropore membrane filters (1-µm pore size, Merck Millipore) and 47-mm quartz filters (Pallflex, Pall Life Sciences) were used to collect fire-integrated total particulate matter for later analysis of particulate phase gravimetric analysis and chemical composition, allowing assessment of whether the fraction of fine fuel and fine woody debris impacted emission factors. The filter measurements were made from filters placed in a holder on top of the same sampling line that was used for the ICOS instruments (Fig. 2).
Samples of the smoke were collected in 5 or 10 L Tedlar bags through Teflon sample lines positioned 840 mm above the wind tunnel floor in the exit section (see Fig. 2). Bags were filled for 1–4 min using differential pressure samplers, from the moment of ignition to the end of the fire, so that all stages of the fires were sampled. Each sampler was equipped with its own battery-operated pump (SKC, Universal XR Sample pump). Bags were filled for 1 min through the early stages of the fires, to capture the rapidly evolving emission profile, and then for 4 min. Typically, only one bag was filled at a time, although for some fires, overlapping samples were collected. Between 6 and 12 Tedlar bag samples were collected during each fire.
Off-line analysis of Tedlar bag samples
The Tedlar bag samples were first analysed on site for CO, CO2, CH4, C2H4 and C2H6 using a Fourier-transform spectrometer coupled with a White cell and then for mercury on a Tekran 2537A analyser (Howard et al. 2019). The bags were then brought to the University of Wollongong to be analysed for a suite of volatile organic compounds (VOCs) using selective ion flow tube mass spectrometry (SIFT-MS). All samples were analysed for VOCs within 48 h of collection, with tests showing losses were minimal (Guerette 2016). The analysis of mercury in the Tedlar bag samples was described in Howard et al. (2019).
A Bomem MB-100 Series FTIR spectrometer (1 cm−1 resolution) coupled to a multi-pass optical (White) cell with a path length of 22.2 m and fitted with an indium antimony (InSb) detector cooled with liquid nitrogen was used to measure CO2, CO, CH4, C2H6 and C2H4 in the Tedlar bag samples of smoke collected during the fires. Part of the sample was transferred to the evacuated White cell and the temperature and pressure inside the cell were logged. Typical temperature and pressure inside the White cell were 22°C and 220 hPa, respectively. A spectrum consisting of 26 scans was acquired for each Tedlar bag sample. Mole fractions of the gases of interest were retrieved using MALT software (Griffith 1996; Griffith et al. 2012) in a similar manner to the analysis for the open-path FTIR spectra. A full description of the measurement method is given in Guerette (2016).
Mole fractions of VOCs in the Tedlar bag samples were measured using a Syft Voice100 SIFT-MS (Milligan et al. 2007) via chemical ionisation by reagent ions (H3O+, NO+, O2+). Typical pressure and temperature inside the flow tube were 67 Pa and 25°C, respectively. The measurement cycle included 34 masses, 28 of which were related to VOCs (the others were for reagent ion monitoring). The measurement cycle length was approximately 90 s, and the dwell time was 200 ms for mass-to-charge ratios related to reagent ions and between 2000 and 8000 ms for VOC-related mass-to-charge ratios. The cycle was repeated five times on each sample. VOC mixing ratios were determined from the product ion counts using normalised calibration factors determined empirically (Guerette 2016).
Gravimetric analysis of the 47-mm Fluoropore filters was performed using a Mettler UMT2 ultramicrobalance with a specialty filter pan in a temperature- and humidity-controlled environment. The 47-mm Fluoropore filters were analysed for major water-soluble ions by suppressed ion chromatography (IC) and for anhydrous sugars including levoglucosan by high-performance anion-exchange chromatography with pulsed amperometric detection (HPAEC-PAD). Carbon analysis was performed on the sampled 47-mm quartz filters using a DRI Model 2001A thermal-optical carbon analyser using the IMPROVE-A temperature protocol (Cheng et al. 2014). Elemental concentrations in collected filter samples were determined using X-ray fluorescence spectroscopy (XRF). Black carbon was measured by light reflectance using the M43D digital smoke stain reflectometer.
Calculating emission factors, emission ratios and modified combustion efficiency
Emission factors (EFs) for biomass burning have been reported in two distinct forms throughout the relevant literature: EFs per unit of dry fuel consumed and emissions per unit of a specific burnt element emitted (usually carbon or nitrogen). In this paper, we report EFs as emissions per unit of dry fuel consumed.
Emission factor per unit of dry fuel consumed
The EF per unit of dry fuel consumed approach is discussed by Yokelson et al. (1999) and Paton-Walsh et al. (2014), and for carbon-containing species follows Eqn 1:
where EFi is the mass in grams of species i emitted per kilogram of dry fuel burned (g kg−1); Fc is the fractional carbon content of the fuel; MMi is the molar mass of species i and 12 is the atomic mass of carbon; and Ci/CT is the number of moles of species i emitted divided by the total number of moles of carbon emitted, and can be calculated directly from excess mole fractions measured according to Eqn 2:
where Δ[i] and Δ[j] are the excess mole fractions of species i and j respectively (i.e. Δ[i] = iplume− ibkgnd) where iplume represents concentrations measured in the smoke and ibkgnd is the mean background mole fraction measured before the fire, nCj is the number of carbon atoms in compound j and the sum is of all carbon-containing species emitted by the fire.
In this work, those carbon-containing species detectable by open path FTIR (CO2, CO, CH4, C2H4, CH2O, CH3OH, HCOOH and CH3COOH) have been included in the EF calculations. Although these do not represent all of the carbon-containing species emitted by a fire, CO2, CO and CH4 account for the vast majority of carbon emissions (>98%) (Andreae and Merlet 2001; Akagi et al. 2011; Andreae 2019). For consistency with other EFs in the literature, the carbon that is burnt but not emitted to the atmosphere (i.e. that is left in the ash residue) is not included in the equations (Surawski et al. 2016). Thus, the EFs are in grams per kilogram of dry fuel consumed and not grams per kilogram of dry fuel burnt.
EFi may also be calculated using emission ratios with respect to a reference species (usually CO2 or CO) using Eqns 3 and 4:
where ERi/y is the emission ratio of species i to reference species y, given by:
where Δ[i] is the excess mole fraction of species i above the background mole fraction. Deriving the emission ratios via the gradient of the best linear fit to a plot of the abundance of species i against the abundance of reference species y removes the requirement for accurate knowledge of the background mole fractions, yet introduces an insignificant degree of error (Wooster et al. 2011).
EFCO2 and EFCO were calculated using Eqn 1, whereas all other EFs were derived using Eqn 3 and the emission ratios with respect to CO.
Modified combustion efficiency
The combustion efficiency is defined as the ratio between the amount of carbon emitted as CO2 and the total amount of carbon emitted. As CO2 and CO represent together ~98% of all carbon emitted, the ratio of CO2 over the sum of CO2 and CO has been generalised as the value reported in the literature (Yokelson et al. 1999; Meyer et al. 2012) and is known as the modified combustion efficiency (MCE):
When the fire is dominated by flaming combustion, the combustion efficiency is high. It decreases as smouldering combustion becomes more dominant.
Combining multiple measurements of different compounds to derive emission factors for the fires
There were three separate sampling configurations used to measure the emissions from these experimental fires:
Open-path configuration (FTIR spectrometer only),
Tedlar bag sample port, used for filling sample bags,
Sampling ports for the continuous ICOS measurements and fire-integrated filters for particle emissions, and for the DustTrak measurements of PM2.5.
Each of these sampling configurations included measurements of CO2 and CO, with some other gases also measured by two separate techniques. The open-path configuration provided the best representative sampling of the whole smoke plume and hence EFs for each fire for CO2 and CO were calculated from the open-path FTIR using Eqn 1. The calculation of emission ratios to CO for each compound used the CO measurements from the relevant sampling configuration (open-path, continuous sampling or Tedlar bag samples) using Eqn 4. EFs were then calculated using Eqn 3 with these emission ratios to CO and the EFCO calculated for the fire from the open-path FTIR.
Several VOCs were measured by multiple methods and in all cases, the measurements agreed within the uncertainties of the measurements (Guerette 2016). The results presented here are from the open-path FTIR, which offered the lowest uncertainties. Similarly, continuous measurements of N2O and CH4 from the ICOS are used here in preference to the open-path FTIR measurements owing to the lower uncertainties. Further details of the methods and results can be found in Guerette (2016) and Desservettaz (2018).
Results and discussion
Variation of emission factors of CO2 and CO against the fraction of fine woody debris fuels in the load
Fig. 3 shows the EFs for CO2 and CO along with MCE for all the heading and backing fires with different fuel loads. The corresponding data along with the fuel moisture from each individual fire are provided in the supplementary section in Supplementary Table S1. The results show no clear trend in the EFs for CO and CO2 and hence no trend in MCE for different amounts of fine woody debris in the load for the heading fires. To confirm this finding, P-values were calculated to determine if there was any dependence on the number of megagrams per hectare of fine woody debris in the fuel load. Values of P = 0.16, 0.41 and 0.21 were found for the dependence of the EFs of CO2, CO and the MCE respectively. Hence, as all results return a P-value above 0.05 (the commonly accepted threshold for significance) (Wasserstein and Lazar 2016), we conclude that there is no dependence on the fine woody debris fuel load.
Emission factors for CO2 and CO, and modified combustion efficiency (MCE) for each of the experimental fires (blue dots), and mean values and s.d. (black dots and error bars) for each load of fine woody debris for the heading fires and for all the backing fires, irrespective of load, together.
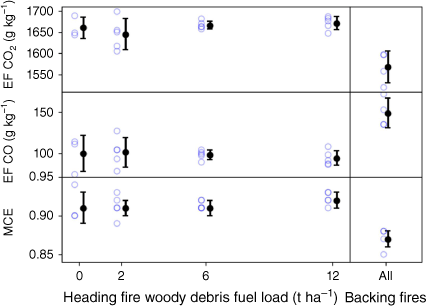
There is only a single backing fire for each fuel load; however, there is also no apparent trend in EFs of CO2 and CO or MCE for different loads within these backing fires (P = 0.94, 0.95 and 0.96 respectively). Elsewhere in this study, we combined these backing fires into a single group and considered the results from these fires separately from the other (heading) fires.
Considered together, the backing fires show on average an EF for CO that is 50% higher than for the heading fires, with a correspondingly lower EF for CO2 and therefore a lower MCE. This result supports similar findings in the literature (e.g. Wooster et al. 2011). This implies that the direction of fire spread should be accounted for in modelling emissions in the fire model but that the EFs for CO and CO2 do not need to be adjusted for the fraction of fine woody debris in the fuels burning.
Variation of emission ratios to CO with the fraction of fine woody debris fuels in the load
Fig. 4 shows emission ratios relative to CO for PM2.5 and a selection of the emitted trace gases measured plotted against the amount of fine woody debris in the fuel load, along with the square of the correlation coefficient (R2) and the P-value for dependence on fuel load. Similar emission ratios with respect to CO for particulate phase compounds measured on the filters are shown in Fig. 5. Data for all measured chemical species are provided in Supplementary Table S2.
Emission ratios with respect to CO for a selection of trace gases measured by open-path FTIR spectrometer and PM2.5 measured by DustTrak versus fine woody debris load. The amount of fine fuel was kept constant at 10 Mg ha−1. None show a significant trend with the amount of fine woody debris in the fuel load.
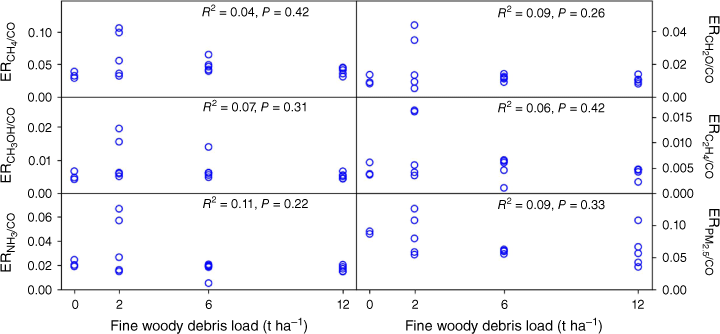
Emission ratios with respect to CO for a selection of particulate phase chemical species measured from the filters versus fine woody debris load, noting that the amount of fine fuel was kept constant at 10 Mg ha−1 (OC, organic carbon; EC, elemental carbon; SO4, sulfates; NO3, nitrates; BC, black carbon; NH4, ammonium). None show a significant trend with the amount of fine woody debris in the fuel load.
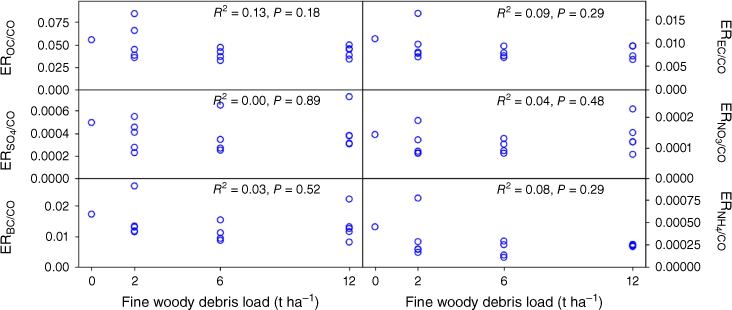
The results show that none of the measured compounds show a significant trend in emission ratio with respect to CO with the amount of fine woody debris in the litter (all P ≥ 0.05). One exception that we measured in this experiment is mercury that is volatilised with the first exposure to heat, which is reported elsewhere (Howard et al. 2019). Thus, we can conclude that we do not need to include different EFs for different fractions of fine woody debris in the fuel mix. Although this simplifies the emissions modelling, the presence of fine woody debris impacts the rate of fire spread and so is still important in fire dynamics (Sullivan et al. 2018).
Variation of emission factors of CO2, CO, CH4 and N2O with fire stage
To model the atmospheric impacts of fires with high temporal resolution, we need to be able to model how the emissions change as the fire progresses. Notes were made for each fire of the time of ignition, the time when the flame spread first reached the end of the fuel bed (end of flame spread), the time of extinction of the fine fuels and the time of extinction of the fine woody debris (see Fig. 6). We used these observations to define three different stages of the fire:
The flaming fire front stage is the period between ignition and the end of flame spread. This stage is dominated by flaming although there is some smouldering behind the flaming fire front.
The residual flaming stage is the period between the end of the fire spread and the extinction of the fine fuels (a mix of flaming and smouldering).
The smouldering stage of the fire is the period after the extinction of the fine fuels (a mix of smouldering and flaming with smouldering dominating).
Time series of the changing concentration of CO2 measured by the open-path FTIR spectrometer as the fire progresses during an example burn (first Load 2 replicate). The time of ignition, time that the fire spread finished and time that the fine fuels were extinguished are shown as vertical dashed lines, along with the definitions of the fire stages (a separate version of this figure that also shows MCE is provided as Supplementary Fig. S1).
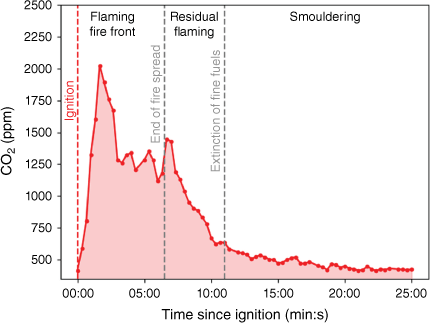
In this definition of the smouldering stage, we do see the occasional flame on some of the fine woody debris, but the predominant mode is smouldering. We created this definition because it was not unusual for some of the woody debris to appear to be extinguished but then to subsequently reignite with visible flames. The very last stages of residual smouldering were not well captured in the laboratory fires and the emissions were insufficient to be measured well against background levels in the open-path format. Thus, it is likely that we underestimate the contribution of smouldering to the total emissions compared with a real-world fire.
It has been established previously that the presence of woody debris changes the dynamics of fire spread, slowing the fire and thereby increasing the length of time taken by each of our three fire stages (Sullivan et al. 2018). Here, we analyse our measurements with 20-s temporal resolution from the open-path FTIR to see how the EFs and the emission ratios change during these different fire stages.
Fig. 7 shows the time series of measured concentrations of CO and greenhouse gases from an example heading fire with each fuel load. From these time series for CO2, CO and CH4, it is possible to subtract background amounts and hence calculate a total cumulative emission of carbon (as shown in the bottom panel of Fig. 7). Using the observed times for the stages of the fire enabled us to calculate the fraction of fuel consumed in each stage. Mean results for all fires showed that approximately 50 ± 10% of fuel was consumed during the flaming fire front stage; 40 ± 10% was consumed during the residual flaming stage and only 13 ± 5% was consumed during the smouldering stage. The bulk of emissions occur in the first two stages of the fire; however, the fraction of emissions occurring in the smouldering stage is likely to be higher in real fires (as described above) and these emissions are more likely to be caught in the nocturnal boundary layer and so are still important for air quality forecasting.
Time series of trace gases and cumulative fraction of carbon emitted during selected burns for Load 1 (fine fuel only, 10 Mg ha−1) through Load 4 (10 Mg ha−1 fine fuel + 12 Mg ha−1 fine woody debris).
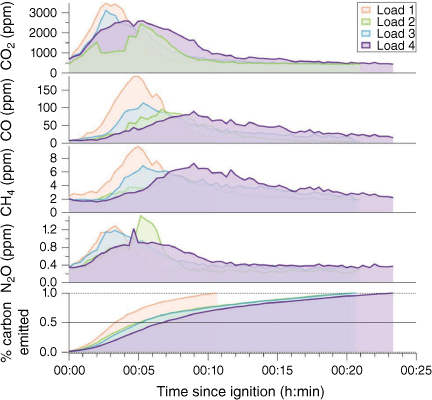
From Fig. 7, it is also clear that the emissions of CO2 peak earlier in the fire than the emissions of CO and CH4. The emission factors of CO and CO2 vary greatly with the fire stage, as can be seen in Table 2. This translates into significant differences in the MCE for each fire stage (Table 2 and Fig. 8). The EFs for CH4 change in a similar pattern to those of CO, whereas the EF for N2O appears relatively constant throughout the fire.
Emission factors (g kg−1 dry fuel) | ||||
---|---|---|---|---|
Flaming fire front | Residual flaming | Smouldering | ||
CO2 | 1790 ± 20 | 1690 ± 50 | 1470 ± 90 | |
CO | 20 ± 10 | 80 ± 30 | 190 ± 40 | |
MCE | 0.98 ± 0.01 | 0.93 ± 0.03 | 0.82 ± 0.04 | |
CH4 | 0.5 ± 0.3 | 2.6 ± 0.7 | 7.3 ± 1.7 | |
N2O | 0.06 ± 0.02 | 0.07 ± 0.03 | 0.06 ± 0.02 | |
Fraction of total carbon emitted | 0.5 ± 0.1 | 0.4 ± 0.1 | 0.13 ± 0.05 |
Plot showing the distribution of modified combustion efficiency (MCE) for each fire stage observed in eucalypt fires at the CSIRO Pyrotron.
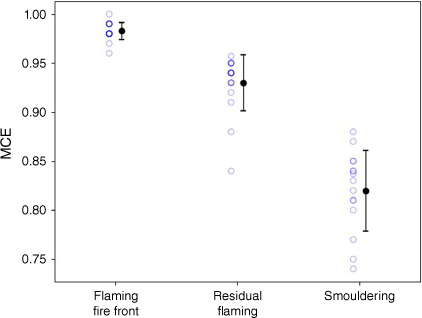
Also apparent from Fig. 7 is that the fire spread progresses rapidly when only fine fuel is present (Load 1). The presence of fine woody debris slows the rate of forward spread, with a clear difference in speed of fire progression between fine fuels only and all fires with some fine woody debris (regardless of the fraction of woody debris in the fuel load), as reported in Sullivan et al. (2018).
Variation of emission ratios of VOCs, ammonia and PM2.5 with fire stage
As well as CO2, CO, CH4 and N2O, the open-path FTIR also measured ethene (C2H4), ammonia (NH3), methanol (CH3OH), formaldehyde (CH2O), formic acid (HCOOH) and acetic acid (CH3COOH) with 20-s temporal resolution, so we can assess how emission ratios to CO change with fire stages for these gases. Fig. 9 shows the emission ratios with respect to CO for each of these gases and for PM2.5 and CH4 for each stage of each of the heading fires (with data from one burn removed as an outlier). The mean and 1σ standard deviations are also shown and these data are provided in the supplementary material in Supplementary Tables S4 and S5 (with and without one outlier removed). The emission ratios from the residual flaming stage show the highest variability, with the smouldering stage showing the least variability. Despite this variability, there is sparse evidence of differences in emission ratios to CO for the different stages of the fire, with all mean values overlapping when all burns are included (see Supplementary Table S4). When the outlier is excluded, there are small differences (within 1σ but not within 2σ) for the emission ratio to CO for methane between the flaming fire front and smouldering and for ethene between residual flaming and smouldering (see Supplementary Table S5). Overall, we conclude that there is insufficient evidence of changes in the emission ratios between fire stages to overturn our original hypothesis that fine woody debris will burn with emission rates and composition similar to that of fine fuels or to justify adding additional complexity to the modelling of fires within the Australian air quality forecasting model (AQFx) (Cope et al. 2019).
Emission ratios (ERs) relative to carbon monoxide of methane (CH4), ethene (C2H4), ammonia (NH3), methanol (CH3OH), formaldehyde (CH2O), formic acid (HCOOH), acetic acid (CH3COOH) as measured by the open-path FTIR system, and PM2.5 as measured by DustTrak, plotted against fire stage.
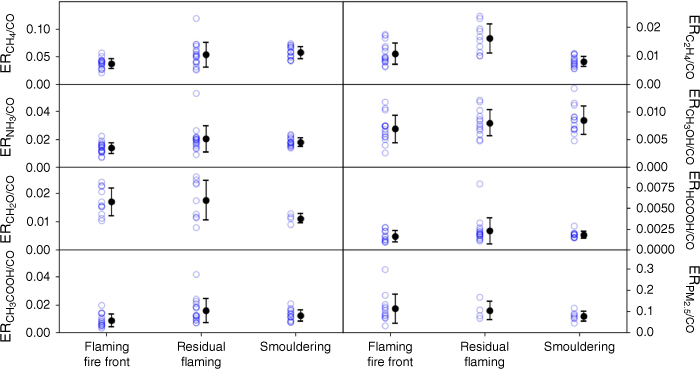
As the emission ratios relative to CO do not vary substantially with fire stage, the EFs for these gases will vary along with the emissions of CO and with the MCE. Thus, if the rate of fire spread is modelled with MCE, then the rate of the emissions can be mapped in terms of CO emissions, with PM2.5 and reactive gases mirroring the emission profile of CO with time.
The VOC species measured by SIFT-MS were limited to the time resolution of the Tedlar bag samples and therefore less data were available to test any dependence on fire stages. Likewise, the particle phase chemical measurements were limited to a single fire-integrated measurement. Nevertheless, given the strong correlation with CO in the whole-fire data, we assume for modelling purposes that these gases will also track with the EF of CO.
The use of experimental fires allows us to test the effect of different variables in a controlled manner, but the experiment may not perfectly replicate conditions in a real forest fire. For this reason, we provide the EFs for the measured chemical components of smoke only in the supplementary material (see Supplementary Table S6) and we recommend that fire models utilise EFs from field measurements of temperate Australian forest fires where available (e.g. Smith et al. 2014; Volkova et al. 2014; Desservettaz et al. 2017; Guérette et al. 2018; Reisen et al. 2018).
Conclusions
We measured the chemical composition of gas and particulate phase emissions from a series of experimental fires with the aim of testing the hypothesis that fine woody debris (between 6 and 50 mm in diameter) burns with similar emission factors and smoke composition to that of fine fuels (<6 mm in diameter). Our results confirmed our hypothesis, with no statistically significant trends in the emission factors of CO2 and CO with different fractions of fine woody debris added to fine fuels. As the emission ratios relative to CO of a large range of chemical species were also insensitive to the fraction of fine woody debris in the fuel, we conclude that the emission factors do not vary with the fraction of fine woody debris in the fuel.
In contrast, the emission factors for CO and CO2 varied significantly between the three defined fire stages, the flaming fire front, residual flaming and smouldering stages, and so each stage had a distinct MCE. For PM2.5 and all the trace gases that were measured with high temporal resolution, the emission ratios to CO did not vary substantially with fire stage. Thus, the emission factors of PM2.5 and trace gases vary with modified combustion efficiency and burn stage, mirroring the emissions of CO, with larger emission factors in the later stages of the fire. When modelling fire emissions, the presence of fine woody debris in the fuel will slow the fire spread (and hence the rate of emissions of CO2 and CO), although the impact appears independent of the quantity of fine woody debris, as reported by Sullivan et al. (2018). However, the emissions of PM2.5, volatile organic compounds and other reactive gases will simply scale with CO emissions, which greatly simplifies the modelling task. Overall, approximately half of the total emissions occurred during the flaming fire front, with the majority of the rest emitted in the residual flaming stage and only 10% during the smouldering combustion stage.
Thus, the lowest emission factors for CO, PM2.5 and VOCs occur during the flaming fire front stage, which consumes approximately half of the fuel in an intense flaming combustion. Emissions from the flaming parts of the fire are likely to be entrained by the convective column created by the fire front and lofted into the atmosphere. These plumes can be transported significant distances and descend with the planetary boundary layer at night, impacting air quality downwind of the fires. The emissions factors during the residual flaming and smouldering stages are higher but fuel consumption is lower. These emissions are less likely to be lofted by convection and thus more likely to impact air quality closer to the fires.
Data availability
The data used in this study are available at the CSIRO data portal: https://data.csiro.au/collection/csiro:62972
Conflicts of interest
Andrew Sullivan is an Associate Editor of International Journal of Wildland Fire. To mitigate this potential conflict of interest, he did not have editor-level access to this paper during the peer review process. Authors declare that they have no other conflicts of interest.
Declaration of funding
The original experiments described in this paper were supported by the Victorian Department of Environment, Land, Water and Planning. The later analysis and write-up were supported by the New South Wales Government and delivered in partnership with NSW Department of Climate Change, Energy, the Environment and Water, within the Bushfire and Natural Hazards Research Centre via the project known as RUSH (Research for Understanding Smoke Hazards).
Acknowledgements
The authors would like to thank Martin Cope for his leadership of the Smoke and Transport Modelling project and Dean Howard, Grant Edwards and Stephen Wilson for their assistance during the experimental laboratory fires. We acknowledge the Ngunnawal and Ngambri peoples, who are the traditional custodians and knowledge holders of the Country on which we conducted this research.
References
Akagi SK, Yokelson RJ, Wiedinmyer C, Alvarado MJ, Reid JS, Karl T, Crounse JD, Wennberg PO (2011) Emission factors for open and domestic biomass burning for use in atmospheric models. Atmospheric Chemistry and Physics 11(9), 4039-4072.
| Crossref | Google Scholar |
Andreae MO (2019) Emission of trace gases and aerosols from biomass burning – an updated assessment. Atmospheric Chemistry and Physics 19(13), 8523-8546.
| Crossref | Google Scholar |
Andreae MO, Merlet P (2001) Emission of trace gases and aerosols from biomass burning. Global Biogeochemical Cycles 15(4), 955-966.
| Crossref | Google Scholar |
Boer MM, Bowman DM, Murphy BP, Cary GJ, Cochrane MA, Fensham RJ, Krawchuk MA, Price OF, De Dios VR, Williams RJ (2016) Future changes in climatic water balance determine potential for transformational shifts in Australian fire regimes. Environmental Research Letters 11(6), 065002.
| Crossref | Google Scholar |
Byrne B, et al. (2021) The carbon cycle of southeast Australia during 2019–2020: drought, fires, and subsequent recovery. AGU Advances 2(4), e2021AV000469.
| Crossref | Google Scholar |
Cheng Y, He K-b, Duan F-k, Du Z-y, Zheng M, Ma Y-l (2014) Ambient organic carbon to elemental carbon ratios: influence of the thermal–optical temperature protocol and implications. Science of The Total Environment 468–469, 1103-1111.
| Crossref | Google Scholar | PubMed |
Cope M, S Lee, M Meyer, F Reisen, C Trindade, A Sullivan, N Surawski, A Wain, D Smith, B Ebert (2019) Smoke emission and transport modelling, June 2019, Research Report 102. Available at https://www.ffm.vic.gov.au/__data/assets/pdf_file/0027/420759/Final-Report_May_2019_v1.1-1.pdf
Crutzen PJ, Andreae MO (1990) Biomass burning in the tropics: impact on atmospheric chemistry and biogeochemical cycles. Science 250(4988), 1669-1678.
| Crossref | Google Scholar | PubMed |
Desservettaz M, et al. (2017) Emission factors of trace gases and particles from tropical savanna fires in Australia. Journal of Geophysical Research: Atmospheres 122(11), 6059-6074.
| Crossref | Google Scholar |
Griffith DWT (1996) Synthetic calibration and quantitative analysis of gas-phase FT-IR spectra. Applied Spectroscopy 50(1), 59-70.
| Crossref | Google Scholar |
Griffith DWT, Deutscher NM, Caldow C, Kettlewell G, Riggenbach M, Hammer S (2012) A Fourier transform infrared trace gas and isotope analyser for atmospheric applications. Atmospheric Measurement Techniques 5(10), 2481-2498.
| Crossref | Google Scholar |
Guérette EA, Paton-Walsh C, Desservettaz M, Smith TEL, Volkova L, Weston CJ, Meyer CP (2018) Emissions of trace gases from Australian temperate forest fires: emission factors and dependence on modified combustion efficiency. Atmospheric Chemistry and Physics 18(5), 3717-3735.
| Crossref | Google Scholar |
Haverd V, Raupach MR, Briggs PR, Canadell JG, Davis SJ, Law RM, Meyer CP, Peters GP, Pickett-Heaps C, Sherman B (2013) The Australian terrestrial carbon budget. Biogeosciences 10(2), 851-869.
| Crossref | Google Scholar |
Howard D, Macsween K, Edwards GC, Desservettaz M, Guérette E-A, Paton-Walsh C, Surawski NC, Sullivan AL, Weston C, Volkova L (2019) Investigation of mercury emissions from burning of Australian eucalypt forest surface fuels using a combustion wind tunnel and field observations. Atmospheric Environment 202, 17-27.
| Crossref | Google Scholar |
Meyer C, Cook GD, Reisen F, Smith T, Tattaris M, Russell‐Smith J, Maier S, Yates C, Wooster M (2012) Direct measurements of the seasonality of emission factors from savanna fires in northern Australia. Journal of Geophysical Research: Atmospheres 117(D20), D20305.
| Crossref | Google Scholar |
Milligan DB, Francis GJ, Prince BJ, McEwan MJ (2007) Demonstration of selected ion flow tube MS detection in the parts per trillion range. Analytical Chemistry 79(6), 2537-2540.
| Crossref | Google Scholar | PubMed |
Muccio Z, Jackson GP (2009) Isotope ratio mass spectrometry. Analyst 134(2), 213-222.
| Crossref | Google Scholar | PubMed |
Paton-Walsh C, Smith TEL, Young EL, Griffith DWT, Guérette ÉA (2014) New emission factors for Australian vegetation fires measured using open-path Fourier transform infrared spectroscopy – Part 1: Methods and Australian temperate forest fires. Atmospheric Chemistry and Physics 14(20), 11313-11333.
| Crossref | Google Scholar |
Price OF, Rahmani S, Samson S (2023) Particulate levels underneath landscape fire smoke plumes in the Sydney region of Australia. Fire 6(3), 86.
| Crossref | Google Scholar |
Rea G, Paton-Walsh C, Turquety S, Cope M, Griffith D (2016) Impact of the New South Wales fires during October 2013 on regional air quality in eastern Australia. Atmospheric Environment 131, 150-163.
| Crossref | Google Scholar |
Reisen F, Meyer CP, Weston CJ, Volkova L (2018) Ground-based field measurements of PM2.5 emission factors from flaming and smoldering combustion in eucalypt forests. Journal of Geophysical Research: Atmospheres 123(15), 8301-8314.
| Crossref | Google Scholar |
Smith T, Paton-Walsh C, Meyer C, Cook GD, Maier SW, Russell-Smith J, Wooster M, Yates CP (2014) New emission factors for Australian vegetation fires measured using open-path Fourier transform infrared spectroscopy – Part 2: Australian tropical savanna fires. Atmospheric Chemistry and Physics 14(20), 11335-11352.
| Crossref | Google Scholar |
Storey MA, Price OF (2022) Prediction of air quality in Sydney, Australia, as a function of forest fire load and weather using Bayesian statistics. PLoS One 17(8), e0272774.
| Crossref | Google Scholar | PubMed |
Sullivan AL (2017a) Inside the inferno: Fundamental processes of wildland fire behaviour: Part 1: Combustion chemistry and heat release. Current Forestry Reports 3, 132-149.
| Crossref | Google Scholar |
Sullivan AL (2017b) Inside the inferno: Fundamental processes of wildland fire behaviour: Part 2: Heat transfer and interactions. Current Forestry Reports 3, 150-171.
| Crossref | Google Scholar |
Sullivan AL, Ball R (2012) Thermal decomposition and combustion chemistry of cellulosic biomass. Atmospheric Environment 47, 133-141.
| Crossref | Google Scholar |
Sullivan AL, WL McCaw, MG Cruz, S Matthews, PF Ellis (2012) Fuel, fire weather and fire behaviour in Australian ecosystems. In ‘Flammable Australia: fire regimes, biodiversity and ecosystems in a changing world’. (Eds Ross A Bradstock, A Malcolm Gill, Richard J Williams) pp. 51–77. (CSIRO Publishing: Melbourne, VIC, Australia)
Sullivan AL, Knight IK, Hurley RJ, Webber C (2013) A contractionless, low-turbulence wind tunnel for the study of free-burning fires. Experimental Thermal and Fluid Science 44, 264-274.
| Crossref | Google Scholar |
Sullivan AL, Surawski NC, Crawford D, Hurley RJ, Volkova L, Weston CJ, Meyer CP (2018) Effect of woody debris on the rate of spread of surface fires in forest fuels in a combustion wind tunnel. Forest Ecology and Management 424, 236-245.
| Crossref | Google Scholar |
Surawski NC, Sullivan AL, Meyer CP, Roxburgh SH, Polglase PJ (2015) Greenhouse gas emissions from laboratory-scale fires in wildland fuels depend on fire spread mode and phase of combustion. Atmospheric Chemistry and Physics 15(9), 5259-5273.
| Crossref | Google Scholar |
Surawski NC, Sullivan AL, Roxburgh SH, Meyer CPM, Polglase PJ (2016) Incorrect interpretation of carbon mass balance biases global vegetation fire emission estimates. Nature Communications 7(1), 11536.
| Crossref | Google Scholar | PubMed |
Urbanski SP (2013) Combustion efficiency and emission factors for wildfire-season fires in mixed conifer forests of the northern Rocky Mountains, US. Atmospheric Chemistry and Physics 13(14), 7241-7262.
| Crossref | Google Scholar |
Volkova L, Meyer CM, Murphy S, Fairman T, Reisen F, Weston C (2014) Fuel reduction burning mitigates wildfire effects on forest carbon and greenhouse gas emission. International Journal of Wildland Fire 23(6), 771-780.
| Crossref | Google Scholar |
Volkova L, Roxburgh SH, Surawski NC, Meyer CM, Weston CJ (2019) Improving reporting of national greenhouse gas emissions from forest fires for emission reduction benefits: an example from Australia. Environmental Science & Policy 94, 49-62.
| Crossref | Google Scholar |
Wasserstein RL, Lazar NA (2016) The ASA’s statement on p values: context, process, and purpose. American Statistician 70(2), 129-133.
| Crossref | Google Scholar |
Wooster MJ, Freeborn PH, Archibald S, Oppenheimer C, Roberts GJ, Smith TEL, Govender N, Burton M, Palumbo I (2011) Field determination of biomass burning emission ratios and factors via open-path FTIR spectroscopy and fire radiative power assessment: headfire, backfire and residual smouldering combustion in African savannahs. Atmospheric Chemistry and Physics 11(22), 11591-11615.
| Crossref | Google Scholar |
Yokelson RJ, Goode JG, Ward DE, Susott RA, Babbitt RE, Wade DD, Bertschi I, Griffith DWT, Hao WM (1999) Emissions of formaldehyde, acetic acid, methanol, and other trace gases from biomass fires in North Carolina measured by airborne Fourier transform infrared spectroscopy. Journal of Geophysical Research 104(D23), 30109-30125.
| Crossref | Google Scholar |