Using hydrological modelling to improve the Fire Weather Index system over tropical peatlands of peninsular Malaysia, Sumatra and Borneo
J. Mortelmans


A
B
C
D
E
F
G
Abstract
Tropical peatland fires contribute to global carbon emissions and air pollution.
Enhance the globally used Canadian Fire Weather Index (FWI) system specifically over drained and undrained tropical peatlands in southeast Asia.
We included simulated tropical peatland hydrology in the FWI, creating a new peatland-specific version of the FWI (FWIpeat). FWIpeat, the original FWI (FWIref) and the drought code (DC) were evaluated against satellite-based active fire occurrence from 2002 to 2018.
The DC shows superior performance in explaining fire occurrence over undrained tropical peatlands. Over drained peatlands, DC and FWIpeat show similar results, both outperforming FWIref. A comparison with an earlier study over boreal peatlands indicates much smaller improvements from FWIpeat for tropical peatlands, possibly due to a lower accuracy of the hydrological input data.
Our results highlight the importance of including information on deeper soil layers, i.e. the DC or groundwater table, when assessing fire danger.
Although this study offers a promising approach for operational fire management over tropical peatlands, we emphasise the need for further research to refine the hydrological input data and explore additional constraints from Earth observation data.
Keywords: Fire Weather Index, FWI, Groundwater table, hydrological data, PEATCLSM, peatland fires, southeast Asia.
Introduction
Tropical peatlands
Tropical peatlands cover at least 44 Mha of land (Page et al. 2022), and likely more, given a 59-Mha best estimate (Leifeld and Menichetti 2018). They are predominantly located in southeast Asia (SEA; Page et al. 2011). The SEA peatlands store approximately 68 Gt carbon (C), constituting 77% of the global C stock in tropical peatlands (Page et al. 2011). Under natural, wet conditions, peatlands act as net C sinks, capturing atmospheric C and storing it in the soil by inhibiting the decomposition of organic plant matter (Gallego-Sala et al. 2018). However, peatland disturbance can threaten these large C stocks and convert them into sources of greenhouse gases. This disturbance can occur gradually, e.g. through the implementation of drainage systems, like ditch networks, for agriculture, forestry, or road construction (Miettinen et al. 2016; Dadap et al. 2021). Consequently, the peat becomes drier, triggering renewed biological activity and resulting in subsidence, i.e. the permanent lowering of the peat surface (Hooijer et al. 2012). This slowly converts the peatland from C sink to source, yet effects are long-lasting (Couwenberg et al. 2010; Hooijer et al. 2012; Jauhiainen et al. 2012).
Peatland fires
Other disturbances, such as wildfires, can result in a more abrupt conversion from sink to source (Loisel et al. 2021). During a peatland fire, the combustion of peat releases a substantial amount of C into the atmosphere in the form of CO, CO2 and CH4. This sudden release occurs when the accumulated organic matter in the peat burns, transforming the peatland from a C sink to a short-lived, yet large C source. Over the last decades, fire has become an increasingly important disturbance for Indonesian peatlands (Dommain et al. 2014), with many large fires occurring during the 1997–1998 El Niño event (Page et al. 2002) and a major subsequent event in 2015–2016 (Huijnen et al. 2016). Page et al. (2002) estimated that the peatland fires during the 1997–1998 El Niño season in Indonesia released between 0.81 and 2.57 Gt C, equalling approximately 13–40% of the mean annual global fossil fuel C emissions. The 2015 fires raged through September and October, emitting on average 0.11 Gt C per day (2.27 Gt C in total), exceeding the daily emissions of fossil fuels from the European Union (Huijnen et al. 2016). The emissions from single fire seasons provide insight into the vulnerability of the C stored in Indonesian peatlands, and highlight the importance of reducing fire risk to protect these peatland areas.
Wildfires in Indonesia are often linked to drought conditions, particularly during strong El Niño years (Taufik et al. 2017), but other factors contribute to anomalous fire seasons as well (Fernandes et al. 2017). Fernandes et al. (2017) showed that temperature anomalies play a significant role in fire occurrence, even during wet years or years with average precipitation. This highlights the complexity of fire management strategies, which typically focus on dry seasons. Peatland drainage exacerbates fire risk by creating a hydrologically dry environment (Taufik et al. 2017; Dadap et al. 2021; Page et al. 2022). Miettinen et al. (2016) showed that only 6% of the SEA peatlands were in pristine condition in 2015, whereas more than 65% of that peatland area contained drainage canals in 2021, according to Dadap et al. (2021). Dadap et al. (2021) did not include narrow drainage canals (<5 m wide) in their analysis, likely underestimating the drained area. Unlike the boreal region, where lightning is the dominant ignition source of peat (Kasischke et al. 2002; Kasischke and Turetsky 2006), fires in Indonesia are predominantly ignited by humans. However, lightning may still play a role in more remote rainforests in Borneo and New Guinea (Janssen et al. 2023).
Forest fires, and vegetation fires in general, are generally dominated by flaming fires burning aboveground vegetation. Peatland fires, in contrast, are typically dominated by smouldering, i.e. a flameless belowground fire (Davies et al. 2013; Rein 2013; Turetsky et al. 2015; Purnomo et al. 2023). Smouldering combustion of peat spreads slowly and deep into the soil. It can persist for long periods, under relatively low temperatures, low oxygen and high moisture content, and even during precipitation events or changing fire weather (Rein et al. 2008, 2013; Turetsky et al. 2015; Huang and Rein 2017). These circumstances often lead to an incomplete combustion process, resulting in higher levels of CO, CH4 and NH3 as compared with flaming fires (Rein 2013; Hu et al. 2018; Hu and Rein 2022). The aerosol emissions caused by the combustion are responsible for large-scale haze events. This haze can cause extreme air pollution and harm human health (Crippa et al. 2016; Koplitz et al. 2016) and regional economies (Glauber et al. 2016).
The Fire Weather Index (FWI)
One of the most well-known fire danger rating systems is the Canadian Fire Weather Index (FWI) system (Van Wagner 1987). The FWI system derives potential fire spread and fuel consumption from weather observations of temperature, relative humidity, precipitation and wind speed. The FWI combines these four variables into a unitless value that represents fire danger, allowing application in other fire environments than a boreal upland forest, for which it was originally developed (Dymond et al. 2005; Taylor and Alexander 2006; de Groot et al. 2007, 2015; Di Giuseppe et al. 2020; Field 2020a; Matondang et al. 2021; BMKG 2024). However, the applicability of the FWI to rate fire danger over non-forested and wetland ecosystem types, including peatlands (Waddington et al. 2012) or drylands (Di Giuseppe et al. 2021), is often questioned.
The FWI empirically combines weather information into three moisture codes, each representing the moisture content of a different fuel class. The Fine Fuel Moisture Code (FFMC) represents the moisture content of the litter layer and the fine fuels on the surface. The Duff Moisture Code (DMC) represents the moisture content in the loosely compacted decomposing organic matter of the mineral soil (5–10 cm deep). The Drought Code (DC) represents the moisture in the deep, compact organic matter of the mineral soil (10–20 cm deep; Van Wagner 1987). Note that, despite representing moisture in organic soil material, the calibration of DMC and DC is specifically designed for predominantly mineral soils, and does not precisely capture the moisture content in different peat layers (Waddington et al. 2012). However, the incorporation of these moisture codes into the FWI highlights the developers’ acknowledgment of the importance of soil moisture in fire danger estimation (Krueger et al. 2022).
Mortelmans et al. (2024) replaced the meteorology-driven moisture codes of the FWI with the output of a peatland-specific hydrological model over boreal peatlands, creating a peatland-specific FWI. Their findings showed the advantages of integrating hydrological information in assessing fire risk. Through different experiments, they demonstrated that making the FWI system more dependent on groundwater table and surface moisture content enhances its predictive capability for fire occurrence.
de Groot et al. (2007) adapted the FWI system by defining thresholds of ‘low’, ‘medium’, ‘high’ and ‘extreme’ fire danger specifically for the Indonesian and Malaysian climate, creating an Indonesian fire danger rating system. This operational system is used by the Indonesian Bureau of Meteorology and Geophysics (BMKG, Badan Meteorologi, Klimatologi, dan Geofisika) to provide daily maps of the FWI and its components based on weather station data (de Groot et al. 2007). Owing to the sparse nature of this weather station network, a near-real-time FWI system was developed based on weather data from satellite observations (Matondang et al. 2021). Although the latter study noted the importance of accurate fire danger predictions for peatland fires, they did not adapt the FWI system to integrate peatlands in their fire danger rating system.
Soil moisture for fire occurrence estimations
Over the last decade, the relationships between soil moisture and groundwater tables and fire activity and risk have been increasingly studied based on the availability of satellite monitoring (Forkel et al. 2012; Le Page et al. 2015; Chaparro et al. 2016; Hantson et al. 2016; Leblon et al. 2016; Holgate et al. 2017; Taufik et al. 2017; Dadap et al. 2019, 2022; Ambadan et al. 2020; Field 2020b; Pettinari and Chuvieco 2020; Di Giuseppe et al. 2021; Mezbahuddin et al. 2023; Pelletier et al. 2023; Purnomo et al. 2023; Mortelmans et al. 2024). Satellite-based soil moisture estimates can indicate locations that pose a high fire activity risk. Ambadan et al. (2020) demonstrated that soil moisture anomalies derived from the European Space Agency (ESA) Soil Moisture and Ocean Salinity (SMOS) satellite mission (Kerr et al. 2010) or the NASA Soil Moisture Active Passive (SMAP) satellite (Entekhabi et al. 2010) could explain wildfire patterns in Canada. However, the use of these data for daily forecasting goals is limited owing to operational limitations, coarse spatial resolutions, low temporal resolution (>1 day), assumptions in the retrieval algorithm and latency of the remote sensing data products (Chaparro et al. 2016). Some of these limitations can be overcome by assimilating satellite-based radiance data into finer-resolution land surface models (De Lannoy and Reichle 2016; Reichle et al. 2019). Only recently was peatland information explicitly included in specific data assimilation products (Bechtold et al. 2020; Reichle et al. 2023).
Objectives and structure of this paper
This study aims to assess the applicability of the approach proposed by Mortelmans et al. (2024) in the undrained and drained tropical peatlands of peninsular Malaysia, Sumatra and Borneo. Although our methodology is based on that of Mortelmans et al. (2024), we anticipate potential variations in outcome. The tropical study area of this study differs from the boreal region of Mortelmans et al. (2024) primarily in terms of the main ignition source, humans versus lightning, climate and land use. Human activities, which can include deliberate actions, may impact the performance of both the original FWI (FWIref) and the peatland-specific FWI (FWIpeat), especially considering that ignition events can occur even when peatland is wet. Mortelmans et al. (2024) distinguished between early and late season fires, hypothesising a greater human influence and lower hydrological control on the occurrence of earlier fires. Their findings revealed a reduced predictive capability of FWIref for early fires compared with late fires, along with a less substantial improvement with the peatland-specific FWI. Consequently, owing to higher human influence in the tropical regions, we hypothesise that FWIref will exhibit inferior performance over tropical peatlands of SEA, with a correspondingly smaller improvement for FWIpeat, relative to the results obtained in the boreal region. Moreover, despite the original calibration of the FWI system for a boreal jack pine forest, suggesting potential limitations in its application to tropical regions, previous studies have shown a positive correlation between the FWI and burned forest area in SEA (Abatzoglou et al. 2018; Jones et al. 2022), illustrating the complexity involved in anticipating the performance of the FWI system across diverse fire regimes.
Regardless of the performance of FWIref, we expect an improvement by including hydrological information. Furthermore, the vast majority (~93%; Leifeld and Menichetti 2018) of boreal peatlands is pristine (undrained), much more than the 6% estimate for tropical SEA (Miettinen et al. 2016). This difference will undoubtedly influence fire behaviour, as drained peatlands are more vulnerable to fires. As the meteorological variables used in the FWI system do not take drainage of peatland into account, incorporating specific hydrology for drained and undrained peatlands is expected to be beneficial in assessing fire danger.
Hereafter, we first discuss the study area, the data used and the evaluation approach. Subsequently, we show and discuss the results together with some shortcomings of this study and datasets used. Lastly, the main conclusions and outlook for future research are summarised.
Methods
Study area and datasets
We studied fires from 2002 through 2018 for the same study area as Miettinen et al. (2016), ranging from 7°S 95°E to 7.4°N 120°E, and comprising peninsular Malaysia, Sumatra and Borneo (Fig. 1). The peatland distribution of this region was derived from peatland land cover maps of Miettinen et al. (2016) for 2007 and 2015 and regridded to 9-km resolution on the EASEv2 grid. As no peatland distribution was available for each year, we used the 2007 map of Miettinen et al. (2016) for all years from 2002 through 2010, and from 2011 onwards, the 2015 map was used. Miettinen et al. (2016) also provided land use maps, which allowed us to classify the peatlands into two hydrological classes, ‘undrained’ and ‘drained’, in line with the hydrological model output that we use in the fire danger estimation. The ‘undrained’ class mainly corresponds to the ‘pristine peat swamp forest’ class in Miettinen et al. (2016), though minor fractions of ‘water’, ‘seasonal water’ and ‘mangrove’ classes were included as well. All other classes, mainly ‘industrial plantation’, ‘small-holder mosaic’, ‘degraded forest’, ‘secondary forest’ and ‘fern/low shrub’ were included in the ‘drained’ class. The resulting ‘undrained’ and ‘drained’ peatland distribution for 2015 is shown in Fig. 1a. The distribution of 2007 is not shown as this was visually very similar to the distribution of 2015.
Maps of the study area showing: (a) the ‘undrained’ and ‘drained’ peatland distribution in 2015, based on the maps of Miettinen et al. (2016); and (b) the average number of peatland fires per year for the years 2002–2018. The different focus regions (Borneo, Sumatra and peninsular Malaysia (PM)) are marked.
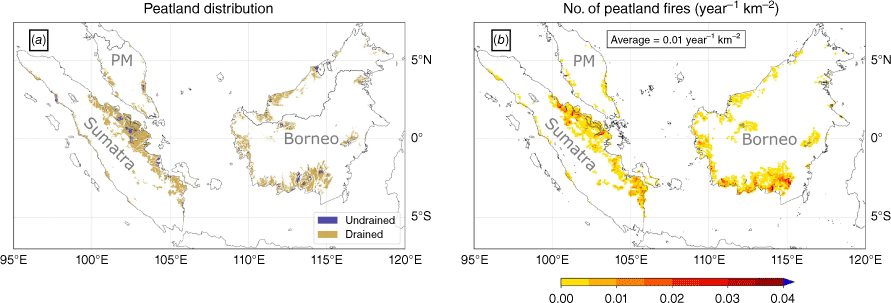
We used hydrological data derived from a peatland-specific land surface model (PEATCLSM; Bechtold et al. 2019), adapted for tropical peatlands (PEATCLSMTrop; Apers et al. 2022) and forced by MERRA-2 (Modern-Era Retrospective analysis for Research and Applications, Version 2) meteorology. The PEATCLSM model is based on NASA’s catchment land surface model (CLSM; Koster et al. 2000) and was run at a 9-km spatial resolution, providing daily output. Apers et al. (2022) adapted and extended the original PEATCLSM module of Bechtold et al. (2019), which was mainly developed to include the hydrology of natural northern peatlands, to simulate the hydrology of tropical natural (undrained; PEATCLSMTrop,Nat) and drained (PEATCLSMTrop,Drain) peatlands. Apers et al. (2022) developed a set of hydrological parameters for both undrained and drained tropical peatlands, based on literature data from peatlands in SEA. Note that the drained version represents only an average drainage intensity although in reality, anthropogenic drainage networks are highly heterogeneous in space (Dadap et al. 2021). PEATCLSMTrop,Drain was used over peatlands classified as drained by the maps of Miettinen et al. (2016) shown in Fig. 1, whereas PEATCLSMTrop,Nat was used over peatlands that fall in the undrained class. From this point forward, unless explicitly stated otherwise, ‘PEATCLSM’ refers to the tropical version of the model, PEATCLSMTrop.
Original FWI
Van Wagner (1987) developed the FWI system to rate fire danger in an upland boreal forest. The FWI system comprises six components: three moisture codes and three behaviour indices. The three moisture codes, each representing the moisture content of a different fuel type, empirically combine four meteorological variables, i.e. 10-m wind speed (V10m), 2-m relative humidity (RH2m), 2-m temperature (T2m) and daily precipitation (P). The structure of the FWI system is represented in Fig. 2. The meteorological input data are extracted from the NASA MERRA2 (Gelaro et al. 2017) as in Mortelmans et al. (2024). These data are at a spatial resolution of 0.5° by 0.625° and bilinearly interpolated to the 9-km PEATCLSM grid. The first moisture code, the FFMC, combines T2m, RH2m, V10m, P and the FFMC of the previous day to give an estimate of the moisture content of litter and fine surface fuels. It is a unitless number ranging from 0 to 99, with values >90 generally considered to be extreme. As a representation of the top fuel layer, the FFMC has a short reaction time to drying, called the time lag. Van Wagner (1987) defined this time lag as the time necessary to lose two-thirds of the free moisture above equilibrium. For the FFMC, this is two-thirds of a day, indicating fast rewetting after rainfall and quick drying thereafter. The second moisture code, the DMC, is a unitless, open-ended estimate of the moisture content in the loosely compacted organic material in the soil and depends on T2m, RH2m, P and the DMC of the day before. Although theoretically, there is no maximum value, values >60 are generally considered to be extreme. At 12 days, the time lag for the DMC is longer than that of the FFMC, resulting in slower responses to rewetting and drying. The third and last moisture code, the DC, represents the moisture content in deep, compact organic soil layers. The DC depends on T2m, P and the DC of the day before and is also unitless and open-ended. Values >400 are generally considered to be extreme. The DC has a time lag of 52 days (Van Wagner 1987).
Structure of the Canadian FWI system and adaptations for FWIpeat (dashed arrows), with T2m the 2-m air temperature, RH2m the 2-m relative humidity, P the daily precipitation, V10m the 10-m wind speed, t the timestep, and f() representing non-linear, empirical functions. ‘zbar’ is the PEATCLSM groundwater table output. Adapted from Mortelmans et al. (2024) (CDF, cumulative density function).
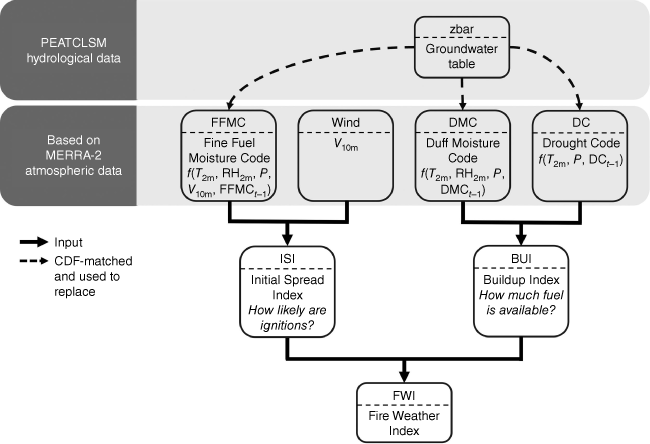
These three moisture codes are combined, together with V10m, to derive the Initial Spread Index (ISI) and the Buildup Index (BUI), two of the three behaviour indices of the FWI system. The ISI empirically combines the FFMC and V10m, and represents the ability of a fire to ignite and spread. The second behaviour index, the BUI, empirically combines the DMC and DC and represents the amount of fuel that is available for a fire to sustain itself. Both the ISI and BUI are unitless and open-ended. Lastly, the ISI and BUI are empirically combined into the third behaviour index, the FWI. The FWI is a measure of potential fire danger and is again unitless and open-ended, with higher values representing higher fire danger (Van Wagner 1987).
FWIpeat
Mortelmans et al. (2024) experimented with different adapted versions of the FWIref system. They concluded that providing the FWI system with peat-specific hydrological data could further improve FWI performance over northern peatlands, especially during the boreal summer. Here, we set up the same experiments as in Mortelmans et al. (2024), and added two additional ones. These new experiments were designed to cope with challenges in simulating peat soil moisture for periods with deep groundwater tables, e.g. during droughts or drainage, which are more common in tropical than boreal peatlands. The exact set-up of all experiments and their results are shown in the Supplementary Figs S1–S4.
For simplicity, Fig. 2 shows only the best-performing experiment. For this experiment, further referred to as FWIpeat, we replaced all the original moisture codes (FFMC, DMC and DC) with the PEATCLSM groundwater table (zbar). The variable zbar represents the mean peatland groundwater level within a grid cell, provided separately for both the drained and undrained fractions of the cell. We emphasise that within each peatland class (drained/undrained), most of the parameters are spatially uniform owing to the lack of datasets available that could constrain the spatial variability of parameter values. Specifically, each peatland class is simulated with a single set of parameters describing the anthropogenic drainage network, microtopography, peat properties, discharge function and plant water stress. The only spatially varying input next to the climate forcing that is used in PEATCLSM is the input datasets that are also used in CLSM to characterise surface and vegetation properties, namely albedo, greenness fraction, leaf area index and land cover type. Those parameters exert strong control on processes related to energy balance calculations, and thus eventually the drawdown of the groundwater level in dry periods.
As zbar typically ranges from −2 to 0, and the components of the FWI system have a vastly different range (e.g. 0 to infinity for DC), a direct replacement of these components would require a recalibration of the whole system. Instead, because we wanted to maintain the integrity of the calibrated FWI system, we matched the distribution of zbar over time to that of each moisture code using cumulative density function (CDF) matching. This method ensures that the statistical properties of the groundwater table data align with those of the moisture codes over time. The CDF-matching was done for each grid cell separately using the pytesmo package in Python (Paulik et al. 2023). This CDF-matching approach allows a conservative replacement of the moisture codes, without the need to recalibrate the entire FWI system to the zbar data. Furthermore, this type of temporal CDF-matching preserves the dynamic features of the PEATCLSM output, while still matching the climatology of the moisture codes. However, by doing this on a per-grid-cell basis, any spatial bias that is still present in the FWI system is maintained in the new FWIpeat. The CDF-matching approach was tested on a per-grid-cell basis and on all available grid cells at once, but the latter resulted in a slight decrease in performance. We therefore preferred to adopt the per-grid-cell approach, to be in line with the work of Mortelmans et al. (2024). By doing so, the results shown here are independent of the domain and thus would not change for the present study if we expanded the approach over all tropical peatlands.
This specific FWI adaptation was not implemented in Mortelmans et al. (2024) for the boreal region. Therefore, we explain the rationale for not using dynamically modelled surface soil moisture in the adjustment of the near-surface codes, i.e. DMC and FFMC. For the boreal region, PEATCLSM model simulations typically estimated a strong capillary connectivity between groundwater table, root zone and surface soil moisture based on the peat soil physical parameters from literature, with only mild hydrostatic disequilibrium states. However, over tropical peatlands, Apers et al. (2022) noticed a frequent disruption of that link during periods with deep groundwater tables when applying available information about peat soil hydraulic parameters in drained peatlands. They only relaxed that decoupling for the connection between groundwater and root zone in PEATCLSMTrop,Drain, while still allowing the surface layer to strongly deviate from the expected value under hydrostatic equilibrium. It could be of great value to include this information in the fire danger estimation; however, owing to the highly heterogeneous conditions (e.g. different disturbance and drainage levels) in degraded tropical peatlands, the model may often be wrong in estimating the timing of the capillary disconnection. Thus, including the strong dynamics of the model in the FWI may cause more harm than good. Therefore, systematically using the smoother dynamics of zbar for all moisture codes may be a better option.
Evaluation
Both FWIref and FWIpeat were computed over the peatlands marked in Fig. 1a. We evaluated their performance against a peatland-specific fire occurrence dataset derived from the global fire atlas (GFA; Andela et al. 2019) for the years 2002 through 2018. To retrieve peatland fire occurrences from this dataset, the GFA fire occurrences were spatially masked based on the peatland maps used in this study, i.e. if the extent of a fire partially or completely overlapped with a peatland area, it was considered a peatland fire. As the peatland dataset was divided into drained and undrained categories, we applied the same classification to fire occurrences. However, in reality, there is no clear boundary between drained and undrained peatlands, as drainage effects often extend into neighbouring undrained areas (Evans et al. 2019). This transitional zone is not considered in the present study. Fire events from the GFA were overlapped with the peatland land use classes and categorised as either ‘undrained’ or ‘drained’ based on the same method used for land use classification (see ‘Study area and datasets’ section).
The GFA is based on the Moderate Resolution Imaging Spectroradiometer (MODIS) Collection 6 MCD64A1 burned area product with 500-m resolution (Giglio et al. 2018). Given the spatial resolution of MCD64A1, small sub-pixel fires usually remain below the MCD64A1 detection threshold and are not included in the GFA (Andela et al. 2019; Chen et al. 2023). Our peatland-specific fire dataset thus included all fires observed by MODIS occurring on peatlands (Fig. 1b).
Next, we used the start and end date of all peatland fire events identified by the GFA to evaluate the FWI as an indicator of danger of active fire occurrence. Therefore, we extracted the FWIref and FWIpeat for each day and location where an active fire was present in the peatland-specific fire dataset, taking both fire occurrence and the duration of the fire into account. In focusing on ‘fire occurrence’, i.e. actively burning fires, rather than ‘fire ignition’, longer-lasting fires carry more weight and have a stronger influence on the evaluation results. Unless specified otherwise, a ‘fire’ hereafter refers to a peat fire.
As fires are rare events, a robust evaluation method that accounts for the abundance of ‘no-fire’ events is necessary. For this reason, we used a receiver operating characteristics (ROC) curve and the area under the curve (AUC) to compare the performance of FWIref and FWIpeat in estimating fire occurrences. An ROC curve considers the full range of FWI values, calculating a true positive rate (TPR) and false positive rate (FPR) for each FWI percentile. The TPR represents the proportion of correctly predicted daily fire occurrences over all data points in space and time. The ROC curve, and specifically the AUC, can then be used to compare the predictive performance of different models. A model with perfect predictive capability (i.e. predicting all fires without false alarms or missed events) would have an AUC of 1 and the ROC curve would be close to the top left corner. In contrast, a model with an AUC ≤ 0.5 is equivalent to a random guess and lacks predictive power, i.e. the model has the same or less predictive capability than a uniform distribution. As the ROC curve is based on a contingency table, we did not take multiple fires occurring in the same 9-km grid cell at the same day into account, but considered this as one fire occurrence.
To improve robustness, we applied bootstrapping by randomly sampling the data (with replacement) used in calculating the ROC curve. By repeating this process 1000 times, we generated a 95% confidence interval around the ROC curve for more reliable results.
Additionally, the ROC analysis was extended to all individual components of the reference FWI system, including all moisture codes and the two behaviour indices (Supplementary Fig. S3). We found that DC outperformed both FWIref and FWIpeat, making it the best predictor for fire occurrence. DC was therefore included in all subsequent analysis for comparison.
Next, to get a more in-depth understanding of the data and to more clearly understand for which range of FWI values we see a difference between FWIref, and FWIpeat, the distributions of fire occurrences, number of grid cells and ratio of pixels with fire occurrences with number of pixels for each FWI value were plotted.
Lastly, the mean seasonal cycles of fire occurrences and various fire weather indices over the tropical study region (in this paper) and the boreal study region (Mortelmans et al. 2024), were compared to understand the differences in performance of the fire weather indices in both regions. Specifically, we determined the seasonality of the fire weather indices of this paper, i.e. FWIref and FWIpeat, and compared those with the observed tropical fire regimes. Similarly, we determined the seasonality of the two best-performing fire weather indices for the boreal region (called ‘EXP2’ for the early fires and ‘EXP4’ for the late fires) and compared those with the observed fire regimes in the boreal zone. For EXP2, the original DC was replaced by PEATCLSM groundwater table and the DMC by PEATCLSM surface moisture content. For EXP4, the FWI was directly replaced by PEATCLSM groundwater table (Mortelmans et al. 2024). Note that for the boreal study region, the original PEATCLSM version is used, as this is mainly designed for northern natural peatlands (Bechtold et al. 2019).
Results and discussion
Spatio-temporal performance assessment for undrained and drained peatlands
Fig. 3 compares the performance of FWIref, DC and FWIpeat using an ROC curve and its corresponding AUC. Based on the AUC in Fig. 3b, it is clear that using zbar as input for all three moisture codes of the FWI or DC directly results in modest improvements for drained peatlands, with an improvement of 0.04 in the AUC compared with FWIref. The 95% confidence intervals of all drained ROC curves are very narrow (and not clearly visible), indicating the robustness of the improvement in AUC. For the undrained peatlands (Fig. 3a), we found a smaller improvement of 0.01 in the AUC for FWIpeat, whereas DC shows an improvement of 0.04. Here, the 95% confidence intervals are wider compared with the drained peatlands, indicating a larger uncertainty. The slightly overlapping confidence intervals of FWIref and FWIpeat suggest that the differences in the AUC might be due to chance rather than a true difference in predictive capability. The confidence interval of DC shows less overlap with that of FWIref for the lower FWI thresholds (right part of the plot), indicating a robust improvement when using DC. This improvement with DC is not seen for drained peatlands.
ROC curves and corresponding AUC for (a) undrained, and (b) drained tropical peatlands. The shaded areas indicate the 95% confidence interval determined by bootstrapping.
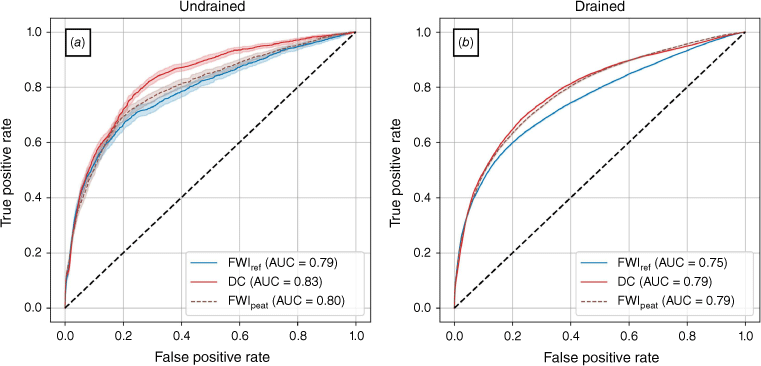
The difference in performance between FWIref and FWIpeat or DC depends on the threshold used. For both the drained and undrained peatlands, all ROC curves show similar predictive capabilities at the lower range of TPR and FPR (left part of the ROC curve, higher threshold values). The main differences are mainly seen for the lower threshold values (right part of the ROC curves), indicating a more accurate fire occurrence prediction under relatively low risk circumstances, i.e. low FWI or DC.
The differences in performance between DC, zbar and the various FWI experiments may be related to the differences in temporal autocorrelation length (τ) of these variables. The value of τ is linked to the time lag described in Van Wagner (1987) and defined as the time (in days) over which the system’s current state is influenced by its past states before becoming effectively independent (here defined as correlation dropping below 1/e). With 70 days (undrained) and 65 days (drained), this τ is longest for zbar. FWIref showed a τ of 42 days, regardless of peatland drainage classification, FWIpeat had a τ of 54 days for undrained peatlands and 50 days for drained peatlands. Lastly, with 59 days for both undrained and drained peatlands, the τ of DC was slightly higher than that of FWIpeat but shorter than that of zbar.
The equal performance that was found over drained peatlands for DC and FWIpeat and the lower performance for the direct use of zbar may indicate that the high τ is an overestimation of the true dynamics. The high diversity in anthropogenic drainage and water management practices poses challenges in modelling water level dynamics of drained tropical peatlands (Dadap et al. 2022). Paired with uncertain profiles of soil hydraulic properties (Apers et al. 2022), this makes modelling of the capillary disconnection between the groundwater table and the surface soil layer very uncertain. This may explain that in an FWI adaptation, the use of highly dynamical (though often erroneous) surface soil moisture eventually performed worse than only using the smoother zbar dynamics.
The reason for the better performance that is found over undrained peatland for DC compared with FWIpeat may be related to various degrees of disturbance at the edges of undrained peatlands where most fires on peatlands occur that were here defined as ‘undrained’ based on the Miettinen classification (Dadap et al. 2021). This may eventually result in DC becoming a more suitable predictor than groundwater level from a model assuming an undrained peatland.
FWI distributions
Fig. 4a, b show the frequency distributions of all grid cells in space and time with a fire occurrence (No. datafire), while Fig. 4c, d show the FWI values of all grid cells regardless of fire occurrence. Fig. 4e, f show the ratio of the frequencies shown in Fig. 4a, c, and the frequencies shown in Fig. 4b, d, respectively. For both the undrained and drained peatland classes, two peaks can be seen for the distributions of fire occurrences. The first small peak is situated at the high-end of the ‘Low’ FWI danger class and dips at ~FWI = 1 (log(FWI) = 0), and originates from a change in empirical equations at FWI = 1. The second, larger peak occurs in the ‘High’ and ‘Extreme’ FWI danger class. This second peak is a bit smaller for FWIpeat than for FWIref for both drainage classes. The first peak is in both cases substantially higher for FWIpeat, with, for the undrained peatlands, more than twice as many grid cells with a fire occurrence for these FWI values for FWIpeat. From these distributions, we can conclude that most fires occurrences coincide with a ‘High’ or ‘Extreme’ FWI value. The first, smaller peak, which coincides with low FWI values, is most likely due to human ignitions, e.g. for slash-and-burn practices, which would take place when conditions are sufficiently dry for a fire to occur, but too wet for fires to spread out of control. As this peak is much narrower for FWIpeat than it is for FWIref, especially at the lower end of the FWI (left on the figure), we can conclude that FWIpeat more accurately describes these conditions and provides a more constrained FWI range for fires occurring during relatively moist conditions. This corroborates with the findings from the ROC curves (Fig. 3), where we mainly saw an improvement in the ‘Low’ FWI danger class.
The frequency of FWI values of all grid cells in space and time (daily resolution) with a fire occurrence (No. datafire) in each bin is shown for (a) undrained and (b) drained peatlands. The frequency of FWI values of all grid cells in space and time regardless of fire occurrence (No. dataall) in the same bins is shown for (c) undrained and (d) drained peatlands. The ratio of the frequencies in a and c, and the frequencies in b and d are shown in e and f, respectively. The Low, Medium, High, and Extreme fire danger are based on the thresholds used by the Indonesian Bureau of Meteorology and Geophysics: Low < 2; Medium = 2–6; High = 7–13; Extreme > 13 (BMKG 2024). The minor thicks on the x-axis indicate the bin edges.
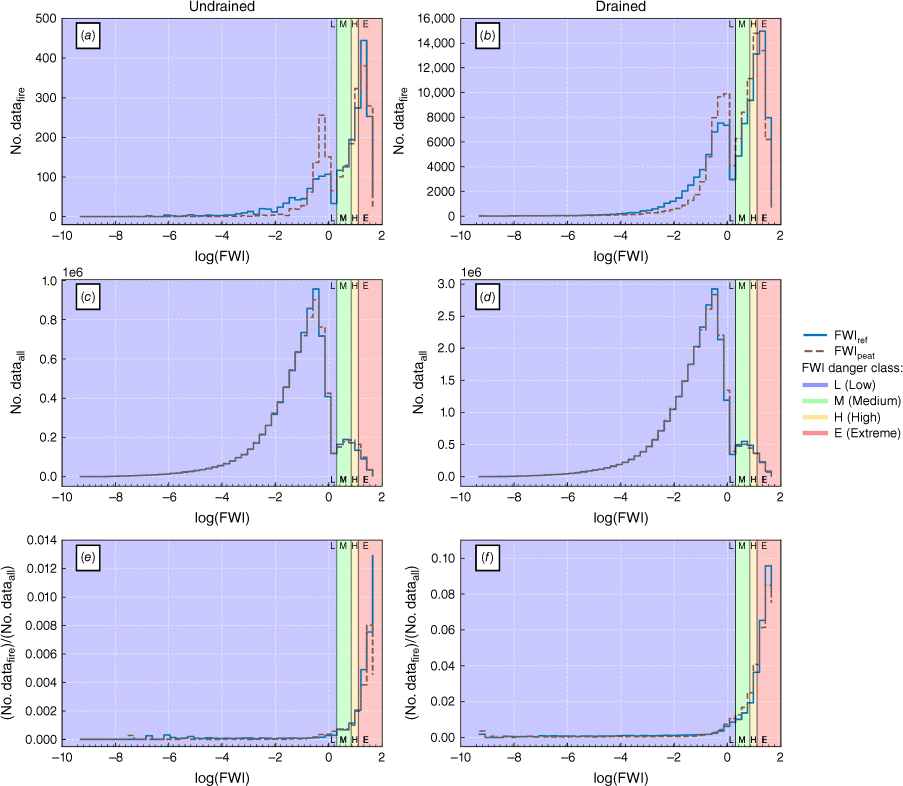
Fig. 4c, d shows similar distributions, with a very high peak at the high-end of the ‘Low’ FWI danger class, coinciding with the first, smaller peak seen in Fig. 4a, b. A smaller, second peak can be seen here as well, in this case in the ‘Medium’ FWI danger class. Of course, if more grid cells have a specific FWI value, it is more likely for a fire to coincide with this value. The distributions of the FWI values indicate relatively few grid cells that fall in the ‘Extreme’ FWI danger class.
A combination of the distributions seen in the first two rows is shown in Fig. 4e, f, with the ratio of the number of grid cells with a fire over the total number of grid cells with that FWI value. From the distributions of fire occurrences (Fig. 4a, b), we can see that there are many fire occurrences when the FWI reaches ‘Extreme’ values. From the distributions of the FWI values (Fig. 4c, d), we also know that there are relatively few grid cells with these ‘Extreme’ values. In Fig. 4e, f, we can then see that grid cells with an ‘Extreme’ FWI value clearly have a disproportionately high number of fire occurrences. The first, smaller peak in fire occurrences seen in Fig. 4a, b, coincides with the main peak in grid cells seen in Fig. 4c, d, so in Fig. 4e, f, we see that even though, in absolute numbers, a fair number of fires occur for these low FWI values, there are relatively few fire occurrences when the FWI is low.
As soon as the FWI reaches ‘Medium’ values (i.e. FWI > 2), there is a good indication that fires become more numerous, and once the FWI reaches ‘High’ (FWI > 7) or ‘Extreme’ (FWI > 13) values, there is a relatively high probability of fires occurring. For drained peatlands, in almost 10% of instances where the FWI reaches ‘Extreme’ values, a fire occurred. For the undrained peatlands, this is the case for approximately 1% of instances where the FWI reaches ‘Extreme’ values. The same conclusions can be made for DC (see Fig. S5). A higher DC indicates that fires become more numerous.
Performance differences between tropical and boreal region
The added value of integrating hydrological data in the FWI is smaller over the tropical study area considered in this study than over the boreal study area of Mortelmans et al. (2024). Mortelmans et al. (2024) observed an AUC improvement of up to 0.14 for their peatland-specific FWI compared with FWIref. For the tropical SEA peatlands, the largest AUC improvement is 0.04. These differences may stem from the above-mentioned differences in regional model configuration, land use and ecosystem characteristics. Firstly, fires in the boreal region are mainly caused by lightning (Kasischke et al. 2002; Kasischke and Turetsky 2006), whereas tropical fires are mainly human-ignited (Janssen et al. 2023). The evaluation of a fuel-based fire danger estimation, such as the FWI, is highly influenced by the dynamics in ignitions. By not taking these ignitions into account, we unavoidably introduced both missed events and false alarms (Di Giuseppe et al. 2020). Possible improvements through including hydrological input data may be partly masked by the influence of ignitions.
Secondly, the hydrology of tropical peatlands is much more difficult to accurately model than that of boreal peatlands owing to the occurrence of capillary disconnection between the peat surface and the groundwater table. This disconnection can occur under dry conditions, such as drainage, as mentioned above. Thirdly, in Mortelmans et al. (2024), the hydrological input data came from a data assimilation product in which SMOS L-band brightness temperature data were assimilated into PEATCLSM, whereas in the present study, the input came from model-only PEATCLSM simulations owing to unsolved challenges of assimilating coarse-scale observations over the coastal peatland areas of the study area.
Another factor causing the lower performance increase is that the FWIref already performed better over tropical SEA than over the boreal study area of Mortelmans et al. (2024). The AUC values of FWIref for both drained and undrained peatlands are higher for tropical SEA (0.79 for undrained and 0.75 for drained) than for boreal peatlands (0.69 for early fires and 0.70 for late fires; Mortelmans et al. 2024). It is important to note that the boreal study area of Mortelmans et al. (2024) was much larger, and much more heterogeneous in terms of climate and human influence, than the area considered here. Therefore, Mortelmans et al. (2024) differentiated between four regions, showing the performance of the FWI for late season fires in each region. Of these regional results, the FWIref performed best over Europe (AUC = 0.88), with an improvement of up to 0.06 for the peatland-specific FWI (Mortelmans et al. 2024). With an AUC of 0.75 (drained) to 0.79 (undrained), FWIref performs second best over tropical SEA. The good performance of FWIref over areas outside Canada, for which it was originally developed, illustrates the complexity of this system and shows its applicability in regions for which it was not originally calibrated, which emerges regionally in global-scale relationships between FWI and burned area, including our study region (Abatzoglou et al. 2018; Jones et al. 2022). It is crucial, however, to highlight that the FWI is being assessed in the present context over peatlands, for which it was not calibrated in the first place. Therefore, the better performance in these geographical regions could be a peatland-specific finding.
The relatively superior performance of both FWIref and FWIpeat over tropical SEA peatlands may be attributed in part to its alignment with the temporal dynamics of active fires, as depicted in the long-term averaged seasonal cycles in Fig. 5. It is essential to recognise, however, that this apparent correlation does not necessarily imply causation, as other factors, such as the dynamics of the ignition source, may influence these dynamics and are not taken into account here. However, the strong correlation between FWIref and active fires does suggest that, unless the other factors show strongly opposite dynamics, the fires are strongly meteorologically driven in the long term. Furthermore, unlike the area considered in our study, the boreal study area of Mortelmans et al. (2024) exhibits greater heterogeneity in fire regimes, not only owing to its larger longitudinal extent but also its larger latitudinal extent. This broader latitudinal range leads to varying peak FWI values at different locations, resulting in a broad peak, as observed in Fig. 5b. Although both study areas display a bimodal seasonal cycle of fires (Fig. 5a, b), with the second peak representing the highest incidence of active fires, the considerable heterogeneity within the study area of Mortelmans et al. (2024) results in divergent FWI system performance across different regions, indicated by their range of AUC values for FWIref (from 0.66 to 0.88; Mortelmans et al. 2024).
Long-term averaged seasonal cycle of active fires and the different FWI for peatlands in (a) tropical SEA, and (b) the boreal region. DOY, day of year. EXP2 and EXP4 refer to different experiments performed by Mortelmans et al. (2024).
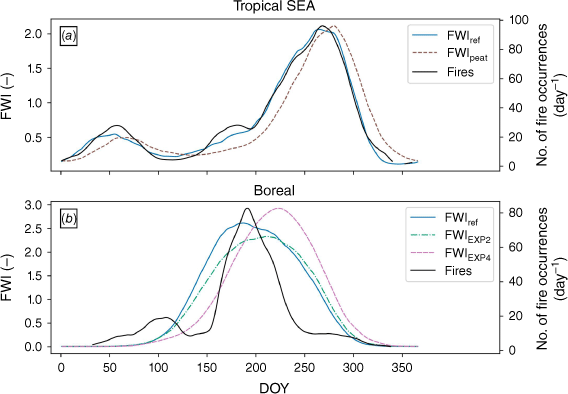
The observed time lag between the FWIpeat peaks and the active fires for the tropical SEA peatlands (Fig. 5a) suggests a potential overestimation of the influence of deep water levels relative to surface drying in the model. As shown in Fig. 2, all moisture codes of FWIpeat are replaced with the PEATCLSM groundwater table, resulting in a greater influence of the long time lags associated with groundwater table changes. This lag is most likely an interplay of several different effects that are not taken into account in this domain-averaged seasonal evaluation. In this regard, it is important to note that short-term spatio-temporal dynamics are not resolved in this long-term averaged seasonal cycle, whereas they are taken into account in the calculation of the ROC curves and corresponding AUC (Fig. 3). Aside from the fact that Fig. 5 represents the domain-averaged long-term seasonality, the observed time lag for FWIpeat is linked to the temporal autocorrelation length (τ), which is 42 days for FWIref and 50–54 days for FWIpeat, indicating a slower response of FWIpeat to rainfall and drying, thus a shift in seasonality.
Several other studies have used hydrological data for fire (danger) estimation in tropical peatlands. Mezbahuddin et al. (2023) demonstrated the potential of using soil moisture and water table dynamics in a machine learning framework for fire danger estimation over peatlands in Indonesia. They showed that a machine learning model that combines weather data with hydrological information resulted in more accurate estimations of fire danger relative to a reference machine learning model that only used weather data (Mezbahuddin et al. 2023). It is important to note that they exclusively evaluated the performance of their two models against each other, and did not include an independent framework, such as the FWI, in their assessment. It is possible that neither of their machine learning models effectively extracted the time-lagged response of weather on the different fuel compartments, as done in the moisture code routines of the FWI. Therefore, the added value of including hydrological information was not proved with respect to the most established framework for fire danger assessment.
Challenges and opportunities of FWIpeat
Land surface models, such as PEATCLSM, depend on representations of soil properties, vegetation, hydrological processes and parameterisations, all of which are associated with significant uncertainties (Clark et al. 2015; Vereecken et al. 2019). Some challenges of the newly proposed FWIpeat for tropical peatlands stem from the uncertainty of the parameterisations used in PEATCLSM. One of these leads to the difficulty of PEATCLSM to accurately estimate the timing of capillary disconnection under drained conditions in peatlands, as indicated by the deterioration of FWIpeat performance when, next to zbar, the dynamics of the surface moisture content is also used. Given that fire managers typically rely on individual moisture codes to assess different fire dangers, such as using DC to estimate smoke hazard and FFMC to gauge ignition potential in tropical peatlands (de Groot et al. 2007), this integrated approach may not align with the needs of operational fire management. However, the improvements presented in the present study show the need to develop new technologies incorporating state-of-the-art groundwater predictions for peatland fire danger estimates.
Given the challenges in modelling surface soil moisture in drained tropical peatlands, satellite-based estimates of surface soil moisture bear promising potential to improve FWIpeat over tropical peatlands, especially for modifying the near-surface FFMC and DMC moisture codes (Dadap et al. 2019; Mortelmans et al. 2024). The usefulness of satellite-derived soil moisture to explain fire activity has been extensively explored (e.g. Chaparro et al. 2016; Ambadan et al. 2020; Field 2020b; Dadap et al. 2022). The primary constraint on the operational use of satellite data lies in its trade-off between spatial and temporal resolution. Typically, satellite products either have a coarse spatial resolution and a high temporal resolution, or a fine spatial resolution and a low temporal resolution. For example, SMOS data have a spatial resolution of 43 km and a temporal resolution of 2.5–3 days (Kerr et al. 2010), and Sentinel-1 C-band data have a spatial resolution of 10 m and a temporal resolution of 12 days (6 days in constellation; Torres et al. 2012) and optical-based retrievals (Burdun et al. 2020a, 2020b; Burdun et al. 2023) typically have a fine resolution but can be hampered by clouds. Furthermore, soil moisture retrieval products over tropical peat areas are only in their infancy and need more research. The assimilation and downscaling of coarse-scale satellite observations within land surface models like PEATCLSM may mitigate some limitations, as in Bechtold et al. (2020).
An operational data assimilation product, the SMAP Level-4 Soil Moisture Product (SMAP L4_SM; Reichle et al. 2019) has recently been updated to incorporate PEATCLSM over peatlands (SMAP_L4_SM v7; Reichle et al. 2023). With a latency time of only 2.5 days, this product holds potential for operational integration into an FWI forecast product. However, it is noteworthy that the current version of the data assimilation product only provides model-only data over coastal areas, because of challenges in assimilating soil moisture in locations with open water (Reichle et al. 2023). Another issue with the current version of SMAP L4_SM is that it globally applies the boreal version of PEATCLSM, as described by Bechtold et al. (2019). The adaptations for tropical peatlands made by Apers et al. (2022) are currently not yet included. As the current version of PEATCLSM in the SMAP L4_SM product was developed for northern, natural peatlands, the drained peatlands in SEA are poorly represented.
Lastly, in line with Mortelmans et al. (2024), we used a conservative approach of CDF-matching to include the PEATCLSM hydrological data in the FWI system. Here, we performed the CDF matching on a per-grid-cell basis, keeping only the temporal information of PEATCLSM and the resulting temporal differences between the drained and undrained peatlands, while keeping any spatial bias present in the FWI. The benefit of this approach is that it does not require the FWI system to be recalibrated and thus results in a user-friendly FWIpeat. Further studies could investigate the benefit of applying another approach, e.g. a different CDF-matching approach or the creation of a new index, to further optimise the inclusion of hydrological information in fire danger assessment.
Limitations of fire danger evaluation
The limitations of this study are partly similar to those discussed in Mortelmans et al. (2024). Firstly, the reference fire dataset used for evaluation has certain limitations. The GFA, being based on MODIS, does not account for fires ≪0.25 km2 (Andela et al. 2019), creating a bias towards larger fires and resulting in false alarms that may not actually be false. Furthermore, owing to the need for cloud-free conditions for a MODIS observation, the reported day of ignition in the GFA can be inaccurate, typically being too late (Veraverbeke et al. 2014; Andela et al. 2019), and possibly resulting in a mismatch between the timing of the ignition and the FWI. As the exact delay for each ignition is not known, this could not be taken into account in this study, even though it can have large implications for our results. To correct for this error, using additional observations from other remote sensing products could help. To provide more spatiotemporal detail, the MODIS observations used for the GFA could be combined with the fire product of the Visible Infrared Imaging Radiometer Suite (VIIRS; Schroeder et al. 2014). Including active fire detections from VIIRS could also help to identify smouldering of deep peat layers as opposed to surface fires, though these observations are only available during cloud free conditions.
Another limitation of our study is the classification of peatlands into drained and undrained based on land use. This differentiation is uncertain owing to variations in drainage density, with reliance solely on land use classes deemed insufficient to determine drainage levels (Dadap et al. 2021). As a consequence, we may have for part of the study domain used the wrong version of peatland-specific hydrological model simulations for the adaptation of the FWI. As we considered only ‘pristine peat swamp forests’ as undrained, while considering all other classes such as ‘secondary forest’ or ‘fern/low shrub’ as drained, we may have overestimated the area of drained peatlands, although this would have limited effect given that most remaining ‘pristine’ forested peatlands in SEA have been impacted indirectly by land use change and drainage in the surrounding landscape (Evans et al. 2019; Dadap et al. 2021).
Furthermore, it is crucial to acknowledge that the evaluation of the FWI against active fires goes beyond the intended use of the FWI, in that a high FWI does not necessarily indicate the occurrence of a fire, nor does a low FWI value guarantee the absence of a fire. However, we considered the occurrence of a fire in the GFA having a minimum size of 0.25 km2 as an indication of high FWI.
Conclusion
Although the Canadian Fire Weather Index (FWI) system is widely used globally (Di Giuseppe et al. 2021), its original development for boreal forests (Van Wagner 1987) raises concerns about its applicability in diverse fire environments, including peatlands, whereas the Drought Code (DC) has been known to show a good relationship with fire occurrence in peatlands (Waddington et al. 2012). Here, we extended previous research of Mortelmans et al. (2024) by evaluating the impact of incorporating peatland-specific hydrological information into the FWI system for tropical peatlands in southeast Asia. We assessed the performance of the newly developed FWIpeat compared with the original FWI (FWIref) and the original DC in explaining active fire occurrence.
Our findings demonstrate that, for undrained peatlands, FWIpeat explains fire occurrence better than FWIref but DC is superior. Over drained peatlands, DC and FWIpeat show a similar performance, both outperforming FWIref. The better performance of DC over undrained peatlands may be related to the various degrees of disturbance at the edge of drained peatlands. Most fires that occurred on peatlands classified as ‘undrained’ were located at the edges of these peatlands, where the effect of neighbouring drainage practices may still affect water levels. In these cases, DC may be a more suitable predictor than the hydrological estimates from a model that assumes an undrained peatland.
The main improvements with both DC and FWIpeat are seen in the lower values, whereas FWIref is capable of capturing the (extremely) high fire danger conditions. This explains, in part, why the improvements shown here for tropical peatlands are smaller than the reported improvements for boreal peatlands (Mortelmans et al. 2024). Other factors explaining these smaller improvements are the different main ignition sources (human vs lightning), and the lower accuracy of the hydrological estimates, which in this study came from model-only runs owing to the lack of a suitable peatland-specific data assimilation product over the tropical peatlands of peninsular Malaysia, Sumatra, and Borneo.
Overall, the findings of this study highlight the importance of giving increased weight to information about moisture content of the deeper soil layers when assessing fire danger over tropical peatlands. Further research on refining this input to the FWI system for different fire regimes is needed to provide a new framework for operational users.
Data availability
Land use data used in this study area are available from Miettinen et al. (2016). The MERRA-2 data (Gelaro et al. 2017) were obtained from https://goldsmr4.gesdisc.eosdis.nasa.gov/data/MERRA2/. In order to access the data, an account at https://urs.earthdata.nasa.gov/home (last access: 22 December 2022) is needed. The GFWED code used for the FWI calculations can be downloaded from https://portal.nccs.nasa.gov/datashare/GlobalFWI/v2.0/20201013.GFWEDCode.tar.gz (Field et al. 2015). The GFA data used for the evaluation were downloaded from 10.3334/ORNLDAAC/1642 (Andela et al. 2019). The CDF matching is done using the Python package pytesmo available at 10.5281/zenodo.8338451 (Paulik et al. 2023).
Declaration of funding
J. Mortelmans thanks the Research Foundation – Flanders (F.W.O.) for funding this research (FWO.G095720N). The computer resources and services used in this work were provided by the High Performance Computing system of the Vlaams Supercomputer Center, funded by F.W.O. and the Flemish Government.
Acknowledgements
We acknowledge the contribution of Tim Kerremans to this study through an initial analysis of the datasets used.
References
Abatzoglou JT, Williams AP, Boschetti L, Zubkova M, Kolden CA (2018) Global patterns of interannual climate–fire relationships. Global Change Biology 24(11), 5164-5175.
| Crossref | Google Scholar | PubMed |
Ambadan JT, Oja M, Gedalof Z, Berg AA (2020) Satellite-observed soil moisture as an indicator of wildfire risk. Remote Sensing 12(10), 1543.
| Crossref | Google Scholar |
Andela N, Morton DC, Giglio L, Paugam R, Chen Y, Hantson S, Van Der Werf GR, Anderson JT (2019) The Global Fire Atlas of individual fire size, duration, speed and direction. Earth System Science Data 11(2), 529-552.
| Crossref | Google Scholar |
Apers S, De Lannoy GJM, Baird AJ, Cobb AR, Dargie GC, del Aguila Pasquel J, Gruber A, Hastie A, Hidayat H, Hirano T, Hoyt AM, Jovani-Sancho AJ, Katimon A, Kurnain A, Koster RD, Lampela M, Mahanama SPP, Melling L, Page SE, et al. (2022) Tropical peatland hydrology simulated with a global land surface model. Journal of Advances in Modeling Earth Systems 14(3), e2021MS002784.
| Crossref | Google Scholar | PubMed |
Bechtold M, De Lannoy GJM, Koster RD, Reichle RH, Mahanama SP, Bleuten W, Bourgault MA, Brümmer C, Burdun I, Desai AR, Devito K, Grünwald T, Grygoruk M, Humphreys ER, Klatt J, Kurbatova J, Lohila A, Munir TM, Nilsson MB, et al. (2019) PEAT-CLSM: a specific treatment of peatland hydrology in the NASA Catchment Land Surface Model. Journal of Advances in Modeling Earth Systems 11(7), 2130-2162.
| Crossref | Google Scholar | PubMed |
Bechtold M, De Lannoy GJM, Reichle RH, Roose D, Balliston N, Burdun I, Devito K, Kurbatova J, Strack M, Zarov EA (2020) Improved groundwater table and L-band brightness temperature estimates for northern hemisphere peatlands using new model physics and SMOS observations in a global data assimilation framework. Remote Sensing of Environment 246, 111805.
| Crossref | Google Scholar |
BMKG (2024) Sistem Peringatan Kebakaran Hutan Dan Lahan. Available at https://www.bmkg.go.id/cuaca/kebakaran-hutan.bmkg?index=fwi&wil=indonesia&day=obs [in Indonesian]
Burdun I, Bechtold M, Sagris V, Komisarenko V, De Lannoy G, Mander Ü (2020a) A comparison of three trapezoid models using optical and thermal satellite imagery for water table depth monitoring in Estonian bogs. Remote Sensing 12(12), 1980.
| Crossref | Google Scholar |
Burdun I, Bechtold M, Sagris V, Lohila A, Humphreys E, Desai AR, Nilsson MB, De Lannoy G, Mander Ü (2020b) Satellite determination of peatland water table temporal dynamics by localizing representative pixels of a SWIR-based moisture index. Remote Sensing 12(18), 2936.
| Crossref | Google Scholar |
Burdun I, Bechtold M, Aurela M, De Lannoy G, Desai AR, Humphreys E, Kareksela S, Komisarenko V, Liimatainen M, Marttila H, Minkkinen K, Nilsson MB, Ojanen P, Salko SS, Tuittila ES, Uuemaa E, Rautiainen M (2023) Hidden becomes clear: optical remote sensing of vegetation reveals water table dynamics in northern peatlands. Remote Sensing of Environment 296, 113736.
| Crossref | Google Scholar |
Chaparro D, Piles M, Vall-llossera M (2016) Remotely sensed soil moisture as a key variable in wildfires prevention services: towards new prediction tools using SMOS and SMAP data. In ‘Satellite soil moisture retrieval: techniques and applications’. (Eds Prashant K. Srivastava, George P. Petropoulos, Yann H. Kerr) pp. 249–269. (Elsevier Inc.) 10.1016/B978-0-12-803388-3.00013-9
Chen Y, Hall J, Van Wees D, Andela N, Hantson S, Giglio L, Van Der Werf GR, Morton DC, Randerson JT (2023) Multi-decadal trends and variability in burned area from the fifth version of the Global Fire Emissions Database (GFED5). Earth System Science Data 15(11), 5227-5259.
| Crossref | Google Scholar |
Clark MP, Fan Y, Lawrence DM, Adam JC, Bolster D, Gochis DJ, Hooper RP, Kumar M, Leung LR, Mackay DS, Maxwell RM, Shen C, Swenson SC, Zeng X (2015) Improving the representation of hydrologic processes in Earth System Models. Water Resources Research 51(8), 5929-5956.
| Crossref | Google Scholar |
Couwenberg J, Dommain R, Joosten H (2010) Greenhouse gas fluxes from tropical peatlands in south‐east Asia. Global Change Biology 16(6), 1715-1732.
| Crossref | Google Scholar |
Crippa P, Castruccio S, Archer-Nicholls S, Lebron GB, Kuwata M, Thota A, Sumin S, Butt E, Wiedinmyer C, Spracklen DV (2016) Population exposure to hazardous air quality due to the 2015 fires in equatorial Asia. Scientific Reports 6, 37074.
| Crossref | Google Scholar | PubMed |
Dadap NC, Cobb AR, Hoyt AM, Harvey CF, Konings AG (2019) Satellite soil moisture observations predict burned area in southeast Asian peatlands. Environmental Research Letters 14(9), 094014.
| Crossref | Google Scholar |
Dadap NC, Hoyt AM, Cobb AR, Oner D, Kozinski M, Fua PV, Rao K, Harvey CF, Konings AG (2021) Drainage canals in southeast Asian peatlands increase carbon emissions. AGU Advances 2(1), e2020AV000321.
| Crossref | Google Scholar |
Dadap NC, Cobb AR, Hoyt AM, Harvey CF, Feldman AF, Im ES, Konings AG (2022) Climate change-induced peatland drying in southeast Asia. Environmental Research Letters 17(7), 074026.
| Crossref | Google Scholar |
Davies GM, Gray A, Rein G, Legg CJ (2013) Peat consumption and carbon loss due to smouldering wildfire in a temperate peatland. Forest Ecology and Management 308, 169-177.
| Crossref | Google Scholar |
de Groot WJ, Wotton BM, Flannigan MD (2015) Wildland fire danger rating and early warning systems. In ‘Wildfire hazards, risks, and disasters’. (Eds JF Shroder, D Paton) pp. 207–228. (Elsevier Inc). 10.1016/B978-0-12-410434-1.00011-7
de Groot WJD, Field RD, Brady MA, Roswintiarti O, Mohamad M (2007) Development of the Indonesian and Malaysian fire danger rating systems. Mitigation and Adaptation Strategies for Global Change 12(1), 165-180.
| Crossref | Google Scholar |
De Lannoy GJM, Reichle RH (2016) Global assimilation of multiangle and multipolarization SMOS brightness temperature observations into the GEOS-5 catchment land surface model for soil moisture estimation. Journal of Hydrometeorology 17(2), 669-691.
| Crossref | Google Scholar |
Di Giuseppe F, Vitolo C, Krzeminski B, Barnard C, MacIel P, San-Miguel J (2020) Fire Weather Index: the skill provided by the European Centre for Medium-Range Weather Forecasts ensemble prediction system. Natural Hazards and Earth System Sciences 20(8), 2365-2378.
| Crossref | Google Scholar |
Di Giuseppe F, Benedetti A, Coughlan R, Vitolo C, Vuckovic M (2021) A global bottom-up approach to estimate fuel consumed by fires using above ground biomass observations. Geophysical Research Letters 48(21), e2021GL095452.
| Crossref | Google Scholar |
Dommain R, Couwenberg J, Glaser PH, Joosten H, Suryadiputra INN (2014) Carbon storage and release in Indonesian peatlands since the last deglaciation. Quaternary Science Reviews 97, 1-32.
| Crossref | Google Scholar |
Dymond CC, Field RD, Roswintiarti O, Guswanto (2005) Using satellite fire detection to calibrate components of the fire weather index system in Malaysia and Indonesia. Environmental Management 35(4), 426-440.
| Crossref | Google Scholar | PubMed |
Entekhabi D, Njoku EG, O’Neill PE, Kellogg KH, Crow WT, Edelstein WN, Entin JK, Goodman SD, Jackson TJ, Johnson J, Kimball J, Piepmeier JR, Koster RD, Martin N, McDonald KC, Moghaddam M, Moran S, Reichle R, Shi JC, et al. (2010) The soil moisture active passive (SMAP) mission. Proceedings of the IEEE 98(5), 704-716.
| Crossref | Google Scholar |
Evans CD, Williamson JM, Kacaribu F, Irawan D, Suardiwerianto Y, Hidayat MF, Laurén A, Page SE (2019) Rates and spatial variability of peat subsidence in acacia plantation and forest landscapes in Sumatra, Indonesia. Geoderma 338, 410-421.
| Crossref | Google Scholar |
Fernandes K, Verchot L, Baethgen W, Gutierrez-Velez V, Pinedo-Vasquez M, Martius C (2017) Heightened fire probability in Indonesia in non-drought conditions: the effect of increasing temperatures. Environmental Research Letters 12(5), 054002.
| Crossref | Google Scholar |
Field RD, Spessa AC, Aziz NA, Camia A, Cantin A, Carr R, de Groot WJ, Dowdy AJ, Flannigan MD, Manomaiphiboon K, Pappenberger F, Tanpipat V, Wang X (2015) Development of a Global Fire Weather Database. Natural Hazards and Earth System Sciences 15(6), 1407-1423.
| Crossref | Google Scholar |
Field RD (2020a) Evaluation of Global Fire Weather Database reanalysis and short-term forecast products. Natural Hazards and Earth System Sciences 20(4), 1123-1147.
| Crossref | Google Scholar |
Field RD (2020b). Using satellite estimates of precipitation for fire danger rating. In ‘Satellite precipitation measurement. Advances in Global Change Research. Vol. 69’. (Eds V Levizzani, C Kidd, D Kirschbaum, C Kummerow, K Nakamura, F Turk) pp. 1131–1154. (Springer: Cham, Switzerland) 10.1007/978-3-030-35798-6_33
Forkel M, Thonicke K, Beer C, Cramer W, Bartalev S, Schmullius C (2012) Extreme fire events are related to previous-year surface moisture conditions in permafrost-underlain larch forests of Siberia. Environmental Research Letters 7(4), 44021.
| Crossref | Google Scholar |
Gallego-Sala AV, Charman DJ, Brewer S, Page SE, Prentice IC, Friedlingstein P, Moreton S, Amesbury MJ, Beilman DW, Björck S, Blyakharchuk T, Bochicchio C, Booth RK, Bunbury J, Camill P, Carless D, Chimner RA, Clifford M, Cressey E, et al. (2018) Latitudinal limits to the predicted increase of the peatland carbon sink with warming. Nature Climate Change 8(10), 907-913.
| Crossref | Google Scholar |
Gelaro R, McCarty W, Suárez MJ, Todling R, Molod A, Takacs L, Randles C, Darmenov A, Bosilovich MG, Reichle R, Wargan K, Coy L, Cullather R, Draper C, Akella S, Buchard V, Conaty A, da Silva AM, Gu W, et al. (2017) The modern-era retrospective analysis for research and applications, version 2 (MERRA-2). Journal of Climate 30(14), 5419-5454.
| Crossref | Google Scholar | PubMed |
Giglio L, Boschetti L, Roy DP, Humber ML, Justice CO (2018) The Collection 6 MODIS burned area mapping algorithm and product. Remote Sensing of Environment 217, 72-85.
| Crossref | Google Scholar | PubMed |
Glauber AJ, Moyer S, Adriani M, Gunawan I (2016) The cost of fire: an economic analysis of Indonesia’s 2015 fire crisis. Indonesia Sustainable Landscapes Knowledge Note No. 1. World Bank, Jakarta. 10.1596/23840
Hantson S, Arneth A, Harrison SP, Kelley DI, Colin Prentice I, Rabin SS, Archibald S, Mouillot F, Arnold SR, Artaxo P, Bachelet D, Ciais P, Forrest M, Friedlingstein P, Hickler T, Kaplan JO, Kloster S, Knorr W, Lasslop G, et al. (2016) The status and challenge of global fire modelling. Biogeosciences 13(11), 3359-3375.
| Crossref | Google Scholar |
Holgate CM, van Dijk AIJM, Cary GJ, Yebra M (2017) Using alternative soil moisture estimates in the McArthur Forest Fire Danger Index. International Journal of Wildland Fire 26(9), 806.
| Crossref | Google Scholar |
Hooijer A, Page S, Jauhiainen J, Lee WA, Lu XX, Idris A, Anshari G (2012) Subsidence and carbon loss in drained tropical peatlands. Biogeosciences 9(3), 1053-1071.
| Crossref | Google Scholar |
Hu Y, Rein G (2022) Development of gas signatures of smouldering peat wildfire from emission factors. International Journal of Wildland Fire 31, 1014-1032.
| Crossref | Google Scholar |
Hu Y, Fernandez-Anez N, Smith TEL, Rein G (2018) Review of emissions from smouldering peat fires and their contribution to regional haze episodes. International Journal of Wildland Fire 27(5), 293-312.
| Crossref | Google Scholar |
Huang X, Rein G (2017) Downward spread of smouldering peat fire: the role of moisture, density and oxygen supply. International Journal of Wildland Fire 26(11), 907-918.
| Crossref | Google Scholar |
Huijnen V, Wooster MJ, Kaiser JW, Gaveau DLA, Flemming J, Parrington M, Inness A, Murdiyarso D, Main B, Van Weele M (2016) Fire carbon emissions over maritime southeast Asia in 2015 largest since 1997. Scientific Reports 6, 26886.
| Crossref | Google Scholar | PubMed |
Janssen TAJ, Jones MW, Finney D, van der Werf GR, van Wees D, Xu W, Veraverbeke S (2023) Extratropical forests increasingly at risk due to lightning fires. Nature Geoscience 16, 1136-1144.
| Crossref | Google Scholar |
Jauhiainen J, Hooijer A, Page SE (2012) Carbon dioxide emissions from an Acacia plantation on peatland in Sumatra, Indonesia. Biogeosciences 9(2), 617-630.
| Crossref | Google Scholar |
Jones MW, Abatzoglou JT, Veraverbeke S, Andela N, Lasslop G, Forkel M, Smith AJP, Burton C, Betts RA, van der Werf GR, Sitch S, Canadell JG, Santín C, Kolden C, Doerr SH, Le Quéré C (2022) Global and regional trends and drivers of fire under climate change. Reviews of Geophysics 60(3), e2020RG000726.
| Crossref | Google Scholar |
Kasischke ES, Turetsky MR (2006) Recent changes in the fire regime across the North American boreal region – Spatial and temporal patterns of burning across Canada and Alaska. Geophysical Research Letters 33(9), L09703.
| Crossref | Google Scholar |
Kasischke ES, Williams D, Barry D (2002) Analysis of the patterns of large fires in the boreal forest region of Alaska. International Journal of Wildland Fire 11(2), 131-144.
| Crossref | Google Scholar |
Kerr YH, Waldteufel P, Wigneron JP, Delwart S, Cabot F, Boutin J, Escorihuela MJ, Font J, Reul N, Gruhier C, Juglea SE, Drinkwater MR, Hahne A, Martin-Neira M, Mecklenburg S (2010) The SMOS L: new tool for monitoring key elements of the global water cycle. Proceedings of the IEEE 98(5), 666-687.
| Crossref | Google Scholar |
Koplitz SN, Mickley LJ, Marlier ME, Buonocore JJ, Kim PS, Liu T, Sulprizio MP, DeFries RS, Jacob DJ, Schwartz J, Pongsiri M, Myers SS (2016) Public health impacts of the severe haze in Equatorial Asia in September–October 2015: demonstration of a new framework for informing fire management strategies to reduce downwind smoke exposure. Environmental Research Letters 11(9), 094023.
| Crossref | Google Scholar |
Koster RD, Suarez MJ, Ducharne A, Stieglitz M, Kumar P (2000) A catchment-based approach to modeling land surface processes in a general circulation model 1. Model structure. Journal of Geophysical Research Atmospheres 105(D20), 24809-24822.
| Crossref | Google Scholar |
Krueger ES, Levi MR, Achieng KO, Bolten JD, Carlson JD, Coops NC, Holden ZA, Magi BI, Rigden AJ, Ochsner TE (2022) Using soil moisture information to better understand and predict wildfire danger: a review of recent developments and outstanding questions. International Journal of Wildland Fire 32(2), 111-132.
| Crossref | Google Scholar |
Leblon B, San-Miguel-Ayanz J, Bourgeau-Chavez L, Kong M (2016) 3-Remote Sensing of Wildfires. In ‘Land Surface Remote Sensing’. (Eds N Baghdadi, M Zribi) pp. 55–95. (Elsevier). 10.1016/B978-1-78548-105-5.50003-7
Leifeld J, Menichetti L (2018) The underappreciated potential of peatlands in global climate change mitigation strategies. Nature Communications 9(1), 1071.
| Crossref | Google Scholar | PubMed |
Le Page Y, Morton D, Bond-Lamberty B, Pereira JMC, Hurtt G (2015) HESFIRE: a global fire model to explore the role of anthropogenic and weather drivers. Biogeosciences 12(3), 887-903.
| Crossref | Google Scholar |
Loisel J, Gallego-Sala AV, Amesbury MJ, Magnan G, Anshari G, Beilman DW, Benavides JC, Blewett J, Camill P, Charman DJ, Chawchai S, Hedgpeth A, Kleinen T, Korhola A, Large D, Mansilla CA, Müller J, van Bellen S, West JB, et al. (2021) Expert assessment of future vulnerability of the global peatland carbon sink. Nature Climate Change 11(1), 70-77.
| Crossref | Google Scholar |
Matondang JS, Sanjaya H, Arifandri R (2021) Development of Google Earth Engine fire weather index calculator for Indonesian Fire Danger Rating System. IOP Conference Series: Earth and Environmental Science 936(1), 012040.
| Crossref | Google Scholar |
Mezbahuddin S, Nikonovas T, Spessa A, Grant RF, Imron MA, Doerr SH, Clay GD (2023) Accuracy of tropical peat and non-peat fire forecasts enhanced by simulating hydrology. Scientific Reports 13(1), 619.
| Crossref | Google Scholar | PubMed |
Miettinen J, Shi C, Liew SC (2016) Land cover distribution in the peatlands of peninsular Malaysia, Sumatra and Borneo in 2015 with changes since 1990. Global Ecology and Conservation 6, 67-78.
| Crossref | Google Scholar |
Mortelmans J, Felsberg A, De Lannoy GJM, Veraverbeke S, Field RD, Andela N, Bechtold M (2024) Improving the fire weather index system for peatlands using peat-specific hydrological input data. Natural Hazards and Earth System Sciences 24(2), 445-464.
| Crossref | Google Scholar |
Page S, Mishra S, Agus F, Anshari G, Dargie G, Evers S, Jauhiainen J, Jaya A, Jovani-Sancho AJ, Laurén A, Sjögersten S, Suspense IA, Wijedasa LS, Evans CD (2022) Anthropogenic impacts on lowland tropical peatland biogeochemistry. Nature Reviews Earth and Environment 3(7), 426-443.
| Crossref | Google Scholar |
Page SE, Siegert F, Rieley JO, Boehm HD, Jaya A, Limin S (2002) The amount of carbon released from peat and forest fires in Indonesia during 1997. Nature 420(6911), 61-65.
| Crossref | Google Scholar | PubMed |
Page SE, Rieley JO, Banks CJ (2011) Global and regional importance of the tropical peatland carbon pool. Global Change Biology 17(2), 798-818.
| Crossref | Google Scholar |
Paulik C, Preimesberger W, s-scherrer, pstradio, Hahn S, Baum D, Plocon A, Mistelbauer T, tracyscanlon, Schmitzer M, alegrub88, teije01 (2023) TUW-GEO/pytesmo: v0.16.0. Zenodo. 10.5281/zenodo.8338451
Pelletier N, Millard K, Darling S (2023) Wildfire likelihood in Canadian treed peatlands based on remote-sensing time-series of surface conditions. Remote Sensing of Environment 296, 113747.
| Crossref | Google Scholar |
Pettinari ML, Chuvieco E (2020) Fire danger observed from space. Surveys in Geophysics 41(6), 1437-1459.
| Crossref | Google Scholar |
Purnomo DMJ, Apers S, Bechtold M, Sofan P, Rein G (2023) KAPAS II: simulation of peatland wildfires with daily variations of peat moisture content. International Journal of Wildland Fire 32(6), 823-835.
| Crossref | Google Scholar |
Reichle RH, Liu Q, Koster RD, Crow WT, De Lannoy GJM, Kimball JS, Ardizzone JV, Bosch D, Colliander A, Cosh M, Kolassa J, Mahanama SP, Prueger J, Starks P, Walker JP (2019) Version 4 of the SMAP Level-4 soil moisture algorithm and data product. Journal of Advances in Modeling Earth Systems 11(10), 3106-3130.
| Crossref | Google Scholar |
Reichle RH, Liu Q, Ardizzone JV, Bechtold M, Crow WT, De Lannoy G, Kimball JS, Koster RD (2023) Soil Moisture Active Passive (SMAP) Project Assessment Report for Version 7 of the L4_SM Data Product. NASA Technical Report Series on Global Modeling and Data Assimilation, NASA/TM-2023-104606, Vol. 64. 87 p. (NASA, Goddard Space Flight Center, Greenbelt: Maryland, USA)
Rein G (2013). Smouldering fires and natural fuels. In ‘Fire phenomena and the Earth system: an interdisciplinary guide to fire science’. (Ed. CM Belcher) pp. 15–33. (John Wiley and Sons) 10.1002/9781118529539.ch2
Rein G, Cleaver N, Ashton C, Pironi P, Torero JL (2008) The severity of smouldering peat fires and damage to the forest soil. Catena 74(3), 304-309.
| Crossref | Google Scholar |
Schroeder W, Oliva P, Giglio L, Csiszar IA (2014) The New VIIRS 375m active fire detection data product: algorithm description and initial assessment. Remote Sensing of Environment 143, 85-96.
| Crossref | Google Scholar |
Taufik M, Torfs PJJF, Uijlenhoet R, Jones PD, Murdiyarso D, Van Lanen HAJ (2017) Amplification of wildfire area burnt by hydrological drought in the humid tropics. Nature Climate Change 7(6), 428-431.
| Crossref | Google Scholar |
Taylor SW, Alexander ME (2006) Science, technology, and human factors in fire danger rating: the Canadian experience. International Journal of Wildland Fire 15(1), 121-135.
| Crossref | Google Scholar |
Torres R, Snoeij P, Geudtner D, Bibby D, Davidson M, Attema E, Potin P, Rommen BÖ, Floury N, Brown M, Traver IN, Deghaye P, Duesmann B, Rosich B, Miranda N, Bruno C, L’Abbate M, Croci R, Pietropaolo A, et al. (2012) GMES Sentinel-1 mission. Remote Sensing of Environment 120, 9-24.
| Crossref | Google Scholar |
Turetsky MR, Benscoter B, Page S, Rein G, Van Der Werf GR, Watts A (2015) Global vulnerability of peatlands to fire and carbon loss. Nature Geoscience 8(1), 11-14.
| Crossref | Google Scholar |
Veraverbeke S, Sedano F, Hook SJ, Randerson JT, Jin Y, Rogers BM (2014) Mapping the daily progression of large wildland fires using MODIS active fire data. International Journal of Wildland Fire 23(5), 655-667.
| Crossref | Google Scholar |
Vereecken H, Weihermüller L, Assouline S, Šimůnek J, Verhoef A, Herbst M, Archer N, Mohanty B, Montzka C, Vanderborght J, Balsamo G, Bechtold M, Boone A, Chadburn S, Cuntz M, Decharme B, Ducharne A, Ek M, Garrigues S, et al. (2019) Infiltration from the Pedon to Global Grid Scales: an overview and outlook for land surface modeling. Vadose Zone Journal 18(1), 1-53.
| Crossref | Google Scholar |
Waddington JM, Thompson DK, Wotton M, Quinton WL, Flannigan MD, Benscoter BW, Baisley SA, Turetsky MR (2012) Examining the utility of the Canadian forest fire weather index system in boreal peatlands. Canadian Journal of Forest Research 42(1), 47-58.
| Crossref | Google Scholar |