An offline coupling of fire spread models to simulate the 2021 Marshall Fire
Fernando Szasdi-Bardales


A
B
C
Abstract
Existing fire spread models focus exclusively on wildland or urban fire simulation.
This study aims at an offline coupling of two fire spread models to enable a continuous simulation of a wildfire incident transitioning from wildland into wildland–urban interface (WUI) communities, evaluate the effects of wind input on simulation results and study the influence of building types on fire spread patterns.
The selected models are WRF-Fire, a wildland fire behaviour simulation platform, and SWUIFT, a model for fire spread inside the WUI. The 2021 Marshall Fire serves as the case study. A map of the fire’s timeline and location is generated using public information. Three simulation scenarios are analysed to study the effects of wind input resolution and building type on the predicted fire spread and damage.
The most accurate results are obtained using a high-resolution wind input and when incorporating different building types.
The offline coupling of models provides a reliable solution for fire spread simulation. Fire-resistant buildings likely helped limit community fire spread during the Marshall Fire.
The research is a first step toward developing simulation capabilities to predict the spread of wildfires within the wildland, WUI and urban environments.
Keywords: fire, Marshall Fire, modelling, offline coupling, SWUIFT, wildfire simulation, wildland–urban interface, WRF-Fire.
Introduction
Since 2010, more than 85,000 structures have been destroyed by wildfires in the United States (Headwaters Economics 2022). This unprecedented level of destruction has been driven by an increasing trend in the frequency of large and destructive wildfires (Dennison et al. 2014). Consequently, the costs of suppression activities, damaged property, loss of natural resources and impact on people’s quality of life have also increased (Moritz et al. 2014).
Mitigation measures aimed at reducing home ignition susceptibility can reduce the potential impacts of wildfires in wildland–urban interface (WUI) communities (Calkin et al. 2014). Observations made from experiments and past wildfire incidents have led to the recommendation of mitigation measures such as vegetation management, fire-resistant construction and accessibility requirements (Federal Emergency Management Agency (FEMA) 2008; International Code Council (ICC) 2017, 2019; National Fire Protection Association (NFPA) 2017). However, implementing mitigation measures in WUI areas has been challenging owing to economic costs and residents’ perceptions of wildfire risk (Martin et al. 2009; Brenkert-Smith et al. 2012; Meldrum et al. 2014; Clifford 2016). Meanwhile, numerical modelling using validated tools can be used to quantify wildfire damage and assess risk. In cases where risk is beyond acceptable thresholds, the effectiveness of different mitigation measures can be evaluated using the models. Such results can be used to guide an efficient allocation of resources and communicate the risk with the public. It is emphasised that the models used in risk assessment procedures should be validated extensively using high-quality experimental datasets or field data, and their limitations should be identified and understood to ensure that evaluations and recommendations are realistic and reliable.
Existing models focus on the simulation of fire spread within wildland (Linn 1997; Finney 1998; Mell et al. 2007; Finney et al. 2011; Coen et al. 2013; Lautenberger 2013) or urban areas (Masoudvaziri et al. 2021; Mastorakos et al. 2022), but they have a limited capacity to simulate fires that transition from one setting to the other. This limitation arises from fundamental differences in their inputs and the mechanics of their modelling procedures. Some researchers have adapted cellular automata models to simulate fire spread across wildland and WUI, but these models often involve significant simplifications or lack sufficient validation and generalisability (Spyratos et al. 2007; Jiang et al. 2021). Connecting existing validated wildland and urban fire models could make simulation of fire spread across different fuel types possible.
This paper documents, for the first time, a continuous large-scale simulation of fire progression from the wildland into nearby WUI communities, achieved through the offline coupling of wildland and urban fire spread models with an explicit physical representation of fire spread modes, making the approach suitable for practical real-world applications. The offline coupling of the models utilises the capabilities of existing modelling platforms to facilitate practical real-world applications. The two models are the Weather Research and Forecasting model with a fire behaviour model (WRF-Fire) (Coen et al. 2013), a semi-empirical surface fire simulation platform with application in the wildland, and the streamlined wildland–urban interface fire tracing (SWUIFT) model (Masoudvaziri et al. 2021), a semi-empirical model for the prediction of fire spread inside WUI communities.
The paper investigates the effect of wind input resolution and the incorporation of buildings with fire-resistant characteristics on the simulated fire spread patterns inside communities. The case study is the 2021 Marshall Fire, an incident that destroyed over 1000 structures in Boulder County, Colorado. Up to this date, the Marshall Fire is the most destructive wildfire in the history of Colorado. Existing work has been limited to the analysis of the fire within the wildlands (Juliano et al. 2023) or vulnerability assessment of the communities without explicit simulation of the fire spread (Chulahwat and Mahmoud 2024). The present paper introduces new maps of the fire spread timeline based on a comprehensive review of available information on the Marshall Fire. The generated maps are used to assess and discuss the simulation results.
Background
WRF-Fire
WRF-Fire (Coen et al. 2013) couples the Advanced Research WRF (ARW) model (Skamarock and Klemp 2008; Skamarock et al. 2021), which is a widely used numerical weather prediction (NWP) model designed for both research and operational applications, with a wildland fire behaviour model based on the Coupled Atmosphere–Wildland Fire Environment Model (CAWFE) (Clark et al. 1996a, 1996b; Coen 2013). WRF-ARW is capable of multiscale atmospheric simulations ranging from mesoscale to microscale. This multiscale simulation capability together with other capabilities of WRF (e.g. detailed land surface parameterisation, radiation, microphysics schemes) can enable WRF to provide localised weather predictions with higher spatial and temporal resolution than the currently available weather data for fire simulation (Shamsaei et al. 2023).
Using fields resolved by WRF’s atmospheric grid, the fire behaviour model simulates surface fire spread with Rothermel’s rate of spread (ROS) model (Rothermel 1972) and a level-set algorithm to track the fire on the surface (Munoz-Esparza et al. 2018). After propagating the fire at each timestep, the fire behaviour model calculates fire-generated sensible and latent heat fluxes based on the combusted fuel. The calculated values are sent back to WRF’s atmospheric grid as temperature and moisture fluxes to simulate the effects of fire heat on atmospheric circulation, allowing the fire to ‘create its own weather’. WRF-Fire has been used for both prescribed burns and landscape-scale historic fires in various studies in the literature (Thomas et al. 2017; DeCastro et al. 2022; Juliano et al. 2023; Shamsaei et al. 2023). WRF-Fire is validated for a number of prescribed burns and wildland fires, especially when fuel characteristics (mass loading, moisture content, etc.), atmospheric winds and topography are quite homogeneous. When any one of these factors becomes increasingly heterogeneous, additional errors may arise in WRF-Fire’s spread prediction. It is important to note that neither canopy fire spread nor fire suppression is presently represented in the model.
SWUIFT
The SWUIFT model (Masoudvaziri et al. 2021) simulates fire spread within WUI communities by simplifying the domain of study into a raster with three different fuel categories: structure, vegetation, or non-combustible. Starting from initially known fire positions, SWUIFT incorporates thermal radiation and fire spotting mechanisms to spread the fire across the domain at regular time intervals (e.g. 5-min increments). The model assumes structure cells to be independent compartments with a height of 3 m and a timber frame construction. The structure cells can ignite by thermal radiation if they are exposed to a radiant heat flux above 14 kW m–2, or by fire spotting if more than 24 g of landed firebrands accumulate within a 78.53 cm2 circle. Once a structure cell’s fire develops, it emits thermal radiation and firebrands. However, vegetation cells ignite if they receive more than 224 g of firebrands, and they can only propagate the fire via fire spotting. The simulation process that has been described is represented in Fig. 1, and further details can be found in Masoudvaziri et al. (2021).
SWUIFT has been used to analyse historical WUI fires such as the 2007 Witch and Guejito Fires (Masoudvaziri et al. 2021), the 2017 Tubbs Fire (Masoudvaziri et al. 2021, 2023), the 2023 Lahaina Fire (Juliano et al. 2024) and the 2018 Camp Fire (Szasdi-Bardales et al. 2024). In all the cases, the simulations provide reasonable estimations for the ROS and the expected location of damaged structures inside the analysed communities. The analysis in the first three cases focused only on fire spread within WUI, whereas the Camp Fire study proposed and evaluated a methodology for smooth fire transition between wildland and community settings where weather radar data were used to track fire propagation in the wildland. Since the analysis of these fires, SWUIFT’s code has not undergone changes in its main fire spread mechanics (i.e. ignition thresholds, structure fire development, or the attributes of the different modes of fire propagation). Nevertheless, the larger scale of recent incidents and the availability of new data and tools have resulted in code modifications that give SWUIFT the capability to process new input layers, including boundaries for domains with different simulation rules, gridded wind velocity and dynamic fire position inputs that can be independently updated at each timestep. These modifications enable SWUIFT to integrate with fire position observations or the output of wildland fire spread models.
The current study employs the methodology that was recently developed for the Camp Fire study, although in this case, WRF-Fire simulates the fire spread within the wildland, making it the first documented case in which the capabilities of fire spread models are complemented to generate a complete simulation of a wildland–WUI fire. Specific details of how the new methodology is applied in this study are provided in the Simulation setup section.
Marshall Fire
On 30 December 2021, a destructive wildfire impacted Boulder County, Colorado. The fire caused 2 deaths, destroyed 1084 homes and 7 commercial structures, and damaged 149 homes and 30 commercial structures (Boulder County 2022a). The Marshall Fire was first reported ~11:05 am local time (UTC–07:00) burning in the vicinity of Marshall Road in unincorporated Boulder County (Boulder County 2022b). The fire rapidly spread eastward through dry grass towards the town of Superior and the city of Louisville, where most of the structural damage was concentrated (Colorado Division of Fire Prevention and Control (DFPC) 2022).
A set of fire weather conditions that facilitated Marshall Fire ignition and rapid expansion were in place on the day of the incident (Fovell et al. 2022; Benjamin et al. 2023; Juliano et al. 2023). The National Weather Service (NWS) (2022) identified strong winds and dry conditions as the two factors that played the most critical role in the disaster. On the morning of the incident, wind gusts up to 45 m s–1 (100 miles h–1) were observed in the area. Additionally, an atypically dry fall (autumn) and early winter of 2021 resulted in the accumulation of dry vegetation, mainly grass and shrubs, that fuelled the expansion of the fire through the wildland. Early fire suppression efforts were overwhelmed by strong winds, which not only accelerated fire spread, but also generated conditions that limited emergency responders’ visibility and compromised their safety. Evacuation orders were put in place to move the residents of Louisville and Superior away from the path of approaching fire (Colorado Division of Fire Prevention and Control (DFPC) 2022; Boulder County 2022b). The fire first affected structures in the Marshall area, but later spread east and reached the commercial and residential structures on the west side of US Highway 36 (US 36) in Superior. The fire jumped over US 36 and continued to move towards Louisville, suggesting that spotting played an important role in fire propagation during the incident.
Simulation setup
An offline coupling implies that the flow of information between coupled components flows in a single direction. Thus, in this study, the integration of WRF-Fire and SWUIFT is classified as an offline coupling because WRF-Fire feeds inputs to SWUIFT, but SWUIFT does not provide feedback to WRF-Fire. WRF-Fire provides SWUIFT with an updated wildland fire position throughout the simulation and, in two of the evaluated scenarios, provides a surface wind field that is updated at each timestep. This section provides details about how, where and when the connection between the two models is set up.
WRF-Fire setup
The WRF-Fire’s model setup used in this study is identical to the one presented in a recent paper by Juliano et al. (2023), in which the wildland spread and meteorological drivers of the 2021 Marshall Fire were investigated. The model utilises two one-way nested domains (Fig. 2) centred at (39.944°N, 105.135°W), with grid sizes of 1 km and 111.11 m for the parent and child domains, respectively, together with 45 vertical levels, which are stretched with altitude. The parent domain covers most of Colorado and southern and western parts of Wyoming and Nebraska, whereas the child domain covers a smaller area limited to Taylor Mountain, CO, on its northwest corner and southern Aurora, CO, on its southeast corner. The initial and lateral boundary conditions for these domains are obtained from the High-Resolution Rapid Refresh (HRRR) model (Benjamin et al. 2016), with the lateral boundary condition provided at an hourly interval. In addition to the atmospheric domains, a fire mesh with grid resolution of 27.78 m is used inside the child domain, which is required for WRF-Fire. The fuel data for this domain are obtained from Landscape Fire and Resource Management Planning Tools (LANDFIRE) (2020), which categorise the fuels based on Anderson’s 13 fuel types, but lack proper representation of WUI fuel categories (Juliano et al. 2023). To simulate the Marshal Fire spread, two ignitions are initiated in coordinates (39.956127°N, 105.230487°W) and (39.951209°N, 105.231441°W) at 11:08 am and 12:00 pm local time (UTC–07:00), respectively. The fire spread through the wildland is simulated in this study from 11:08 am to 9:00 pm local time on 30 December 2021. Further details on the WRF-Fire model setup can be found in Juliano et al. (2023).
SWUIFT setup
The spatial domain for SWUIFT simulations (Fig. 3) is defined as a rectangular area of 158 km2 limited by the following coordinates: 40.004° (to the north), 39.898° (to the south), −105.105° (to the east) and −105.263° (to the west). This domain was selected to fully cover the observed fire perimeter, the city of Louisville and the town of Superior. The spatial domain is divided into a raster of 13.89 by 13.89 m cells, with cells categorised as structure, vegetation, or non-combustible. These dimensions are compatible with WRF-Fire’s child domain’s and fire mesh’s grids (i.e. 1/8 and 1/2 of their cell size, respectively). The vegetation landcover information is obtained from the same LANDFIRE fuel maps used in WRF-Fire domains (Landscape Fire and Resource Management Planning Tools (LANDFIRE) 2020), and the location of structures is obtained from Microsoft’s US Building Footprints database (Microsoft 2022). The temporal domain comprises the period between 11:10 am and 9:00 pm local time on 30 December 2021, with the first fire location input taken from WRF-Fire at 11:10 am. The simulation is run at 5-min timesteps.
For the coupled simulations (community fire spread), three scenarios are analysed:
LRW-AS: in this scenario the wind input for SWUIFT is obtained from the Sugarloaf Remote Automatic Weather Station (RAWS) (Western Regional Climate Center (WRCC) 2021). This input provides single average wind and gust speeds for the full spatial domain at 1-h intervals. For ease of reference, this wind input is referred to as ‘low-resolution wind input’ throughout this study. All structures within the spatial domain are included in the simulation and treated as timber-frame residential structures (the default structure type in SWUIFT) for ignition criteria and fire development purposes (Masoudvaziri et al. 2021).
HRW-AS: this scenario uses as an input a surface wind field generated by WRF. This wind input has higher spatial and temporal resolutions (111.11 × 111.11 m grid size updated for each 5-min timestep) compared with the wind input in LRW-AS. For ease of reference, this wind input is referred to as ‘high-resolution wind input’ throughout this study. Like in LRW-AS, all structures are included as timber-frame residential structures.
HRW-NFRS: this scenario uses the same wind input as HRW-AS. Additionally, all structures that cannot be classified as residential and wood light-frame structures according to sections 4.1 and 4.2.1 of the Hazus Inventory Technical Manual (Federal Emergency Management Agency (FEMA) 2022) are removed from the spatial domain and replaced with non-combustible cells. Examples of different structure types are provided in Fig. 4. The building categorisation is manually performed through the analysis of satellite and Google Street View images (Google Maps 2019). For simplification, all removed structures are referred to as ‘fire-resistant structures’ throughout this study. Non-residential structures in the area are classified as non-combustible because they usually have characteristics (Fig. 4b) that reduce their vulnerability to ignition, such as fire-resistant materials, sufficient defensible space and active fire protection (e.g. fire sprinkler systems). This approach provides a reasonable approximation to capture the contribution of structures with improved fire-resistant features in reducing the ROS inside communities. Developing capabilities to incorporate hardened structures (i.e. differentiating the ignition and fire development criteria for different structure categories) into the simulation framework is outside the scope of this study.
(a) Wood light-frame residential structures usually have combustible exterior materials and small defensible space around the building, and may not incorporate active fire protection. (b) Non-residential structures usually have fire-resistant exterior materials, are surrounded by large defensible spaces due to parking lots and sidewalks, and are more likely to have active fire protection (e.g. fire sprinkler systems) in place.
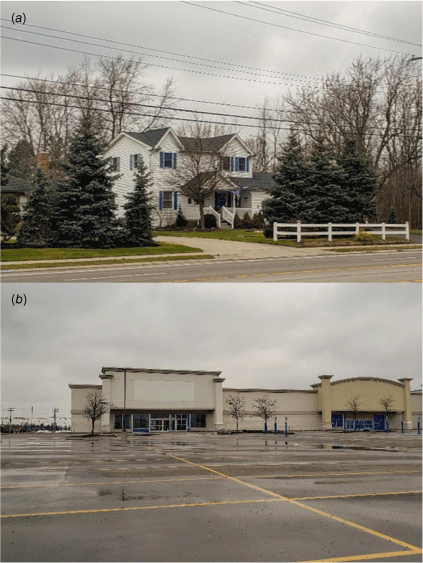
Post-incident damage reports provide evidence of the improved performance of non-residential structures, as the proportion of destroyed versus damaged commercial buildings (0.2:1) is significantly lower in comparison with that of residential buildings (7.3:1) (Colorado Division of Fire Prevention and Control (DFPC) 2022; Boulder County 2022a). Fig. 5 shows the location of structures removed for the third simulation scenario, and Table 1 summarises the differences between the three coupled simulation cases that are analysed in this study.
Structures removed from the spatial domain (red) and replaced with non-combustible cells for simulation scenario HRW-NFRS.
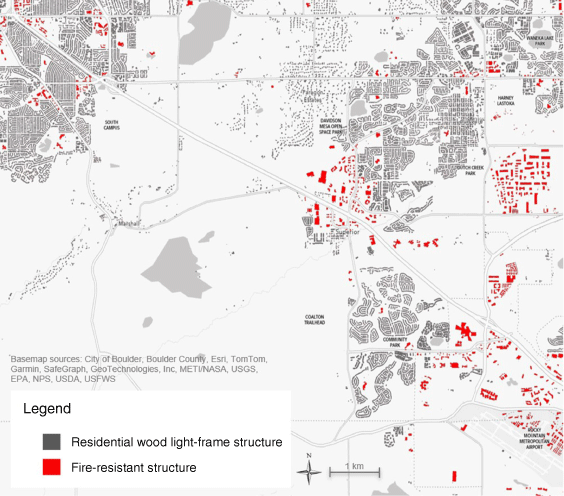
Scenario | Wind input source | Wind input resolution | Structures removed from the simulation | |
---|---|---|---|---|
LRW-AS | RAWS | Low: single wind velocity updated every hour | None | |
HRW-AS | WRF | High: 111.11 × 111.11 m grid updated every 15 min | None | |
HRW-NFRS | WRF | High: 111.11 × 111.11 m grid updated every 15 min | Those assumed to be fire-resistant: non-residential occupancy and/or building types other than wood light-frame |
Offline coupling of models
To enable a smooth fire line transition between the wildland and communities, the ‘three-domain solution’ proposed by Szasdi-Bardales et al. (2024) is implemented. This procedure divides SWUIFT’s spatial domain into three different regions with specific rules. As shown in Fig. 6, fire spread in the ‘wildland domain’ is exclusively dictated by WRF-Fire. In the ‘transition domain’, WRF-Fire simulates the fire spread, while SWUIFT’s fire spotting module is activated to track the embers that could potentially land and cause ignitions inside the communities. Finally, fire spread inside the ‘community domain’ is exclusively simulated by SWUIFT. In this study, SWUIFT and WRF-Fire run asynchronously on different platforms. The inter-model communication occurs when the fire position output and the gridded wind velocity from WRF inform the SWUIFT simulations. These outputs are represented in a higher resolution format in SWUIFT because it operates at higher spatial and temporal resolutions than WRF-Fire (Fig. 6).
Three-domain solution applied to an offline coupling of WRF-Fire and SWUIFT (NWSC: NCAR-Wyoming Supercomputer Center; FBFM: Fire Behavior Fuel Models; HRRR: High-Resolution Rapid Refresh model; MS: Microsoft).
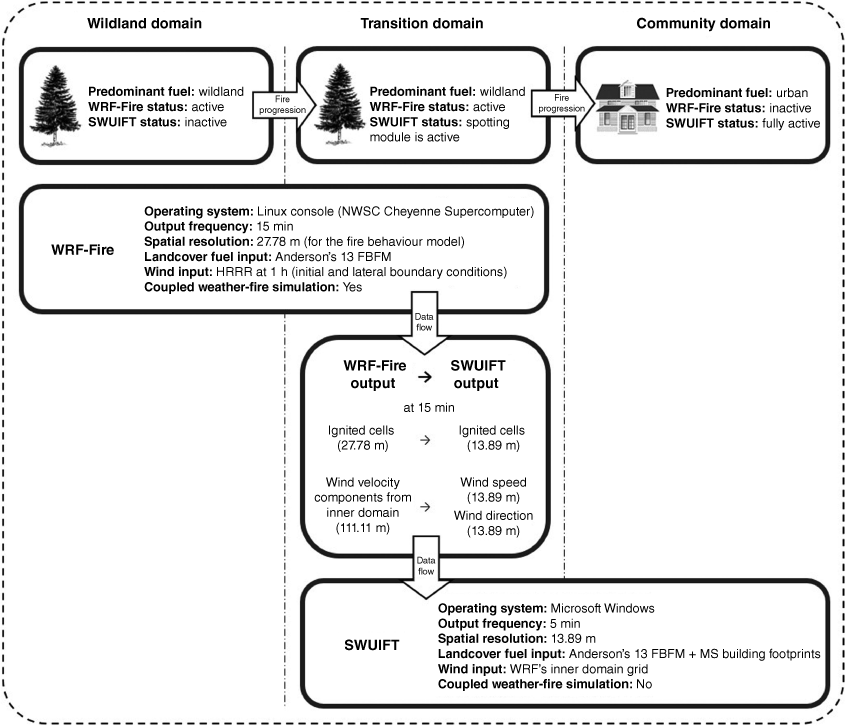
The definitions of the boundaries for the community and transition domains follow the Neighbourhood-Based Housing Density (NBHD) method, also proposed in Szasdi-Bardales et al. (2024). In this method, each structure (regardless of its area) is represented as a point located at its geometrical centre. Subsequently, a density raster is built by defining a squared-shaped neighbourhood around each cell and dividing the number of points inside the neighbourhood by its area. This procedure is illustrated in Fig. 7.
NBHD method: (a) buildings are represented by their footprints; (b) each footprint is simplified into a point located at its geometric centre; and (c) a density raster is generated by assigning each cell the density of its square-shaped neighbourhood.
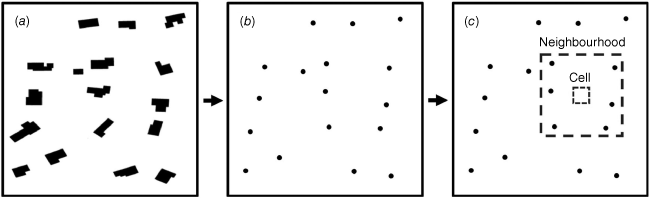
The NBHD method is applied in the current study using a raster of 50 m cells and square-shaped neighbourhoods of 500 m per side (0.25 km2). The community domain is defined as all the areas with a minimum density threshold of 1 structure acre–1 (247 structures km–2), with a minimum size of 1 km2. The transition domain is defined as a buffer of 1.4 km around the community domain. This domain is implemented for computational efficiency (i.e. to prevent the generation and transportation of firebrands that are not expected to land inside the community domain). The buffer is defined such that no more than 1% of the firebrands generated beyond this distance within the wildland domain could be expected to fall inside the community domain, even under the most favourable wind conditions. The same buffer size is used for all the fire scenarios. Fig. 8 shows the areas covered by the community, transition and wildland domains for the Marshall Fire case study.
The generated community domain covers the location of the main clusters of structures while leaving out several buildings located west of Louisville and in the commercial area along US 36 in Superior. This is owing to the large size of several commercial buildings, which reduces the density in the region because the NBHD method does not account for building areas. The low density of structures outside the community domain is expected to result in fire behaviour that is better represented with a wildland fire model than an urban fire model. In the coupled set-up, any structure located outside the community domain can ignite when reached by the fire spread from WRF-Fire, and by fire spotting if located inside the transition domain. Further analysis of the proposed methodology and its influence on the simulation results is provided in the Results and discussion section, where a sensitivity analysis is performed to show how varying neighbourhood sizes and minimum density thresholds impact on the precision of the simulation results.
Documented damage, fire locations and suppression activities during the Marshall Fire
This section provides information on (1) documented active fire locations, (2) documented fire suppression activities, and (3) the final report of damaged and destroyed structures.
Timeline of fire progression
Detailed documentation of fire progression inside communities affected by wildfires can provide vital information to understand fire behaviour and can be used to validate simulation results. Although satellite observations can be used to track fire perimeters in the wildland, they lack the temporal and spatial resolutions needed for fire tracking inside communities (Lareau et al. 2022). Meanwhile, data documented by official agencies and ordinary citizens can offer an alternative for detailed tracking of the fire progression inside the affected communities.
A collection of more than 550 videos recorded during the Marshall Fire is publicly available (KUSA-TV 2022). The compilation of these videos focused on broad documentation of the events during the incident rather than on the fire spread pattern, but several of the videos show structures, vegetation, or other elements ablaze. Thus, as part of this study, all the videos were analysed, and the location and time of active fires were manually extracted.
Fig. 9 presents 193 active fire locations classified by fire type and time of observation. All the locations were visually confirmed by comparing the videos against Google Street View images. Each mark on the map represents an observed fire location rather than the location from which the video was recorded (in many cases a single video resulted in several individual fire locations). All times are taken as reported in the videos. When a fire is observed in the same location at different times, the earliest time is reported in Fig. 9. Reported times must not be assumed to be ignition times.
Documented active fire times and locations during the 2021 Marshall Fire, extracted and verified from publicly available videos (KUSA-TV 2022).
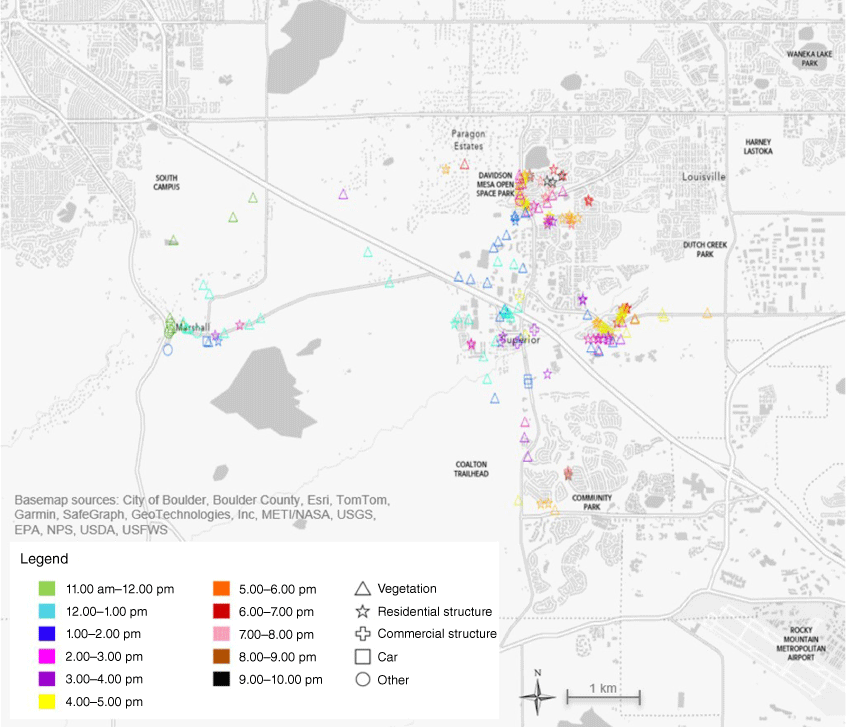
Fire suppression activities
A summary of fire suppression activities observed during the Marshall Fire is presented in Fig. 10. Twenty-one of the locations were manually extracted from the videos that were analysed to obtain active fire locations (KUSA-TV 2022), whereas the rest of the points were collected from other sources (Brennan 2022; Fischer et al. 2022; Boulder County 2022b).
Damaged buildings
An inventory of affected residential and commercial structures is available (Boulder County 2022a). In contrast to the reported active fire locations, this source provides spatial information of all affected structures, but without indicating the times of ignition. Therefore, this inventory is appropriate only for the assessment of the final simulation results (fire line location at 9:00 pm). Fig. 11 displays the location of destroyed or damaged structures.
Reported location of damaged and destroyed structures after the Marshall Fire; map generated based on data from the Boulder County (2022a).
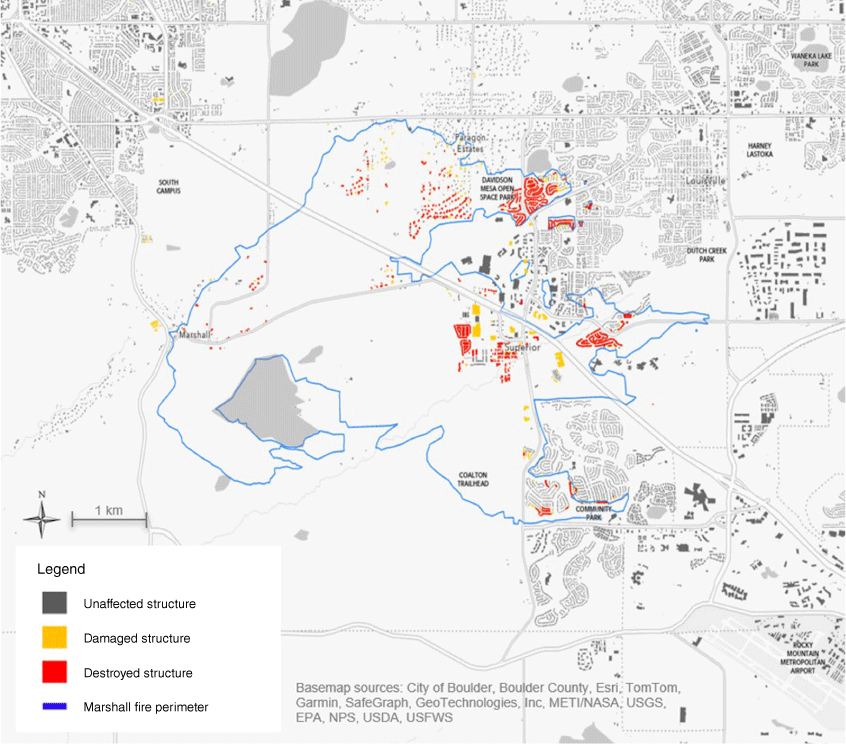
Results and discussion
Wildland fire spread
The wildland fire spread results generated exclusively by the WRF-Fire simulation are presented in Fig. 12. In this case, no structure ignitions are allowed within the wildland domain as the ignition of structures is a feature that will be analysed with the coupled simulations.
Predicted fire spread according to WRF-Fire simulation only at (a) 1:00 pm, (b) 3:00 pm, (c) 5:00 pm, (d) 7:00 pm, and (e) 9:00 pm.
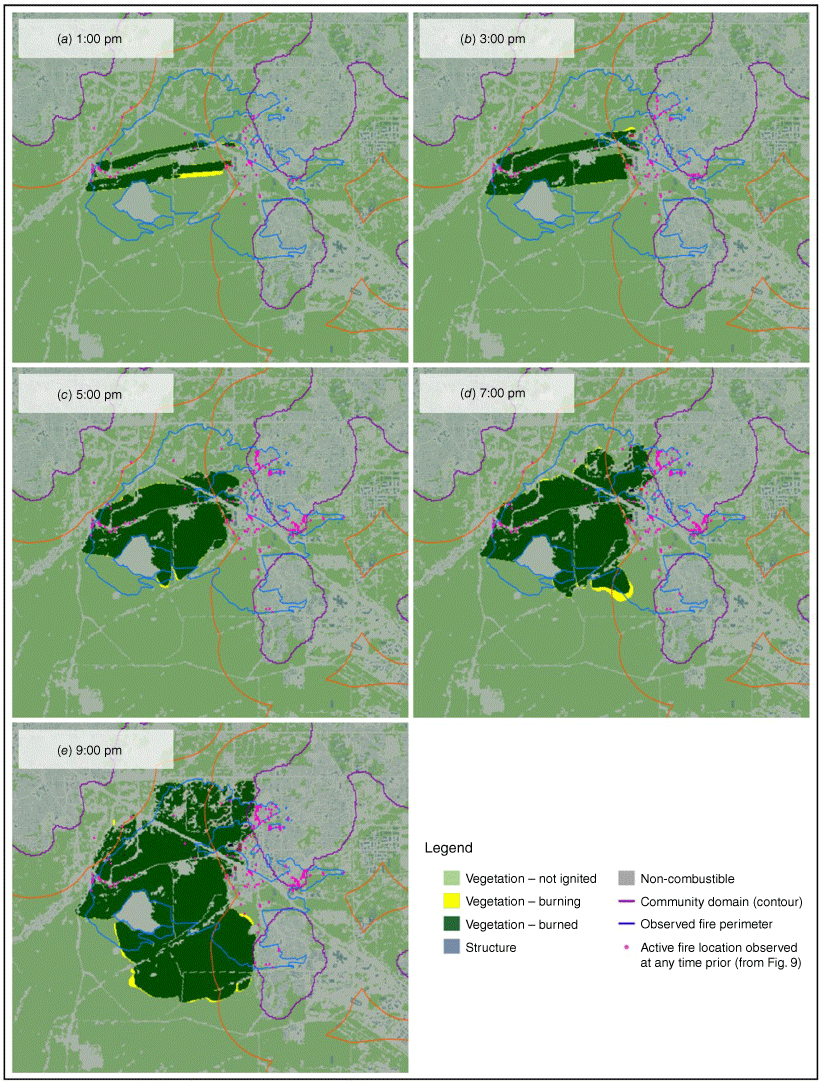
A comparison between Figs 9 and 12 shows that the WRF-Fire simulation captures well the initial eastward spread of the fire. By 1:00 pm (Fig. 12a), simulation results agree with the documented fire locations (Fig. 9, green and cyan symbols) that indicate that the fire has reached Superior and crossed US 36. At later times, the simulation results present different discrepancies with respect to the documented fire locations. These discrepancies will be relevant for the discussion of coupled simulation results because any errors from the wildland simulation are propagated into SWUIFT’s urban fire simulations. In the case of the wildland fire simulation performed by WRF-Fire, most of the factors contributing to modelling errors that were mentioned in the Background section do not apply for the Marshall Fire (the fire spread in primarily short grass fuels homogeneously distributed across the landscape, canopy fire spread was not important and topography was mostly uniform over the region). The fire spread was largely controlled by unusually strong and turbulent winds, including an atmospheric hydraulic jump (i.e. wind discontinuity) near the leading edge of the fire (Juliano et al. 2023). Although WRF-Fire allows for heat and moisture feedback to the atmospheric model, prior results suggest that this feedback had a negligible impact on the fire propagation and the hydraulic jump characteristics. Therefore, the WRF atmospheric model primarily controls the wind field in this particular case owing to the dominant atmospheric forcing. Despite the thorough validation of WRF over the last two decades, small-scale mesoscale and microscale variability is anticipated owing to turbulence and other physics parameterisations. Such deviations likely contributed to the simulation’s overestimation of fire spread south of the observed perimeter line (Fig. 12). Inconsistencies in the fuel map (e.g. representing road breaks as burnable fuel) may also have contributed to this overprediction. Nonetheless, the Marshall Fire case represents a scenario where the WRF-Fire model performs well when simulating wildland fire spread prior to reaching the built environment.
Among the most important observations is that the simulated fire does not cross US 36 in the undeveloped area between Louisville and Superior, whereas the documented locations show fire presence in this area at least since 3:00 pm (Fig. 9, magenta symbols). The discrepancy can be explained by the non-combustible barrier formed by the urban land cover in Superior. Additionally, the simulated fire spread towards the north and south is delayed with respect to the documented observations. The fire was observed inside Louisville by 3:00 pm (Fig. 9, magenta symbols), and reached the cluster of residential structures in Superior by 5:00 pm (Fig. 9, yellow symbols), but the simulation results reached Louisville and Superior at 7:00 pm (Fig. 12d) and 9:00 pm (Fig. 12e), respectively. A comparison of the results at 9:00 pm (Fig. 12e) with the official fire perimeter (Fig. 10) shows that the simulation overestimates the extent of fire spread in the South.
The WRF-Fire simulation stops close to the proposed community domain boundaries in both Louisville and Superior. This confirms that the community domain (Fig. 8) produced by the NBHD method provides an appropriate representation of the WUI areas, identifying important clusters of pixels that are considered non-burnable by WRF-Fire.
Coupled simulations: community fire spread
This section presents the results (Figs 13–15) from the three coupled simulation scenarios (Table 1). All the scenarios share the wildland fire spread simulation from WRF-Fire (Fig. 12) but differ in their prediction of fire spread inside the community owing to the variation in wind and structure fuel inputs.
Predicted fire spread for scenario LRW-AS at (a) 1:00 pm, (b) 3:00 pm, (c) 5:00 pm, (d) 7:00 pm, and (e) 9:00 pm.
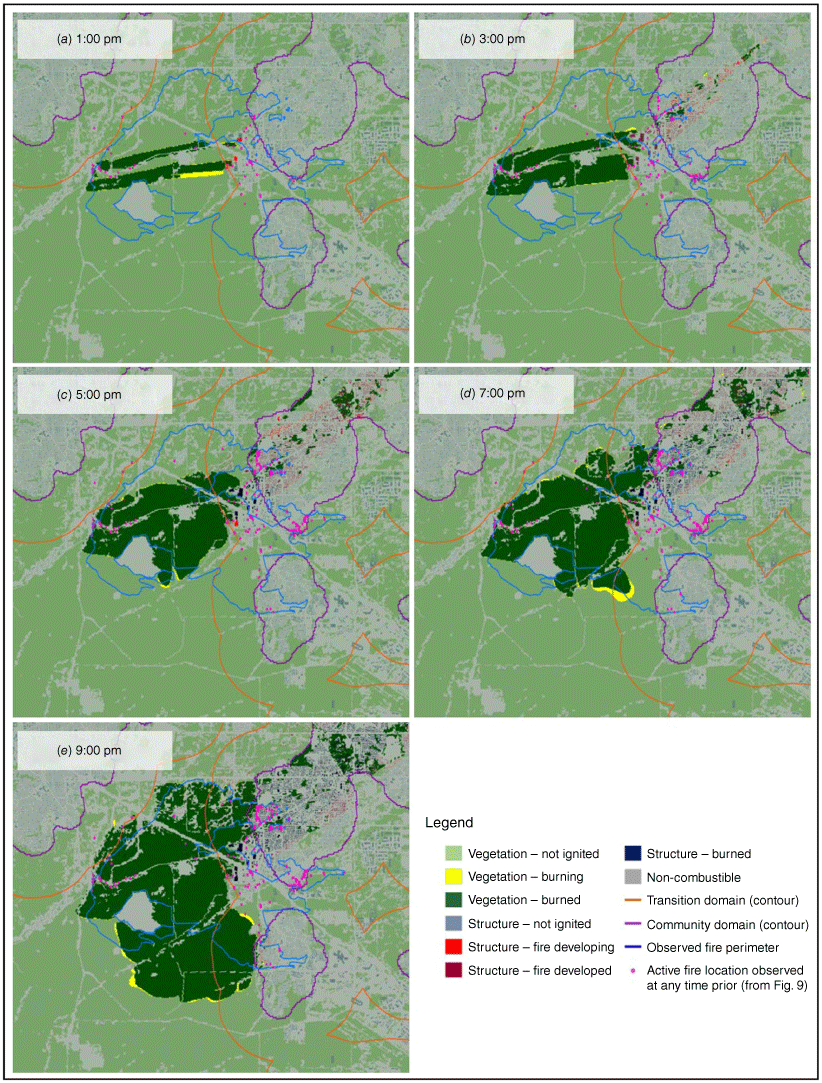
Predicted fire spread for scenario HRW-AS at (a) 1:00 pm, (b) 3:00 pm, (c) 5:00 pm, (d) 7:00 pm, and (e) 9:00 pm.
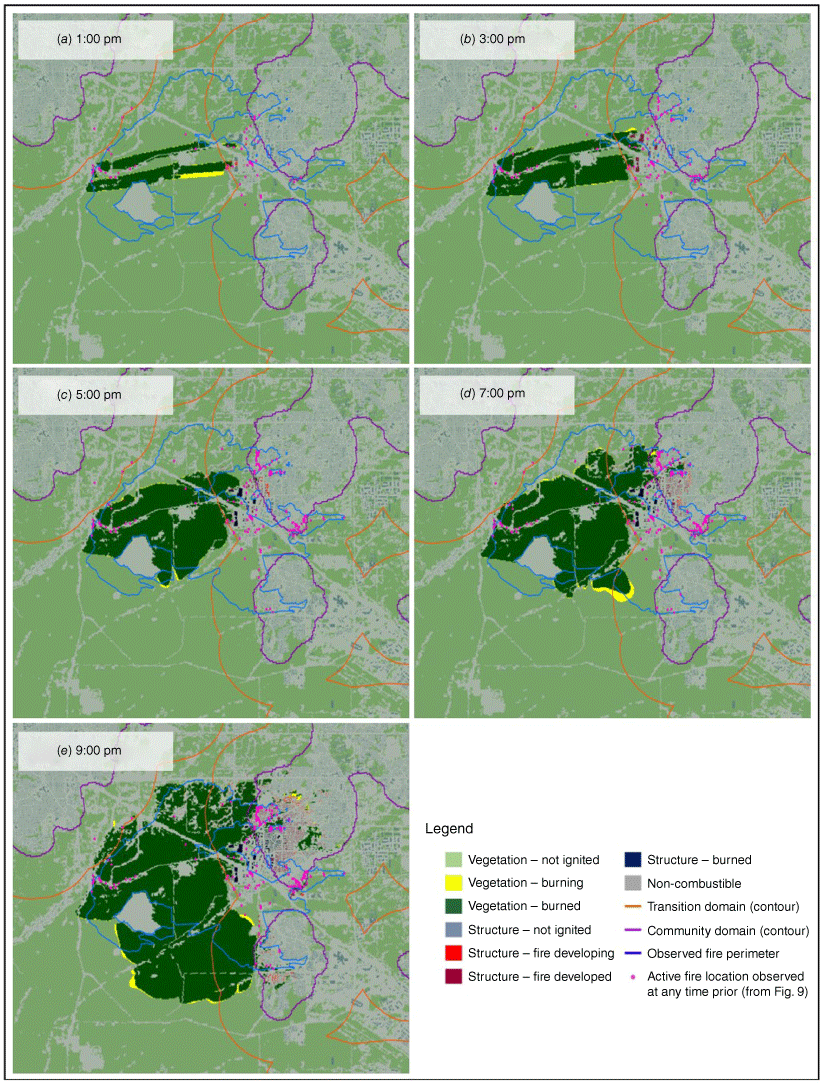
Predicted fire spread for scenario HRW-NFRS at (a) 1:00 pm, (b) 3:00 pm, (c) 5:00 pm, (d) 7:00 pm, and (e) 9:00 pm.
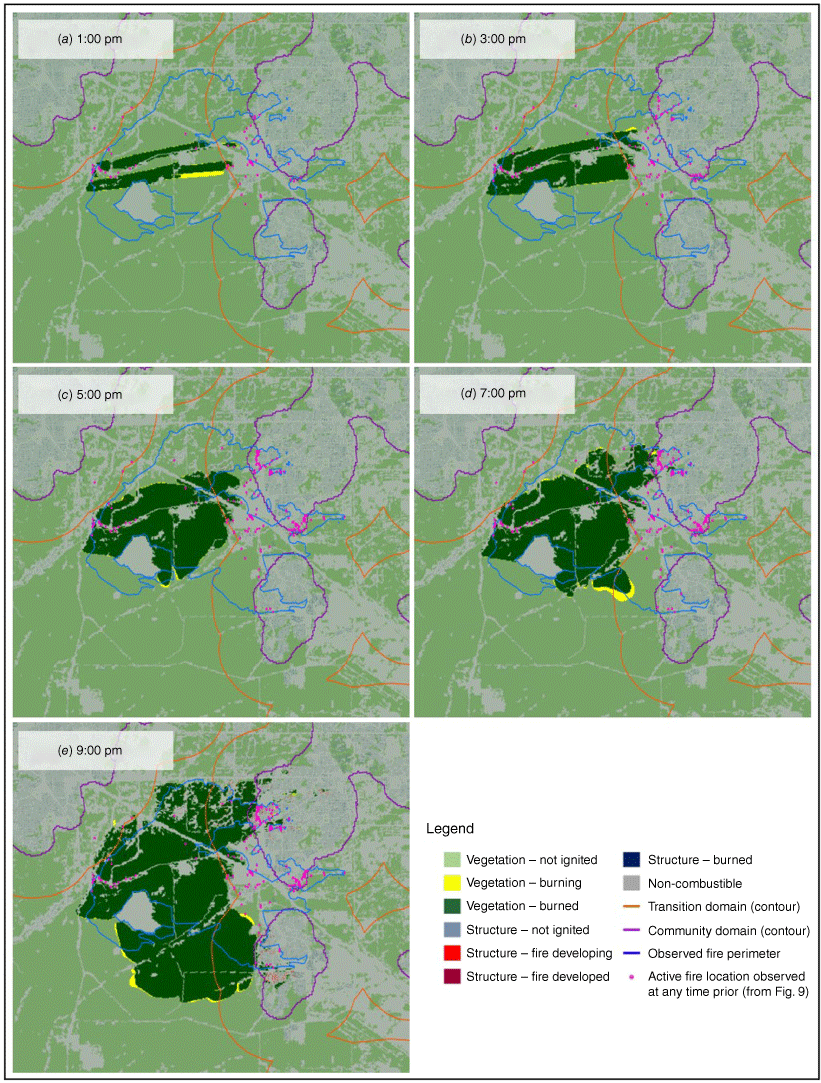
The results from the coupled simulation for Scenario LRW-AS show that by 1:00 pm (Fig. 13a), the fire ignited commercial structures on both sides of US 36 inside the transition domain. Two hours later (Fig. 13b), the fire enters the community domain and makes significant advances inside Louisville. At 5:00 pm (Fig. 13c), the fire continues to spread inside Louisville, with a noticeable widening of the main fire front, reaching the north-east limit of the simulation domain (Fig. 3). By the end of the simulation at 9:00 pm (Fig. 13e), this scenario results in 6410 ignited structures in Louisville and Superior, excluding structures located outside the simulation domain that could have been reached after 5:00 pm. These results clearly deviate from the observed fire progression (Fig. 9) and reported damage (Fig. 10) in terms of the number (1270) and location of damaged and destroyed structures. This overestimation represents an error of 405%.
In Scenario HRW-AS, the low-resolution wind input for the SWUIFT simulation is replaced by a high-resolution input generated by WRF. At 1:00 pm, the simulation results (Fig. 14a) resemble those from LRW-AS, with the fire affecting only a small number of commercial structures on both sides of US 36 inside the transition domain. Additional timesteps (Fig. 14b–e) show a fire expansion that better resembles the observed fire spread (Figs 9 and 10). This can be the result of WRF’s ability to simulate a hydraulic jump, which resulted in slower fire spread eastward, but wider north–south spread. Scenario HRW-AS results in a final estimate of 2867 ignited structures, which represents an overestimation of 126% compared with the observed damage. Additionally, the fire in HRW-AS does not reach the limits of the spatial domain by the end of the simulation, implying that the actual decrease in the simulated number of ignited structures is potentially higher. It can, therefore, be concluded that the resolution of the wind input greatly affects the simulated fire spread and predicted damage. Using a WRF-generated wind field as an input introduces more realistic wind conditions into the simulation, improving SWUIFT’s performance.
Despite the improvement of the results through the introduction of a high-resolution wind input, the simulated fire spread inside the affected communities still overestimates the number of affected structures and the reach of the different fire fronts. As discussed earlier, the default version of SWUIFT does not differentiate the ignition criteria based on the level of structure hardening and requires manual adjustment. To address this limitation, fire-resistant structures are identified and replaced with non-combustible pixels in the simulation. Most of the excluded structures are non-residential buildings made from material other than wood, located in the commercial area between Superior and Louisville, around US 36, as well as in the vicinity of the Rocky Mountain Regional Airport, southeast of Superior as shown in Fig. 5.
The results from Scenario HRW-NFRS (Fig. 15) show a delayed fire entrance into Louisville, due to the limited number of combustible pixels within the area around US 36, where the wildland fire front approached the community domain in the other two scenarios. As a result, the fire does not enter Louisville until 7:00 pm (Fig. 15d) and it does so from a position further north. Although the WRF-Fire simulation in the wildland lags by ~4 h (Fig. 9, magenta symbols), a fire entrance into Louisville from the north results in a SWUIFT simulation that better resembles the observed fire progression (Fig. 9) and damage distribution (Fig. 11). The fire spread in Superior’s residential areas is similar to that of HRW-AS, which is expected as the removal of fire-resistant structures did not affect this region. The fire enters Superior’s residential areas at ~9:00 pm (Fig. 15e), a 3-h delay relative to observations (Fig. 9, orange symbols) that can be explained by the slow southward wildland fire spread from the WRF-Fire simulation. The results from HRW-NFRS estimate a total of 1110 ignited structures, which represents an underestimation with a 13% error compared with the observed damage (Boulder County 2022a).
A comparison of the three coupled simulation scenarios shows how wind input resolution and building type consideration affect the accuracy of the simulation results. The most accurate results were obtained for scenario HRW-NFRS, which used a high-resolution wind field generated by WRF and considered fire-resistant structures as non-combustible. This scenario, along with the observations from Fig. 9, suggests that the improved fire-resistant characteristics of structures located in the area south of Louisville along US 36 prevented hundreds of ignitions that might have occurred had the fire entered the residential areas earlier. This observation highlights the potential applications of model coupling for analysing large-scale WUI wildfires.
Sensitivity analysis of the thresholds defining the community domain
Different neighbourhood sizes and minimum density thresholds result in different community domain boundaries, which can affect the simulated fire spread patterns and prediction results (Szasdi-Bardales et al. 2024). In general, varying the neighbourhood size creates a trade-off between the homogeneity and precision of the generated community domains (i.e. larger neighbourhoods yield more homogeneous but less precise domains). Meanwhile, the minimum density threshold can be adjusted to control how closely borders separating the structure clusters from the vegetation areas are followed. In this section, a sensitivity analysis is conducted to evaluate how varying threshold values impact the accuracy of the simulation results. Nine simulations of the HRW-NFRS scenario are run using a combination of different neighbourhood sizes and minimum density thresholds that result in the community domain boundaries shown in Fig. 16. For all cases, the transition domain is defined as a 1.4 km buffer area around each community domain boundaries.
Contours of community domains defined with different minimum density thresholds for neighbourhood sizes of (a) 250 m, (b) 500 m, and (c) 750 m.
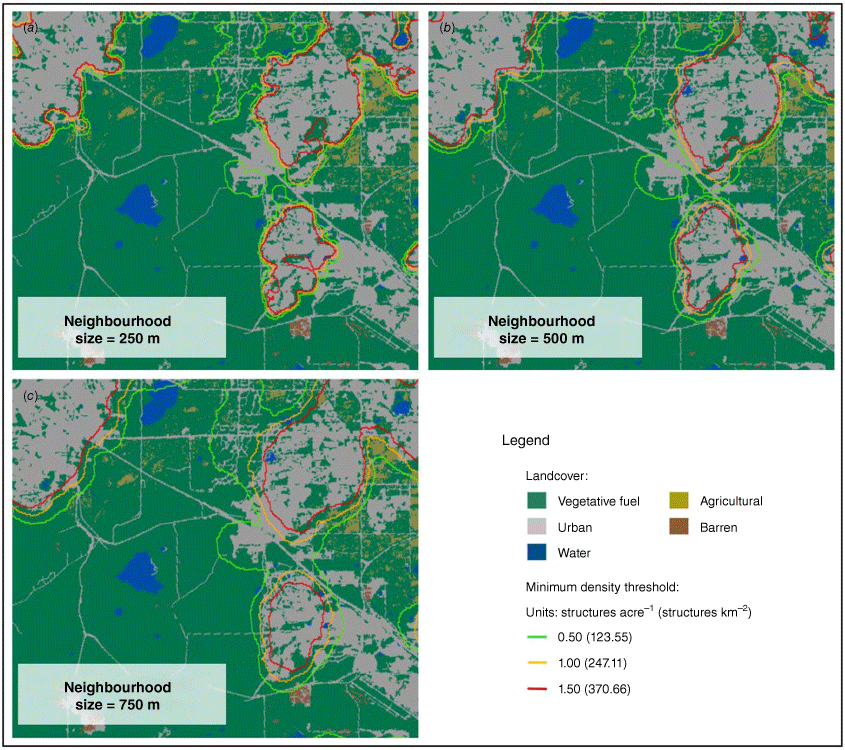
The fire progression over time for each of the nine simulations for the HRW-NFRS scenario is compared in Fig. 17 against the observed final fire perimeter. Table 2 presents different metrics to numerically assess the accuracy of the simulation results, including the Jaccard Index J (Eqn 1) and the Sørensen–Dice Index, SDI (Eqn 2). Both indexes range from 0 to 1, with 0 indicating total dissimilarity and 1 total similarity. For both cases, A is the area inside the observed fire perimeter and B is the ignited area predicted by the simulation.
The results of the sensitivity analysis show that the accuracy of the fire spread prediction is more sensible to variations in the minimum density threshold than to changes in the neighbourhood size. Specifically, community domains defined by a minimum density threshold of 0.5 structures acre–1 (124 structures km–2) are found to produce particularly inaccurate predictions in terms of the number of ignited buildings compared with the other considered thresholds. This inaccuracy can be attributed to the inclusion of large areas predominantly covered by vegetation fuel inside the community domain. Meanwhile, variations in the similarity metrics between the predicted and observed fire perimeters are not as significantly affected when different thresholds are used to define the community domain. Overall, the thresholds originally selected for this study (i.e. 0.25 km2 square-shaped neighbourhood and a minimum density threshold of 247 structures km–2) provide an accurate fire spread prediction that offers a balanced precision between the estimated number of ignited structures and the similarity of the final fire perimeter.
Temporal progression of the fire spread in the HRW-NFRS scenario for community domains defined with neighbourhood sizes and minimum density thresholds of (a) 250 m and 0.5 structures acre–1, (b) 250 m and 1.0 structures acre–1, (c) 250 m and 1.5 structures acre–1, (d) 500 m and 0.5 structures acre–1, (e) 500 m and 1.0 structures acre–1, (f) 5000 m and 1.5 structures acre–1, (g) 750 m and 0.5 structures acre–1, (h) 750 m and 1.0 structures acre–1, and (i) 750 m and 1.5 structures acre–1. Note: 1 structures acre–1 = 247 structures km–2.
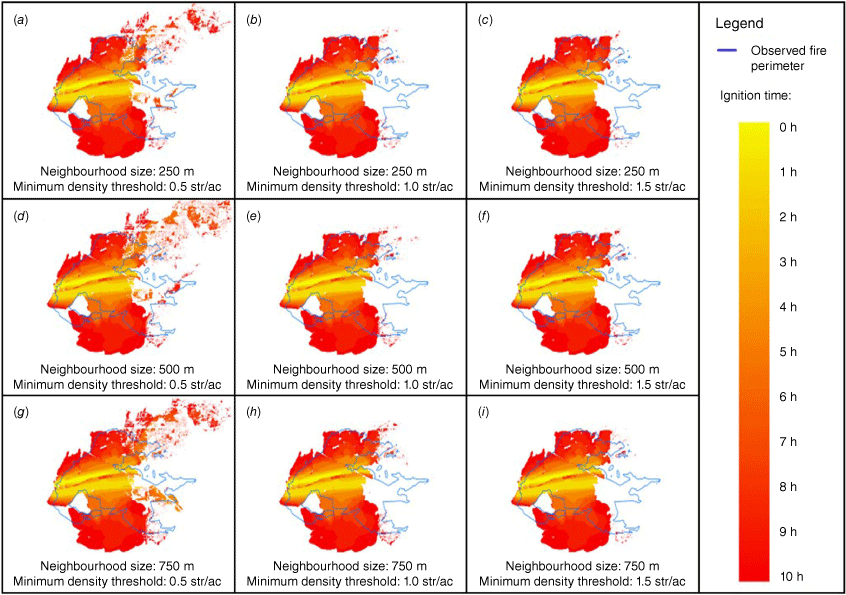
Square-shaped neighbourhood size (m) | Minimum density threshold (structures acre–1) | Ignited structures estimation error (%) | Jaccard Index | Sørensen–Dice Index | |
---|---|---|---|---|---|
250 | 0.5 | 161 | 0.46 | 0.63 | |
250 | 1.0 | −24 | 0.50 | 0.67 | |
250 | 1.5 | −38 | 0.51 | 0.67 | |
500 | 0.5 | 319 | 0.42 | 0.59 | |
500 | 1.0 | −13 | 0.50 | 0.67 | |
500 | 1.5 | −31 | 0.51 | 0.67 | |
750 | 0.5 | 245 | 0.45 | 0.62 | |
750 | 1.0 | −3 | 0.50 | 0.66 | |
750 | 1.5 | −35 | 0.50 | 0.66 |
Note: 1 structures acre–1 = 247 structures km–2.
Summary and conclusions
This study aimed to complement the capabilities of existing fire spread models for a complete simulation of a wildfire incident. The paper also assessed how variations in wind conditions affect simulation outcomes and analysed how different building types impact fire spread patterns. This work performed an offline coupling of WRF-Fire, a fully coupled wildland fire–atmosphere simulation platform, and SWUIFT, a semi-empirical model for the simulation of fire spread in the WUI. The 2021 Marshall Fire in Colorado was selected as a case study, where the fire ignited in fine fuels and expanded to the nearby suburban communities of Superior and Louisville. Simulations were performed for a timeframe between 11:08 am and 9:00 pm local time. The offline coupling of the models followed a Three-Domain Solution, including wildland, transition and community domains, with the relevant definitions and details discussed in Szasdi-Bardales et al. (2024). Maps of the time and location of the fire during the event were generated using publicly available information.
Three different simulation scenarios were analysed to evaluate the effects of two key inputs on the results of fire simulation inside the WUI: (1) resolution of the wind input, and (2) consideration of structures with improved fire-resistant features compared with wood light-frame residential structures. The scenario that included a high-resolution wind input (i.e. surface wind field generated by WRF with a 111.11 m resolution and updated every 15 min) and consideration of fire-resistant structures led to results that best resembled the fire spread and number of damaged structures observed after the actual incident (underestimation with 13% error). These results confirmed that the simulation benefited from more detailed and realistic wind conditions. It was also shown that structures with characteristics that make them less vulnerable to ignition can be expected to act as barriers and slow down or stop fire spread in a community.
This study demonstrated the potential of fire spread model coupling for the simulation of complete wildland–WUI fires, but several limitations and assumptions should be accounted for in the conclusions of this work. For instance, the location of community domain boundaries can affect the simulation results. In this study, the area with buildings located close to US 36 in Superior was not classified within the community domain, because the large size of commercial buildings resulted in a lower density for the area (the NBHD method does not consider building size). Future improvements to the NBHD method may be applied to address this issue. The simplification of structures with fire-resistant characteristics as non-combustible cells provided a reasonable approximation to demonstrate how buildings with improved fire-resistant features can limit fire spread inside communities. This simplification represents a best-case scenario. Future work will explore methodologies for a more precise classification of fire-resistant features, developing capabilities to incorporate hardened structures into the simulation framework. Finally, the spread of urban fire back into the wildland is not considered, which affects the capacity of the simulation to reach isolated vegetation areas. Future work will include enhancing the integrated simulation capabilities towards developing an online coupled atmosphere–wildland and urban fire spread platform.
Data availability
The data that support this study will be shared on reasonable request to the corresponding author.
Declaration of funding
This work is supported through the National Science Foundation’s Leading Engineering for America’s Prosperity, Health, and Infrastructure (LEAP HI) program by grant number CMMI-1953333. The opinions and perspectives expressed in this study are those of the authors and do not necessarily reflect the views of the sponsor. Moreover, this work received high-performance computing support from Cheyenne (doi:10.5065/D6RX99HX) provided by the US NSF National Center for Atmospheric Research (NCAR) Computational and Information Systems Laboratory. NCAR is a major facility sponsored by the National Science Foundation under Cooperative Agreement 1852977.
References
Benjamin SG, Weygandt SS, Brown JM, Hu M, Alexander CR, Smirnova TG, Olson JB, James EP, Dowell DC, Grell GA, Lin H, Peckham SE, Smith TL, Moninger WR, Kenyon JS (2016) A North American hourly assimilation and model forecast cycle: the rapid refresh. Monthly Weather Review 144(4), 1669-1694.
| Crossref | Google Scholar |
Benjamin SG, James EP, Szoke EJ, Schlatter PT, Brown JM (2023) The 30 December 2021 Colorado Front Range windstorm and Marshall Fire: evolution of surface and 3D structure, NWP guidance, NWS forecasts, and decision support. Weather and Forecasting 38, 2551-2573.
| Crossref | Google Scholar |
Boulder County (2022a) List of structures damaged or destroyed in the Marshall Fire. Available at https://assets.bouldercounty.gov/wp-content/uploads/2022/01/marshall-fire-damage-assessment-list.pdf [accessed February 2023]
Boulder County (2022b) Marshall Fire operational after-action report. Available at https://assets.bouldercounty.gov/wp-content/uploads/2022/06/marshall-fire-after-action-report.pdf [accessed February 2023]
Brenkert-Smith H, Champ P, Flores N (2012) Trying not to get burned: understanding homeowners’ wildfire risk–mitigation behaviors. Environmental Management 50, 1139-1151.
| Crossref | Google Scholar | PubMed |
Brennan N (2022) Firefighter shares photos, video from Marshall Fire front lines. Available at https://www.9news.com/article/news/local/wildfire/photos-video-marshall-fire-front-lines/73-ede64f54-2ec6-4403-aee3-dbf8100e8c10 [accessed June 2022]
Calkin DE, Cohen JD, Finney MA, Thompson MP (2014) How risk management can prevent future wildfire disasters in the wildland–urban interface. Proceedings of the National Academy of Sciences 111(2), 746-751.
| Crossref | Google Scholar | PubMed |
Chulahwat A, Mahmoud H (2024) The impact of wind characteristics on the spatial distribution of damage to the built environment during wildfire events: the 2022 Marshall Fire. Natural Hazards Review 25(1), 06023003.
| Crossref | Google Scholar |
Clark TL, Jenkins MA, Coen JL, Packham DR (1996a) A coupled atmosphere–fire model: convective feedback on fire-line dynamics. Journal of Applied Meteorology and Climatology 35(6), 875-901.
| Crossref | Google Scholar |
Clark TL, Jenkins MA, Coen JL, Packham DR (1996b) A coupled atmosphere-fire model: role of the convective Froude Number and dynamic fingering at the fireline. International Journal of Wildland Fire 6(4), 177-190.
| Crossref | Google Scholar |
Clifford E (2016) Predicting homeowner wildfire mitigation behaviors in the wildland–urban interface. Doctoral Dissertation, Arizona State University, Tempe, AZ, USA. Available at https://keep-dev.lib.asu.edu/items/155106
Coen JL (2013) Modeling wildland fires: a description of the Coupled Atmosphere–Wildland Fire Environment model (CAWFE). (No. NCAR/TN-500+STR). 10.5065/D6K64G2G
Coen JL, Cameron M, Michalakes J, Patton EG, Riggan PJ, Yedinak KM (2013) WRF-Fire: coupled weather–wildland fire modeling with the weather research and forecasting model. Journal of Applied Meteorology and Climatology 52(1), 16-38.
| Crossref | Google Scholar |
Colorado Division of Fire Prevention and Control (DFPC) (2022) Marshall Fire facilitated learning analysis. Available at https://storymaps.arcgis.com/stories/83af63bd549b4b8ea7d42661531de512 [accessed June 2022]
DeCastro AL, Juliano TW, Kosovic B, Ebrahimian H, Balch JK (2022) A computationally efficient method for updating fuel inputs for wildfire behavior models using sentinel imagery and random forest classification. Remote Sensing 14(6), 1447.
| Crossref | Google Scholar |
Dennison PE, Brewer SC, Arnold JD, Moritz MA (2014) Large wildfire trends in the western United States, 1984–2011. Geophysical Research Letters 41(8), 2928-2933.
| Crossref | Google Scholar |
Federal Emergency Management Agency (FEMA) (2008) Home builder’s guide to construction in wildfire zones. technical fact sheet series, FEMA P-737. Availble at https://www.govinfo.gov/content/pkg/GOVPUB-HS5-PURL-gpo60104/pdf/GOVPUB-HS5-PURL-gpo60104.pdf
Federal Emergency Management Agency (FEMA) (2022) Hazus inventory technical manual, Hazus 6.0. Available at https://www.fema.gov/sites/default/files/documents/fema_hazus-6-inventory-technical-manual.pdf [accessed June 2023]
Finney MA (1998) FARSITE: Fire Area Simulator – model development and evaluation. Research Paper RMRS-RP-4. (USDA Forest Service, Rocky Mountain Research Station: Ogden, UT) 10.2737/RMRS-RP-4
Finney MA, McHugh CW, Grenfell IC, Riley KL, Short KC (2011) A simulation of probabilistic wildfire risk components for the continental United States. Stochastic Environmental Research and Risk Assessment 25(7), 973-1000.
| Crossref | Google Scholar |
Fischer E, Wham B, Dashti S, Javernick-Will A, Liel A, Whelton A, Berty N, Klingaman J, Metz A, Ramos J, Rose H (2022) ‘The 2021 Marshall Fire, Boulder County, Colorado (Version 1.0).’ (GEER Association) 10.18118/G6KT04
Fovell RG, Brewer MJ, Garmong RJ (2022) The December 2021 Marshall Fire: predictability and gust forecasts from operational models. Atmosphere 13(5), 765.
| Crossref | Google Scholar |
Google Maps (2019) Street view images, Boulder County, CO. Available at https://www.google.com/maps [accessed September 2023]
Headwaters Economics (2022) Wildfires destroy thousands of structures each year. Available at https://headwaterseconomics.org/natural-hazards/structures-destroyed-by-wildfire/ [accessed February 2023]
International Code Council (ICC) (2017) ‘2018 International Wildland–Urban Interface Code.’ (Washington, DC, USA) Available at https://codes.iccsafe.org/content/IWUIC2018P3 [accessed June 2023]
International Code Council (ICC) (2019) ‘2019 California Referenced Standards Code. California Code of Regulations, Title 24, Part 12.’ (Washington, DC, USA) Available at https://codes.iccsafe.org/content/CRSC2019P1 [accessed June 2023]
Jiang W, Wang F, Linghang F, Zheng X, Qiao X, Li Z, Meng Q (2021) Modelling of wildland–urban interface fire spread with the heterogeneous cellular automata model. Environmental Modelling & Software 135, 104895.
| Crossref | Google Scholar |
Juliano TW, Lareau N, Frediani ME, Shamsaei K, Eghdami M, Kosiba K, Wurman J, DeCastro A, Kosovic B, Ebrahimian H (2023) Toward a better understanding of wildfire behavior in the wildland–urban interface: a case study of the 2021 Marshall Fire. Geophysical Research Letters 50(10), e2022GL101557.
| Crossref | Google Scholar |
Juliano TW, Szasdi-Bardales F, Lareau NP, Shamsaei K, Kosovic B, Elhami-Khorasani N, James EP, Ebrahimian H (2024) Brief communication: the Lahaina Fire disaster – how models can be used to understand and predict wildfires. Natural Hazards and Earth System Sciences 24(1), 47-52.
| Crossref | Google Scholar |
KUSA-TV (2022) Burned: the story behind the Marshall Fire. Available at https://www.marshallfiremap.com/ [accessed July 2023]
Landscape Fire and Resource Management Planning Tools (LANDFIRE) (2020) ‘13 Fire Behavior Fuel Models Anderson. LANDFIRE 2.0.0.’ (US Department of the Interior, Geological Survey, and US Department of Agriculture) Available at https://www.landfire.gov/viewer/ [accessed November 2022]
Lareau NP, Donohoe A, Roberts M, Ebrahimian H (2022) Tracking wildfires with weather radars. Journal of Geophysical Research: Atmospheres 127(11), e2021JD036158.
| Crossref | Google Scholar |
Lautenberger C (2013) Wildland fire modeling with an Eulerian level set method and automated calibration. Fire Safety Journal 62, 289-298.
| Crossref | Google Scholar |
Linn RR (1997) ‘A transport model for prediction of wildfire behavior’. LA-13334-T. (Los Alamos National Laboratory: Los Alamos, NM, USA) 10.2172/505313
Martin W, Martin I, Kent B (2009) The role of risk perceptions in the risk mitigation process: the case of wildfire in high risk communities. Journal of Environmental Management 91(2), 489-498.
| Crossref | Google Scholar | PubMed |
Masoudvaziri N, Szasdi Bardales F, Keskin O, Sarreshtehdari A, Sun K, Elhami Khorasani N (2021) Streamlined wildland–urban interface fire tracing (SWUIFT): modeling wildfire spread in communities. Environmental Modelling and Software 143, 105097.
| Crossref | Google Scholar |
Masoudvaziri N, Elhami-Khorasani N, Sun K (2023) Toward probabilistic risk assessment of wildland–urban interface communities for wildfires. Fire Technology 59, 1379-1403.
| Crossref | Google Scholar |
Mastorakos E, Gkantonas S, Efstathiou G, Giusti A (2022) A hybrid stochastic Lagrangian–cellular automata framework for modelling fire propagation in inhomogeneous terrains. Proceedings of the Combustion Institute 39(3), 3853-3862.
| Crossref | Google Scholar |
Meldrum J, Champ P, Warziniack T, Brenkert-Smith H, Barth CM, Falk LC (2014) Cost shared wildfire risk mitigation in Log Hill Mesa, Colorado: survey evidence on participation and willingness to pay. International Journal of Wildland Fire 23(4), 567-576.
| Crossref | Google Scholar |
Mell W, Jenkins MA, Gould J, Cheney P (2007) A physics-based approach to modelling grassland fires. International Journal of Wildland Fire 16(1), 1-22.
| Crossref | Google Scholar |
Microsoft (2022) US Building Footprints (2022 release). Available at https://github.com/microsoft/USBuildingFootprints [accessed February 2023]
Moritz MA, Batllori E, Bradstock RA, Malcolm Gill A, Handmer J, Hessburg PF, Leonard J, McCaffrey S, Odion DC, Schoennagel T, Syphard A (2014) Learning to coexist with wildfire. Nature 515, 58-66.
| Crossref | Google Scholar | PubMed |
Munoz-Esparza D, Kosovic B, Jimenez PA, Coen JL (2018) An accurate fire-spread algorithm in the Weather Research and Forecasting Model using the level-set method. Journal of Advances in Modeling Earth Systems 10(4), 908-926.
| Crossref | Google Scholar |
National Fire Protection Association (NFPA) (2017) Standard for reducing structure ignition hazards from wildland fire. NFPA 1144-2018. Available at https://www.nfpa.org/codes-and-standards/1/nfpa-1/4/nfpa-1144
National Weather Service (NWS) (2022) Summary of the Marshall Fire and high wind event on December 30, 2021. Available at https://www.weather.gov/bou/HighWinds12_30_2021 [accessed February 2023]
Rothermel RC (1972) A mathematical model for predicting fire spread in wildland fuels. Research Paper INT-115. (USDA Forest Service, Intermountain Forest and Range Experiment Station: Ogden, UT) Available at https://www.fs.usda.gov/rm/pubs_int/int_rp115.pdf
Shamsaei K, Juliano TW, Roberts M, Ebrahimian H, Kosovic B, Lareau NP, Taciroglu E (2023) Coupled fire–atmosphere simulation of the 2018 Camp Fire using WRF-Fire. International Journal of Wildland Fire 32(2), 195-221.
| Crossref | Google Scholar |
Skamarock WC, Klemp JB (2008) A time-split nonhydrostatic atmospheric model for weather research and forecasting applications. Journal of Computational Physics 227(7), 3465-3485.
| Crossref | Google Scholar |
Skamarock WC, Klemp JB, Dudhia J, Gill DO, Liu Z, Berner J, Wang W, Powers JG, Duda MG, Barker D, Huang X (2021) A Description of the Advanced Research WRF Version 4.3. (NCAR/TN-556+STR) 10.5065/1dfh-6p97
Spyratos V, Bourgeron P, Ghil M (2007) Development at the wildland–urban interface and the mitigation of forest-fire risk. Proceedings of the National Academy of Sciences 104(36), 14272-14276.
| Crossref | Google Scholar | PubMed |
Szasdi-Bardales F, Shamsaei K, Lareau NP, Juliano TW, Kosovic B, Ebrahimian H, Elhami-Khorasani N (2024) Integrating dynamic wildland fire position input with a community fire spread simulation: a case study of the 2018 Camp Fire. Fire Safety Journal 143, 104076.
| Crossref | Google Scholar |
Thomas CM, Sharples JJ, Evans JP (2017) Modelling the dynamic behaviour of junction fires with a coupled atmosphere–fire model. International Journal of Wildland Fire 26(4), 331.
| Crossref | Google Scholar |
Western Regional Climate Center [WRCC] (2021) Sugarloaf Colorado Daily Summary. Available at https://wrcc.dri.edu/cgi-bin/wea_daysum2.pl [accessed February 2023]