Influence of fuel structure on gorse fire behaviour
Andres Valencia




A Department of Civil and Natural Resources Engineering, University of Canterbury, Christchurch 8140, New Zealand.
B University Centre for Atmospheric Research, School of Earth and Environment, University of Canterbury, Christchurch, New Zealand.
C New Zealand Forest Research Institute, Scion, Christchurch, New Zealand.
D Fire and Emergency New Zealand, Christchurch, New Zealand.
E USDA Forest Service, Missoula Fire Science Laboratory, Missoula, Montana, USA.
International Journal of Wildland Fire 32(6) 927-941 https://doi.org/10.1071/WF22108
Submitted: 1 July 2022 Accepted: 11 April 2023 Published: 15 May 2023
© 2023 The Author(s) (or their employer(s)). Published by CSIRO Publishing on behalf of IAWF. This is an open access article distributed under the Creative Commons Attribution-NonCommercial-NoDerivatives 4.0 International License (CC BY-NC-ND)
Abstract
Background: Complex interactions between fuel structure and fire substantially affect fire spread and spatial variability in fire behaviour. Heterogeneous arrangement of the fuel coupled with variability in fuel characteristics can impact heat transfer efficiency, preheating of unburned fuel and consequent ignition and spread.
Aim: Study the influence of pre-burn fuel structure (canopy height, spatial arrangement) on fire behaviour (rate of spread, flame residence time) derived from high-resolution video of a prescribed gorse fire.
Method: Rate of spread and flame residence time are calculated and mapped from high-resolution overhead visible-spectrum video, and compared with the Canopy Height Model derived from pre-burn Light Detection and Ranging (Lidar) scans.
Results: Geospatial analytics can provide precision observations of fire behaviour metrics. Rates of spread under high wind conditions are influenced by local changes in canopy height and may be more dependent on other fuel characteristics, while flame residence time is better correlated with canopy height.
Conclusions: These observational technology and spatio-temporal analytical techniques highlight how detailed fire behaviour characteristics can be derived from these data.
Implications: The results have implications for wildfire modelling and Wildland–Urban Interface (WUI) building design engineers, as the reported dataset is suitable for model validation and the analysis contributes to further understanding of gorse fire hazard.
Keywords: fire behaviour, gorse, image analysis, image velocimetry, lidar, rate of spread, residence time, UAV, Ulex europaeus, wildfires.
Introduction
Wildfire behaviour is driven by complex interactions between fire energy, fuel load and structure, and coupled fire–atmosphere dynamics (Clements et al. 2007; Dahale et al. 2013; Finney et al. 2015; Sullivan 2017; Katurji et al. 2021). Understanding these interactions is essential for informed firefighting operations (Beer 1991; Page and Butler 2017), building design in the wildland–urban interface (WUI) (Penney et al. 2020, 2022) and development of wildfire simulation models (Mell et al. 2007, 2011; Morvan 2011; Hoffman et al. 2016). Field-scale shrubland fire behaviour has primarily been characterised for a whole burn plot by using metrics such as average rate of spread, residence time and fire intensity, generally accounting for first-order mechanisms (Martins Fernandes 2001; Santoni et al. 2006; Cruz et al. 2013). Although this practical approach is deemed reasonable for numerous applications, it falls short of the level of detail necessary to progress the current knowledge on fire spread mechanisms and to assess the ability of physics-based models, such as WFDS (Wildland–Urban Interface Fire Dynamics Simulator) (Mell et al. 2011; Sánchez-Monroy et al. 2019), FIRETEC (Linn and Cunningham 2005; Canfield et al. 2014) and FIRESTAR (Morvan et al. 2009) to predict fire behaviour.
Several efforts have been made to develop deployable techniques that can accurately characterise spatial and transient fire behaviour and their interactions with the fuel and the overlying atmosphere at the field scale. Rossi et al. (2010) developed a 3D imaging technique capable of measuring morphological characteristics of wildfires and estimating rate of spread of complex fire fronts. This technique has been expanded to include Unmanned Aerial Vehicle (UAV) technology (Ciullo et al. 2020; Fayad et al. 2022) with the aim of enhancing spatial coverage and fire geometry definition, as well as exploring fuel–fire interactions. Recently, Katurji et al. (2021) developed a novel velocimetry technique based on high-resolution infrared images suitable for measuring interactions between the flaming zone and the overlying atmospheric turbulent boundary layer. The technique was initially tested during cereal crop stubble prescribed fires and validated with in-field instrumentation (Finney et al. 2018), and recently used gorse fires dynamics analysis (Katurji et al. 2022).
These new-generation experimental techniques are capable of capturing complex wildfire interactions at the field scale, and will contribute to further characterising the dynamics of the flaming zone essential for the development of future fire spread models (Finney et al. 2015). In this context, the current experimental study links spatially resolved fire behaviour properties at field scale to high-fidelity fuel structure data obtained with remote sensing. In this work, recently developed non-intrusive UAV-based methods (Hartley et al. 2022; Schumacher et al. 2022) are leveraged to study the influence of pre-burn fuel structure (canopy height and spatial arrangement) on fire behaviour (rate of spread and flame residence time) derived from high-resolution overhead RGB (Red, Green, Blue or visible) videos collected during a 4-ha prescribed gorse fire.
In the second section of this paper, the burn site and the different experimental methods used in this work are described. The third section presents the methods applied to derive detailed fire behaviour metrics from UAV platforms. Results from this work and their associated uncertainties are reported in the fourth and fifth sections, respectively. Finally, conclusions and relevant discussions are presented in the final section of this work.
Methods
Site description and burning conditions
The research site is located at a latitude of −43.409, longitude of 171.568, and altitude above the sea level of 300 m, 15 km north of the Rakaia Gorge in Mid-Canterbury, New Zealand. The experimental burn layout is presented in Fig. 1, showing the six burn blocks, hereafter referred to as plots P1, P2, P3, P4, P5 and P6. The data presented here were acquired from the P2 experimental burn as part of the wider project (video available in: https://youtu.be/fdSvSVKFrMw), which was ignited on 2 March 2020 at 12:11 pm New Zealand Daylight Time (NZDT) and lasted 5 min. The plot was ignited as a wind-driven line fire, with the downwind edge lit by drip torch, with five igniters each lighting a short section to establish a completed line as quickly as possible. Lateral ignitions at each side of the plot were also used to keep a line-like fire front. Detailed information about UAV capabilities used to collect fire spread and fuel data is provided in the next section.
Experimental burn layout showing plot sizes, fire spread overlay on Plot 2, fire spread direction and other instrumentation locations. The top-view picture of the flaming zone was taken from an Unmanned Aerial Vehicle (UAV) and overlaid on the map at the 1-min time point after the ignition.
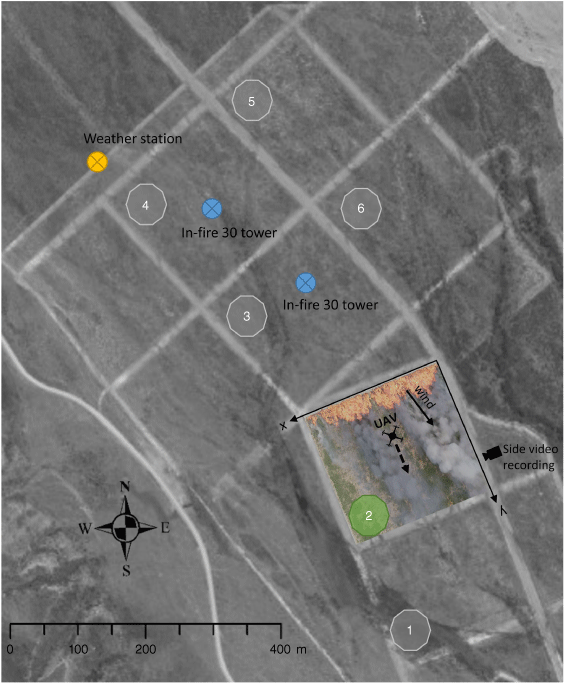
Temperature, relative humidity, wind direction and wind speed were recorded every minute of the burn by an on-site 10-m weather station (yellow in Fig. 1). Information about the experimental conditions is provided in Table 1. P3 and P4 were instrumented with two 30 m sonic anemometer towers (shown in blue in Fig. 1) (SATI/3(K) series 3-dimensional ultrasonic anemometer, K-probe, 150 mm vertical and horizontal measurement path length, sampling frequency 20 Hz; Applied Technologies Inc., Loughborough, UK). The two towers were near centrally located in the burn blocks as the fireline flaming zone passed across. Infrared and visible videos (not used in this work) were also acquired from the side of the P1, P2, P3 and P4 burn experiments.
Temperature (°C) | Relative humidity (%) | Wind direction (°) | Wind speed (m/s) | Moisture content (live gorse) (%) | Moisture content (dead gorse) (%) | Moisture content (grass) (%) | |
Average | 22.9 | 33.2 | 313 | 9.80 | 175.6 | 9.9 | 70.0 |
Min. | 22.9 | 32.8 | 306 | 8.42 | – | – | – |
Max. | 23.0 | 34.5 | 322 | 11.40 | – | – | – |
The dominant vegetation type was gorse (Ulex europaeus L.), ranging from 0.2 to 2.0 m in height. Gorse height and percentage cover were heterogeneous across the site, with some areas containing tall dense vegetation, and other areas being relatively open with short clumps of gorse interspersed with grassy patches. Apart from gorse, the overstorey contained a small component of matagouri (Discaria toumatou Raoul) shrub, and the understorey consisted mostly of grasses with a component of wild rose (Rosa sp.), California thistle (Cirsium arvense (L.) Scop) and Russell lupin (Lupinus polyphyllus Lindl).
The research site had a range of fuel types and size classes, with fuel loads and the resulting fire intensity and spread rates varying across the site owing to differences in vegetation height, density, coverage, proportion of dead material, and the presence of other species. Three different fuel classes were identified:
Standing shrubs: predominantly gorse with a component of matagouri, with live and dead leaves and needles, twigs and stems elevated above ground surface.
Understorey fuels: grasses and other herbaceous species (thistle, lupin) found below the shrub canopy.
Surface fuels: dead leaf and needle litter and downed twigs and branches from shrubs that form a distinct stratum on the ground surface.
Within the area, a 200 × 200-m burn plot was established on flat ground (a dry riverbed with slight undulations), ensuring that it aligned with the prevailing up- and down-valley wind directions. In order to characterise the fuel composition in the plot, pre-burn fuel sampling was conducted in three 4 × 1-m subplots within the burn plot. Following the methods described by Pearce et al. (2010), fuel sampling consisted of both non-destructive and destructive measurements: (1) non-destructive estimation of average fuel height and percentage cover per square metre for each fuel class; and (2) destructive sampling, undertaken by cutting and collecting all aboveground biomass associated with each fuel class, and oven-drying it to obtain total biomass and fuel loading.
Information about height, percentage cover and fuel load of each class is provided in Table 2, with standing shrub fuels split into gorse and matagouri, understorey fuel split into grass and other understorey, and surface fuels split into woody debris and litter. Thermochemical properties such as specific yield and heat of pyrolysis were not measured as part of this work.
Fuel class | Height (m) mean (min–max) | Cover (%) mean (min–max) | Total aboveground biomass (kg/m2) mean (min–max)B | Aboveground available fuel load (kg/m2) mean (min–max)B |
Gorse (dead and live) | 1.10 (0.45–2.15) | 43.4 (5–100) | 4.10 (1.74–7.91) | 0.93 (0.36–1.91) |
Matagouri | 1.07 (0.58–1.55) | 5.4 (0–10) | 0.31 (0–0.94) | 0.12 (0–0.37) |
Grass | 0.13 (0.09–0.16) | 59.9 (37–77) | 0.30 (0.21–0.37) | 0.30 (0.21–0.37) |
Other herbs | – | – | 0.04 (0–0.12) | 0.04 (0–0.12) |
Woody debris | – | 14.0 (11–18) | 1.65 (0.74–2.24) | 1.65 (0.74–2.24) |
Litter | 58.6A (50.8–66.7) | 37.5 (0–60) | 1.33 (0.55–2.5) | 1.33 (0.55–2.5) |
Total | 7.73 (4.66–12.70) | 4.37 (2.71–6.70) |
ANote that Litter fuel height is given in millimetres.
BTotal aboveground biomass and Aboveground available fuel load were determined using the methodology described in Pearce et al. (2010).
UAV and lidar acquisition
UAV capabilities were used for two different purposes: to record nadir RGB videos of the flaming zone, and to obtain a pre-burn high-density point cloud from Unmanned aerial Laser Scanning (ULS). In advance of any flight operations, an extensive set of Ground Control Points (GCPs) was established throughout the study site. Eight GCPs evenly distributed across the study area ensured a suitable level of accuracy of the ULS data. A further 36 GCPs were established around the individual research burn blocks to be used during the georectification of the acquired frames from the visible imagery and the alignment of the two data streams. These GCPs comprised purpose-built fire-proof steel plates that were pinned to the ground and painted in a pattern of four smaller squares of two high-contrast colours that made identification of the centre of the targets more accurate. Steel was used to allow the placement of some GCPs within the burn block. The eight GCPs for the ULS data were surveyed for approximately 3 min to obtain ~180 point fixes using a Trimble Geo7X differential GPS (Global Positioning System) unit (Trimble Inc., Sunnyvale, CA, USA) together with a Trimble Zephyr Model 2 external antenna for higher accuracy. An additional point was surveyed in the centre of the study area for a longer period to attain a highly accurate point fix. This was then used as the location for the base station of the Lidar (Light Detection and Ranging) to enable enhanced accuracy through post-processed kinematic (PPK) positioning.
Aerial video data of the flame zone was captured using a DJI Zenmuse XT2 dual thermal and RGB sensor with integrated 1/17 inch (1.49 mm) 12MP RGB camera (DJI Ltd., Shenzhen, China), mounted on a DJI Matrice 210 UAV (DJI Ltd., Shenzhen, China) (shown in Fig. 1). Video was captured in MP4 format at a frame rate of 30 fps (frames per second). Pre-burn ULS data were captured with a LidarUSA Snoopy V-series system (Fagerman Technologies, INC., Somerville, AL, USA) that incorporates a Riegl MiniVUX-1 UAV scanner (Riegl, Horn, Austria). This sensor was attached to a DJI Matrice 600 Pro UAV.
Flights for the visual capture were carried out using the DJI Pilot flight control application at a height of 250 m above ground level (AGL) to ensure full coverage of the burn block in a single frame. Flights for the ULS data capture were carried out using the UgCS flight control software (SPH Engineering, Riga, Latvia) at a height of 60 m AGL and a line spacing of 30 m to maximise characterisation of the vegetation. The resulting pulse density was 306 ppm2. UgCS software was selected owing to its ability to create flight paths that follow terrain and to build in banking turns to the ends of flight lines, which help to minimise potential errors in the sensors’ Inertial Measurement Unit (IMU).
Lidar data were converted from native LidarUSA format to the universal LAS (LASer) file format in two processing steps. Initially, the raw trajectory data from the rover (type GNSS) of the LidarUSA system were post-processed with the PPK GPS data from the CHCX900B base station (CHC Navigation, Shanghai, China) in Inertial Explorer Express (NovAtel Inc., Calgary, AB, Canada). The post-processed trajectory data were then combined with the raw sensor data in the ScanLook Point Cloud Export (ScanLook PC) software (Fagerman Technologies INC., Somerville, AL, USA), and to apply boresight calibrations and lever arm offsets. The resulting point cloud was then output in LAS format. Processing of the raw point cloud from Lidar was then carried out using two pieces of software. First, the point cloud was tiled and had basic noise filtering applied using the LasTools software version 210,418 (Isenburg 2021). This tile output from LasTools was then imported into R statistical software version 4.0.4 (R Core Team 2020) and processed using a data processing pipeline developed using the LidR library (Roussel et al. 2018). First ground points were classified and then a digital terrain model (DTM) with a resolution of 1 m was derived from these points. The point cloud was then height-normalised using the DTM, more noise filtering was applied to remove spurious points, and finally a pit-free canopy height model (CHM) with a resolution of 0.25 m was calculated.
Description of techniques
Fire perimeter tracking – rate of spread
The Fire Perimeter Tracking algorithm, explained and evaluated by Schumacher et al. (2022), and provided by Melnik (2021) was used to calculate the rate of spread (ROS) of the fire from the nadir RGB video. This method allows for high-resolution 2D pixel-wise calculation of the ROS (time-varying fire front) and further derived characteristics such as 2D maps of ROS, streamwise rate of spread (ROSy), radial rate of spread (ROSx) and spread direction (θROS). In this work, the ROS is defined as speed of propagation, or as the magnitude of the vector composed of ROSx and ROSy, which is not always exactly aligned with the experimental plot, as shown in the next section. This choice was made to reduce the uncertainties related to the misalignments between the wind direction and the plot, as well as to account for changes of fire progression direction due to heterogeneity of fuel structure.
The method is described in detail in Schumacher et al. (2022), and is summarised below. First, the video footage was stabilised using Blender (Blender Online Community 2019), and down-sampled from 30 to 1 fps. Each frame of the stabilised 1-fps video was analysed to identify which pixels contained active flames by applying manually identified colour thresholds in the hue, saturation and value (HSV) colour space. In order to calculate the pixel-wise rate of fire spread, a 41 × 41 pixel (5.3 × 5.3 m) moving window was established across the ignition array, with every pixel of the ignition array taking its turn to serve as the centre pixel of the moving window. Within the moving window, only timestamps immediately preceding or following the timestamp of the central pixel were retained. Vectors going from the preceding timestamp pixels to the central pixel and advancing from the central pixel to the following timestamp pixels were calculated, and these vectors averaged to obtain the rate and direction of spread of the central pixel. The window then moved over and the process was repeated for the next pixel in the ignition array to serve as the central pixel. The resulting array contained the computed rate and direction of fire perimeter spread for each pixel in the burn plot.
Flame residence time
Flame Residence Time (FRT) was the second fire behaviour variable calculated in this work. It was defined as the pixel-wise duration of the observable fire from the nadir RGB video acquired with the UAV. It was calculated using a technique illustrated in Fig. 2. After the stabilisation and HSV-based flame identification previously described, two 2D maps were created: (1) an ignition map in which each pixel contained the first timestamp of active flaming in the given pixel of the burn plot, and (2) an extinction map in which each pixel contained the last timestamp of active flaming. The ignition array was subtracted from the extinction array in order to create the flame residence time array, which contained the burning duration of each pixel across the burn plot. The result was a high-resolution map containing a pixel-wise duration of the observable fire.
Results and discussions
Description of fuel structure and fire spread mechanism
Fig. 3a shows an image of the fire extracted from the RGB UAV footage approximately 1 min after ignition. This figure highlights the observable flaming zone and fire front. Fire spread was characterised by rapid progressions of the fire front involving intermittent coverage and subsequent ignition of immediate preheated unburned fuel, resulting in localised progression in short periods of time. This behaviour is shown in Fig. 3a1, which highlights the change of the observable fire front during a short period of time of approximately 20 ms (white) which included instances where flames extending horizontally but then withdrew as they stood up more vertically.
Plan view of the colour sensed with the UAV-mounted RGB videos at two timesteps: (a) approximately 1 min after ignition and (b) approximately 3 min after ignition. The expanded image (a1) shows the observable fire front for two consecutive frames, with the white line illustrating the location of the observed fire approximately 20 ms after the black line.
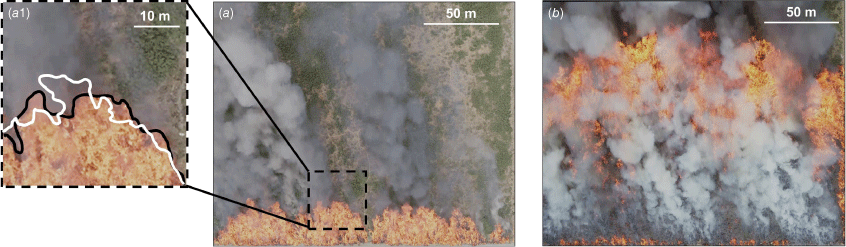
One of the main technical challenges related to the image and fire behaviour analysis was obscuration produced by the thick, white smoke emitted by the smouldering fuel behind the fire, as shown in Fig. 3b. It was found that smoke obstruction considerably hindered the identification of the flaming zone after ~160 m (~3 min after the ignition) in the streamwise direction (y axis), and reduced the quality of the ROS and residence time results, as discussed in the next section. In this work, the results associated with the entire duration of the fire, including the region affected by the smoke are reported. However, the analysis is limited to locations where clear identification of the flaming zone was achieved.
Fig. 4 shows the pre-burn CHM obtained for plot P2 from ULS data. The canopy height was heterogeneous throughout the burn plot, with interspersed zones of high and low vegetation. The mean canopy height was 0.75 m with a standard deviation 0.56 m. The right side of the plot was predominantly composed of low-height fuel with occasional areas of tall fuel, while the left side contained mostly tall vegetation with some interspersed short patches. The location of the fire front at different times after ignition is also shown in Fig. 4, depicted as black contour lines. Fire front position and development were found to be considerably influenced by local variability in fuel structure. Three regions (a., b. and c.) outlined in white in Fig. 4 highlight areas of low canopy height. Regions a. and b. exhibit a similar pattern, with fire fronts initially showing a convex structure (e.g. at 60 s) smoothly transitioning to a concave structure (e.g. at 180 s). This transition is highlighted with purple arrows in Fig. 4.
In colour (blue to red): 2D map of average height of the vegetation canopy obtained from ULS acquisitions; colour values in metres. The resolution of the Canopy Height Model (CHM) map is 7.8 × 10−2 m/pixel. In contour (black): location of fire front over time from ignition; contour values in seconds. Areas a., b. and c. (white and violet) highlight fire propagation patterns in regions of low canopy height.
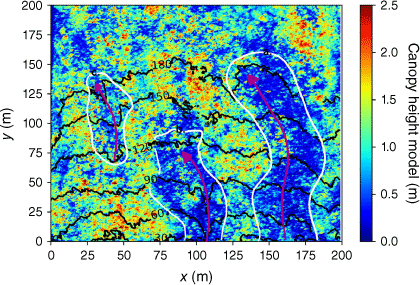
Among the different complex interactions between fuel, fire and atmosphere contributing to the aforementioned behaviour, it is hypothesised that the various morphological changes of the fire during the early stage can explain some of the observed patterns. A careful analysis of the overhead videos and of the 2D ROS map showed that the fire front evolved from an initial acceleration phase with a thin flaming zone to an established phase with a constant ROS and a deeper flaming zone during the first 20–40 m (from ~60 to ~90 s). In the initial phase, the fire spread was driven by a localised heat transfer mechanism characterised by a thin flaming zone and low burning rate. Consequently, the fire front was highly driven by fuel, resulting in localised changes of fire front as a function of the canopy height. Once the fire front was established (after 90 s), with flaming zone depth reaching up to ~50 m and an observed increase in flame heights, a wider region of vegetation was affected by the emitted radiative and convective heat flux, resulting in a less localised and more ‘connected’ fire across and along the flaming zone. This connection can be linked to the quasi-steady state in which the equilibrium between the ignition and burning rate seems to take place (as further discussed in the next section). In the case of regions a. and b., it is possible that the overall inflow of wind is modified by the fuel structure, creating a ‘channel’ effect in regions of low canopy height surrounded by taller vegetation, ultimately modifying both the speed and direction of the incoming fresh air feeding the fire front. Region c. highlighted in Fig. 4 shows an ~15 m wide canopy gap starting from y ≈ 75 m. The fire front, which reached the gap ~120 s after ignition, seems to be initially disturbed by the abrupt change in fuel height, developing a convex structure localised around the surroundings of the gap. This structure seems to be conserved during the next minute over 50 m of fire progression. This behaviour could be linked to an increase in the ignition time associated with the sudden discontinuity of the fuel structure, characterised by the heat needing to reach lower vegetation levels to achieve ignition.
The influence of the fuel structure on the spatial variability of fire spread reported in this section through the description and analysis of the CHM and fire front progression maps are further discussed in the following section using ROS and FRT as fire behaviour metrics.
Influence of canopy height on ROS and residence time
Fig. 5a, b show the maps of FRT and ROS, respectively. The low canopy height regions shown in Fig. 4 are also included in Fig. 5a for reference. On one hand, FRT ranged from 10 to 90 s, with extreme values at the plot edges where drip torch ignition occurred. Regions a., b. and c. in the FRT map are also associated with low canopy height values, which may be linked to the low amount of vegetative fuel available in these regions resulting in a limited amount of energy available for combustion. This hypothesis is strengthened by the presence of regions of high canopy height associated with high FRT (e.g. bottom left, x = 50 m and y = 25 m). On the other hand, the ROS map captured the previously described rapid progressions of the fire front in the form of localised high ROS values, which could be explained by the presence of greater flame heights and longer fire activity in regions with higher canopy height, most likely associated with higher fuel load.
Derived datasets of the burn plot where the ignition took place along the x-axis, with the x-axis showing plot width in metres, the y-axis showing the plot length in metres, and the value corresponding to: (a) the flame residence time derived from the Unmanned Aerial Vehicle (UAV) video footage, and (b) rate of fire spread also derived from the footage. The resolution of both maps is 7.8 × 10−2 m/pixel.
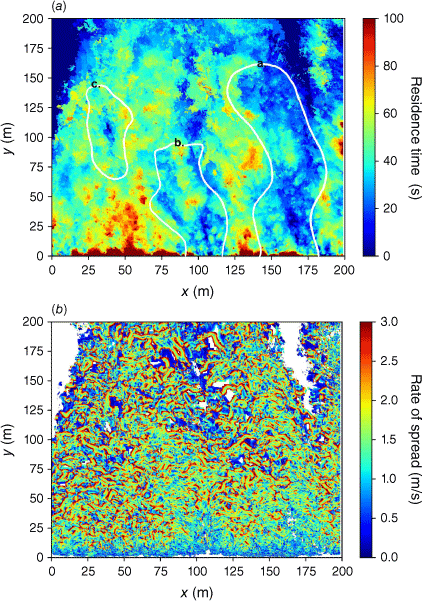
Fig. 6b shows the evolution of ROS through the streamwise direction for high canopy height (top) and low canopy height (bottom). The ROS and FRT in Fig. 6a were averaged over a flame front width of Δx = 20 m, with ±1 s.d. shown in grey. The plots are accompanied by the corresponding CHM values (blue) averaged over the same width. ROS resulting from high canopy height was found to be low during the first ~20 m in the streamwise direction, where the fire front was mainly accelerating with a progressively bigger flaming zone attached to the ignition line. After ~20 m, the fire was more established, with a quasi-steady value of ROS ~1.2 m/s and a larger flaming zone, even though the averaged canopy height from the CHM considerably decreased from ~1.6 to 1.0 m. For lower canopy heights, however, the fire seems to undergo a longer period of acceleration until ~40 m, where a maximum average ROS of ~1.0 m/s is reached. Those differences suggest that canopy height could play a relevant role on ROS during the acceleration period for the experimental conditions studied in this work.
(a) Flame residence time (FRT) and (b) rate of spread (ROS) compared with canopy height for high (top) and low (bottom) vegetation heights. The y axis represents the streamwise direction from the ignition point (y = 0 m). The black lines are the FRT and ROS averaged over a width of Δx = 20 m. The grey ribbon shows 1 s.d. for the FRT or ROS values, and the blue lines are the average canopy height.
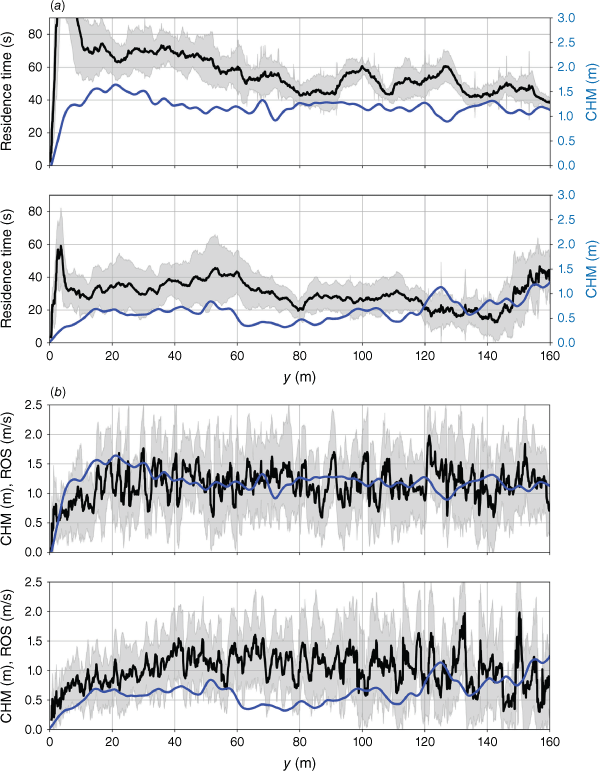
FRT, shown in Fig. 6a, displays a different behaviour and seems to be considerably more dependent on canopy height for both studied cases. This dependency is mostly perceivable over the first half of the plot. For tall vegetation (top plot), FRT progressively decreases by approximately 30% from y = 20 to 80 m, while the CHM decreases ~25% in the same range. After y = 80 m, FRT and CHM are overall both relatively constant except for some localised changes.
The same trend can be seen in the second studied case for the region of short vegetation (Fig. 6a, bottom plot), where changes in FRT values seem to be driven by variation in vegetation height during the development of the fire. This correlation, further explored in the next section, is associated with the strong linkage between the canopy height and fuel load (Pearce et al. 2010), which is supported by results from destructive sampling measurements carried out before the prescribed experimental burns (not shown in this work). Areas with high fuel load contain a greater amount of biomass available to participate in combustion, leading to longer combustion duration and FRT. However, it is particularly challenging to assess the extent to which wind, moisture content, fuel morphology and other variables simultaneously influence the ROS and FRT. For instance, wind speed may play a relevant role in increasing combustion efficiency by providing oxygen to feed the reaction, while fuel condition (dead-to-live ratio) and particle size modify the rate at which the reaction takes place. Fuel type, which captures these differences in the properties and structure associated with different vegetation types, may also play a significant role in these low canopy height areas owing to a change from shrubs to grass as the dominant fuel carrying the fire. Grass fuels are known to produce faster spread rates, especially under high wind speeds and when dead or cured, owing to the predominance of fine fuels, high proportion of dead fuel and lower moisture contents (Cruz et al. 2022). The next section provides further insight into the complex coupling between FRT, ROS and CHM addressed in this work by exploring mathematical correlations and statistical properties describing this interaction. This approach does not aim to provide a definitive explanation or description on how this interaction works, but rather intends to bring quantitative clarity on the first-order nature of this relationship.
Empirical correlations and statistical analysis
The ROS and FRT maps shown in Fig. 5 are each composed of more than 6 million data points owing to the high resolution of the acquired video and subsequent methodology applied to derive them. The datasets were initially filtered to improve readability of the results by averaging the ROS and FRT, as shown in Fig. 7. A detailed analysis of the non-filtered dataset is shown in Figs 8 and 9.
Scatter plot of Flame Residence Time (FRT) as a function of the Canopy Height Model (CHM) and rate of spread (ROS) (in colour). Results correspond to data located from x = 30 to 170 m to reduce edge effects, and from y = 30 to 90 m to ensure low smoke obscuration and a steady-state fire. The results were filtered using a 20 × 20 m2 non-overlapping square kernel on the three maps (CHM, FRT and ROS).
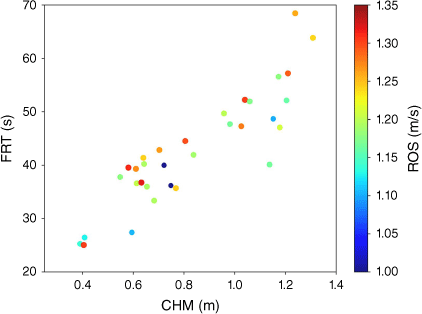
2D probability density plots of rate of spread (ROS) as a function of the Canopy Height Model (CHM) for six different locations. Plots (a–f) are shown as 2D kernel density estimations and histograms of a subsample of datapoints. The size of the regions of interest (black squares) is 20 × 20 m2.
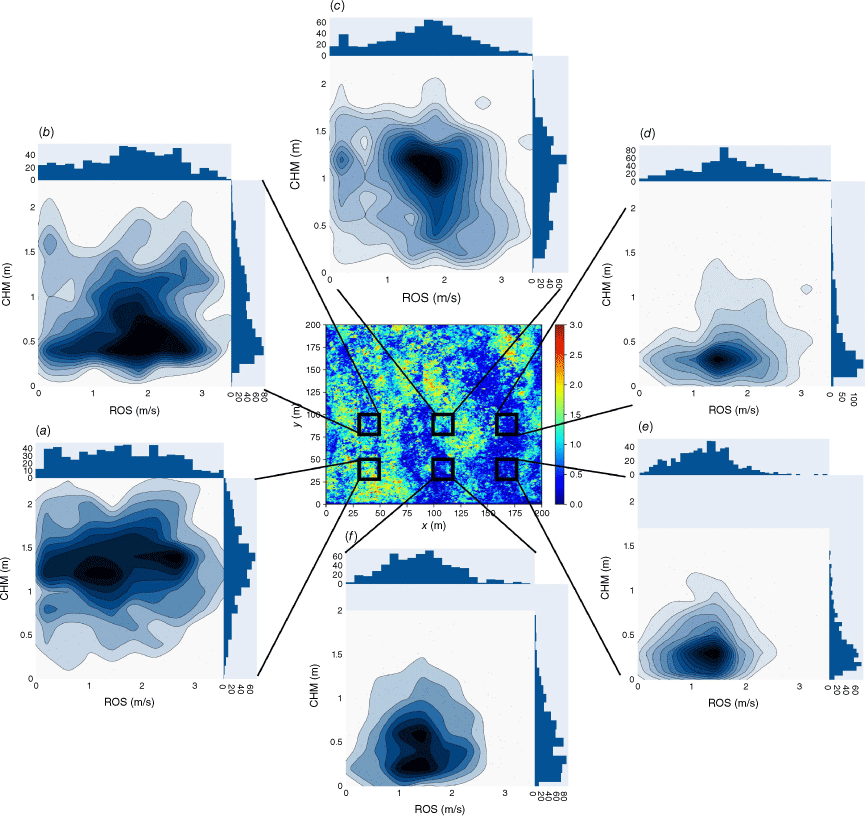
2D probability density plots of Flame Residence Time (FRT) as a function of the Canopy Height Model (CHM) for six different locations. Plots (a–f) are shown as 2D kernel density estimations and histograms of a subsample of datapoints. The size of the regions of interest (black squares) is 20 × 20 m2.
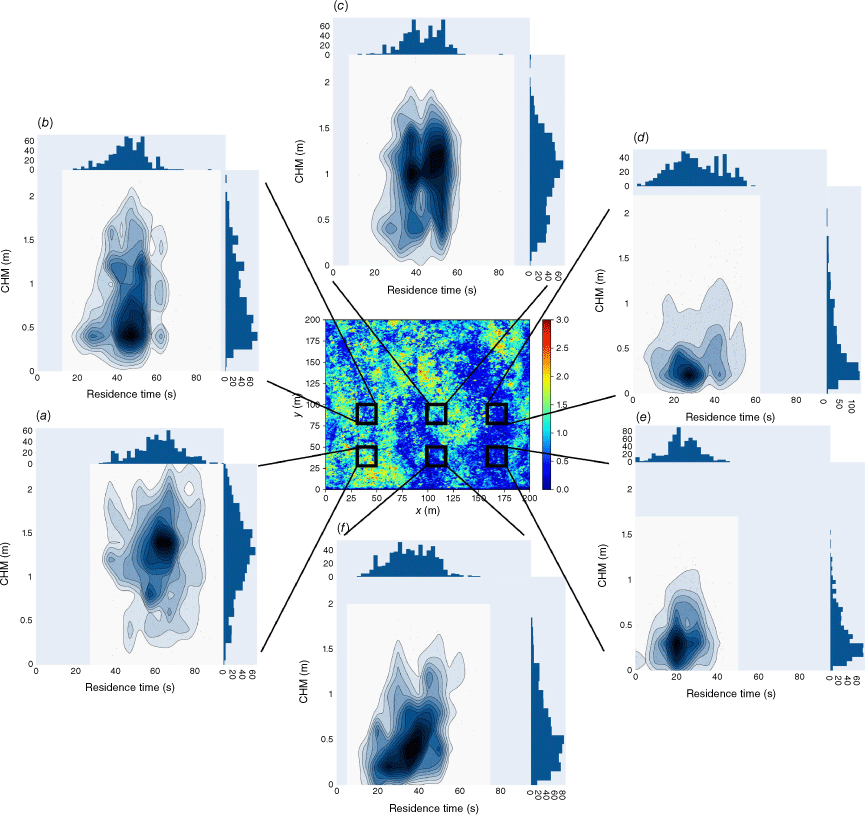
Fig. 7 shows a scatter plot of FRT and ROS (in colour) as a function of CHM. The plot was generated by applying a median filter using a basic 20 × 20 m2 non-overlapping square kernel on the three maps, accounting for 10% of the experimental plot, which was found to represent a suitable quality/size trade-off. Results correspond to data located from x = 30 to 170 m to reduce edge effects, and from y = 30 to 90 m to ensure low smoke obscuration and a steady-state fire.
A linear regression analysis was performed to explore the relationship between the average FRT and the average CHM, which yielded the following equation:
where CHM is in metres and FRT is in seconds. This equation is valid in the studied range of applicability, and yields a reasonably high regression coefficient of determination of R2 = 0.74 as expected from results shown in the previous section. Most of the dispersed points are associated with high average CHM values (>1.2 m), potentially due to dissociation between fuel load and canopy height, as well as due to uncertainties linked to smoke obscuration and the calculation method. The linear regression between the average ROS and CHM yielded the following relationship:
where ROS is in metres per second and CHM in metres. This equation is valid in the studied range of applicability provided in Fig. 7. The resulting coefficient of R2 = 0.26 is low, showing that unlike FRT, CHM alone is not a primary variable dictating the speed of progression of the fire for the studied case. Furthermore, another potential reason explaining the observed disparity involves a high dependency of ROS on the fuel structure (e.g. local changes in CHM). This hypothesis is further explored in Fig. 8, which shows a scatterplot of the non-filtered ROS versus CHM for different 20 × 20 m2 regions of interest. Owing to the large number of datapoints (64 000 points per region of interest), the plots are shown as 2D kernel density estimations and histograms of a subsample of datapoints randomly selected (1:100 or 640 datapoints). This was necessary to facilitate readability and interpretation of the results.
In general, regions with lower canopy height (average canopy height <0.5 m – plots d and e in Fig. 8) are associated with low ROS values, presenting narrow distributions centred around 1.5 m/s. Regions with higher CHM (average canopy height >1.0 m – plots a and c in Fig. 8) present wider distributions of ROS values, reaching both high magnitudes greater than 3 m/s and values close to quiescent conditions. This behaviour is associated with quick progressions or fire flickering, as previously described, involving greater flame heights and longer fire activity. Regions covering low CHM vegetation (averaged canopy height lower than 0.5 – plots d and e in Fig. 8) showed narrow distributions, suggesting that the fire progression was considerably more ‘stable’. Regions with medium CHM values (averaged canopy height between 0.5 and 1.0 m – plots b and f in Fig. 8) were found to be in a transition regime. On one hand, Figure 8f involves a low ROS region surrounded by low CHM vegetation, associated with thin flaming zones and low FRT, whereas Fig. 8b covers high ROS channel-like vegetation structure, surrounded by high CHM vegetation linked to high FRT, and associated with a considerably larger flaming zone. It is likely that these differences in fuel structure played an important role in enhancing or hindering heat transfer mechanisms associated with the rate of progression of the fire. In the case of Fig. 8b, the favourable characteristics of the surrounding vegetation can be associated with higher flames and larger flame depth, which could strengthen the radiative heat transfer required for ignition of the unburnt fuel. Additionally, it is possible that the channel-like structure enhances convective heat transfer by increasing the flow of hot gases and the surface area of exposed fuel.
Fig. 9 shows histograms and scatterplots for FRT at the locations discussed above. The distribution of FRT for all the plots is considerably narrower than in the case of ROS, showing less dispersion and in most cases a higher correlation between the variables of interest. Similarly to the ROS cases, low CHM regions are linked to low FRT values, with distributions centred between FRTs of 20 and 30 s, and high CHM regions with distributions centred between 40 and 60 s. FRT values shown in Fig. 9a are by far the highest, with FRT values ranging between 31 and 96 s. Interestingly, the results presented in Fig. 9c show a bi-modal distribution of FRT centred on 38 and 52 s, which can be associated with high heterogeneity of vegetation heights in the region of interest. In summary, results reported in Fig. 9 are in accordance with previous findings showing a clear dependency on canopy height.
Methodological uncertainties
The studied experimental burn involved considerable heterogeneities in fuel structure and fire behaviour, which resulted in high variability in the ROS, sporadic fast progressions of the fire and complex flame front ignition patterns. This, combined with obscuration from the smoke, introduced a series of methodological uncertainties that were carefully addressed in this work to assess the accuracy and the range of applicability of the techniques used to quantify the ROS and the FRT.
First, the uncertainties linked to the derivation of the ROS from fire front position were quantified. This was achieved by implementing the methodology illustrated in Fig. 10a to estimate the average location of the fire front in the streamwise direction y. This methodology included: (i) calculating the average streamwise ROS (ROSy), and (ii) calculating the average location of the fire front yf by integrating ROSy over time. The result was compared with the observed fire front location measured from the nadir videos and the estimate of the error was obtained by comparing both quantities. Fig. 10b shows both the ‘calculated’ (from ROS maps) and the ‘measured’ (directly from nadir videos) fire front location over time, as well as the estimated error. The error oscillates between 0 and 16% depending on the location of the fire, with an average value of 5.25%, which is suitable for the purposes of this work. As expected, the error was found to increase considerably with the video frame rate owing to the quick and intermittent fire progressions. For instance, at 3 fps, the average error was 50%, whereas for the original frame rate (30 fps), the average error surpassed 100%. A frame rate of 1 fps was chosen and a correction factor of 0.61 was used to minimise the error. This approach allowed a suitable trade-off between accuracy and the temporal resolution required for the quality of the results reported.
(a) Illustration of methodology used to estimate uncertainties of rate of spread (ROS) results, where the colours and shades represent relative changes in pixel ROS from slow (blue) through intermediate (green) to faster (yellow and orange) spread rates. (b) Evolution of average fire front location (yf) over time derived from the nadir videos (blue) and calculated using the averaging methodology presented in in (a) above (dark grey), with percentage error (red).
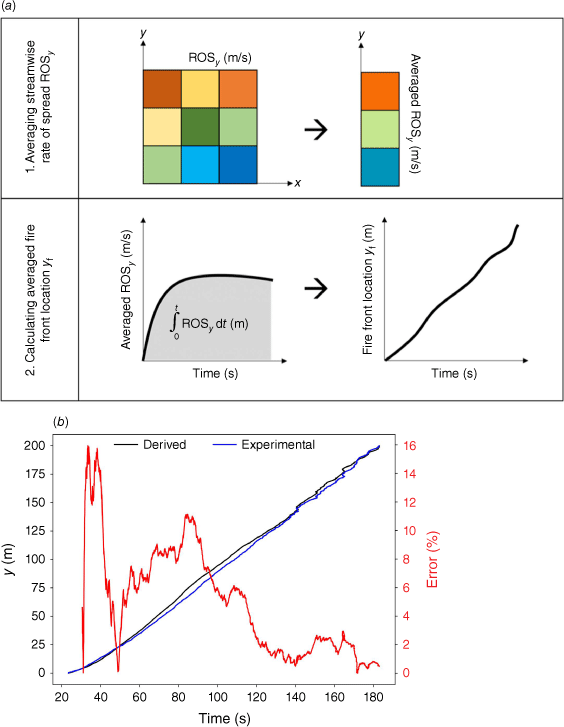
Concerning the FRT, the methodology involves well-known image processing approaches using high-resolution videos and low uncertainties linked to the method per se were therefore expected. The main source of uncertainty resulted from the obscuration produced by the thick, white smoke emitted from the smouldering fuel behind the fire. The strategy put in place to mitigate this issue was to limit the region of study down to 40% of the studied plot, where the flaming zone could be clearly identified throughout the duration of the burn.
Similarly, the ignition pattern used to light the burn plot could have had an influence on the ROS and FRT observed. However, the use of multiple igniters each lighting small sections of the main ignition line ensured a continuous fireline was established quickly, within 1 min of ignition commencing. The subsequent ignition along the sides of the plot to maintain a straight-line fire front is also considered to have had minimal influence on the results presented here, with analyses focused on the more central areas of the burn plot (x = 30–170 m; see Figs 7–9) where the flame front is unlikely to have been affected by these edge effects.
Discussions and conclusions
Detailed measurements of fire behaviour of a 4-ha prescribed gorse fire are reported in this work, and then used to study the influence of the heterogeneity of the fuel structure on fire behaviour. Results and corresponding analysis from this work have implications principally for wildfire modellers and WUI building design engineers, as the reported experimental dataset can be used for model validation and the associated analysis contributes to further understanding of the level of hazard associated with gorse fires.
In terms of contribution to the emerging field of WUI building design engineering, reduced-order models for hazard calculations are currently widely used in regulations (WAPC 2017; SAI-Global 2018; NSW Rural Fire Service 2019) to estimate the level of exposure of the built environment to fire. In this context, ROS plays a major role in calculations of fire severity in the WUI environment, as ROS relates to the intensity of the fire and the level of exposure. For instance, methods for designing fire suppression and people evacuation protocols make use of ROS, as it provides key information for estimating heat flux exposure levels and time available to evacuate (Penney and Richardson 2019). Generally, and for practical purposes, ROS is assumed to be constant and dependent on several first-order averaged variables such as wind strength, plant species, canopy height and fuel load. The results presented in this work show that this assumption is reasonable for gorse fires once the steady-state phase of fire spread is achieved, but also that the acceleration phase is not negligible, as it was found to cover the first 20–40 m depending on the canopy height. Similarly, the results discussed show that fire progression under the specific experimental wind conditions is considerably more unpredictable and intermittent in regions of high canopy height, behaviour that can hinder suppression efforts and planning. A similar behaviour was observed in channel-like structures composed of low CHM covered by high vegetation (width ~15 m), suggesting that these types of complex fuel structures can be associated with higher local values of the level of hazard. Additional investigation is required to further assess the role of these structures in fire behaviour. FRT is also a key variable used in WUI building design. Unfortunately, the lack of literature on FRT for bushland species has limited its full implementation in WUI building design severity calculations. Our analysis showed that FRT reached values up to 90 s, and that it is a function of canopy height following Eqn 1 in the range of applicability of the prescribed burn studied here. These results can be used for estimating shrubland fire exposure duration and in severity calculations in general. It is worth noting that FRT should not be confused with the total reaction time, as it only represents the time of flaming combustion, excluding other type of reactions such as smouldering combustion. FRT is essential primarily for tenability calculations as it provides an estimate of the exposure time, which is critical for structure ignition estimation (Vacca et al. 2020) and thermal heat dose for both responders and people evacuating (Penney et al. 2019). The results also provide a suitable dataset for validating Computational Fluid Dynamics (CFD) wildland fire simulation tools. Generally, variables such as fire front location and averaged ROS are used as metrics for model performance assessment. Some of the reported results can help expand these metrics to include fire behaviour characteristics (e.g. ROS and FRT histograms as a function of canopy height) in order to assess the model simulation results for coupled fuel–fire interactions in heterogeneous fuel loading settings.
Several future areas of research are expected to proceed from the work presented here. More specifically, this paper demonstrates that geospatial analytics from UAV platforms can provide precision observations of ROS and FRT, expanding opportunities to study other fire behaviour metrics. In this context, a future focus involves further developing the reported methods to allow incorporation of fuel load maps from CHM into fire intensity estimation and mapping, which is considered an important descriptor for wildfire management (Johnston et al. 2017). Future work also involves extending the results to include the additional experimental burn plots that took place under different weather conditions, allowing the further study of fire behaviour dependency on canopy height, as well as the role of wind and fuel moisture in these processes. Finally, one limitation of this work was the obscuration produced by the thick, white smoke emitted from the smouldering fuel behind the fire. In future study, we aim to assess the ability of nadir infrared videos acquired during the experimental campaign to improve the quality of the ROS and FRT measurements, with emphasis on extending the effective measurement time and enhancing the identification of flaming zone surface area throughout the duration of the fire.
Nomenclature
Variable | Symbol (Units) |
Canopy height model or vegetation height from Unmanned Laser Scanning. | CHM (m) |
Flaming residence time or duration of observable flaming combustion | FRT (s) |
Rate of spread or fire propagation speed | ROS (m/s) |
Streamwise rate of spread or fire propagation speed in y direction | ROSy (m/s) |
Radial rate of spread or fire propagation speed in the x direction | ROSx (m/s) |
Time from ignition | t (s) |
Radial direction relative to the burn plot | x (m) |
Streamwise direction relative to the burn plot | y (m) |
Fire front location in the y direction | yf (m) |
Data availability
The data that support the findings of this study are available on request from the corresponding author.
Declaration of funding
This research was co-funded by the Ministry of Business, Innovation and Employment (MBIE), New Zealand, grant number C04X1603 entitled ‘Preparing New Zealand for Extreme Fire’, grant number C04X2103 ‘Extreme wildfire: Our new reality – are we ready?’ and the Royal Society of New Zealand grant number RDF-UOC170. MBIE was not involved in the preparation of the data or the manuscript or the decision to submit the publication.
Acknowledgements
We would like to thank to all the field support teams including technical and general staff who assisted with data collection. The research team would especially like to acknowledge the very well organised and proactive support received from all the volunteer firefighting crews and fire agency personnel from Fire Emergency New Zealand (FENZ). We also thank the landowner for their contribution leading to the success of the field campaign. A special thanks to the Scion field crew: David Glogoski, Max Novoselov, Richard Parker, Brooke O’Connor, Emma Percy and Ilze Pretorius. We would also like to acknowledge Peter Massam from Scion’s UAV team for assistance with UAV data capture and processing.
References
Beer T (1991) Bushfire rate‐of‐spread forecasting: Deterministic and statistical approaches to fire modelling. Journal of Forecasting 10, 301-317.
| Crossref | Google Scholar |
Blender Online Community (2019) ‘Blender - a 3D modelling and rendering package.’ (Stichting Blender Foundation, 508 Blender Institute: Amsterdam) Available at http://www.blender.org
Canfield JM, Linn RR, Sauer JA, Finney M, Forthofer J (2014) A numerical investigation of the interplay between fireline length, geometry, and rate of spread. Agricultural and Forest Meteorology 189–190, 48-59.
| Crossref | Google Scholar |
Ciullo V, Rossi L, Pieri A (2020) Experimental fire measurement with UAV multimodal stereovision. Remote Sensing 12, 3546.
| Crossref | Google Scholar |
Clements CB, Zhong S, Goodrick S, Li J, Potter BE, Bian X, Heilman WE, Charney JJ, Perna R, Jang M, Lee D, Patel M, Street S, Aumann G (2007) Observing the dynamics of wildland grass fires. Bulletin of the American Meteorological Society 88, 1369-1382.
| Crossref | Google Scholar |
Cruz MG, McCaw WL, Anderson WR, Gould JS (2013) Fire behaviour modelling in semi-arid mallee-heath shrublands of southern Australia. Environmental Modelling & Software 40, 21-34.
| Crossref | Google Scholar |
Cruz MG, Alexander ME, Kilinc M (2022) Wildfire rates of spread in grasslands under critical burning conditions. Fire 5, 55.
| Crossref | Google Scholar |
Dahale A, Ferguson S, Shotorban B, Mahalingam S (2013) Effects of distribution of bulk density and moisture content on shrub fires. International Journal of Wildland Fire 22, 625-641.
| Crossref | Google Scholar |
Fayad J, Rossi L, Frangieh N, Awad C, Accary G, Chatelon FJ, Morandini F, Marcelli T, Cancellieri V, Cancellieri D, Morvan D, Pieri A, Planelles G, Costantini R, Meradji S, Rossi JL (2022) Numerical study of an experimental high-intensity prescribed fire across Corsican Genista salzmannii vegetation. Fire Safety Journal 131, 103600.
| Crossref | Google Scholar |
Finney MA, Cohen JD, Forthofer JM, McAllister SS, Gollner MJ, Gorham DJ, Saito K, Akafuah NK, Adam BA, English JD (2015) Role of buoyant flame dynamics in wildfire spread. Proceedings of the National Academy of Sciences 112, 9833-9838.
| Crossref | Google Scholar |
Finney MA, Pearce G, Strand T, et al. (2018) New Zealand prescribed fire experiments to test convective heat transfer in wildland fires. In Viegas DX (ed) ‘Advances in Forest Fire Research 2018’ 1288-1292.
| Crossref | Google Scholar |
Hartley RJL, Davidson SJ, Watt MS, Massam PD, Aguilar-arguello S, Melnik KO, Pearce HG, Clifford VR (2022) A mixed methods approach for fuel characterisation in gorse (Ulex europaeus L.) scrub from high-density UAV laser scanning point clouds and semantic segmentation of UAV imagery. Remote Sensing 14, 4775.
| Crossref | Google Scholar |
Hoffman CM, Canfield J, Linn RR, Mell W, Sieg CH, Pimont F, Ziegler J (2016) Evaluating crown fire rate of spread predictions from physics-based models. Fire Technology 52, 221-237.
| Crossref | Google Scholar |
Isenburg M (2021) LAStools-efficient LiDAR processing software (version 210418). Available at lastools.github.io [accessed on 10 October 2021]
Johnston JM, Wooster MJ, Paugam R, Wang X, Lynham TJ, Johnston LM (2017) Direct estimation of Byram’s fire intensity from infrared remote sensing imagery. International Journal of Wildland Fire 26, 668-684.
| Crossref | Google Scholar |
Katurji M, Zhang J, Satinsky A, McNair H, Schumacher B, Strand T, Valencia A, Finney M, Pearce G, Kerr J, Seto D, Wallace H, Zawar‐Reza P, Dunker C, Clifford V, Melnik K, Grumstrup T, Forthofer J, Clements C (2021) Turbulent thermal image velocimetry at the immediate fire and atmospheric interface. Journal of Geophysical Research: Atmospheres 126, e2021JD035393.
| Crossref | Google Scholar |
Katurji M, Noonan B, Zhang J, Valencia A, Shumacher B, Kerr J, Strand T, Pearce G, Zawar-Reza P (2022) Atmospheric turbulent structures and fire sweeps during shrub fires and implications for flaming zone behaviour. International Journal of Wildland Fire 32, 43-55.
| Crossref | Google Scholar |
Linn RR, Cunningham P (2005) Numerical simulations of grass fires using a coupled atmosphere–fire model: Basic fire behavior and dependence on wind speed. Journal of Geophysical Research: Atmospheres 110, D13107.
| Crossref | Google Scholar |
Martins Fernandes PA (2001) Fire spread prediction in shrub fuels in Portugal. Forest Ecology and Management 144, 67-74.
| Crossref | Google Scholar |
Mell W, Jenkins MA, Gould J, Cheney P (2007) A physics-based approach to modelling grassland fires. International Journal of Wildland Fire 16, 1-22.
| Crossref | Google Scholar |
Melnik K (2021) Fire perimeter tracking. Available at https://doi.org/10.5281/zenodo.5138773
Morvan D (2011) Physical phenomena and length scales governing the behaviour of wildfires: a case for physical modelling. Fire Technology 47, 437-460.
| Crossref | Google Scholar |
Morvan D, Méradji S, Accary G (2009) Physical modelling of fire spread in grasslands. Fire Safety Journal 44, 50-61.
| Crossref | Google Scholar |
NSW Rural Fire Service (2019) Planning for Bush Fire Protection (PBP). Available at https://www.rfs.nsw.gov.au/__data/assets/pdf_file/0008/4400/Complete-Planning-for-Bush-Fire-Protection-2006.pdf
Page WG, Butler BW (2017) An empirically based approach to defining wildland firefighter safety and survival zone separation distances. International Journal of Wildland Fire 26, 655-667.
| Crossref | Google Scholar |
Pearce HG, Anderson WR, Fogarty LG, Todoroki CL, Anderson SAJ (2010) Linear mixed-effects models for estimating biomass and fuel loads in shrublands. Canadian Journal of Forest Research 40, 2015-2026.
| Crossref | Google Scholar |
Penney G, Richardson S (2019) Modelling of the radiant heat flux and rate of spread of wildfire within the urban environment. Fire 2, 4.
| Crossref | Google Scholar |
Penney G, Habibi D, Cattani M (2019) Firefighter tenability and its influence on wildfire suppression. Fire Safety Journal 106, 38-51.
| Crossref | Google Scholar |
Penney G, Habibi D, Cattani M (2020) ‘A handbook of wildfire engineering: guidance for wildfire suppression and resilient urban design.’ (Bushfire and Natural Hazards CRC: Melbourne) Available at https://www.bnhcrc.com.au/publications/handbook-of-wildfire-engineering
Penney G, Baker G, Valencia A, Gorham D (2022) The CAED Framework for the development of performance‐based design at the wildland–urban interface. Fire 5, 54.
| Crossref | Google Scholar |
R Core Team (2020) ‘R: A Language and Environment for Statistical Computing.’ (R Foundation for Statistical Computing: Vienna, Austria) Available at https://www.r-project.org/
Rossi L, Molinier T, Akhloufi M, Tison Y, Pieri A (2010) A 3D vision system for the measurement of the rate of spread and the height of fire fronts. Measurement Science and Technology 21, 105501.
| Crossref | Google Scholar |
Sánchez-Monroy X, Mell W, Torres-Arenas J, Butler BW (2019) Fire spread upslope: Numerical simulation of laboratory experiments. Fire Safety Journal 108, 102844.
| Crossref | Google Scholar |
Santoni PA, Simeoni A, Rossi JL, Bosseur F, Morandini F, Silvani X, Balbi JH, Cancellieri D, Rossi L (2006) Instrumentation of wildland fire: Characterisation of a fire spreading through a Mediterranean shrub. Fire Safety Journal 41, 171-184.
| Crossref | Google Scholar |
Schumacher B, Melnik KO, Katurji M, Zhang J, Clifford V, Pearce HG (2022) Rate of spread and flaming zone velocities of surface fires from visible and thermal image processing. International Journal of Wildland Fire 31, 759-773.
| Crossref | Google Scholar |
Sullivan AL (2017) Inside the Inferno: Fundamental Processes of Wildland Fire Behaviour: Part 2: Heat Transfer and Interactions. Current Forestry Reports 3, 150-171.
| Crossref | Google Scholar |
Vacca P, Caballero D, Pastor E, Planas E (2020) WUI fire risk mitigation in Europe: A performance-based design approach at home-owner level. Journal of Safety Science and Resilience 1, 97-105.
| Crossref | Google Scholar |
WAPC (2017) Guidelines for Planning in Bushfire Prone Areas. (Western Australian Planning Commission: Perth, Western Australia) Available at https://apo.org.au/node/102666