Comparison of temperature and humidity measurements from two separate weather stations at Camden Airport, Australia
Matthew L. Riley
A
Abstract
In Australia, meteorological measurements from air quality monitoring networks are an overlooked source of data for urban climatology and meteorology research and operations. The reasons for this are not clear but may include uncertainty in the quality of the observations. Here I compare over 1 million 1-min near surface air temperature (n = 516,334) and relative humidity (n = 516,717) measurements from two distinct observational stations at Camden Airport in New South Wales (NSW), Australia – the Bureau of Meteorology automatic weather station (AWS) and the NSW Department of Climate Change, Energy, the Environment and Water’s air quality monitoring station (AQMS). Annual mean bias in the AQMS temperature measurements was −0.14°C. There were seasonal and diurnal variations in temperature bias, with monthly mean bias varying from −0.27 to +0.06°C and mean hourly bias varying between −0.39 and +0.11°C. Annual mean bias in AQMS humidity measurements was −0.37%, monthly mean bias varied from −2.21 to +1.44% and bias in mean hourly measurements varied between −2.64 and +2.66%. Temperature and humidity mean biases were both within the range of the combined instrument uncertainties. The seasonal and diurnal signal in the bias is likely due to differences in instrument siting and the different radiation shields (Stevenson and multi-plate). This analysis suggests that temperature and humidity measurements from the NSW AQMS are of high quality. The performance of the AQMS measurements matches the AWS measurements and, for most circumstances, the temperature and humidity measurements are comparable. Urban climatologists and meteorologists should consider data from air quality networks in their research and can use this data with confidence.
Keywords: air quality monitoring station, AQMS, automatic weather station, AWS, humidity measurement, intercomparison, temperature measurement, thermometer screen.
1.Introduction
To better understand atmospheric processes that influence air quality, environmental management agencies in sub-national jurisdictions have long acquired meteorological measurements within their air quality monitoring networks. Typically, air quality monitoring stations and sites (AQMS) in Australia collect horizontal wind, temperature and humidity data, with many also measuring rainfall, pressure and radiation (e.g. total solar and net). Although the design requirements and siting of AQMS differ from World Meteorological Organisation (WMO) guidance for national meteorological and hydrological agencies, measurements from AQMS may nevertheless provide valuable additional information to climate and weather researchers and forecasters working in urban and peri-urban environments.
Air quality monitoring networks generally focus on measurements of air pollutants within urban environments and are most typically concerned with micro- or meso-scale processes (Seigneur 2019; Pérez et al. 2020). Similarly, much urban climate research focusses on micro- to meso-scale phenomena (e.g. urban heat-island effect, sea–land breeze circulation and mechanics of the urban canopy layer) (Oke, 2002; Mills 2014; Naserikia et al. 2023). High-quality observations at these scales and locations can deliver multiple benefits and is often cited as an area for further research activity (Hamdi et al. 2020; Lucas‐Picher et al. 2021). For example, modern weather forecasting systems increasingly use data assimilation to improve or enhance forecasts (Lean et al. 2021; de Rosnay et al. 2022) and support warning systems for high-impact weather (Zhang et al. 2019), with a growing focus on urban–city forecasts (Rennie et al. 2022). However, as modelling and forecasting systems move to finer resolutions to better capture high-impact weather events, the need for more fine-scale data to support model development, verification and evaluation increases (Marsigli et al. 2021; Koopmans et al. 2023).
The benefits of incorporating data from second- and third-party networks into the data ecosystems of national meteorological services has been long recognised (Dutton 2002; National Research Council 2009; Stalker et al. 2013) and the WMO’s vision for the WMO Integrated Global Observing System (WIGOS) in 2040 adopts a tiered approach to observing network design, with observations integrated across different levels of performance (World Meteorological Organisation 2019). Much work has focussed on the assessment of the quality of data from crowd-sourced observations (Nipen et al. 2020; Garcia-Marti et al. 2023) or on automated quality control processes for temperature data from these networks (Båserud et al. 2020; Li et al. 2024). In Australia, the Bureau of Meteorology’s third-party automatic weather stations (TPAWS) program has recently investigated approaches to evaluate the quality of daily maximum and minimum temperature from third-party networks (TPNs) using synthetic data and a case study from Western Australia (Li et al. 2024).
Even though there is a clear overlap between urban climate research, air pollution research and observational systems supporting each, in Sydney there is limited evidence of urban climatologists systematically using meteorological observations from air quality monitoring networks. A search of recent (2017–2023) research in the Web of Science Core Collection on keywords ‘heat waves’ or ‘urban heat’ and ‘Sydney’ returned 63 articles, of which 40 included observations from Sydney, but only 9 of these used observations from AQMS. Even when the aim of the research was to identify micro- to meso-scale meteorological phenomena (Santamouris et al. 2017; Hirsch et al. 2021; Khan et al. 2021), rarely did researchers use AQMS observations. Of interest is that of the nine articles that used AQMS observations, five included assessments of air pollution (e.g. Ulpiani et al. 2021; Ulpiani et al. 2022), and all involved investigators from the University of New South Wales, perhaps indicating that an awareness of the local AQMS observations assists urban climate researchers in their choices of observations.
Another reason that researchers may not be using freely and readily available meteorology data from air quality monitoring networks is a lack of confidence in the measurements. Even though meteorological measurements from AQMS are often governed by strict siting and instrumentation requirements, researchers may not have confidence in the measurements from AQMS given their different siting requirements compared with AWS. Consider for example the information from the WMO in its Guide to Instruments and Methods of Observations Volume I – Measurement of Meteorological Variables (World Meteorological Organisation 2021a). The AQMS usually comprise a building with regulated internal temperature (i.e. air conditioning or heating), meaning that under the classifications in the WMO guide for temperature observations, the siting typical of AQMS would rarely meet the classifications of a Class 1–3 station and most likely would be classified as a Class 4 station. The guide notes that this classification can lead to ‘additional estimated uncertainty added by siting up to 2°C’ (World Meteorological Organisation 2021a, section 2.5, p. 48), a range likely inconsistent with data quality requirements for many urban climate studies. However, I am not aware of any published research that compares the performance of AQMS and AWS in Sydney, or elsewhere in Australia, that would support that assessment of temperature measurement uncertainty due to AQMS siting. This analysis will begin to address this.
This research does not seek to understand the reasons why researchers are overlooking AQMS meteorological observations in NSW. Nor is the aim to classify appropriate uses for AQMS observations or to assess the accuracy of the Bureau of Meteorology’s measurements. The research also does not seek to systematically assess what methods may be appropriate to integrate measurements from different, professionally operated networks into Bureau of Meteorology observations or assimilation systems. Rather the analysis aims to assess if the temperature and humidity measurements from the NSW AQMS are sufficiently robust to be considered more explicitly in urban climate research design, data assimilation in operational forecasting systems or as independent observations for use in model or forecast evaluation studies. Quantifying the biases in the AQMS temperature and humidity measurements with respect to the AWS measurements, and characterising those in the context of stated measurement uncertainties, provides the information that researchers may need to assess if temperature and humidity measurements from AQMS are suitable for their needs. Additionally, this analysis may assist in highlighting the existence of robust observations from AQMS, bringing attention to this over-looked source of urban climate data.
2.Measurements and methods
2.1. Site context, measurement locations and comparison period
The Camden Airport is located ~50 km south-west of the Sydney CBD at an elevation of 70 m in a semi-rural area. The airport is close to a large meander in the Nepean River with the Nepean Valley generally aligned north-to-south. To the west lies the foothills of the Great Dividing Range and elevation increases to over 600 m within 18 km of the airport. Major peaks of the range exceed 1300-m elevation ~70 km west of the airport. The Tasman Sea is ~35 km to the south-east, separated by low, forested hills and a coastal escarpment of ~275-m elevation. Camden Airport has a Köppen classification of humid subtropical (Cfa). Long-term climate statistics show annual mean maximum temperature of 23.8°C and mean minimum of 10.3°C. Annual mean rainfall is 797 mm, warm season dominant. Mean daily solar exposure ranges from 22.5 MJ m–2 in December to 8.8 MJ m–2 in June. Mean annual 09:00- and 15:00-hours wind speeds are 1.9 and 4.4 m s−1 respectively (Bureau of Meteorology 2024).
The Bureau of Meteorology station is an airport meteorological station that has operated since 1943, first operating with daily 09:00-hours observations until December 1971 and at least 09:00- and 15:00-hours observations from then onwards. The site has operated as an AWS (Site number 068192, latitutde 34°02′21″S, longitude 150°41′21″E) since June 1997 (Bureau of Meteorology 2023). Roughness length is assumed to be ~0.1 m (Wieringa 1980). Site details are provided in Table 1.
AQMS | AWS | ||
---|---|---|---|
Site ID | 2570 | 068192 | |
Location | 34°02′30″S, 150°41′25″E | 34°02′21″S, 150°41′21″E | |
Altitude (m) | 74 | 74 | |
Operational | December 2012–present (1 min) | June 1997–present (hourly) | |
Aug 2010–present (1 min) | |||
Classification | NEPM Performance | Synoptic, Aeronautical |
The Camden AQMS was established in December 2012. The AQMS is located ~300 m SSW (bearing 162°) from the AWS (Fig. 1) and comprises a temperature controlled portable building, fencing and a meteorological mast of 10-m height. The station is classified as a performance monitoring station under the National Environment Protection (Ambient Air Quality) Measure (NEPM, see https://www.dcceew.gov.au/environment/protection/air-quality/ambient-air-quality-nepm). Surface characteristics vary somewhat between the sites as the siting requirements for air quality meteorology are less strict than the WMO guidelines for synoptic and aeronautical stations. In particular, the AQMS is situated closer to low buildings (<5-m height) and low trees (<10-m height) than the AWS. The AQMS also houses a reverse-cycle air conditioner for ensuring internal temperature control of the portable building, which is a source of artificial heat. The AQMS broadly fits the Bureau of Meteorology criteria of a Special Purpose Station (Bureau of Meteorology 2013). Applying the WMO Siting Classification to the AQMS shows the site would be classified as Class 4 for temperature and Class 6 for wind (World Meteorological Organisation 2021a), with roughness length (z0) of ~0.5 m (Wieringa 1980).
The analysis focusses on 1-min averages from each station over a single complete calendar year from 1 January 2019 through to 31 December 2019, providing a potential 525,600 observations for comparison for each of temperature and humidity. All times are given in Australian Eastern Standard Time. Full-year comparison data are available from 2013 onwards and this analysis could have considered using multiple years or the full comparison period. However, quality assurance (QA) processes at the AQMS (see below) require the temperature and humidity instrument to be swapped out annually, which potentially introduces another aspect of uncertainty in this comparison. To reduce this, I focus on the single year of 2019 where the instrument swap occurred on 18 December 2019, minimising any major influence on results from inter-instrument variability at the AQMS. The year assessed here included some extreme conditions (see Results) and the periods of high temperatures and low rainfall observed in the latter may affect the extrapolation of these results to other climate conditions.
2.2. Instrumentation
Both systems use a single combined sensor with thin-film capacitance for humidity measurements and platinum resistance thermometers for temperature observations. Both sensors operate with Teflon filter caps.
The AWS houses a Rotronic MP101 (Rotronic AG, Switzerland). The manufacturer reports accuracy for temperature measurements of ±0.3°C and ±1.0% for relative humidity (RH) (Table 2). Response time (without the filter cap) for the Rotronic is given as 10 s but will be much longer with the filter cap in place as deployed (Payne et al. 2002). The instrument is housed in a standard non-aspirated Stevenson screen at ~1.2 m AGL.
AQMS – Vaisala HMP155D | AWS – Rotronic MP101a | ||||
---|---|---|---|---|---|
Temperature | Relative humidity | Temperature | Relative humidity | ||
Type | Pt100 Class F | Thin-film capacitor | Pt100 Class B | Thin-film capacitor | |
Range | −80 to +60°C | 0−100% | −40 to +60°C | 0−100% | |
Accuracy | ±(0.18 – 0.003t)°C (<20°C) | ±(1.0 + 0.01RH) | ±0.3°C (20–25°C) | ±1.0% (20–25°C) | |
±(0.07 + 0.003t)°C (>20°C) | ±1.7% (RH ≥ 90%) | ||||
Response time | 20 s (63%) | 20 s (63%) | 10 s | 10 s | |
60 s (90%) | 60 s (90%) |
The AQMS uses a Vaisala HMP155D (Vaisala Oyj, Finland) for the measurement of RH and temperature. Humidity measurement is by a thin-film polymer capacitance sensor (HUMICAP R2) whereas temperature measurements are from a resistive platinum sensor (Pt100 RTD, Class F 0.1 IEC 60751). The manufacturer reports accuracy of temperature measurements of ~0.1°C at 20°C and ±(1.0 + 0.01RH)% when RH is below 90%. The response time (with the filter cap) is given as 20 s for 63% (1 − e−1) and 60 s for 90% response. The instrument is housed in a non-aspirated radiation shield (Vaisala DTR500) at a height of ~4 m above ground on an arm that protrudes ~1.5 m beyond the wall of the portable building and away from the air conditioning unit.
Temperature and humidity measurements at the AQMS are taken at 2 Hz and averaged over the full minute. This varies from the Bureau of Meteorology AWS approach in which the last 1-Hz measurement taken in the minute is logged as the mean over the minute (Ayers 2019). However, Ayers and Warne (2020) show that there is little variance between different approaches to averaging high frequency temperature measurements, due mainly to the time response rates of the sensor.
2.3. QA procedures
The temperature and humidity sensors at the AQMS are calibrated annually at the NSW Department of Climate Change, Energy, the Environment and Water Calibration Reference Laboratory (National Association of Testing Authorities (NATA) Accreditation 14209). The RH is calibrated using a chilled mirror dew point sensor (Omega RHCL-2, Omega Engineering, Inc., CT, USA) traceable to National Institute of Standards and Technology (NIST) standards for dry bulb temperature and dew point transfer standards. Humidity calibrations are conducted typically at 15, 50 and 80% with acceptance limits of ±2%. The AQMS temperature sensors are calibrated using a fluid bath (Fluke Calibration 7102 Micro-Bath, Fluke Corporation, WA, USA) with typical set points of 2, 23 and 45°C and acceptance limits of 0.3°C.
To minimise environmental degradation of the instrument in the field (Matsuguchi et al. 2000), the AQMS sensors are swapped annually using a two-step process. Firstly, before removing an instrument a comparison with an in situ measurement from a transfer standard is taken. After installing the new instrument and allowing some time to reach thermal equilibrium, a test is performed at ambient temperature against the same transfer standard. The removed instrument is returned to the laboratory for an initial three-point test under laboratory conditions. After characterising the results, the instrument is cleaned and re-calibrated before being added again to the available instrument pool. In this study the swap occurred on 18 December 2019 (S/N G2610037 to S/N H1540026).
Scheduled maintenance includes monthly visual inspection of the radiation shield and sensor, cleaning when necessary (Richardson et al. 1999). Additionally, every 6 months an in-field single point precision check under ambient conditions is completed where the station sensor performance is compared to a portable reference instrument. Acceptance limits for this test are ±0.6°C for temperature and for RH ±5% (at RH < 35%) or ±7% (RH ≥ 35%). The AQMS radiation shield is replaced annually with a fully serviced screen, minimising the occurrence of environmental degradation that can affect shield radiation reflectance (Richardson et al. 1999).
Automated QA initially occurs at datalogging, flagging any communication issues and recording status flags from the sensor. A series of plausibility checks including measurement ranges and step changes is also performed. Further checks on 1-min data beyond the automated processes are not routinely performed for the AQMS, with QA focused on hourly data to align with air quality monitoring requirements. Consequently, some invalid data can be passed through the 1-min data system. For this study, additional QA of the 1-min data was undertaken, which identified invalid data on some occasions due primarily to interruptions to power supply and datalogger communications.
2.4. Uncertainty of the measurements
Data are assessed against expanded uncertainty estimates from the sensors and the measurement systems. The uncertainty (Δ) of a measurand (x) is calculated using the root of the sum of the individual uncertainties squared:
The major system uncertainties are due to sensor siting, housing and data handling. For the AWS measurement system, I assume that the system uncertainties are nil, treating the AWS measurement system as a reference. This infers no uncertainty in the temperature and humidity measurements due to calibration uncertainty, which certainly exists as no calibration method is without uncertainty. It assumes no systematic uncertainties in the AWS measurements due to instrument siting, data sampling and data averaging and no uncertainties due to the Stevenson screen, even though non-aspirated Stevenson screens have systematic biases (Brandsma and Van der Meulen 2008; Hoover and Yao 2018). This choice is driven by the aim of this work, which is to characterise the AQMS measurements in terms of the AWS measurements and not to assess the accuracy of the AWS measurements, which would necessitate a formal intercomparison as opposed to this comparison of convenience.
The sensor uncertainty estimates assume that all appropriate maintenance protocols have been followed. This infers perfectly operated instruments free from uncertainties due to sensor drift and assumes that other mechanical or electrical uncertainties have been captured adequately in the manufacturers’ stated uncertainty.
The temperature calibration uncertainty of the HMP155 (including non-linearity, hysteresis and repeatability) is estimated at 0.27°C. The AQMS calibration procedure has a tolerance of 0.3°C, which includes fluid bath uncertainty estimated at 0.12°C. Hence the expanded uncertainty of laboratory readings from the HMP155 is ΔTsensor = 0.57°C. In lieu of detailed information on AWS calibrations, I treat calibration uncertainty for the AWS as ±0°C, effectively assigning the AWS calibrations as ‘perfect’. For the AWS, I assign ΔTsensor = 0.3°C based solely on the reported accuracy of the Rotronic instrument.
The non-aspirated multiplate radiation shield used in the AQMS introduces biases, especially at low wind speeds (Richardson et al. 1999). These biases are primarily due to internal heating within the shield under low ventilation conditions. Navarro-Serrano et al. (2019) found temperature bias in multi-plate radiation shields of ~±0.5°C, which is comparable with earlier studies (see references within). In this analysis I therefore assign ΔTsystem = 0.5°C for the AQMS.
By contrast, Harrison and Burt (2021) found that temperatures in non-aspirated Stevenson screens typically have errors of ~±0.07°C, but that errors can increase to −0.66 to 0.47°C in low wind flows. Hoover and Yao (2018) found a mean bias (MB) in non-aspirated screens of +0.11°C and differences of up to +2.1°C under high insolation and low wind speed conditions. Nevertheless, as stated earlier, for this analysis I assume that ΔTsystem = 0°C for the AWS.
The combined expanded uncertainty for the measurements is given by the root sum square of the system and sensor uncertainty:
It is important to note that this is a conservative estimate of the combined uncertainty using stated sensor accuracies from the manufacturers and other estimates of potential systematic observations biases from, for example, radiation screening. Almost certainly the actual expanded uncertainty is much higher than stated here as field assessments of sensor response time (Burt and de Podesta 2020) and ventilation of radiation shields and their effects on temperature response (Burt 2022) show significant variance from the manufacturers' stated response times.
The expanded uncertainty of the AQMS humidity calibration using the RHCL-2 is estimated at 1.2% and is incorporated into the AQMS calibration procedure tolerance of 2%. The estimated uncertainty of the HMP155 probe (including non-linearity, hysteresis and repeatability) is reported by the manufacturer as ±(1.0 + 0.01RH%) or ±1.7% when RH ≥ 90%. I hence estimate the sensor uncertainty for AQMS humidity measurements from the HMP155 as 3.7%.
In lieu of detailed information on AWS calibrations, I treat calibration uncertainty for the AWS as 0%, effectively assigning the AWS calibrations as ‘perfect’. For the AWS I assign ΔRHsensor = 1%, based solely on the reported accuracy of the Rotronic instrument. Therefore, the combined sensor uncertainty for this comparison is estimated as ±3.8%.
Biases in humidity measurements due to radiation shield characteristics are less well-studied than for temperature. Harrison and Wood (2012) found that low ventilation in a Stevenson screen can bias humidity measurements by several percent. By contrast, during a field comparison in Algeria, Lacombe et al. (2011) found variations between humidity measuring instruments but no clear influence from different radiation screens. In lieu of clear evidence quantifying screen biases in humidity measurements, I chose to assign no system bias, i.e. ΔRHsystem = 0%. Hence the combined expanded uncertainty for the measurements is given solely by root sum square of the sensor uncertainty:
As with temperature, this is a conservative estimate of the combined uncertainty using stated sensor accuracies from the manufacturers. In field conditions the actual uncertainties are likely to be much higher.
2.5. Analyses
I assess the overall performance of the sensors in each system through time series analysis to discuss seasonal and diurnal variations between the two systems. I assess these variations in the context of the expanded uncertainties of the measurements (assuming the AWS provides the reference or close to the ‘true’ measure) and the confidence intervals of various mean measurements from both systems. This approach assesses uncertainties from sampling bias (i.e. statistical uncertainty or Type A uncertainty) and systematic uncertainty (Type B) (Farrance and Frenkel 2012). The true uncertainty is combination of the two. Type A uncertainty is assessed using statistical means, here the 95% confidence interval of the mean for daily and hourly means. By contrast, Type B uncertainty is assessed using non-statistical approaches as described in Section 2.4. In very large samples, such as the 1-min observations presented here, the confidence interval approaches zero as n → ∞ and the uncertainty is best represented by the Type B component (i.e. the bias).
I test for bias using tests of MB, mean absolute error (MAE), and root-mean-square error (RMSE), defined as follows:
After assessing the overall performance of the sensors, I provide a more detailed analysis of two case studies: 1 day each of extreme high and low temperatures.
Base data are the validated 1-min measurements. For the analysis period, this provides a potential 525,600 observations for comparison. The 1-min data are organised into a paired time series, that is, a valid 1-min observation is included in the analysis only when both AQMS and AWS data are valid for that period. Following the quality procedures outlined previously provides 516,334 paired temperatures and 516,717 paired humidity measurements for comparison. Data are also aggregated into 1-h average time series using strict data availability criteria of 95% or greater, that is at least 57 1-min averages in each hour need to be valid for the 1-h calculation.
The data are also summarised as daily returns, with the daily mean, maximum and minimum 1-min observations compiled into an annual time series. Daily maxima and minima for each calendar day are derived from the 1-min observations, that is the daily maximum (minimum) is assigned to the highest (lowest) 1-min average from each day for each of the AQMS and AWS. This varies from the approach for reporting daily maximum and minimum used by the Bureau of Meteorology where the highest and lowest temperature of the minute (1-Hz sampling) is logged, and the daily maximum and minimum temperature reported as the highest (lowest) of the 1440 1-min extremes during the standard observation day (Trewin 2022). For a valid day to be included in the analysis, I apply strict data availability rules of at least 95% (1368) of all paired 1-min observations valid for 1 day to be added to the daily time series. This delivers 343 valid days for temperature and 354 valid days for humidity. Missing data are mainly due to power interruptions to the AQMS during Black Summer bushfires (7 days) and a communications failure in the AQMS datalogger during 6–10 September.
3.Results
3.1. Temperature
I begin by examining all the paired 1-min temperature data (Table 3). There are 516,334 paired data, that is 98.2% of all 1-min periods during the year, with all months having high data return rates (the lowest, September at 87.6% due to an AQMS datalogger problem). Annual mean temperatures were 17.22 and 17.07°C at the AWS and AQMS respectively. Monthly means had ranges of 10.69–25.18°C at the AWS and 10.48–25.24°C at the AQMS. Assessing the biases of the AQMS with respect to the AWS showed annual MB of −0.14°C and monthly MBs between −0.27°C in August and +0.06°C in January. The MAEs were 0.37°C for the annual mean and 0.37–0.46°C for the monthly means. Annual RMSE was 0.49°C with monthly RMSEs of 0.37–0.57°C.
Period | n | AWS (°C) | AQMS (°C) | r | MB (°C) | MAE (°C) | RMSE (°C) | |
---|---|---|---|---|---|---|---|---|
January | 44,633 | 25.18 | 25.24 | 0.997 | 0.06 | 0.30 | 0.41 | |
February | 40,135 | 22.45 | 22.41 | 0.997 | −0.04 | 0.29 | 0.41 | |
March | 44,638 | 21.11 | 21.00 | 0.997 | −0.11 | 0.27 | 0.37 | |
April | 43,180 | 17.41 | 17.28 | 0.997 | −0.13 | 0.31 | 0.40 | |
May | 44,625 | 13.40 | 13.27 | 0.997 | −0.13 | 0.36 | 0.48 | |
June | 43,145 | 11.01 | 10.79 | 0.995 | −0.22 | 0.41 | 0.50 | |
July | 44,416 | 10.69 | 10.48 | 0.995 | −0.21 | 0.43 | 0.57 | |
August | 44,197 | 11.25 | 10.98 | 0.996 | −0.27 | 0.46 | 0.57 | |
September | 37,856 | 14.51 | 14.32 | 0.996 | −0.19 | 0.40 | 0.53 | |
October | 44,074 | 17.40 | 17.31 | 0.997 | −0.10 | 0.37 | 0.50 | |
November | 43,079 | 20.31 | 20.20 | 0.997 | −0.11 | 0.39 | 0.53 | |
December | 42,356 | 22.10 | 21.90 | 0.996 | −0.19 | 0.40 | 0.55 | |
Annual | 516,334 | 17.22 | 17.07 | 0.998 | −0.14 | 0.37 | 0.49 |
The minimum 1-min observation occurred on 24 August with the AWS reporting −2.8°C (06:42–06:45 hours AEST) and the AQMS reporting −3.1°C (06:44 hours AEST). The variance in this minimum temperature (−0.3°C) is well within the combined sensor uncertainty of ±0.82°C. The maximum temperature recorded was on 31 December 2019, with 43.8°C at the AWS and 43.3°C at the AQMS. Even at this extreme of temperature, and with the differences in station siting, sensor height and radiation shields, the difference between sensors (0.5°C) is well within the combined sensor uncertainty (±0.82°C). This combined with the separation of the stations (300 m), the differences in close-to-site landscapes and the difference in radiation shielding (Stevenson vs non-aspirated beehive) is a significant result.
Next, I consider the daily data (Table 4). Annual mean daily maximum temperatures were 25.62 and 25.29°C at the AWS and AQMS respectively. Monthly means of the daily maximum had range of 18.76–33.17°C at the AWS and 18.16–33.38°C at the AQMS. Assessing the biases of the AQMS with respect to the AWS showed annual MB for daily maximum temperatures of −0.33°C and monthly MBs between −0.51°C in August and +0.21°C in January. The MAEs for daily maximum temperatures were 0.46°C for the annual mean and 0.29–0.64°C for the monthly means. Annual mean daily minimum temperatures were 10.40 and 10.35°C at the AWS and AQMS respectively. Monthly means of the daily minimum ranged within 2.80–19.94°C at the AWS and 2.58–19.58°C at the AQMS. Assessing the biases of the AQMS with respect to the AWS showed annual MB for daily minimum temperatures of −0.05°C and monthly MBs between −0.21°C in July and +0.17°C in November. The MAEs for daily minimum temperatures were 0.24°C for the annual mean and 0.16–0.34°C for the monthly means.
Daily maxima | Daily minima | |||||||||||
---|---|---|---|---|---|---|---|---|---|---|---|---|
Period | n | AWS | AQMS | MB | MAE | r | AWS | AQMS | MB | MAE | r | |
January | 31 | 33.17 | 33.38 | 0.21 | 0.35 | 0.998 | 19.94 | 19.98 | 0.04 | 0.20 | 0.988 | |
February | 26 | 29.88 | 29.66 | −0.22 | 0.37 | 0.995 | 16.48 | 16.51 | 0.03 | 0.16 | 0.995 | |
March | 31 | 27.95 | 27.69 | −0.26 | 0.40 | 0.995 | 15.78 | 15.72 | −0.06 | 0.20 | 0.995 | |
April | 30 | 25.24 | 25.04 | −0.20 | 0.43 | 0.990 | 10.91 | 10.82 | −0.10 | 0.17 | 0.998 | |
May | 31 | 22.05 | 21.66 | −0.38 | 0.43 | 0.990 | 6.21 | 6.15 | −0.06 | 0.25 | 0.996 | |
June | 28 | 18.60 | 18.16 | −0.45 | 0.49 | 0.990 | 4.69 | 4.61 | −0.08 | 0.27 | 0.992 | |
July | 27 | 18.76 | 18.51 | −0.25 | 0.29 | 0.988 | 2.80 | 2.58 | −0.21 | 0.34 | 0.991 | |
August | 29 | 19.61 | 19.10 | −0.51 | 0.53 | 0.995 | 3.74 | 3.55 | −0.19 | 0.34 | 0.996 | |
September | 25 | 23.57 | 23.05 | −0.52 | 0.53 | 0.996 | 6.66 | 6.51 | −0.14 | 0.24 | 0.998 | |
October | 27 | 26.41 | 26.11 | −0.30 | 0.48 | 0.996 | 9.38 | 9.29 | −0.09 | 0.22 | 0.996 | |
November | 29 | 29.96 | 29.46 | −0.50 | 0.56 | 0.997 | 11.85 | 12.01 | 0.17 | 0.27 | 0.993 | |
December | 29 | 31.25 | 30.67 | −0.58 | 0.64 | 0.997 | 14.95 | 15.03 | 0.08 | 0.23 | 0.995 | |
Annual | 343 | 25.62 | 25.29 | −0.33 | 0.46 | 0.998 | 10.40 | 10.35 | −0.05 | 0.24 | 0.999 |
There is some seasonality and diurnal variation in the MBs of the temperature measurements, with the greatest biases in the cooler months (Fig. 2). Although these MBs remain well within the combined sensor uncertainty (±0.87°C), there is likely a physical explanation for them. It is beyond the scope of this study to characterise the sources of the biases precisely; however, they are likely to be due to a combination of the height of the observations, the thermal properties of the radiation shields, and differences in the landscape in the immediate vicinity of the two stations. Of note is the variation in MB between 06:00 and 08:00 hours. This is potentially due to AQMS siting, which may affect the thermal response of the AQMS building and immediately adjacent land at and near sunrise. Although apparent in the data, the effect is still minimal at <0.05°C and does not obviously affect observations at other hours.
Diurnal (left) and seasonal (right) variation in the AQMS mean temperature bias (black) and bias in daily mean minimums (blue) and maximums (red).
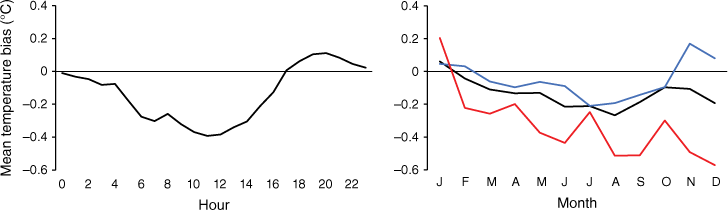
Bias in the daily summary data (daily minimum, maximum and mean temperatures) is shown in Fig. 3. The bias in the AQMS daily mean temperature lies within the expanded sensor uncertainty range of ~±0.87°C for all days. For 5 days there is record bias in daily minimum temperatures outside the expanded sensor uncertainty range whereas 50 days record bias in daily maximum outside that range. Fig. 4 shows the comparison of monthly means of the daily minimum, maximum and mean temperature with respect to the 95% confidence interval of each mean. For all months there is significant overlap in the confidence intervals of each. Fig. 5 summarises the seasonal and diurnal means for the 1-h averaged temperature. For the hourly observations the 95% confidence intervals overlap for all hourly (diurnal) and monthly means.
Bias in AQMS daily mean, maximum and minimum temperatures in comparison with expanded instrument, sensor (calibration + instrument) and system uncertainties.
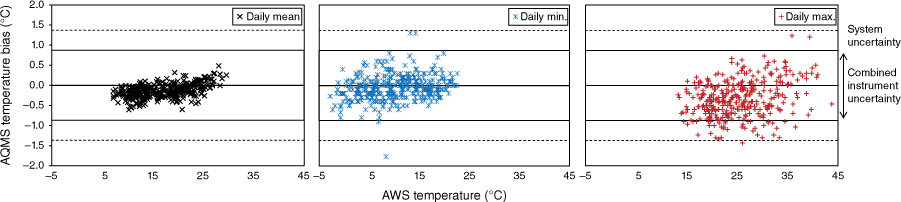
3.2. Relative humidity
There are 516,717 paired 1-min humidity data (Table 5). Annual mean RH was 67.00 and 66.97% at the AWS and AQMS respectively. Monthly means ranged within 55.89–77.48% at the AWS and 57.99–75.71% at the AQMS. Assessing the biases of the AQMS with respect to the AWS showed annual MB of −0.37% and monthly MBs between −2.35% in June and +1.44% in December. The MAEs were 2.71% for the annual mean and 2.17–3.27% for the monthly means. All biases are within the combined uncertainty of the two instruments (±3.8%). Annual RMSE was 3.50% with monthly RMSEs of 2.66–4.43%. Correlation across all data is 0.993.
Period | n | AWS (%) | AQMS (%) | r | MB (%) | MAE (%) | RMSE (%) | |
---|---|---|---|---|---|---|---|---|
January | 44,612 | 71.89 | 72.80 | 0.995 | 0.93 | 2.17 | 2.66 | |
February | 40,117 | 65.84 | 67.18 | 0.996 | 1.28 | 2.27 | 2.83 | |
March | 44,630 | 72.39 | 73.01 | 0.995 | 0.62 | 2.23 | 2.76 | |
April | 43,164 | 76.69 | 75.71 | 0.994 | −0.96 | 2.57 | 3.19 | |
May | 44,621 | 71.99 | 70.15 | 0.992 | −1.84 | 3.07 | 4.02 | |
June | 43,143 | 77.48 | 75.21 | 0.988 | −2.25 | 3.14 | 4.15 | |
July | 44,537 | 68.63 | 66.35 | 0.991 | −2.21 | 3.27 | 4.43 | |
August | 44,622 | 60.89 | 59.72 | 0.993 | −1.17 | 2.79 | 3.79 | |
September | 37,831 | 64.31 | 66.20 | 0.993 | −1.12 | 2.96 | 3.80 | |
October | 44,052 | 61.61 | 61.96 | 0.995 | −0.12 | 2.75 | 3.33 | |
November | 43,048 | 55.89 | 56.95 | 0.995 | 1.02 | 2.74 | 3.31 | |
December | 42,340 | 56.53 | 57.99 | 0.994 | 1.44 | 2.53 | 3.21 | |
Annual | 51,6717 | 67.00 | 66.97 | 0.993 | −0.37 | 2.71 | 3.50 |
Like the temperature measurements, there is a diurnal profile and some seasonality to the biases, with the AQMS reading higher than the AWS in the warmer months and lower in the cooler months (Fig. 6). For mean daily maximum RH (Table 6), the AQMS is consistently lower throughout the year (−2.63%), with monthly MB between −3.70 and −1.41% with largest bias in the cooler months. By contrast, the mean daily minimum RH from the AQMS is biased high at +2.60% annually and 1.67–3.55% monthly. The MBs all remain within the combined sensor uncertainty of ±3.8%. Fig. 7 summarises the seasonal and diurnal means for the 1-h averaged RH. For the hourly observations, the 95% confidence intervals overlap for all hourly (diurnal) and monthly means.
Daily maxima | Daily minima | |||||||||||
---|---|---|---|---|---|---|---|---|---|---|---|---|
Period | n | AWS | AQMS | MB | MAE | r | AWS | AQMS | MB | MAE | r | |
January | 31 | 96.10 | 94.52 | −1.57 | 1.78 | 0.980 | 42.61 | 45.30 | 2.68 | 2.68 | 0.998 | |
February | 27 | 94.44 | 93.03 | −1.41 | 1.57 | 0.987 | 34.89 | 38.44 | 3.55 | 3.55 | 0.998 | |
March | 31 | 95.65 | 94.08 | −1.57 | 1.93 | 0.985 | 42.13 | 45.21 | 3.08 | 3.12 | 0.995 | |
April | 30 | 99.13 | 96.62 | −2.51 | 2.51 | 0.877 | 41.83 | 44.45 | 2.62 | 2.62 | 0.995 | |
May | 31 | 95.84 | 92.78 | −3.06 | 3.06 | 0.991 | 38.13 | 40.52 | 2.39 | 2.92 | 0.990 | |
June | 30 | 98.50 | 95.30 | −3.20 | 3.20 | 0.757 | 47.20 | 48.87 | 1.67 | 2.26 | 0.992 | |
July | 30 | 94.17 | 90.62 | −3.55 | 3.78 | 0.971 | 36.33 | 38.12 | 1.78 | 2.00 | 0.995 | |
August | 31 | 88.45 | 85.11 | −3.35 | 3.41 | 0.993 | 30.10 | 32.05 | 1.96 | 2.18 | 0.995 | |
September | 25 | 97.88 | 94.42 | −3.46 | 3.46 | 0.888 | 30.76 | 32.91 | 2.15 | 2.34 | 0.997 | |
October | 30 | 94.77 | 91.06 | −3.70 | 3.70 | 0.987 | 27.23 | 30.30 | 3.06 | 3.06 | 0.997 | |
November | 29 | 91.72 | 89.21 | −2.51 | 2.56 | 0.989 | 22.41 | 25.71 | 3.29 | 3.29 | 0.997 | |
December | 29 | 88.48 | 86.84 | −1.64 | 2.10 | 0.989 | 25.76 | 28.76 | 3.00 | 3.11 | 0.994 | |
Annual | 354 | 94.57 | 91.94 | −2.63 | 2.76 | 0.982 | 35.11 | 37.71 | 2.60 | 2.76 | 0.995 |
3.3. Event analysis
Next, I examine the temperature and humidity measurements for two events: 1 day of extreme heat (31 December 2019) and 1 day of extreme cold (24 August 2019) to explore the relative performance of the stations on days of temperature extremes. For both analyses, I consider the 1-min temperature and humidity time series alongside 10-m horizontal wind data from the AQMS. I use the AQMS anemometer measurements as its sonic anemometer has a lower wind speed threshold than the cup or vane anemometer of the AWS and thus is better able to monitor winds under low flow conditions.
Extreme heat across Sydney on the 31 December 2019 saw the record for the highest December temperature ever recorded at the Camden AWS (43.8°C). This day also experienced a large diurnal temperature range of 27.2°C (26.8°C as measured by the AQMS). The daily mean temperature was 26.87°C at the AWS and 26.85°C at the AQMS. Daily mean humidity was 43.8% at the AWS and 44.2% at the AQMS. Mean temperature and humidity measurements are well within the combined instrument uncertainties.
Fig. 8 shows the time series for the day. Note that temperature and humidity recorded at both stations follow each other closely. Sunrise was at 06:48 hours AEST and sunset at 19:12 hours AEST. In the early morning, winds were predominantly from the south with speeds of 0.1–0.8 m s–1, typical of cold air drainage along the Nepean River Valley. A cold front arrived at the site at c. 16:39 hours AEST with increased southerly winds of up to 10 m s−1 leading to temperatures dropping more than 10°C between 16:38 and 16:52 hours AEST. Winds remained southerly for the remainder of the day, averaging 3.5 m s−1 until c. 23:15 hours AEST when winds decreased to average ~0.8 m s−1 through to midnight.
The 1-min time series of AQMS and AWS temperature (top), humidity (middle) and their differences and AQMS 10-m horizontal wind (bottom) at Camden on a hot day (31 December 2019). ws, wind speed; wd, wind direction.
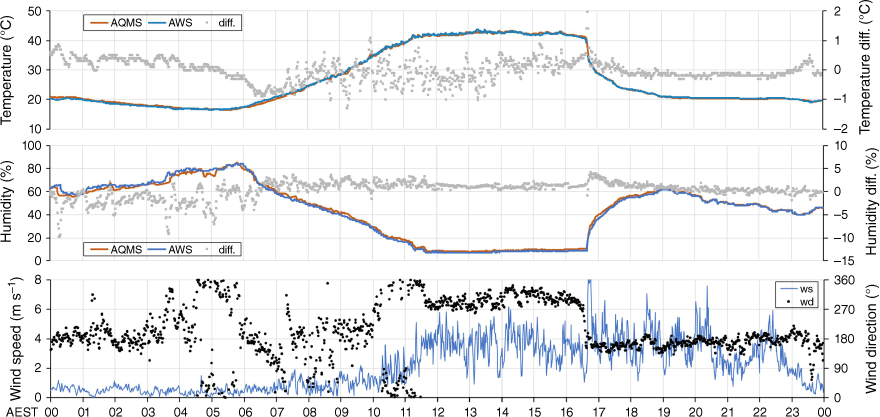
Minimum overnight temperature was 16.5°C (AQMS, 05:28–05:29 hours AEST) and 16.6°C (AWS, 04:55–05:04 hours AEST). After sunrise, temperatures increased throughout the day and reached their maximum of 43.8°C at 13:30 hours AEST as measured by the AWS and 43.33°C at the AQMS at 14:18 and 14:24 hours AEST. At the time of the AWS maximum, temperature at the AQMS was 42.9°C, whereas the AQMS maximum occurred at two different times where temperature at the AWS was 42.9 and 43.0°C. Temperature difference ranged between −1.3°C at 09:14 hours AEST and +2.0°C at 16:42 hours AEST during the passage of the cold front.
There is greater variability in the respective temperature measurements during the day after sunrise through to the passage of the cold front. A component of this variation is likely due to the differences in radiation shielding at the stations; however, the most significant contributor to the variation is probably from temperature differences in small-scale convective turbulence (eddies) exacerbated by the separation of the stations. Overall, however, from sunrise until the arrival of the cold front, the mean temperature difference between the stations was −0.1°C, again well within combined instrument uncertainty. Over the same period, the mean minute-to-minute variation in temperatures at the AWS was 0.04°C (AQMS 0.04°C) and the mean absolute variation was 0.14°C (AQMS 0.11°C), showing that the difference between instruments is comparable to the difference between sequential measurements for an individual instrument.
In contrast to temperature, there was more variability in humidity measurements prior to sunrise compared to post-sunrise and throughout the remainder of the day. Prior to sunrise the mean humidity difference was −1.7%, whereas during the day it was +1.2%. Throughout the entire day, mean humidity difference was +0.4%. The maximum negative variation in humidity between the stations (AQMS – AWS) was −9.7% at 00:17 hours AEST and the greatest positive variation was +4.3% at 16:43 hours during the initial passage of the cold front.
Fig. 9 shows the time series for the 24 August 2019, the day with the lowest observed temperature of the year and a day with a large diurnal temperature range (28°C). Sunrise was at 06:27 hours AEST and sunset at 17:23 hours AEST. Overnight and in the early morning, temperatures dropped steadily from 2.4°C at midnight to the minimum of −2.8°C (as measured by AWS). The minimum temperature of −2.8°C was recorded at the AWS from 06:42 to 06:45 hours AEST, whereas the AQMS minimum was −3.1°C recorded at 06:44 hours AEST. Daily maximum temperature at the AWS was 25.2°C at 14:18 hours AEST, whereas at the AQMS it was 24.9°C at 14:27 hours AEST. Daily mean temperature was 11.1°C at the AWS and 10.94°C at the AQMS. The variation in the daily maximum and minimum of −0.3°C is again well within the combined instrument uncertainties, as too is the variation in the daily mean (−0.16°C).
The 1-min time series of AQMS and AWS temperature (top), humidity (middle) and their differences and AQMS 10-m horizontal wind (bottom) at Camden during a cold night (24 August 2019). ws, wind speed; wd, wind direction.
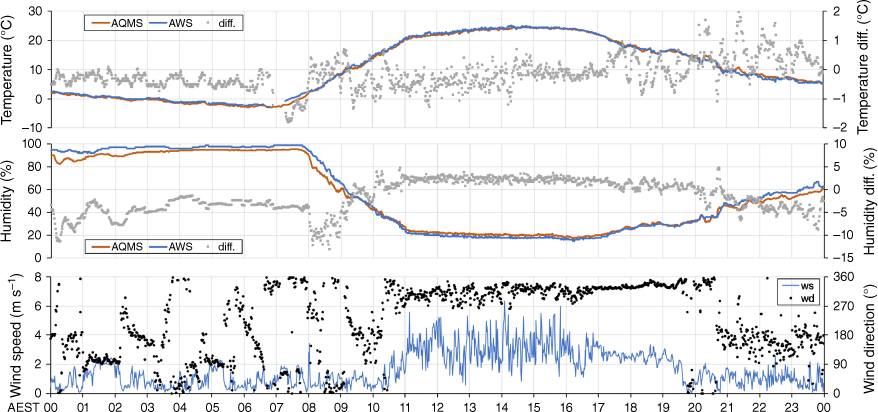
Over this day the temperatures recorded at both stations follow each other closely, whereas there is more variation in the humidity measurements compared with the hot day assessed earlier. Daily mean humidity was 55.7% at the AWS and 54.1% at the AQMS, with daily minimum of 15.0 and 17.5% respectively. Again, these variations are within the combined instrument uncertainties. When considering daily maximum humidity, the AWS recorded 99% and the AQMS 95.5% for a variation of 3.5%, again within the combined instrument uncertainty. Of note is the greater variation in humidity between the two stations overnight, early morning and late evening with many periods of variation between instruments that exceed 5%. This is likely due to the difference in instrument sampling heights between the stations.
4.Discussion
In this paper, I have compared measurements of surface air temperature and RH from the two separate meteorological stations at Camden Airport. The Bureau of Meteorology AWS is a typical airport measurement system with a greater focus on reliability for measurement of synoptic significance under WMO guidance. The NSW Department of Climate Change, Energy, the Environment and Water AQMS is a typical AQMS that has less stringent siting requirements than the AWS. Siting aside, there are several similarities between the stations. Both use thin-film capacitance and platinum resistance sensors for RH and temperature respectively and both systems also have well developed and described data quality management systems with independent oversight. The study is an intercomparison of convenience, relying on in situ measurements from the existing systems, which are separated by ~300 m. Although the two systems possess similar designs, some key elements differ such as siting and instrument performance requirements. Nevertheless, the systems are sufficiently similar and proximate that we can draw some firm conclusions from the analysis.
Firstly, temperature measurements can be considered under most circumstances and for most use cases as equivalent. Both systems use platinum resistance thermometers to measure air temperature, whereas the AWS houses the sensor within a Stevenson screen at 1.5 m and the AQMS houses the sensor in non-aspirated plate radiation shield at 4 m. This difference in siting does not appear to significantly affect comparability of measurements. Annual MB in the temperature measurements is −0.14°C. There are seasonal and diurnal variations in temperature bias with monthly MB varying from −0.27 to +0.06°C and mean hourly bias varying between −0.39 to +0.11°C. The MBs are within the range of the combined instrument uncertainty of ±0.82°C. The 95% confidence interval for the daily mean, minimum and maximum temperatures from the 1-min average data overlap for all months. Similarly, when assessing the hourly observations, the 95% confidence intervals overlap for all months and for all hours in the day, signifying little variance due to seasonal or diurnal patterns. Variations in some aspects of sampling design (e.g. observation heights and radiation shields) appear to have limited effect on the overall quality of the AQMS temperature observations. Users can therefore be confident that temperature measurements from the AWS and the AQMS are equivalent.
Similarly, for most situations the RH measurements are equivalent. Both systems use thin-film capacitance sensors and, although their siting specifics differ (Stevenson screen at 1.5 m and non-aspirated radiation shield at 4 m), the variation between measurements is within the expanded uncertainty range of ±3.8% for most situations. Annual MB in humidity measurements is −0.37%, monthly MB varies from −2.21 to +1.44% and bias in mean hourly measurements varies between −2.64 and +2.66%. Users can also be confident that humidity measurements from the AWS and the AQMS are comparable.
Formal intercomparisons of differing AWS appear limited in peer-reviewed literature. The WMO has conducted a few laboratory intercomparisons of specific instruments focused on Regional Instrument Centres. The most recent of these was in 2018–19, which included HMP155 sensors (World Meteorological Organisation 2021b). Of note is that the acceptance limits of these well-designed, laboratory-controlled intercomparisons are ±0.2°C for temperature and ±3% for RH (Bojkovski et al. 2019), placing the results here from a field comparison of convenience at Camden into further context.
By contrast, comparisons of convenience are more numerous but often focus on comparing AWS with manual observations (Leeper et al. 2015; Acquaotta et al. 2019; Trewin et al. 2020). A comparison in Greenland found that two different AWS on the Greenland Summit separated by ~1 km differed in measurements by ~0.5°C on average, with a seasonal component (Shuman et al. 2001). In another comparison in Antarctica, Genthon et al. (2011) found a bias of greater than 5°C between two AWS separated by 3 km in flat, homogenous terrain occurred 47% of the time and that a bias of greater than 2°C occurred 80% of the time even under well-mixed atmospheric conditions.
5.Conclusion
This is a comparison at one location only but should give confidence to researchers in the robustness of AQMS measurements. Here I not only demonstrate statistical equivalency of temperature and humidity measurements under most situations at this station, but also describe the comprehensive quality control and assurance processes that support the observations here and across the AQMS network.
Urban climatologists and meteorologists can consider using data from the NSW air quality monitoring network in their research with confidence. The AQMS can greatly expand the observations available for research across a range of endeavours, including the study of urban climates, urban heat island effects, temperature extremes and mesoscale processes such as land–sea breeze circulations. Modellers could also benefit from using AQMS data as an independent data set to assist in model validation and verification. The Bureau of Meteorology could benefit from the inclusion of NSW AQMS (and other AQMS) data into data assimilation systems for both routine forecasting and forecasting during high-impact weather events. It is surprising that given the existing effort of the Bureau of Meteorology’s TPAWS program that this has not yet occurred, perhaps highlighting the lack of awareness of AQMS observations.
Further work is required to more explicitly assess the quality of the AQMS observations under a broader range of conditions and across more monitoring locations. Additional analysis using other relatively closely paired stations in NSW is warranted, for example at Richmond, Penrith, Narrabri and Albion Park where stations are separated by less than 3 km (and as little as 300 m at Narrabri). The analysis can also extend to consideration of wind speed, pressure and other relevant variables. However, these will still be comparisons of convenience and cannot replace the robust results that would be delivered by a well-designed, formal field inter-comparison. National meteorological services should consider field intercomparisons of AWS.
Data availability
The 1-min data from the AQMS are available upon request. Hourly AQMS data are available from the NSW air quality data services https://www.airquality.nsw.gov.au/air-quality-data-services. The 1-min data from the AWS are available upon request from the Bureau of Meteorology.
Acknowledgements
I acknowledge the diligent work of all technical and scientific staff in the Air Quality Monitoring and Quality Systems and Reporting teams of the Department of Climate Change, Energy, the Environment and Water for their continued professionalism in providing robust air quality and meteorology data for the community.
References
Acquaotta F, Fratianni S, Aguilar E, Fortin G (2019) Influence of instrumentation on long temperature time series. Climatic Change 156(3), 385-404.
| Crossref | Google Scholar |
Ayers G (2019) A comment on temperature measurement at automatic weather stations in Australia. Journal of Southern Hemisphere Earth Systems Science 69(1), 172-182.
| Crossref | Google Scholar |
Ayers G, Warne J (2020) Response time of temperature measurements at automatic weather stations in Australia. Journal of Southern Hemisphere Earth Systems Science 70(1), 160-165.
| Crossref | Google Scholar |
Båserud L, Lussana C, Nipen TN, Seierstad IA, Oram L, Aspelien T (2020) TITAN automatic spatial quality control of meteorological in situ observations. Advances in Science and Research 17, 153-163.
| Crossref | Google Scholar |
Brandsma T, Van der Meulen J (2008) Thermometer screen intercomparison in De Bilt (the Netherlands)—part II: description and modeling of mean temperature differences and extremes. International Journal of Climatology: A Journal of the Royal Meteorological Society 28(3), 389-400.
| Crossref | Google Scholar |
Bojkovski J, Drnovšek J, Groselj D, Knez J, Begeš G (2019) WMO interlaboratory comparison in Europe with loops linkage and data processing. Journal of Atmospheric and Oceanic Technology 36(2), 257-267.
| Crossref | Google Scholar |
Bureau of Meteorology (2013) Observation specification number 2013.1. Guidelines for the siting and exposure of meteorological instruments and observing facilities. (The Bureau) Available at http://www.bom.gov.au/climate/cdo/about/observation_specification_2013.pdf [Verified 20 March 2024]
Bureau of Meteorology (2023) Climate Data Sites: Site information, Site Summary: CAMDEN AIRPORT AWS. (The Bureau) Available at http://www.bom.gov.au/climate/averages/tables/cw_068192_Info.shtml [Verified 20 March 2024]
Bureau of Meteorology (2024) Climate statistics for Australian locations. Monthly climate statistics: all years of record. Summary statistics: CAMDEN AIRPORT AWS. (The Bureau) Available at http://www.bom.gov.au/climate/averages/tables/cw_068192.shtml [Verified 20 March 2024]
Burt S (2022) Measurements of natural airflow within a Stevenson screen and its influence on air temperature and humidity records. Geoscientific Instrumentation, Methods and Data Systems 11(2), 263-277.
| Crossref | Google Scholar |
Burt S, de Podesta M (2020) Response times of meteorological air temperature sensors. Quarterly Journal of the Royal Meteorological Society 146(731), 2789-2800.
| Crossref | Google Scholar |
de Rosnay P, Browne P, de Boisséson E, Fairbairn D, Hirahara Y, Ochi K, Schepers D, Weston P, Zuo H, Alonso‐Balmaseda M (2022) Coupled data assimilation at ECMWF: current status, challenges and future developments. Quarterly Journal of the Royal Meteorological Society 148(747), 2672-2702.
| Crossref | Google Scholar |
Dutton JA (2002) Opportunities and priorities in a new era for weather and climate services. Bulletin of the American Meteorological Society 83(9), 1303-1311.
| Crossref | Google Scholar |
Farrance I, Frenkel R (2012) Uncertainty of measurement: a review of the rules for calculating uncertainty components through functional relationships. The Clinical biochemist. Reviews 33(2), 49-75.
| Google Scholar | PubMed |
Garcia-Marti I, Overeem A, Noteboom JW, de Vos L, de Haij M, Whan K (2023) From proof-of-concept to proof-of-value: approaching third-party data to operational workflows of national meteorological services. International Journal of Climatology 43(1), 275-292.
| Crossref | Google Scholar |
Genthon C, Six D, Favier V, Lazzara M, Keller L (2011) Atmospheric temperature measurement biases on the Antarctic Plateau. Journal of Atmospheric and Oceanic Technology 28(12), 1598-1605.
| Crossref | Google Scholar |
Hamdi R, Kusaka H, Doan Q-V, Cai P, He H, Luo G, Kuang W, Caluwaerts S, Duchêne F, Van Schaeybroek B (2020) The state-of-the-art of urban climate change modeling and observations. Earth Systems and Environment 4, 631-646.
| Crossref | Google Scholar |
Harrison R, Wood C (2012) Ventilation effects on humidity measurements in thermometer screens. Quarterly Journal of the Royal Meteorological Society 138(665), 1114-1120.
| Crossref | Google Scholar |
Harrison RG, Burt SD (2021) Quantifying uncertainties in climate data: measurement limitations of naturally ventilated thermometer screens. Environmental Research Communications 3(6), 061005.
| Crossref | Google Scholar |
Hirsch AL, Evans JP, Thomas C, Conroy B, Hart MA, Lipson M, Ertler W (2021) Resolving the influence of local flows on urban heat amplification during heatwaves. Environmental Research Letters 16(6), 064066.
| Crossref | Google Scholar |
Hoover J, Yao L (2018) Aspirated and non‐aspirated automatic weather station Stevenson screen intercomparison. International Journal of Climatology 38(6), 2686-2700.
| Crossref | Google Scholar |
Khan HS, Santamouris M, Paolini R, Caccetta P, Kassomenos P (2021) Analyzing the local and climatic conditions affecting the urban overheating magnitude during the Heatwaves (HWs) in a coastal city: a case study of the greater Sydney region. Science of The Total Environment 755, 142515.
| Crossref | Google Scholar |
Koopmans S, van Haren R, Theeuwes N, Ronda R, Uijlenhoet R, Holtslag AA, Steeneveld GJ (2023) The set‐up and evaluation of fine‐scale data assimilation for the urban climate of Amsterdam. Quarterly Journal of the Royal Meteorological Society 149(750), 171-191.
| Crossref | Google Scholar |
Lacombe M, Bousri D, Leroy M, Mezred M (2011) WMO field intercomparison of thermometer screens/shields and humidity measuring instruments, Ghardaia, Algeria, November 2008–October 2009. World Meteorological Organization, Instruments and Observing Methods Report 106, 101.
| Google Scholar |
Lean P, Hólm E, Bonavita M, Bormann N, McNally A, Järvinen H (2021) Continuous data assimilation for global numerical weather prediction. Quarterly Journal of the Royal Meteorological Society 147(734), 273-288.
| Crossref | Google Scholar |
Leeper RD, Rennie J, Palecki MA (2015) Observational perspectives from US Climate Reference Network (USCRN) and Cooperative Observer Program (COOP) network: temperature and precipitation comparison. Journal of Atmospheric and Oceanic Technology 32(4), 703-721.
| Crossref | Google Scholar |
Li M, Shao Q, Dabrowski JJ, Rahman A, Powell A, Henderson B, Hussain Z, Steinle P (2024) Developing a statistical approach of evaluating daily maximum and minimum temperature observations from third-party automatic weather stations in Australia. Quarterly Journal of the Royal Meteorological Society 150(760), 1624-1642.
| Crossref | Google Scholar |
Lucas‐Picher P, Argüeso D, Brisson E, Tramblay Y, Berg P, Lemonsu A, Kotlarski S, Caillaud C (2021) Convection‐permitting modeling with regional climate models: latest developments and next steps. Wiley Interdisciplinary Reviews: Climate Change 12(6), e731.
| Crossref | Google Scholar |
Marsigli C, Ebert E, Ashrit R, Casati B, Chen J, Coelho CA, Dorninger M, Gilleland E, Haiden T, Landman S (2021) Observations for high-impact weather and their use in verification. Natural Hazards and Earth System Sciences 21(4), 1297-1312.
| Crossref | Google Scholar |
Matsuguchi M, Hirota E, Kuroiwa T, Obara S, Ogura T, Sakai Y (2000) Drift phenomenon of capacitive‐type relative humidity sensors in a hot and humid atmosphere. Journal of the Electrochemical Society 147(7), 2796.
| Crossref | Google Scholar |
Mills G (2014) Urban climatology: history, status and prospects. Urban Climate 10, 479-489.
| Crossref | Google Scholar |
Naserikia M, Hart MA, Nazarian N, Bechtel B, Lipson M, Nice KA (2023) Land surface and air temperature dynamics: the role of urban form and seasonality. Science of The Total Environment 905, 167306.
| Crossref | Google Scholar | PubMed |
Navarro-Serrano F, López-Moreno JI, Azorin-Molina C, Buisán S, Domínguez-Castro F, Sanmiguel-Vallelado A, Alonso-González E, Khorchani M (2019) Air temperature measurements using autonomous self-recording dataloggers in mountainous and snow covered areas. Atmospheric Research 224, 168-179.
| Crossref | Google Scholar |
Nipen TN, Seierstad IA, Lussana C, Kristiansen J, Hov Ø (2020) Adopting citizen observations in operational weather prediction. Bulletin of the American Meteorological Society 101(1), E43-E57.
| Crossref | Google Scholar |
Pérez IA, García MÁ, Sánchez ML, Pardo N, Fernández-Duque B (2020) Key points in air pollution meteorology. International Journal of Environmental Research and Public Health 17(22), 8349.
| Crossref | Google Scholar | PubMed |
Rennie S, Cooper S, Steinle P, Dietachmayer G, Krysta M, Franklin C, Bridge C, Marshall M, Xiao Y, Sgarbossa D (2022) ACCESS-C: Australian convective-scale NWP with hourly 4D-Var data assimilation. Weather and Forecasting 37(7), 1287-1303.
| Crossref | Google Scholar |
Richardson SJ, Brock FV, Semmer SR, Jirak C (1999) Minimizing errors associated with multiplate radiation shields. Journal of Atmospheric and Oceanic Technology 16(11), 1862-1872.
| Crossref | Google Scholar |
Santamouris M, Haddad S, Fiorito F, Osmond P, Ding L, Prasad D, Zhai X, Wang R (2017) Urban heat island and overheating characteristics in Sydney, Australia. An analysis of multiyear measurements. Sustainability 9(5), 712.
| Crossref | Google Scholar |
Shuman CA, Steffen K, Box JE, Stearns CR (2001) A Dozen Years of Temperature Observations at the Summit: central Greenland Automatic Weather Stations 1987–99. Journal of Applied Meteorology 40(4), 741-752.
| Crossref | Google Scholar |
Stalker J, Lasley J, Frederick G, McPherson R, Campbell P, Philips B, Pasken B (2013) A nationwide network of networks. Bulletin of the American Meteorological Society 94(10), 1602-1606.
| Crossref | Google Scholar |
Trewin B (2022) A climatology of short-period temperature variations at Australian observation sites. Journal of Southern Hemisphere Earth Systems Science 72(2), 117-125.
| Crossref | Google Scholar |
Trewin B, Braganza K, Fawcett R, Grainger S, Jovanovic B, Jones D, Martin D, Smalley R, Webb V (2020) An updated long-term homogenized daily temperature data set for Australia. Geoscience Data Journal 7(2), 149-169.
| Crossref | Google Scholar |
Ulpiani G, Duhirwe PN, Yun GY, Lipson MJ (2022) Meteorological influence on forecasting urban pollutants: long-term predictability versus extreme events in a spatially heterogeneous urban ecosystem. Science of The Total Environment 814, 152537.
| Crossref | Google Scholar | PubMed |
Ulpiani G, Ranzi G, Santamouris M (2021) Local synergies and antagonisms between meteorological factors and air pollution: a 15-year comprehensive study in the Sydney region. Science of The Total Environment 788, 147783.
| Crossref | Google Scholar | PubMed |
Wieringa J (1980) Representativeness of wind observations at airports. Bulletin of the American Meteorological Society 61(9), 962-971.
| Crossref | Google Scholar |
Zhang C, Fan C, Yao W, Hu X, Mostafavi A (2019) Social media for intelligent public information and warning in disasters: an interdisciplinary review. International Journal of Information Management 49, 190-207.
| Crossref | Google Scholar |