A historical monthly upper-air humidity dataset for Australia
Branislava Jovanovic

A Environmental Prediction Services – Climate, Australian Bureau of Meteorology, GPO Box 1289, Melbourne, Vic. 3001, Australia.
B Environmental Prediction Services – Water, Australian Bureau of Meteorology, Melbourne, Vic., Australia.
C School of Earth, Atmosphere and Environment, Monash University, Melbourne, Vic., Australia.
Journal of Southern Hemisphere Earth Systems Science 73(2) 148-167 https://doi.org/10.1071/ES22022
Submitted: 1 July 2022 Accepted: 1 June 2023 Published: 21 June 2023
© 2023 The Author(s) (or their employer(s)). Published by CSIRO Publishing on behalf of the Bureau of Meteorology. This is an open access article distributed under the Creative Commons Attribution-NonCommercial-NoDerivatives 4.0 International License (CC BY-NC-ND)
Abstract
Monthly humidity (represented as dew point temperature, DWPT) data from 22 land and 5 island Australian upper-air sites were analysed, with trends estimated over the 1965–2017 period at four pressure levels. Humidity data were selected to ensure that data collected under consistent sampling conditions were used (‘modified data’). The quality control process involved examining station metadata and applying an objective statistical test that detected discontinuities in the data series. At each station and pressure level, modified data series were adjusted (homogenised) on a monthly timescale when discontinuities were identified. Analysis of the homogenised (adjusted) modified DWPT data indicates that, over the 1965–2017 period, linear trends are mostly positive and smaller compared to unadjusted modified data. The all-Australian time-series show positive trends at the 850–400-hPa levels. The total increases in DWPT since 1965 at 850-, 700-, 500- and 400-hPa levels are ~0.5, ~1.2, ~1.3 and ~0.8°C respectively. The increase in humidity in the lower and middle troposphere is in accordance with the expectation that, as the troposphere warms, the amount of moisture in it should increase (at a differential rate of ~7% °C–1 at low altitudes globally, following Clausius–Clapeyron scaling) due to increasing surface evaporation and moisture-holding capacity of the air. However, changes in atmospheric dynamics also influence the magnitude and distribution of humidity trends. The homogenised modified Australian radiosonde data for the 850-hPa level show that the amount of moisture at this level increased ~8.8% °C–1 during 1965–2015
Keywords: Australia, dew point temperature, historical monthly dataset, homogenisation, humidity, radiosondes, trends, upper-air.
1.Introduction
Humidity measurements made by radiosondes are used in different operational and research applications, including weather forecasting, initialising and evaluating numerical models, validating remotely sensed water vapour data, parameterising cloud processes, calculating precipitable water and assessing extreme rainfall potential. These records are also used for constructing water vapour climatologies and monitoring their trends (Elliott and Gaffen 1991; Miloshevich et al. 2004) as they are the only high-resolution, multi-decadal in situ observations of tropospheric water vapour content.
Water vapour plays a key role in the global hydrological cycle, as it is linked to the formation of clouds and precipitation (Trenberth et al. 2007; Sherwood et al. 2010). Further, through latent heat exchanges, water vapour is the main method of energy transport throughout the atmosphere, and its phase changes provide one of the largest heat sources and sinks modulating the large-scale circulation patterns of the atmosphere (Hense et al. 1988). Its effect on the radiation balance is particularly important because of the polarity of the water vapour molecule, which results in a strong absorption in the infrared spectrum. Consequently, as a very potent and abundant greenhouse gas (it accounts for ~60% of the natural greenhouse effect for clear skies, Kiehl and Trenberth 1997), it is very important in studies of climate change. This is because much of the projected temperature increase, which results from the enhanced greenhouse effect, comes from the concurrent increase in atmospheric moisture (Held and Soden 2000; Philipona et al. 2005). Water vapour increase is a key feedback that determines climate sensitivity (Dai et al. 2011).
In contrast to the generally uniform global distribution of other greenhouse gases (CO2, CH4 and N2O), the distribution of water vapour in the atmosphere has more regional variability. Owing to the short residence time of water vapour in the atmosphere (~9 days), the typical horizontal (zonal) distances between its sources and sinks remain near 2000 km, which may explain large regional deviations (Hense et al. 1988; Van der Ent and Savenije 2011; Gimeno et al. 2021) and non-uniform distribution of water vapour over the globe. Therefore, understanding its historic variability and evolution is very important for understanding the present and estimating future regional climatic changes.
Some studies estimated trends in humidity from selected stations that were assumed to be mostly homogeneous (e.g. Hense et al. 1988). McCarthy et al. (2009) and Durre et al. (2009) applied methods developed for homogenising temperature data to detect discontinuities and adjust monthly radiosonde humidity data. Both studies assumed the existence of homogeneous reference series, produced from humidity data of neighbouring stations. Dai et al. (2011) developed a different method of homogenising historical records of tropospheric dew point depression (DPD), based on two statistical tests used to detect break points, which were most apparent in histograms, and occurrence frequencies of the daily DPD. Before applying adjustments, Dai et al. (2011) estimated missing DPD values using empirical relationships at each station between the anomalies of air temperature and vapour pressure derived from recent observations when DPD reports were available.
Previous studies have shown that relative humidity (RH) near the surface and in the lower troposphere stayed mostly constant since the 1970s, despite the increase in air temperature, although a downward trend since c. 2000 was reported in surface RH over land (Dai et al. 2011; Byrne and O’Gorman 2018; Masson-Delmotte et al. 2021). By contrast, there was an increase, over both land and ocean, in specific humidity and precipitable water in response to the increased temperatures both at the surface and in the troposphere (Gaffen et al. 1991; Trenberth et al. 2005; Masson-Delmotte et al. 2021), with the temperature–moisture relationship being modified by changes in dynamical processes, for example poleward expansion of the Hadley cell (Nguyen et al. 2015; Tabari 2020). On the regional scale, Gaffen et al. (1991) and Ross and Elliott (1996, 2001) reported an increase in specific humidity over most of North America and a small decrease over Europe; Darand et al. (2019) found a decrease in specific humidity over most of Iran apart from coastal areas; Hense et al. (1988) reported an upward trend of 700–500-hPa precipitable water and RH over the western Pacific; McCarthy et al. (2009) found that homogenised radiosonde data show an increase in specific humidity over northern hemisphere extra-tropics; whereas Xie et al. (2011) and Zhao et al. (2012) found an increase in precipitable water associated with the slight warming in the lower to mid-troposphere over China.
Many researchers investigated changes in trends of different variables that are generally linked to the humidity of the atmosphere over Australia. Lucas (2010) found an upward trend in observed near-surface humidity. Cai et al. (2012) showed that the drying trend in south-eastern Australia since the late 1970s in the austral autumn coincides with a poleward expansion of the subtropical dry zone in the same season; Nguyen et al. (2015) found that the increasing trends of droughts in southern Australia could be explained by expansion of the Hadley cell forced by an increasing surface global warming; whereas Dey et al. (2020), applying a rainfall event-based approach, concluded that the north of Australia has experienced an increase in frequency of long-duration persistent rain events.
The purpose of this study is to develop a homogeneous upper-air humidity dataset, examine the spatial distribution and estimate trends at 850-, 700-, 500- and 400-hPa levels for the period 1965–2017. The upper-air network is identified, and monthly data discussed (Section 2). Following this, an analysis is carried out on the biases in the humidity data (Section 3) and identification of data inhomogeneities using statistical testing (Section 4). The long-term annual and seasonal climatologies and trends based on homogenised modified humidity data series are presented in Section 5 and conclusions are summarised in Section 6.
2.Australian radiosonde network and data
In this work we analyse dew point temperature (DWPT) data for 22 Australian sites located over the mainland and Tasmania, which have long records (40 or more years). A long record enables the detection of any underlying signal (trend), as it becomes distinct from the ‘noise’ introduced by natural climate variability. In addition, data from five island sites are also analysed: the subantarctic Macquarie Island, Lord Howe Island in the Tasman Sea, Norfolk Island in the south-west Pacific Ocean, Willis Island in the Coral Sea and Cocos Island (formally known as Cocos (Keeling) Islands) in the eastern Indian Ocean. The list of stations included in the upper-air network is shown in Table 1 and their spatial distribution in Fig. 1. The distribution of the stations is uneven across Australia, with stations concentrated in the more highly populated areas of the south-east and south-west and sparse coverage across the central and northern parts of the continent. The low density of upper-air stations in northern Australia is partly due to lower spatial and temporal variations in upper-air conditions in equatorial than in high-latitude regions (Bureau of Meteorology 1980).
Station numbers | Station names | Latitude (°S) | Longitude (°E) | Start year of the analysis |
---|---|---|---|---|
3003 | Broome AP | 17.95 | 122.24 | 1965 |
4032 | Port Hedland AP | 20.37 | 118.63 | 1965 |
5007/6011 | Learmonth AP | 22.24 | 114.10 | 1965 |
9021 | Perth AP | 31.93 | 115.98 | 1965 |
9741 | Albany AP | 34.94 | 117.80 | 1966 |
9789 | Esperance | 33.83 | 121.89 | 1969 |
12038 | Kalgoorlie-Boulder AP | 30.78 | 121.45 | 1965 |
13017 | Giles MO | 25.03 | 128.30 | 1965 |
14015 | Darwin AP | 12.42 | 130.89 | 1965 |
15590 | Alice Springs AP | 23.80 | 133.89 | 1965 |
23034 | Adelaide AP | 34.95 | 138.52 | 1965 |
29127 | Mount Isa Aero | 20.68 | 139.49 | 1975 |
32040 | Townsville Aero | 19.25 | 146.77 | 1965 |
39083/39123 | Rockhampton Aero | 23.38 | 150.48 | 1974 |
40842/40223 | Brisbane Aero | 27.39 | 153.13 | 1965 |
44021 | Charleville Aero | 26.41 | 146.26 | 1965 |
48027 | Cobar MO | 31.48 | 145.83 | 1965 |
53115/53048 | Moree Aero | 29.49 | 149.85 | 1965 |
61078 | Williamtown RAAF | 32.79 | 151.84 | 1965 |
72150 | Wagga Wagga AMO | 35.16 | 147.46 | 1966 |
86282/87031 | Melbourne AP | 37.67 | 144.83 | 1965 |
94008 | Hobart AP | 42.83 | 147.50 | 1965 |
200283 | Willis Island | 16.29 | 149.97 | 1965 |
200284 | Cocos Island | 12.19 | 96.83 | 1973 |
200288 | Norfolk Island | 29.04 | 167.94 | 1965 |
200839 | Lord Howe Island | 31.54 | 159.08 | 1965 |
300004 | Macquarie Island | 54.50 | 158.94 | 1965 |
The starting year of the analysis of monthly data is indicated in the last column. Double station numbers indicate that data from two neighbouring sites were merged. AMO, Aeronautical Meteorological Office; AP, Airport; MO, Meteorological Office; RAAF, Royal Australian Air Force.
Locations of 27 stations included in the historical upper-air humidity dataset; also indicated are different Australian states and territories (abbreviations: Vic., Victoria; SA, South Australia; NSW, New South Wales; Qld, Queensland; NT, Northern Territory; WA, Western Australia).
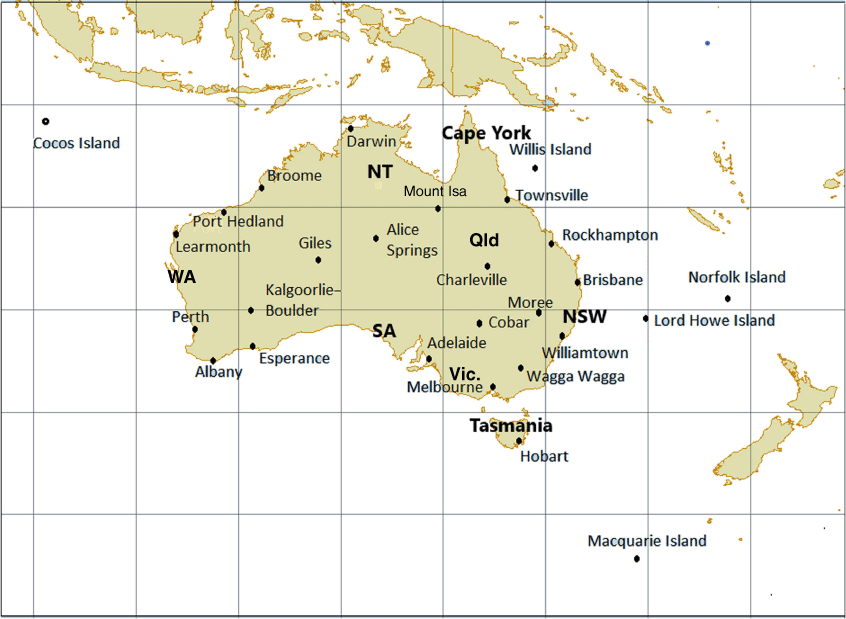
The information on the history of measurements and observation sites is important for a successful detection of inhomogeneities in climate data series as non-climatic influences can be confirmed and attributed only with the use of good-quality metadata. Metadata contain information about how observational datasets were created, the level of quality control, observational times and methods, instrumentation, observation sites and other relevant details. Supplementary Table S1 summarises historic information related to changes in instrumentation and observation practices, taken from the Bureau of Meteorology’s paper history files and, for more recent times, electronic metadata database (Sites DB, established in 1997). Additional information was found in a World Meteorological Organization (WMO) report summarising results of an international survey of historical changes in radiosonde instruments and practices (Gaffen 1993), and Vaisala technical documentation. Supplementary Table S1 summarises the potential causes for inhomogeneities in DWPT data series based on changes in radiosonde or sensor types and algorithms for processing data received from radiosondes. In Australia, these changes occurred approximately at the same time at all stations in the upper-air network. For the study period, the majority of examined data series had two discontinuities, associated with changes in types of radiosondes or humidity sensors. More information about the Australian upper-air network, as well as the collected metadata, can be found in Jovanovic (2014) and Jovanovic et al. (2017).
The measured radiosonde RH data are stored in the Australian Data Archive for Meteorology (ADAM) climate database as DWPT. By definition, DWPT is the temperature to which a parcel of moist air has to be cooled, under constant conditions (i.e. constant pressure and no evaporation), in order for the water vapour to condense. We could have chosen to convert daily DWPT to RH or specific humidity before undertaking further analysis. However, we instead decided to keep DWPT, in order to be consistent with the work of Lucas (2010), who evaluated trends in the near-surface humidity over Australia. In addition, the DWPT has a physical representation that is easy to understand, as well as familiar units of degrees Celsius.
From 1958, upper-air soundings in Australia were performed at 23:00 hours UTC, which corresponded to 09:00 hours Australian Eastern Standard Time (AEST). The second sounding time at 11:00 hours UTC (21:00 hours AEST) was added to the observation program in the late 1990s. Data series for the latter sounding time were not used in this study as they were deemed too short for trend analysis.
The DWPT data for 23:00 hours UTC time were retrieved from the ADAM database for the 850-, 700-, 500- and 400-hPa levels, and monthly data were generated for each station by averaging all available daily values. Prior to 1987–1988, when the Vaisala RS-80 radiosonde was introduced at all sites in the upper-air network, there were no DWPT data at levels above 400 hPa. This is because DWPT was calculated using a mixing ratio calculator (Bureau of Meteorology 1976). As measurements of RH were difficult to make under the cold and dry conditions, the quality of humidity data was generally thought to decrease with decreasing water vapour content, temperature and pressure (Elliott and Gaffen 1991). Hence, humidity measurements were not recorded above the 400-hPa level.
3.Biases in upper-air humidity data
Measuring water vapour in the upper atmosphere is very difficult, as absolute concentrations decrease by an order of magnitude from the surface to the stratosphere (McCarthy et al. 2009). Humidity sensor performance depends on the fast exchange of water molecules with air, and low water vapour mixing ratios combined with the cold temperatures make measurement difficult. This, together with inhomogeneities in the data, has a limiting effect on our ability to detect temporal changes in global atmospheric water vapour (McCarthy et al. 2009).
The apparent drop in DWPTs at the end of the 1980s (shown in Fig. 2 for Hobart) is a result of a sampling bias in the original (raw) humidity data caused by missing cold observations (McCarthy et al. 2009) that occurred during the earlier period. Prior to 1982–1983, it was standard practice in Australia to report humidity measurements as missing if the air temperature was below −40°C. This was because an early type of humidity sensor (the lithium chloride strip) was considered unreliable at and below these temperatures (Elliott and Gaffen 1991). After 1982–1983, when the VIZ carbon humidity element was introduced, humidity was reported as missing if the ambient temperature was below −60°C (Gaffen 1993), whereas after the Vaisala Humicap sensor came into use in 1987–1988, RH was measured when the ambient temperature was down to −90°C (Bureau of Meteorology 1999).
Monthly mean DWPT (original data) for the 700- and 400-hPa levels, recorded at Hobart Airport (monthly means calculated if at least 10 daily measurements per month were available), for the period 1965–2017.
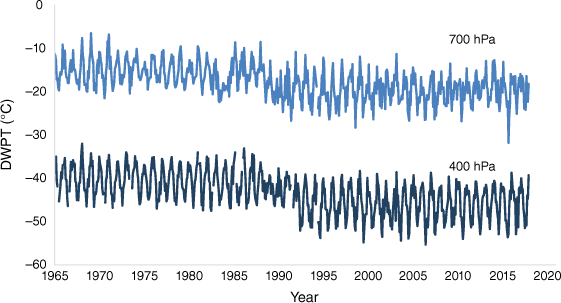
Another sampling problem is related to missing dry observations. Due to low humidity measurements being considered unreliable, if the measured RH was below 20%, in some countries it was either recorded as a RH of 19% or as a DPD of 30°C (McCarthy et al. 2009). Prior to the 1980s, the practice in Australia was to report a constant humidity record (below ~20%) as a DPD of 30°C (Gaffen 1993), as early humidity sensors performed poorly at low humidities. In particular, at very low humidities, the sensors lacked sensitivity to changes in humidity (as the instrument response depends on the number of water molecules present). When the stage of a more or less constant humidity trace was reached, it was assumed that the sensor had gone beyond its measurement capability, and the air was considered dry (Bureau of Meteorology 1986); this was reported as a DPD of 30°C, and in the conversion and storage within the Bureau’s climate database it was recorded as missing DWPT.
These biases are known by the common name of ‘sampling biases’. As radiosonde humidity sensors have been improved over time to have shorter response times and smaller errors under cold conditions, there has been increased sampling and reporting of cold and dry conditions at a given pressure level (Dai et al. 2011). However, both mentioned sampling practices resulted in a bias in monthly means during the period 1965–1988 and in spurious trends during 1965–2017 because of the termination of these practices in the late 1980s (McCarthy et al. 2009; Sherwood et al. 2010).
Apart from sampling biases, which have caused discontinuities at the times of changes in observational practices, the introduction of new types of humidity sensors with different sensitivities and response times, as well as changes in the humidity sensor housing, could also have caused discontinuities in monthly mean data series (known as ‘measurement biases’, McCarthy et al. 2009). In Australia, the lithium chloride strip sensor was replaced in 1982–1983 with the VIZ carbon element. A further improved humidity sensor (Humicap) was introduced in 1987–1988 when the Vaisala RS80 radiosonde came into use. Also, from 1983 humidity sensors were mounted in a new ‘hygristor duct’, which provided effective radiation shielding for the humidity sensor. Other potential reasons for inhomogeneities in DWPT data series can be related to changes in the data processing (i.e. improved humidity measurement algorithm and revised solar radiation correction table c. 2010), or in the algorithms used for converting RH to DWPT as in the case of the introduction of modern, automated systems (i.e. PC-Cora in 1990–1991) (Dai et al. 2011). Obviously, simply using only the existing (raw) data would introduce a bias that would influence estimated trends (Fig. 2).
To estimate trends in DWPT over the longest possible period, it is necessary to use only data collected under the same sampling conditions. This is enabled by modifying DWPT time series by removing the daily DWPT data (from the later period of record) if the ambient temperature is below −40°C and the RH below 20%. The algorithm for converting RH to DWPT follows:
where t is the measured ambient temperature, and:
where a and b are empirical constants (a = 17.502 and b = 240.97). This equation was proposed by the International Organising Committee for the WMO Hygrometer Intercomparison (Gaffen 1993) and is used here as, from the available historic information, it was not possible to determine the algorithm used in Australia for calculating DWPT based on the measured RH and ambient temperature.
The number of removed daily data varied depending on the station location and, in particular, on the level, with the number of removed data increasing from lower to the higher levels (2–12% at 850 hPa, and up to 25% at 400 hPa).
Gaffen et al. (1991) concluded that at least three observations per month were needed to estimate a monthly mean that falls within the 0.10 confidence bands. We decided to produce monthly means (based on modified DWPT data) if at least five observations in a month were available. However, most months, especially at the 850- and 700-hPa levels, had more observations, and estimates of monthly means had generally much higher confidence levels. Note that the modified data series for 1965–2017 did not include daily DWPT data if the ambient temperature was below −40°C and RH below 20%, which resulted in monthly means being biased high.
If less than five observations in a month were available, the monthly mean was classified as missing. Missing monthly means were generally located at the 500- and 400-hPa levels and were replaced with averages for respective months (from the period between break points); this was justified by generally lower interannual variability in DWPT at these levels, which correspond to ~5.5 and ~7.0 km above the mean sea level respectively, as well as by high consistency between the neighbouring sites.
A comparison between the original (raw) and modified DWPT monthly data series for the 850-hPa level (Charleville Aero, 44021) is given in Fig. 3. After removing part of the daily data (as described above), the resultant modified data series is more uniform (Fig. 3a), as it contains only data measured under the same conditions. This has an obvious consequence on the analysed trends (Fig. 3b): original (raw) data show a slightly positive trend (0.04°C decade–1, monthly values outside the three standard deviations were deleted), whereas modified data show a much stronger positive trend of 0.53°C decade–1. Note that we will further examine and discuss results based on the modified DWPT monthly data, as the original (raw) data contain different biases and were not suitable for the further analysis.
Monthly time series (850 hPa): (a) original dew point temperature (DWPT) data (red line) and modified data (blue line); note the increase in magnitude of lower monthly DWPTs in the most recent part of the original (raw) data record; (b) the same as above but for the shorter 2005–2017 period; and (c) corresponding annual DWPT time series for both original (raw) and modified data with fitted least squares linear regression. Data are for Charleville Aero station (44021).
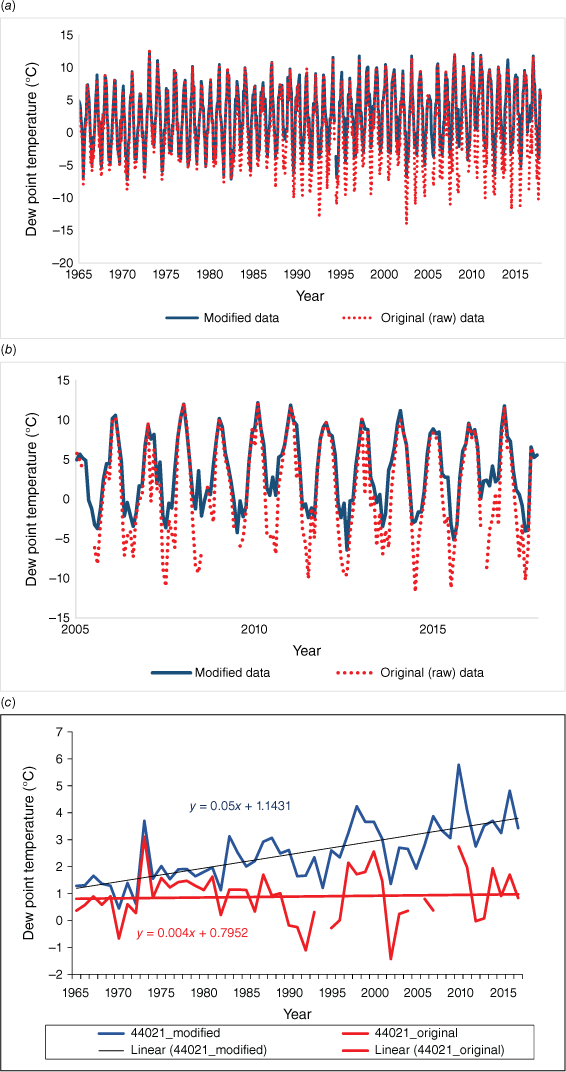
4.Determination of inhomogeneities in data series
In general, detection of break points in upper-air data is more complicated than for surface data because of the sparsity of the station network, simultaneous changes in radiosonde or sensor types, or operational procedures across the network, and the absence of reliable reference series.
To objectively determine discontinuities (break points), modified monthly data series were tested using RHTests software (ver. 4, see https://github.com/ECCC-CDAS/RHtests; Wang and Feng 2013), both without and with reference series (at both 99 and 95% confidence levels). The statistical test used in the software for the case without a reference series is the penalised maximal F test. This allows the time series to have a linear trend throughout the whole period of record but without a shift in the trend itself. When a reference series is available, the t statistic is used to identify unknown break points by locating the point in the difference data series where there is a significant shift in the mean (Wang et al. 2007; Wang 2008a, 2008b); in other words, a modified DWPT data series is compared to a reference series that is homogeneous (free of discontinuities), and any disagreement between the two series is likely to be due to a discontinuity in the modified DWPT series.
Using data from neighbouring sites as reference series is not appropriate for upper-air DWPT due to the simultaneous changes of radiosondes, sensors or operational procedures. There are also no reliable independent reference series (for example, long homogeneous satellite humidity records). However, due to the link between water vapour in the atmosphere and the formation of clouds and precipitation, we decided to use as reference series monthly (log-transformed) precipitation data from the candidate site or from neighbouring sites included in the Australian climate change site network (see http://www.bom.gov.au/climate/change/#tabs=Tracker&tracker=timeseries). These were previously tested and considered to be homogeneous. The use of precipitation data from neighbouring sites is justified as Jones and Weymouth (1997) examined the spatial correlations of monthly rainfall observations and found significant correlations across large distances (correlation greater than 0.5 over distances of 300 km or more), thus confirming considerable spatial homogeneity in monthly variance structures. Initial testing for selected candidate sites gave promising results, as identified break points were generally supported by the metadata. Note that the choice of rainfall as reference series does not have any impact on resulting climatology and trends.
Break points found in the majority of modified data series, obtained by applying the statistical test, are predominantly related to the introduction of new types of radiosondes or humidity sensors in 1982–1983, 1987–1988 and 2006. These results are consistent with the results obtained by analysing radiosonde temperature data, where major break points were found mostly in the same years (Jovanovic et al. 2017). This is because, in general, the humidity sensor transmits a signal that is proportional to the RH, but which is also affected by the temperature of the hygristor. The radiosonde temperature is used both to correct the reading of the hygristor, and to convert the RH to DWPT (Elliott and Gaffen 1991), thus resulting in temperature and DWPT time series generally exhibiting discontinuities at the same time.
The most frequent break point at the 850- and 700-hPa levels was in 1982–1983, when in the MARK radiosonde, the lithium chloride strip sensor was replaced with the VIZ carbon element located in the hygristor duct, which provided effective radiation shielding. At the 500- and 400-hPa levels the most frequent break point was in 1987–1988, when the MARK radiosonde was replaced by the Vaisala RS80-15 radiosonde, which had a thin film capacitor humidity sensor (Humicap); in addition, solar radiation corrections were also applied for the first time. The third most frequent break point was in 2006–2007 and was related to the change from the Vaisala RS80 to Vaisala RS92 which had a heated twin-Humicap. Note that for five sites with merged data (where data series from two neighbouring sites with shorter records were combined to get one longer data series), statistical testing did not indicate break points at the time of the data merge, except for the Learmonth AP where a pronounced discontinuity was found in 1988 at all four levels. This could be a result of merging dew point data series from two sites ~300 km apart. However, a new type of radiosonde was introduced at approximately the same time, and it cannot be confidently concluded which of the two was the primary source of the discontinuity.
Modified DWPT series were homogenised (by adjusting data in identified break points) if the same break points were determined using at least two different rainfall data series as reference series (the exception were Giles and remote island sites where only one rainfall series was used, as there were no nearby sites and hence no additional available rainfall data series). Additionally, identified break points also had to be supported by the metadata. For a given level, adjustments for each month were calculated using a change in mean, calculated for each month from the discontinuity-free period of modified DWPT data on either side of the change point; usually 6–8-year-long segments were used in order to minimise the influence of low-frequency variability (e.g. radiative forcing or anthropogenic causes, Lanzante et al. 2003). Adjustments were applied by a uniform shift to bring each segment of the time series into agreement with the most recent homogeneous part of the series, assuming that sensors have generally improved in more recent times.
Most data series needed two adjustments. The size of most adjustments was 0.5–1.5°C (annual value). The adjustments were positive, indicating that changes of radiosonde or sensor types in 1982–1983, 1987–1988 (also including application of solar radiation corrections) and 2006–2007 lead to an increase in measured DWPT. This is likely the result of reductions in a dry bias caused by solar heating of the sensors (Gaffen et al. 1991; Moradi et al. 2013).
The number of validated break points, magnitude of adjustments and estimated linear DWPT trends (based on annual means in homogenised modified data series) are given in Supplementary Table S2. At all four levels, trends calculated after adjustments all show lower magnitudes than before adjustments were made, because increases in measured DWPT caused by introduction of new radiosonde or sensor types were eliminated by homogenisation. It is likely, however, that natural variability was partly removed as well, because 1982–1983, 1987–1988 and 2006–2007 were years of ENSO events. Despite this, the trends estimated from homogenised modified data series for the period 1965–2017 are considered to be closer to the real trends than those estimated from the modified DWPT data series prior to homogenisation. An example of a modified monthly DWPT series, and the unhomogenised and homogenised modified annual DWPT data series for one station (Charleville Aero) for the 700-hPa level is shown in Fig. 4. A comparison between the all-Australia DWPT time series based on the unhomogenised and homogenised modified data for the 700-hPa level is shown in Fig. 5a, whereas strong correlations that exist between unhomogenised and homogenised modified data for four periods that correspond to major changes in equipment are shown on Fig. 5b.
(a) Monthly dewpoint temperature (DWPT) anomalies (with the mean annual cycle subtracted) for the 700-hPa level at Charleville Aero (break points in 1983 and 2006 are indicated by arrows), and (b) unhomogenised and homogenised modified annual DWPT series for the same level.
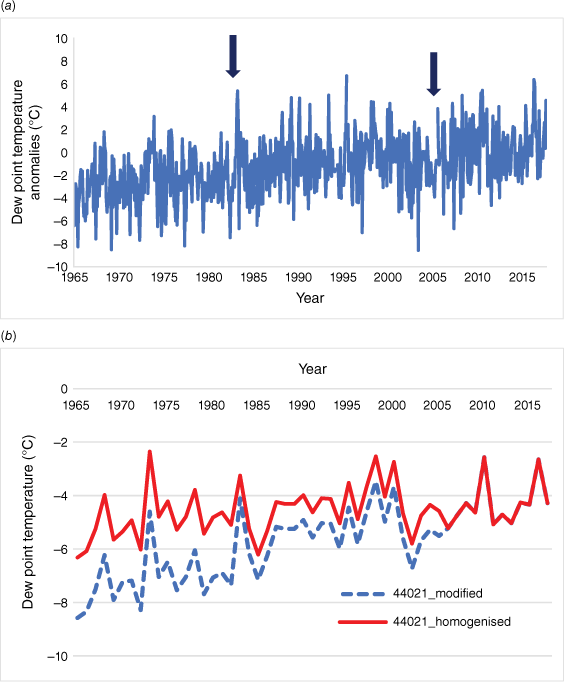
(a) The all-Australia dew point temperature time series based on unhomogenised modified data (‘Modified’) and homogenised modified data (‘Homogenised’) for the 700-hPa level and (b) the corresponding scatter diagram showing relationships between the data for four indicated periods, selected mostly in accordance with the major changes in equipment.
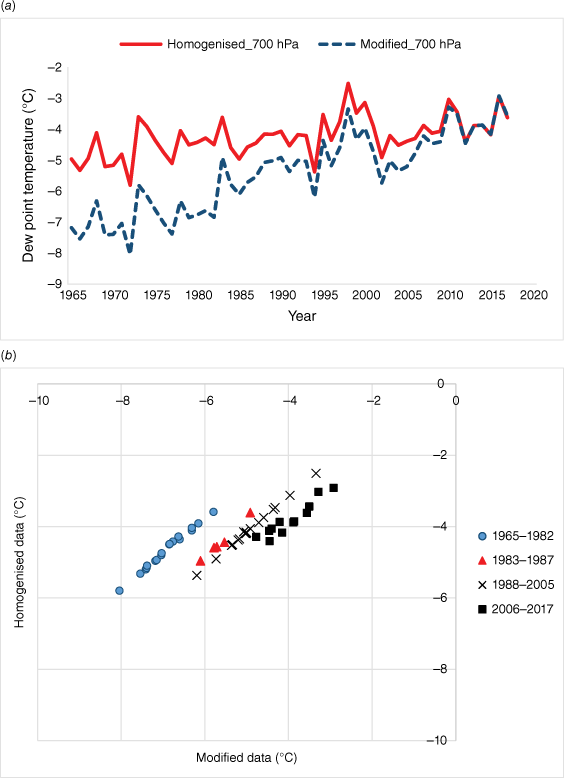
5.Results
The upper-air DWPT dataset (based on homogenised modified data) was analysed in order to determine the spatial distribution as well as the interannual variability and trends in DWPT at the 850–400-hPa levels on annual and seasonal time scales.
For gridding data, we used the objective analysis method of Barnes (1964) to produce annual and seasonal climatological and trend maps for Australia. Using this method, the analysis is derived in which the grid point values are based on data at the closest observation points. Before applying the Barnes analysis, the analysis parameters were optimised using a minimum root-mean-square error estimate (Seaman 1989).
The long effective length scale determined from the optimised process allow for coverage across Australia.
5.1. Climatology
For producing upper-air DWPT climatology maps shown in Fig. 6 and 7, we used the 1971–2000 period as the base climatology. Note that the colour bar on the right-hand side of each map changes with each level.
Maps of 1971–2000 mean 850-hPa DWPT for (a) annual, (b) autumn (MAM), (c) winter (JJA), (d) spring (SON) and (e) summer (DJF).
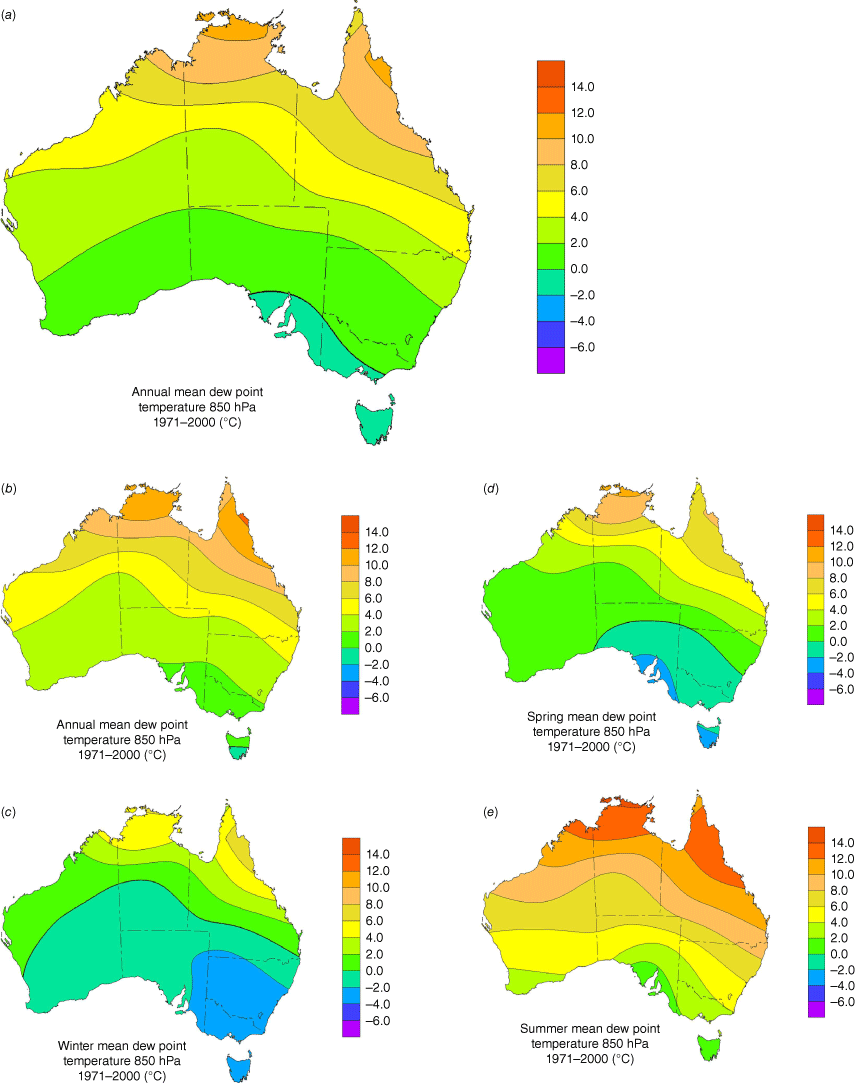
The 850-hPa climatology map (Fig. 6a) shows that the annual mean DWPTs decrease from the northern parts of Australia towards the south, indicating higher humidity values over the tropical latitudes. This is the result of the high evaporation in the low latitudes and the prevalence of warm air masses, which can contain large amounts of water vapour (Peixoto and Oort 1992). Maximum 850-hPa DWPT values (10–12°C) are found over the far north of the Australian continent and eastern part of the Cape York peninsula, and somewhat lower DWPTs (6–10°C) over most of the north and north-east of the country. Over the south-eastern parts of Australia, annual mean DWPTs have lower values, reaching minima (between 0 and −2°C) over south-eastern South Australia, parts of western and southern Victoria, and Tasmania. There is a broadly zonal gradient in humidity, with some departure from the zonal pattern, for example along the east coast (where higher DWPTs extend down the coast, likely a result of the predominant moist south-easterly trade wind flow, as well as the impact of topographic characteristics, i.e. the Great Dividing Range). The distribution of DWPT at the 850-hPa level is in contrast with distribution of DWPT at the surface (not shown here), where minimum values are reached over the arid centre of the continent (Lucas 2010).
Seasonal maps for the 850-hPa level indicate considerable intraannual variability. The austral summer map shows high values of DWPTs over most of Australia, with highest values above the far north of the continent (due to advection of moisture by monsoonal flows). By contrast, the austral winter map shows low (negative) DWPTs approximately over the southern two-thirds of the country, with driest air extending throughout the south-eastern parts, as a result of the low moisture-holding capacity of cold air. In spring and autumn, the distribution of DWPTs mostly follows the annual pattern. However, note that at this level, seasonal maps are influenced by the diurnal cycle and heating and mixing of air in the convective boundary layer; these are particularly strong in the austral summer.
At the 700-, 500- and 400-hPa levels (Fig. 7), there is an overall decrease in specific humidity reflected in the progressively lower DWPT at each level. The decrease in annual mean DWPT from the northern parts of Australia towards the south is more zonal at these levels compared to the 850-hPa level, and gradients are stronger over the southern half of the country.
Similar to the 850-hPa level, seasonal maps for the 700–400-hPa levels (not shown here) indicate considerable intraannual variability. The austral summer maps show relatively high DWPT values over the northern third of Australia, whereas during the austral winter, which coincides with the dry season in the region, DWPT gradients are weak. The lowest DWPTs and strongest gradients are over south-eastern Australia during the austral winter.
This zonal distribution of humidity over Australia is in agreement with the distribution of upper-air temperature (Jovanovic et al. 2017). This was expected because the temperature determines the upper bound of the water vapour content in the air (Ross and Elliott 1996). It is also in agreement with the description given by Peixoto and Oort (1992). However, because the observations made under the cold and dry conditions were not included in the homogenised modified DWPT data series, climatology maps show that DWPTs are biased high (Supplementary Fig. S1 and S2).
5.2. DWPT trends
For estimating trends in DWPT we used a least squares linear regression method for 1965–2017. The all-Australia annual mean DWPTs at the 850-, 700-, 500- and 400-hPa levels show high interannual variability and positive trends with magnitudes of 0.09 ± 0.12, 0.23 ± 0.11, 0.25 ± 0.11 and 0.15 ± 0.12°C decade–1 respectively (Fig. 8). Trends at the 700-, 500- and 400-hPa levels are significant. These are in accordance with trend magnitudes within ±0.4°C decade–1 found by Dai et al. (2011), although for the shorter 1973–2008 period. The total increases in DWPT since 1965 at 850-, 700-, 500- and 400-hPa levels are 0.50, 1.23, 1.30 and 0.82°C respectively; when expressed as specific humidity these increases respectively correspond to ~0.1, 0.3, 0.1 and 0.04 g kg–1. For reference against these changes, national mean specific humidity for the 850-, 700-, 500- and 400-hPa levels are 5.8, 3.9, 1.5 and 0.74 g kg–1 respectively.
1965–2017 annual mean DWPTs for Australia at the 850-, 700-, 500- and 400-hPa levels show positive trends with magnitudes of 0.09 ± 0.12, 0.23 ± 0.11, 0.25 ± 0.11 and 0.15 ± 0.12°C decade–1 respectively. Also shown is total annual rainfall.
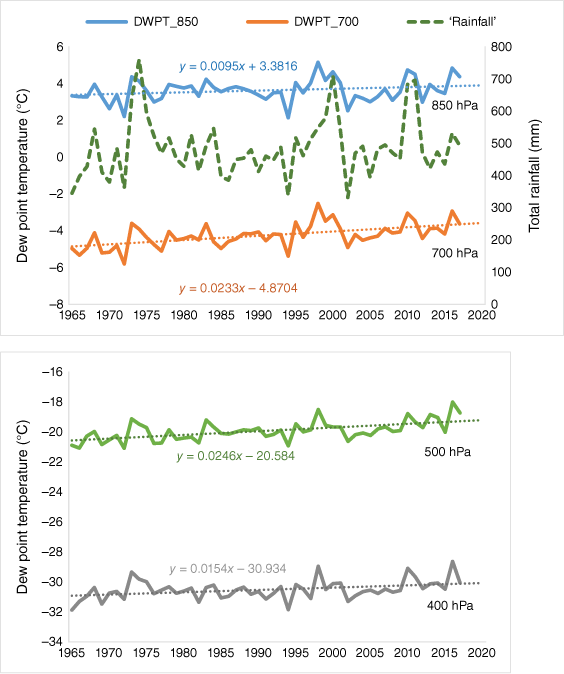
Expressed as an increase in the annual mean DWPT, all-Australian time series indicate an increase of moisture content at the 850–400-hPa levels. Physical principles suggest that, as the troposphere warms, the amount of moisture in it should increase ~7% °C–1 at lower altitudes (Stocker et al. 2013; Allan et al. 2020). The homogenised modified Australian radiosonde data for the 850-hPa level show that the amount of moisture at this level increased ~8.8% °C–1 during 1965–2015. This value was obtained by dividing a change in normalised specific humidity with a change in temperature at the 850-hPa level; as the homogenised radiosonde temperature data are available only to 2015, the increase of the amount of moisture at this level was calculated for 1965–2015. The general increase in DWPT at the 850-hPa level over Australia is in agreement with an upward trend in observed near-surface humidity (for 1957–2003) previously found by Lucas (2010), with the magnitude of the nationally averaged trend in surface dew point at just over 0.1°C decade–1.
We did not compare our results with the output from the reanalysis because previous work (Dai et al. 2011) showed that water vapour trends in atmospheric reanalysis were significantly affected by inhomogeneities in radiosonde humidity data, and thus cannot be trusted.
The increasing moisture content in the Australian region coincides with the general increase in the annual sea surface temperatures (SSTs) (Richardson and Pattiaratchi 2020; Masson-Delmotte et al. 2021; Cheng et al. 2022) around Australia over the same period of time, consistent with the strong relationships between atmospheric humidity and SSTs on global scales (Trenberth et al. 2005). The warming SSTs are associated with positive trends in evaporation in the oceans surrounding Australia (Holgate et al. 2020a). Ocean evaporation is the primary source of atmospheric moisture for rainfall across Australia (Holgate et al. 2020a) and plays an important role in drought development and recovery (Holgate et al. 2020b). Increasing SSTs also lead to increasing occurrence of heavy rain events (Trenberth 2012) from atmospheric systems that advect moisture particularly from warm waters around northern Australia.
Fig. 8 shows the relationship between the all-Australian DWPT time series at the 850–400-hPa pressure levels compared to the all-Australian rainfall totals. The interannual variability in all time series is similar, with periods of high rainfall corresponding to periods of higher DWPT values at all levels. Similarly, periods of lower rainfall agree well with periods of lower DWPTs. Correlation coefficients (R) between rainfall series and DWPT series at the 850-, 700-, 500- and 400-hPa levels are 0.71, 0.68, 0.57 and 0.72 respectively. Although R for all four levels are significant (P < 0.001), only R at the 500-hPa level is of much lower magnitude at the other levels. At the 850- and 400-hPa levels, the variance in DWPT is still explained by only 50% of the variance in rainfall.
The interannual variability in the Australian annual mean DWPT time series results from a strong periodic influence by climate drivers such as El Niño–Southern Oscillation (ENSO), the Indian Ocean Dipole (IOD) and the Southern Annular Mode (Risbey et al. 2009; King et al. 2014). During La Niña (El Niño) phase of ENSO, the atmospheric circulation enhances (reduces) humidity over eastern Australia. Similarly, when IOD is positive (negative), Indian Ocean waters to the north-west of the continent are cooler (warmer) than average and there is less (more) moisture in the air.
The influences of both ENSO and IOD are shown in a change in the amount of total rainfall. During the warm ENSO phase (El Niño), total rainfall measured across Australia is generally lower than normal. By contrast, during the cold ENSO phase (La Niña), conditions are generally wetter in Australia. For example, La Niña periods 1973–1975, 1998–2001 and 2010–2011 are characterised by high DWPT values at all levels and the high total rainfall amounts (Fig. 8). The opposite is seen for the 1993–1994 and 2002–2003 periods, which correspond to El Niño events (i.e. very low DWPTs and total annual rainfall). Similarly, when the IOD is positive (i.e. in 1972, 1994, 2012 and 2015), parts of Australia experience drier conditions, leading to lower than normal total rainfall for Australia, whereas a negative IOD results in above average rainfall (i.e. in 1974, 1998, 2010 and 2016).
Correlations between the annual DWPT and the Southern Oscillation Index (SOI) were calculated for each site over a 50-year period (1965–2015). The strongest correlations at the 850-hPa level (Fig. 9a) were for the south-east of the continent and for Willis and Norfolk Islands (not shown on the map), whereas the weakest correlations were over Australia’s south-west. Correlations between the annual DWPT and Dipole Mode Index (DMI1) for the 850-hPa level (Fig. 9b)were strongest for the north-west and south-east of the continent, and for Cocos Island. The weak negative or positive correlations were found for Australia’s south-west. Despite a spatial pattern of correlation between DWPT and SOI, and DWPT and DMI, that broadly resembles the pattern of correlation between rainfall and SOI and DMI, R values were not significant at any site with the exception of Melbourne AP (between DWPT and SOI).
Maps of 1965–2015 correlations between 850-hPa level annual DWPT and (a) SOI and (b) DMI for each site in the upper-air network; open circles indicate radiosonde sites. Low magnitude correlation contours close to zero are spread across −0.1 and 0.1. Correlation coefficients are not statistically significant.
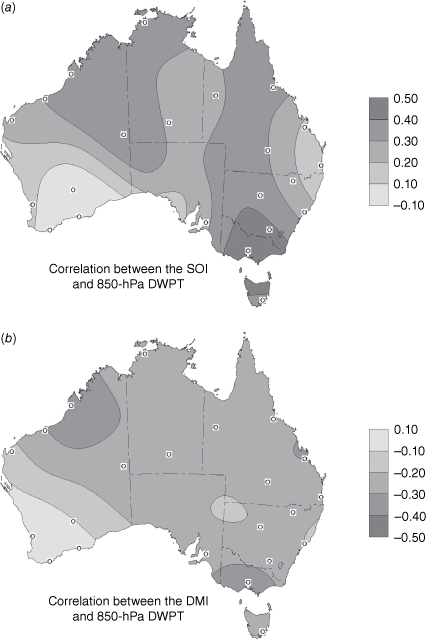
These patterns of correlation between the annual homogenised modified DWPT data and the SOI and DMI are broadly consistent with patterns of correlation between these indices and rainfall. Risbey et al. (2009) showed that ENSO influences rainfall in eastern and northern Australia, whereas the IOD influences rainfall in the south and west of the continent.
Fig. 10 shows maps of 1965–2017 trends in annual mean DWPT (based on the homogenised modified data) at the 850–400-hPa levels. There is a general increase in humidity at all levels, with strongest increases during the summer (not shown). Trends for each site and information about their statistical significance are shown in Supplementary Table S2.
Maps of 1965–2017 trends in annual mean DWPT at (a) 850-, (b) 700-, (c) 500- and (d) 400-hPa levels. Units are degrees Celcius per decade. Maps are based on the homogenised modified data.
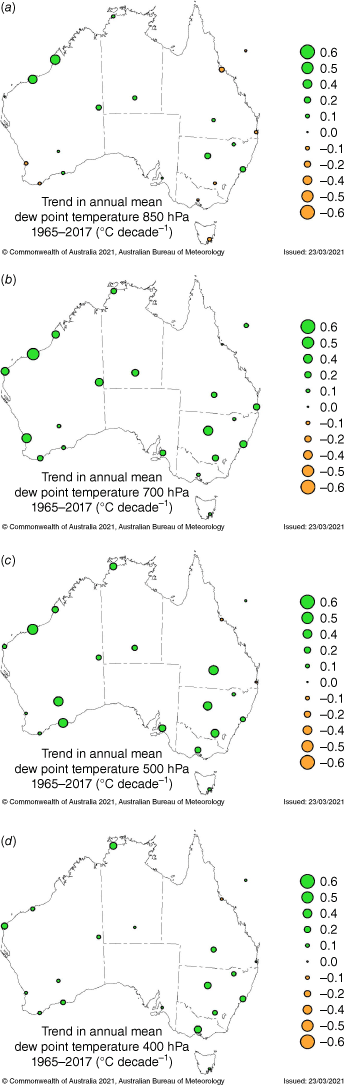
Fig. 11 shows annual and seasonal contour maps of 1965–2017 trends in mean DWPT at the 850-hPa level based on the homogenised modified data. For comparison, a map of annual trends for the same period of time based on the original (raw) data is shown in Fig. 12. Naturally, using original (raw) data on its own would produce erroneous results due to the biases they contain.
Maps of 1965–2017 trends in mean dew point temperature (DWPT) at the 850-hPa level: (a) annual, (b) autumn (MAM), (c) winter (JJA), (d) spring (SON) and (e) summer (DJF). Maps are based on the homogenised modified data. Units are degrees Celcius per decade.
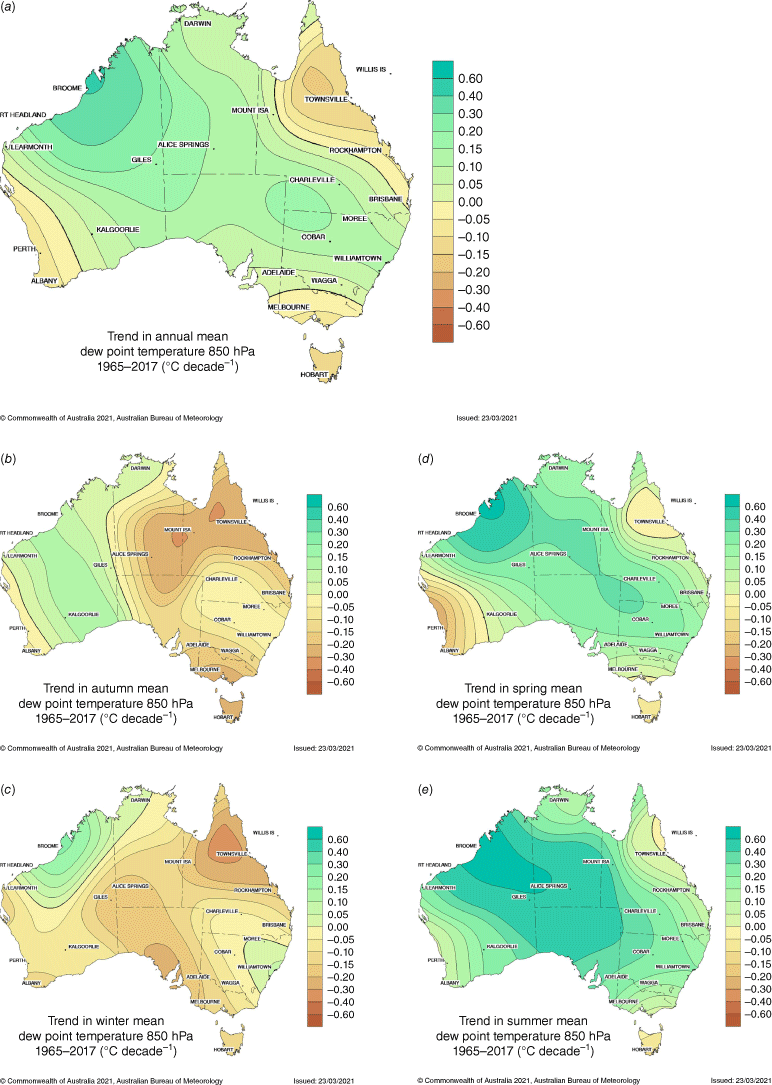
Annual map of 1965–2017 trends in mean dew point temperature (DWPT) at the 850-hPa level based on the original (raw) data. Units are degrees Celcius per decade.
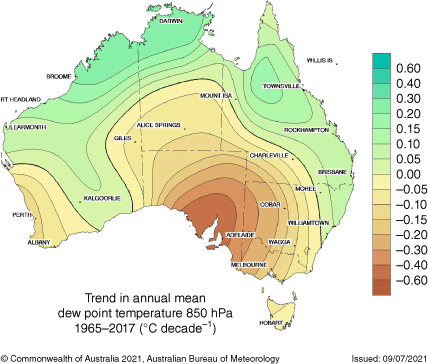
The 850-hPa level annual DWPT trend map for the period 1965–2017 based on homogenised modified data (Fig. 11a) shows increasing trends above most of Australia. The strongest increase in DWPT is in the north-west of Western Australia, where it is generally 0.3–0.4°C decade–1. Most of the Northern Territory, South Australia, New South Wales and south-western Queensland show an increase in DWPT of 0.1–0.2°C decade–1. Trends are negative in south-west Western Australia, Tasmania, most of Victoria, and north-east and far east Queensland, with the strongest decreases over Tasmania and north-east Queensland (0.1–0.2°C decade–1).
We also examined seasonal long-term trends (Fig. 11b–e). Autumn and winter show decreasing trends in DWPT over much of Australia. In spring, negative DWPT trends are found over south-west Western Australia, Tasmania and north-east Queensland, whereas positive trends (mostly 0.20–0.40°C decade–1) are found over the north-west, central and most of the southern and eastern parts of the continent. In summer, DWPTs increase generally everywhere except over Tasmania.
The increase in humidity at the 850-hPa level over northern Australia is in accordance with observed increases in rainfall across all seasons, but especially in the north-west in the period of October–April (Clark et al. 2018). This is also in accordance with an increase in the annual number of days with the Australian north-west cloudband in recent decades (Reid et al. 2019) and with an increase in frequency of long-duration persistent rain events (Dey et al. 2020).
The decreasing humidity at 850 hPa over the south-west and south-east of Australia during winter months is consistent with observed reductions in rainfall in these areas (Hope et al. 2006; Risbey et al. 2009). It was previously found that large decreases for both seasonal and annual rainfall in south-west Western Australia and southern Australia are primarily due to the southward shift in rain-bearing synoptic circulations since the 1970s, which have been partly attributed to natural variability, to expansion of the Hadley cell forced by an increasing surface global warming (Nguyen et al. 2015) and stratospheric ozone depletion (Hope et al. 2006; Frederiksen and Frederiksen 2011; Dey et al. 2020). The decreasing trend in humidity over north-east Queensland could potentially be a response to changes in the atmospheric circulation that generate anomalous subsidence at high and middle levels of the atmosphere, inhibiting convective formation of clouds over the region; there is also evidence of weakened tropical easterlies that consequently reduce the moisture advection onto the coast (Taschetto and England 2009).
Maps of 1965–2017 trends in mean DWPT at the 850-hPa level (Fig. 11) are much smoother than maps of trends in mean rainfall (Fig. 13) given that there are far fewer stations in the upper-air network (22) than in the rainfall network, resulting in less detail shown on the 850-hPa trend maps. However, there is broad consistency between respective maps (Fig. 11 and 13), reflecting corresponding trends in both variables.
Maps of 1965–2017 trends in mean rainfall: (a) annual, (b) autumn (MAM), (c) winter (JJA), (d) spring (SON) and (e) summer (DJF). Units are millimetres per decade.
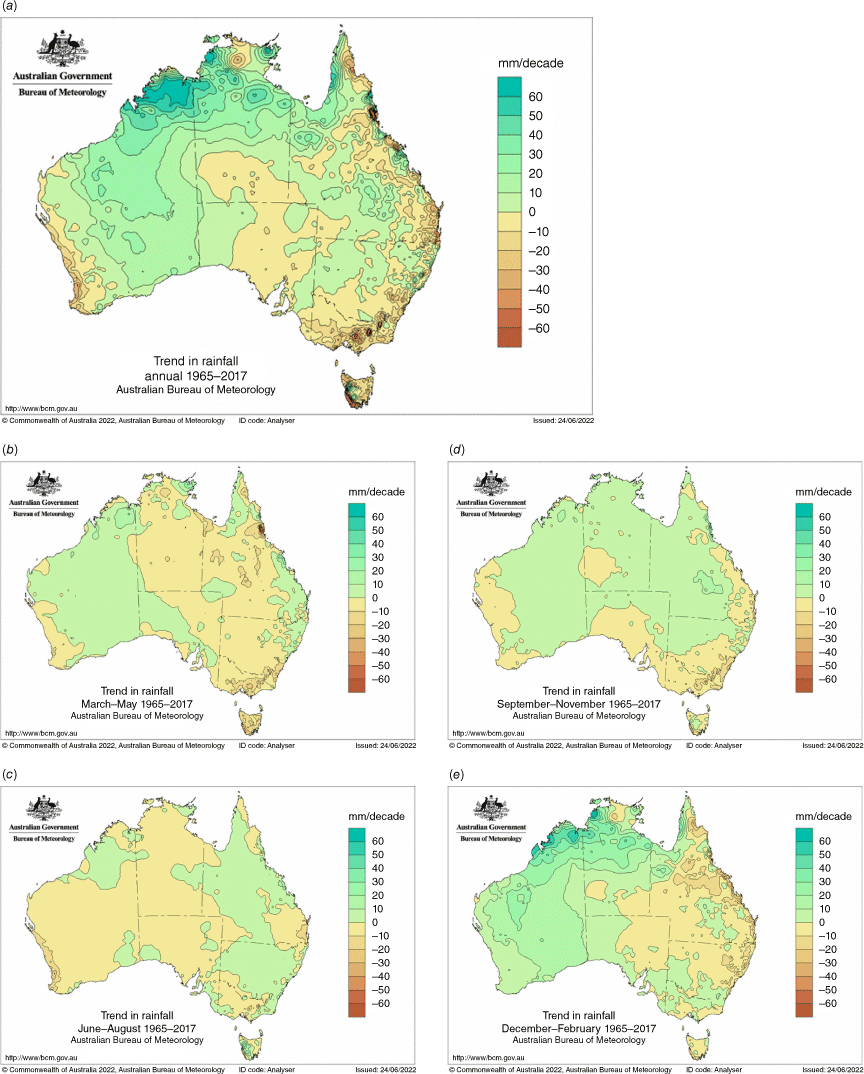
Analysis of trend maps for the shorter period 1992–2017 (Fig. 14), which covers the time interval after the change to Vaisala radiosondes, revealed that maps at each level show generally increasing (and stronger) DWPT, confirming that the increases since 1965 are not the artificial result of the change to a new radiosonde or sensor type. The only exceptions are negative trends over parts of north-eastern Australia found at all four levels for the shorter period.
Maps of 1992–2017 trends in annual mean dew point temperature at (a) 850-, (b) 700-, (c) 500- and (d) 400-hPa levels. Units are degrees Celcius per decade. Maps are based on the homogenised modified data.
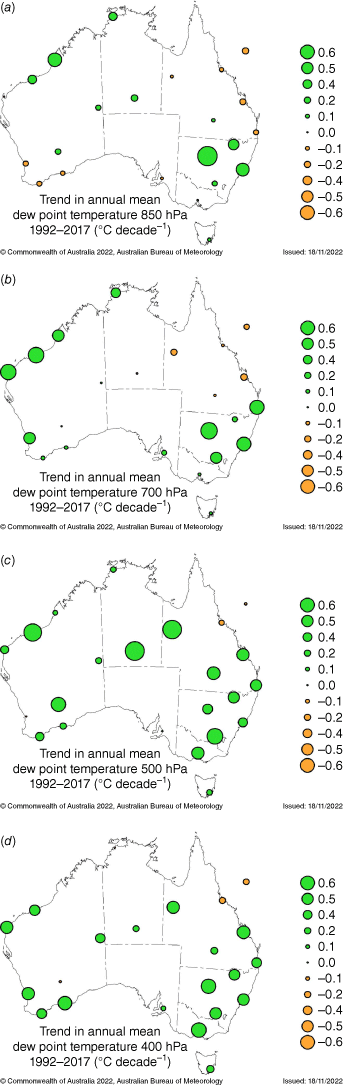
Australian remote tropical and subtropical islands show decreasing trends in DWPT at the 850-hPa level (Supplementary Table S2); by contrast, subantarctic Macquarie Island shows an increasing DWPT trend at this level. These results are generally consistent with changes in rainfall at these sites (Jovanovic et al. 2013) over the same period. At 700–400-hPa levels, there is an increasing trend in DWPT for all remote island sites except Norfolk Island, where trends are generally weak and, at the 400-hPa level, there is a decreasing DWPT trend.
6.Conclusions
The climatology, long-term trends and temporal variability of radiosonde humidity over Australia were analysed using homogenised modified monthly data from 22 stations in the Australian upper-air network. In addition, we also analysed data from the five stations located on the Australian remote islands.
Radiosonde humidity data contained sampling biases due to the inability of earlier sensors to perform sampling and reporting of cold and dry conditions. To be able to estimate trends over the longest possible period of time, it was necessary to use only data collected under the same sampling conditions. This was enabled by modifying raw DWPT time series by removing the daily DWPT data (from the later period of record) if the ambient temperature was below −40°C and the RH below 20%. This procedure removed an artificial temporal trend towards drying that existed in the raw data.
Discontinuities in the modified DWPT data were identified using a combination of historical information that explained changes in the way data were collected and processed, and objective statistical tests. Detection of discontinuities in radiosonde data was generally difficult, because of the low spatial density of the network, the absence of reliable reference series and the simultaneous changes of radiosonde or sensor types and operational procedures at all stations.
To identify major discontinuities in the modified DWPT data series, we used monthly (log-transformed) precipitation data from the candidate site or neighbouring sites as references. Break points found in the majority of data series were predominantly related to the introduction of new types of radiosondes or humidity sensors in 1982–1983, 1987–1988 and 2006–2007. Identified discontinuities were removed by adjusting data series in break points supported by the metadata. The difference between adjusted and unadjusted data was significant, with strong influence on trends at all examined levels. The adjusted (homogenised) modified data series are spatially more coherent across the Australian region and better represent long-term changes in DWPT than both the raw (original) and unhomogenised modified data. However, because the observations made under the cold and dry conditions were not included in the data series, climatology maps show higher DWPTs than is the actual case.
Trend maps for the 1965–2017 period show a general increase of humidity at the 850–400-hPa levels. Decreasing trends were found at the 850-hPa level over the south-west, south-east and north-east of Australia, consistent with the decrease of rainfall observed over these areas, particularly during winter.
The all-Australia DWPT time series showed a modulating influence from the ENSO phenomenon and increasing trends at the 850–400-hPa levels. Trends at the 700-, 500- and 400-hPa levels are significant. For 1965–2017, increasing trends are stronger at the 700- and 500-hPa levels (0.23 and 0.25°C decade–1 respectively) compared to the 850- and 400-hPa levels (0.09 and 0.15°C decade–1 respectively). This is in accordance with trend magnitudes within ±0.4°C decade–1 found by Dai et al. (2011), although these were for the shorter 1973–2008 period. The homogenised modified Australian radiosonde data for the 850-hPa level show that the amount of moisture at this level increased ~8.8% °C–1 during 1965–2015.
Finally, we compared the original (raw) and homogenised modified DWPT data over the period 1992–2017 for selected sites, to gain a better understanding of the obtained trends. During that time the only change was in 2006–2007 when the Vaisala RS80 containing a Humicap sensor was replaced with Vaisala RS92 which had a heated twin-Humicap, but the measurement range (for ambient temperature and RH) stayed the same. This comparison showed that trends in the homogenised modified data were also influenced by the absence of the observations made under the cold and dry conditions, resulting in stronger positive magnitudes over the 1992–2017 period, especially at the 500- and 400-hPa levels. This is likely also true for the longer 1965–2017 period. Nevertheless, the obtained trends are spatially coherent, better represent the pattern of change in humidity than the original (raw) data and correctly indicate a general increase in moisture at 850–400-hPa levels over the Australian region.
Further work should be directed towards the homogenisation and analysis of observed (original) data for the period starting in 1992, extending the analysis to levels above 400 hPa. Other humidity variables could also be calculated using Australian homogenised radiosonde temperature records.
Data availability
The data that support this study are available at http://www.bom.gov.au/climate/data/radiosondes/humidity.tar.gz.
Conflicts of interest
Steven Siems is an editor for the Journal of Southern Hemisphere Earth Systems Science (JSHESS) but did not at any stage have editor-level access to this manuscript while in peer review, as is the standard practice when handling manuscripts submitted by an editor to this journal. JSHESS encourages its editors to publish in the journal and they are kept totally separate from the decision-making processes for their manuscripts. The authors have no further conflicts of interest to declare.
Acknowledgements
The authors thank Dr Bertrand Timbal and Dr Chris Lucas from the Bureau of Meteorology for their comments and suggestions in the initial phases of this project, which started as a MSc thesis. Dr Blair Trewin and Dr Doerte Jakob provided valuable comments on an earlier version of the manuscript. Comments from two anonymous reviewers further improved this publication.
References
Allan RP, Barlow M, Byrne MP, Cherchi A, Douville H, Fowler HJ, Gan TY, Pendergrass AG, Rosenfeld D, Swann ALS, Wilcox LJ, Zolina O (2020) Advances in understanding large-scale responses of the water cycle to climate change. Annals of the New York Academy of Sciences 1472(1), 49-75.
| Crossref | Google Scholar |
Barnes SL (1964) A technique for maximizing details in numerical weather-map analysis. Journal of Applied Meteorology 3(4), 396-409.
| Crossref | Google Scholar |
Byrne MP, O’Gorman PA (2018) Trends in continental temperature and humidity directly linked to ocean warming. Proceedings of the National Academy of Sciences 115(19), 4863-4868.
| Crossref | Google Scholar |
Cai W, Cowan T, Thatcher M (2012) Rainfall reductions over southern hemisphere semi-arid regions: the role of subtropical dry zone expansion. Scientific Reports 2, 702.
| Crossref | Google Scholar |
Cheng L, Abraham J, Trenberth KE, et al. (2022) Another record: ocean warming continues through 2021 despite La Niña conditions. Advances in Atmospheric Sciences 39, 373-385.
| Crossref | Google Scholar |
Clark S, Reeder MJ, Jakob C (2018) Rainfall regimes over northwestern Australia. Quarterly Journal of the Royal Meteorological Society 144(711), 458-467.
| Crossref | Google Scholar |
Dai A, Wang J, Thorne PW, Parker DE, Haimberger L, Wang XL (2011) A new approach to homogenize daily radiosonde humidity data. Journal of Climate 24, 965-991.
| Crossref | Google Scholar |
Darand M, Pazhooh F, Saligheh M (2019) Trend analysis of tropospheric specific humidity over Iran during 1979–2016. International Journal of Climatology 39(10), 4058-4071.
| Crossref | Google Scholar |
Dey R, Gallant AJE, Lewis S (2020) Evidence of a continent-wide shift of episodic rainfall in Australia. Weather and Climate Extremes 29, 100274.
| Crossref | Google Scholar |
Durre I, Williams CN, Jr, Yin X, Vose RS (2009) Radiosonde-based trends in precipitable water over the Northern Hemisphere: an update. Journal of Geophysical Research: Atmospheres 114, D05112.
| Crossref | Google Scholar |
Elliott WP, Gaffen DJ (1991) On the utility of radiosonde humidity archives for climate studies. Bulletin of the American Meteorological Society 72(10), 1507-1520.
| Crossref | Google Scholar |
Frederiksen JS, Frederiksen CS (2011) Twentieth century winter changes in southern hemisphere synoptic weather modes. Advances in Meteorology 2011, 1-16.
| Crossref | Google Scholar |
Gaffen DJ, Barnett TP, Elliott WP (1991) Space and time scales of global tropospheric moisture. Journal of Climate 4, 989-1008.
| Crossref | Google Scholar |
Gimeno L, Eiras-Barca J, Durán-Quesada AM, et al. (2021) The residence time of water vapour in the atmosphere. Nature Reviews Earth & Environment 2, 558-569.
| Crossref | Google Scholar |
Held IM, Soden BJ (2000) Water vapor feedback and global warming. Annual Review of Energy and the Environment 25, 441-475.
| Crossref | Google Scholar |
Hense A, Krahe P, Flohn H (1988) Recent fluctuations of tropospheric temperature and water vapour content in the Tropics. Meteorology and Atmospheric Physics 38, 215-227.
| Crossref | Google Scholar |
Holgate CM, Evans JP, van Dijk AIJM, Pitman AJ, Virgilio GD (2020a) Australian precipitation recycling and evaporative source regions. Journal of Climate 33, 8721-8735.
| Crossref | Google Scholar |
Holgate CM, Van Dijk AIJM, Evans JP, Pitman AJ (2020b) Local and remote drivers of southeast Australian drought. Geophysical Research Letters 47(18), e2020GL090238.
| Crossref | Google Scholar |
Hope PK, Drosdowsky W, Nicholls N (2006) Shifts in the synoptic systems influencing southwest Western Australia. Climate Dynamics 26(7–8), 751-764.
| Crossref | Google Scholar |
Jovanovic B, Braganza K, Collins D, Jones D (2013) Climate variations and change evident in high-quality climate data for Australia’s Antarctic and remote island weather stations. Australian Meteorological and Oceanographic Journal 62(4), 247-261.
| Crossref | Google Scholar |
Jovanovic B, Smalley R, Timbal B, Siems S (2017) Homogenized monthly upper-air temperature data set for Australia. International Journal of Climatology 37(7), 3209-3222.
| Crossref | Google Scholar |
Kiehl JT, Trenberth KE (1997) Earth’s annual global mean energy budget. Bulletin of the American Meteorological Society 78, 197-208.
| Crossref | Google Scholar |
King AD, Klingaman NP, Alexander LV, Donat MG, Jourdain NC, Maher P (2014) Extreme rainfall variability in Australia: patterns, drivers and predictability. Journal of Climate 27, 6035-6050.
| Crossref | Google Scholar |
Lanzante JR, Klein SA, Seidel DJ (2003) Temporal homogenization of monthly radiosonde temperature data. Part I: methodology. Journal of Climate 16, 224-240.
| Crossref | Google Scholar |
Lucas C (2010) A high-quality historical humidity database for Australia. CAWCR Technical Report Number 024. (The Centre for Australian Weather and Climate Research) Available at http://cawcr.gov.au/technical-reports/CTR_024.pdf
McCarthy MP, Thorne PW, Titchner HA (2009) An analysis of tropospheric humidity trends from radiosondes. Journal of Climate 22, 5820-5838.
| Crossref | Google Scholar |
Masson-Delmotte V, Zhai P, Pirani A, Connors SL, Péan C, Berger S, Caud N, Chen Y, Goldfarb L, Gomis MI, Huang M, Leitzell K, Lonnoy E, Matthews JBR, Maycock TK, Waterfield T, Yelekçi O, Yu R, Zhou B (Eds) (2021) ‘Climate Change 2021: The Physical Science Basis. Contribution of Working Group I to the Sixth Assessment Report of the Intergovernmental Panel on Climate Change.’ (Cambridge University Press: Cambridge, UK, and New York, NY, USA) 10.1017/9781009157896
Miloshevich LM, Paukkunen A, Vömel H, Oltmans SJ (2004) Development and validation of a time-lag correction for vaisala radiosonde humidity measurements. Journal of Atmospheric and Oceanic Technology 21, 1305-1327.
| Crossref | Google Scholar |
Moradi I, Soden B, Ferraro R, Arkin P, Vömel H (2013) Assessing the quality of humidity measurements from global operational radiosonde sensors. Journal of Geophysical Research: Atmospheres 118, 14, 8040-8053.
| Crossref | Google Scholar |
Nguyen H, Lucas C, Evans A, Timbal B, Hanson L (2015) Expansion of the southern hemisphere Hadley cell in response to greenhouse gas forcing. Journal of Climate 28, 8067-8077.
| Crossref | Google Scholar |
Philipona R, Dürr B, Ohmura A, Ruckstuhl C (2005) Anthropogenic greenhouse forcing and strong water vapor feedback increase temperature in Europe. Geophysical Research Letters 32, L19809.
| Crossref | Google Scholar |
Reid KJ, Simmonds I, Vincent CL, King AD (2019) The Australian northwest cloudband: climatology, mechanisms, and association with precipitation. Journal of Climate 32, 6665-6684.
| Crossref | Google Scholar |
Risbey JS, Pook MJ, McIntosh PC, Wheeler MC, Hendon HH (2009) On the remote drivers of rainfall variability in Australia. Monthly Weather Review 137(10), 3233-3253.
| Crossref | Google Scholar |
Richardson AJ, Pattiaratchi CB (2020) Long-term changes in temperature around Australia. In ‘State and Trends of Australia’s Ocean Report’. (Eds AJ Richardson, R Eriksen, T Moltmann, I Hodgson-Johnston, JR Wallis) pp. 1.1.1–1.1.4. (Integrated Marine Observing System) 10.26198/5e169fa949e73
Ross RJ, Elliott WP (1996) Tropospheric water vapor climatology and trends over North America: 1973–93. Journal of Climate 9, 3561-3574.
| Crossref | Google Scholar |
Ross RJ, Elliott WP (2001) Radiosonde-based northern hemisphere tropospheric water vapor trends. Journal of Climate 14, 1602-1612.
| Crossref | Google Scholar |
Seaman RS (1989) Tuning the Barnes objective analysis parameters by statistical interpolation theory. Journal of Atmospheric and Oceanic Technology 6, 993-1000.
| Crossref | Google Scholar |
Sherwood SC, Roca R, Weckwerth TM, Andronova NG (2010) Tropospheric water vapour, convection, and climate. Reviews of Geophysics 48(2), RG2001.
| Crossref | Google Scholar |
Stocker TF, Qin D, Plattner G-K, Tignor MMB, Allen SK, Boschung J, Nauels A, Xia Y, Bex V, Midgley PM (Eds) (2013) ‘Climate Change 2013: The Physical Science Basis. Contribution of Working Group I to the Fifth Assessment Report of the Intergovernmental Panel on Climate Change.’ (Cambridge University Press: Cambridge, UK, and New York, NY, USA) 10.1017/CBO9781107415324
Tabari H (2020) Climate change impact on flood and extreme precipitation increases with water availability. Scientific Reports 10, 13768.
| Crossref | Google Scholar |
Taschetto AS, England MH (2009) An analysis of late twentieth century trends in Australian rainfall. International Journal of Climatology 29, 791-807.
| Crossref | Google Scholar |
Trenberth KE (2012) Framing the way to relate climate extremes to climate change. Climatic Change 115, 283-290.
| Crossref | Google Scholar |
Trenberth KE, Fasullo J, Smith L (2005) Trends and variability in column-integrated atmospheric water vapor. Climate Dynamics 24, 741-758.
| Crossref | Google Scholar |
Trenberth KE, Smith L, Qian T, Dai A, Fasullo J (2007) Estimates of the global water budget and its annual cycle using observational and model data. Journal of Hydrometeorology 8, 758-769.
| Crossref | Google Scholar |
Van der Ent RJ, Savenije HHG (2011) Length and time scales of atmospheric moisture recycling. Atmospheric Chemistry and Physics 11, 1853-1863.
| Crossref | Google Scholar |
Wang XL (2008a) Penalized maximal F test for detecting undocumented mean shift without trend change. Journal of Atmospheric and Oceanic Technology 25, 368-384.
| Crossref | Google Scholar |
Wang XL (2008b) Accounting for autocorrelation in detecting mean shifts in climate data series using the penalized maximal t or F tests. Journal of Applied Meteorology and Climatology 47, 2432-2444.
| Crossref | Google Scholar |
Wang XL, Feng Y (2013) RHTestV4 User Manual. Available at http://etccdi.pacificclimate.org/RHtest/RHtestsV4_UserManual_20July2013.pdf
Wang XL, Wen QH, Wu Y (2007) Penalized maximal t test for detecting undocumented mean change in climate data series. Journal of Applied Meteorology and Climatology 46, 916-931.
| Crossref | Google Scholar |
Xie B, Zhang Q, Ying Y (2011) Trends in precipitable water and relative humidity in China 1979–2005. Journal of Applied Meteorology and Climatology 50, 1985-1994.
| Crossref | Google Scholar |
Zhao T, Dai A, Wang J (2012) Trends in tropospheric humidity from 1970 to 2008 over China from a homogenized radiosonde dataset. Journal of Climate 25(13), 4549-4567.
| Crossref | Google Scholar |