Evaluation of ACCESS-S1 seasonal forecasts of growing season precipitation for Western Australia’s wheatbelt region
Rebecca Firth

A Environmental and Conservation Sciences and Harry Butler Institute, Centre for Terrestrial Ecosystem Science and Sustainability, Murdoch University, Murdoch, WA 6150, Australia.
B Australian Bureau of Meteorology, Melbourne, Vic., Australia.
C Centre for Crop and Food Innovation, Murdoch University, Murdoch, WA 6150, Australia.
Journal of Southern Hemisphere Earth Systems Science 73(2) 131-147 https://doi.org/10.1071/ES22031
Submitted: 24 September 2022 Accepted: 16 May 2023 Published: 7 June 2023
© 2023 The Author(s) (or their employer(s)). Published by CSIRO Publishing on behalf of the Bureau of Meteorology. This is an open access article distributed under the Creative Commons Attribution-NonCommercial-NoDerivatives 4.0 International License (CC BY-NC-ND)
Abstract
Seasonal forecasts are increasingly important tools in agricultural crop management. Regions with Mediterranean-type climates typically adopt rain-fed agriculture with minimal irrigation, hence accurate seasonal forecasts of rainfall during the growing season are potentially useful in decision making. In this paper we examined the bias and skill of a seasonal forecast system (ACCESS-S1) in simulating growing season precipitation (GSP) for south-west Western Australian (SWWA), a region with a Mediterranean-type climate and significant cereal crop production. Focusing on July–September (3-month) and May–October (6-month) forecasts, with 0- and 1-month lead times, we showed that overall ACCESS-S1 had a dry bias for SWWA rainfall and a tendency to simulate close to average rainfall during both wetter and drier than average rainfall years. ACCESS-S1 showed particularly poor skill at these timeframes for very wet and very dry years. The limitations in ACCESS-S1 for SWWA GSP were associated with inaccuracies in the timing of heavy rainfall events. In addition, limitations of the ACCESS-S1 model in accurately capturing SST and wind anomaly patterns over the tropical Indian Ocean during extreme rainfall years also contributed to errors in SWWA GSP forecasts. Model improvements in these regions have the potential to improve seasonal rainfall forecasts for SWWA.
Keywords: ACCESS-S1, agriculture, Bureau of Meteorology, model evaluation, rainfall, seasonal climate forecasting, south-west Western Australia, wheatbelt.
1.Introduction
Advances in our modelling and understanding of the earth–atmosphere system over recent decades have resulted in significant improvements in the skill and usefulness of intra-seasonal and seasonal climate forecasts (Meza et al. 2008). This provides opportunities for the cereal crop industry to use these forecasts to improve decision making and potentially minimise risks and increase profits. In rain-fed cereal crop regions, rainfall variability has a significant effect on crop yield and episodes of extreme conditions such as droughts can have dire consequences for crop productivity (e.g. Giunta et al. 1993; Wittwer et al. 2002). If agricultural producers are well informed about the likelihood of the next season being dryer (or wetter) than average, there is the opportunity to adjust management practices so that losses can be minimised, and gains maximised. As such, the availability of accurate and relevant intra-seasonal to seasonal forecasts can be highly valuable to agricultural producers when making management decisions such as which crop types and varieties to select, and when to sow, harvest and fertilise.
The wheatbelt of south-west Western Australia (SWWA) (Fig. 1) is home to a world-class agricultural industry and is the nation’s largest grain producing region (Department of Agriculture 2019). Grain production, which is the largest agricultural sector in the state, delivers ~A$4.6 billion to the economy of Western Australia (WA) each year, the majority of which is from cereal crops (Department of Primary Industries and Regional Development 2018). The SWWA experiences a Mediterranean-type climate that is characterised by hot, dry summers and cool, wet winters (Gentilli 1972) with over 80% of the annual rainfall falling during the cooler months (May–October) (Wright 1974). Cereal crops are grown from winter to spring and are rain-fed. Consequently, crop yields are heavily affected by inter-annual variations in precipitation (Stephens and Lyons 1998). Given the significant contribution that the agricultural industry makes to WA’s economy, accurate and meaningful forecasts of precipitation during the growing season can play an important role in supporting the future of the state’s agricultural industry.
Map showing Western Australia’s wheatbelt indicated by the potentially arable area (Geographic Information Services 2016).
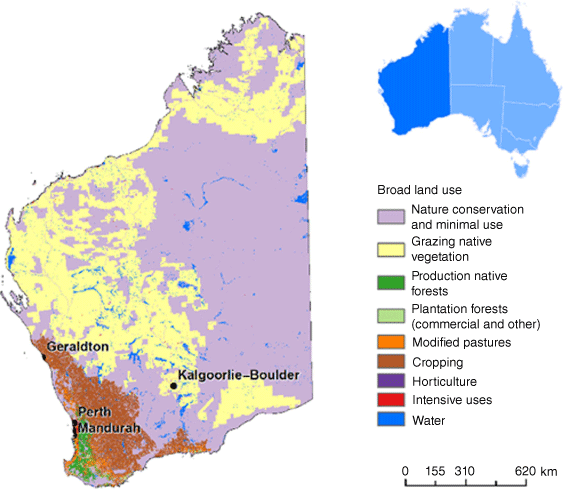
The Australian Bureau of Meteorology has been using dynamical models to provide seasonal outlooks since 2002, beginning with the Predictive Ocean Atmosphere Model for Australia (POAMA; Alves et al. 2003; Charles et al. 2015) and continuing with the ACCESS-S1 (Australian Community Climate and Earth-System Simulator – Seasonal; Hudson et al. 2017a) system, which became operational in 2018. The Bureau provides operational seasonal forecasts of the phase of the El Niño–Southern Oscillation (ENSO) and the Indian Ocean Dipole (IOD), as well as intra-seasonal and seasonal forecasts of Australian climate (http://www.bom.gov.au/climate/ahead/). ACCESS-S1 was developed as a collaboration between the Bureau of Meteorology and the UK Meteorological Office (UKMO) specifically for seasonal forecasts and offers several improvements over its predecessor, POAMA. These include an increased horizontal resolution of the atmospheric model of 60 km (compared to 250 km for POAMA) with 85 vertical levels in the atmosphere (compared to 17 levels in POAMA), and updated physics parameterisation schemes (Hudson et al. 2017a). A new version of the system, ACCESS-S2, became operational in October 2021, which incorporates a new data assimilation scheme for the ocean and land surface (Wedd et al. 2022). The focus of this study, however, is on ACCESS-S1.
ACCESS-S1 has been evaluated for a variety of applications and purposes. Several studies have compared the performance of ACCESS-S1 with that of POAMA and these have generally shown an overall improvement (Hudson et al. 2017b; Camp et al. 2018; Marshall and Hendon 2018; Gregory et al. 2019). Lim et al. (2016) assessed ACCESS-S1 skill in comparison to POAMA in forecasting seasonal rainfall and temperature for the state of Victoria in south-eastern Australia, including analysing the large-scale drivers of climate variability: ENSO, IOD and Southern Annular Mode (SAM). Several improvements were identified with ACCESS-S1, including better forecast reliability for Victorian seasonal rainfall, improved prediction of the early stages of the development of ENSO and the IOD, and greater skill in predicting Victorian rainfall in late autumn, winter and spring with up to 3 months of lead time (lead time refers to the time from when the forecast is available to when the forecast period starts). Lim et al. (2016) also showed that ACCESS-S1 tends to overestimate the magnitude of ENSO and IOD and underestimate the teleconnection of ENSO, IOD and SAM to Victorian rainfall. Although these findings are informative for seasonal rainfall prediction in Victoria where large-scale modes of variability are strongly correlated with rainfall, phenomena such as ENSO have a much weaker influence on rainfall in SWWA (Smith et al. 2000) and as such these findings have less relevance for SWWA rainfall forecast skill.
Previous studies also evaluated the ability of ACCESS-S1 to forecast rainfall extremes. For example, Marshall et al. (2021a) evaluated the predictability of extreme rainfall for each season across Australia using ACCESS-S1, with a particular focus on the influence of the Madden–Julian Oscillation (MJO). They identified that the MJO has a strong influence on the variation of rainfall extremes across Australia. However, although ACCESS-S1 did well in predicting the MJO with a lead time of up to 28 days, its ability to simulate the MJO’s relationship with extreme rainfall across Australia was limited thus inhibiting the model’s skill in forecasting extreme rainfall. The MJO is more strongly linked to rainfall patterns in northern Australia, and so again, these findings are less informative for SWWA forecasts.
King et al. (2020) evaluated ACCESS-S1’s ability to forecast various indices of rainfall extremes and how well ACCESS-S1 simulates the relationships between modes of variability and the extreme rainfall indices across Australia. They used hindcasts that were calibrated to daily rainfall observations and carried out their analysis for 0–2-month lead times. Most relevant to this study are forecasts of total rainfall for WA. King et al. (2020) demonstrated a significant correlation between ACCESS-S1 forecasts and observations of total rainfall in WA for January, May, July, August and September at a 0-month lead time. There was no significant correlation found for 1-month lead time. The results were averaged over the entire state of WA (fig. 3 of King et al. 2020). However, SWWA and northern WA experience almost opposite rainfall climatology; SWWA experiences the majority of rainfall in winter, whereas northern WA experiences a tropical climate with the majority of rainfall in summer (Gentilli 1972). Further work is therefore required which focuses only on SWWA.
Other studies that explored ACCESS-S1 and rainfall prediction include Cowan et al. (2020) who assessed ACCESS-S1 skill in predicting the northern rainfall onset (NRO) by evaluating the raw forecasts as well as the mean bias corrected forecasts and the forecasts calibrated against observations. The raw forecasts performed well in simulating the observed median NRO over the given timeframe, whereas the calibrated forecasts were most skilful in capturing the interannual variability of the NRO. Cowan et al. (2022) also explored ACCESS-S1 hindcasts to evaluate the model’s skill in predicting tropical burst activity in northern Australia during summer, with results demonstrating reasonable predictive skill with up to 2-weeks lead time. Although these studies identified skill in ACCESS-S1 in simulating rainfall indices in northern Australia, they have little relevance for rainfall forecasts for WA’s wheatbelt region.
Marshall et al. (2021b) evaluated ACCESS-S1 skill in simulating and predicting extreme fire weather across Australia during spring and summer, focusing on the roles of various modes of variability. ACCESS-S1 scored highly for forecast skill of extreme fire weather in SWWA during September, October and November (SON). In terms of the roles of the various climate drivers on extreme fire weather prediction in SWWA, improvements in predictive skill were identified during La Niña in SON, the positive SAM phase in SON and in phases six and seven of the MJO when it is strong also in SON. Although this study shows some promising results for extreme fire weather prediction in SWWA using ACCESS-S1, the focus is on spring and summer and therefore provides limited information about the wheat growing season (May–October) in SWWA.
Some studies have assessed ACCESS-S1 seasonal forecasts for agricultural applications in Australia. Zhao et al. (2019a, 2019b) used ACCESS-S1 forecasts at daily, monthly and seasonal time-scales to investigate the skill of predicting reference crop evapotranspiration in north-east, south and south-east Australia with various lead times. Raw forecasts as well as forecasts that were post-processed using a Bayesian joint probability modelling approach were evaluated. Results demonstrated that, although raw forecasts performed poorly, the post-processed forecasts demonstrated significant skill.
Seasonal rainfall forecasts from climate models are generally shown to be more skilful when the relationship between the large-scale climate drivers and mean and extreme rainfall is robust (e.g. King et al. 2020). For example, the Southern Oscillation Index (SOI) correlates significantly with rainfall in many regions of the world, including eastern Australia, and is therefore often used as a predictive tool for seasonal forecasts (e.g. Stone et al. 1996). However, the relationship between the SOI (and other indicators of ENSO) and rainfall in SWWA is very weak in comparison and therefore limited in its usefulness for SWWA rainfall prediction (Smith et al. 2000). Rainfall during the wheat-growing season (May–October) in SWWA can largely be attributed to frontal systems and cut-off lows (Pook et al. 2012). Some studies have shown a link between Indian Ocean sea surface temperature (SST) and SWWA rainfall (e.g. Smith et al. 2000; England et al. 2006; Ummenhofer et al. 2008; Evans et al. 2020). For example, England et al. (2006) showed that there was a distinctive dipole pattern in Indian Ocean SST evident during extreme rainfall years that switched sign between wet and dry years and differed from previously identified dipoles in the region. The dipole was characterised by anomalously cool waters in the tropical–subtropical eastern Indian Ocean, alongside an area of anomalously warm water in the subtropics off SWWA during dry years, with the opposite occurring during wet years. The alternating dipole is shown to coincide with anomalous winds in the region that drive the SST changes and alter the large-scale advection of moisture onto the SWWA coast. The change in winds is also shown to correlate with stronger anticyclonic motion in the Indian Ocean during dry years and the opposite in wet years. It was also demonstrated that during some extreme rainfall years, the IOD acted to reinforce anomalous conditions when in either a positive or negative phase.
To our knowledge, no studies have evaluated ACCESS-S1 with a specific focus on the wheatbelt of SWWA during the wheat growing season (May–October). This presents an area where further research could potentially bring significant value, especially considering the importance of the cereal crop industry to WA’s economy. The aim of this study is to evaluate ACCESS-S1 precipitation forecasts during the growing season for SWWA, with particular focus on their use for agricultural decision-making, and to explore the dynamical processes which lead to model biases.
2.Methods
2.1. Data
The ACCESS-S1 forecast system includes a coupled atmosphere–ocean–land surface model based on UKMO’s global coupled model seasonal forecast system GloSea5-GC2 (Global Seasonal forecast system version 5 using the Global Coupled model configuration 2; MacLachlan et al. 2015). It comprises the Unified Model (UM; Williams et al. 2015; Walters et al. 2017) for the atmosphere, the Joint UK Land Environment Simulator (JULES; Best et al. 2011; Walters et al. 2017), the Nucleus for European Modelling of the Ocean (NEMO; Madec and The NEMO team 2008; Megann et al. 2014) and the Los Alamos sea ice model (CICE; Hunke and Lipscomb 2008). ACCESS-S1 has a horizontal resolution of ~60 km (N216) with 85 vertical levels in the atmosphere, four soil levels in the land surface model and an ocean model resolution of 0.25° with 75 vertical levels. For more details on ACCESS-S1, see Hudson et al. (2017a).
The ACCESS-S1 forecasts are evaluated based on a set of retrospective forecasts known as hindcasts. Initial conditions for the hindcasts come from ERA-Interim (Dee et al. 2011) for the atmosphere and from the FOAM (Forecast Ocean Assimilation Model) analyses (Blockley et al. 2014) for the ocean. The ACCESS-S1 hindcasts are available for the period 1990–2012 and start on the 1st, 9th, 17th and 25th of each month. The hindcasts include an 11-member ensemble forecast 7 months into the future. We created a time-lagged 22-member ensemble for each of the lead times by combining the forecasts initialised on the 1st of the month with those from the 25th of the previous month. For example, the May–October forecast with 1-month lead time, used forecasts from 1 April together with those from 25 March (i.e. 11 members from 1 April combined with 11 members from 25 March). For the July–September forecast with 0-month lead time, forecasts from 1 July were combined with those from 25 June. For the 6-month forecasts for May–October, the last 4 days of October were removed from the forecasts initialised on 1 April because the forecasts initialised on 25 March only went out to 27 October. Creation of a time-lagged ensemble is common practice in seasonal prediction to increase the ensemble size and better capture uncertainties. The Bureau’s real-time forecast products utilise a time-lagged ensemble by combining 9 successive days of forecasts for the seasonal timescale (11 members are run every day, thus this approach builds a 99-member ensemble; Hudson et al. 2017a; Wedd et al. 2022). We did, however, repeat our analysis of the mean biases (Section 3.1) to compare the results of an 11-member ensemble from the 1st of the month with an 11-member ensemble on the 25th of the month – the results were very similar to each other and to those from the 22-member ensemble (not shown).
To assess ACCESS-S1 rainfall, we compared the forecasts to daily 5-km gridded observations from the Australian Water Availability Project (AWAP) dataset (Jones et al. 2009). This dataset is an interpolation of direct surface measurements recorded from a network of weather stations across Australia. The number of stations recording data varies in time and by variable, and precipitation is interpolated from 5000 to 7000 stations across Australia. A map of the rainfall stations used across Australia showing the density within the SWWA region can be found in fig. 2a of Jones et al. (2009). The AWAP dataset has been used for model evaluation purposes in several studies (e.g. Kala et al. 2015; Zhao et al. 2021; Shao et al. 2022). To be consistent with ACCESS-S1, the last 4 days of October were also removed from the AWAP dataset. To enable comparison of the ACCESS-S1 forecasts with observed precipitation, the AWAP precipitation data were re-gridded to the 60-km ACCESS-S1 grid using the first-order conservative remapping tool, remapcon, from Climate Data Operators (https://code.mpimet.mpg.de/projects/cdo/), and a land fraction of 0.3 was chosen as the threshold to determine the land–sea mask.
To explore the large-scale processes in ACCESS-S1, we used the ERA5 reanalysis (Hersbach et al. 2020) as a surrogate truth for key atmospheric variables such as winds and SST. The ERA5 is a re-analysis produced from the European Centre for Medium Range Weather Forecasts, replacing and improving upon the previous ERA-Interim reanalysis (Dee et al. 2011). It has a horizontal resolution of ~30 km and there are 137 vertical levels from the surface up to 0.01 hPa. To enable comparison with ACCESS-S1, the ERA5 data were re-gridded to the 60-km ACCESS-S1 grid and the last 4 days of October were removed. We compared ERA5 growing season precipitation (GSP) to AWAP, and these were very similar (not shown), hence, warranting the use of ERA5 data to explain precipitation biases in ACCESS-S1.
2.2. Analysis
Two forecast periods during the growing season were selected for analysis based on the timing of key rainfall-related management decisions. One of the most important management decisions involves choosing how much fertiliser to apply so that favourable conditions are either exploited to maximise profits, or resources are conserved during less favourable conditions to minimise losses. Fertiliser is initially applied at the beginning of the growing season based on the rainfall outlook for the season ahead. Decisions are then made as to whether to apply a ‘top up’ of fertiliser approximately mid-way through the season based on rainfall that has already fallen and the outlook for the remainder of the season. To tie into these two critical decision-making time periods, this study evaluated May–October (MJJASO) and July–September (JAS) precipitation forecasts with both 0- and 1-month lead times.
The forecasts were analysed based on wet, dry and average rainfall years. To classify years as wet, dry and average, the SWWA domain (36–28°S; 114–122°E; Fig. 2) averaged anomaly for the MJJASO growing season (GS) was calculated from the AWAP observational data for each year. Based on an evaluation of all of these values together, a subjective decision was made to use the anomaly thresholds as follows: <−40 mm per GS = dry year, >40 mm per GS = wet year and >−40 mm per GS and <40 mm per GS = average year. This resulted in 6 dry years (2000, 2002, 2006, 2007, 2010 and 2012), 6 wet years (1992, 1996, 1998, 1999, 2005 and 2011) and 11 average years (1990, 1991, 1993, 1994, 1995, 1997, 2001, 2003, 2004, 2008 and 2009) for analysis. We note that because years are classified as either wet, dry or average based on the entire growing season, for some years, the JAS anomaly may not necessarily show a positive (negative) anomaly for a wet (dry) year. Although it could have been possible to also use the JAS anomaly to classify years, this would have resulted in a different set of years being classified as wet, dry and average for JAS compared with MJJASO. Because the interest is largely in whether a particular growing season will be either very wet or very dry overall, for simplicity we just used the anomaly for the MJJASO period to classify years.
Anomalies for the ACCESS-S1 hindcasts were calculated by computing the climatology (1990–2012) from the ensemble mean hindcasts for each start date. The climatology was then subtracted from the ensemble mean (or individual ensemble members) for each start date to produce the forecast anomalies, and in so doing a first-order linear correction for model bias or drift was made (Hudson et al. 2017b).
The AWAP dataset was used as a reference to estimate the errors in ACCESS-S1 GSP, and the ERA5 dataset allowed an exploration of the dynamical processes driving the errors. We examined the ACCESS-S1 ensemble mean precipitation during the growing season spatially over the SWWA domain and compared it with the AWAP observations, for all years, as well as wet, dry and average years. We also assessed the ensemble spread, displayed as box and whisker plots for the SWWA region.
To contribute to an understanding of the model errors in rainfall over SWWA, the ability of ACCESS-S1 to capture the frequency and timing of rain-days of different intensities, i.e. light to heavy rainfall events, was evaluated. Five rain-day categories were defined based on the 25th, 50th, 75th and 95th percentiles for the daily domain sum of rainfall from ACCESS-S1 and AWAP (Table 1). ACCESS-S1 had lower rainfall values than AWAP across all percentiles (Table 1). The percentiles were used to generate five rain-day categories (Table 2). We examined the frequency of different rain-day categories in ACCESS-S1 and AWAP for wet, dry and average years. Additionally, we examined the timing of the most extreme rainfall (i.e. categories 4 and 5; Table 2), as these heavy rainfall events can have significant impacts. The rain-day category analysis was based on the entire growing season and 0-month lead time.
Percentile | ACCESS-S1 | AWAP |
---|---|---|
25 | 4.1 | 5.8 |
50 | 27.3 | 35.6 |
75 | 120.9 | 168.7 |
95 | 493.6 | 604.4 |
Category | Percentile range |
---|---|
1 | ≤25th |
2 | >25th and ≤50th |
3 | >50th and ≤75th |
4 | >75th and ≤95th |
5 | >95th |
The final part of the analysis focused on the large-scale circulation, including SST and wind anomalies, and how well these were captured by ACCESS-S1 during wet and dry years.
3.Results and discussion
3.1. Spatial analyses of mean bias
The first stage of our analysis involved evaluating the ensemble mean bias in ACCESS-S1 spatially across SWWA for the full growing season of MJJASO as well as for the JAS forecast period for lead times of 0- and 1-month. This allowed us to initially assess how the model errors varied regionally. Fig. 3 shows the total precipitation for JAS and MJJASO averaged over 1990–2012 for the AWAP observations (re-gridded to ACCESS-S1 resolution) and for the ACCESS-S1 ensemble mean at 0- and 1-month lead times. The observations showed that most rainfall occurred in the south-west corner and there was a clear south-west to north-east gradient with rainfall decreasing moving further inland. Although ACCESS-S1 captured this rainfall pattern well, rainfall in the south-west corner was markedly underestimated, with a notable dry bias evident. This was apparent in both forecast periods and there was no obvious drift in the bias with lead time. These findings are consistent with those of Hudson et al. (2017a), who showed that although the rainfall climatology in ACCESS-S1 is much improved compared to POAMA, its predecessor, there is still a dry bias over SWWA.
Total precipitation averaged over 1990–2012 from observations (left panels) and ACCESS-S1 with 0-month (middle panels) and 1-month lead times (right panels) for (a) July–September and (b) May–October.
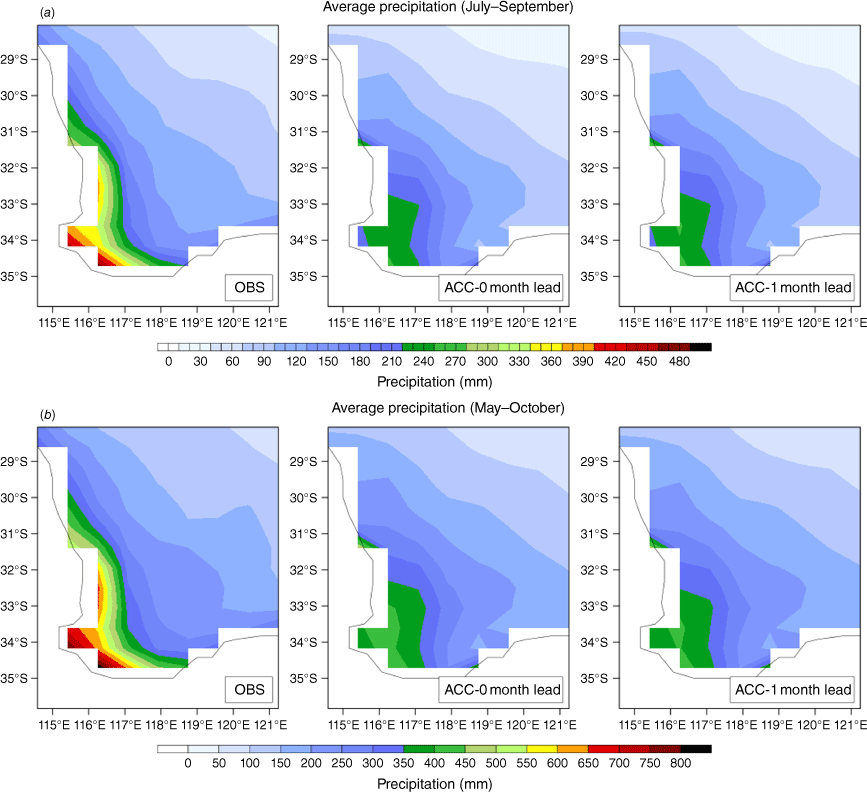
Similarly, Fig. 4–6 show the precipitation anomalies for dry, wet and average years respectively (refer to Methods for definitions of wet, dry and average). For dry years (Fig. 4), although the ACCESS-S1 anomalies were negative in some areas of SWWA, the forecasts did not capture the significant deficiency of rainfall for these years. The ACCESS-S1 forecasts suggested closer-to-average conditions rather than the dry conditions that were observed. This bias appeared more pronounced for the 1- compared with the 0-month lead time particularly for the MJJASO forecast (Fig. 4b). ACCESS-S1 better captured the wet years compared to dry years in that the precipitation anomalies were largely positive across most of SWWA (Fig. 5). However, the forecasts still showed a dry bias, slightly more evident at 1- compared to 0-month lead time. During average years, forecasts at 0-month lead time showed slightly drier conditions whereas the forecast at 1-month lead time showed average to neutral conditions (Fig. 6).
Precipitation anomalies using a 1990–2012 climatology averaged across dry years for observations (OBS) and ACCESS-S1 with 0- and 1-month lead time for (a) July–September and (b) May–October.
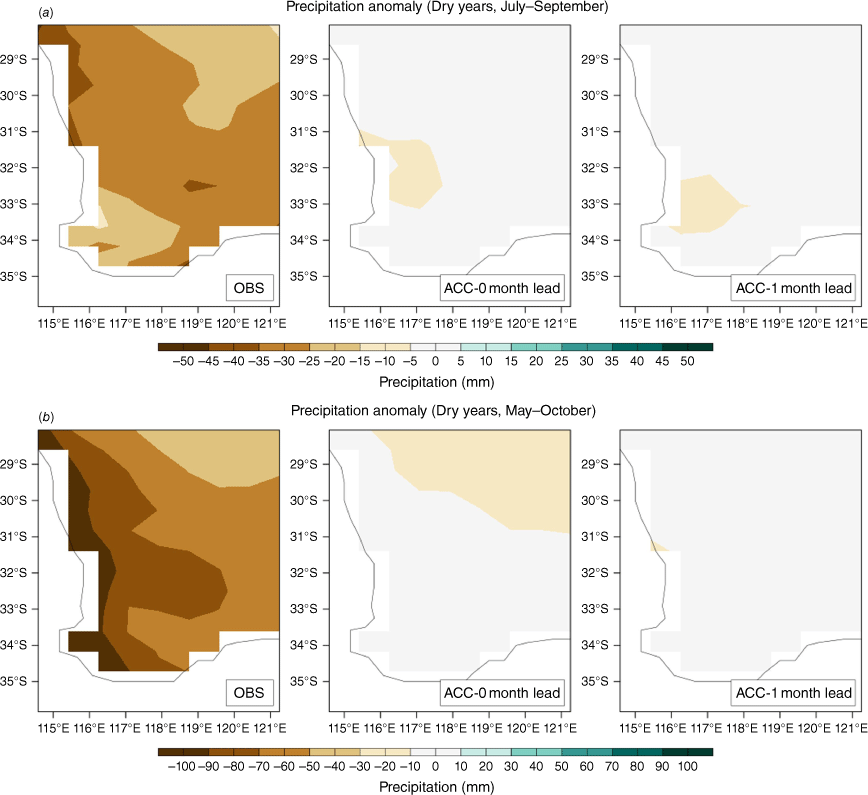
Precipitation anomalies using a 1990–2012 climatology averaged across wet years for observations (OBS) and ACCESS-S1 with 0- and 1-month lead time for (a) July–September and (b) May–October.
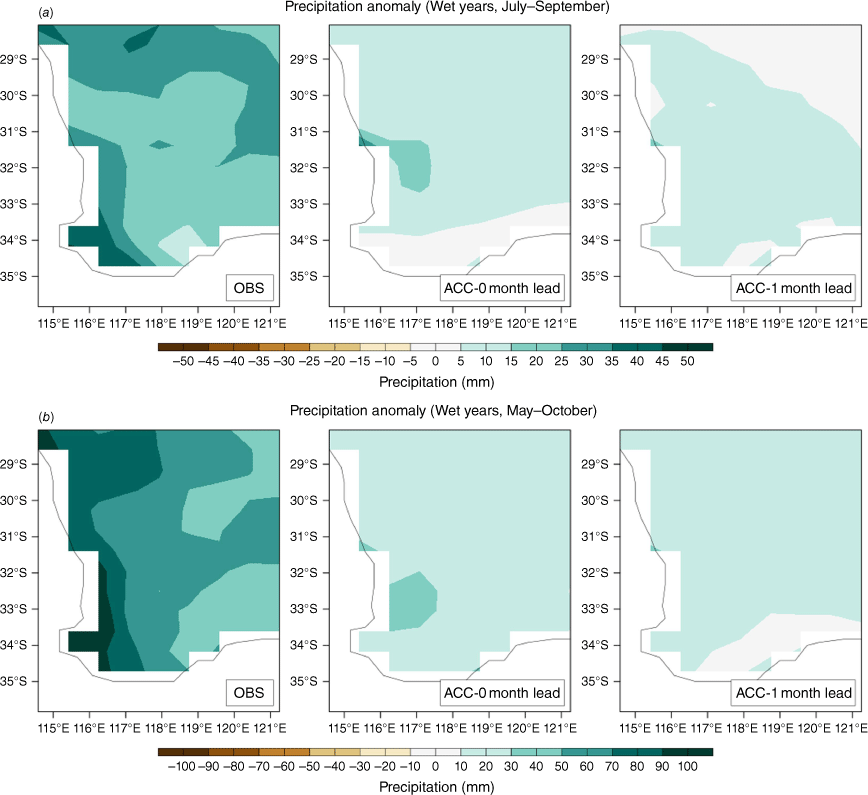
Precipitation anomalies using a 1990–2012 climatology averaged across average years for observations (OBS) and ACCESS-S1 with 0- and 1-month lead time for (a) July–September and (b) May–October.
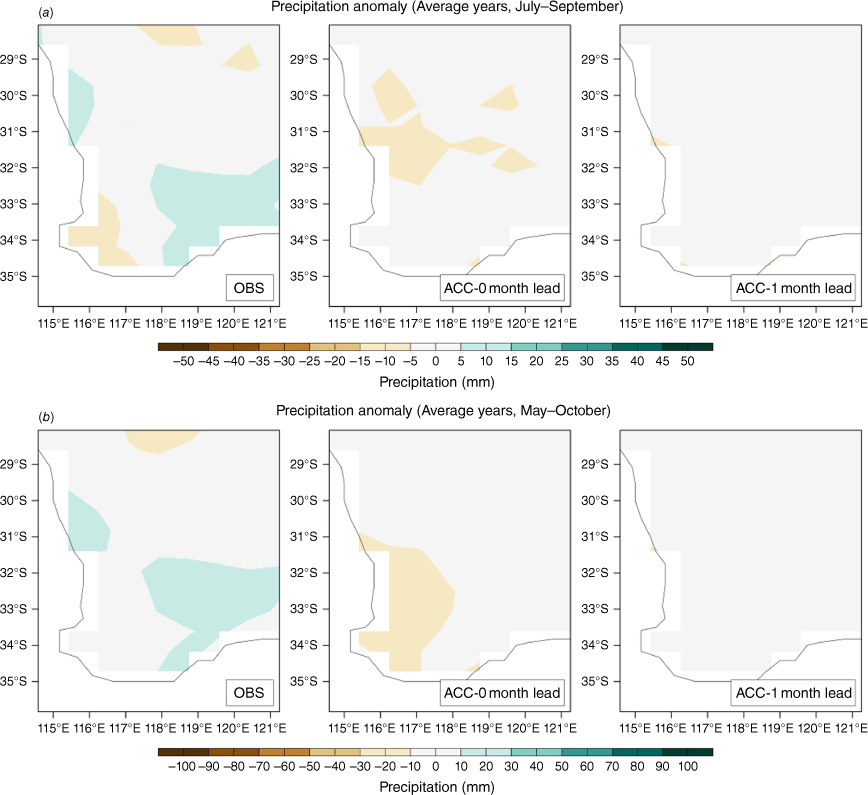
The results in Fig. 4–6 demonstrated an overall poor performance of ACCESS-S1 in simulating GSP for SWWA. Having identified the bias in the ensemble mean forecasts, we took a more probabilistic approach, and examined the ensemble spread for all years combined as well as each individual year, to provide an assessment of forecast skill.
3.2. Ensemble spread and forecast skill
The ensemble spread for the SWWA region is illustrated in Fig. 7–9. These show the median and spread of the ACCESS-S1 ensemble precipitation anomalies averaged across SWWA compared with observed for dry, wet and average years respectively. The JAS and MJJASO forecasts are shown for both 0- and 1-month lead times. All years are plotted together in panel (a) and each year is plotted separately in panel (b) to provide an indication of forecast skill.
Median and spread of ACCESS-S1 ensemble anomalies averaged over SWWA compared with observed anomaly (red dots) for 0- and 1-month lead times and for July–September (JAS) and May–October (MJJASO) forecasts for (a) all dry years combined and (b) each dry year (boxes show 25th to 75th percentiles, whiskers show Q1 − 1.5 × IQR and Q3 + 1.5 × IQR, outliers (open circles) show >1.5 × IQR).
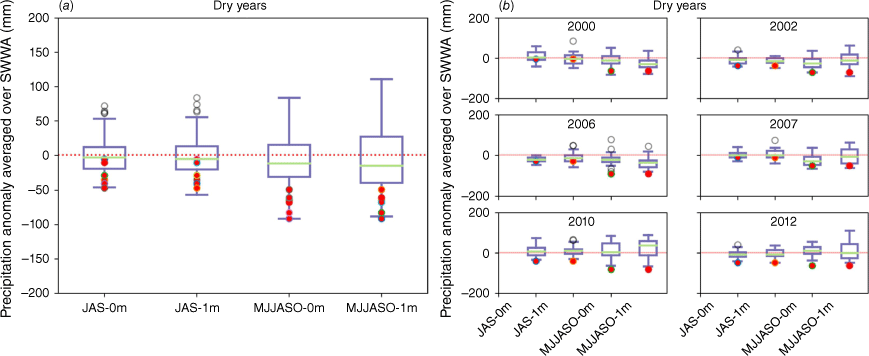
Median and spread of ACCESS-S1 ensemble anomalies averaged over SWWA compared with observed anomaly (red dots) for 0- and 1-month lead times and for July–September (JAS) and May–October (MJJASO) forecasts for (a) all wet years combined and (b) each wet year (boxes show 25th to 75th percentiles, whiskers show Q1 − 1.5 × IQR and Q3 + 1.5 × IQR, outliers (open circles) show >1.5 × IQR).
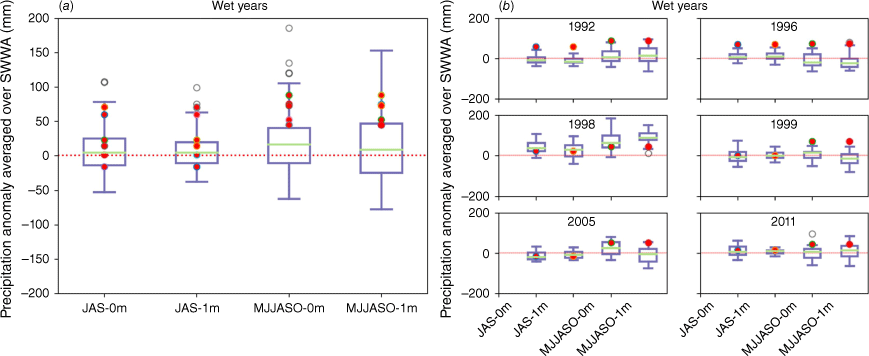
Median and spread of ACCESS-S1 ensemble anomalies averaged over SWWA compared with observed anomaly (red dots) for 0- and 1-month lead times and for July–September (JAS) and May–October (MJJASO) forecasts for (a) all average years combined and (b) each average year (boxes show 25th to 75th percentiles, whiskers show Q1 − 1.5 × IQR and Q3 + 1.5 × IQR, outliers (open circles) show >1.5 × IQR).
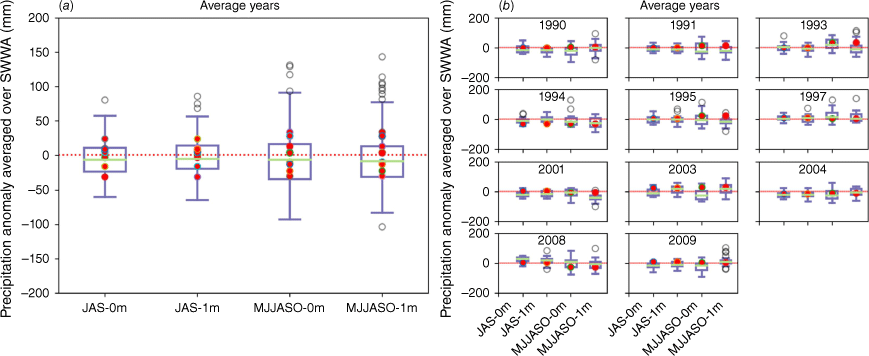
Overall, Fig. 7–9 show that regardless of whether the season was very wet or very dry, the ACCESS-S1 ensemble median forecast anomaly was generally weak. This is consistent with our spatial analysis of the ensemble mean (Fig. 4–6) that showed close to average mean forecasts during both dry and wet years. For dry years, the ACCESS-S1 ensemble spread generally captured the observed precipitation anomalies, but mostly within the lower tail of the distribution, explaining why the ensemble mean precipitation for dry years was not dry enough (Fig. 4). For the JAS forecasts, the ensemble median anomaly was closer to the observed anomaly compared with the MJJASO forecasts for most years, with the exception of 2010 and 2012. Notably, these were both particularly dry years. We note that all years were classified as wet, dry or average based on the precipitation anomaly of the MJJASO period. Hence, for some years, the observed JAS rainfall anomaly for the dry or wet years may be closer to zero if these anomalies happened predominantly during May–June or in October.
The years when the observed anomalies were most negative, i.e. the driest years (2006, 2010 and 2012) were the years when the ACCESS-S1 ensemble spread least consistently captured the observed anomaly. For example, in 2010, one of the driest years on record, none of the forecasts for either time frame or lead time captured the observed anomalies in its ensemble spread. Furthermore, for the MJJASO forecast at 1-month lead time, the ensemble median actually showed a forecast with a positive anomaly. This implies that ACCESS-S1 had limited skill in capturing more extreme conditions. When examining all years together, the ensemble spread increased with lead time for dry years. However, this pattern was not consistent for each individual year. For example, for the year 2000 the ensemble spread decreased with increasing lead time for both the JAS and the MJJASO forecast periods.
For wet years (Fig. 8), the opposite was shown, with the observations mostly in the upper tail of the ensemble spread. We note that the observed anomalies for some years for the JAS period were close to zero or negative, suggestive of average or dry conditions. The classification of those years being wet was due to the wetter-than-average conditions experienced in May–June or October rather than the JAS period. ACCESS-S1 performed marginally better for wet years compared to dry years in that the average anomaly was generally positive across the region. When examining the forecasts for all wet years, for the MJJASO forecast period, the observations were mostly within the upper tail of the ensemble spread. This can explain why ACCESS-S1 overall did not simulate large enough positive precipitation anomalies during wet years, as illustrated in Fig. 5. For the 1-month lead time for MJJASO, it is worth noting that the spread in ACCESS-S1 was much larger above the median and the observations generally lay in the lower part of the upper tail. The larger upper tail in the ensemble spread for the 1-month lead time forecast shows that there was a large range of values among the higher rainfall forecasts and hence less agreement among ensemble members. When examining all wet years, the ensemble spread decreased for increasing lead time for the JAS forecast but increased for the MJJASO forecast. It is expected that with increasing lead time there would be more uncertainty with a forecast and increased spread, as demonstrated for MJJASO, and it may be that the spread difference between the two lead times for JAS was not significantly different. For wet years (Fig. 8b), forecast skill appeared poorest during the wettest years (1992, 1996 and 1999), supporting the premise that ACCESS-S1 is limited in its forecasts of extreme rainfall in this region and time of year. Notably, for 1992 and 1996, when a significant proportion of the rainfall occurred during the JAS period, the observed anomaly was still not captured within the ensemble spread even for the shorter forecast period of 3 months and 0-month lead time.
For average years (Fig. 9), the observed anomalies fell within the middle range of the ensemble spread, which is to be expected since ACCESS-S1 tends to forecast average conditions for these forecast periods and lead times as shown earlier. Across all years, the ensemble spread increased slightly with increasing lead time for the JAS forecast but decreased for the MJJASO forecast.
The results above (Fig. 7–9) showed that model skill was limited, especially for very dry and very wet years. Previous studies that investigated seasonal precipitation forecasts in ACCESS-S1 have focused on periods of 1 month (e.g. King et al. 2020) and up to a 3-month season (e.g. Hudson et al. 2017b; Marshall et al. 2021a). King et al. (2020) examined the area-averaged correlation coefficient matrices for each state of Australia for each calendar month and for both 0- and 1-month lead times. Relevant to the present study, the correlation for total precipitation averaged across WA at 0-month lead time with observed, was statistically significant for forecasts for May, July, August and September. However, with 1-month lead time, none of the monthly forecasts had significant correlation coefficients for WA, demonstrating that forecasts beyond the first month performed poorly for the broader region. Similarly, Hudson et al. (2017b) evaluated ACCESS-S1 skill for forecasts of four separate seasons of 3 months duration for the key horticultural regions across Australia at both 0- and 1-month lead times. For the SWWA region, there were no significant correlations with observations for any of the forecasts (refer to fig. 2, A1 and A2 of Hudson et al. 2017b). Marshall et al. (2021a) examined correlations between the MJO and extreme precipitation across Australia. For SWWA during strong phases of the MJO, they showed some skill during SON and DJF for small parts of SWWA (fig. 11 of Marshall et al. (2021a)), but not during MAM and JJA, which are the key seasons during the growing season. Our results demonstrated that forecasts for a longer period, i.e. a 6-month growing season, were similarly limited in terms of skill.
3.3. Rain-day category frequency and timing based on percentiles
We next investigated how accurately ACCESS-S1 captured the frequency and timing of light to heavy rainfall events (rainfall was aggregated over the SWWA domain, i.e. gridboxes receiving rainfall were summed to create the domain total) to understand how these variables contributed to the inaccuracies in the ACCESS-S1 GSP forecasts. Fig. 10 shows the relative frequency of the different rain-day categories for ACCESS-S1 and AWAP for dry and wet years. For dry years, ACCESS-S1 overestimated the frequency of categories 4 and 5 but underestimated the driest category (rainfall less than the 25th percentile). The overestimation of the heavier rainfall categories contributed to ACCESS-S1 not sufficiently capturing the dry conditions during dry years (Fig. 4 and 7). For wet years, ACCESS-S1 had a very similar rainfall distribution to AWAP, perhaps slightly underestimating the heaviest rainfall categories.
Relative frequency of rain-day categories (Table 2) for (a) dry years and (b) wet years for ACCESS-S1 at 1-month lead time and AWAP (error bars for ACCESS-S1 denote the standard deviation of the ensemble mean for each category).
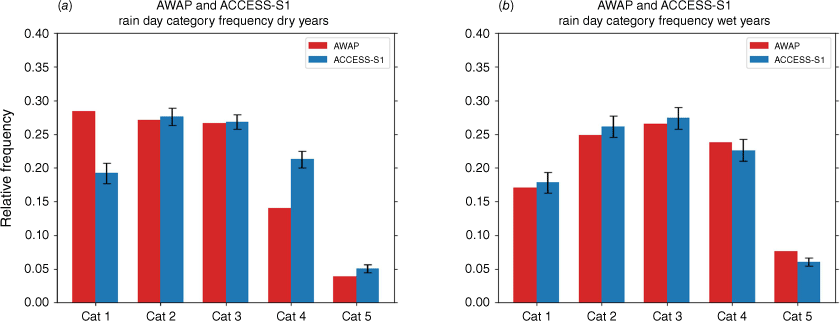
We next examined the timing of the heavy rainfall events showing the relative frequency of rain-day categories 4 and 5 for ACCESS-S1 compared with AWAP for dry and wet years for each month of the growing season (Fig. 11). For dry years, it is most notable that ACCESS-S1 forecast too many extreme (category 5) rainfall days in May and June but too few for July–October. The differences between ACCESS-S1 and AWAP were less marked for the timing of category 4 rain-days. Category 5 rain-days represent extreme rainfall events which, in this region, are most commonly driven by strong cold fronts traversing the region during winter (Hope et al. 2014). These results therefore suggest that during dry years, ACCESS-S1 likely simulated the intense cold fronts that bring the heavy rainfall days in SWWA too early in the season. Since these mid-latitude fronts are not strongly directly linked to any one mode of natural climate variability, this inevitably makes their predictability on seasonal timescales more challenging. King et al. (2020) showed in their evaluation of ACCESS-S1 that model performance was superior in the northern areas and the interior where climate modes of variability such as ENSO have a greater influence in comparison to the south and coastal regions. Nonetheless, as far as we are aware, this inconsistency in the timing of extreme rainfall events in SWWA has not been identified in previous evaluations of ACCESS-S1 and should be explored further when developing and improving the model.
Relative frequency for each month of the growing season (May–October) for dry years (a) category 4 and (b) category 5 rain-days and wet years (c) category 4 and (d) category 5 rain-days for AWAP and ACCESS-S1. Error bars for ACCESS-S1 denote standard deviations for ensemble means.
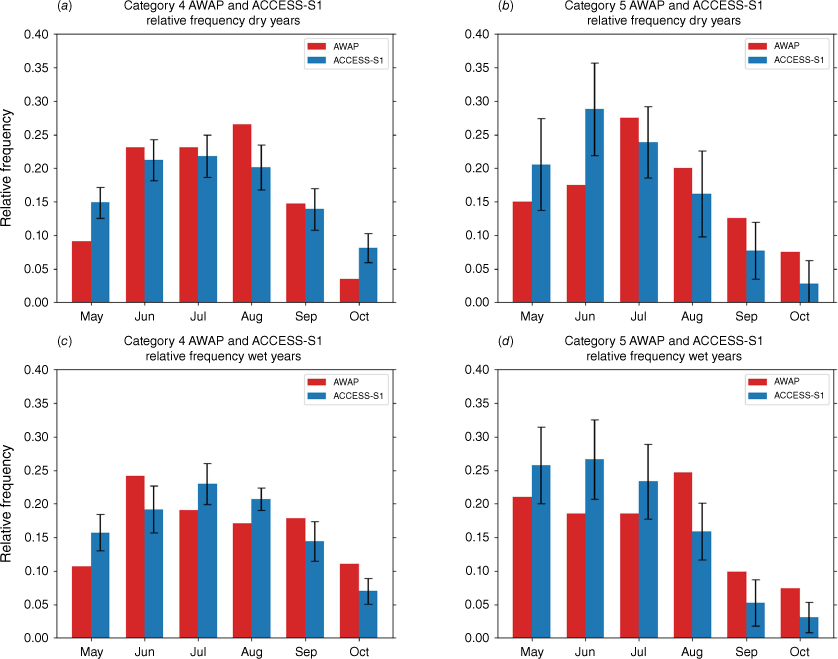
For wet years, there was a similar pattern of differences between ACCESS-S1 and AWAP for category 5 rain-days (Fig. 11b). Early growing season heavy rainfall was overestimated (May–July) and later in the season was underestimated (August–October). It is likely that during wet years too many cold fronts were simulated early in the season but too few later in the season.
The analysis of the frequency and timing of low to heavy rainfall events was based on the domain sum of rainfall across the south-west region. It is acknowledged that the majority of this rainfall comes from the south-west coast (Fig. 3) and is therefore outside of the agricultural region which is the main focus of this study and by using the domain sum the spatial differences were lost. We re-performed the analysis but focused on a smaller region of the south-west (36–28°S; 114–117.5°E), where the majority of rainfall occurs. The results obtained (not shown) were very similar to Fig. 10 and 11, demonstrating that these results were largely driven by the rainfall from this smaller region in the south-west corner. This highlights a limitation due to the relatively coarse 60-km resolution of ACCESS-S1 when examining a small region like SWWA. Higher spatial-resolution seasonal forecasts in the future would allow a better understanding of rainfall biases within the inland wheatbelt region. However, given that the main rain-bearing frontal systems would also bring rainfall inland, although with lower rainfall amounts, it is not unreasonable to suggest that a similar distribution frequency would be obtained if the percentile thresholds were relative to the inland regions.
3.4. Large-scale circulation
It was established in previous work that variations in Indian Ocean SST and surface winds can partly explain very dry–wet years in SWWA (e.g. England et al. 2006; Ummenhofer et al. 2008). Fig. 12 shows wind vector and wind speed anomalies over the growing season averaged across dry years and wet years for ERA5 compared with ACCESS-S1 at 0- and 1-month lead times. It is clear from ERA5 that during dry years, there is anomalous strong offshore (easterly–south-easterly) anticyclonic circulation across SWWA, suggestive of the drier conditions that were observed. The wind pattern identified here during dry years is similar to previous results (fig. 4 of England et al. 2006) despite the different reanalysis (NCEP v. ERA5 re-analysis) and timeframes (yearly v. growing season) used for the analysis. It is evident that ACCESS-S1 failed to capture this wind pattern driving dry conditions in the region. Instead, ACCESS-S1 simulated anomalous southerly–south-westerly winds moving across the region at 0-month lead time and weaker south-easterlies at 1-month lead time. During wet years, ERA5 showed an anomalous strong onshore (north-westerly) cyclonic circulation across SWWA, which would advect more moist air into the region, again very similar to results in England et al. (2006). There was some cyclonic motion evident in ACCESS-S1 at both lead times over SWWA; however, this was weaker than in ERA5. In ERA5 there were also strong northerly anomalies extending from the Maritime continent region and eastern tropical Indian Ocean down over WA, which were not captured in the model. Additionally, the anomalously strong wind speeds present north of Papua New Guinea and south-west of Sumatra during wet years were absent in the ACCESS-S1 simulations.
Ten-metre wind speed and direction anomalies (reference period 1990–2012) averaged for May–October for (a) dry years and (b) wet years, for ERA5 and ACCESS-S1 at 0- and 1-month lead times. Vectors indicate direction only, with the wind speed anomalies shown as colour shading.
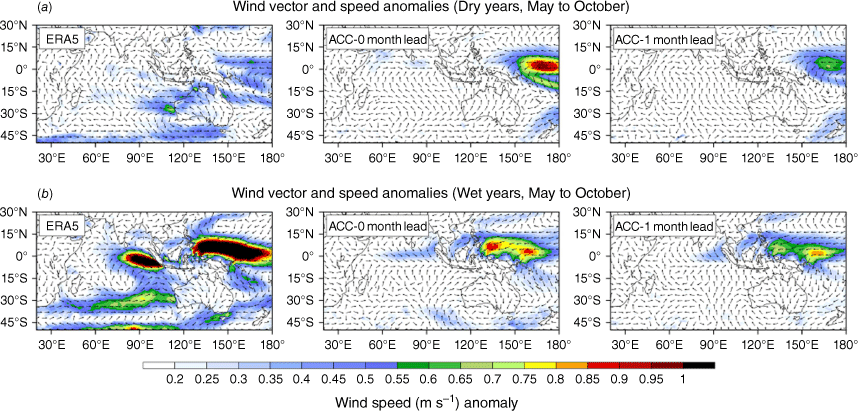
Fig. 13 shows SST anomalies averaged for May–October for ERA5 compared with ACCESS-S1 at 0- and 1-month lead times averaged for dry and wet years. England et al. (2006) identified a dipole of SST anomalies associated with wet and dry years for SWWA. This dipole has peak amplitudes in the eastern Indian Ocean adjacent to the west coast of Australia – off the north-west and south-east coast (fig. 5 in England et al. 2006). The dipole was also evident here in the ERA5 SST (Fig. 13), but with differences in the magnitude and spatial distribution due to different models, SST datasets and timeframes. During dry years there were anomalously cooler temperatures to the north of Australia and extending to Sumatra similar to the SST pole (P1) shown in England et al. (2006) (their fig. 5a) but evidently much weaker here compared to their study. Another pole of warmer temperatures was shown in the Indian Ocean to the west of WA, similar to the second pole (P2) identified by England et al. (2006). These poles switched sign during wet years; however, it is notable that, although there is symmetry between the wet and dry years in England et al. (2006), the dipoles identified in our study were asymmetrical. As described by England et al. (2006), this dipole in SST develops as a result of the anomalous wind fields in the region during extreme rainfall years. In addition, in dry years the SST anomaly in the tropics shows another dipole − a weak positive IOD pattern. The IOD is the SST anomaly difference between the western (50–70°E; 10°S–10°N) and eastern (90–110°E; 10°S–0°) tropical Indian Ocean (Saji et al. 1999). In ACCESS-S1 dry years, the anomalously cooler waters to the north of Australia were absent for both lead times, with the model simulating warmer than average SST. In addition, the tropical west–east SST anomalies in the model are indicative of a negative (0-month lead) or neutral (1-month lead) IOD.
SST anomalies (reference period 1990–2012) averaged for May–October for dry years (top row) and wet years (bottom row), for ERA5 (left) and ACCESS-S1 at 0- (middle) and 1-month (right) lead times.
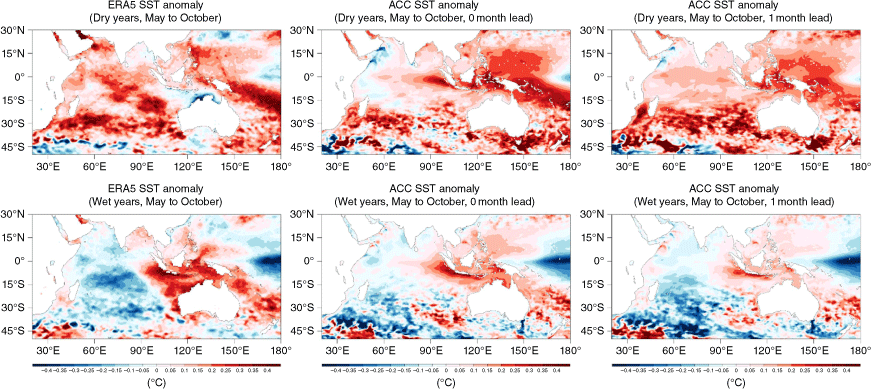
During wet years there are warm SST anomalies surrounding much of Australia, particularly the north and north-west, but there are cold SST anomalies over the central Indian Ocean and the signature over the tropics is for a negative IOD. ACCESS-S1 captured the general large-scale pattern of SST anomalies, but the warm anomalies north of Australia and the central Indian Ocean cold anomalies were much weaker than observed. The limited skill of ACCESS-S1 to capture the large-scale circulation and SSTs associated with wet and dry years in SWWA likely contributed to the errors identified in our analysis of GSP forecasts during the wheat growing season in SWWA.
These results highlight how errors in ACCESS-S1’s simulation of the Indian Ocean SSTs and wind fields potentially drive the shortcomings in SWWA rainfall prediction. Previous work also highlighted that model errors in simulating the relationship between Indian Ocean SST and Australian weather are potentially causing issues in forecasting for Australia. For example, Lim et al. (2016) demonstrated how the inability of ACCESS-S1 to accurately simulate the teleconnection between the tropical western Indian Ocean SST and Victorian rainfall limited the skill for rainfall forecasts in the region. Similarly, Marshall et al. (2021b) showed that, although ACCESS-S1 was able to capture the relationship between the negative IOD phase and extreme fire weather over Australia quite well, there were clear inaccuracies in the depiction of this relationship during the positive phase.
4.Conclusion
In this study we have investigated the ability of the Bureau of Meteorology’s dynamical seasonal forecasting model, ACCESS-S1, to simulate and forecast rainfall for the wheat growing season (May–October) in SWWA. The timing and frequency of light to heavy rainfall events was compared between AWAP observations and ACCESS-S1 to understand how these contributed to mean biases in the model’s GSP forecasts. Additionally, ACCESS-S1’s ability to accurately capture the large-scale drivers of SWWA rainfall over the Indian Ocean was also explored using the ERA5 reanalysis. We acknowledge that the short hindcast period (23 years) for ACCESS-S1 means that the sample sizes for the analysis were relatively small (6 dry years, 6 wet years and 11 average years). The recently released ACCESS-S2 system has a larger hindcast period (38 years). Our future work will focus on ACCESS-S2, as well as other forecast models, for example from the S2S database (Vitart et al. 2017). In addition, slight differences may be obtained from our results if a different observation dataset or reanalysis is used for the verification. However, the main conclusions are unlikely to change.
We showed that ACCESS-S1 had an overall dry bias for SWWA particularly for the most south-western corner. However, regardless of whether a given season was notably wetter or drier than average, ACCESS-S1 ensemble mean and median tended to forecast close-to-average rainfall. Specifically, dry years were generally forecast as dry but not dry enough, whereas wet years were forecast as wet, but not wet enough. We note that it is promising that in several instances, the ACCESS-S1 ensemble spread captured the observed anomalies even for this extended 6-month forecast period (which is quite a long period for a seasonal rainfall forecast). Our results also showed that a short lead time of 0 months was not always consistently more skilful than longer lead time forecasts of 1 month. In some instances, 0-month lead time showed slighter better results such as for the spatial analysis of the mean bias for wet and dry years (Fig. 4 and 5). When considering the ensemble spread and forecast skill (Section 3.2), some results showed improvement for 0-month lead time and for others 1-month lead time was more skilful. Similarly, when considering wind anomalies (Fig. 12) and SST anomalies (Fig. 13), the differences between the two lead-times were minimal. We also note that King et al. (2020) showed in their analysis that extended lead-times had a more noticeable effect on reducing the performance of the model during the warmer months in comparison to the cooler months, and our analysis focused largely on the cooler seasons. Overall, for the extended forecast periods considered in our study, the difference between 0- and 1-month lead times was less obvious compared to studies that focused on shorter forecast periods.
We identified that the SWWA rainfall biases were likely driven by inaccuracies in the timing of heavy rainfall events as well as Indian Ocean SST and wind anomaly biases. Seasonal forecasts of SWWA rainfall are generally challenging due to the weak teleconnections between the large-scale climate drivers such as ENSO and rainfall when compared to eastern Australia. For example, King et al. (2020) noted that ACCESS-S1 generally performs better when the relationship between the large-scale climate drivers and Australian mean and extreme rainfall is strongest. However, there is an established association between an Indian Ocean dipole in SSTs and SWWA rainfall, as identified here and in previous work (e.g. England et al. 2006; Ummenhofer et al. 2008). Ummenhofer et al. (2008) provided evidence that these Indian Ocean SSTs are key in forcing mid-latitude rainfall changes over SWWA. In addition, there is a weak association between the IOD and SWWA rainfall (e.g. Fig. 13 and England et al. 2006). ACCESS-S1 did not capture these SST anomalies and associated circulation during wet and dry years, which led to the poor forecast skill. The Indian Ocean SSTs are a potential source of seasonal predictability for SWWA and improvements in the model could lead to improved forecasts.
Model biases in the tropical eastern Indian Ocean limit our seasonal prediction skill for Australia in general (Hudson et al. 2017a). These persistent biases are currently the focus of a key collaboration between the Bureau, UKMO and other organisations. Areas of model development currently being investigated, with a key focus on the Indian Ocean region, are improving the model’s convection scheme and the modelling of the ocean in the Indonesian Throughflow region (which affects SSTs north of Australia). These future developments have the potential to improve seasonal forecasts of rainfall for SWWA.
To conclude, our study suggests that the ensemble mean or median seasonal rainfall forecasts for the growing season in SWWA are not skilful sources of information for wheat growers of SWWA particularly when growing season conditions are going to be very wet or very dry. Although a probability forecast that would translate the information contained in the ensemble spread of the forecasts would provide more useful information, they should still be interpreted with caution.
Data availability
All datasets used as part of this study are available at the NCI (https://nci.org.au/). Scripts used in this study are available by request to the corresponding author.
Acknowledgements
This research and project was undertaken with the assistance of resources and services from the National Computational Infrastructure (NCI), which is supported by the Australian Government. We thank the Australian Research Council Centre of Excellence for Climate Extremes and Prof. Andy Pitman for providing access to computer and data storage resources at the NCI. All this assistance is gratefully acknowledged.
References
Alves O, Wang G, Zhong A, Smith N, Tseitkin F, Warren G, Schiller A, Godfrey S, Meyers G (2003) POAMA: Bureau of Meteorology operational coupled model seasonal forecast system. In ‘Science for Drought: Proceedings of National Drought Forum’, 15–16 April 2003, Brisbane, Qld, Australia. (Eds R Stone, I Partridge) pp. 22–32. (Queensland Department of Primary Industries) Available at https://www.ecmwf.int/sites/default/files/elibrary/2003/7694-poama-bureau-meteorology-coupled-model-seasonal-forecast-system.pdf
Best MJ, Pryor M, Clark DB, Rooney GG, Essery RLH, Ménard CB, Edwards JM, Hendry MA, Porson A, Gedney N, Mercado LM, Sitch S, Blyth E, Boucher O, Cox PM, Grimmond CSB, Harding RJ (2011) The Joint UK Land Environment Simulator (JULES), model description – Part 1: energy and water fluxes. Geoscientific Model Development 4(3), 677-699.
| Crossref | Google Scholar |
Blockley EW, Martin MJ, McLaren AJ, Ryan AG, Waters J, Lea DJ, Mirouze I, Peterson KA, Sellar A, Storkey D (2014) Recent development of the Met Office operational ocean forecasting system: an overview and assessment of the new Global FOAM forecasts. Geoscientific Model Development 7(6), 2613-2638.
| Crossref | Google Scholar |
Camp J, Wheeler MC, Hendon HH, Gregory PA, Marshall AG, Tory KJ, Watkins AB, MacLachlan C, Kuleshov Y (2018) Skilful multiweek tropical cyclone prediction in ACCESS-S1 and the role of the MJO. Quarterly Journal of the Royal Meteorological Society 144(714), 1337-1351.
| Crossref | Google Scholar |
Charles AN, Duell RE, Wang X, Watkins AB (2015) Seasonal forecasting for Australia using a dynamical model: improvements in forecast skill over the operational statistical model. Australian Meteorological and Oceanographic Journal 65(3), 356-375.
| Crossref | Google Scholar |
Cowan T, Stone R, Wheeler MC, Griffiths M (2020) Improving the seasonal prediction of northern Australian rainfall onset to help with grazing management decisions. Climate Services 19, 100182.
| Crossref | Google Scholar |
Cowan T, Wheeler MC, Sharmila S, Narsey S, de Burgh-Day C (2022) Forecasting northern Australian summer rainfall bursts using a seasonal prediction system. Weather and Forecasting 37(1), 23-44.
| Crossref | Google Scholar |
Dee DP, Uppala SM, Simmons AJ, Berrisford P, Poli P, Kobayashi S, Andrae U, Balmaseda MA, Balsamo G, Bauer P, Bechtold P, Beljaars ACM, van de Berg L, Bidlot J, Bormann N, Delsol C, Dragani R, Fuentes M, Geer AJ, Haimberger L, Healy SB, Hersbach H, Hólm EV, Isaksen L, Kållberg P, Köhler M, Matricardi M, McNally AP, Monge-Sanz BM, Morcrette J-J, Park B-K, Peubey C, de Rosnay P, Tavolato C, Thépaut J-N, Vitart F (2011) The ERA‐Interim reanalysis: Configuration and performance of the data assimilation system. Quarterly Journal of the Royal Meteorological Society 137(656), 553-597.
| Crossref | Google Scholar |
Department of Primary Industries and Regional Development (2018) Western Australian grains industry. Available at https://www.agric.wa.gov.au/grains-research-development/western-australian-grains-industry
England MH, Ummenhofer CC, Santoso A (2006) Interannual rainfall extremes over southwest Western Australia linked to Indian Ocean climate variability. Journal of Climate 19(10), 1948-1969.
| Crossref | Google Scholar |
Evans FH, Guthrie MM, Foster I (2020) Accuracy of six years of operational statistical seasonal forecasts of rainfall in Western Australia (2013 to 2018). Atmospheric Research 233, 104697.
| Crossref | Google Scholar |
Geographic Information Services (2016) Potentially arable areas in the Western Australian wheatbelt. (Department of Primary Industries and Regional Development, Western Australia: Perth, WA, Australia) Available at https://library.dpird.wa.gov.au/gis_maps/20/
Giunta F, Motzo R, Deidda M (1993) Effect of drought on yield and yield components of durum wheat and triticale in a Mediterranean environment. Field Crops Research 33(4), 399-409.
| Crossref | Google Scholar |
Gregory PA, Camp J, Bigelow K, Brown A (2019) Sub‐seasonal predictability of the 2017–2018 Southern Hemisphere tropical cyclone season. Atmospheric Science Letters 20(4), e886.
| Crossref | Google Scholar |
Hersbach H, Bell B, Berrisford P, Hirahara S, Horányi A, Muñoz‐Sabater J, Nicolas J, Peubey C, Radu R, Schepers D, Simmons A, Soci C, Abdalla S, Abellan X, Balsamo G, Bechtold P, Biavati G, Bidlot J, Bonavita M, Chiara G, Dahlgren P, Dee D, Diamantakis M, Dragani R, Flemming J, Forbes R, Fuentes M, Geer A, Haimberger L, Healy S, Hogan RJ, Hólm E, Janisková M, Keeley S, Laloyaux P, Lopez P, Lupu C, Radnoti G, Rosnay P, Rozum I, Vamborg F, Villaume S, Thépaut JN (2020) The ERA5 global reanalysis. Quarterly Journal of the Royal Meteorological Society 146(730), 1999-2049.
| Crossref | Google Scholar |
Hope P, Keay K, Pook M, Catto J, Simmonds I, Mills G, McIntosh P, Risbey J, Berry G (2014) A comparison of automated methods of front recognition for climate studies: a case study in southwest Western Australia. Monthly Weather Review 142(1), 343-363.
| Crossref | Google Scholar |
Hudson D, Alves O, Hendon HH, Lim E-P, Liu G, Luo J-J, MacLachlan C, Marshall AG, Shi L, Wang G, Wedd R, Young G, Zhao M, Zhou X (2017a) ACCESS-S1: the new Bureau of Meteorology multi-week to seasonal prediction system. Journal of Southern Hemisphere Earth Systems Science 67(3), 132-159.
| Crossref | Google Scholar |
Hudson D, Shi L, Alves O, Hendon H, Young G (2017b) Performance of ACCESS-S1 for key horticultural regions. Bureau Research Report Number BRR020. (Bureau of Meteorology: Melbourne, Vic., Australia) Available at http://www.bom.gov.au/research/publications/researchreports/BRR-020.pdf [Verified 18 May 2023]
Jones DA, Wang W, Fawcett R (2009) High-quality spatial climate data-sets for Australia. Australian Meteorological and Oceanographic Journal 58(4), 233-248.
| Crossref | Google Scholar |
Kala J, Andrys J, Lyons TJ, Foster IJ, Evans BJ (2015) Sensitivity of WRF to driving data and physics options on a seasonal time-scale for the southwest of Western Australia. Climate Dynamics 44(3–4), 633-659.
| Crossref | Google Scholar |
King AD, Hudson D, Lim E-P, Marshall AG, Hendon HH, Lane TP, Alves O (2020) Sub-seasonal to seasonal prediction of rainfall extremes in Australia. Quarterly Journal of the Royal Meteorological Society 146(730), 2228-2249.
| Crossref | Google Scholar |
Lim E, Hendon H, Hudson D, Zhao M, Shi L, Alves O, Young G (2016) Evaluation of the ACCESS-S1 hindcasts for prediction of Victorian seasonal rainfall. Bureau Research Report 19. (Bureau of Meteorology Australia) Available at http://www.bom.gov.au/research/publications/researchreports/BRR-019.pdf
MacLachlan C, Arribas A, Peterson KA, Maidens A, Fereday D, Scaife AA, Gordon M, Vellinga M, Williams A, Comer RE, Camp J, Xavier P, Madec G (2015) Global seasonal forecast system version 5 (GloSea5): a high-resolution seasonal forecast system. Quarterly Journal of the Royal Meteorological Society 141(689), 1072-1084.
| Crossref | Google Scholar |
Madec G, The NEMO team (2016) NEMO ocean engine: Note du pole de modélisation de l’Institut Pierre-Simon Laplace nombre 27. (IPSL: Guyancourt, France) Available at https://www.nemo-ocean.eu/doc/
Marshall AG, Hendon HH (2018) Multi-week prediction of the Madden–Julian Oscillation with ACCESS-S1. Climate Dynamics 52(5–6), 2513-2528.
| Crossref | Google Scholar |
Marshall AG, Hendon HH, Hudson D (2021a) Influence of the Madden–Julian Oscillation on multiweek prediction of Australian rainfall extremes using the ACCESS-S1 prediction system. Journal of Southern Hemisphere Earth Systems Science 71(2), 159-180.
| Crossref | Google Scholar |
Marshall AG, Gregory PA, de Burgh-Day CO, Griffiths M (2021b) Subseasonal drivers of extreme fire weather in Australia and its prediction in ACCESS-S1 during spring and summer. Climate Dynamics 58(1), 523-553.
| Crossref | Google Scholar |
Megann A, Storkey D, Aksenov Y, Alderson S, Calvert D, Graham T, Hyder P, Siddorn J, Sinha B (2014) GO 5.0: the joint NERC–Met Office NEMO global ocean model for use in coupled and forced applications. Geoscientific Model Development 7(3), 1069-1092.
| Crossref | Google Scholar |
Meza FJ, Hansen JW, Osgood D (2008) Economic value of seasonal climate forecasts for agriculture: review of ex-ante assessments and recommendations for future research. Journal of Applied Meteorology and Climatology 47(5), 1269-1286.
| Crossref | Google Scholar |
Pook MJ, Risbey JS, McIntosh PC (2012) The synoptic climatology of cool-season rainfall in the central wheatbelt of Western Australia. Monthly Weather Review 140(1), 28-43.
| Crossref | Google Scholar |
Saji NH, Goswami BN, Vinayachandran PN, Yamagata T (1999) A dipole mode in the tropical Indian Ocean. Nature 401(6751), 360-363.
| Crossref | Google Scholar |
Shao Y, Wang QJ, Schepen A, Ryu D (2022) Introducing long‐term trends into sub‐seasonal temperature forecasts through trend‐aware post‐processing. International Journal of Climatology 42(9), 4972-4988.
| Crossref | Google Scholar |
Smith IN, McIntosh P, Ansell TJ, Reason CJC, McInnes K (2000) Southwest Western Australian winter rainfall and its association with Indian Ocean climate variability. International Journal of Climatology 20(15), 1913-1930.
| Crossref | Google Scholar |
Stephens DJ, Lyons TJ (1998) Rainfall–yield relationships across the Australian wheatbelt. Australian Journal of Agricultural Research 49(2), 211-224.
| Crossref | Google Scholar |
Stone RC, Hammer GL, Marcussen T (1996) Prediction of global rainfall probabilities using phases of the Southern Oscillation Index. Nature 384(6606), 252-255.
| Crossref | Google Scholar |
Ummenhofer CC, Sen Gupta A, Pook MJ, England MH (2008) Anomalous rainfall over southwest Western Australia forced by Indian Ocean sea surface temperatures. Journal of Climate 21(19), 5113-5134.
| Crossref | Google Scholar |
Vitart F, Ardilouze C, Bonet A, Brookshaw A, Chen M, Codorean C, Déqué M, Ferranti L, Fucile E, Fuentes M, Hendon H, Hodgson J, Kang HS, Kumar A, Lin H, Liu G, Liu X, Malguzzi P, Mallas I, Manoussakis M, Mastrangelo D, MacLachlan C, McLean P, Minami A, Mladek R, Nakazawa T, Najm S, Nie Y, Rixen M, Robertson AW, Ruti P, Sun C, Takaya Y, Tolstykh M, Venuti F, Waliser D, Woolnough S, Wu T, Won DJ, Xiao H, Zaripov R, Zhang L (2017) The Subseasonal to Seasonal (S2S) Prediction Project Database. Bulletin of the American Meteorological Society 98(1), 163-173.
| Crossref | Google Scholar |
Walters D, Boutle I, Brooks M, Melvin T, Stratton R, Vosper S, Wells H, Williams K, Wood N, Allen T, Bushell A, Copsey D, Earnshaw P, Edwards J, Gross M, Hardiman S, Harris C, Heming J, Klingaman N, Levine R, Manners J, Martin G, Milton S, Mittermaier M, Morcrette C, Riddick T, Roberts M, Sanchez C, Selwood P, Stirling A, Smith C, Suri D, Tennant W, Vidale PL, Wilkinson J, Willett M, Woolnough S, Xavier P (2017) The Met Office unified model global atmosphere 6.0/6.1 and JULES global land 6.0/6.1 configurations. Geoscientific Model Development 10(4), 1487-1520.
| Crossref | Google Scholar |
Wedd R, Alves O, de Burgh-Day C, Down C, Griffiths M, Hendon HH, Hudson D, Li S, Lim E-P, Marshall AG, Shi L, Smith P, Smith G, Spillman CM, Wang G, Wheeler MC, Yan H, Yin Y, Young G, Zhao M, Xiao Y, Zhou X (2022) ACCESS-S2: the upgraded Bureau of Meteorology multi-week to seasonal prediction system. Journal of Southern Hemisphere Earth Systems Science 72(3), 218-242.
| Crossref | Google Scholar |
Williams KD, Harris CM, Bodas-Salcedo A, Camp J, Comer RE, Copsey D, Fereday D, Graham T, Hill R, Hinton T, Hyder P, Ineson S, Masato G, Milton SF, Roberts MJ, Rowell DP, Sanchez C, Shelly A, Sinha B, Walters DN, West A, Woollings T, Xavier PK (2015) The Met Office Global Coupled Model 2.0 (GC2) configuration. Geoscientific Model Development 8(5), 1509-1524.
| Crossref | Google Scholar |
Wittwer G, Adams PD, Horridge M, Madden JR (2002) Drought, regions and the Australian economy between 2001-02 and 2004-05. Australian Bulletin of Labour 28(4), 231-246.
| Google Scholar |
Wright PB (1974) Seasonal rainfall in southwestern Australia and the general circulation. Monthly Weather Review 102(3), 219-232.
| Crossref | Google Scholar |
Zhao T, Wang QJ, Schepen A (2019a) A Bayesian modelling approach to forecasting short-term reference crop evapotranspiration from GCM outputs. Agricultural and Forest Meteorology 269-270, 88-101.
| Crossref | Google Scholar |
Zhao T, Wang QJ, Schepen A, Griffiths M (2019b) Ensemble forecasting of monthly and seasonal reference crop evapotranspiration based on global climate model outputs. Agricultural and Forest Meteorology 264, 114-124.
| Crossref | Google Scholar |
Zhao P, Wang QJ, Wu W, Yang Q (2021) Which precipitation forecasts to use? Deterministic versus coarser‐resolution ensemble NWP models. Quarterly Journal of the Royal Meteorological Society 147(735), 900-913.
| Crossref | Google Scholar |