Towards a wildfire vulnerability index using expert judgement
M. Papathoma-Köhle

A
B
C
Abstract
Climate change is expected to significantly change wildfire frequency and magnitude, which poses particular challenges for countries with limited experience in managing wildfires, such as Austria.
To develop a vulnerability index, this study aims at weighting the characteristics of buildings and their surroundings (vulnerability indicators) by involving different national stakeholders (Austria) and international experts.
Expert judgement and the analytic hierarchy process (AHP) were used for weighting indicators identified in a previous study through a literature review.
The judgement of two expert groups regarding the weighting of the vulnerability indicators is compared. A wildfire vulnerability index that combines all the indicators into a single vulnerability index assigned to each building is introduced.
The wildfire vulnerability index for buildings is a tool for decision-makers and other end users in Austria. The index sets the foundations for ongoing research in the field of vulnerability assessment and is based on trans-disciplinary approaches involving both academia and stakeholders.
The index can be used to support decision-making, risk reduction and climate change adaptation strategies but it can also guide local adaptation at the building level.
Keywords: analytic hierarchy process, Austria, buildings, expert judgement, index, indicators, stakeholders, vulnerability, wildland–urban interface, wildfire.
Introduction
Climatic conditions favourable to wildfires are increasing worldwide, including in Europe, but trends in the number of fires and the area burnt vary regionally (Jones et al. 2022). Additionally, according to Cunningham et al. (2024), the frequency of energetically extreme events associated with extreme environmental, social and economic impacts has increased by a factor of 2.2 over the past 20 years. The global costs of wildfires have risen substantially (Ritchie et al. 2022). Moreover, countries with limited experience in managing wildfires in northern and central Europe are expected to experience more wildfires in the future (Cardil et al. 2021). Specifically in Austria, which is the focus of the present study, the effects of climate change are expected to lead to an increase in heat waves and a decrease in precipitation (Olefs et al. 2021) and, consequently, an increase in fire weather days, which will ultimately increase wildfire risk in the country (Müller et al. 2020).
Increased wildfire risk is influenced not only by climate change but also by socio-economic dynamics. Austrian forests, for example, serve as recreational areas and as a source of timber as well as protection against other natural hazards such as snow avalanches and landslides. Until now, wildland fires in Austria have had only a minor impact on human lives, settlements and infrastructure within the wildland-urban interface (WUI). However, focusing on the WUI is important as 85% of forest fires in Austria have been caused directly or indirectly by human activity, and the remaining 15% by lightning (Müller et al. 2020). The interaction between forests and settlements is twofold, as on the one hand, human activities increase the likelihood of wildfires, but on the other hand, wildfires threaten the population and infrastructure in the WUI. It is, therefore, pivotal to focus on the vulnerabilities within the WUI that affect overall wildfire risk to our society.
Studies focusing on the vulnerability of the built environment do exist, but they are mostly limited to the interaction between buildings and fire, rather than providing a tool to measure this vulnerability for risk reduction purposes. However, some of them focus on assessing the vulnerability of buildings within the WUI in Europe. These studies include the study by Samora-Arvela et al. (2023), who investigated the factors that contribute to the resistance of buildings to the impact of wildfire to support municipal mitigation strategies in Portugal. Moreover, the relationship between building characteristics and the resulting wildfire damage to buildings was investigated by Dossi et al. (2023) using data from California and Portugal. In terms of expert judgement, Àgueda et al. (2023) developed a tool for homeowners based on fuzzy logic (fuzzy preferences of WUI experts) to help them assess and reduce the physical vulnerability of buildings to wildfire, which was applied in two case studies in Spain. One of the first attempts to develop a wildfire vulnerability index was the study by Papathoma-Köhle et al. (2022b), based on building characteristics and damage data from the wildfire in Mati, Greece (July 2018).
Nevertheless, all these studies use damage data from countries with long experience of wildfire and a large amount of empirical data. The lack of empirical data in countries with a poor record of wildfire events is challenging for vulnerability and risk assessment. A promising approach to overcome this gap is to compensate for the lack of data with expert judgement or other participatory methods.
In this paper, we focus on such an approach. By examining buildings located within the WUI and considering their characteristics along with their surroundings, we have developed a vulnerability index for these structures. This index can guide disaster risk reduction strategies, building reinforcement efforts and preparedness policies for future wildfire events. The outcomes of our approach are essential for reducing the vulnerability and increasing the resilience of the built environment. Herein, ‘vulnerability’ is considered to be the conditions determined by physical (in this case) factors that increase the susceptibility of an asset to the impacts of hazards, and ‘resilience’ is considered to be the capacity to resist, absorb, accommodate, adapt to, transform and recover from these impacts (UNISDR 2009).
Wildfire risk in Austria and research needs
In Austria, wildfires are not as frequent as in other European countries (e.g. Portugal, Greece); nevertheless, when they do occur, they can have costly consequences. For example, a wildfire in the Austrian Federal State of Tyrol in 2014 resulted in costs of ~€3 million (Müller et al. 2020). In 2014 and 2015, Austria experienced two large wildfires, each burning approximately 100 ha. In 2021, a wildfire in the Austrian Federal State of Lower Austria (Hirschwang an der Rax) was recorded as one of the largest wildfires ever in Austria, burning more than 115 ha. Nevertheless, in the following year (2022) the burnt area in Austria increased dramatically mainly owing to a single event (Allensteig, Lower Austria) that was responsible for burning more than 800 ha (including 550 ha of forest) (Müller 2023). To monitor wildfires in Austria, an online database (Vacik and Müller 2013) with more than 7000 data records of current and historical forest fires has been established. Based on this database, wildfire events in Austria since 2000 are shown in Fig. 1. Most wildfires (85%) were caused by humans, with tourism and recreational activities being the most important factors for their initiation (Müller et al. 2020).
Annual number of wildfires (bars) and burnt area (dots) in Austria since 2000 (data source: https://fire.boku.ac.at).
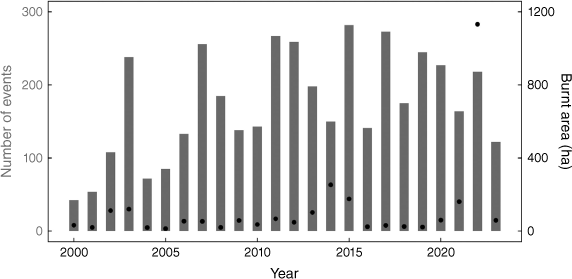
In recent years, significant effects of climate change have become manifest in the greater Alpine region, leading to severe precipitation deficits in Austria (Haslinger and Mayer 2023). Long intense dry periods in 2011 and 2012 led to severe spring fires, whereas summer fires increased in 2013 (Fig. 1). In 2015, an extremely dry late autumn resulted in wildfires that lasted until the end of December in the high mountains. Rising temperatures and the resulting dry conditions caused by human-induced climate change have repeatedly been blamed for these anomalies (Vacik et al. 2020). The changes observed in Austria have also been recorded at the European level by EFFIS (European Forest Fire Information System). Based on these data and according to the European Commission: Joint Research Centre et al. (2022), there is an increasing trend towards a higher number and larger area of forest fires in the European Union (EU). As wildfires occur in Austria and other regions with limited historical records of such events (e.g. Scandinavia, Siberia), central and northern European countries are seeking ways to reduce wildfire risk. In Austria, although efforts have been made to assess wildfire risk, most of them do not include a vulnerability study. Current efforts are focusing on hazard assessment (Müller et al. 2020), which is an essential component for the assessment of associated risks, but it needs to be complemented by vulnerability studies.
Method
As empirical data on wildfire losses are missing, the methodological approach used herein relied on expert judgment to determine the physical vulnerability of buildings located in the WUI. The workflow included the following steps: (a) a thorough literature review on vulnerability indicators of buildings exposed to wildfire; (b) stakeholder mapping to identify Austrian and international experts able to assess the importance of these indicators; (c) the identification of relevant vulnerability indicators and the design of a questionnaire to assess these indicators; (d) the implementation of the analytic hierarchy process (AHP) to weight the indicators; and (e) the development of a wildfire vulnerability index. The workflow of the study is shown in Fig. 2.
Literature review
In the literature review that was completed during a previous study (Papathoma-Köhle et al. 2022b), 35 peer-reviewed scientific articles published over approximately the last 30 years (1991–2020) were examined. Their focus was buildings and their interaction with wildfire in the WUI in case studies in Europe, the USA and Australia (e.g. Xanthopoulos 2004; Australian Standards 2009; Lampin-Maillet et al. 2010; Maranghides et al. 2013; Quarles et al. 2013; Laranjeira and Cruz 2014; Alexandre et al. 2016a; Syphard and Keeley 2019; Vacca et al. 2020). Although vulnerability was not always explicitly addressed in the papers, information was provided on the characteristics of buildings and their surroundings and how these contribute to overall wildfire vulnerability, allowing a list of potential indicators to be compiled (Papathoma-Köhle et al. 2022b).
Stakeholder mapping
Two main pools of experts were invited as the basis for expert judgement: Austrian stakeholders and international experts. These two groups have different strengths and advantages. On the one hand, the Austrian stakeholders are familiar with the Austrian built environment, but, on the other hand, the international experts bring experience and scientific knowledge from many places in the world. Fourteen Austrian stakeholders were identified as experts on the basis of their involvement in research, decision making or response to wildfires. Their professional backgrounds included research related to forest fires, engineering, natural hazard insurance, emergency services (firefighters) and public administration related to natural hazards in Austria (Table 1). Nine out of the fourteen Austrian stakeholders responded and sent a completed questionnaire, resulting in a response rate of approximately 64%.
Professional background | Invited (14) | Responded (9) | |
---|---|---|---|
University and research | 4 | 2 | |
Authorities | 3 | 1 | |
Fire brigade | 6 | 5 | |
Insurance | 1 | 1 |
More than 50 (54) international experts were invited to provide expert judgement on the project. The main criterion for their selection was their involvement in research related to wildfire impacts in the WUI, as evidenced by their scientific publications over the last 20 years. Consequently, they all had a similar professional background (university, research institute), but with a wide geographical distribution (Table 2), in order to benefit from their experience and knowledge from countries with experience in wildfire management. Of the 54 international experts invited to participate in the survey, 19 completed the questionnaire (35% response rate).
Design of questionnaire
A questionnaire was designed (see Supplementary Appendix S1) on the basis of the literature review and subsequently sent to the two expert groups described in the previous section. This questionnaire was used to weight the different indicators against each other in order to obtain a ranking of the important variables that determine the vulnerability of buildings to wildfire. The set of indicators was divided into the following three categories:
Building environment: characteristics of nearby forest including information on the settlement the building is located in and nearby forest (e.g. vegetation density and type).
Building exterior: characteristics of the immediate surroundings of the building including ground cover and vegetation.
Building characteristics: characteristics of the building including materials, design, shape, etc.
All the indicators considered in the study can be seen in Table 3. It is clear to us that some indicators, especially those in the ‘building environment’ category, are related to the hazard and less to the element at risk. Nevertheless, as physical vulnerability is hazard-specific, characteristics of the hazard that are related to its impact on buildings are also included in the assessment.
Category | Indicator | Abbreviation | Weight (AT) | Weight (INT) | Delta | |
---|---|---|---|---|---|---|
Building environment | Protective strips/firebreaks | Firebreaks | 0.2858 | 0.1979 | 0.088 | |
Building environment | Vegetation density | Vegetype | 0.1705 | 0.1861 | −0.0156 | |
Building environment | Distance to neighbouring buildings | Neighbours | 0.1632 | 0.1335 | 0.0297 | |
Building environment | Forest type | Forest | 0.1543 | 0.1696 | −0.0153 | |
Building environment | Water sources | Water | 0.1269 | 0.0912 | 0.0357 | |
Building environment | Building density | Density | 0.0993 | 0.1099 | −0.0106 | |
Building exterior | Distance of tree crowns/vegetation | Vegdist | 0.2579 | 0.2118 | 0.0461 | |
Building exterior | Distance to forest edge | Forestdist | 0.2443 | 0.1629 | 0.0815 | |
Building exterior | Type of vegetation | Vegproperty | 0.1349 | 0.1411 | −0.0062 | |
Building exterior | Combustible materials and objects | Combustibles | 0.1252 | 0.1241 | 0.0011 | |
Building exterior | Terrain slope | Slope | 0.094 | 0.1076 | −0.0137 | |
Building exterior | Ground covering | Cover | 0.0761 | 0.0811 | −0.005 | |
Building exterior | Property boundary | Boundary | 0.0676 | 0.0752 | −0.0076 | |
Building characteristics | Roof material | Roofmat | 0.2032 | 0.1636 | 0.0396 | |
Building characteristics | Façade | Facade | 0.1929 | 0.1229 | 0.0699 | |
Building characteristics | Building material | Structure | 0.1336 | 0.1319 | 0.0018 | |
Building characteristics | Roof overhang | Roofoverhang | 0.1217 | 0.1077 | 0.0141 | |
Building characteristics | Door/window material | Doorwin | 0.1003 | 0.1233 | −0.0229 | |
Building characteristics | Shutters | Shutters | 0.0939 | 0.1196 | −0.0257 | |
Building characteristics | Roof shape | Roofcomplex | 0.0833 | 0.1086 | −0.0253 | |
Building characteristics | Number of floors | Stories | 0.0711 | 0.038 | 0.0331 |
Application of analytic hierarchy process (AHP)
The AHP was used to analyse the results of the questionnaires sent out to the stakeholders. The AHP is part of a prescriptive decision theory and is used to solve multi-criteria decision problems (Saaty 1987). AHP can break down complex decisions into hierarchical structures providing a quantitative basis to support decision-making and, for this reason, is widely used for evaluating expert judgement in various scientific fields. The procedure of the AHP follows the publications of Saaty (1987), Riedl (2005) and Ronninger (2019). The steps of the AHPs are described in detail below:
Questionnaire and pairwise comparisons: based on the literature review, a questionnaire was designed. The questionnaire was used to perform indicator weighting based on expert assessment by leveraging the AHP methodology in order to account for the different effects on the vulnerability of a building to wildfires. Starting with the list of indicators identified in three categories of ‘building environment’ (six indicators), ‘building exterior’ (seven indicators) and ‘building characteristics’ (eight indicators) as described above, a survey (questionnaire) featuring a total of pairwise comparisons was designed. The number of indicators per group was chosen in accordance with Saaty and Ozdemir (2003) and Ozdemir (2005), i.e. limiting the number of elements in a group to seven plus or minus two. The AHP method is based on quantifying the weights of decision criteria by leveraging an exhaustive set of pairwise comparisons, where the relative importance between indicators is estimated by gathering information on the direction and magnitude of relative preference between each pair. Thus, experts estimate the relative importance of indicators on the physical vulnerability of a building for each pair of indicators across all three categories. Instead of the fundamental 1–9 Saaty scale, we opted to use a reduced four-point ratio scale coded as: 1, equal importance; 2, slight importance; 3, moderate importance; and 4, strong importance. If two indicators are considered to exert identical influence on wildfire vulnerability, the middle checkbox stating ‘equal importance’ can be selected in the questionnaire. However, if the subjective assessment is associated with a tendency towards one of the two opposite indicators, there is a three-level classification (slight, moderate, strong) for each of the two indicators in which the influence can be selected. The questionnaire was designed as a fillable PDF form structured into the three categories described above to make it easily accessible. PDF form data of the responses were dumped to csv-files using pdftk (Steward 2004) for further processing in R (R Core Team 2024). The three categories were not separately weighted.
Derivation of weights: aggregated preference weights were computed by analysing the pairwise comparison matrices of all responses k = {1, …, m} and for each of the three categories c = {1, 2, 3}. m denotes the total number of responses (9 and 19 for the national and international group, respectively). Each of these pairwise comparison matrices is a positive reciprocal matrix of order n that is defined as:
where ai,j > 0 and . ai,j represents the pairwise comparison between the attributes i and j by means of their weights, i.e. . Thus, ai,j takes the integer value of the ratio scale if i is more important than j, and its reciprocal if j is more important than i. n is the number of indicators per category, and thus takes the values 6, 7 and 8 in the present case.
First, the individual priorities of each expert are derived for each attribute by computing the geometric average of the normalised preference weights per row. Subsequently, these individual priority weights are aggregated to total weights for each attribute, using the geometric mean as an aggregation function. The geometric mean is used as an aggregation function as it satisfies the reciprocal property of pairwise comparisons, i.e. aj,i = 1/ai,j when aggregating individual judgements to group judgements (Aczél and Saaty 1983; Grošelj and Dolinar 2023). The R package ahpsurvey (Cho 2019) was used for conducting the AHP analysis.
Index development
Several studies use indicators to assess physical vulnerability (Barroca et al. 2006; Dall’Osso et al. 2009; Agliata et al. 2021; Papathoma-Köhle et al. 2022a). The PTVA (Papathoma Tsunami Vulnerability Assessment) method (Papathoma and Dominey-Howes 2003; Papathoma et al. 2003; Dominey-Howes and Papathoma 2007; Dall’Osso et al. 2009, 2016; Dominey-Howes et al. 2010; Papathoma-Köhle et al. 2019) originally designed for tsunami hazards is a representative indicator-based method. Based on PTVA, a similar approach was used for hydro-geomorphic hazards in France (Kappes et al. 2012), Peru (Thouret et al. 2014; Ettinger et al. 2016) and India (Thennavan et al. 2016). The most recent development is a Physical Vulnerability Index (PVI) for dynamic flooding in the European Alps (Papathoma-Köhle et al. 2019) and an index for wildfire vulnerability based on empirical data from a wildfire event in Greece in 2018 (Papathoma-Köhle et al. 2022b). The steps in the development of a vulnerability index are shown in Fig. 3. Following the selection of the relevant indicators, the indicators need to be weighted (in this case study using AHP) and then normalised and aggregated into a single index. The index can be calculated as:
In the above equation, wi expresses the weighting (Table 3) of the scores for each indicator Ii that receives a value of 1, 2, 3, 4 or 5 according to how the specific characteristic affects the vulnerability of the building. In the present study, we assumed that the three categories are equally important for the vulnerability of the building. The reason for assigning equal weights is mainly the fact that these three categories express three different aspects: (a) vulnerability related to whether and at which intensity the fire approaches the settlements where the building is located (surroundings); (b) vulnerability related to the fire reaching the building (exterior of the building); and finally, (c) the way the building reacts to the fire (building characteristics).
The assessment of each building can be done in a spreadsheet in the form of an assessment tool, as shown in Fig. 4. In this figure, the scoring for each indicator can be found, ranging from 1 to 5, depending on whether it reduces vulnerability or not. The scale of 1–5 was chosen to capture the variation of each indicator within the area under investigation. Some of the indicators have five scoring classes to choose from (e.g. building material), others have fewer (e.g. roof shape) and others can be scored either 1 or 5 (present or not present), e.g. water sources, buffer strips or firebreaks in the adjacent forest.
This scoring can be modified according to the specifics of each study area to reflect local conditions. Alternatively, the scoring can be based on the judgement of local experts who are familiar with local conditions. The weighting used for the index in this study is based on the national experts, as they have knowledge of the Austrian context. The weighting of the international experts is important because of their long experience with wildfire events, and it is used to validate the weighting of the local stakeholders.
The final step of the vulnerability assessment process is the classification of the vulnerability classes. There are many different methods, depending on the user and the purpose of the study (Papathoma-Köhle et al. 2019), including equal intervals, quantiles and natural breaks. It is clear that the choice of classification can guide different actions to reduce vulnerability.
Results and discussion
Despite their different backgrounds and experience with wildfires, the two teams of experts came to very similar conclusions (Fig. 5 and Table 3). For both teams, the presence of protective strips or fire breaks in the nearby forest, the distance of tree crowns to the building and the roof material were the most important features. However, in terms of building characteristics, the Austrian experts considered the roof overhang to be much more important than the international experts. This may be due to local characteristics. Specifically, in some regions of Austria, buildings have wooden roofs with large overhangs. In this case, it may be easier for the roof to burn. In buildings with concrete roofs, the overhang may not be as important. Furthermore, regarding the building environment, the international experts, in contrast to their Austrian counterparts, considered the type of forest more important than the distance to neighbouring buildings. Finally, regarding the building exterior, the distance to the neighbouring forest was the most important indicator for the international experts in contrast to the type of forest, which seemed to be the most important indicator in this category for the Austrian stakeholders.
Weighting of the indicators based on the international experts (a), and the Austrian stakeholders (b). Dots indicate the weights derived using the geometric mean as an aggregation function. Error bars indicate the standard deviation of the aggregated weights.
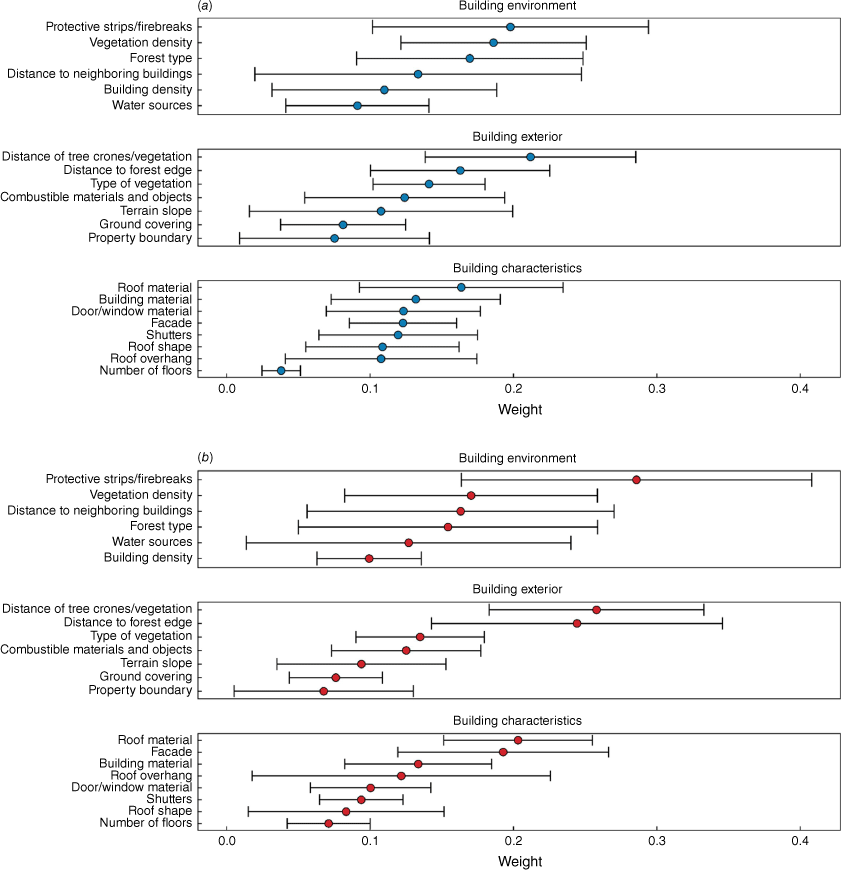
Investigating the aggregated individual judgements of the two groups of experts allows one to analyse and compare the average pairwise comparisons between the two groups (Fig. 6a and b). Specifically, investigating differences (Δ) in the weighting of the two groups (Fig. 6c) allows us to unearth patterns of agreement or disagreement between them. Whereas the category ‘building exterior’ shows comparable judgements between the two groups, major differences can be observed within the categories ‘building environment’ and ‘building characteristics’, where Δ is further away from zero. More specifically, Austrian stakeholders consider the presence of water resources to be slightly more important than vegetation density, whereas international experts have the opposite opinion, with a rather pronounced judgement favouring vegetation density over the presence of water resources. This can be explained by the lack of experience with wildfires and the sufficient availability of water in Austria (given the scale of wildfires so far), and the greater water scarcity in countries with regular large-scale wildfires. Furthermore, the presence of vegetation and fuel is directly correlated with the probability of fire occurrence and the potential for fire spread (e.g. Alexandre et al. 2016a, 2016b). Consequently, the experts from countries with a higher incidence of wildfires may have assigned greater importance to vegetation density as a risk factor. Moreover, the Austrian group considers the façade more important than the material of the windows and doors, whereas the international experts conclude the opposite. This can be explained by the predominant architecture in each country, with different types of cladding materials in Austria (including wooden shingles) and more homogeneous façade types in other countries. Nevertheless, as in different geographical regions some construction materials are more common than others (Syphard et al. 2017; Syphard and Keeley 2019), this indicator is highly case-sensitive. In contrast to the international experts, Austrian stakeholders rate forest type as less important than both the presence of water and vegetation density. This is surprising, given that most wildfires in Austria occur in pine forests, which are not a predominant forest type. Finally, the façade is also considered more important than the building material for the Austrians, whereas the international experts provide the opposite judgement. This can also be attributed to the locally predominant architecture, with brick, stone and concrete being more widespread in Austria.
Aggregated individual judgements of the international experts (a), the Austrian stakeholders (b), and a demonstration of the differences among the two groups (c). Positive values indicate preference of the indicator on the x-axis while negative values indicate preference of the indicator on the y-axis. Δ is derived as the difference between the international and the Austrian group. See Table 3 for attribute characteristics and definitions of terms.
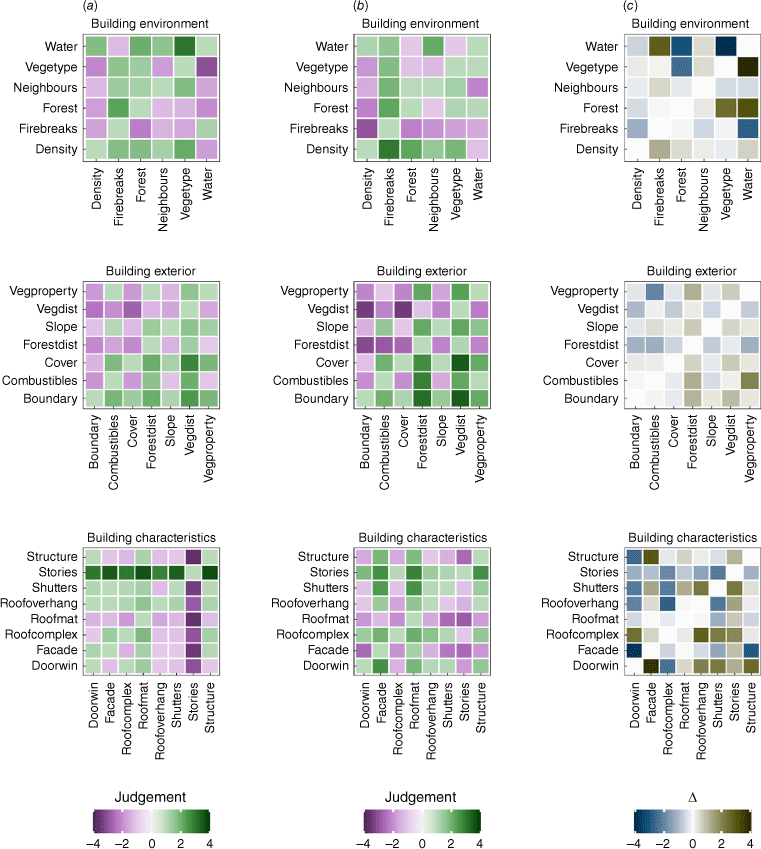
The consistency of the AHP results was assessed (Fig. 7). According to Saaty (1987), responses with a consistency ratio greater than 0.1 are rated inconsistent. Although most of the responses of both groups of experts were consistent, the inconsistency that appeared in the cluster ‘building environment’ is unexpected. This cluster showed the highest inconsistency (especially from the international experts) despite containing the lowest number of indicators. This inconsistency may reflect the perceived complexity of the cause-effect relationships in this context, which poses a challenge for a consistent ranking of indicators. The higher consistency rate of some responses in the building characteristics group is probably due to the large number of attributes in this class, which inherently increases the risk of inconsistent responses. For this reason, in the future, we can either reduce the number of indicators, inform the decision makers about inconsistencies and ask them to revise their pairwise comparison (Tavana et al. 2023), or try to remove inconsistencies computationally (Cao et al. 2008).
Distribution of the consistency ratio of all responses for both the international experts and the Austrian stakeholders. Responses with a consistency ratio larger than 0.1 are deemed to be inconsistent according to Saaty (1987).
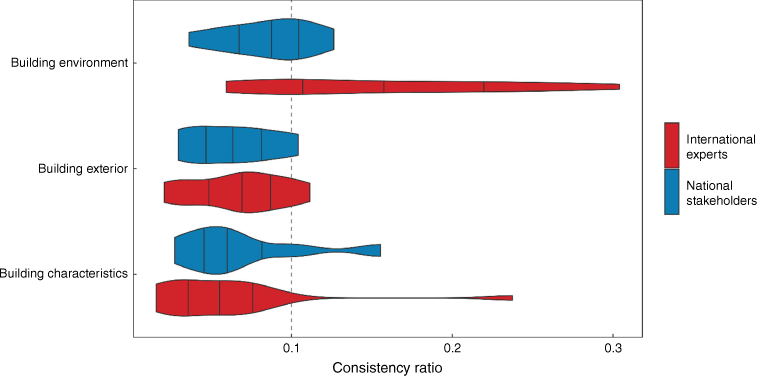
The questionnaires sent to the experts also included open questions, giving them the opportunity to either question an indicator or suggest a new one. Their responses questioned the relevance of some indicators (e.g. number of storeys, roof overhang, water sources and terrain slope, roof shape). However, some additional indicators were suggested including distance from roads, the defensive potential of the building (firefighting infrastructure), leeward land use, type and cleanliness of the gutters, vegetation connectivity, vegetation maintenance, and maintenance level and the overall condition of the building and surrounding vegetation. Furthermore, some experts suggested indicators related to neighbouring buildings, details of windows and shutters, concavities in the building envelope and the presence of a slab separating the roof from the rest of the building. Some experts also discussed the inclusion of information on preparedness (ability to defend the building, existence of preventive measures) but also on the response of the homeowners (e.g. presence of people in the building). Finally, one of the experts noted that the intensity of the wildfire on each building should also be taken into account as well as the prevailing winds (direction and speed). However, the majority of the responses were in line with the existing indicators and the additional ones suggested will need to be discussed with the local stakeholders in further development of the study to see how relevant they are to the Austrian context (through additional workshops and interviews). In more detail, a summary of the responses of the experts to the open questions can be found in Table 4.
International experts | ||
---|---|---|
Not relevant | Additional | |
Slope (1) | Distance to roads (1) | |
Roof overhang (1) (unless design is the focus) | Fire characteristics and fire suppression infrastructure (1) | |
Number of floors (2) | Absence/presence of people (1) | |
Roof complexity (1) | Potential for the building to be defended (1) | |
Water sources (1) | Upwind land use (1) | |
Preventive measures and self-protection systems (2) | ||
Data on recent wildfire events (1) | ||
Ember screens on the openable portion of the window (1) | ||
Shutter material (1) | ||
Type of window glass (1) | ||
Framing used in the roof space (1) | ||
Concavities or breakages in the building envelope (2) | ||
Presence of exterior combustible elements (2) | ||
Subfloor area used for the storage of combustible material (1) | ||
Wind speed and direction (1) | ||
Deck attached to the building (1) | ||
Fence near the building (1) | ||
Building (1) and vegetation maintenance (1) | ||
Fuel moisture content (1) | ||
Gutter type (material) and cleanliness from leaf debris (1) | ||
Existence of an inert plate between the roof and the interior of the building (1) | ||
Condition of vegetation and existence of combustible materials or abandoned cars in the neighbouring property (1) | ||
Connectivity of vegetation (1) |
Austrian stakeholders | ||
---|---|---|
Not relevant | Additional | |
Roof overhang (1) | Orientation of the slope where the building is located (1) | |
Altitude (geographical position) (1) | ||
Ground humidity (1) | ||
Population density in WUI (1) | ||
Temporal storage of material between the fire and the building (1) | ||
Infrastructure (gas tanks, auxiliary buildings) between the fire and the building (1) | ||
Response time and equipment of the fire brigade (1) | ||
Exposure of the building to the prevailing wind (1) |
The assessment tool, including all the indicators and the scoring (Fig. 4), was tested on 23 buildings along the Gladiolenweg in the village of Oberschütt (Carinthia, Austria), located in the WUI on the lower southern slope of the Dobratsch mountain. Classification of the vulnerability classes was based on division into equal intervals. It becomes obvious that not all the buildings in the settlement under investigation are equally vulnerable (Fig. 8), demonstrating the spatial variability of the index. Buildings closer to the edge of the forest are more vulnerable, but not always (there are buildings very close to the edge of the forest with moderate vulnerability). Local authorities or individual homeowners can use this information to recommend or implement local adaptation measures (changes to the building) or other activities (pruning of nearby trees) to increase the resilience of specific buildings. The community can also use this information to plan evacuation routes and prioritise actions. The spatial pattern of vulnerability could also be overlaid with information on the location of vulnerable groups.
Implementation of the vulnerability index in an Austrian WUI (village of Oberschütt, Carinthia, Austria - the exact location (yellow dot) in Austria and in Carinthia can be seen in the inset map).
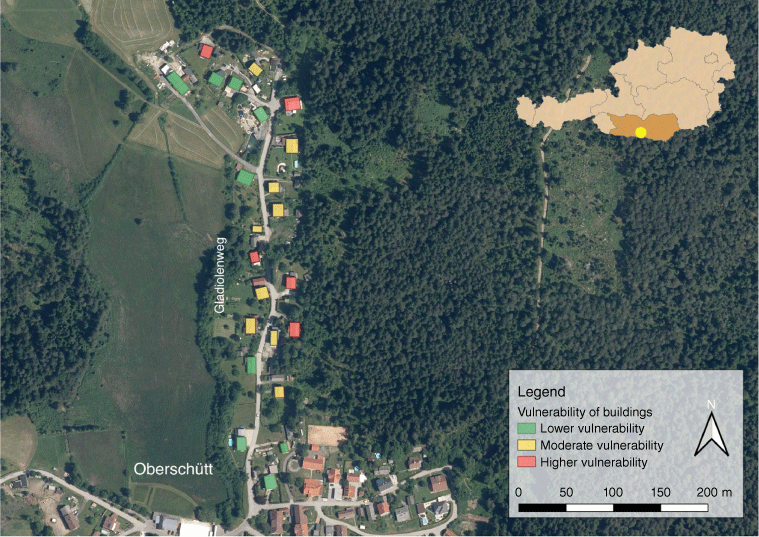
Ideally, the relevance and weighting of indicators can be validated using statistical methods (e.g. Boruta selection) after a future disaster (Papathoma-Köhle et al. 2022b). The relevance of the indicators in question can be checked and additional indicators can be included.
The weighting of the indicators presented here is based on the expert judgement of Austrian stakeholders. It is noteworthy that the weighting of the international experts was not much different. However, if we compare the results with the index developed for Greece using empirical damage data (Papathoma-Köhle et al. 2022b), we see that in some cases, the results are similar (roof material is the most important indicator for building characteristics in both studies) but in some cases, they are not. For example, although terrain slope is the third most important indicator in the Greek study, it is not particularly important in the present study. However, it should be noted that in the Greek case study, the indicators were not divided into categories. The comparison of the two studies, however, has highlighted the challenges in terms of the transferability of results between countries, given the differences in construction design. It is clear that it is challenging to develop a ‘universal model’ using expert judgement, but statistical analysis based on damage data may provide more reliable results on the relevance and weighting of indicators. More research is therefore needed on the transferability of such indices.
The Austrian stakeholders who participated in this study were national or regional stakeholders and as such, they understand the Austrian status quo. They may, however, lack local knowledge of particularities and special needs at the municipality level. It would be interesting in the future to see if local stakeholders come to similar conclusions.
Conclusion
The development of a wildfire vulnerability index based on expert judgement is presented with a special focus on the Austrian context. Vulnerability indicators were divided into three categories and then weighted by two different groups of experts using AHP. The results of the weighting showed many similarities between the groups, but also differences. This is the very first attempt to develop such a tool for a country with a poor record of wildfire events and little experience with the phenomenon. Nevertheless, the role of such a tool may be crucial in the near future, as wildfire events are expected to increase in the European Alps. The results are therefore of interest to other countries facing similar challenges. In this case, local decision-makers will need methods and tools that allow them to focus their resources more efficiently. Given the scarcity of similar studies in the specific geographical area, there is still room for future research, including improving the index to include information on the expected fire and local factors that contribute to its propagation (e.g. prevailing winds), but also local features of buildings, landscape and spatial planning.
Data availability
Data used to generate the results in the paper are available on request from the corresponding author (anonymously). The questionnaire can be found on GitLab at https://gitlab.com/Rexthor/wildfire-indicators-ahp and as supplementary material The code used for data preparation, data processing, statistical analysis and graphical visualisation of results is available on GitLab at https://gitlab.com/Rexthor/wildfire-indicators-ahp.
Declaration of funding
This study was funded by the Austrian Ministry of Agriculture, Forestry, Regions, and Water Management under the Austrian Waldfonds (BMLRT/III-2023-M6/1 (FAI.69) – Project ‘Wildfire: local vulnerability assessment for buildings in Austria’).
Acknowledgements
The authors would like to thank the Austrian and international experts that took part in this survey.
References
Aczél J, Saaty TL (1983) Procedures for synthesizing ratio judgements. Journal of Mathematical Psychology 27, 93-102.
| Crossref | Google Scholar |
Agliata R, Bortone A, Mollo L (2021) Indicator-based approach for the assessment of intrinsic physical vulnerability of the built environment to hydro-meteorological hazards: review of indicators and example of parameters selection for a sample area. International Journal of Disaster Risk Reduction 58, 102199.
| Crossref | Google Scholar |
Àgueda A, Vacca P, Planas E, Pastor E (2023) Evaluating wildfire vulnerability of Mediterranean dwellings using fuzzy logic applied to expert judgement. International Journal of Wildland Fire 32, 1011-1029.
| Crossref | Google Scholar |
Alexandre PM, Stewart SI, Keuler NS, Clayton MK, Mockrin MH, Bar-Massada A, Syphard AD, Radeloff VC (2016a) Factors related to building loss due to wildfires in the conterminous United States. Ecological Applications 26, 2323-2338.
| Crossref | Google Scholar | PubMed |
Alexandre PM, Stewart SI, Mockrin MH, Keuler NS, Syphard AD, Bar-Massada A, Clayton MK, Radeloff VC (2016b) The relative impacts of vegetation, topography and spatial arrangement on buildings to wildfires in case studies of California and Colorado. Landscape Ecology 31, 415-430.
| Crossref | Google Scholar |
Australian Standards (2009) Construction of buildings in bushfire-prone areas, AS 3959–2009, Standards Australia, Sydney, Australia
Barroca B, Bernardara P, Mouchel J-M, Hubert G (2006) Indicators for identification of urban flooding vulnerability. Natural Hazards and Earth Systems Sciences 6, 553-561.
| Crossref | Google Scholar |
Cao D, Leung LC, Law JS (2008) Modifying inconsistent comparison matrix in analytic hierarchy process: a heuristic approach. Decision Support Systems 44, 944-953.
| Crossref | Google Scholar |
Cardil A, Monedero S, Schag G, de-Miguel S, Tapia M, Stoof CR, Silva CA, Mohan M, Cardil A, Ramirez J (2021) Fire behavior modeling for operational decision-making. Current Opinion in Environmental Science & Health 23, 100291.
| Crossref | Google Scholar |
Cho F (2019) ahpsurvey: Analytic Hierarchy Process for survey data. R package version 0.4.1. Available at https://CRAN.R-project.org/package=ahpsurvey
Cunningham CX, Williamson GJ, Bowman DMJS (2024) Increasing frequency and intensity of the most extreme wildfires on Earth. Nature Ecology & Evolution 8, 1420-1425.
| Crossref | Google Scholar | PubMed |
Dall’Osso F, Gonella M, Gabbianelli G, Withycombe G, Dominey-Howes D (2009) A revised (PTVA) model for assessing the vulnerability of buildings to tsunami. Natural Hazards and Earth System Sciences 9, 1557-1565.
| Crossref | Google Scholar |
Dall’Osso F, Dominey-Howes D, Tarbotton C, Summerhayes S, Withycombe G (2016) Revision and improvement of the PTVA-3 model for assessing tsunami building vulnerability using ‘international expert judgement’: introducing the PTVA-4 model. Natural Hazards 83, 1229-1256.
| Crossref | Google Scholar |
Dominey-Howes D, Papathoma M (2007) Validating the ‘Papathoma Tsunami Vulnerability Assessment Model (PTVAM)’ using field data from the 2004 Indian Ocean tsunami. Natural Hazards 53, 43-61.
| Crossref | Google Scholar |
Dominey-Howes D, Dunbar P, Varner J, Papathoma-Köhle M (2010) Estimating probable maximum loss from a Cascadia tsunami. Natural Hazards 53, 43-61.
| Crossref | Google Scholar |
Dossi S, Messerschmidt B, Ribeiro LM, Almeida M, Rein G (2023) Relationships between building features and wildfire damage in California, USA and Pedrógão Grande, Portugal. International Journal of Wildland Fire 32, 296-312.
| Crossref | Google Scholar |
Ettinger S, Mounaud L, Magill C, Yao-Lafourcade A-F, Thouret J-C, Manville V, Negulescu C, Zuccaro G, De Gregorio D, Nardone S, Luque Uchuchoque JA, Arguedas A, Macedo L, Manrique Llerena N (2016) Building vulnerability to hydro-geomorphic hazards: estimating damage probability from qualitative vulnerability assessment using logistic regression. Journal of Hydrology 541, Part A, 563-581.
| Crossref | Google Scholar |
European Commission: Joint Research Centre, Oom D, De Rigo D, Pfeiffer H, Branco A, Ferrari D, Grecchi R, Artés Vivancos T, Houston Durrant T, Boca R, Maianti P, Libertà G, San-Miguel-Ayanz J, Lelouvier R, Onida M, Benchikha A, Abbas M, Humer F, Vacik H, Müller M, Heil K, Baetens J, Konstantinov V, Pešut I, Kaliger A, Petkoviček S, Papageorgiou K, Petrou P, Toumasis I, Pecl J, Ruuska R, Richoilley L, Chassagne F, Savazzi R, Gonschorek A, Panteli M, Debreceni P, Nagy D, Nugent C, Ben Zaken A, Di Fonzo M, Sciunnach R, Micillo G, Fresu G, Marzoli M, Pompei E, Ferlazzo S, Ascoli D, Romano R, Leisavnieks E, JaunķIķis Z, Mitri G, Repšienė S, Glazko Z, Assali F, Mharzi Alaoui H, Kok E, Stoof C, Timovska M, Botnen D, Piwnicki J, Szczygieł R, Kaczmarowski J, Pinho J, Moreira J, Cruz M, Sbirnea R, Mara S, Milanović S, Longauerová V, Jakša J, Lopez-Santalla A, Sandahl L, Andersson S, Beyeler S, Sautter M, Conedera M, Pezzatti B, Tolgay Dursun K, Baltaci U, Gazzard R, Moffat A, Sydorenko S (2022) ‘Pan-European wildfire risk assessment.’ (Publications Office of the European Union) 10.2760/9429
Grošelj P, Dolinar G (2023) Group AHP framework based on geometric standard deviation and interval group pairwise comparisons. Information Sciences 626, 370-389.
| Crossref | Google Scholar |
Haslinger K, Mayer K (2023) Early spring droughts in Central Europe: indications for atmospheric and oceanic drivers. Atmospheric Science Letters 24, e1136.
| Crossref | Google Scholar |
Jones MW, Abatzoglou JT, Veraverbeke S, Andela N, Lasslop G, Forkel M, Smith AJP, Burton C, Betts RA, van der Werf GR, Sitch S, Canadell JG, Santín C, Kolden C, Doerr SH, Le Quéré C (2022) Global and regional trends and drivers of fire under climate change. Reviews of Geophysics 60, e2020RG000726.
| Crossref | Google Scholar |
Kappes M, Papathoma-Köhle M, Keiler M (2012) Assessing physical vulnerability for multi-hazards using an indicator-based methodology. Applied Geography 32, 577-590.
| Crossref | Google Scholar |
Lampin-Maillet C, Mantzavelas A, Galiana L, Jappiot M, Long M (2010) Wildland–urban interfaces, fire behaviour and vulnerability, mapping and assessment. In ‘Towards Integrated Fire Management – Outcomes of the European Project Fire Paradox’. (Eds J Sande Silva, F Regio, PM Fernandes, E Rigolot) pp. 71–93. (European Forest Institute: Joensuu, Finland)
Laranjeira J, Cruz H (2014) Building vulnerabilities to fires at the wildland urban interface. In ‘Advances in Forest Fire Research’. (Ed. DX Viegas) pp. 673–684. (Imprensa da Universidade de Coimbra: Coimbra, Portugal) 10.14195/978-989-26-0884-6_76
Müller MM (2023) Jahresrückblick 2022. Waldbrand-Blog Österreich. Available at https://fireblog.boku.ac.at/2023/01/12/jahresrueckblick-2022/[In German]
Olefs M, Formayer H, Gobiet A, Marke T, Schöner W, Revesz M (2021) Past and future changes of the Austrian climate – Importance for tourism. Journal of Outdoor Recreation and Tourism 34, 100395.
| Crossref | Google Scholar |
Ozdemir MS (2005) Validity and inconsistency in the analytic hierarchy process. Applied Mathematics and Computation 161, 707-720.
| Crossref | Google Scholar |
Papathoma M, Dominey-Howes D (2003) Tsunami vulnerability assessment and its implications for coastal hazard analysis and disaster management. Natural Hazards and Earth System Sciences 6, 733-744.
| Crossref | Google Scholar |
Papathoma M, Dominey-Howes D, Zong Y, Smith D (2003) Assessing tsunami vulnerability, an example from Herakleio, Crete. Natural Hazards and Earth System Sciences 3, 377-389.
| Crossref | Google Scholar |
Papathoma-Köhle M, Schlögl M, Fuchs S (2019) Vulnerability indicators for natural hazards: an innovative selection and weighting approach. Scientific Reports 9, 15026.
| Crossref | Google Scholar | PubMed |
Papathoma-Köhle M, Schlögl M, Dosser L, Roesch F, Borga M, Erlicher M, Keiler M, Fuchs S (2022a) Physical vulnerability to dynamic flooding: vulnerability curves and vulnerability indices. Journal of Hydrology 607, 127501.
| Crossref | Google Scholar |
Papathoma-Köhle M, Schlögl M, Garlichs C, Diakakis M, Mavroulis S, Fuchs S (2022b) A wildfire vulnerability index for buildings. Scientific Reports 12, 6378.
| Crossref | Google Scholar |
R Core Team (2024) ‘R: A language and environment for statistical computing.’ (R Foundation for Statistical Computing: Vienna, Austria) Available at https://www.R-project.org/
Riedl R (2005) Der Analytic Hierarchy Process: Ein geeignetes Verfahren für komplexe Entscheidungen in der Wirtschaftsinformatik? HMD-Praxis Der Wirtschaftsinformatik 42, 104-114 [In German].
| Google Scholar |
Ritchie H, Rosado P, Roser M (2022) Natural disasters. Our world in data. Available at https://ourworldindata.org/natural-disasters
Saaty RW (1987) The analytic hierarchy process – what it is and how it is used. Mathematical Modelling 9, 161-176.
| Crossref | Google Scholar |
Saaty TL, Ozdemir MS (2003) Why the magic number seven plus or minus two. Mathematical and Computer Modelling 38, 233-244.
| Crossref | Google Scholar |
Samora-Arvela A, Aranha J, Correia F, Pinto DM, Magalhães C, Tedim F (2023) Understanding building resistance to wildfires: a multi-factor approach. Fire 6, 32.
| Crossref | Google Scholar |
Syphard AD, Keeley JE (2019) Factors associated with structure loss in the 2013-2018 California wildfires. Fire 2, 49.
| Crossref | Google Scholar |
Syphard AD, Brennan TJ, Keeley JE (2017) The importance of building construction materials relative to other factors affecting structure survival during wildfire. International Journal of Disaster Risk Reduction 21, 140-147.
| Crossref | Google Scholar |
Tavana M, Soltanifar M, Santos-Arteaga FJ (2023) Analytical hierarchy process: revolution and evolution. Annals of Operations Research 326, 879-907.
| Crossref | Google Scholar |
Thennavan E, Ganapathy GP, Chandra Sekaran SS, Rajawat AS (2016) Use of GIS in assessing building vulnerability for landslide hazard in The Nilgiris, Western Ghats, India. Natural Hazards 82, 1031-1050.
| Crossref | Google Scholar |
Thouret J-C, Ettinger S, Guitton M, Santoni O, Magill C, Martelli K, Zuccaro G, Revilla V, Charca JA, Arguedas A (2014) Assessing physical vulnerability in large cities exposed to flash floods and debris flows: the case of Arequipa (Peru). Natural Hazards 73, 1771-1815.
| Crossref | Google Scholar |
Vacca P, Caballero D, Pastor E, Planas E (2020) WUI fire risk mitigation in Europe: a performance-based design approach at home-owner level. Journal of Safety Science and Resilience 1, 97-105.
| Crossref | Google Scholar |
Vacik H, Müller M (2013) Waldbrand-Datenbank für Österreich. Wildbach - Und Lawinenverbauung 172, 188-189 [In German].
| Google Scholar |
Vacik H, Müller MM, Degenhart J, Sass O (2020) Auswirkungen von Waldbränden auf die Schutzfunktionalität alpiner Wälder. In ‘ExtremA 2019 – Aktueller Wissenstand zu Extremereignissen alpiner Naturgefahren in Österreich’. (Eds T Glade, M Mergili, K Sattler) pp. 173–201. (Vandenhoeck & Ruprecht: Vienna, Austria) Available at https://library.oapen.org/handle/20.500.12657/43512
Xanthopoulos G (2004) Factors affecting the vulnerability of houses to wildland fire in the Mediterranean region. In ‘II International workshop on forest fires in the wildland–urban interface and rural areas in Europe: an integral planning and management challenge’. pp. 85–92. (Mediterranean Agronomic Institute of Chania: Athens, Greece)