Heading and backing fire behaviours mediate the influence of fuels on wildfire energy
Joseph D. Birch





A Department of Plant, Soil and Microbial Sciences, Michigan State University, East Lansing, MI, USA. Email: mieselje@msu.edu
B Program in Ecology and Evolutionary Biology, Michigan State University, East Lansing, MI, USA.
C USDA Forest Service, Northern Research Station, Delaware, OH, USA. Email: matthew.b.dickinson@usda.gov
D Geospatial Technology and Applications Center, USDA Forest Service, Asheville, NC, USA. Email: alicia.reiner@usda.gov
E USDA Forest Service, Pacific Southwest Research Station, Redding, CA, USA. Email: eric.e.knapp@usda.gov
F USDA Forest Service, Enterprise Program, Reno, NV, USA. Email: scott.dailey@usda.gov
G USDA Forest Service, PSW Region, Stanislaus National Forest, Sonora, CA, USA.
H Department of Wildland Resources, Utah State University, Logan, UT, USA. Email: james.lutz@usu.edu
International Journal of Wildland Fire 32(8) 1244-1261 https://doi.org/10.1071/WF22010
Submitted: 9 February 2022 Accepted: 30 June 2023 Published: 21 July 2023
© 2023 The Author(s) (or their employer(s)). Published by CSIRO Publishing on behalf of IAWF. This is an open access article distributed under the Creative Commons Attribution-NonCommercial-NoDerivatives 4.0 International License (CC BY-NC-ND)
Abstract
Background: Pre-fire fuels, topography, and weather influence wildfire behaviour and fire-driven ecosystem carbon loss. However, the pre-fire characteristics that contribute to fire behaviour and effects are often understudied for wildfires because measurements are difficult to obtain.
Aims: This study aimed to investigate the relative contribution of pre-fire conditions to fire energy and the role of fire advancement direction in fuel consumption.
Methods: Over 15 years, we measured vegetation and fuels in California mixed-conifer forests within days before and after wildfires, with co-located measurements of active fire behaviour.
Key results: Pre-fire litter and duff fuels were the most important factors in explaining fire energy and contributed similarly across severity categories. Consumption was greatest for the forest floor (litter and duff; 56.8 Mg ha−1) and 1000-h fuels (36.0 Mg ha−1). Heading fires consumed 13.2 Mg ha−1 more litter (232%) and 24.3 Mg ha−1 more duff (202%) than backing fires. Remotely sensed fire severity was weakly correlated (R2 = 0.14) with fuel consumption.
Conclusions: 1000-h fuels, litter, and duff were primary drivers of fire energy, and heading fires consumed more fuel than backing fires.
Implications: Knowledge of how consumption and fire energy differ among contrasting types of fire behaviours may inform wildfire management and fuels treatments.
Keywords: backing fire, burn severity, carbon loss, FBAT, fire effects, flanking fire, forest change, heading fire, Klamath Mountains, Sierra Nevada.
Introduction
Wildfire impacts are often assessed over extended post-fire timescales and by using remote sensing techniques that characterise near-term (i.e. ~1 year) to delayed fire effects over landscape scales. However, these approaches may not adequately capture immediate fire effects such as fuel consumption, carbon emissions, and the release of damaging or lethal fire energy into surrounding vegetation, or the fire behaviours that produce those effects (Johnson 1996; Dickinson 2002; De Groot et al. 2009). Measuring the drivers of fire behaviour and immediate fire effects is important for improving knowledge about how fire behaviour influences the post-fire environment and long-term forest recovery. Understanding these relationships depends on accurate measures of immediate fire effects and their drivers, because relatively small absolute differences in fire behaviour and fuel consumption may produce outsized ecosystem effects. For example, the consumption of overstorey foliage, despite it being a relatively small proportion of overall forest biomass (Miesel et al. 2018), may result in tree mortality, loss of carbon sequestration potential, and eventual increases in downed woody fuels (Furniss et al. 2020b; Jeronimo et al. 2020; Lutz et al. 2020). In contrast, equivalent consumption of litter or duff mass may have lesser direct impacts on overstorey trees but result in high carbon loss and smoke emissions (McRae et al. 2006; De Groot et al. 2009; but see Cansler et al. (2019)).
Understanding relationships among forest fuels, fire behaviour, and effects in differing fuel strata (e.g. canopy or ground fuels) is important for refining our knowledge of how these specific wildfire characteristics influence post-fire ecosystem responses (sensu Keeley et al. 2009). Relatively recent advancements in applications of remote sensing data over the past decade(s) have expanded our ability to characterise post-fire landscapes, but these techniques rely most directly on detected changes in overstorey vegetation reflectance that occur within the first post-fire year (van Wagtendonk et al. 2004; Miller and Thode 2007). These increasingly standard measures of remotely sensed fire severity may not relate directly to immediate fire effects because delayed tree mortality and additional factors such as insect infestation or drought may also occur during and after the first post-fire year and contribute to longer-term ecosystem responses (van Mantgem et al. 2018; Lutz et al. 2020). Given the widespread application of remote sensing data and derived burn severity metrics in post-wildfire management (Eidenshink et al. 2007), evaluating their ability to represent direct fire effects such as fuel consumption will be important for strengthening linkages between immediate and longer-term fire effects.
Fire effects are inherently driven by fire behaviour, which varies as a function of fuel, topography, and fire weather. Interactions among these factors cause wildfires to transition from smouldering to more energetic flaming phases (Rein 2016), leading fires to advance rapidly through surface fuels such as shrubs, herbaceous plants, or fine litter and woody fuels. The amount of fuel consumed and its heat of combustion together determine fire energy (Van Wagner 1972; Johnson 1996). Fire energy, an estimate of the amount of total heat released by fuel consumption and modified by fuel moisture, may be more directly related to fire effects than measures of fuel consumption alone. Differences in fire energy and behaviour may cause variable fire effects with long-term consequences for forest health and fuel loadings. For example, heading fires (fire advancement with the wind or slope) may exhibit faster rates of pre-conditioning or drying of nearby fuels, faster rates of spread, and greater fireline intensities than backing fires (Rothermel 1972). Although the interactions between fire spread, topography and fuel consumption have been relatively well-studied for prescribed fires (Fahnestock and Hare 1964; Knapp et al. 2011; Skowronski et al. 2020), scientific understanding of how these factors influence fire energy during wildfires burning under more extreme conditions remains largely lacking. A better understanding of relationships among pre-fire fuel loadings, environmental conditions, and fire behaviour may help support tactical decision-making on wildfires, particularly relative to ignition and holding operations that are intended to reduce fireline intensities and fire effects on vegetation (Ingalsbee and Raja 2015; Fillmore et al. 2021).
To address these knowledge gaps, we analysed a globally unique dataset of immediate pre- and post-fire forest composition and structure and fuel measurements coupled with in situ fire behaviour measurements collected across 15 years on wildfires occurring in Sierra Nevada mixed-conifer forests (California, USA). Our primary objective was to define the immediate drivers and effects of contrasting wildfire behaviour. Specifically, we evaluated the following questions:
What are the immediate effects of wildfire on forest fuel loadings, and how does the magnitude of change differ among forest fuel strata?
What are the drivers of wildfire energy and how do they differ with burn severity?
How do ground-based measurements of fuel consumption relate to satellite remote sensing burn severity?
How do fuel consumption and fire energy differ among contrasting directions of fire advancement (e.g. heading, backing, and flanking fires)?
Methods
Study area
Sierra Nevada mixed-conifer forests represent a continuum of pine- (Pinus spp.) and fir- (Abies spp.) dominated forests whose distribution is jointly controlled by fire and climate (Parks et al. 2018; van Wagtendonk et al. 2020). Since the early 1900s, fire suppression and land use change have caused a shift in forest composition, structure, and biomass. These forests, which historically consisted of a higher proportion of Pinus species, have become denser and increasingly encroached by shade-tolerant species such as Abies concolor (Gordon & Glendinning) Hildebrand (white fir) (Parsons and DeBenedetti 1979; McIntyre et al. 2015; Hagmann et al. 2021). Similar to forests across western North America, these forests are experiencing pronounced periodic drought (Young et al. 2020), a longer fire season (Westerling et al. 2006), and greater forest mortality rates (van Mantgem et al. 2009) relative to their pre-Euro-American settlement conditions.
Our study consisted of 112 plots established across 18 wildfires that occurred in mixed-conifer forests between 2005 and 2020, spanning an elevation range of 419–2656 m (Fig. 1). All data were collected by the USDA Forest Service Fire Behaviour Assessment Team (FBAT) (Table 1). The FBAT collects pre-fire, during-fire, and post-fire data to predict and quantify fire behaviour and understand short- and long-term fire effects. The plots occur predominantly within US National Forests (n = 106); an additional six plots were located inside Yosemite National Park, including four in the Yosemite Forest Dynamics Plot (YFDP) (Lutz et al. 2012; Davies et al. 2021). All plots burned between 18 June and 20 October (June: 5% of plots; July 30%; August 25%; September 35%; October 3%) and usually during the daytime.
The location of the 112 (circles) plots established by the Fire Behaviour Assessment Team from 2005 to 2020 in California, USA. Plot locations are marked with shaded circles. Elevation sourced from 1 × 1-degree 1/3 arc-second digital elevation models (United States Geological Survey 2020).
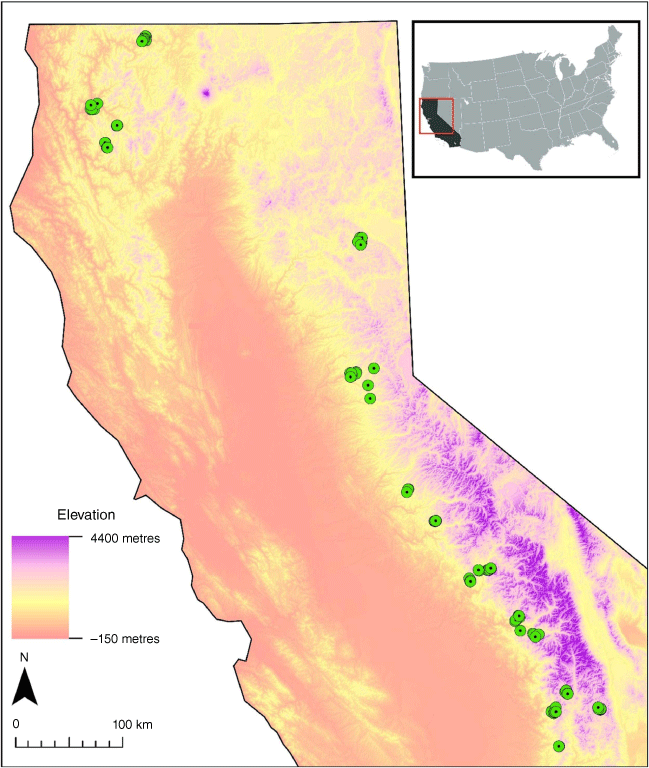
Name | Ecoregion | Start date | Source | Area burned (ha) | Number of plots | Elevation range (m) | Dominant tree species |
---|---|---|---|---|---|---|---|
Antelope Complex | Sierra Nevada | 5 July 2007 | Lightning | 9037 | 9 | 1696–1840 | P. jeffreyi (0.92) – A. concolor (0.05) |
Aspen | Sierra Nevada | 22 July 2013 | Lightning | 9282 | 6 | 1543–1679 | A. concolor (0.42) – P. ponderosa (0.34) |
Bake-Oven | Klamath | 23 July 2006 | Lightning | 26 324 | 4 | 610–1120 | P. menziesii (0.61) – A. menziesii (0.12) |
Beaver | Klamath | 30 July 2014 | Lightning | 13 149 | 7 | 715–1441 | P. menziesii (0.73) – P. ponderosa (0.15) |
Cedar | Sierra Nevada | 16 Aug 2016 | Human | 11 776 | 3 | 1776–1824 | C. decurrens (0.55) – A. concolor (0.34) |
Clover | Sierra Nevada | 31 May 2008 | Lightning | 6389 | 6 | 2456–2525 | P. jeffreyi (0.44) – A. concolor (0.26) |
Crag | Sierra Nevada | 24 July 2005 | Lightning | 479 | 1 | 2640 | P. jeffreyi (0.66) – J. occidentalis (0.19) |
French | Sierra Nevada | 27 July 2014 | Human | 5597 | 2 | 1579–1601 | P. ponderosa (0.51) – C. decurrens (0.30) |
King | Sierra Nevada | 13 Sept 2014 | Human | 39 531 | 3 | 1499–1672 | P. ponderosa (0.27) – A. concolor (0.27) |
Lion | Sierra Nevada | 8 July 2011 | Lightning | 8369 | 9 | 2042–2185 | P. ponderosa (0.79) – A. concolor (0.18) |
Pier | Sierra Nevada | 29 Aug 2017 | Human | 14 819 | 6 | 1486–1904 | C. decurrens (0.47) – A. concolor (0.20) |
Ralston | Sierra Nevada | 5 Sept 2006 | Unknown | 3407 | 14 | 922–1301 | P. ponderosa (0.26) – P. menziesii (0.24) |
Red Salmon Complex | Klamath | 27 July 2020 | Lightning | 46 436 | 4 | 656–712 | P. sabiniana (0.31) – P. menziesii (0.20) |
Rim | Sierra Nevada | 17 Aug 2013 | Human | 103 670 | 9 | 1576–1875 | A. concolor (0.25) – P. lambertiana (0.20) |
Rough | Sierra Nevada | 31 July 2015 | Lightning | 61 328 | 14 | 1854–2485 | A. concolor (0.44) – S. giganteum (0.19) |
Somes | Klamath | 25 July 2006 | Unknown | 6275 | 8 | 419–1634 | P. menziesii (0.65) – Q. densiflorus (0.15) |
Walker | Sierra Nevada | 4 Sept 2019 | Unknown | 22 101 | 3 | 1174–1710 | P. ponderosa (0.96) – P. menziesii (0.04) |
Willow | Sierra Nevada | 25 July 2015 | Human | 2307 | 4 | 1324–1700 | P. ponderosa (0.54) – C. decurrens (0.28) |
Number of plots denotes the number of plots that were measured pre-fire, burned and remeasured for immediate post-fire change. Dominant forest type denotes the most abundant overstorey species and their proportional pre-fire basal area in parenthesis. Plot ecoregions were classified according to Miles and Goudey (1997) and are within the Klamath Mountains section (M261A) or the Sierra Nevada sections (M261E).
The plots encompass a range of topography and forest compositions (Supplementary Appendix S1, Supplementary Table S1). Common tree species included Abies concolor (white fir), Calocedrus decurrens (Torrey) Florin (incense cedar), Pinus jeffreyi Greville and Balfour (Jeffrey pine), Pinus lambertiana Douglas (sugar pine), Pinus ponderosa Douglas ex Lawson and C. Lawson (ponderosa pine), Pseudotsuga menziesii var. menziesii (Mirbel) Franco (Douglas-fir), and Quercus kelloggii Newberry (California black oak) (Flora of North America Editorial Committee e. 1993+). Prior to establishment, many encompassing areas had been treated by land managers to address specific management objectives. We did not analyse pre-fire treatment history because any remaining treatment outcomes (e.g. lower fuel loadings or altered forest structure) were inherently reflected in our pre-fire fuel and vegetation structure, composition, and fuel measurements.
Plot establishment
The FBAT field methods were chosen to balance data collection with speed, which is particularly important for pre-wildfire measurements when opportunities for data collection may be fleeting. Detailed protocols are available online (https://www.frames.gov/fbat/home). Plots were established between 1 and 3 days prior to wildfire reaching the location and were re-measured within 10 days post-burn. Plot locations were opportunistically selected to provide representative conditions of the local landscape while also being located within several hundred metres (µdistance = 244 m) of the nearest road; this helped maximise the number of plots established and provided for escape routes should they be needed. Each plot was monumented with rebar at plot centre and at the end of each fuel transect to aid post-fire remeasurement. In each plot, we placed a fire-protected camera mounted on a tripod at 1.2 m above ground level, which was oriented to maximise the likelihood that the fire would spread across the plot perpendicular to the camera. Camera systems were designed with heat-sensitive trip wires that initiated recording on fire arrival. We measured forest vegetation and fuels for each fuel stratum (e.g. forest canopy to ground fuels) at the time of plot establishment and after each plot burned. We determined elevation, slope, and aspect using 1 × 1-degree 1/3 arc-second digital elevation models (United States Geological Survey 2020) in ArcGIS Desktop 10.6.1 (ESRI 2020).
Tree measurements
We used a Spiegel Relaskop (Silvanus Inc.; Salzburg, Austria) to select trees for measurement in a variable radius plot, varying the basal area factor based on the stem density of each plot. In general, we chose a basal area factor so that 5–10 pole-sized trees (2.5–15.0 cm diameter at breast height (1.37 m, DBH)) and 5–10 overstorey trees (>15.0 cm DBH) were measured in each plot. The same basal area factor was generally used across plots within a fire. This criterion balanced the need for a comprehensive assessment of the forest with measurement expedience in the face of advancing wildfires. Variable radius plots with 5–10 trees per plot are largely similar to fixed-radius plots in estimating basal area and trees per hectare (Bitterlich 1984; Piqué et al. 2011). We used the azimuth and distance from plot centre to relocate the same trees for post-fire measurements.
Trees were identified to species, measured for DBH, tree height, and health-related conditions (e.g. pitch, insect galleries, fire scars, or evidence of mechanical damage) noted. To assess canopy characteristics, we used a TruPulse laser hypsometer (Laser Tech; Colorado, USA) to measure tree height, canopy base height (i.e. height of the lowest live foliage), and height to live crown (height to the nearest branch that is contiguous across ≥30° of the canopy). For the post-fire measurements, we revisited and assessed each tree for mortality, bole scorch height, partial bole consumption, and percentage of the canopy scorched or torched. Trees that were live at the pre-fire measurement were classified as newly dead if all foliage was either consumed or scorched. Tree mortality was likely underestimated due to tree remeasurements occurring only a few days post-fire, thereby capturing only immediate mortality in contrast with delayed mortality, which can occur over months to years after fire. We present pole and overstorey tree data together in all tree-layer analyses.
Surface and ground fuel measurements
In each plot, we established one to three 15.24 m transects originating distal to the plot centre to minimise disrupting surface fuels at plot centre (Fig. 2a). These transects were used as the centerline of belt transects for assessing surface vegetation (FBAT 2022), and for a modified version of Brown’s Planar Intercept Method for assessing downed woody fuels (hereafter, Brown’s transects; Brown 1974). Plots established prior to 2010 (n = 43) had one or two transects oriented at random azimuths around plot centre, whereas plots established in and after 2010 (n = 69) had three transects oriented at 0°, 120°, and 240° azimuths. We measured tree seedlings, shrubs, grass, and forbs using 1.0 m × 15.24 m belt transects along the fuel transects described above (Fig. 2a). We measured live and dead cover (% ground surface area) and mean height, and classified the bulk density and morphology for each species using the shrub and grass photoseries in Burgan and Rothermel (1984), modified to also classify tree seedlings and forbs. We used the bulk density, average cover (%), and height to estimate the pre- and post-fire loadings of seedlings, shrubs, grasses, and forbs. We also used photos taken along each transect from the transect origin and end point to qualitatively assess change in surface vegetation between pre-fire and post-fire images.
A diagram of (a) the Fire Behaviour Assessment Team (FBAT) plot measurements and (b) modified Brown’s fuels transects. Measurements include variable radius tree plots, three modified Brown’s fuel transects (Brown 1974), three vegetation transects, thermocouples for fire arrival and rate of spread, and a video camera to assess fire behaviour.
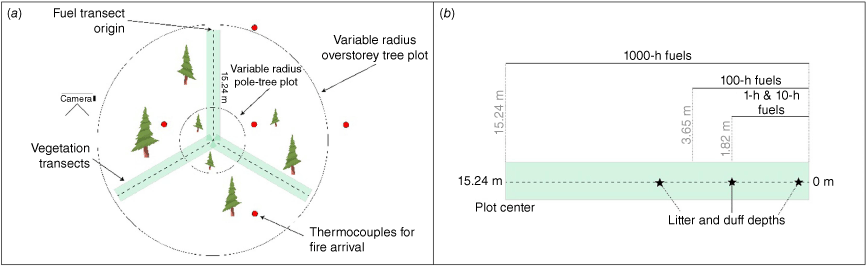
We assessed downed woody fuels, litter, and duff in each of the three 15.24 m Brown’s transects (Brown 1974). We assessed 1-h (≤0.6 cm diameter) and 10-h (>0.6–2.54 cm) fuels from 0 to 1.82 m along the transect, 100-h fuels (>2.54–7.6 cm) from 0 to 3.65 m, and 1000-h fuels (>7.6 cm) along the entire transect (Fig. 2b). These size classes are hereafter referred to cumulatively as ‘downed woody fuels’. Litter and duff depths were measured at three locations on each transect (0.3, 1.8, and 4.8 m from the origin).
Biomass calculations
We used allometric equations to estimate aboveground bole biomass of living trees (Chojnacky et al. 2014) (Supplementary Appendix S1, Supplementary Table S2). For the biomass of consumed foliage, we used the component parameters for foliage (Jenkins et al. 2003) and multiplied biomass estimates by the post-fire percentage of the canopy with unconsumed needles. For standing dead trees of decay class one or two (Lutz et al. 2020), we used species-specific conversion factors from Cousins et al. (2015), and general decay coefficients from Harmon et al. (2008) after subtracting estimates of foliage biomass (Supplementary Appendix S1, Supplementary Tables S3, S4). We modelled standing dead trees of decay class ≥3 as conic frustums with a top diameter of 0.01 cm and used the volume to estimate mass following the species-specific and general density values of Harmon et al. (2008). For 130 trees (53% of dead trees) the decay classification was not recorded in the field, and they were treated as decay class one.
Downed woody fuels, litter, and duff fuel loadings were calculated following van Wagtendonk et al. (1998). Plots measured during the 2007 Antelope Complex (n = 9) and 2006 Ralston fire (n = 14) did not have post-fire understorey vegetation measurements. To estimate post-fire loadings for these plots, we compared pre- and post-fire photos of the vegetation transect to estimate the percentage change in understorey vegetation cover (Supplementary Appendix S1, Supplementary Fig. S1; Supplementary Appendix S1, Supplementary Table S5). Four FBAT plots located within the Yosemite Forest Dynamics Plot (YFDP) had spatially explicit mapping of pre- and post-fire tree, surface fuel, and understorey vegetation data of a higher resolution than typical FBAT measurements (Lutz et al. 2017; Cansler et al. 2019), which we compiled and used for these analyses (see Supplementary Appendix S1 Methods for a description of data collection at the YFDP).
Fire weather, fuel moistures, and environmental characteristics
Because fire behaviour is influenced by local fine-scale weather, we generated interpolated hourly weather (air temperature, relative humidity, precipitation, and wind speed and direction) for each plot. The remote automated weather stations (RAWS) are a widespread system of nearly 2200 weather-recording stations across the USA. Despite their abundance, many areas have been historically under-represented by RAWS stations, particularly in California (Horel and Dong 2010); therefore, we used the program BioSIM 11.0 (Régnière et al. 2018) to generate interpolated hourly weather for our plots to better represent local conditions at the time of the fires. Prior to interpolation, BioSIM weights the four nearest RAWs station data according to their distance from the plot and the similarity in elevation and aspect (Supplementary Appendix S2). After interpolation, we visually assessed the interpolated data for outliers and removed any missing or anomalous measurements.
We used interpolated RAWS hourly weather data during the year of plot establishment in Fire Family Plus 5.0 software (Bradshaw and McCormick 2000) to estimate fuel moistures for the hour of fire arrival. In cases where we did not know the hour of arrival (N = 58 plots), we used the moisture values generated for 1300 hours on the day the plot burned. For 1000-h fuels, we used a minimum of 5 months of pre-fire hourly weather to incorporate seasonal scale. All fuel moisture variables were calculated using the NFDRS 2016 timber fuel model.
We converted wind speeds from 10 m height to midflame using the ‘waf’ function in the R package firebehavioR 0.1.2 (Ziegler et al. 2019) to account for surface fuel height, tree height, and canopy ratio following the formula of Andrews (2012). To assess the accuracy of the interpolated winds we compared them with anemometer data from a subset of plots where wind data were measured (Supplementary Appendix S1, Supplementary Fig. S2). For five plots with missing fuel bed height, we used the wind adjustment factor from the nearest (<1 km) comparable plot.
To estimate heat load of each plot, we calculated the McCune Heat Load in spatialEco 1.3.7 (Evans and Ram 2015). The McCune Heat Load is a variable used to estimate the potential incident solar radiation at a given location using latitude, slope, and aspect (McCune 2007). To estimate the influence of topography on soil and fuel wetness we calculated a one-step topographic wetness index using a multiple-flow-direction algorithm in SAGA 7.9.1 (Conrad et al. 2015).
Calculation of remotely sensed burn severity
To evaluate burn severity, we retrieved the differenced normalised burn ratio (dNBR) and the relative dNBR (RdNBR) from the Monitoring Trends in Burn Severity (MTBS) database, which utilises paired pre-fire and 1-year post-fire imagery to assess changes in vegetation reflectance (Eidenshink et al. 2007; Miller and Thode 2007). We used the MTBS-provided dNBR offset to account for changes in pre- and post-fire reflectance due to vegetation phenology and weather. To derive categorical burn severity, we used the RdNBR cutoff values of Miller and Thode (2007), which are empirically linked to ground-based composite burn index (CBI) plots in mixed-vegetation types in Sierra Nevada, many of which were mixed-conifer forests. All remotely sensed severity estimates (hereafter ‘burn severity’) were extracted from 30 × 30 m pixels. The categorical burn severity, in order of increasing severity, include ‘Unchanged’ (RdNBR < 69), ‘Low’ (69 ≤ RdNBR ≤ 315), ‘Moderate’ (315 < RdNBR < 641), and ‘High’ (641 ≤ RdNBR) categories (Miller and Thode 2007).
Total and flaming fire energy calculations
To estimate total fire energy produced during the frontal and post-frontal phases of combustion, we multiplied the low heat of combustion (18 600 kJ kg−1) by the amount of fuel consumed in tree foliage, shrub, seedling, herbaceous, grass, 1- to 1000-h fuels, litter, and duff (Johnson 1996) for each plot. We modified the heat of combustion by −24 kJ kg−1 per percentage of fuel moisture (Van Wagner 1972) for each straum of consumed fuels. One-hour fuel moisture was used to estimate the moisture content of the litter layer. To calculate the energy produced principally during the frontal phase of combustion (hereafter flaming fire energy), we used the consumption values of tree foliage, shrub, seedling, herbaceous, 1- to 100-h fuels, and litter, which is the fuel stratum most likely to directly contribute to frontal phase combustion.
Statistical analyses
Analyses were conducted with the statistical program R 4.0.4 (R Core Team 2021), using the graphical user interface R Studio 1.4.1106 (R Studio Team 2020). We created graphs using ggplot2 3.35 (Wickham 2016) and ggpubr 0.2.5 (Kassambara 2020). We used the Forest Vegetation Simulator version 20200903 (Dixon 2002) to calculate trees per hectare using plot-specific basal area factors.
To assess differences in fuel loadings and relative changes between pre- and post-fire fuel strata, we used pairwise Wilcoxon Signed-rank tests with a false-discovery-rate p-adjustment from the stats 3.6.2 package (R Core Team 2021). To calculate the relative change in loading by fuel stratum, we used the formula:
The relative change by fuel stratum was not bounded between 0 and 1 because in some cases, the post-fire measurements recorded slightly increased surface or litter and duff fuels relative to the pre-fire conditions. Such increases were likely the result of new fuel deposition on a transect (a possibility we attempted to identify in the field as part of the protocol) or a transect location placement slightly off from the original location. Fuel-specific relative change values are calculated only for plots that had pre-fire fuel loading >0.
To identify the best explanatory variables for fire energy we used a multi-model inference approach with model averaging. Multi-model inference uses Akaike’s Information Criterion (AICc) to rank all possible models chosen from an a priori list of reasonable models. Prior to model averaging we generated an a priori list of reasonable explanatory variables and plotted pairwise correlations between variables using the ‘ggpairs’ function in GGally 1.5.0 (Schloerke et al. 2020). We visually assessed correlations among variables and evaluated each variable’s variance inflation factor (VIF) using the ‘vif’ function in the car 3.0-7 package (Fox and Weisberg 2019). We removed the highly collinear (VIF > 2.5) variables: air temperature; pre-fire overstorey foliage; 1-h fuel moisture; 100-h fuel moisture; herbaceous fuel moisture; and the topographic moisture index (Table 2).
Variable | Mean | Origin |
---|---|---|
Slope (%) | 25.00 (1.00) | 1 × 1-degree 1/3 arc-second DEM |
Elevation (m)A | 1586.00 (48.00) | 1 × 1-degree 1/3 arc-second DEM |
McCune Heat Index | 0.73 (0.01) | Calculated with DEM and spatialEco 1.37 |
Topographic moisture indexA | −16.50 (0.20) | Calculated with SAGA |
Air temperature (°C)A | 24.00 (1.10) | Interpolated from RAWS |
Midflame windspeed (km h−1) | 2.30 (0.10) | Interpolated from RAWS and FireBehavoR |
Relative humidity (%) | 31.20 (1.30) | Interpolated from RAWS |
1-h fuel moisture (%)A | 8.40 (0.60) | Interpolated from RAWS and estimated with FireFamily 5.0 |
10-h fuel moisture (%) | 9.50 (0.30) | Interpolated from RAWS and estimated with FireFamily 5.0 |
100-h fuel moisture (%)A | 10.40 (0.20) | Interpolated from RAWS and estimated with FireFamily 5.0 |
1000-h fuel moisture (%) | 11.10 (0.20) | Interpolated from RAWS and estimated with FireFamily 5.0 |
Live woody fuel moisture (%) | 111.10 (4.60) | Interpolated from RAWS and estimated with FireFamily 5.0 |
Herbaceous fuel moisture (%)A | 138.60 (3.40) | Interpolated from RAWS and estimated with FireFamily 5.0 |
Pre-fire overstorey foliage (Mg ha−1)A | 13.40 (1.20) | Estimated using parameter components (Jenkins et al. 2003) and empirical measurements |
Pre-fire tree mass (Mg ha−1) | 261.20 (21.40) | Estimated using allometry (Chojnacky et al. 2014) and empirical measurements |
Pre-fire standing dead tree mass (Mg ha−1) | 25.40 (4.80) | Estimated using allometry (Harmon et al. 2008; Chojnacky et al. 2014; Cousins et al. 2015) and empirical measurements |
Pre-fire shrub mass (Mg ha−1) | 5.50 (1.70) | Estimated using allometry (Burgan and Rothermel 1984) and empirical measurements |
Pre-fire seedling mass (Mg ha−1) | 3.30 (0.30) | Estimated using allometry (Burgan and Rothermel 1984) and empirical measurements |
Pre-fire forb mass (Mg ha−1) | 0.02 (0.00) | Estimated using allometry (Burgan and Rothermel 1984) and empirical measurements |
Pre-fire grass mass (Mg ha−1) | 0.05 (0.02) | Estimated using allometry (Burgan and Rothermel 1984) and empirical measurements |
Pre-fire 1-h fuel mass (Mg ha−1) | 0.80 (0.10) | Estimated using allometry (van Wagtendonk et al. 1998) and empirical measurements |
Pre-fire 10-h fuel mass (Mg ha−1) | 2.50 (0.20) | Estimated using allometry (van Wagtendonk et al. 1998) and empirical measurements |
Pre-fire 100-h fuel mass (Mg ha−1) | 4.10 (0.40) | Estimated using allometry (van Wagtendonk et al. 1998) and empirical measurements |
Pre-fire 1000-h fuel mass (Mg ha−1) | 47.20 (8.40) | Estimated using allometry (van Wagtendonk et al. 1998) and empirical measurements |
Pre-fire litter mass (Mg ha−1) | 20.80 (2.00) | Estimated using allometry (van Wagtendonk et al. 1998) and empirical measurements |
Pre-fire duff mass (Mg ha−1) | 45.50 (3.60) | Estimated using allometry (van Wagtendonk et al. 1998) and empirical measurements |
Mean values are provided with standard errors in parentheses.
AVariables removed from the model averaging due to unacceptable collinearity with other variables.
We generated all possible models, including a null model, and ranked the models according to the best (lowest) AICc score and their difference in AICc from the best performing model (ΔAICc). Models with a ΔAICc < 3 are considered excellent models (Burnham and Anderson 2002) and suitable for global model averaging. In multi-model inference, variables are ranked according to relative variable importance (RVI), which is the sum of model weights in which a variable occurs. Variables with an RVI value >0.5 are considered highly influential. For the averaged model, we used the conservative full coefficient estimates, which biases the variable coefficients towards ‘0’ by averaging variables across models where they did not appear. We used the ‘dredge’ function in the MuMIn 1.43.17 package (Barton 2020) to weight models prior to averaging. Four plots with overall post-fire fuel loads higher than pre-fire (potentially due to newly deposited fuels) were excluded from any fire-energy analyses due to possible negative calculated energy values. To investigate differences in fire energy among types of fire behaviour, we used a subset of our data (n = 56) where video recordings and post-fire observations allowed for fire categorisation (i.e. heading, backing, flanking). This subset included heading (n = 15), backing (n = 31), and flanking (n = 10) plots from 14 wildfires. We used a pairwise Wilcoxon rank-sum test with a false-discovery rate p-adjustment to test for differences in total fire energy, flaming fire energy, and fuel consumption of each stratum across the fire types. To assess differences in the relative consumption of litter and duff by transect, we used an analysis of variance with a Tukey Honest Significant Differences comparison.
Results
Pre-fire fuel loadings and weather differences among satellite-based burn severity categories
Mean pre-fire fuel loadings varied substantially among fuel strata (Fig. 3) and by fire event (Supplementary Appendix S1, Supplementary Table S6). The largest portion of pre-fire mass was contained in tree boles (both living and dead) (Table 3). Relatively little pre-fire fuel was contained within seedlings (3.3 ± 1.9 Mg ha−1), shrubs (5.5 ± 1.7 Mg ha−1), grass (0.05 ± 0.02 Mg ha−1), and forbs (0.02 ± 0.00 Mg ha−1) (Fig. 3c–f). There were no significant differences in pre-fire fuel loadings among burn severity categories for 100- to 1000-h fuels (Fig. 3j–i), whereas pre-fire 1-h fuel loadings were greater in high severity plots (1.3 ± 0.3 Mg ha−1) relative to unchanged plots (0.9 ± 0.3 Mg ha−1). Pre-fire litter (20.8 ± 2.0 Mg ha−1) and duff (45.4 ± 3.6 Mg ha−1) fuel loadings were similar among burn severity categories.
The pre- and post-fire fuel loadings (Mg ha−1) by fuel strata for 112 mixed-conifer forests in California, USA. Error bars are ±1 standard error. Biomass is plotted for pre-, post-fire and the change in biomass for (a) live overstorey foliage biomass, (b) standing dead tree bole fuels, (c) seedling fuels, (d) shrub fuels, (e) grass fuels, (f) forb fuels, (g) 1000-h fuel fuels, (h) 100-h fuel fuels, (i) 10-h fuel fuels, (j) 1-h fuels, (k) litter fuels, and (l) duff fuels. Lowercase letters indicate significant differences in pre-fire biomass and post-fire change (P < 0.05) among severity categories. Non-significant differences are denoted by ‘ns’.
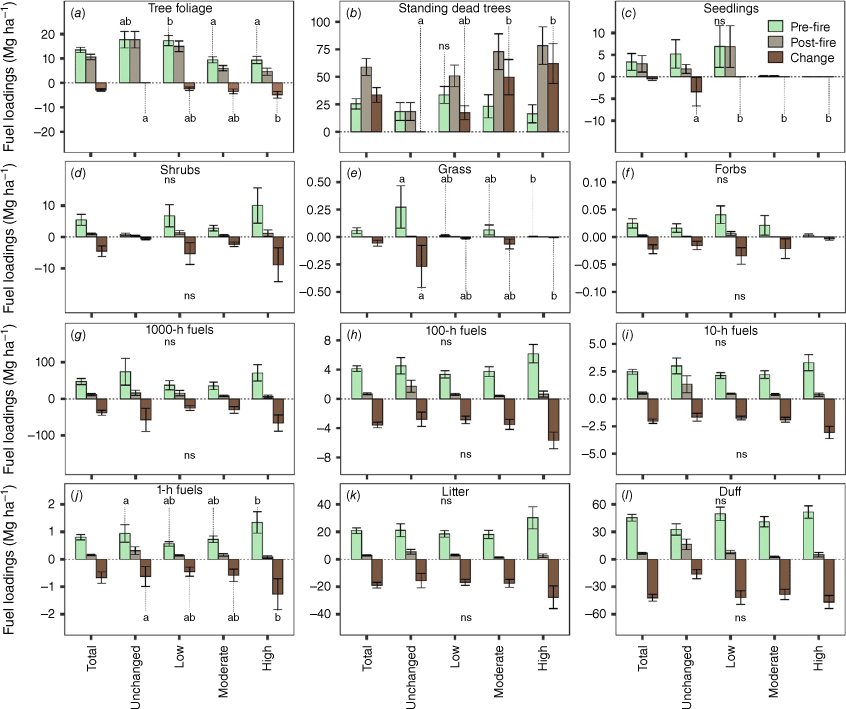
# of plots | Total | Trees | Standing dead trees | Shrub and seedlings | Grass and forbs | 1000-h | 100-h | 10-h | 1-h | Litter | Duff | |
---|---|---|---|---|---|---|---|---|---|---|---|---|
Pre-fire fuels | ||||||||||||
Unchanged | 13 | 510.9 (79.6) | 350.2 (66.3) | 18.3 (8.1) | 6.0 (3.2) | 0.2 (0.1) | 73.8 (36.5) | 4.5 (1.1) | 2.9 (0.7) | 0.9 (0.3) | 21.1 (4.6) | 32.5 (6.1) |
Low | 44 | 510.1 (47.9) | 351.5 (41.3) | 33.3 (7.7) | 13.6 (5.7) | 0.0 (0.0) | 37.2 (12.6) | 33 (0.4) | 2.1 (0.2) | 0.5 (0.0) | 18.4 (2.3) | 49.7 (7.2) |
Moderate | 34 | 300.2 (28.9) | 172.7 (21.9) | 23.1 (10.4) | 3.0 (0.9) | 0.0 (0.0) | 35.4 (10.2) | 3.7 (0.6) | 2.2 (0.3) | 0.7 (0.1) | 18.0 (2.9) | 41.2 (5.6) |
High | 21 | 349.6 (36.5) | 159.6 (25.2) | 16.3 (8.2) | 10.0 (5.6) | 0.0 (0.0) | 70.8 (22.2) | 6.1 (1.2) | 3.2 (0.7) | 1.3 (0.3) | 30.2 (8.0) | 51.6 (6.7) |
Post-fire fuels | ||||||||||||
Unchanged | 13 | 412.2 (77.3) | 350.2 (66.3) | 18.3 (8.1) | 2.1 (1.1) | 0.0 (0.0) | 16.3 (7.0) | 1.7 (0.8) | 1.3 (0.7) | 0.3 (0.1) | 5.8 (1.7) | 16.3 (5.8) |
Low | 44 | 408.0 (42.3) | 321.0 (43.0) | 50.8 (9.8) | 8.3 (4.7) | 0.0 (0.0) | 15.5 (7.3) | 0.6 (0.1) | 0.4 (0.0) | 0.1 (0.0) | 3.0 (0.6) | 7.9 (1.7) |
Moderate | 34 | 198.1 (23.5) | 111.7 (19.2) | 72.8 (18.1) | 0.6 (0.3) | 0.0 (0.0) | 7.8 (2.3) | 0.4 (0.1) | 0.4 (0.0) | 0.1 (0.1) | 1.4 (0.3) | 2.6 (0.7) |
High | 21 | 175.7 (25.0) | 81.0 (25.7) | 78.3 (17.0) | 1.2 (1.0) | 0.0 (0.0) | 6.4 (3.7) | 0.6 (0.4) | 0.3 (0.1) | 0.0 (0.0) | 2.6 (1.2) | 4.9 (2.4) |
The pre- and post-fire fuel loadings (Mg ha−1) by fuel strata within mixed-conifer forests of California, USA. Total loadings includes living and dead tree boles, foliage, seedling, shrub, forbs, grass, 1- to 1000 h fuels, litter and duff. Standard errors are provided in parentheses. Categorical burn severity was derived from remote sensing severity cutoffs of relative difference normalised burn ratio (Miller and Thode 2007) and include, in order of increasing severity, ‘Unchanged’, ‘Low’, ‘Moderate’, and ‘High’.
Pre-fire relative humidity (µ = 31 ± 1.4% s.e.) did not vary significantly with burn severity category (Supplementary Appendix S1, Supplementary Fig. S3). Downed wood fuel moistures were uniformly low (µ < 12%) and also did not vary significantly by burn severity category (Supplementary Appendix S1, Supplementary Fig. S4).
Fuel consumption and relative change among satellite-based burn severity categories
Total fuel consumption averaged 131.2 ± 9.2 Mg ha−1 and was greater with increasing dNBR values (Table 3, Supplementary Appendix S1, Supplementary Fig. S5a). Overstorey tree foliage had low absolute consumption (2.9 ± 0.4 Mg ha−1) and was greater in high severity (4.8 ± 1.3 Mg ha−1) relative to unchanged severity (0.0 ± 0.0 Mg ha−1) plots. Standing dead tree fuels increased post-fire, with high severity plots having greater gains in standing dead tree fuels (33.5 ± 6.7 Mg ha−1) compared with unchanged severity (0.0 ± 0.0 Mg ha−1; Fig. 3b). Seedling, shrub, grass, and forb fuels decreased markedly for all burn severities (Fig. 3d–f, Table 3) but accounted for little of the total fuel consumption. Absolute consumption was high for 100- to 1000-h fuels but was similar across burn severity categories (Fig. 3g–i). Consumption of 1-h fuels was greater for high severity (1.2 ± 0.5 Mg ha−1) relative to unchanged areas (0.6 ± 0.3 Mg ha−1). Litter (18.8 ± 2.0 Mg ha−1) and duff (42.0 ± 3.7 Mg ha−1) consumption were similar across severity categories.
Absolute consumption (Mg ha−1) and relative change (%) were better explained by dNBR than by RdNBR (Supplementary Appendix S1, Supplementary Fig. S5). Relative change had a stronger relationship (R2 = 0.34) with dNBR than did absolute consumption (R2 = 0.14), a trend that was also present with RdNBR (R2 = 0.31 vs R2 = 0.06). Relative change varied significantly among burn severity categories for duff and seedling fuels (Fig. 4, Table 4) despite few differences in absolute consumption (Fig. 2). A majority of plots (66%) had incomplete consumption of either litter, duff, or both strata (Table 4, Supplementary Appendix S1, Supplementary Figs S6, S7). Post-fire available fuels (standing dead tree fuels, tree foliage, downed woody fuels, shrub, and herbaceous fuels, litter, and duff) were strongly related to pre-fire available fuels for low (R2 = 0.47; P < 0.001), moderate (R2 = 0.18; P = 0.006), and high (R2 = 0.25; P = 0.010) burn severity categories. No relationship between post-fire and pre-fire available fuels were found for the unchanged severity category (Fig. 5).
The proportional change (loss) of fuel loadings by burn severity and fuel strata, across 112 mixed-conifer forests in California, USA. Error bars represent ±1 standard error. (a) Change in seedling, shrub, grass and herbaceous fuels by burn severity. (b) Change in 1- to 1000-h fuels by burn severity. (c) The change in litter and duff fuels by severity. Different lowercase letters indicate significantly different values among categories. Non-significance across all categories is denoted as ‘ns’.
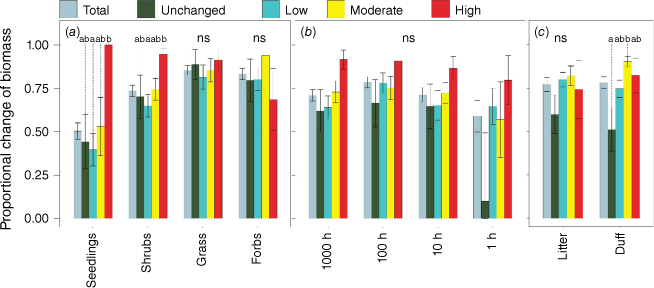
# of plots | Total | Live trees | Shrubs | Seedlings | Herbs | Grass | 1000-h | 100-h | 10-h | 1-h | Litter | Duff | |
---|---|---|---|---|---|---|---|---|---|---|---|---|---|
Total | 112 | 0.31 (0.02) | 0.26 (0.03) | 0.73 (0.03) | 0.50 (0.04) | 0.83 (0.03) | 0.85 (0.02) | 0.70 (0.03) | 0.78 (0.03) | 0.71 (0.04) | 0.58 (0.08) | 0.77 (0.04) | 0.78 (0.03) |
Unchanged | 13 | 0.18 (0.06) | 0.00 (0.00) | 0.70 (0.12) | 0.44 (0.15) | 0.79 (0.12) | 0.88 (0.08) | 0.62 (0.12) | 0.66 (0.13) | 0.64 (0.12) | 0.09 (0.39) | 0.59 (0.11) | 0.51 (0.12) |
Low | 44 | 0.22 (0.02) | 0.14 (0.04) | 0.64 (0.06) | 0.39 (0.09) | 0.80 (0.06) | 0.81 (0.06) | 0.63 (0.06) | 0.78 (0.05) | 0.65 (0.08) | 0.64 (0.10) | 0.79 (0.04) | 0.74 (0.06) |
Moderate | 34 | 0.38 (0.04) | 0.31 (0.05) | 0.74 (0.06) | 0.52 (0.16) | 0.93 (0.04) | 0.85 (0.06) | 0.72 (0.06) | 0.75 (0.06) | 0.72 (0.05) | 0.56 (0.21) | 0.82 (0.05) | 0.90 (0.03) |
High | 21 | 0.47 (0.06) | 0.61 (0.09) | 0.94 (0.03) | 1.0 (0.00) | 0.68 (0.10) | 0.91 (0.04) | 0.91 (0.05) | 0.90 (0.05) | 0.86 (0.06) | 0.79 (0.14) | 0.74 (0.16) | 0.82 (0.10) |
Standard errors are provided in parentheses. Categorical burn severity was derived from remote sensing severity cutoffs of relative difference normalised burn ratio (Miller and Thode 2007) and include, in order of increasing severity, ‘Unchanged’, ‘Low’, ‘Moderate’, and ‘High’.
The pre-fire available fuel (standing dead tree fuels, tree foliage, downed woody fuels, shrub and herbaceous fuels, litter, and duff; Mg ha−1; x-axis) and post-fire available fuels (Mg ha−1) for (a) unchanged, (b) low, (c) moderate, and (d) high severities for 112 California mixed-conifer forests, California, USA. The dashed diagonal line is a 1:1 line. Points above the 1:1 line indicate greater post-fire fuels from conversion of overstorey mortality or downed woody pools. Linear regression equations are labelled in each graph with significant relationships plotted with shaded confidence intervals.
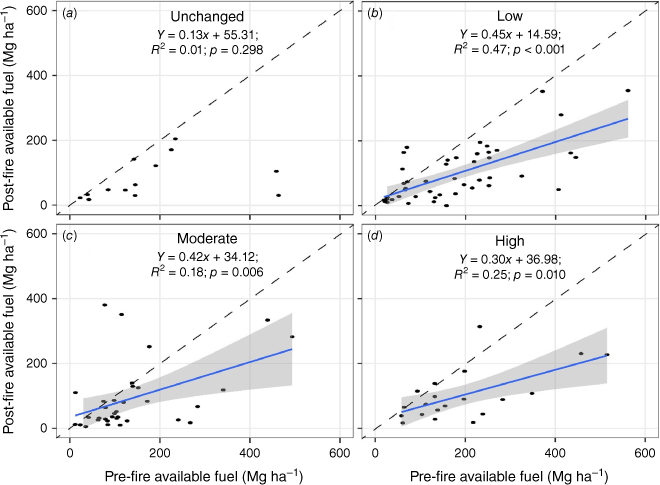
Relative and absolute fuel contributions to fire energy
Total fire energy (kJ m−2) increased with increasing continuous values of dNBR (y = 299.2x + 134 361.8; R2 = 0.13, P < 0.001), but not RdNBR (y = 152.5x + 159 768.3; R2 = 0.05, P = 0.10). This was in contrast with categorical burn severity where values were similar (Fig. 6a). Total fire energy was largely dominated by the high consumption of 1000-h fuels, litter, and duff (Fig. 6b). However, the absolute consumption of most fuel strata did not vary significantly among burn severity categories despite a trend of increasing consumption with higher burn severity (Fig. 2, Supplementary Appendix S1, Supplementary Fig. S5). Across the best-performing models (ΔAICc < 3), the most important variables for predicting total fire energy (RVI of 1.00) were pre-fire loadings of shrubs, 1000-h fuels, litter, and duff, as well as pre-fire standing dead tree loadings (RVI = 0.83). Total fire energy was also positively associated with higher relative humidity (RVI = 0.53), indicating higher fuel consumption with higher humidity (Table 5, Supplementary Appendix S1, Supplementary Fig. S8). Consumption of both 10-h and 1000-h fuels were negatively associated with fuel moisture.
(a) Distribution of fire energy (MJ m2) by burn severity classification. (b) Proportional contribution to fire energy by fuel strata from 112 mixed-conifer plots in California, USA. No significant differences (ns) exist between fire energy by categorical burn severity.
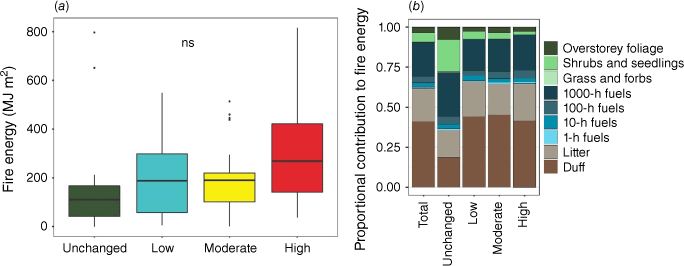
Variable | Estimate | Std. Error | Adjusted SE | z value | Pr(>|z|) | RVI |
---|---|---|---|---|---|---|
(Intercept) | 14 924.10 | 36 199.20 | 36 464.30 | 0.41 | 0.68 | NA |
Duff loadings | 1519.09 | 224.47 | 226.99 | 6.69 | P < 0.001 | 1.00 |
Litter loadings | 1868.54 | 385.18 | 389.60 | 4.80 | P < 0.001 | 1.00 |
Shrub loadings | 1208.06 | 419.01 | 423.92 | 2.85 | 0.004 | 1.00 |
1000-h fuel loadings | 1341.16 | 90.64 | 91.66 | 14.63 | P < 0.001 | 1.00 |
Standing snag loadings | 250.31 | 133.61 | 135.17 | 1.85 | 0.06 | 0.83 |
Relative humidity (%) | 936.27 | 592.66 | 598.85 | 1.56 | 0.12 | 0.53 |
100-h fuel loadings | 2747.37 | 1942.05 | 1965.00 | 1.40 | 0.16 | 0.42 |
Mid-flame windspeed (km h−1) | −9844.10 | 6517.23 | 6591.99 | 1.49 | 0.14 | 0.38 |
1000-h fuel moisture (%) | −4355.20 | 3692.44 | 3732.03 | 1.17 | 0.24 | 0.22 |
Grass loadings | −14 330.00 | 14 443.5 | 14 609.10 | 0.98 | 0.33 | 0.13 |
Live woody fuel moisture (%) | 248.65 | 258.88 | 261.49 | 0.95 | 0.34 | 0.12 |
10-h fuel moisture (%) | −2781.10 | 3013.14 | 3043.50 | 0.91 | 0.36 | 0.10 |
10-h fuel loadings | 3160.30 | 3458.19 | 3497.97 | 0.90 | 0.37 | 0.10 |
Seedling loadings | −327.97 | 370.07 | 374.35 | 0.88 | 0.38 | 0.08 |
Slope (%) | 254.44 | 454.49 | 459.76 | 0.55 | 0.58 | 0.05 |
McCune heatload | −26 493.00 | 53 414.70 | 54 048.90 | 0.49 | 0.62 | 0.04 |
1-h fuel loadings | 1932.75 | 8092.41 | 8184.08 | 0.24 | 0.81 | 0.03 |
Herbaceous loadings | −10 526.00 | 43 260.90 | 43 771.80 | 0.24 | 0.81 | 0.03 |
Live tree loadings | −2.13 | 33.82 | 34.22 | 0.06 | 0.95 | 0.03 |
The variable coefficients, significance, and relative variable importance (RVI) from the models with the lowest AIC and a ΔAICc of <3.0 that were averaged to create to estimate the drivers of total fire energy (kJ m2). All fuel variables (Mg ha−1) represent pre-fire conditions. Significant P-values and RVI values greater than 0.5 are bolded for clarity.
Flaming fire energy (derived from litter, seedling, shrub, grass, forb, and 1–100-h fuels) was similar among fire severity categories (Supplementary Appendix S1, Supplementary Fig. S9), with litter consumption the primary contributor (µ = 0.59 ± 0.02%). Across the best-performing models (ΔAICc < 3), the most important variables in predicting flaming fire energy were pre-fire loadings of shrubs, 100-h fuels, and litter (RVI = 1.0), along with 10-h fuels (RVI = 0.95) and standing dead tree fuel loadings (RVI = 0.65) (Supplementary Appendix S1, Supplementary Table S7).
Fuel consumption and fire energy among heading, backing, and flanking fires
Heading fires produced greater total fire energy than backing fires (P = 0.04) (Fig. 7a). Flaming fire energy showed no significant differences among fire advancement categories (Fig. 7b). The fuel differences in total fire energy between heading and backing fires were driven by greater litter (+212%; P = 0.002) and duff (+202%; P = 0.04) consumption relative to backing fires (Fig. 7c–d). No significant differences between direction of fire advancement and consumption were found for other fuels. There were no differences (P < 0.05) in pre-fire fuel loadings, moisture, or relative humidity among direction of fire advancement categories. Heading fires produced more complete consumption of litter (84 ± 6%; P < 0.001) and duff (89 ± 4% P < 0.001) than backing fires (69 ± 8% litter; 79 ± 5% duff; Supplementary Appendix S1, Supplementary Figs S10, S11). Flanking fires resulted in more complete consumption of litter (95 ± 2%; P < 0.001) and duff (97 ± 1%; P < 0.001) than backing fires and were similar to heading fires.
(a) Total and (b) flaming fire energy (MJ m2) and amount of (c) consumed litter and (d) consumed duff (Mg ha−1) under different wildfire behaviours in California, USA. Different lowercase letters indicate significant differences among categories. Fire energy was estimated from pre- and post-fire fuel consumption for (a) all fuel classes and from (b) fuel strata (litter, 1–100 h fuels, foliage, and vegetation) that are expected to drive the flaming phase of combustion.
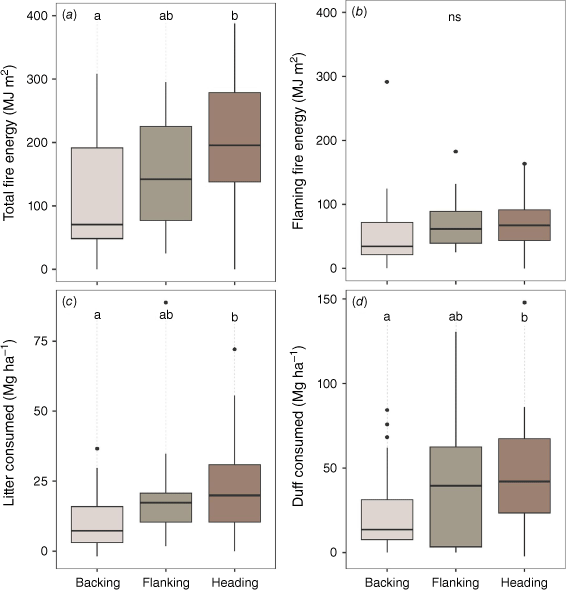
Discussion
In this study, we quantified the relative and absolute fuel consumption for wildfires in mixed-conifer forests and their contribution to fire energy for each fuel stratum and related these changes to remotely sensed burn severity and to direction of fire advancement.
Litter, duff, and 1000-h fuels are the primary contributors of fire energy
The amount of litter, duff, and 1000-h fuels overwhelmingly contributed to fire energy across all burn severity categories. Our consumption estimates are similar to those from others reporting high fuel consumption in these strata with wildfires in long-unburned forests (Lutz et al. 2020), which are 10–20% greater than the consumption percentages typical for prescribed burns (Vaillant et al. 2009; Levine et al. 2020). However, the similar absolute consumption of fuels across severity classes emphasises that satellite-derived burn severity metrics have limited ability to detect fuel consumption differences, likely due to their indirect connections with fine-scale surface and ground fuel consumption (Blomdahl et al. 2019). Indirect fire effects such as interactions between fire-induced tree injuries and post-fire environmental stressors, such as drought or bark beetles, may contribute to the weak relationship between remotely sensed fire severity and fuel consumption.
Although pre-fire fuel loadings were similar across all burn severity categories, the relative change indicates that 47–82% of aboveground fuels were not consumed and therefore could be consumed in subsequent fires. A mean total of 71% of unconsumed biomass was in live tree boles, which – at least in moderate to high severity wildfire – are likely to suffer substantial conversion from live to dead biomass pools (i.e. standing dead tree and downed woody debris) due to immediate and delayed mortality (Miesel et al. 2018; Lutz et al. 2020; Stephens et al. 2022). These dead wood fuels are then vulnerable to near-total loss during a later fire (Miesel et al. 2018). Additionally, incomplete combustion of at least one of the two litter and duff strata occurred in 74 plots (66%), with incomplete combustion of both litter and duff occurring in 44 (39%) of the 112 plots. Currently, fire consumption models such as BURNUP or FOFEM often assume high or complete combustion of litter and duff in wildfires (Lydersen et al. 2014; Lutes 2020) and may therefore overestimate wildfire emissions.
Our averaged model indicated that topography and fire weather had relatively little influence on total or flaming fire energy; this result aligns with other reports that fires in mixed-conifer forests of California are often fuel-limited (Parks et al. 2018), even though broad-scale filters, such as fire weather, can have important impacts (Kane et al. 2015a; Povak et al. 2020). The relationship between relative humidity and fire energy was weakly positive (R2 = 0.05) but identified as ‘important’ in our averaged model; this was surprising because higher relative humidity is intrinsically linked with higher fuel moisture and lower energy release, heats of combustion, spread rates, and fireline intensities (Van Wagner 1972; Johnson 1996). Although it is possible that slower spread rates could lead to more complete consumption by locally drying fuels, the variability in our dataset was quite high. Thus, we believe the weakly positive relationship was likely spurious or a result of confounding factors also varying with relative humidity. The relatively poor ability for fire weather variables to predict total fire energy may be influenced by a dataset weighted towards lower severity plots, as well as by the inability to accurately capture extreme weather conditions due to the inaccuracy of our hourly fire weather interpolations at higher wind speeds (Supplementary Appendix S1, Supplementary Fig. S2). It is likely that topography (Kane et al. 2015a, 2015b) or fire weather (Lydersen et al. 2017) may be more strongly associated with differences in fire energy than was represented in our results because these variables were not adequately characterised by FBAT measurements or are less important for low- and moderate-severity fires.
Fuel consumption poorly correlates with satellite remote sensing of burn severity
The poor performance of remote sensing metrics supports a body of literature, suggesting that these metrics are useful for some applications but insufficient for others, such as estimating fuel consumption (Murphy et al. 2008; Miesel et al. 2018; Szpakowski and Jensen 2019). Our results were consistent with other studies (Furniss et al. 2020a), indicating that dNBR had greater correlations for both the absolute and relative change in fuels than did RdNBR or categorical severities. Results confirmed our expectation that the relative dNBR (RdNBR) performed more poorly than dNBR in predicting absolute fuel consumption. Because remote sensing metrics are particularly sensitive to changes in photosynthetic vegetation and exposed mineral soil (van Wagtendonk et al. 2004), relatively large changes in dead woody fuels, litter, and duff – as observed in our study – may be undetected. In similar mixed-conifer forests, dNBR and RdNBR have more than 30% uncertainty in predicting basal area and stem mortality (Furniss et al. 2020a), highlighting the inherent difficulties in relating 30 × 30 m pixel scale burn severity to fine scale effects (Kolden et al. 2012; Blomdahl et al. 2019).
Our immediate sampling of fire effects identified relatively little overstorey mortality (Table 3), but tree mortality captured in remote sensing imagery may have occurred from time-lag mortality factors, which could be a combination of fuel consumption and other unmeasured factors such as post-fire drought, beetle attack, or pathogens (van Mantgem et al. 2018; Furniss et al. 2020b). The inherent variability in fuel consumption estimates and lack of clear relationships with categorial or continuous metrics of burn severity could be particularly relevant when attempting to accurately estimate carbon emissions from wildfires or predict long-term ecosystem effects (Stenzel et al. 2019). Continued development and implementation of new tools, such as hyperspectral imagery (Veraverbeke et al. 2018), airborne LiDAR (McCarley et al. 2020), or combinations of active and passive sensing may help address some of these issues and provide for better fuel mapping and consumption estimations from wildfire.
Heading fires consume more litter and duff than backing fires
Backing fires produced significantly lower total fire energy than heading fires, indicating important potential differences in fire effects and fire management considerations. Differences in fuel preconditioning or continuity of fire spread (i.e. less patchy spread) may explain the differences in greater consumption from heading fires, relative to backing fires, despite similar pre-fire fuel loadings. However, more research is needed to identify the mechanisms underlying the differences in fuel consumption.
These results may be important for forest management decisions because substantial duff consumption can cause mortality to large-diameter trees (Cansler et al. 2019), which are typically desirable to retain as live in mixed-conifer and other historically frequent-fire forests. Fire that occurs at relatively higher duff moisture content has greater propensity for incomplete or smouldering combustion (Frandsen 1987), which may help mitigate the risk of tree mortality (Varner et al. 2007). Backing fires may produce relatively lower rates of delayed overstorey tree mortality and associated loss of carbon sequestration potential, owing to the lower total fire energy compared with heading fires. In contrast, heading fires may be more likely to result in complete canopy scorching and mortality of large trees than backing fires. Additionally, heading fires will likely produce greater carbon emissions than backing fires and thus larger direct contributions to climate change. More long-term research is needed to describe how backing and heading fire effects alter long-term forest resilience and accumulation of new fuels. Our results suggest that forest management actions such as thinning or prescribed fires that target consuming or removing litter, duff, and 1000-h fuels would most directly reduce total fire energy, which may reduce the injury and mortality of overstorey trees.
Conclusion
Our study showed that litter, duff, and 1000-h fuels represented the primary contributors to wildfire energy, and that the consumption of litter and duff differ markedly with the direction of fire advancement. Managing wildfires to achieve more backing fire behaviour may limit effects on live trees that support the greatest aboveground biomass, and therefore carbon pools, and consume less of the fuels that contribute the most to fire energy. By reducing surface and ground fuels, such fire management strategies may help set the stage for prescribed fire treatments that more closely mimic historic fire regimes, thereby improving forest health and resilience and decreasing the risk of future catastrophic fire. Further research on heading and backing wildfires across a range of conditions may help parse the exact mechanisms by which heading fires consume greater amounts of litter and duff, and the consequences for long-term forest resilience.
Data availability
Data collected by the Fire Behaviour Assessment Team (FBAT) are publicly available through the frames.gov clearinghouse.
Acknowledgements
We thank the USFS Northern Research Station, Washington Office, and Pacific Southwest Region Fire and Aviation Management programs, as well as the Joint Fire Science Program for logistical and financial support for the Fire Behaviour Assessment Team since its establishment. Thanks to Dr. JoAnn Fites-Kauffman, Nicole Vaillant, Chelsea Morgan, and the many on-call FBAT team members for their contributions to wildfire operations and datasets. We appreciate the R Project and the many developers who support the statistical and processing packages. We thank Faith Ann Heinsch for assistance importing data into Fire Family Plus and calculating outputs, R. J. DeRose for equipment assistance, and Craig Baker for assistance calculating remote sensing severity metrics. This project was supported by the California Department of Forestry and Fire Protection (Grant number 8GG19804) as part of the California Climate Investments Program, and the McIntire-Stennis (Project MICL06033).
References
Barton K (2020) MuMIn: multi-model inference. R package version 1. 43.17. Available at http://r-forge.r-project.org/projects/mumin/
Blomdahl EM, Kolden CA, Meddens AJH, Lutz JA (2019) The importance of small fire refugia in the central Sierra Nevada, California, USA. Forest Ecology and Management 432, 1041-1052.
| Crossref | Google Scholar |
Cansler CA, Swanson ME, Furniss TJ, Larson AJ, Lutz JA (2019) Fuel dynamics after reintroduced fire in an old-growth Sierra Nevada mixed-conifer forest. Fire Ecology 15, 16.
| Crossref | Google Scholar |
Chojnacky DC, Heath LS, Jenkins JC (2014) Updated generalized biomass equations for North American tree species. Forestry 87, 129-151.
| Crossref | Google Scholar |
Conrad O, Bechtel B, Bock M, Dietrich H, Fischer E, Gerlitz L, Wehberg J, Wichmann V, Böhner J (2015) System for automated geoscientific analyses (SAGA) v. 2.1. 4. Geoscientific Model Development 8, 1991-2007.
| Crossref | Google Scholar |
Cousins SJM, Battles JJ, Sanders JE, York RA (2015) Decay patterns and carbon density of standing dead trees in California mixed conifer forests. Forest Ecology and Management 353, 136-147.
| Crossref | Google Scholar |
Davies SJ, Abiem I, Abu Salim K, Aguilar S, Allen D, Alonso A, Anderson-Teixeira K, Andrade A, Arellano G, Ashton PS, Baker PJ, Baker ME, Baltzer JL, Basset Y, Bissiengou P, Bohlman S, Bourg NA, Brockelman WY, Bunyavejchewin S, Burslem D, Cao M, Cárdenas D, Chang LW, Chang-Yang CH, Chao KJ, Chao WC, Chapman H, Chen YY, Chisholm RA, Chu C, Chuyong G, Clay K, Comita LS, Condit R, Cordell S, Dattaraja HS, de Oliveira AA, den Ouden J, Detto M, Dick C, Du X, Duque Á, Ediriweera S, Ellis EC, Obiang N, Esufali S, Ewango C, Fernando ES, Filip J, Fischer GA, Foster R, Giambelluca T, Giardina C, Gilbert GS, Gonzalez-Akre E, Gunatilleke I, Gunatilleke C, Hao Z, Hau B, He F, Ni H, Howe RW, Hubbell SP, Huth A, Inman-Narahari F, Itoh A, Janík D, Jansen PA, Jiang M, Johnson DJ, Jones FA, Kanzaki M, Kenfack D, Kiratiprayoon S, Král K, Krizel L, Lao S, Larson AJ, Li Y, Li X, Litton CM, Liu Y, Liu S, Lum S, Luskin MS, Lutz JA, Luu HT, Ma K, Makana JR, Malhi Y, Martin A, McCarthy C, McMahon SM, McShea WJ, Memiaghe H, Mi X, Mitre D, Mohamad M, Monks L, Muller-Landau HC, Musili PM, Myers JA, Nathalang A, Ngo KM, Norden N, Novotny V, O’Brien MJ, Orwig D, Ostertag R, Papathanassiou K, Parker GG, Pérez R, Perfecto I, Phillips RP, Pongpattananurak N, Pretzsch H, Ren H, Reynolds G, Rodriguez LJ, Russo SE, Sack L, Sang W, Shue J, Singh A, Song GM, Sukumar R, Sun IF, Suresh HS, Swenson NG, Tan S, Thomas SC, Thomas D, Thompson J, Turner BL, Uowolo A, Uriarte M, Valencia R, Vandermeer J, Vicentini A, Visser M, Vrska T, Wang X, Wang X, Weiblen GD, Whitfeld T, Wolf A, Wright SJ, Xu H, Yao TL, Yap SL, Ye W, Yu M, Zhang M, Zhu D, Zhu L, Zimmerman JK, Zuleta D (2021) ForestGEO: Understanding forest diversity and dynamics through a global observatory network. Biological Conservation 253, 108907.
| Crossref | Google Scholar |
De Groot WJ, Pritchard JM, Lynham TJ (2009) Forest floor fuel consumption and carbon emissions in Canadian boreal forest fires. Canadian Journal of Forest Research 39, 367-382.
| Crossref | Google Scholar |
Eidenshink J, Schwind B, Brewer K, Zhu ZL, Quayle B, Howard S (2007) A project for monitoring trends in burn severity. Fire Ecology 3(1), 3-21.
| Crossref | Google Scholar |
Evans JS, Ram K (2015) Package ‘spatialEco’. R Package. Version 1.3.7. Available at https://cran.r-project.org/web/packages/spatialEco/index.html
Fahnestock GR, Hare RC (1964) Heating of tree trunks in surface fires. Journal of Forestry 62(11), 799-805.
| Crossref | Google Scholar |
FBAT (2022) ‘Fire Behavior Assessment Team measurement protocols.’ (United States Department of Agriculture) Available at https://www.frames.gov/documents/fbat/resources/FBAT_Protocols_1August2022.pdf [accessed 13 March]
Fillmore SD, McCaffrey SM, Smith AMS (2021) A mixed methods literature review and framework for decision factors that may influence the utilization of managed wildfire on federal lands, USA. Fire 4(3), 62.
| Crossref | Google Scholar |
Frandsen WH (1987) The influence of moisture and mineral soil on the combustion limits of smoldering forest duff. Canadian Journal of Forest Research 17, 1540-1544.
| Crossref | Google Scholar |
Furniss TJ, Kane VR, Larson AJ, Lutz JA (2020a) Detecting tree mortality with Landsat-derived spectral indices: Improving ecological accuracy by examining uncertainty. Remote Sensing of Environment 237, 111497.
| Crossref | Google Scholar |
Furniss TJ, Larson AJ, Kane VR, Lutz JA (2020b) Wildfire and drought moderate the spatial elements of tree mortality. Ecosphere 11(8), e03214.
| Crossref | Google Scholar |
Hagmann RK, Hessburg PF, Prichard SJ, Povak NA, Brown PM, Fulé PZ, Keane RE, Knapp EE, Lydersen JM, Metlen KL, Reilly MJ, Sánchez Meador AJ, Stephens SL, Stevens JT, Taylor AH, Yocom LL, Battaglia MA, Churchill DJ, Daniels LD, Falk DA, Henson P, Johnston JD, Krawchuk MA, Levine CR, Meigs GW, Merschel AG, North MP, Safford HD, Swetnam TW, Waltz A (2021) Evidence for widespread changes in the structure, composition, and fire regimes of western North American forests. Ecological Applications 31, e02431.
| Crossref | Google Scholar |
Horel JD, Dong X (2010) An evaluation of the distribution of remote automated weather stations (RAWS). Journal of Applied Meteorology and Climatology 49, 1563-1578.
| Crossref | Google Scholar |
Jenkins JC, Chojnacky DC, Heath LS, Birdsey RA (2003) National-scale biomass estimators for United States tree species. Forest Science 49, 12-35.
| Crossref | Google Scholar |
Jeronimo SMA, Lutz JA, Kane VR, Larson AJ, Franklin JF (2020) Burn weather and three-dimensional fuel structure determine post-fire tree mortality. Landscape Ecology 35, 859-878.
| Crossref | Google Scholar |
Kane VR, Cansler CA, Povak NA, Kane JT, McGaughey RJ, Lutz JA, Churchill DJ, North MP (2015a) Mixed severity fire effects within the Rim fire: relative importance of local climate, fire weather, topography, and forest structure. Forest Ecology and Management 358, 62-79.
| Crossref | Google Scholar |
Kane VR, Lutz JA, Cansler CA, Povak NA, Churchill DJ, Smith DF, Kane JT, North MP (2015b) Water balance and topography predict fire and forest structure patterns. Forest Ecology and Management 338, 1-13.
| Crossref | Google Scholar |
Kassambara A (2020) ggpubr: ‘ggplot2’ based publication ready plots. R Package. Version 0.2.5. Available at https://cran.r-project.org/web/packages/ggpubr/index.html
Keeley JE, Safford H, Fotheringham CJ, Franklin J, Moritz M (2009) The 2007 southern California wildfires: lessons in complexity. Journal of Forestry 107(6), 287-296.
| Crossref | Google Scholar |
Knapp EE, Varner JM, Busse MD, Skinner CN, Shestak CJ (2011) Behaviour and effects of prescribed fire in masticated fuelbeds. International Journal of Wildland Fire 20(8), 932-945.
| Crossref | Google Scholar |
Kolden CA, Lutz JA, Key CH, Kane JT, van Wagtendonk JW (2012) Mapped versus actual burned area within wildfire perimeters: characterizing the unburned. Forest Ecology and Management 286, 38-47.
| Crossref | Google Scholar |
Levine JI, Collins BM, York RA, Foster DE, Fry DL, Stephens SL (2020) Forest stand and site characteristics influence fuel consumption in repeat prescribed burns. International Journal of Wildland Fire 29, 148-159.
| Crossref | Google Scholar |
Lutz JA, Larson AJ, Swanson ME, Freund JA (2012) Ecological importance of large-diameter trees in a temperate mixed-conifer forest. PLoS One 7, e36131.
| Crossref | Google Scholar |
Lutz JA, Furniss TJ, Germain SJ, Becker KML, Blomdahl EM, Jeronimo SMA, Cansler CA, Freund JA, Swanson ME, Larson AJ (2017) Shrub communities, spatial patterns, and shrub-mediated tree mortality following reintroduced fire in Yosemite National Park, California, USA. Fire Ecology 13, 104-126.
| Crossref | Google Scholar |
Lutz JA, Struckman S, Furniss TJ, Cansler CA, Germain SJ, Yocom LL, McAvoy DJ, Kolden CA, Smith AMS, Swanson ME, Larson AJ (2020) Large-diameter trees dominate snag and surface biomass following reintroduced fire. Ecological Processes 9, 41.
| Crossref | Google Scholar |
Lydersen JM, Collins BM, Ewell CM, Reiner AL, Fites JA, Dow CB, Gonzalez P, Saah DS, Battles JJ (2014) Using field data to assess model predictions of surface and ground fuel consumption by wildfire in coniferous forests of California. Journal of Geophysical Research: Biogeosciences 119(3), 223-235.
| Crossref | Google Scholar |
Lydersen JM, Collins BM, Brooks ML, Matchett JR, Shive KL, Povak NA, Kane VR, Smith DF (2017) Evidence of fuels management and fire weather influencing fire severity in an extreme fire event. Ecological Applications 27, 2013-2030.
| Crossref | Google Scholar |
McCarley TR, Hudak AT, Sparks AM, Vaillant NM, Meddens AJH, Trader L, Mauro F, Kreitler J, Boschetti L (2020) Estimating wildfire fuel consumption with multitemporal airborne laser scanning data and demonstrating linkage with MODIS-derived fire radiative energy. Remote Sensing of Environment 251(2020), 112114.
| Crossref | Google Scholar |
McCune B (2007) Improved estimates of incident radiation and heat load using non‐parametric regression against topographic variables. Journal of Vegetation Science 18, 751-754.
| Crossref | Google Scholar |
McIntyre PJ, Thorne JH, Dolanc CR, Flint AL, Flint LE, Kelly M, Ackerly DD (2015) Twentieth-century shifts in forest structure in California: denser forests, smaller trees, and increased dominance of oaks. Proceedings of the National Academy of Sciences 112, 1458-1463.
| Crossref | Google Scholar |
McRae DJ, Conard SG, Ivanova GA, Sukhinin AI, Baker SP, Samsonov YN, Blake TW, Ivanov VA, Ivanov AV, Churkina TV, Hao WM, Koutzenogij KP, Kovaleva N (2006) Variability of fire behavior, fire effects, and emissions in Scotch pine forests of Central Siberia. Mitigation and Adaptation Strategies for Global Change 11, 45-74.
| Crossref | Google Scholar |
Miesel J, Reiner A, Ewell C, Maestrini B, Dickinson M (2018) Quantifying changes in total and pyrogenic carbon stocks across fire severity gradients using active wildfire incidents. Frontiers in Earth Science 6, 41.
| Crossref | Google Scholar |
Miller JD, Thode AE (2007) Quantifying burn severity in a heterogeneous landscape with a relative version of the delta Normalized Burn Ratio (dNBR). Remote Sensing of Environment 109, 66-80.
| Crossref | Google Scholar |
Murphy KA, Reynolds JH, Koltun JM (2008) Evaluating the ability of the differenced Normalized Burn Ratio (dNBR) to predict ecologically significant burn severity in Alaskan boreal forests. International Journal of Wildland Fire 17, 490-499.
| Crossref | Google Scholar |
Parks SA, Holsinger LM, Panunto MH, Jolly WM, Dobrowski SZ, Dillon GK (2018) High-severity fire: evaluating its key drivers and mapping its probability across western US forests. Environmental Research Letters 13, 044037.
| Crossref | Google Scholar |
Parsons DJ, DeBenedetti SH (1979) Impact of fire suppression on a mixed-conifer forest. Forest Ecology and Management 2, 21-33.
| Crossref | Google Scholar |
Piqué M, Obon B, Condés S, Saura S (2011) Comparison of relascope and fixed-radius plots for the estimation of forest stand variables in northeast Spain: an inventory simulation approach. European Journal of Forest Research 130, 851-859.
| Crossref | Google Scholar |
Povak NA, Kane VR, Collins BM, Lydersen JM, Kane JT (2020) Multi-scaled drivers of severity patterns vary across land ownerships for the 2013 Rim Fire, California. Landscape Ecology 35, 293-318.
| Crossref | Google Scholar |
Schloerke B, Cook D, Larmarange J, Briatte F, Marbach M, Thoen E, Elberg A, Crowley J (2020) GGally: Extension to ‘ggplot2’. R Package. Version 1.5.0. Available at https://cran.r-project.org/web/packages/GGally/index.html
Skowronski NS, Gallagher MR, Warner TA (2020) Decomposing the interactions between fire severity and canopy fuel structure using multi-temporal, active, and passive remote sensing approaches. Fire 3(1), 7.
| Crossref | Google Scholar |
Stenzel JE, Bartowitz KJ, Hartman MD, Lutz JA, Kolden CA, Smith AMS, Law BE, Swanson ME, Larson AJ, Parton WJ, Hudiburg TW (2019) Fixing a snag in carbon emissions estimates from wildfires. Global Change Biology 25, 3985-3994.
| Crossref | Google Scholar |
Stephens SL, Bernal AA, Collins BM, Finney MA, Lautenberger C, Saah D (2022) Mass fire behavior created by extensive tree mortality and high tree density not predicted by operational fire behavior models in the southern Sierra Nevada. Forest Ecology and Management 518, 120258.
| Crossref | Google Scholar |
Szpakowski DM, Jensen JL (2019) A review of the applications of remote sensing in fire ecology. Remote Sensing 11, 2638.
| Crossref | Google Scholar |
United States Geological Survey (2020) ‘USGS National Elevation Dataset.’ (U.S. Department of the Interior) Available at https://apps.nationalmap.gov/viewer/ [accessed 10 November 2020]
Vaillant NM, Fites-Kaufman J, Reiner AL, Noonan-Wright EK, Dailey SN (2009) Effect of fuel treatments on fuels and potential fire behavior in California, USA, national forests. Fire Ecology 5, 14-29.
| Crossref | Google Scholar |
van Mantgem PJ, Stephenson NL, Byrne JC, Daniels LD, Franklin JF, Fulé PZ, Harmon ME, Larson AJ, Smith JM, Taylor AH, Veblen TT (2009) Widespread increase of tree mortality rates in the western United States. Science 323, 521-524.
| Crossref | Google Scholar |
van Mantgem PJ, Falk DA, Williams EC, Das AJ, Stephenson NL (2018) Pre‐fire drought and competition mediate post‐fire conifer mortality in western US National Parks. Ecological Applications 28(7), 1730-1739.
| Crossref | Google Scholar |
van Wagtendonk JW, Benedict JM, Sydoriak WM (1998) Fuel bed characteristics of Sierra Nevada conifers. Western Journal of Applied Forestry 13, 73-84.
| Crossref | Google Scholar |
van Wagtendonk JW, Root RR, Key CH (2004) Comparison of AVIRIS and Landsat ETM+ detection capabilities for burn severity. Remote Sensing of Environment 92, 397-408.
| Crossref | Google Scholar |
van Wagtendonk JW, Moore PE, Yee JL, Lutz JA (2020) The distribution of woody species in relation to climate and fire in Yosemite National Park, California, USA. Fire Ecology 16, 22.
| Crossref | Google Scholar |
Varner JM, Hiers JK, Ottmar RD, Gordon DR, Putz FE, Wade DD (2007) Overstory tree mortality resulting from reintroducing fire to long-unburned longleaf pine forests: the importance of duff moisture. Canadian Journal of Forest Research 37, 1349-1358.
| Crossref | Google Scholar |
Veraverbeke S, Dennison P, Gitas I, Hulley G, Kalashnikova O, Katagis T, Kuai L, Meng R, Roberts D, Stavros N (2018) Hyperspectral remote sensing of fire: State-of-the-art and future perspectives. Remote Sensing of Environment 216, 105-121.
| Crossref | Google Scholar |
Westerling AL, Hidalgo HG, Cayan DR, Swetnam TW (2006) Warming and earlier spring increase western US forest wildfire activity. Science 313, 940-943.
| Crossref | Google Scholar |
Young DJN, Meyer M, Estes B, Gross S, Wuenschel A, Restaino C, Safford HD (2020) Forest recovery following extreme drought in California, USA: natural patterns and effects of pre‐drought management. Ecological Applications 30, e02002.
| Crossref | Google Scholar |
Ziegler JP, Hoffman CM, Mell W (2019) firebehavioR: An R Package for Fire Behavior and Danger Analysis. Fire 2, 41.
| Crossref | Google Scholar |