Avoid getting burned: lessons from the McKinley wildfire in rural Alaska, USA
Jennifer I. Schmidt
A
B
Abstract
Climate change and continued development in the wildland–urban interface (WUI) have increased risks to property and infrastructure from destructive wildfires.
A better understanding of the factors associated with building survival will promote resilience of WUI communities.
We studied factors associated with the likelihood that a building burned during the 2019 McKinley fire in the Alaska boreal forest, USA. We examined the potential influence of both ecological or socio-economic factors on building loss.
The probability of a building burning was significantly associated (P < 0.001) with a building burning nearby (within 30 m). Having less flammable deciduous cover nearby (within 100 m) improved survival. Buildings with lower value on larger parcels were more likely to burn, as were buildings with larger perimeters. Other important factors associated with burning included the number of buildings both nearby (within 30 m) and within the property parcel boundary.
Our results suggest that social and ecological factors contribute to building survival, indicating that a comprehensive social-ecological approach would provide the most effective support to WUI communities with wildfire risks.
A comprehensive approach that integrates social, economic, and ecological factors is important in understanding building loss in WUI wildfires.
Keywords: Alaska, Arctic, decision making, mitigation, planning, risk, SES, socio-economic, wildland fire, WUI.
Introduction
Increased wildfire activity fuelled by climate change and human activities, including continued development into forested areas, is contributing to a growing number of severe and catastrophic wildfires in the wildland–urban interface (WUI) (Hammer et al. 2009; Moritz et al. 2012; Radeloff et al. 2018; Haynes et al. 2019). As the number of lives, homes, and property lost to wildfire has increased (Karter 2010a; Haynes et al. 2019; Badger 2021), many communities and governments are responding to the increased risk by urging the use of wildfire safety standards (US Government 2016), and promoting Firewise USA (NFPA 2018) or FireSmart in Canada (FireSmart 2018). Meanwhile, insurance companies have stopped offering new and cancelling existing policies (Adriano 2023; Blood 2023). The current state of affairs stresses the need to learn more about what homeowners and communities can do to reduce wildfire risk and prevent property destruction and loss of life.
Research on wildfires in smaller communities (<2500 residents) within the WUI is scarce (Bar Massada et al. 2009; Dye et al. 2021) despite these areas facing a higher incidence of fires (Karter 2010b). Existing studies often address larger communities (Gibbons et al. 2012; Syphard et al. 2012, 2013; Alexandre et al. 2016; Gibbons et al. 2018; Knapp et al. 2021). In contrast, our research focuses on a specific wildfire that destroyed 139 buildings in the relatively sparsely populated WUI between two communities with fewer than 2500 residents (US Census Bureau 2020). Previous research on smaller communities focused on modelling and simulations and did not explore spatial auto-correlation (Bar Massada et al. 2009; Dye et al. 2021). Strategies for mitigating wildfire risks in sparsely populated regions may differ due to limited suppression resources, lower incomes, and the challenge of engaging residents who may be wary of government involvement (Jacobs and Cramer 2020). Additionally, these areas may experience future development. Collaborating with homeowners, developers, and local governments to plan for wildfire-adapted communities is essential for mitigating risks to life and property (Moritz et al. 2014; Schoennagel et al. 2017). Producing actionable research findings can inform smart planning and reduce the negative consequences of wildfires on society (Syphard et al. 2013).
Promoting resilience to wildfires involves understanding the WUI as a socio-ecological system (SES) where societal and ecological elements interact (Chapin et al. 2006; Moritz et al. 2014; Steelman 2016). Social attributes include building design, land use, risk perception, and suppression efforts, while ecological characteristics encompass the type of ecosystem, fire history, and weather. Activities like vegetation manipulation and fuel reduction have both social and ecological effects. Understanding WUI SESs can enhance safety and reduce property loss (Lampin-Maillet et al. 2010; Galiana-Martin et al. 2011). The best strategies are likely to include a combination of social and ecological actions (Moritz et al. 2022). Our unique study analyses social, economic, and ecological factors that predict which buildings burn and which survive during wildfires, emphasising the importance of combined socio-ecological actions for effective strategies.
Previous research on building survival in WUI fires typically focused on specific aspects of the SES, often combining ecological features with building spatial arrangement or land management. (Gibbons et al. 2012; Syphard et al. 2013; Alexandre et al. 2016; Syphard and Keeley 2019). However, no empirical studies have comprehensively examined a broad range of social-ecological parameters simultaneously, and few have explored the issue of spatial scale (Alexandre et al. 2016; Syphard and Keeley 2019). Context-specific and place-based approaches are crucial, given the diverse SES combinations in the WUI. Despite its extensive wildfire history and WUI development, no research has investigated building loss in Alaska’s boreal forest. Our research addresses three critical gaps: (1) enhancing understanding of building loss in boreal forest ecosystems; (2) evaluating SES characteristics influencing building loss at multiple scales; and (3) discerning factors that may affect building loss differently in sparsely populated WUI areas. By exploring various socio-ecological factors, we aim to contribute insights for promoting wildfire-adapted communities in boreal forest regions.
Materials and methods
Study area
The McKinley wildfire started on 17 August 2019, during one of the hottest and driest summers on record in south-central Alaska (AICC 2019; Bhatt et al. 2021) and was believed to be caused by human activity. Driven by high winds, the fire moved rapidly, burning 1331 ha (3288 acres) over 3 days, destroying 52 residential buildings, 84 outbuildings, and three commercial buildings. In total, 1028 properties were affected, and approximately 350–400 people were evacuated (McDonald 2019; Zak 2019).
The McKinley wildfire swept from north to south along the George Parks Highway and the Alaska Railroad, shutting down the only direct surface transportation links between Alaska’s two main urban centres, Anchorage and Fairbanks (Fig. 1, inset, lower right). Although the area receives heavy use from travellers and recreationists, it is sparsely populated. Based on building density, this area does not meet the housing density threshold of one house per 40 acres (16.2 ha) to classify it as a wildland–urban interface (WUI) (USDA and USDOI 2001). However, it is managed as WUI in Alaska and falls under the highest wildfire suppression category (AKDFFP 2023). The area affected by the fire contains no incorporated communities but lies within the Matanuska-Susitna Borough (MSB), a large (65,420 km2) county-level government that provides limited public services financed through local property taxes. About 70% of the area within the fire perimeter was undeveloped in 2019, but the 118 parcels, averaging 3 ha (7.5 acres) per parcel, contained buildings. Developed parcels, including homes, recreational cabins, and a few commercial establishments serving travellers, were scattered in clusters near the highway.
Location of burned and unburned buildings along with (a) 100-m hazardous fuels, (b) vegetation used to create hazardous fuels, and (c) burn severity within the 2019 McKinley wildfire perimeter. En. growth means enhanced growth after fire with low to moderate burn severity.
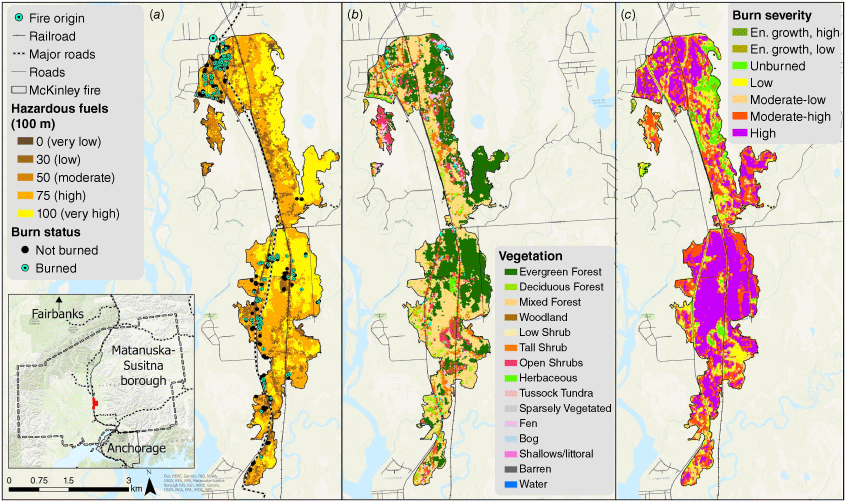
Although much of the boreal forest where the McKinley widlfire burned remains in the surrounding unoccupied parcels, there are occupied portions where buildings are closely spaced (<30 m), and the forest has been thinned and cleared. Pre-fire aerial imagery shows that many landowners retained deciduous trees while clearing evergreens. Boreal forests are a fire-driven ecosystem with highly flammable black and white spruce (Picea), and their natural fire return intervals range from 75 to >100 years (Hoecker et al. 2020). However, this interval has been shortening (Flannigan et al. 2009; Kasischke et al. 2010). Lightning-ignited fires typically begin in mid-June and are suppressed naturally by rains in August (Bieniek et al. 2012). Even though lightning-caused wildfires burn the most area, most ignitions in Alaska, especially in the WUI, are caused by humans (DeWilde and Chapin 2006; Calef et al. 2017). Human-caused fires are more likely to occur outside of a typical wildfire season than lightning-caused ones (Calef et al. 2017), though the wildfire season has been expanding due to climate change (Grabinski and McFarland 2020).
Data sources
Building burn status
The MSB created a damage assessment database from reports by property owners after the wildfire that documented physical addresses receiving building damage due to the McKinley wildfire. However, not all residents participated in this process, so we also used aerial imagery before (2017, 0.3 m) and after (2020, 0.3 m) the wildfire to determine which buildings were lost during the wildfire (MSB 2023). We linked areal building images to the spatial buildings outline database obtained from MSB (MSB 2021). Buildings present in the pre-fire aerial imagery but absent in post-fire imagery were digitised and classified as burned. Building outlines were also removed if they were not visible at either time point. Buildings appearing only in the post-fire imagery were excluded from the analysis.
Explanatory variables
We used a variety of social and ecological variables to determine why some buildings burned and others survived (Table 1). The goal was to include variables capturing SES aspects based on available data. For detailed information about the variables, see Supplementary Appendices S1 and S2.
Explanatory variable | Theme | Type | Data source | |
---|---|---|---|---|
Parcel lot size | Social | Building | MSB A | |
Mobile home on the property | Social | Building | MSB A | |
Building size | Social | Building | MSB A | |
Building wall surface area length | Social | Building | MSB A | |
Land value | Social | Value | MSB A | |
Building value | Social | Value | MSB A | |
Number of residential units | Social | Land use | MSB A | |
Commercial building | Social | Land use | MSB A | |
Total buildings on parcel | Social | Land use | MSB | |
Junkyard on the property, adjacent to property (yes/no) | Social | Land use | Aerial photography | |
Distance to nearest building (m) | Social | Land use | Aerial photography | |
Building within 10, 30, 100 m buffer around the building (yes/no) | Social | Land use | Aerial photography | |
Number of buildings within 30 m buffer around the building | Social | Land use | Aerial photography | |
Number of neighbouring parcels within 10, 30, 100 m buffer around the building | Social | Land use | MSB A | |
Distance to nearest burnt building (m) | Social/Ecological | Land use/Fire | Aerial photography | |
Burned building within 30 m | Social/Ecological | Land use/Fire | Aerial photography | |
Distance to fire starting point (m) | Ecological | Fire | Georeferenced photo | |
Within fire perimeter on day 1 (yes/no) | Ecological | Fire | VIIRS B | |
Average burn severity for the parcel (dNBR) | Ecological | Fire | Sentinel-2 C | |
Average burn severity within a 30 m buffer | Ecological | Fire | Sentinel-2 C | |
Average burn severity in 10, 30, and 500 m buffer, excluding building footprint (dNBR) | Ecological | Fire | Sentinel-2 C | |
Percent of tree cover within 100 m (%) | Ecological | Vegetation | Aerial photography/GIS | |
Undetectable vegetation within 10 m building (yes/no) | Ecological | Vegetation | Aerial photography/GIS | |
Average or maximum 100 m or 500 m hazardous vegetation value within 30, 100, and 500 m buffer | Ecological | Hazard | ABoVE D | |
Average merged wildfire exposure value for the parcel | Ecological | Exposure | ABoVE D | |
Average or maximum 100, 500 m, or merged wildfire exposure value within 30, 100, 500 m buffer | Ecological | Exposure | ABoVE D | |
Average merged wildfire exposure within the four cardinal quadrants of 100 m buffer around the building | Ecological | Exposure | ABoVE (30 m) |
Building and property characteristics can be important for understanding loss due to wildfire (Syphard and Keeley 2019; Knapp et al. 2021; Pierce et al. 2022). We consider characteristics of the built environment social variables, as they reflect people’s income, personal preferences, and land use rules. Information on the characteristics of the parcels and buildings came primarily from the property appraisal records maintained by the MSB to assess properties for tax purposes. Alaska requires local governments to appraise property at full market value (AS 29.45.110). However, taxable assessed values may be less than appraised if properties qualify for one or more exemptions under state and local statutes (AS 29.45.010). Information available in the appraisal records is limited, as building permits are not required outside incorporated cities in the MSB. Data included parcel size, number and type of buildings, floor area of the main building, appraised value of land, and total building value on the property. The building wall surface length was calculated by taking the square root of the building size (ft2).
The spatial arrangement of buildings was explored by calculating the distance to the nearest building and the presence or absence of a building within 30 and 100 m (Alexandre et al. 2016; Knapp et al. 2021). Development density was assessed by summing the number of buildings and parcels within 10, 30, and 100 m buffers. Discussions with firefighters revealed that unkempt properties (i.e. junkyards) could hinder suppression efforts. Therefore, pre-fire aerial imagery was used to identify which buildings occurred on parcels with junkyards and adjacent parcels with junkyards.
Previous research found that the distance and time from the start of a fire might influence building loss (Maranghides et al. 2013). The starting location of the McKinley wildfire was Mile 91 on the George Parks Highway. This location was digitised, and then the Euclidean distance from the fire start to each building was calculated. We used Visible Infrared Imaging Radiometer Suite remote sensed data (VIIRIS I-Band 375 m (NASA 2023)) to determine which buildings were reached by the fire on the first day. Burn severity can also be an important factor for explaining building loss; we used remote sensed Sentinel-2 (10 m, Copernicus S2 (ESA 2020)) to calculate the Normalised Burn Ratio (NBR) before (July 2019) and after the wildfire (July 2020) and then calculated the differenced NBR (dNBR), which is the pre-fire NBR minus the post-fire NBR. To ensure that our remote sensed dNBR accurately captured burn severity, we also conducted 61 composite-based index (CBI) plots to assess burn severity on the ground (Appendix S3). Average burn severity was calculated for each parcel and assigned to the buildings on that parcel. Average burn severity was calculated within 10, 30, 100, and 500 m buffers of the building footprint, including and excluding the building footprint. This was done because research shows that buildings can be fuel (Knapp et al. 2021); thus, we wanted to explore whether the inclusion of the building affected measured burn severity.
Vegetation cover and type can influence the survival of buildings during a wildfire (Alexandre et al. 2016; Gibbons et al. 2018). In summer, deciduous trees tend to have higher moisture content in their leaves than spruce needles, and they may extinguish embers (Wilson and Ralph 1985) and influence the spread of fire (Rothermel 1972). The presence of green vegetation has been associated with building loss (Gibbons et al. 2018; Knapp et al. 2021). To capture the amount of green cover around buildings, we generated a grid of hexagons with 10 m buffer (2 m side length), 30 m (4 m side length), and 100 m (12.5 m side length). The hexagon was classified as green if the deciduous tree cover was observed. We then determined the total percent of green hexagons around each building at various scales. Given the promotion and success of Firewise principles (NFPA 2018), we classified buildings as being ‘Firewise’ if they had undetectable vegetation within 10 m or 30 m. Few buildings had no visible vegetation within 100 m.
In Alaska, we used a new wildfire exposure approach (Schmidt et al. 2024) modified from previous research in the Canadian boreal forest (Beverly et al. 2010, 2021). Exposure reflects the potential that a wildfire will get close enough to impact a location. Areas with higher exposure will be more exposed to intense wildfire activity than areas with lower exposure. This approach used a land cover layer with 15 cover types (Wang et al. 2019), reclassified into a flammability hazard rating based on wildfire torching, spotting, and spread potential of each cover type. High hazard fuels include tall woody vegetation (>3 m) such as evergreen species while lower hazard fuels are water-soaked areas like bogs and littorals and sparsely vegetated areas. Moderate fuels are shrubs and grasses, which are typically green in summer. Focal statistics were used in ArcGIS Pro to calculate the average flammability hazard rating within a 100 and 500 m circle, which reflect short and long-distance ember dispersal, respectively. The products are two wildfire exposure layers (100 and 500 m). The merged wildfire exposure layer was created by taking the maximum from the 100 and 500 m within a 500-m buffer of buildings and outside the buffer using the 500 m. Average and maximum hazardous vegetation scores and wildfire exposure were also calculated at different scales within 30, 100, and 500 m buffers around buildings. The McKinley wildfire spread from north to south. Directionality of hazards can be important (Gibbons et al. 2012; Syphard et al. 2012), so we calculated the average exposure in four quadrants: (1) northern (315°–45°); (2) eastern (45°–135°); (3) southern (135°–225°); and (4) western (225°–315°).
A complete list of the variables used for this analysis and data sources is in Supplementary Appendix S1. Several parameters were derived from each data source, which are explained below. Some parameters were also calculated at various scales. We typically used scales of 10, 30, and 100 m to capture the distances used to explore human ignition zones around buildings and Firewise and FireSmart principles (Syphard et al. 2012, 2014; Alexandre et al. 2016; FireSmart 2018; NFPA 2018). Longer-distance ember dispersal and dynamics were explored at 500 m (Beverly et al. 2010; Alexandre et al. 2016; Gibbons et al. 2018). The scales have also been used in other research on the distance embers travel, allowing fire to move across the landscape (Bierwagen 2005; Cohen 2008; Beverly et al. 2010; Syphard et al. 2014; Alexandre et al. 2016).
Statistical methods
We modelled the probability that building i burned, bi, generally as a function of ecological variables affecting wildfire risk, xi, and social characteristics of property parcels and buildings, zi:
Since wildfire tends to spread spatially outward, the error term, ui, may contain spatial auto-correlation. We considered both spatial errors, where the error term ui includes a random error ɛi and a correlation parameter ρ times a weighted sum of nearby error terms, and spatial lags, where ui contains ɛi plus a correlation parameter λ times a weighted sum of nearby burn probabilities, bj: (Anselin 1988):
where dijj represents the inverse distance between building i and building j. Observations represent binary outcomes, burned vs unburned buildings, rather than probabilities. Given the nature of the errors for the binary outcome, we started by specifying Eqn 1 as logistic regression:
with constant α, and parameter vectors β and γ estimated via maximum likelihood. Since the theory of spatial correlation is incompletely developed for logistic regression, we also estimated Eqn 1 using a linear probability model, testing residuals for spatial correlation using the standard Moran global I statistic (Moran 1950). If the Moran test indicated significant spatial correlation, we estimated spatially auto-correlated linear regression equations adjusting for spatial lag and spatial error in ui (Drukker et al. 2013b):
where ui is given by Eqn 2 and ɛi ~ (N(0, σ2In).
The inclusion of the dependent variable in the spatially correlated error term implies a potential for feedback from an individual building i’s burn status to the probability that neighbouring building j burned. The feedback makes it difficult to infer the effects on structural survival from the estimated coefficients of Eqn 4 alone. To address the full effects of the diverse ecological and social attributes, we estimated total effect (LeSage and Pace 2009), which is the sum of two components: the direct effect of the parcel’s attributes on building i’s survival, βxi, +γzi, and the indirect effects, which measure the feedback effect coming from nearby buildings included in the error term ui.
Intense wildfire may exhibit locally chaotic behaviour, as it generates its own local weather. Evidence of the chaotic component may show up as random variation in observed burn severity. The noise of this inherently unpredictable component of burn severity may obscure the signals coming from the surrounding vegetation and property development characteristics that are more helpful to inform policy and planning to reduce wildfire risk to buildings. A simple strategy for keeping the focus on potentially policy-relevant characteristics is to ignore the burned status of neighbouring buildings and include the presence or absence of nearby buildings, regardless of their burn status. A more complex approach would be to estimate the degree to which burn status of a building might be correlated with the component of neighbourhood burn severity that is contributed by the characteristics of the local vegetation and built environment. To implement the latter strategy, we estimated an equation that estimates burn severity without the influence of surrounding buildings (x0i) as a linear combination of exogenous ecological and development variables, xi and zi:
and ωi is a normally distributed random error term.
We then estimated a version of Eqn 4 correcting for potential spatial elation, replacing measured burn severity with predicted burn severity, δxi, +μzi from Eqn 5, using generalised spatial two-stage least squares instrumental variable regression (Drukker et al. 2013a).
The large number of potential explanatory variables for the small case study population requires a strategy for eliminating variables that are not associated with risk to buildings. Since the literature on wildfire in Alaska boreal forest ecosystems does not provide guidance for which spatial scale is most relevant for each particular characteristic of the site, we constructed ecological and some social variables with spatial buffers at varying scales. Measures of the same characteristic at different spatial scales are highly correlated. To determine the relevant set of explanatory variables, we tested potential effects with stepwise entry and removal, using P < 0.2 as a criterion for retention. For each characteristic that could be measured at multiple spatial scales, we examined spatial effects by starting with the smallest spatial scale for which a characteristic could be measured reliably. Then, we expanded to the next largest spatial scale. If a given characteristic met the P < 0.2 criterion at any spatial scale, we retained the one spatial scale for that characteristic that provided the best fit. We estimated variance inflation factors (VIF) to evaluate multi-collinearity among the remaining variable set. Strong winds spread the fire from north to south during the 2-day period when the fire burned out of control through the area, so we tested whether effects on the north side of buffers differed from effects coming from other directions.
After obtaining the preliminary set of regressors with the stepwise procedure, we tested the potential significance of every excluded variable again, including the different spatial scales, by entering the excluded variable again into the equation and testing for P < 0.2.
Results
Results from logistic regression (Eqn 4) estimated for the odds ratio that a building burned show that a nearby burned building was the most salient predictor of a building burning, followed by the 100-m exposure index measured within the 100-m building radius (Column 1 of Table 2). Vegetation cover within 100 m was negatively associated with a burned outcome, suggesting that vegetation such as deciduous trees may mitigate the effect of more flammable evergreen trees associated with higher exposure values. Burn severity, using the measure that attempted to exclude the building footprint, was also positively associated with burning, although the correlation is weaker (P = 0.057). Using the alternative measurement of burn severity that included all cells within the 30-m buffer provided a more significant correlation with burned status (P = 0.048); however, we cannot rule out that the variable was biased by, in some instances, measuring the burning building’s contribution to burn severity.
(1) | (2) | (3) | (4) | (5) | ||
---|---|---|---|---|---|---|
Equation | Eqn 3 | Eqn 3 | Eqns 2, 4 | Eqn 5 | Eqns 2, 4, 5 | |
Dependent variable, specification | Probabilty that a building burned, controlling for nearby burned buildings | Probability that a building burned, ignoring burn status of nearby buildings | Probability that a building burned, same as Column 2 adjusting for spatial auto-correlation | Burn severity without the influence of buildings | Probability that a building burned, including burn severity without the influence of buildings | |
Estimation method | Logistic regression | Logistic regression | Spatial GLS regression | Least squares regression | Spatial IV GLS regression | |
Average burn severity in a 30 m buffer, excluding building footprint | 0.00282 (1.90)* | 0.00537 (4.48)*** | 0.000721 (3.49)*** | |||
Predicted average burn severity in 30 m buffer, excluding building footprint | 0.000620 (2.27)** | |||||
Percent of tree cover within 100 m | −0.0411 (−2.91)*** | −0.0503 (−4.53)*** | −0.00700 (−3.79)*** | 1.500 (2.76)*** | −0.00619 (−3.66)*** | |
Undetectable vegetation within 10 m of building | −42.3 (−1.87) | |||||
Average 100 m wildfire exposure value within 100 m buffer | 0.0567 (2.96)*** | 0.0396 (2.67)*** | 0.00696 (2.54)** | 0.00587 (2.38)** | ||
Maximum 100 m wildfire exposure value within a 100 m buffer | 2.90 (2.88)*** | |||||
Average 100 m hazardous vegetation value within a 30 m buffer | 2.12 (2.19)** | |||||
Maximum 500 m hazardous vegetation value within a 100 m buffer | −2.87 (−4.03)*** | |||||
Distance to fire starting point | −0.0101 (−4.71)*** | |||||
Building value (000s) | −0.0105 (−2.74)*** | −0.0135 (−4.64)*** | −0.00200 (−4.23)*** | −0.00210 (−5.24)*** | ||
Total buildings on parcel | 0.551 (2.50)** | 0.737 (4.21)*** | 0.112 (3.34)*** | 0.116 (4.04)*** | ||
Building wall perimeter | 0.0364 (1.88)* | 0.0179 (1.30) | 0.0391 (1.83)* | 0.00387 (2.06)** | ||
Parcel lot size (acres) | −0.0128 (−1.45) | −0.0182 (−2.31)*** | −0.00232 (−1.87)* | |||
Burned building within 30 m | 3.75 (9.77)*** | |||||
Building within 30 m | 0.743 (2.33)** | 0.105 (1.98)** | −22.7 (−1.43) | 0.108 (1.91)* | ||
Number of buildings within 30 m | 0.260 (2.79)*** | −16.0 (−3.60)*** | ||||
Constant term | −1.51 (−1.61) | −3.15 (−3.62)*** | −0.202 (−1.31) | 295 (5.55)*** | −0.0725 (−0.51) | |
Spatial lag (λ) | 0.882 (4.18)*** | 1.12 (7.05)*** | ||||
Spatial error (ρ) | 0.676 (1.53) | |||||
Observations | 325 | 325 | 325 | 325 | 325 | |
Log likelihood | −113.0 | −173.5 | −168.2 | |||
Wald/F | 223.8*** | 102.8*** | 61.45*** | 30.31*** | 52.09*** | |
d.f. | 8 | 9 | 8 | 8, 316 | 8 | |
R2/Pseudo R2 | 0.498 | 0.229 | 0.286 | 0.43 | 0.255 |
A negative sign indicates an increased probability of building survival. Figures enclosed in parentheses below coefficients represent coefficient z or t statistics.
*P < 0.10
**P < 0.05
***P < 0.001
Higher-valued buildings were more likely to survive, but controlling for value, larger buildings with more surface area were more prone to burning. Multiple buildings on the property reduced survival odds. These findings might be influenced by firefighting decisions favouring valuable properties and ensuring their safety. A junkyard near the building was not a significant predictor of building loss.
Although the wind came from the north on the day of the fire, burn severity on that side, or any side, of a building was not as good a predictor as burn severity around the entire building, suggesting that radiative heat was more likely to drive building ignition than wind-driven flames.
Significant variables appeared to be relatively robust with respect to the different specifications of Eqn 1. The parallel linear regression (Eqn 5, results not shown) showed very similar results. Multi-collinearity among remaining explanatory variables in Eqn 5 was modest (VIF mean 1.56, maximum of 2.15). The Moran global I test reported no significant spatial auto-correlation (χ2 = 0.257, P = 0.61). Including a nearby burned building in the list of explanatory variables essentially specifies a spatial lag model, complicating attribution. Column 2 of Table 2 shows results from estimating Eqn 3 but replacing a burned building as an explanatory variable with any building, burned or not, within the 30-m buffer. These results showed that nearby buildings were a highly significant predictor of the risk of loss, although the equation explained less than half as much of the likelihood of a building burning. Without the direct spatial lag in the equation, the Moran I test reported that the residuals were highly spatially correlated (χ2 = 39.47, P < 0.001).
The high degree of spatial auto-correlation in the equation could theoretically be due to the fact that the nearby building is a risk factor for direct ignition, implying a spatial lag model, or that the spatial scale of the ecological risk; i.e. exposure to radiative heat from burning vegetation is larger than the distance between buildings, generating a spatial error. The results of estimating Eqn 5 with both a spatial lag and spatial error terms, displayed in Column 3 of Table 2, show a highly significant spatial lag (λ) (P < 0.001), but the spatial error was not significant (ρ) (P = 0.13). One should note that the ecological variables are constructed from gridded data by drawing spatial buffers ranging from 30 to 100 m around the building. The data, therefore, build in and account for spatial error in the form of contiguity matrices with distances to neighbours corresponding to buffer radii in the variable definitions.
We do not have measurements of fire intensity at the relevant scales; however, burn severity within 30 m of the building footprint represents evidence for fire intensity and is, not surprisingly, a positive and significant explanatory variable for the probability that the building survives the wildfire. It is possible that a burning building may have ignited the surrounding vegetation and, therefore, increased the fire intensity in nearby vegetation. We addressed that potential feedback by estimating Eqn 5 as a spatially auto-correlated equation with burn intensity as an endogenous variable (Drukker et al. 2013a). In this case, the spatial error was not significant (P = 0.2), so we show the results in Column 5 of Table 2, which includes only the spatial lag (λ). The equation shown in Column 5 includes the predicted values of the equation for burn severity without the influence of buildings from the equation shown in Column 4 rather than the measured values of burn severity. The results are very similar to those of Column 3. The only real difference is that the coefficient for burn severity has a larger standard error.
The equation for burn severity (Column 4 of Table 2) reveals additional factors relevant to building survival that were not visible directly but appear to affect building survival indirectly through their impact on burn severity. First, the nearby building has a negative sign (although not statistically significant, P = 0.15). These results suggest that a building within 30 m ignites nearby buildings with radiant heat rather than by igniting vegetation. Although many of the same vegetation characteristics are the same as those directly affecting survival, they are significant at smaller spatial scales. Additional significant factors associated with burn severity include distance to the fire start location and absence of vegetation in the immediate 10 m around the building. The negative coefficient on the distance to the fire start location likely indicates the ramping up of the fire suppression effort, including aerial water drops to reduce the intensity (Gabbert 2019). The lack of observed vegetation around the building represents efforts of homeowners to protect the building by clearing brush near the building. Although clearing brush around the house did not make the P = 0.2 cut-off for inclusion in the equations for building survival, it was marginally significant in reducing fire intensity around the home (P = 0.07).
The way the effects from the variety of social and ecological variables interact to predict the probability of a structure burning, after adjusting for spatial auto-correlation, is spatially complex. Fig. 2 illustrates two important contributing factors that interact to predict burn probability: (1) social, the spatial clustering of buildings; and (2) ecological, the exposure. The figure overlays the estimated probability of burning and actual burn status with wildfire exposure, an important contributing factor to building ignition directly, as well as indirectly through its impact on burn severity. The estimated probability of burning, calculated from the coefficients in Column 5 of Table 2 is higher in areas with high exposure values (lighter colours). Supplementary Appendix S4 shows the logistic curve fit between predicted burn probabilities and burn status.
Location of buildings, with predicted probability of burning (dot interior colour) and burn status (dot boundary colour), overlaid on wildfire exposure. Predicted probability of burning is derived from coefficients in Column 5 of Table 2. Lighter exposure colours represent higher exposure.
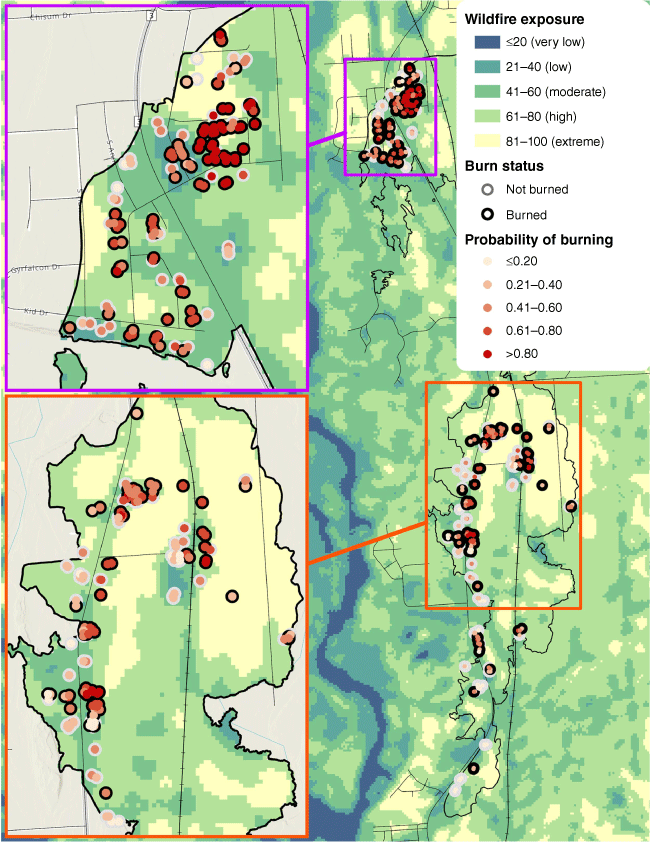
As described in the Materials and Methods section, the full effect of each explanatory variable in the regression equation with spatial auto-correlation includes a direct effect and an indirect effect (LeSage and Pace 2009). The direct effect represents the effect of a characteristic of a given building on that building’s burn probability. That same characteristic also affects the potential ignition of nearby buildings. That effect coming through ignition from nearby buildings is included in the indirect effects. The total effect is the sum of direct and indirect effects. Table 3 shows the direct, indirect, and total effects of a one-unit change in each characteristic derived from the equation in Column 3 of Table 2. The results show that indirect effects are broadly similar to the direct effects, although somewhat smaller. The total effects are generally more statistically significant than the regression coefficients in this case. The pattern of burned buildings is spatially clustered, reflecting the spatial clustering of development in the region (Fig. 2). However, the characteristics of those buildings within the burn perimeter of the McKinley wildfire are locally spatially diverse. Some properties contain homes with non-residential outbuildings, while neighbours may include only a single building; small recreational cabins may be adjacent to large homes, commercial buildings, or mobile homes. Such diversity at local spatial scales causes the spatial errors to be uncorrelated with the spatial lags, contributing to the lack of difference between the relative strength of direct and indirect effects.
Explanatory variable | Direct effect (z statistic) | Indirect effect (z statistic) | Total effect (z statistic) | |
---|---|---|---|---|
Burn severity (30 m) | 0.000729 (3.51)*** | 0.000565 (2.73)*** | 0.00129 (4.41)*** | |
Percent of tree cover within 100 m | −0.00707 (−3.80)*** | −0.00548 (−2.73)*** | −0.0125 (−4.58)*** | |
Average 100 m wildfire exposure value within 100 m buffer | 0.00703 (2.55)** | 0.00545 (2.11)** | 0.0125 (3.31)*** | |
Value of improvements (000s) | −0.00202 (−4.23)*** | −0.00158 (−2.80)*** | −0.00360 (−4.87)*** | |
Total buildings on parcel | 0.113 (3.35)*** | 0.0873 (2.35)** | 0.200 (3.99)*** | |
Building wall surface area length | 0.0395 (1.84)* | 0.0306 (1.39) | 0.0702 (2.28)** | |
Parcel lot size (acres) | −0.00234 (−1.88)* | −0.00182 (−1.60) | −0.00416 (−2.47)** | |
Number of buildings within 30 m | 0.106 (1.99)** | 0.0825 (1.82)* | 0.189 (2.70)*** |
A negative sign indicates an increased probability of building survival. Figures in parentheses below coefficients represent coefficient z or t statistics.
*P < 0.10.
**P < 0.05.
***P < 0.001.
Fig. 3 illustrates the relative magnitude of the direct and indirect effects of the various ecological and social characteristics. They show the effect of a 1% change in each characteristic on building survival. The direct effect represents the effect of that characteristic on the probability that buildings burn. In contrast, the indirect effect represents the effect of the characteristic of a building on the sum of the changes in probabilities of loss of nearby buildings, considering the effect of the building burning on the loss of its neighbours. For example, Fig. 3 indicates that a 1% increase in burn severity around a building increases the probability that it burns by about 0.3%, and it increases the sum of the changes in probabilities that neighbouring buildings burn by 0.28%. Among all the significant predictors of building loss, the exposure index has the largest percentage effect, with a 1% increase associated with a direct effect of about 0.4% and an indirect effect of about 0.35%.
The relative direct and indirect effects of different explanatory factors in the spatial regression equation for the probability that a building burned are derived from Table 3 and measured as the effect of a 1% increase in each explanatory variable.
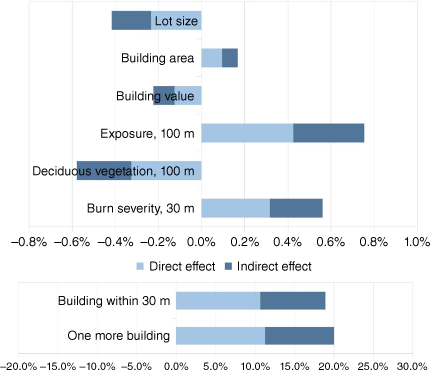
The bottom panel of Fig. 3 shows that adding one more building to a parcel increases the probability of burning by 20%. Additionally, placing another building within 30 m of another adds a 10% increase in burn probability for each additional building. The mean number of nearby buildings is relatively small (2.32). The indirect effect (i.e. the sum effects on all the other nearby buildings) is only a little smaller than the direct effect.
Discussion
Statistical results consistently showed that the presence of nearby buildings significantly predicted building loss. Additionally, the indirect effects of a building on its neighbours were nearly as important as the direct effects (Table 3 and Fig. 3). These findings support the view that neighbourhood efforts are part of the solution to improving wildfire preparedness and reducing vulnerability (Cohen 2008; Paveglio et al. 2012). Despite the rapid spread of the McKinley wildfire, the absence of fatalities in this rural area indicates the effectiveness of community networks. Post-wildfire discussions revealed the use of communication networks for evacuation, emphasising their importance for adaptive capacity (Byrd and Schmidt 2020; Schmidt 2020). Paveglio et al. (2016a) and Lambrou et al. (2023) showed that neighbourhood efforts and good communication are important for adaptive capacity toward wildfire. Steffey et al. (2020) also noted that community groups, communication networks, and local firefighters play vital roles in shaping attitudes and reducing building vulnerability in rural areas. Bringing like-minded individuals together as a collective group with positive risk reduction attitudes has been shown to reduce building loss to wildfire in these areas (Lucas et al. 2022).
Our results underscore the significance of policy-related factors such as zoning, lot size, and building codes. Building spacing and density have been identified as important factors influencing building vulnerability in other studies (Alexandre et al. 2016; Knapp et al. 2021; Mockrin et al. 2023). Despite rural areas being inherently sparsely populated, clustering occurs, and our findings indicate that placing buildings within 30 m of each other increases vulnerability. When buildings are in lower densities, the risk depends on the exposure to hazardous vegetation surrounding the buildings, not how far they are from another house. Crafting policies that balance affordable housing needs with wildfire risk reduction is a top priority for many WUI communities.
Information about the exterior characteristics of buildings, such as roofing materials and siding used in Alaska, which might explain more of the variation in ignition (see Meldrum et al. 2022), was unfortunately unavailable for the study and is a relevant topic for future research. Even with our limited data, building characteristics such as value and age are more important than demographic characteristics (Paveglio et al. 2016b, 2018). Better construction may help explain why more expensive homes were less likely to burn. However, it could also be that firefighting crews prioritised saving more expensive homes during suppression efforts.
Wildfire impact on buildings depends on the fire’s ability to reach them, influenced by factors like flammable vegetation and fire intensity. Wildfire exposure measures how capable the flammability hazards surrounding a specific location are to reach and impact that location. A 10% increase in wildfire exposure values at the 100-m scale (short-distance ember dispersal) within 100 m of a building resulted in nearly a 4% increase in the probability of building loss, both for the building itself (directly) and for nearby buildings (indirectly; Fig. 3). Burn severity, rather than the direction of fire spread, highlighted the increased vulnerability generated by flammable vegetation near homes. Practices like Firewise and Firesmart suggest removal of flammable vegetation, which homeowners may avoid undertaking, thinking it implies a total loss of vegetation (Nelson et al. 2005; Paveglio and Kelly 2018). However, this drastic approach is neither necessary nor in alignment with Firewise. Like others (Gibbons et al. 2018), our results show that leaving deciduous trees on the property (although not abutting the house) can decrease loss.
Diverse weather conditions, terrain, vegetation, and suppression actions all contribute to variability in individual wildfires (Finney 2005; Carmel et al. 2009). Previous studies that linked vegetation characteristics to building loss emphasised the role of weather on wildfire characteristics (Finney et al. 2010; Maranghides et al. 2013; Prichard and Kennedy 2014; Stevens et al. 2014; Johnson et al. 2019). Weather conditions varied little during the brief 2-day period when the McKinley wildfire spread uncontained, and the relatively flat terrain precluded consideration of topographical variations (Haire and McGarigal 2009).
The McKinley wildfire underscores the need to reassess WUI definitions (Radeloff et al. 2005, 2018; Carlson et al. 2022). The minimum development threshold for the WUI definition poses challenges for Alaska and rural areas, where people and property remain at risk, despite lower development densities (Cottrell 2005). The formulaic approach to defining the WUI may exclude these areas from mitigation funding (CWSF 2023; FEMA 2023). Small communities or states with limited resources may struggle to determine effective approaches. Collaboration between academia, agencies, and communities in community-driven research to understand vulnerability would promote wildfire-adapted communities.
Conclusion
This research adds to the growing body of work highlighting the complexity of building vulnerability to wildfire in the WUI. The statistical findings on building loss provide policy suggestions to reduce wildfire vulnerability in the boreal forest region and potentially globally in the WUI, especially in areas with relatively low population density. Significantly, the spatial arrangement of buildings underscores the importance of land-use codes, such as building setbacks, in mitigating wildfire vulnerability during initial development stages. Fundamental Firewise and FireSmart principles, including vegetation management around buildings and on the property level, remain crucial, even in forest-dominated areas. Recommendations include avoiding buildings within 30 m of another building to prevent radiant heat spread and discouraging construction in high wildfire exposure areas. If buildings are constructed in such areas, fuel management actions to clear flammable vegetation to reduce wildfire exposure would decrease building loss during wildfires.
Our findings emphasise the importance of a comprehensive SES approach that integrates social and ecological factors to understand building loss to wildfire in the WUI. As (Calkin et al. 2023) noted, WUI fires are not solely a wildfire problem but are closely tied to buildings and their surroundings. We found that wildfire may still spread from building to building in sparsely populated areas. Storage sheds and other outbuildings, popular in more sparsely populated areas where self-sufficiency and identity are valued, pose a risk to residences. Instead of creating WUI building codes, which may face resistance, empowering people through knowledge and awareness initiatives, such as outreach at home builder conferences or outdoor symposiums/shows, may be more effective in reducing wildfire risk by making residents aware of the hazards from outbuildings near their homes.
Many WUI residents, especially in Alaska, enjoy living in the forest and value the ecosystem services they provide (Hansen and Naughton 2013; Little et al. 2018). Work in Alaska showed that shaded and thinned fuel treatments were more acceptable than cleared treatments (Hansen and Naughton 2013; Little et al. 2018). Our finding that deciduous trees reduce the likelihood of building loss provides a potential pathway for living with forests in a fire-safe manner. Property owners should still heed Firewise recommendations regarding the placement of trees and removal of debris, but this could be an acceptable trade-off for mitigating wildfire risk.
Data availability
After acceptance, the dataset used for the modelling will be archived with the NSF Arctic data centre.
Declaration of funding
Funding for this work was provided by the US National Science Foundation, Award No. 1927563/1927537: NNA: Arctic Urban Risks and Adaptations (AURA): a co-production framework for addressing multiple changing environmental hazards and the Alaska EPSCoR and the state of Alaska provided funds for this project (NSF award #OIA- 1757348).
Author contributions
J.S.: Conceptualisation, methodology, data collection and curation, writing. M.B.: Methodology, formal analysis, and writing. C.W.: Conceptualisation, methodology.
References
Adriano L (2023) Farmers Insurance continues to cancel policies due to wildfire risk. Insurance Business. Available at https://www.insurancebusinessmag.com/us/news/breaking-news/farmers-insurance-continues-to-cancel-policies-due-to-wildfire-risk-436573.aspx
AICC (2019) ‘Fire season weather summary 2019.’ (Alaska Interagency Coordination Center) Available at https://fire.ak.blm.gov/content/Weather%20Folder/Fire%20Season%20Summaries/2019%20Fire%20Season.pdf
AKDFFP (2023) Fire management plans. Available at https://www.forestry.alaska.gov/fire/fireplans
Alexandre PM, Stewart SI, Mockrin MH, Keuler NS, Syphard AD, Bar-Massada A, Clayton MK, Radeloff VC (2016) The relative impacts of vegetation, topography and spatial arrangement on building loss to wildfires in case studies of California and Colorado. Landscape Ecology 31, 415-430.
| Crossref | Google Scholar |
Bar Massada A, Radeloff VC, Stewart SI, Hawbaker TJ (2009) Wildfire risk in the wildland-urban interface: a simulation study in northwestern Wisconsin. Forest Ecology and Management 258, 1990-1999.
| Crossref | Google Scholar |
Beverly JL, Bothwell P, Conner JCR, Herd EPK (2010) Assessing the exposure of the built environment to potential ignition sources generated from vegetative fuel. International Journal of Wildland Fire 19, 299-313.
| Crossref | Google Scholar |
Beverly JL, McLoughlin N, Chapman E (2021) A simple metric of landscape fire exposure. Landscape Ecology 36, 785-801.
| Crossref | Google Scholar |
Bhatt US, Lader RT, Walsh JE, Bieniek PA, Thoman R, Berman M, Borries-Strigle C, Bulock K, Chriest J, Hahn M, Hendricks AS, Jandt R, Little J, McEvoy D, Moore C, Rupp TS, Schmidt J, Stevens E, Strader H, Waigl C, White J, York A, Ziel R (2021) Emerging anthropogenic influences on the southcentral Alaska temperature and precipitation extremes and related fires in 2019. Land 10, 82.
| Crossref | Google Scholar |
Bieniek PA, Bhatt US, Thoman RL, Angeloff H, Partain J, Papineau J, Fritsch F, Holloway E, Walsh JE, Daly C, Shulski M, Hufford G, Hill DF, Calos S, Gens R (2012) Climate divisions for Alaska based on objective methods. Journal of Applied Meteorology and Climatology 51, 1276-1289.
| Crossref | Google Scholar |
Bierwagen BG (2005) Predicting ecological connectivity in urbanizing landscapes. Environment and Planning B-Planning & Design 32, 763-776.
| Google Scholar |
Byrd A, Schmidt JI (2020) The McKinley Fire: Rebuilding and Lessons Learned. Available at https://www.youtube.com/watch?v=no8dvnjbdHY
Calef MP, Varvak A, McGuire AD (2017) Differences in Human versus Lightning Fires between Urban and Rural Areas of the Boreal Forest in Interior Alaska. Forests 8, 422.
| Crossref | Google Scholar |
Calkin DE, Barrett K, Cohen JD, Finney MA, Pyne SJ, Quarles SL (2023) Wildland-urban fire disasters aren’t actually a wildfire problem. Proceedings of the National Academy of Sciences 120, e2315797120.
| Crossref | Google Scholar | PubMed |
Carlson AR, Helmers DP, Hawbaker TJ, Mockrin MH, Radeloff VC (2022) The wildland–urban interface in the United States based on 125 million building locations. Ecological Applications 32, e2597.
| Crossref | Google Scholar | PubMed |
Carmel Y, Paz S, Jahashan F, Shoshany M (2009) Assessing fire risk using Monte Carlo simulations of fire spread. Forest Ecology and Management 257, 370-377.
| Crossref | Google Scholar |
Chapin FS, III, Robards MD, Huntington HP, Johnstone JF, Trainor SF, Kofinas GP, Ruess RW, Fresco N, Natcher DC, Naylor RL (2006) Directional changes in ecological communities and social‐ecological systems: a framework for prediction based on Alaskan examples. The American Naturalist 168, S36-S49.
| Crossref | Google Scholar |
Cohen JD (2008) The wildland-urban interface fire problem. Forest History Today 20-26 https://research.fs.usda.gov/treesearch/33787.
| Google Scholar |
Cottrell A (2005) Communities and bushfire hazard in Australia: more questions than answers. Global Environmental Change Part B: Environmental Hazards 6, 109-114.
| Crossref | Google Scholar |
CWSF (2023) Wildand Urban Interface Grant Program. Available at https://www.westernforesters.org/wui-grants
DeWilde L, Chapin FS (2006) Human impacts on the fire regime of interior Alaska: Interactions among fuels, ignition sources, and fire suppression. Ecosystems 9, 1342-1353.
| Crossref | Google Scholar |
Drukker DM, Prucha IR, Raciborski R (2013a) A command for estimating spatial-autoregressive models with spatial-autoregressive disturbances and additional endogenous variables. Stata Journal 13, 287-301.
| Crossref | Google Scholar |
Drukker DM, Prucha IR, Raciborski R (2013b) Maximum likelihood and generalized spatial two-stage least-squares estimators for a spatial-autoregressive model with spatial-autoregressive disturbances. Stata Journal 13, 221-241.
| Crossref | Google Scholar |
Dye AW, Kim JB, McEvoy A, Fang F, Riley KL (2021) Evaluating rural Pacific Northwest towns for wildfire evacuation vulnerability. Natural Hazards 107, 911-935.
| Crossref | Google Scholar |
ESA (2020) Overview of Sentinel-2 Mission. Available at https://sentiwiki.copernicus.eu/web/s2-mission
FEMA (2023) Wildfire and the Wildand Urban Interface (WUI). Available at https://www.usfa.fema.gov/wui/
Finney MA (2005) The challenge of quantitative risk analysis for wildland fire. Forest Ecology and Management 211, 97-108.
| Crossref | Google Scholar |
Finney MA, Cohen JD, Grenfell IC, Yedinak KM (2010) An examination of fire spread thresholds in discontinuous fuel beds. International Journal of Wildland Fire 19, 163-170.
| Crossref | Google Scholar |
FireSmart (2018) About FireSmart Canada. Available at https://firesmartcanada.ca/about-firesmart/
Flannigan M, Stocks B, Turetsky M, Wotton M (2009) Impacts of climate change on fire activity and fire management in the circumboreal forest. Global Change Biology 15, 549-560.
| Crossref | Google Scholar |
Gabbert B (2019) McKinley Fire burns 50 structures south of Talkeetna, Alaska. Wildfire Today. August 19. Available at https://wildfiretoday.com/2019/08/19/mckinley-fire-burns-50-structures-south-of-talkeetna-alaska/
Galiana-Martin L, Herrero G, Solana J (2011) A wildland-urban interface typology for forest fire risk management in Mediterranean areas. Landscape Research 36, 151-171.
| Crossref | Google Scholar |
Gibbons P, van Bommel L, Gill AM, Cary GJ, Driscoll DA, Bradstock RA, Knight E, Moritz MA, Stephens SL, Lindenmayer DB (2012) Land management practices associated with house loss in wildfires. Plos One 7, 29212.
| Crossref | Google Scholar | PubMed |
Gibbons P, Gill AM, Shore N, Moritz MA, Dovers S, Cary GJ (2018) Options for reducing house-losses during wildfires without clearing trees and shrubs. Landscape and Urban Planning 174, 10-17.
| Crossref | Google Scholar |
Haire SL, McGarigal K (2009) Changes in fire severity across gradients of climate, fire size, and topography: a landscape ecological perspective. Fire Ecology 5, 86-103.
| Crossref | Google Scholar |
Hammer RB, Stewart SI, Radeloff VC (2009) Demographic trends, the wildland-urban interface, and wildfire management. Society & Natural Resources 22, 777-782.
| Crossref | Google Scholar |
Hansen WD, Naughton HT (2013) The effects of a spruce bark beetle outbreak and wildfires on property values in the wildland-urban interface of south-central Alaska, USA. Ecological Economics 96, 141-154.
| Crossref | Google Scholar |
Hoecker TJ, Higuera PE, Kelly R, Hu FS (2020) Arctic and boreal paleofire records reveal drivers of fire activity and departures from Holocene variability. Ecology 101, 3096.
| Crossref | Google Scholar | PubMed |
Jacobs DB, Cramer LA (2020) The relationships between social capital and concerns for climate change with increasing wildfire risks in rural communities in Central Oregon. Journal of Environmental Studies and Sciences 10, 12-30.
| Crossref | Google Scholar |
Johnson MC, Kennedy MC, Harrison S (2019) Fuel treatments change forest structure and spatial patterns of fire severity, Arizona, USA. Canadian Journal of Forest Research 49, 1357-1370.
| Crossref | Google Scholar |
Kasischke ES, Verbyla DL, Rupp TS, McGuire AD, Murphy KA, Jandt R, Barnes JL, Hoy EE, Duffy PA, Calef M, Turetsky MR (2010) Alaska’s changing fire regime - implications for the vulnerability of its boreal forests. Canadian Journal of Forest Research-Revue Canadienne De Recherche Forestiere 40, 1313-1324.
| Crossref | Google Scholar |
Knapp EE, Valachovic YS, Quarles SL, Johnson NG (2021) Housing arrangement and vegetation factors associated with single-family home survival in the 2018 Camp Fire, California. Fire Ecology 17, 25.
| Crossref | Google Scholar |
Lambrou N, Kolden C, Loukaitou-Sideris A, Anjum E, Acey C (2023) Social drivers of vulnerability to wildfire disasters: a review of the literature. Landscape and Urban Planning 237, 104797.
| Crossref | Google Scholar |
Lampin-Maillet C, Jappiot M, Long M, Bouillon C, Morge D, Ferrier JP (2010) Mapping wildland-urban interfaces at large scales integrating housing density and vegetation aggregation for fire prevention in the South of France. Journal of Environmental Management 91, 732-741.
| Crossref | Google Scholar | PubMed |
Little JL, Jandt R, Drury S, Molina A, Lane B (2018) Evaluating the Effectiveness of Fuel Treatments in Alaska. Final Report to the Joint Fire Science Program. JFSP Project No. 14-5-01-27. University of Alaska-Fairbanks, Fairbanks, AK. 97 p. Available at https://research.fs.usda.gov/treesearch/58856
Lucas CH, Williamson GJ, Bowman D (2022) Neighbourhood bushfire hazard, community risk perception and preparedness in peri-urban Hobart, Australia. International Journal of Wildland Fire 31, 1129-1143.
| Crossref | Google Scholar |
Maranghides A, McNamara D, Mell W, Trook J, Toman B (2013) ‘A case study of a community affected by the witch and guejito fires report: #2 – evaluating the effects of hazard mitigation actions on structure ignitions.’ (National Institute of Standards and Technology, U.S. Department of Commerce: Gaithersburg, MD)
McDonald N (2019) ‘McKinley fire incident summary.’ (Alaska Incident Management Team) Available at https://fire.ak.blm.gov/content/aicc/team_left/03. Alaska IMT Incident Archive/Alaska IMT Incident Summaries/2019 Summaries/2019 08-19 to 09-02 McKinley Summary (AK Green).pdf
Meldrum JR, Barth CM, Goolsby JB, Olson SK, Gosey AC, White J, Brenkert-Smith H, Champ PA, Gomez J (2022) Parcel-level risk affects wildfire outcomes: insights from pre-fire rapid assessment data for homes destroyed in 2020 East Troublesome Fire. Fire 5, 24.
| Crossref | Google Scholar |
Mockrin MH, Locke DH, Syphard AD, O’Neil-Dunne J (2023) Using high-resolution land cover data to assess structure loss in the 2018 Woolsey Fire in Southern California. Journal of Environmental Management 347, 118960.
| Crossref | Google Scholar | PubMed |
Moran PAP (1950) Notes on continuous stochastic phenomena. Biometrika 37, 17-23.
| Crossref | Google Scholar | PubMed |
Moritz MA, Parisien MA, Batllori E, Krawchuk MA, Van Dorn J, Ganz DJ, Hayhoe K (2012) Climate change and disruptions to global fire activity. Ecosphere 3, 1-22.
| Crossref | Google Scholar |
Moritz MA, Batllori E, Bradstock RA, Gill AM, Handmer J, Hessburg PF, Leonard J, McCaffrey S, Odion DC, Schoennagel T, Syphard AD (2014) Learning to coexist with wildfire. Nature 515, 58-66.
| Crossref | Google Scholar | PubMed |
Moritz MA, Hazard R, Johnston K, Mayes M, Mowery M, Oran K, Parkinson AM, Schmidt DA, Wesolowski G (2022) Beyond a focus on fuel reduction in the WUI: the need for regional wildfire mitigation to address multiple risks. Frontiers in Forests and Global Change 5, 848254.
| Crossref | Google Scholar |
MSB (2021) Infrastructure Buildings. Available at https://data1-msb.opendata.arcgis.com/datasets/MSB::infrastructure-buildings/explore?location=62.001326%2C-149.012900%2C9.24
MSB (2023) MSB Aerial Imagery. Available at https://data1-msb.opendata.arcgis.com/pages/msb-aerial-imagery
NASA (2023) VIIRS I-Band 375 m Active Fire Data. Available at https://www.earthdata.nasa.gov/learn/find-data/near-real-time/firms/viirs-i-band-375-m-active-fire-data
Nelson KC, Monroe MC, Johnson JF (2005) The look of the land: homeowner landscape management and wildfire preparedness in Minnesota and Florida. Society & Natural Resources 18, 321-336.
| Crossref | Google Scholar |
NFPA (2018) Firewise USA®: Residents Reducing Wildfire Risks. Available at https://www.nfpa.org/Public-Education/By-topic/Wildfire/Firewise-USA
Paveglio TB, Kelly E (2018) Influences on the Adoption and Implementation of a Wildfire Mitigation Program in an Idaho City. Journal of Forestry 116, 47-54.
| Crossref | Google Scholar |
Paveglio TB, Carroll MS, Jakes PJ, Prato T (2012) Exploring the Social Characteristics of Adaptive Capacity for Wildfire: Insights from Flathead County, Montana. Human Ecology Review 19, 110-124.
| Google Scholar |
Paveglio TB, Abrams J, Ellison A (2016a) Developing Fire Adapted Communities: The Importance of Interactions Among Elements of Local Context. Society & Natural Resources 29, 1246-1261.
| Crossref | Google Scholar |
Paveglio TB, Prato T, Edgeley C, Nalle D (2016b) Evaluating the characteristics of social vulnerability to wildfire: demographics, perceptions, and parcel characteristics. Environmental Management 58, 534-548.
| Crossref | Google Scholar |
Paveglio TB, Edgeley CM, Stasiewicz AM (2018) Assessing influences on social vulnerability to wildfire using surveys, spatial data and wildfire simulations. Journal of Environmental Management 213, 425-439.
| Crossref | Google Scholar | PubMed |
Pierce G, Gabbe CJ, Rosser A (2022) Households Living in Manufactured Housing Face Outsized Exposure to Heat and Wildfire Hazards: Evidence from California. Natural Hazards Review 23, 04022009.
| Crossref | Google Scholar |
Prichard SJ, Kennedy MC (2014) Fuel treatments and landform modify landscape patterns of burn severity in an extreme fire event. Ecological Applications 24, 571-590.
| Crossref | Google Scholar | PubMed |
Radeloff VC, Hammer RB, Stewart SI, Fried JS, Holcomb SS, McKeefry JF (2005) The wildland-urban interface in the United States. Ecological Applications 15, 799-805.
| Crossref | Google Scholar |
Radeloff VC, Helmers DP, Kramer HA, Mockrin MH, Alexandre PM, Bar-Massada A, Butsic V, Hawbaker TJ, Martinuzzi S, Syphard AD, Stewart SI (2018) Rapid growth of the US wildland-urban interface raises wildfire risk. Proceedings of the National Academy of Sciences of the United States of America 115, 3314-3319.
| Crossref | Google Scholar | PubMed |
Rothermel RC 1972. A mathematical model for predicting fire spread in wildland fuels. Rocky Mountain Research Station. Res. Pap. INT-115. Ogden, UT: U.S. Department of Agriculture, Intermountain Forest and Range Experiment Station. 40 p. https://research.fs.usda.gov/treesearch/32533
Schmidt JI, Ziel RH, Calef MP, Varvak A (2024) Spatial distribution of wildfire threat in the far north: exposure assessment in boreal communities. Natural Hazards 120, 4901-4924.
| Crossref | Google Scholar |
Schoennagel T, Balch JK, Brenkert-Smith H, Dennison PE, Harvey BJ, Krawchuk MA, Mietkiewicz N, Morgan P, Moritz MA, Rasker R, Turner MG, Whitlock C (2017) Adapt to more wildfire in western North American forests as climate changes. Proceedings of the National Academy of Sciences of the United States of America 114, 4582-4590.
| Crossref | Google Scholar | PubMed |
Steelman T (2016) U.S. wildfire governance as social-ecological problem. Ecology and Society 21, 3.
| Crossref | Google Scholar |
Steffey E, Budruk M, Vogt C (2020) The mitigated neighborhood: exploring Homeowner Associations’ role in resident wildfire-mitigation actions. Journal of Forestry 118, 613-624.
| Crossref | Google Scholar |
Stevens JT, Safford HD, Latimer AM (2014) Wildfire-contingent effects of fuel treatments can promote ecological resilience in seasonally dry conifer forests. Canadian Journal of Forest Research 44, 843-854.
| Crossref | Google Scholar |
Syphard AD, Keeley JE (2019) Factors associated with structure loss in the 2013-2018 California wildfires. Fire-Switzerland 2, 49.
| Crossref | Google Scholar |
Syphard AD, Keeley JE, Bar Massada A, Brennan TJ, Radeloff VC (2012) Housing arrangement and location determine the likelihood of housing loss due to wildfire. PLoS One 7, 33954.
| Crossref | Google Scholar | PubMed |
Syphard AD, Bar Massada A, Butsic V, Keeley JE (2013) Land use planning and wildfire: development policies influence future probability of housing loss. PLoS One 8, 71708.
| Crossref | Google Scholar | PubMed |
Syphard AD, Brennan TJ, Keeley JE (2014) The role of defensible space for residential structure protection during wildfires. International Journal of Wildland Fire 23, 1165-1175.
| Crossref | Google Scholar |
US Census Bureau (2020) U.S. Decadal Census: Profile of general population and housing characteristics: 2020. https://data.census.gov/table/DECENNIALDHC2020.P1?t=Populations and People&g=040XX00US02$1600000&y=2020&d=DEC Demographic and Housing Characteristics
USDA, USDOI (2001) ‘Urban–wildland interface communities within vicinity of federal lands that are at high risk from wildland fire.’ (U.S. Department of Agriculture and U.S. Department of the Interior) Available at https://www.federalregister.gov/documents/2001/01/04/01-52/urban-wildland-interface-communities-within-the-vicinity-of-federal-lands-that-are-at-high-risk-from
Wilson JR, Ralph A (1985) Observations of extinction and marginal burning states in free burning porous fuel beds. Combustion Science and Technology 44, 179-193.
| Crossref | Google Scholar |