Comparing gas composition from fast pyrolysis of live foliage measured in bench-scale and fire-scale experiments
David R. Weise













A
B
C
D
E
F
G
H
I
J
K
L
Abstract
Fire models have used pyrolysis data from oxidising and non-oxidising environments for flaming combustion. In wildland fires pyrolysis, flaming and smouldering combustion typically occur in an oxidising environment (the atmosphere).
Using compositional data analysis methods, determine if the composition of pyrolysis gases measured in non-oxidising and ambient (oxidising) atmospheric conditions were similar.
Permanent gases and tars were measured in a fuel-rich (non-oxidising) environment in a flat flame burner (FFB). Permanent and light hydrocarbon gases were measured for the same fuels heated by a fire flame in ambient atmospheric conditions (oxidising environment). Log-ratio balances of the measured gases common to both environments (CO, CO2, CH4, H2, C6H6O (phenol), and other gases) were examined by principal components analysis (PCA), canonical discriminant analysis (CDA) and permutational multivariate analysis of variance (PERMANOVA).
Mean composition changed between the non-oxidising and ambient atmosphere samples. PCA showed that flat flame burner (FFB) samples were tightly clustered and distinct from the ambient atmosphere samples. CDA found that the difference between environments was defined by the CO-CO2 log-ratio balance. PERMANOVA and pairwise comparisons found FFB samples differed from the ambient atmosphere samples which did not differ from each other.
Relative composition of these pyrolysis gases differed between the oxidising and non-oxidising environments. This comparison was one of the first comparisons made between bench-scale and field scale pyrolysis measurements using compositional data analysis.
These results indicate the need for more fundamental research on the early time-dependent pyrolysis of vegetation in the presence of oxygen.
Keywords: CH4, CO, CO2, compositional data, Fourier transform infrared spectroscopy, gas composition, H2, log-ratio, longleaf pine, phenol.
Introduction
Wildland fire is a complex phenomenon involving many chemical and physical processes at various length scales. Moldoveanu (2021) categorised wildland fire as burning which is a process that includes both pyrolysis and combustion. The two processes are not clearly separable in a natural setting since the products of one process are linked to and can influence the other process. During pyrolysis, a dead, solid wildland fuel composed of cellulose, hemicellulose, lignin, water and trace elements is heated and breaks down into constituent parts consisting of gases, tars and a solid material called char (Shafizadeh and Fu 1973; Shafizadeh 1982; Di Blasi 2008; Neves et al. 2011). Living wildland fuels additionally contain plant metabolites (Jolly et al. 2012, Matt et al. 2020). While Shafizadeh and others examined pyrolysis in non-oxidising and oxidising environments, Di Blasi’s modelling review (2008) limited pyrolysis to a non-oxidising environment. In a wildland fire, pyrolysis occurs in the presence of oxygen and combustion products (Leroy et al. 2006). For porous plant materials, oxygen and water vapour can modify the pyrolysis process, either as catalysts to the celluloses or char oxygen chemisorption (DeGroot and Shafizadeh 1983; Moldoveanu 2021). Smouldering combustion produces pyrolysis products that are a result of a low heating rate in a relatively oxygen-deficient environment (Frandsen 1991; McKenzie et al. 1995; Carvalho et al. 2002; Torero et al. 2020). During flaming combustion, gaseous pyrolysis products generated by higher heating rates can react with oxygen releasing energy (heat) and a large assortment of gaseous and solid chemical compounds and solid products (char) which can also react further with oxygen via the glowing combustion process.
An extensive body of knowledge about pyrolysis of wildland fuels in both non-oxidising and oxidising environments exists. Numerous compounds have been identified from pyrolysis of wood and foliage. For example, 213, 224, and 326 compounds have been previously listed (Goos 1952; Soltes and Elder 1981; Weise et al. 2022b), respectively, and other recent work identified potentially hundreds of compounds (Moore et al. 2021). The composition of pyrolysis products is dependent on heating rate and temperature (e.g. Shafizadeh 1982; Safdari et al. 2019). Temperatures below 300°C favour char, H2O, CO and CO2 production, intermediate temperatures (300–500°C) favour production of tar containing many different compounds and higher temperatures (>500°C) produce a mixture of low molecular weight gaseous products (Shafizadeh 1982). Safdari et al., (2019) found that fast pyrolysis of foliage from live fuels resulted in higher tar and lower char yield when compared to slow pyrolysis that was like thermogravimetric analysis (TGA). Gas yield between the two pyrolysis types was not statistically different. In a study of the O2 effect on cellulose degradation in smouldering combustion, the difference in pyrolysis rate at a heating rate of 0.25°C s−1 under nitrogen and air atmospheres disappeared at 310°C (Shafizadeh and Bradbury 1979).
Much of the work describing pyrolysis kinetics has occurred in benchtop-scale TGA-type experiments at low or high heating rates and the results have been assumed to be applicable to wildland fires (Philpot 1970; Dimitrakopoulos 2001). However, TGA experiments typically only use slow heating rates (<1°C s−1) whereas measured heating rates of 75–5000°C s−1 in wildland fires have been reported (Butler et al. 2004; Tachajapong et al. 2008). TGA experiments are applicable to conditions under which smouldering combustion occurs and have been critical to defining processes associated with smouldering combustion (Torero et al. 2020). In addition, TGA and other similar methods most often grind and dry fuels to produce uniform samples to yield more consistent, least adulterated results, but this method raises the question about loss of organic material from the drying and grinding process and the effect of fuel particle shape. While most TGA experiments occur in inert atmospheres, some have been conducted in normal atmospheres with the intent to be more applicable to the wildland fire setting (Hillado 1977; Dimitrakopoulos 2001). Conditions under which pyrolysis data from inert atmospheres can be extended to pyrolysis under an oxidising environment have been identified: (1) oxygen cannot reach the surface of a pyrolysing particle or (2) the type of environment (inert, oxidising) does not affect the kinetics and the pyrolysis mechanisms (Senneca et al. 2004); however, it is unknown if these conditions are implicitly assumed to be met in pyrolysis models used in wildland fire. A quick review of the literature reveals that the type of environment may or may not affect the kinetics of pyrolysis.
Tihay et al. (2009a) described three general approaches to modelling gas phase combustion in physical fire models: using global rates and thermodynamic parameters, using reduced reaction mechanisms, and using CO burning in air. In a review of wildland fire models developed between 1990 and 2009, Sullivan (2009a, 2009b, 2009c) described how several models implemented pyrolysis and combustion. Simplified reactions and chemical kinetics, often involving char and gases, were used in the physical models because of the high computational demand needed to implement detailed chemistry. Many of the physics-based fire models used a variety of mixtures of key pyrolysis products including H2, CO, CH4, and C2H6 (Grishin 1997; Grishin and Perminov 1998; Morvan and Dupuy 2001; Zhou and Mahalingam 2001; Mell et al. 2009; Clark et al. 2010; Borujerdi et al. 2022) and solid char (Grishin 1997; Grishin and Perminov 1998; Morvan and Dupuy 2001; Zhou and Mahalingam 2001; Mell et al. 2009; Clark et al. 2010; Borujerdi et al. 2022) and the process of char oxidation. The assumed mixture of gases used in these models was typically chosen based on a simplified pyrolysis reaction model, with reaction kinetic parameters provided by thermogravimetric analysis (TGA) experiments of wood and other wildland fuels performed in either a non-oxidising or oxidising environment.
QUIC-Fire is an example of a complex physical model that is being simplified for ‘field’ use (Linn et al. 2020). The chemistry has been simplified to a single equation representing the burning of wood with oxygen. In the United States, today’s widely used operational fire behaviour model was based on data from homogenous beds of dead fuels and associated theory (Rothermel 1972). The chemical aspects of combustion were assumed to occur at much faster rates than heat transfer and the only chemical aspect of this model related to fuel heat content (kJ kg−1). Thus, heat content has been determined for many common wildland fuels and used in the basic calculation of fire spread (Hough 1969; Countryman and Philpot 1970; Rothermel 1972; Countryman 1982; Susott 1982a, 1982b; Rogers et al. 1986).
While TGA and fast pyrolysis techniques have been applied to a variety of lignocellulosic fuels, fast pyrolysis techniques have seldom been applied to wildland fuels, particularly the intact foliar portion which is typically the most readily ignited component in a wildland fuel complex. Similarly, the results from the many lab-based, tightly controlled experiments have seldom been compared to measurements made in wildland fires which are more complex to ascertain similarity between lab and field conditions. The applicability of the assumptions and simplifications made in the models to the actual conditions under which a wildland fire pyrolyses unburned fuels is largely undescribed. We found no literature supporting the assumption that bench-scale experiments are applicable to the wildland fire setting (Weise et al. 2022a).
It is well known that primary pyrolysis products that enter a hot environment may further react even in the absence of O2. One pathway is for the primary tars to crack into lower molecular weight gas species. Another pathway is for the aromatic tars to polymerise, possibly with the help of some of the light gases such as acetylene and ethylene, forming polyaromatic hydrocarbons (PAH) and eventually forming soot. The polymerisation and condensation reactions release H2 in the process. Pathways in the presence of O2 in a fire include combustion, gasification, and water-gas shift reactions, depending on the mixing, local temperature, and local species concentrations. Comparison of the oxidative and non-oxidative pyrolysis results can help shed light on which processes are important in the flames studied.
In a project (Weise et al. 2022a) designed to improve the understanding and modelling capability of pyrolysis in physics-based fire spread models, three goals were identified: (1) provide more detailed description of pyrolysis and the evolution of its products for a greater variety of southern United States fuels than is currently known, (2) determine how convective and radiative heat transfer from flames to live fuel particles influenced pyrolysis and ignition at laboratory and field scales and (3) gain more detailed insight into pyrolysis, combustion and heat transfer processes in wildland fire spread through the use of high-fidelity physics-based models. These goals were achieved by (1) characterising the physical, chemical, compositional and spatial structure of wildland fuels (Safdari et al. 2018; Hudak et al. 2020; Matt et al. 2020; Herzog et al. 2022), (2) characterising pyrolysis products by measurement of a variety of live and dead foliar fuels in laboratory and small-scale field experiments (Safdari et al. 2018; Amini et al. 2019a; Scharko et al. 2019a, 2019b; Phillips et al. 2020; Banach et al. 2021; Moore et al. 2021), (3) determining the effects of convective and radiant heat transfer on pyrolysis (Safdari et al. 2019, 2020; Weise et al. 2022b) and (4) performing high-fidelity physics-based modelling of pyrolysis for bench-scale and wind tunnel experiments (Yashwanth et al. 2015, 2016; Shotorban et al. 2018; Borujerdi et al. 2019, 2020, 2022; Borujerdi and Shotorban 2022). Pyrolysis gases were generated in non-oxidising environments from individual leaves at bench-scale by slow heating in a pyrolyser (Hillier et al. 2013) and fast heating in a flat-flame burner at heating rates and temperatures typical of wildland fires (approximately 100°C s−1, 100 kW m−2 s−1 and 765°C) (Safdari et al. 2018). Pyrolysis gases from fuel beds of live and dead fuels were measured in a wind tunnel and in the field in small prescribed burns at Ft. Jackson, SC by capturing gases in canisters (Weise et al. 2022c) or in real-time using nonintrusive Fourier-transform infrared (FTIR) spectroscopy (Scharko et al. 2019a; Banach et al. 2021).
At the bench scale, heating rate/mode, moisture status and plant species affected the composition and yield of pyrolysis products with higher heating rates yielding relatively more tars (Safdari et al. 2019; Weise et al. 2022b). In the oxidising (normal atmosphere) environment of the wind tunnel and small prescribed burns, burning phase (pyrolysis, flaming combustion) affected the gas composition. Log-ratios of measured gases with CO2 were larger in the pyrolysis samples indicating relatively more of these gases in the pyrolysis samples. The presence of live plants affected the gas composition. The relative composition of pyrolysis gases was affected by the fire location (wind tunnel, field) (Weise et al. 2022c). Comparison of the FTIR and gas chromatography (GC)/flame ionization detector (FID) gas compositions found that trace gas composition differed between methods but each method yielded comparable description of the primary fuel gases (CO, CH4) (Weise et al. 2023). This paper presents the comparison of the pyrolysis data derived from these experiments to test the assumption that TGA-like and fast pyrolysis are representative of wildland fire conditions.
Methods
A brief description of each of the three experiments follows. The interested reader is referred to the referenced papers for more details. All papers from the Forest Service are available through the www.fs.usda.gov/research/treesearch document repository and the data sets are available through the Wildland Fire Science Initiative (WFSI) Data Portal (Weise et al. 2024a, 2024b, 2024c).
Bench-scale non-oxidative pyrolysis experiments
Bench-scale experiments at Brigham Young University (BYU) used a flat-flame burner (FFB) with and without a radiant panel to produce a hot, oxygen-free post flame environment to heat single species samples, typically leaves, at high heating rates (180 and 195°C s−1) in a non-oxidising environment (Safdari et al. 2020). The FFB setup (Fig. 1a) yielded some permanent gases and many tars comprising the measured pyrolysis products. These gas samples and all others described below are time-integrated samples. While the BYU FFB setup has been used previously to measure mass loss associated with ignition of live fuels (e.g. Fletcher et al. 2007) and mass loss recorded during the current experiments (Safdari 2018), the composition of the gas samples was determined off-line. Foliage from 15 different plant species was heated in the FFB. Tars, which can undergo secondary pyrolysis producing additional simpler pyrolysis products, and kinetic modelling, were the primary foci of this set of experiments (Safdari et al. 2018, 2019, 2020; Amini et al. 2019a, 2019b, 2021). The yield and concentrations of over 100 tar compounds were determined using a gas chromatograph equipped with a mass spectrometer (GC/MS). A gas chromatograph equipped with a thermal conductivity detector (GC/TCD) was used for permanent (light or non-condensable at ambient conditions) gases. The permanent gases consisted of CO, CO2, CH4 and H2. Molecular mass of the identified gases ranged from 2 to 250 amu. As the primary focus of the experiment was to measure tars and other secondary pyrolysis products, GC/MS was selected for detection. To eliminate the effect of heating rate on composition in this analysis, only data resulting from convective or radiant/convective high heating rates, denoted Conv and RadConv, respectively, were used from the bench-scale experiments. In an on-going companion bench-scale study at the USDA Forest Service Forest Products Laboratory, a cone calorimeter has been used to heat leaf samples at irradiance of 35 kW m−2 to achieve fast pyrolysis (10°C s−1) in air for both unpiloted and piloted conditions; however, those data are not yet published and they do not provide as detailed a description of the composition of permanent gases and tars as the BYU data. The pyrolysis gas composition determined at BYU was used in the physics-based modelling of leaf scale flames (Borujerdi et al. 2022). While not pertinent to the present paper, related measurements and modelling to estimate kinetic parameters for pyrolysis of the BYU leaf samples and longleaf pine needles based on slow pyrolysis are available (Amini et al. 2019a, 2021; McGrattan et al. 2023, sec. 12.9.4).
Experimental setups to determine composition of fast pyrolysis gases in non-oxidising (a) and oxidising (b–d) environments. (a) Flat-flame burner setup used to heat southern U.S. wildland fuels in an O2-free (fuel rich) post-flame environment. (b) canister sampling for flaming (F) and pyrolysis gases (P) in a wind tunnel where the fire's flame heated fuels. (c) FTIR and GC/FID sampling setup in the wind tunnel. (d) canister sampling for FTIR and GC/FID in the field.
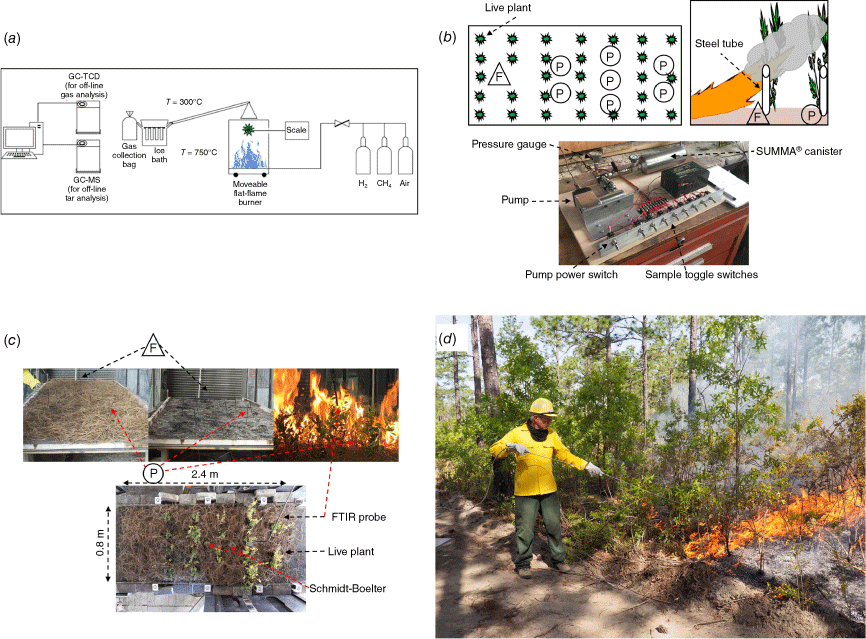
Wind tunnel oxidative pyrolysis experiments
The second set of experiments used simplified fuel beds of longleaf pine (Pinus palustris Mill.) needles with a subset of the 15 plant species used above (Fig. 1b). These fuel beds were heated by a spreading flame in a wind tunnel under normal atmospheric composition (oxidising environment) (Banach et al. 2021; Weise et al. 2022c, 2023). Pyrolysis gases were collected using an array of eight stainless-steel sample tubes to fill a sample canister with multiple small aliquots of pyrolysis gases and a separate canister to characterise flaming emissions for comparison using off-line identification and quantification. At sample tube ‘F’, flaming emissions (smoke), were collected in a canister for 30 s before the flame reached the sample tube. While pyrolysis gases may have been entrained into the plume and included in the flaming sample, the preponderance of gases resulted from flaming combustion because the plume extended downstream of the visible flame (Aminfar et al. 2020). The flaming canister was then replaced by a new canister. A verbal command to initiate and end sampling at the ‘P’ sample tubes based on fire progression resulted in a short sampling interval at each tube prior to flame front arrival. The plume was well above the tube inlet so minimal flaming combustion gases were collected (Fig. 1b). Each pyrolysis canister was filled with multiple small aliquots from the seven ‘P’ (pyrolysis) sample tubes. Contamination of ‘P’ samples by residual smouldering gases was nonexistent. Between each fire, ambient air was pumped through the sampling system for 30 min to purge any residual gases. Sampling gases from replicated experiments with controlled fuel bed composition, quasi-steady state repeatable heating conditions (flame) and sequentially collected gas samples from several points along the length of the fuel bed as the flame advanced limited the variability of the gas composition from the seven pyrolysis samples.
All canisters were analysed using EPA method TO-14A (EPA 1999) for CO2, CO, CH4, and C2 to C7 hydrocarbon gases with an Agilent model 7890 gas chromatograph configured with two columns running simultaneously.1 A 3.175 mm diameter, 2 m Carbosphere packed column with a nickel catalyst methaniser was used for analysis of CO2 and CO using a flame ionisation detector (FID) (Colket et al. 1974). The second column, a 0.53 mm in diameter × 50 m length Agilent Al/S column, separated hydrocarbons and methane. Both columns went to FID detectors and were run simultaneously. Chromatogram data were collected and processed by Agilent OpenLab software. Pyrolysis gases were also collected by a single probe connected via 3/8″ heated metal tubing (70oC) to a Bruker Tensor 37 (T37) spectrometer/gas cell system to extract the gases before the passage of the flame front down the wind tunnel. The White cell was held at approximately 55°C to keep the gases and particulates from condensing inside the cell and gases identified via Fourier transform infrared spectroscopy (FTIR) (Fig. 1c). The GC/FID provided measurements of CO, CO2, and C1 to C6 hydrocarbons. A Trace Analytical RGA3 reduction gas analyser was used to measure H2 concentrations. FTIR provided measurements of CO, CO2, CH4, several other light hydrocarbons as well as some aromatics, other oxygenated hydrocarbons and nitrogen compounds (see table 1 in Weise et al. (2023)). The FTIR was typically run in extractive (static) mode to provide higher resolution detection of pyrolysis gases (Banach et al. 2021). Less than 10 percent of the wind tunnel experiments were measured by FTIR in dynamic mode; this mode enabled visualisation of the evolving composition of gases. Analysis of the dynamic data has not been completed. Collectively, these instruments determined the concentrations of 48 gases with molecular mass ranging from 2 to 128 amu (Weise et al. 2022c, 2023). Gas samples for both pyrolysis and flaming combustion were collected and shown to differ, supporting the assertion that the gas samples described the composition of pyrolysis gases.
Field oxidative pyrolysis experiments
In a third set of experiments, similar measurement methods were used to collect and analyse the same pyrolysis gases from prescribed burns on 0.1 ha plots in longleaf pine stands at Ft. Jackson, SC (Scharko et al. 2019a, 2019b; Hudak et al. 2020; Banach et al. 2021; Herzog et al. 2022; Weise et al. 2022c, 2023). To sample gases during the prescribed burns safely (Fig. 1d), we used a gas sampling probe built from 2.5 m of 6 mm stainless-steel tubing connected to the sampling package with flexible stainless tubing. A swing Piston KNF Neuberger Pump, 12-volt gel cell rechargeable battery and stainless-steel tubing coupled to a pressure relief valve and gauge comprised the sampling package. The flow rate to fill the SUMMA® canisters was 250 mL s−1. A remotely triggered sampling system such as the Fire Atmosphere Sampling System (Susott et al. 1991; Ward et al. 1992) was not practical for this study as expert judgement was needed to locate the sample probe to increase the probability of collecting only pyrolysis gases. The sampling strategy was to identify plants with sufficient foliage along the edge of the burn unit where they could be safely sampled. At the base of the advancing flame, the probe collected short interval sample aliquots when it was likely that pyrolysis was occurring. Due to this uncertainty, the field samples were not initially labelled as flaming or pyrolysis samples; subsequent statistical analysis (logistic regression) classified these samples (Weise et al. 2022c). Based on thermal camera imagery of shrub foliage, the mean heating rates for these prescribed burns ranged from 107 to 372°C s−1. Further detail on instrumentation, fuels, and measurements are available in the cited works and in the project’s final report (Weise et al. 2022a). In all three sets of experiments, the reported gas concentrations were excess concentrations (i.e. concentration in excess of ambient levels in the test environment).
Statistical data analysis
In this paper, to determine if non-oxidising environment FFB pyrolysis results which are typical of many pyrolysis studies were related to the oxidising environment wherein wildland fires occur, the subset of gases common to all three experiments were analysed. The statistical characteristics of these data sets and the techniques used to analyse them follow. All data analyses and modelling were conducted on the R system for statistical computing version 4.3.1 (R Core Team 2023).
Smoke and pyrolysis gases are compositional data (e.g. Bandeen-Roche 1994; Engle et al. 2011; Jarauta-Bragulat et al. 2016; Gibergans-Baguena et al. 2020; Weise et al. 2020a, 2020b) that are multivariate and contain relative information. Compositional data analysis (CoDA) relies on log-ratio coordinate representations of the data. Note that in the air pollution and smoke communities, the amount of a particular gas has often been expressed as a simple ratio with a common gas such as CO or CO2 (Darley et al. 1966; Crutzen et al. 1979; Andreae et al. 1988). While this is a common approach, we have shown that with an additional calculation step an emission ratio can in fact be transformed into so-called additive log-ratio (alr) coordinates (Aitchison 1986; Weise et al. 2022b). The presence of zero values in a compositional data set hampers the log-ratio approach, as neither dividing by zero nor computing the logarithm of zero are feasible mathematical operations.
Assuming that zero values actually corresponded with unobserved values below the minimum observed concentrations (i.e. left-censored data), those instances where the gas concentration was reported as 0 were imputed by random values below such minimum observed concentrations. Such values were generated from a lognormal probability distribution fitted to the observed concentrations using the multLN method in the zCompositions R package (Palarea-Albaladejo and Martín-Fernández 2013, 2015). This is designed to ensure that the log-ratios between other gases are not affected by the imputation. After imputation, the measured gases common to all three experimental scales were identified (H2, CO, CO2, CH4, C6H6O (phenol)), and the concentrations of the remaining gases were amalgamated into a single category ‘Other’. Amalgamation does not affect the relative relationships between the other parts of the resulting (reduced) composition provided that CoDA techniques are used (Aitchison 1986; van den Boogaart and Tolosana-Delgado 2013; Greenacre 2020). The three data sets involved varying heating rates which is known to affect both the reaction kinetics and gas composition. While the rates and temperatures were somewhat different, they all fell within the category of fast pyrolysis (Maschio et al. 1992; Demirbas and Arin 2002). Direct comparison of the absolute measures in the data sets would include this difference as a confounding factor that could not be isolated. However, as in Weise et al. (2022b) and other data analyses involving compositional data, the relative amount of one gas (or group of gases) to another gas (or group of gases) is the variable that is being compared and not the absolute values. The compositional approach filters out the instrument effect which mostly determines the absolute values (Aitchison 1986).
While GC/FID has been shown to be more sensitive than GC/TCD for propane measurement (Budiman et al. 2015), for the purposes of this analysis, we assumed that the sensitivity of GC/FID and GC/TCD was similar. In summary, H2, CO, CO2 and CH4 were measured using GC/TCD or GC/FID, phenol was measured by FTIR or GC/MS, and ‘Other’ was measured by FTIR, GC/MS or GC/FID. The gas composition was normalised so that the gases were expressed as proportions of the total, summing up to 1. Note that because of the use of different instruments in the non-oxidising (bench) and oxidising (wind tunnel, field) environments, any effect on the composition due to instrumentation was confounded with the environment effect.
Summary measures for compositional data were calculated, including the centre (mean composition), total variance and contributions of individual gases to this (see e.g. van den Boogaart and Tolosana-Delgado 2013). Geometric mean barplots were used to visually examine differences between the pyrolysis environments (see e.g. Butler et al. 2020).
Multivariate data analysis consisted of principal components analysis (PCA) (Mardia et al. 1979), permutational multivariate analysis of variance (PERMANOVA) (Anderson 2001, 2017) and canonical discriminant analysis (CDA) (Rencher 1992). Following the CoDA methodology, the data were expressed in appropriate log-ratio coordinates for analysis and interpretation of results. Thus, PCA is usually applied on so-called centred log-ratio (clr) transformed data to reduce the data dimensionality and to facilitate the visualisation of possible trends using a biplot graphical display (Aitchison and Greenacre 2002; Gower et al. 2011). PCA partitions the total variance in the data set sequentially so that the first principal component accounts for the largest portion of the total variance, the second component accounts for the next largest portion, and so on.
A special class of log-ratio coordinates used here, so-called log-ratio balances or simply balances, considers ratios representing contrasts or comparisons between subsets of parts of the composition (aggregated by their respective geometric means) that facilitate scientifically meaningful interpretations in the context of application. In our case, comparisons between subsets of the D = 6 gases were represented by (D − 1) = 5 balances with the general expression given by
A balance then compares the geometric mean of rk gases in one subset (in the numerator, indicated with + symbol) with the geometric mean of sk gases in another subset (in the denominator, indicated with − symbol). The preceding multiplicative factor is a normalisation factor formally required to ensure comparability of the balances and orthonormality of the resulting coordinate system. A simpler equivalent expression as the normalised difference between the means of log-transformed data is given by
These balances can be obtained by a sequential binary partition of the original gas composition (Egozcue and Pawlowsky-Glahn 2005) (see Table 1 for the selection of balances used in this study). Considering such log-ratio coordinate representation as a basis, PERMANOVA was applied to statistically test the potential effect of location (bench, wind tunnel, field) on the mean composition of gases using the adonis function in the vegan R package (Oksanen et al. 2020). Moreover, CDA was performed on the selected balances using the candisc package in R (Friendly and Fox 2021). CDA is a multivariate technique that derives optimal linear combinations of the original variables with the goal to provide the maximal separation between the pyrolysis environments in our case. Like PCA, the scores obtained by CDA can be used for a low-dimensional graphical representation of samples and variables which facilitates interpretation. After the exploratory analyses suggested that the gas composition differed between environments, pairwise comparisons were made based on the entire composition and individual balances. Boxplots were used along with Kruskal–Wallis tests to look at the differences between environments for each balance. P-values resulting from statistical significance testing were adjusted for multiple comparisons using Benjamini and Hochberg’s method (1995).
Balance | H2 | CO | CO2 | CH4 | Phenol | Other | |
---|---|---|---|---|---|---|---|
Common vs Other | + | + | + | + | + | − | |
CO, CO2 vs H2, CH4, Phenol | − | + | + | − | − | ||
CO vs CO2 | + | − | |||||
CH4 vs H2, Phenol | − | + | − | ||||
H2 vs Phenol | + | − |
‘+’ denotes gases in numerator and ‘−’ denotes gases in denominator of the balance (see Eqn 1).
Results
The combined data set consisted of 306 observations (bench = 174, wind tunnel = 107, field = 25). In the non-oxidising bench scale experiments at BYU, all six gases were measured. In the oxidising environment experiments in the wind tunnel and in the field, the GC/FID analysis of the canisters did not measure phenol. Infrared spectroscopy is unable to detect homonuclear diatomic molecules of which H2 is one. These unmeasured/undetected gases were assigned below detection limit (BDL) values in these samples using the multLN procedure as described above resulting in a BDL percentage of 34% for phenol (105/306) and 8.8% for H2 (27/306).
The sample mean compositions changed notably between the non-oxidative pyrolysis (bench) samples and the oxidative (wind tunnel, field) samples (Table 2 top). Except for CO2, the mean values listed in Table 2 were higher in the bench measurements than in the wind tunnel or field experiments. Because of dilution factors and familiarity with gas ratios in syngas produced by pyrolysis, we examined the untransformed ratios of H2/CO or H2/CO2 as an indicator of the validity of the bench measurements. These ratios were negligible in the wind tunnel and field experiments. In the bench experiments the ratios were 0.38 and 1.31, respectively, which was similar to syngas ratios produced from coal and biomass (Di Blasi 1999; Grieco 2011).
Table 2 (bottom) shows the percentage contributions of the individual gases to the metric variance of the composition. The contribution of CO was relatively small and comparable between the locations; those percentages of CO2 and CH4 were similarly low for the wind tunnel and field. While the contribution for H2 was relatively small in the bench-scale measurements, it was more than half of the variability in the wind tunnel and field. Similarly, phenol (C6H6O) accounted for somewhat more than half of the variability at the bench-scale. In addition to phenol, 18 other phenolic compounds were identified in the tars collected in the bench-scale experiments (Safdari et al. 2018) and both plant species and heating mode/rate affected the relative amounts of these compounds (Weise et al. 2022b). The variability of the individual phenolic compounds ranged up to 2% of the total variance in these experiments. In the wind tunnel measurements, the GC/FID did not measure phenol which was assigned a BDL value described previously, resulting in a smaller contribution to total variance. In the wind tunnel, a heated line was used to keep sampled gases from condensing prior to injection in the White cell used with the FTIR and a HEPA (high efficiency particulate air) filter was used to remove any condensed tars and char particles (Banach et al. 2021). In the field, gas samples were collected in SUMMA canisters; gas samples were extracted from the unheated canisters within 24 h via a 70°C heated line and injected into the White cell (Scharko et al. 2019b). This temperature is not sufficient to keep tars from condensing. These sampling processes likely decreased phenol’s relative amount and contribution to total variance in the wind tunnel and field locations.
In the compositional PCA biplot of the gas composition (Fig. 2), the first principal component (PC1) accounted for 60.7% of the variation and the second principal component (PC2) accounted for an additional 33.1% for a total of 93.8%. Several characteristics of the data can be discerned from Fig. 2. The length of rays approximates the standard deviations of the clr-transformed gases. These were small for CO and CH4 relative to the other gases in the composition, which is consistent with their overall low contribution to the total variability of the composition as reported in Table 2. Having the rays for CH4, CO and CO2 pointing in the same direction and close to each other indicated that these gases were highly proportional in concentration. Proportionality is the CoDA analog to correlation (Egozcue et al. 2018, Lovell et al. 2015). Contrarily, the ray for phenol pointing in the opposite direction, and exhibiting long links between the corresponding arrowheads, suggested a negligible association with CO2, CH4 and CO concentrations. Similar pattern was observed between H2 and the Other gases. These results showed that the bench-scale observations (non-oxidative environment) were tightly clustered and distinct from the wind tunnel and field observations, particularly along the direction defining the contrast between phenol and CO2 concentrations, with bench-scale observations presenting higher concentrations of phenol and lower concentrations of CO2, comparatively. This result is consistent with the observation that non-oxidative conditions favour tar formation (of which phenol is a major component) and that these tar components decrease as oxygen increases (Chen et al. 2008). The wind tunnel and field results tended to cluster together. However, the biplot suggested that the FTIR and GC/FID methods differed, particularly along the H2 against Other direction, which has been recently corroborated in a separate analysis (Weise et al. 2023).
Compositional principal components analysis (PCA) biplot for pyrolysis gases distinguishing observations by location and method (instrument). Note the separation between the non-oxidising (bench) and oxidising (wind tunnel, field) pyrolysis environments. Arrows indicate clr-transformed gas concentrations. Points represent the original samples through their PCA scores. Field = 0.1 ha prescribed burn plots, Ft. Jackson, SC, US.
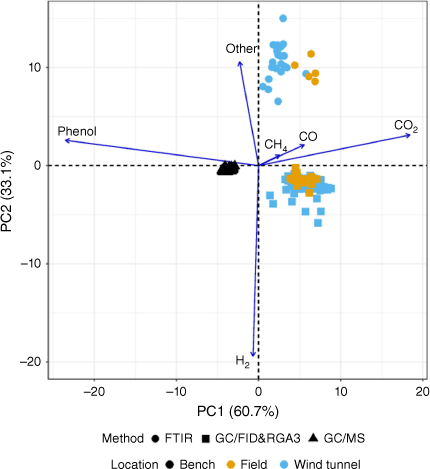
Geometric mean barplots, showing the average deviations of the individual geometric means relative to the overall means, indicated similar trends (Fig. 3). Deviations by instrument showed that FTIR and GC/FID were similar and opposite the GC/MS means. Note that the deviations labelled GC/MS also include gases measured with GS/TCD. The plot by location showed a similar pattern which also indicated the confounding with the instrument/method. When the wind tunnel and field data were combined as a single ‘oxidising environment’ data set, the deviation plot showed consistent differences with the non-oxidising environment. Examination of the mean fitted values of the five balances (as defined in Table 1) also suggested differences between locations (Table 3). To identify the balances between gases which best separated the oxidising environment from the non-oxidising environment, CDA was performed using the balance values. Fig. 4 shows the resulting first two CDA dimensions (those accounting for the highest fraction of the between-group variability). The CDA scores of the samples at the three locations showed that these locations were essentially arranged along the first CDA dimension (x-axis; 99.9% of the between-group variation accounted for), with this being mostly defined by the contrast between the CO-CO2 balance and the other balances (except for pointing at a nearly orthogonal direction). The mean scores per location, indicated by the large red symbols, also illustrated the separation between the bench scale (with higher values of the balance and lower values of the ((CO, CO2) vs. (H2, CH4, phenol)) and (CH4 vs (H2, phenol)) balances) and the other two locations.
Geometric mean barplots (in log-ratio scale) by instrument, location and environment for pyrolysis gases measured from wildland fuels. The reference line at zero indicates the overall mean composition.2
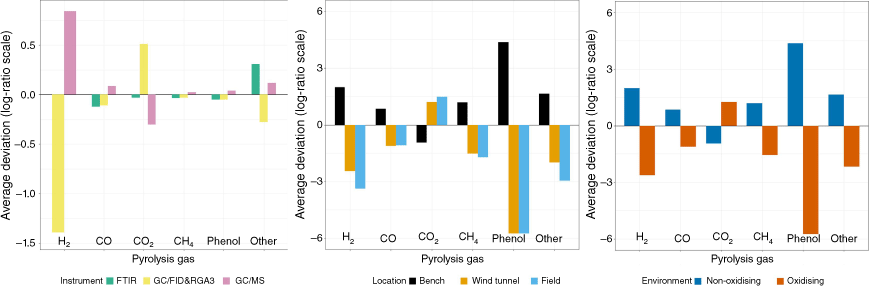
Plot of first two dimensions resulting from canonical discriminant analysis (CDA) stressing the difference in scientifically meaningful balances (Table 1, ). between the oxidising (wind tunnel, field) and non-oxidising environments (bench). These two dimensions accounted for 100% of original variability. Arrows indicate the balances that separated the two environments. Points indicate CDA scores of the original observations. The large red symbols indicate the mean values of the CDA scores for each group. Field = 0.1 ha prescribed burn plots, Ft. Jackson, SC, US.
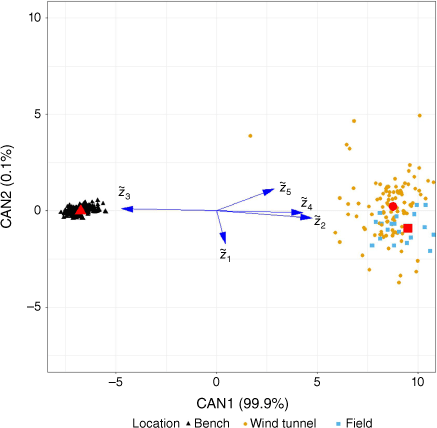
PERMANOVA testing the effect of location on the gas composition found that location was highly significant at the usual statistical significance threshold of 0.05 (P-value < 0.001), and pairwise comparison of the individual effects found that the non-oxidising environment results differed from the other two locations (P-values = 0.001), but no significant differences were found between field and wind tunnel (P-value = 0.294). Based on these tests and the evidence provided by the previous exploratory data analyses, the wind tunnel and field data were grouped together as an oxidising environment for further comparison with the non-oxidising environment results.
Fig. 5 summarises boxplots and results from Kruskal–Wallis tests looking at how each balance was affected by the environment. All five balances differed significantly between the oxidising and non-oxidising environments according to the results. For all balances except CO vs CO2, their values were larger in the oxidising environment. As would be expected, the CO vs CO2 balance indicated that there was significantly more CO relative to CO2 in the non-oxidising environment. This has been observed in other less oxidative environments, including the pyrolysis and smouldering stages of fire (Yokelson et al. 1997; Tihay et al. 2009b; El Houssami et al. 2016). The data analyses above all suggested differences between the three locations with the wind tunnel and field locations (oxidising) being more similar compared to the bench (non-oxidising) compositions.
Box and whiskers plots for selected balances of pyrolysis gases for non-oxidising (fuel rich, no O2) and oxidising (ambient atmosphere) environments. Adjusted P-values from Kruskal–Wallis testing for comparisons of balances by environment shown. See Table 1 for balance description.
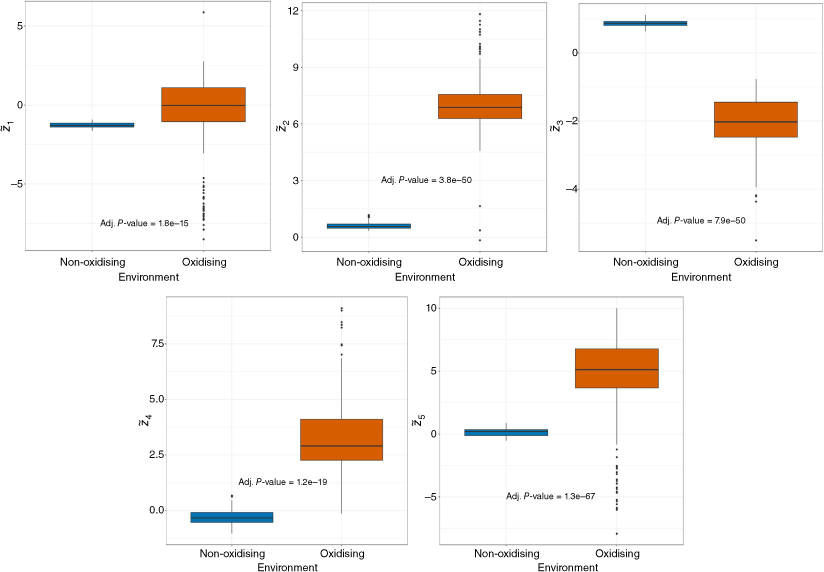
Discussion
A wildland fire is a chaotic event and the gases which are produced by the pyrolysis of wildland fuels may (a) rapidly oxidise producing (and sustaining) the flame, (b) react within the fuel-rich high temperature flame zone to either form lower molecular weight gases or (c) polymerise and condense to form PAH and soot, (d) pass through the flame and only partially oxidise, or (e) cool and condense without passing through the flame zone (Ryan and McMahon 1976) yielding a rich mixture of gases and particulates collectively known as smoke. This last process is prevalent in smouldering combustion. While these chemical processes occur on small time scales as short as a few milliseconds and there are myriad pathways along which the reactions can occur (Sullivan 2017), the data used in the present analysis were collected under largely controlled conditions with the hope that they represented the ‘average’ or ‘most frequent’ composition of gases for these fuels in both non-oxidising and oxidising environments. In the non-oxidising FFB experiments which measured pyrolysis products with a focus on tars and major light gases associated with a range of plant species, different heating rates and modes and foliar moisture contents, differences in composition due to heating mode/rate and species were found (Weise et al. 2022b). Foliar moisture also affected some of the other log-ratios. However, when compared to the oxidising environment compositions, the non-oxidising results were tightly grouped indicating less variability in composition which was not surprising as the oxidising environment was less controlled.
In the oxidising environment experiments, care was taken to capture gases immediately before the flame front arrived, i.e. during the preheating stage. In the wind tunnel experiments, pyrolysis and flaming combustion samples were collected and their compositions were shown to differ (Weise et al. 2022c). The sampling setup was designed to collect flaming combustion and pyrolysis samples at different locations relative to the flame and the convective plume (Aminfar et al. 2020); the sample inlets for the pyrolysis samples were below the convective plume. Relatively more H2 was observed in the pyrolysis samples. In the oxidising environment, CO2 was the dominant component (relative amounts of 0.93 and 0.95 for pyrolysis and flaming, respectively). The relatively high CO2 fraction is consistent with reported CO2 mass fractions of 0.62–0.72 from pyrolysis studies using the Fire Propagation Apparatus (Tihay et al. 2009b; El Houssami et al. 2016). It is not known if these reported CO2 mass fractions were based on excess concentrations as is standard practice when handling fire emissions. Prior to flame front arrival, maximum radiometric leaf temperatures adjacent to the FTIR probe in the wind tunnel ranged from 406 to 933 K (Supplementary Table A1 in Weise et al. 2022c) which is well-within the temperature range associated with primary degradation of biomass (Di Blasi 2008). While we are confident that our reported measurements resulted primarily from pyrolysis, we are aware of the idea of buoyant turbulent eddies forcing hot gases in front of the flame (Finney et al. 2015) which could have affected the measured composition by the introduction of flame gases. The background-oriented schlieren imagery used to visualise convective heating of the plants in the wind tunnel experiments did not reveal these forward-thrusting turbulent jets (Aminfar et al. 2020) in the no-wind experiments. This imagery viewed the flow field parallel to the flame front (perpendicular to fire spread direction). While the IR imagery taken vertically looking downward at the fuel bed may suggest the presence of the forward-thrusting turbulent jets (Banach et al. 2021, p. 2368), the camera was looking through the slightly tilted flames which appear to be horizontal jets due to the 2D nature of the imagery. While there is much evidence to suggest that mixing of flame gases did not occur or was minimal during the pyrolysis gas sampling in the wind tunnel experiments, the possibility of this mixing cannot be conclusively eliminated. More detailed measurements, imagery and analysis that include local temperature, velocity, and O2 measurements in addition to the current measurements might be able to help in determining the physical processes involved in this pre-flame region.
The one thing that seems clear from the data analysis conducted is that pyrolysis products measured in the bench experiments were much richer in H2 and other hydrocarbons than those in the wind tunnel and field experiments. The small amount of pyrolysis products detected in the wind tunnel and field experiments do not seem to resemble the compositions of similar products in the bench experiments, as indicated by the PCA results. This is likely a result of the instrumentation and methods used in these relatively unique experiments measuring pyrolysis in a wildland flame environment.
Recognising the importance of time in the sequences of chemical reactions that can occur as pyrolysates released into an environment containing O2 gas, it is difficult to confidently state that the gas samples represent only that stage in the sequences of reactions. This is due in part to the sampling method used for the field experiments (canister samples, static FTIR samples). In the wind tunnel experiments, however, a subset of the FTIR samples were dynamic samples meaning that one could observe the evolution of the gas mixture over time (Banach et al. 2021). As seen in Fig. 6 and explained in (Banach et al. 2021), the dynamic FTIR data were synchronised with thermal imagery of the fuel bed, which was used to identify the different stages of pyrolysis and combustion. Such a temporal series of gas composition has several potential uses. Using CoDA techniques, empirical models of the change in the gas composition could be derived and used for an improved representation of pyrolysis and combustion processes in physical models (e.g. Borujerdi et al. 2022). The temporal series could be compared with the static samples to locate the time step where the dynamic composition is closest to a static sample using some suitable multidimensional distance approach.
Burn 87 from project RC2640, inkberry with longleaf pine needles: (a) CO and CO2 spectral profile from 2250 to 2000 cm−1. Purple traces indicate the ambient stage, green and orange traces indicate the pre-combustion/pyrolysis stage, red spectral traces indicate the flaming stage and blue traces indicate smouldering. (b) Largely C2H4 (ethylene)spectral waterfall plot from 1000 to 900 cm−1 with accompanying C2H4 reference spectrum is the black trace. Spectra are recorded/produced every 0.79 s (Banach et al. 2021).
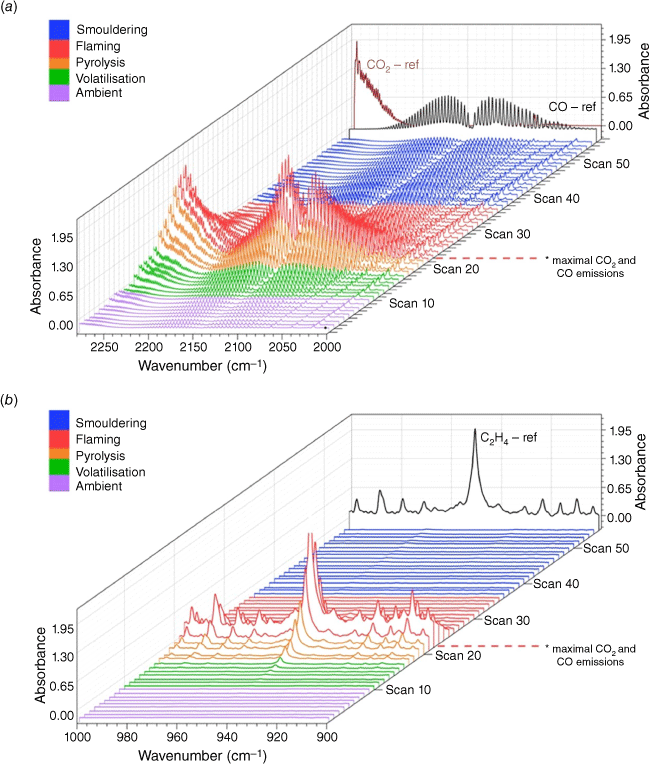
In physical models, the gas composition from a non-oxidising environment can be the starting point to represent the pyrolysis gas mixture and to predict the evolution of the gas mixture to the point of ignition. However, possible modifications to the modelling may be needed due to oxidative pyrolysis. The gas composition from the oxidising environment could serve as validation data for a physical model describing pyrolysis in an oxidising environment. As the composition evolves in time, identifying the time step in the simulation where the multidimensional distance between the modelled composition and the measured composition is minimised for a static FTIR sample (Banach et al. 2021) could prove to be informative. If a dynamic gas composition was available, such a comparison would be between the modelled and observed time sequences of gas composition. These are just a few possibilities of the future work that needs to be performed to support the continued use of non-oxidising environment pyrolysis data as the starting point for physical modelling of fire.
Conclusions
Data from a series of carefully controlled experiments measuring the composition of pyrolysis gases in non-oxidising and oxidising environments, determined using gas chromatography and FTIR spectroscopy that have been previously reported, were analysed within a compositional data analysis framework. Principal component analysis, canonical discriminant analysis and permutational analysis of variance showed that the subcomposition of gases (H2, CO, CO2, CH4, phenol, and other gases) common to all three experiments differed significantly between the non-oxidising flat-flame burner environment and the oxidising environments of small fires in a wind tunnel and in prescribed burns at Ft. Jackson, SC, US. Results seem to indicate the need for more fundamental research on the early time-dependent pyrolysis of vegetation in the presence of oxygen in an environment where a flame is the primary heat source rather than using a bulk composition for the entire pyrolysis process taken from non-oxidising experiments. The complete composition of measured gases resulting from pyrolysis of live foliage from common shrub and tree species in the southeastern United States is available to be used in physically based models of fire to model pyrolysis.
Data availability
The data are available through the WFSI Data Portal (Weise et al. 2024a, 2024b, 2024c).
Conflicts of interest
Andrew T. Hudak and Bret Butler are Associate Editors of the International Journal of Wildland Fire. Bret Butler is also a member of the Editorial Advisory Committee; both were blinded from the peer-review process for this paper. The authors declare no other conflicts of interest.
Declaration of funding
This work was supported by the DOD/DOE/EPA Strategic Environmental Research and Development Program project RC-2640. JPA was supported by the project PID2021-123833OB-I00, funded by the Spanish Ministry of Science and Innovation (MCIN/AEI/10.13039/501100011033) and by ERDF A way of making Europe. USDA Forest Service annual research appropriations provided in-kind support from 2016 to 2022.
References
Aitchison J, Greenacre M (2002) Biplots of compositional data. Journal of the Royal Statistical Society: Series C (Applied Statistics) 51, 375-392.
| Crossref | Google Scholar |
Aminfar A, Cobian-Iñiguez J, Ghasemian M, Rosales Espitia N, Weise DR, Princevac M (2020) Using background-oriented schlieren to visualize convection in a propagating wildland fire. Combustion Science and Technology 192, 2259-2279.
| Crossref | Google Scholar |
Amini E, Safdari M-S, DeYoung JT, Weise DR, Fletcher TH (2019a) Characterization of pyrolysis products from slow pyrolysis of live and dead vegetation native to the southern United States. Fuel 235, 1475-1491.
| Crossref | Google Scholar |
Amini E, Safdari M-S, Weise DR, Fletcher TH (2019b) Pyrolysis kinetics of live and dead wildland vegetation from the southern United States. Journal of Analytical and Applied Pyrolysis 142, 104613.
| Crossref | Google Scholar |
Amini E, Safdari M-S, Johnson N, Weise DR, Fletcher TH (2021) Pyrolysis kinetics of wildland vegetation using model-fitting methods. Journal of Analytical and Applied Pyrolysis 157, 105167.
| Crossref | Google Scholar |
Anderson MJ (2001) A new method for non-parametric multivariate analysis of variance. Austral Ecology 26, 32-46.
| Crossref | Google Scholar |
Anderson MJ (2017) Permutational Multivariate Analysis of Variance (PERMANOVA). In ‘Wiley StatsRef: Statistics Reference Online’. (Eds N Balakrishnan, T Colton, B Everitt, W Piegorsch, F Ruggeri, JL Teugels) pp. 1–15. (John Wiley & Sons, Ltd: Chichester, UK) 10.1002/9781118445112.stat07841
Andreae MO, Browell EV, Garstang M, Gregory GL, Harriss RC, Hill GF, Jacob DJ, Pereira MC, Sachse GW, Setzer AW, Dias PLS, Talbot RW, Torres AL, Wofsy SC (1988) Biomass-burning emissions and associated haze layers over Amazonia. Journal of Geophysical Research 93, 1509.
| Crossref | Google Scholar |
Banach CA, Bradley AM, Tonkyn RG, Williams ON, Chong J, Weise DR, Myers TL, Johnson TJ (2021) Dynamic infrared gas analysis from longleaf pine fuel beds burned in a wind tunnel: observation of phenol in pyrolysis and combustion phases. Atmospheric Measurement Techniques 14, 2359-2376.
| Crossref | Google Scholar |
Bandeen-Roche K (1994) Resolution of additive mixtures into source components and contributions: a compositional approach. Journal of the American Statistical Association 89, 1450-1458.
| Crossref | Google Scholar |
Benjamini Y, Hochberg Y (1995) Controlling the false discovery rate: a practical and powerful approach to multiple testing. Journal of the Royal Statistical Society Series B (Methodological) 57, 289-300.
| Crossref | Google Scholar |
Borujerdi PR, Shotorban B (2022) Pyrolysis and combustion characteristics of leaf-like fuel under convection and radiation heating. Combustion Science and Technology 194, 2558-2579.
| Crossref | Google Scholar |
Borujerdi PR, Shotorban B, Mahalingam S, Weise DR (2019) Modeling of water evaporation from a shrinking moist biomass slab subject to heating: Arrhenius approach versus equilibrium approach. International Journal of Heat and Mass Transfer 145, 118672.
| Crossref | Google Scholar |
Borujerdi PR, Shotorban B, Mahalingam S (2020) A computational study of burning of vertically oriented leaves with various fuel moisture contents by upward convective heating. Fuel 276, 118030.
| Crossref | Google Scholar |
Borujerdi PR, Shotorban B, Mahalingam S, Weise DR (2022) Influence of pyrolysis gas composition and reaction kinetics on leaf-scale fires. Combustion Science and Technology 1-24.
| Crossref | Google Scholar |
Budiman H, Nuryatini , Zuas O (2015) Comparison between GC-TCD and GC/FID for the determination of propane in gas mixture. Procedia Chemistry 16, 465-472.
| Crossref | Google Scholar |
Butler BW, Cohen J, Latham DJ, Schuette RD, Sopko P, Shannon KS, Jimenez D, Bradshaw LS (2004) Measurements of radiant emissive power and temperatures in crown fires. Canadian Journal of Forest Research 34, 1577-1587.
| Crossref | Google Scholar |
Butler BM, Palarea-Albaladejo J, Shepherd KD, Nyambura KM, Towett EK, Sila AM, Hillier S (2020) Mineral–nutrient relationships in African soils assessed using cluster analysis of X-ray powder diffraction patterns and compositional methods. Geoderma 375, 114474.
| Crossref | Google Scholar | PubMed |
Carvalho ER, Gurgel Veras CA, Carvalho Jr JA (2002) Experimental investigation of smouldering in biomass. Biomass and Bioenergy 22, 283-294.
| Crossref | Google Scholar |
Chen Y, Duan J, Luo Y (2008) Investigation of agricultural residues pyrolysis behavior under inert and oxidative conditions. Journal of Analytical and Applied Pyrolysis 83, 165-174.
| Crossref | Google Scholar |
Clark MM, Fletcher TH, Linn RR (2010) A sub-grid, mixture–fraction-based thermodynamic equilibrium model for gas phase combustion in FIRETEC: development and results. International Journal of Wildland Fire 19, 202-212.
| Crossref | Google Scholar |
Colket MB, Naegeli DW, Dryer FL, Glassman I (1974) Flame ionization detection of carbon oxides and hydrocarbon oxygenates. Environmental Science & Technology 8, 43-46.
| Crossref | Google Scholar |
Countryman CM (1982) Physical characteristics of some northern California brush fuels. General Technical Report PSW-61. (USDA Forest Service, Pacific Southwest Forest and Range Experiment Station: Berkeley, CA). https://doi.org/10.2737/PSW-GTR-61
Countryman CM, Philpot CW (1970) Physical characteristics of chamise as a wildland fuel. Research Paper PSW-66. (USDA Forest Service, Pacific Southwest Forest and Range Experiment Station: Berkeley, CA). Available at https://www.fs.usda.gov/psw/publications/documents/psw_rp066/psw_rp066.pdf
Crutzen PJ, Heidt LE, Krasnec JP, Pollock WH, Seiler W (1979) Biomass burning as a source of atmospheric gases CO, H2, N2O, NO, CH3Cl and COS. Nature 282, 253-256.
| Crossref | Google Scholar |
Darley EF, Burleson FR, Mateer EH, Middleton JT, Osterli VP (1966) Contribution of burning of agricultural wastes to photochemical air pollution. Journal of the Air Pollution Control Association 16, 685-690.
| Crossref | Google Scholar |
DeGroot WF, Shafizadeh F (1983) Influence of inorganic additives on oxygen chemisorption on cellulosic chars. Carbon 21, 61-67.
| Crossref | Google Scholar |
Demirbas A, Arin G (2002) An overview of biomass pyrolysis. Energy Sources 24, 471-482.
| Crossref | Google Scholar |
Di Blasi C (2008) Modeling chemical and physical processes of wood and biomass pyrolysis. Progress in Energy and Combustion Science 34, 47-90.
| Crossref | Google Scholar |
Di Blasi C, Signorelli G, Portoricco G (1999) Countercurrent fixed-bed gasification of biomass at laboratory scale. Industrial & Engineering Chemistry Research 38(7), 2571-2581.
| Crossref | Google Scholar |
Dimitrakopoulos AP (2001) Thermogravimetric analysis of Mediterranean plant species. Journal of Analytical and Applied Pyrolysis 60, 123-130.
| Crossref | Google Scholar |
Egozcue JJ, Pawlowsky-Glahn V (2005) Groups of parts and their balances in compositional data analysis. Mathematical Geology 37, 795-828.
| Crossref | Google Scholar |
Egozcue JJ, Pawlowsky-Glahn V, Gloor GB (2018) Linear association in compositional data analysis. Austrian Journal of Statistics 47(1), 3-31.
| Crossref | Google Scholar |
El Houssami M, Thomas JC, Lamorlette A, Morvan D, Chaos M, Hadden R, Simeoni A (2016) Experimental and numerical studies characterizing the burning dynamics of wildland fuels. Combustion and Flame 168, 113-126.
| Crossref | Google Scholar |
Engle M, Martín-Fernández JA, Geboy N, Olea RA, Peucker-Ehrenbrink B, Kolker A, Krabbenhoft D, Lamothe P, Bothner M, Tate M (2011) Source apportionment of atmospheric trace gases and particulate matter: comparison of log-ratio and traditional approaches. In ‘Proceedings of the 4th International Workshop on Compositional Data Analysis’, Girona, Spain. 10. (Universitat de Girona: Girona, Spain). Available at http://hdl.handle.net/10256/13626
EPA (1999) Compendium Method TO-14A Determination of volatile organic compounds (VOCs) in ambient air using specially prepared canisters with subsequent analysis by gas chromatography. In ‘Compendium of Methods for the Determination of Toxic Organic Compounds in Ambient Air’. p. 86. (Environmental Protection Agency: Cincinnati, OH, USA) Available at https://www.epa.gov/sites/production/files/2019-11/documents/to-14ar.pdf
Finney MA, Cohen JD, Forthofer JM, McAllister SS, Gollner MJ, Gorham DJ, Saito K, Akafuah NK, Adam BA, English JD (2015) Role of buoyant flame dynamics in wildfire spread. Proceedings of the National Academy of Sciences 112, 201504498.
| Crossref | Google Scholar | PubMed |
Fletcher TH, Pickett BM, Smith SG, Spittle GS, Woodhouse MM, Haake E, Weise DR (2007) Effects of moisture on ignition behavior of moist California chaparral and Utah leaves. Combustion Science and Technology 179, 1183-1203.
| Crossref | Google Scholar |
Frandsen WH (1991) Burning rate of smoldering peat. Northwest Science 65, 166-172.
| Google Scholar |
Friendly M, Fox J (2021) Candisc: visualizing generalized canonical discriminant and canonical correlation analysis. Available at https://CRAN.R-project.org/package=heplots
Gibergans-Baguena J, Hervada-Sala C, Jarauta-Bragulat E (2020) The quality of urban air in Barcelona: a new approach applying compositional data analysis methods. Emerging Science Journal 4, 113-121.
| Crossref | Google Scholar |
Greenacre M (2020) Amalgamations are valid in compositional data analysis, can be used in agglomerative clustering, and their logratios have an inverse transformation. Applied Computing and Geosciences 5, 100017.
| Crossref | Google Scholar |
Grieco EM, Baldi G (2011) Predictive model for countercurrent coal gasifiers. Chemical Engineering Science 66(23), 5749-5761.
| Crossref | Google Scholar |
Grishin A, Perminov V (1998) Mathematical modeling of the ignition of tree crowns. 34, 378-386.
| Crossref | Google Scholar |
Herzog MM, Hudak AT, Weise DR, Bradley AM, Tonkyn RG, Banach CA, Myers TL, Bright BC, Batchelor JL, Kato A, Maitland JS, Johnson TJ (2022) Point cloud based mapping of understory shrub fuel distribution, estimation of fuel consumption and relationship to pyrolysis gas emissions on experimental prescribed burns. Fire 5, 118.
| Crossref | Google Scholar |
Hillado CJ (1977) The correlation of laboratory test results with behavior in real fires. Journal of Fire and Flammability 8, 202-209.
| Google Scholar |
Hillier JL, Fletcher TH, Solum MS, Pugmire RJ (2013) Characterization of macromolecular structure of pyrolysis products from a Colorado Green River oil shale. Industrial & Engineering Chemistry Research 52, 15522-15532.
| Crossref | Google Scholar |
Hough WA (1969) Caloric value of some forest fuels of the southern United States. Research Note SE-120. (USDA Forest Service, Southeastern Forest Experiment Station: Asheville, NC). Available at https://research.fs.usda.gov/treesearch/2778
Hudak AT, Kato A, Bright BC, Loudermilk EL, Hawley C, Restaino JC, Ottmar RD, Prata GA, Cabo C, Prichard SJ, Rowell EM, Weise DR (2020) Towards spatially explicit quantification of pre- and postfire fuels and fuel consumption from traditional and point cloud measurements. Forest Science 66, 428-442.
| Crossref | Google Scholar |
Jarauta-Bragulat E, Hervada-Sala C, Egozcue JJ (2016) Air Quality Index revisited from a compositional point of view. Mathematical Geosciences 48, 581-593.
| Crossref | Google Scholar |
Jolly WM, Parsons RA, Hadlow AM, Cohn GM, McAllister SS, Popp JB, Hubbard RM, Negron JF (2012) Relationships between moisture, chemistry, and ignition of Pinus contorta needles during the early stages of mountain pine beetle attacked. Forest Ecology and Management 269, 52-59 ked.k.
| Crossref | Google Scholar |
Leroy V, Cancellieri D, Leoni E (2006) Thermal degradation of ligno-cellulosic fuels: DSC and TGA studies. Thermochimica Acta 451, 131-138.
| Crossref | Google Scholar |
Linn RR, Goodrick SL, Brambilla S, Brown MJ, Middleton RS, O’Brien JJ, Hiers JK (2020) QUIC-fire: A fast-running simulation tool for prescribed fire planning. Environmental Modelling & Software 125, 104616.
| Crossref | Google Scholar |
Lovell D, Pawlowsky-Glahn V, Egozcue JJ, Marguerat S, Bähler J (2015) Proportionality: a valid alternative to correlation for relative data. PLoS Computational Biology 11(3), e1004075.
| Crossref | Google Scholar | PubMed |
Maschio G, Koufopanos C, Lucchesi A (1992) Pyrolysis, a promising route for biomass utilization. Bioresource Technology 42, 219-231.
| Crossref | Google Scholar |
Matt FJ, Dietenberger MA, Weise DR (2020) Summative and ultimate analysis of live leaves from southern US forest plants for use in fire modeling. Energy & Fuels 34, 4703-4720.
| Crossref | Google Scholar |
McGrattan K, McDermott R, Vanella M, Mueller E, Hostikka S, Floyd J (2023) ‘Fire Dynamics Simulator Technical Reference Guide Volume 2: Verification’. Special Publication 1018–2. (US Department of Commerce, National Institute of Standards and Technology) 10.6028/NIST.SP.1018
McKenzie LM, Hao WM, Richards GN, Ward DE (1995) Measurement and modeling of air toxins from smoldering combustion of biomass. Environmental Science & Technology 29, 2047-2054.
| Crossref | Google Scholar | PubMed |
Mell W, Maranghides A, McDermott R, Manzello SL (2009) Numerical simulation and experiments of burning douglas fir trees. Combustion and Flame 156, 2023-2041.
| Crossref | Google Scholar |
Moore RK, Dietenberger MA, Mann DH, Lebow PK, Weise DR (2021) Utilizing two-dimensional gas chromatography time of flight mass spectrometry (GCxGC ToFMS) to characterize volatile products from pyrolysis of living vegetation foliage. BioResources 17, 862-889.
| Crossref | Google Scholar |
Morvan D, Dupuy JL (2001) Modeling of fire spread through a forest fuel bed using a multiphase formulation. Combustion and Flame 127, 1981-1994.
| Crossref | Google Scholar |
Neves D, Thunman H, Matos A, Tarelho L, Gómez-Barea A (2011) Characterization and prediction of biomass pyrolysis products. Progress in Energy and Combustion Science 37, 611-630.
| Crossref | Google Scholar |
Oksanen J, Blanchet FG, Friendly M, Kindt R, Legendre P, McGlinn D, Minchin PR, O’Hara RB, Simpson GL, Solymos P, Stevens MHH, Szoecs E, Wagner H (2020) vegan: Community Ecology Package. Available at https://CRAN.R-project.org/package=vegan
Palarea-Albaladejo J, Martín-Fernández JA (2013) Values below detection limit in compositional chemical data. Analytica Chimica Acta 764, 32-43.
| Crossref | Google Scholar | PubMed |
Palarea-Albaladejo J, Martín-Fernández JA (2015) zCompositions — R package for multivariate imputation of left-censored data under a compositional approach. Chemometrics and Intelligent Laboratory Systems 143, 85-96.
| Crossref | Google Scholar |
Phillips MC, Myers TL, Johnson TJ, Weise DR (2020) In-situ measurement of pyrolysis and combustion gases from biomass burning using swept wavelength external cavity quantum cascade lasers. Optics Express 28, 8680-8700.
| Crossref | Google Scholar | PubMed |
Philpot CW (1970) Influence of mineral content on the pyrolysis of plant materials. Forest Science 16, 461-471.
| Crossref | Google Scholar |
R Core Team (2023) ‘R: A Language and Environment for Statistical Computing.’ (R Foundation for Statistical Computing: Vienna, Austria) Available at https://www.R-project.org/
Rencher AC (1992) Interpretation of canonical discriminant functions, canonical variates, and principal components. The American Statistician 46, 217-225.
| Crossref | Google Scholar |
Rogers JM, Susott RA, Kelsey RG (1986) Chemical composition of forest fuels affecting their thermal behavior. Canadian Journal of Forest Research 16, 721-726.
| Crossref | Google Scholar |
Rothermel RC (1972) ‘A mathematical model for predicting fire spread in wildland fuels.’ Research Paper INT-115. (USDA Forest Service, Intermountain Forest and Range Experiment Station: Ogden, UT) Available at https://www.fs.usda.gov/rm/pubs_int/int_rp115.pdf
Safdari M-S, Rahmati M, Amini E, Howarth JE, Berryhill JP, Dietenberger M, Weise DR, Fletcher TH (2018) Characterization of pyrolysis products from fast pyrolysis of live and dead vegetation native to the Southern United States. Fuel 229, 151-166.
| Crossref | Google Scholar |
Safdari M-S, Amini E, Weise DR, Fletcher TH (2019) Heating rate and temperature effects on pyrolysis products from live wildland fuels. Fuel 242, 295-304.
| Crossref | Google Scholar |
Safdari M-S, Amini E, Weise DR, Fletcher TH (2020) Comparison of pyrolysis of live wildland fuels heated by radiation vs. convection. Fuel 268, 117342.
| Crossref | Google Scholar |
Scharko NK, Oeck AM, Myers TL, Tonkyn RG, Banach CA, Baker SP, Lincoln EN, Chong J, Corcoran BM, Burke GM, Ottmar RD, Restaino JC, Weise DR, Johnson TJ (2019a) Gas-phase pyrolysis products emitted by prescribed fires in pine forests with a shrub understory in the southeastern United States. Atmospheric Chemistry and Physics 19, 9681-9698.
| Crossref | Google Scholar |
Scharko NK, Oeck AM, Tonkyn RG, Baker SP, Lincoln EN, Chong J, Corcoran BM, Burke GM, Weise DR, Myers TL, Banach CA, Griffith DWT, Johnson TJ (2019b) Identification of gas-phase pyrolysis products in a prescribed fire: first detections using infrared spectroscopy for naphthalene, methyl nitrite, allene, acrolein and acetaldehyde. Atmospheric Measurement Techniques 12, 763-776.
| Crossref | Google Scholar |
Senneca O, Chirone R, Salatino P (2004) Oxidative pyrolysis of solid fuels. Journal of Analytical and Applied Pyrolysis 71, 959-970.
| Crossref | Google Scholar |
Shafizadeh F (1982) Introduction to the pyrolysis of biomass. Journal of Analytical and Applied Pyrolysis 3, 283-305.
| Crossref | Google Scholar |
Shafizadeh F, Bradbury AGW (1979) Thermal degradation of cellulose in air and nitrogen at low temperatures. Journal of Applied Polymer Science 23, 1431-1442.
| Crossref | Google Scholar |
Shafizadeh F, Fu YL (1973) Pyrolysis of cellulose. Carbohydrate Research 29, 113-122.
| Crossref | Google Scholar | PubMed |
Shotorban B, Yashwanth BL, Mahalingam S, Haring DJ (2018) An investigation of pyrolysis and ignition of moist leaf-like fuel subject to convective heating. Combustion and Flame 190, 25-35.
| Crossref | Google Scholar |
Sullivan AL (2009a) Wildland surface fire spread modelling, 1990–2007. 1: Physical and quasi-physical models. International Journal of Wildland Fire 18, 349-368.
| Crossref | Google Scholar |
Sullivan AL (2009b) Wildland surface fire spread modelling, 1990–2007. 2: Empirical and quasi-empirical models. International Journal of Wildland Fire 18, 369-386.
| Crossref | Google Scholar |
Sullivan AL (2009c) Wildland surface fire spread modelling, 1990–2007. 3: Simulation and mathematical analogue models. International Journal of Wildland Fire 18, 387-403.
| Crossref | Google Scholar |
Sullivan AL (2017) Inside the inferno: fundamental processes of wildland fire behaviour: part 1: combustion chemistry and heat release. Current Forestry Reports 3, 132-149.
| Crossref | Google Scholar |
Susott RA (1982a) Characterization of the thermal properties of forest fuels by combustible gas analysis. Forest Science 28, 404-420.
| Crossref | Google Scholar |
Susott RA (1982b) Differential scanning calorimetry of forest fuels. Forest Science 28, 839-851.
| Crossref | Google Scholar |
Susott R, Ward DE, Babbitt R, Latham D (1991) The measurement of trace emissions and combustion characteristics for a mass fire. In ‘Global Biomass Burning: Atmospheric, Climatic, and Biospheric Implications’. (Ed. JS Levine) pp. 245–257. (The MIT Press: Cambridge, MA, USA) 10.7551/mitpress/3286.003.0036
Tachajapong W, Lozano J, Mahalingam S, Zhou X, Weise DR (2008) An investigation of crown fuel bulk density effects on the dynamics of crown fire initiation in shrublands. Combustion Science and Technology 180, 593-615.
| Crossref | Google Scholar |
Tihay V, Santoni P-A, Simeoni A, Garo J-P, Vantelon J-P (2009a) Skeletal and global mechanisms for the combustion of gases released by crushed forest fuels. Combustion and Flame 156, 1565-1575.
| Crossref | Google Scholar |
Tihay V, Simeoni A, Santoni P-A, Garo J-P, Vantelon J-P (2009b) A global model for the combustion of gas mixtures released from forest fuels. Proceedings of the Combustion Institute 32, 2575-2582.
| Crossref | Google Scholar |
Torero JL, Gerhard JI, Martins MF, Zanoni MAB, Rashwan TL, Brown JK (2020) Processes defining smouldering combustion: integrated review and synthesis. Progress in Energy and Combustion Science 81, 100869.
| Crossref | Google Scholar |
Ward DE, Susott RA, Kauffman JB, Babbitt RE, Cummings DL, Dias B, Holben BN, Kaufman YJ, Rasmussen RA, Setzer AW (1992) Smoke and fire characteristics for cerrado and deforestation burns in Brazil: BASE-B experiment. Journal of Geophysical Research 97, 14601-14619.
| Crossref | Google Scholar |
Weise DR, Jung H, Palarea-Albaladejo J, Cocker DR (2020a) Compositional data analysis of smoke emissions from debris piles with low-density polyethylene. Journal of the Air & Waste Management Association 70, 834-845.
| Crossref | Google Scholar |
Weise DR, Palarea‐Albaladejo J, Johnson TJ, Jung H (2020b) Analyzing wildland fire smoke emissions data using compositional data techniques. Journal of Geophysical Research: Atmospheres 125, e2019JD032128.
| Crossref | Google Scholar |
Weise DR, Fletcher TH, Johnson TJ, Hao W, Dietenberger MA, Princevac M, Butler BW, McAllister S, O’Brien JJ, Loudermilk EL, Ottmar RD, Hudak AT, Kato A, Shotorban B, Mahalingam S, Mell WE, Boardman CR, Myers TL, Baker SP, Bright BC, Restaino JC (2022a) ‘Fundamental measurements and modeling of prescribed fire behavior in the naturally heterogeneous fuel beds of southern pine forests.’ Final Report RC-2640. (USDA Forest Service, Pacific Southwest Research Station: Albany, CA). Available at https://apps.dtic.mil/sti/pdfs/AD1180629.pdf
Weise DR, Fletcher TH, Safdari M-S, Amini E, Palarea-Albaladejo J (2022b) Application of compositional data analysis to determine the effects of heating mode, moisture status and plant species on pyrolysates. International Journal of Wildland Fire 31, 24-45.
| Crossref | Google Scholar |
Weise DR, Hao WM, Baker S, Princevac M, Aminfar A-H, Palarea-Albaladejo J, Ottmar RD, Hudak AT, Restaino J, O’Brien JJ (2022c) Comparison of fire-produced gases from wind tunnel and small field experimental burns. International Journal of Wildland Fire 31, 409-434.
| Crossref | Google Scholar |
Weise DR, Johnson TJ, Myers TL, Hao WM, Baker S, Palarea-Albaladejo J, Scharko NK, Bradley AM, Banach CA, Tonkyn RG (2023) Comparing two methods to measure oxidative pyrolysis gases in a wind tunnel and in prescribed burns. International Journal of Wildland Fire 32, 56-77.
| Crossref | Google Scholar |
Weise DR, Fletcher T, Safdari M-S, Amini E, Dietenberger MA, Matt FJ (2024a) Pyrolysis gases produced by fast and slow pyrolysis of foliage samples from 15 plants common to the southeastern US coastal plain. WFSI Data Portal. 10.60594/W4WC78
Weise DR, Hao WM, Baker S, Lincoln E, Hudak AT, Bright BC, Princevac M, Aminfar AH, Ottmar RD, O’Brien JJ, Kato A, Batchelor JL, Herzog MM, Chong J, Corcoran BM, Burke GM (2024b) Pyrolysis gases measured by gas chromatography and mass spectrometry from fires in a wind tunnel and at Ft. Jackson, SC. WFSI Data Portal. 10.60594/W4MW22
Weise DR, Johnson TJ, Myers TL, Scharko NK, Bradley AM, Tonkyn RG, Banach CA, Phillips, M (2024c) Pyrolysis gases measured by FTIR in a wind tunnel and at Ft. Jackson, SC. WFSI Data Portal. 10.60594/W41596
Yashwanth BL, Shotorban B, Mahalingam S, Weise DR (2015) An investigation of the influence of heating modes on ignition and pyrolysis of woody wildland fuel. Combustion Science and Technology 187, 780-796.
| Crossref | Google Scholar |
Yashwanth BL, Shotorban B, Mahalingam S, Lautenberger CW, Weise DR (2016) A numerical investigation of the influence of radiation and moisture content on pyrolysis and ignition of a leaf-like fuel element. Combustion and Flame 163, 301-316.
| Crossref | Google Scholar |
Yokelson RJ, Susott R, Ward DE, Reardon J, Griffith DWT (1997) Emissions from smoldering combustion of biomass measured by open-path Fourier transform infrared spectroscopy. Journal of Geophysical Research-Atmospheres 102, 18865-18877.
| Crossref | Google Scholar |
Zhou X, Mahalingam S (2001) Evaluation of reduced mechanism for modeling combustion of pyrolysis gas in wildland fire. Combustion Science and Technology 171, 39-70.
| Crossref | Google Scholar |
Footnotes
1 The use of trade or firm names in this publication is for reader information and does not imply endorsement by the U.S. Department of Agriculture of any product or service.
2 Comas-Cufí M (2024). _coda.plot: Compositional Data Related Plots_. R package version 0.1.7, commit 6c8cf1247d71092eeb96320d9f0b3dad85765f7f, <https://github.com/mcomas/coda.plot>.