Live trial performance of the Australian Fire Danger Rating System – Research Prototype†
S. Grootemaat A C , S. Matthews A D , B. J. Kenny A E , J. W. Runcie A , J. J. Hollis A F * , S. Sauvage B , P. Fox-Hughes
A
B Research Program,
C Present address:
D Present address:
E
F Present address:
Abstract
The Australian Fire Danger Rating System program (AFDRS) has built a new fire danger rating system for Australia. A live trial of the system’s Research Prototype (AFDRSRP), based on fire behaviour thresholds, was run and evaluated between October 2017 and March 2018.
Live trial results are critically analysed, and knowledge gaps and recommendations for future work discussed.
Australian bushfire experts assessed wildfires and prescribed burns across a range of vegetation types and weather conditions. Forecast fire danger ratings calculated using: (1) AFDRSRP; and (2) Forest Fire Danger Index (FFDI) and Grassland Fire Danger Index (GFDI) were compared against ratings derived by expert opinion for each evaluation fire (n = 336).
Overall performance of AFDRSRP was superior to the FFDI/GFDI system (56 vs 43% correct), with a tendency to over-predict rather than under-predict fire potential. AFDRSRP also demonstrated its value to assess fire danger in fuel types not conforming to current grassland or forest models; e.g. for fuels that were grouped to use mallee-heath, spinifex and shrubland fire spread models.
The AFDRSRP live trial was successful, outperforming the existing operational fire danger system.
Identified improvements would further enhance AFDRSRP performance, ensuring readiness for operational implementation.
Keywords: bushfire risk, fire behaviour, fire behaviour models, fire danger forecast, fire management, forecast system, fuel types, system evaluation.
Introduction
Fire danger ratings are a valuable tool for decision making in fire preparedness, fire suppression operations, and prescribed burn planning. Fire danger rating systems are also arguably the most visible medium used by fire and land management agencies worldwide to communicate fire danger to the general public (for example, Deeming et al. 1977; Chandler et al. 1983; Burgan 1988; Willis et al. 2001; Taylor and Alexander 2006).
Fire danger ratings estimate the fire potential at a certain time and place, given the weather and fuel conditions (and other elements, if available, such as ignition likelihood, controllability and safety). During the early development of fire ratings, Du Bois (1914) in the United States of America recognised the need for a systematic approach and concept of estimating fire danger, and the elements affecting it. It was not until the 1930s that the first fire danger meters and methods were developed (Gisborne 1928; Shank 1935; Gisborne 1936a, 1936b). In Australia, fire danger rating estimations have been made since the mid 1930s, starting with the introduction of ‘hazard sticks’ in Western Australia (Wallace 1936; Cromer 1946). This was followed by meteorological fire danger tables (Foley 1947) and ‘difficulty of suppression tables’ in southern Australia (Douglas 1957). See Hollis et al. (2024b) for an extensive overview on fire danger ratings in Australia.
Prior to 2022, when the AFDRS was implemented throughout Australia, fire danger ratings were based on the empirically derived McArthur Forest Fire Danger Index (FFDI) and Grassland Fire Danger Index (GFDI) (McArthur 1967, 1973, 1977). These indices were developed based on empirical studies during the 1950s and 1960s, and were specifically built for dry eucalypt forest (10–20 m tall, with a fine fuel load of 1.25 kg m−2) and open continuous grasslands.
Given that only 7% of Australia’s vegetation can be classified as forest and 61% as grass (Kenny et al. 2024), this means that 32% of the Australian vegetation was potentially misrepresented in the FFDI/GFDI system. Further, in the McArthur system, the FFDI and GFDI do not allow for the wide variation in vegetation across the Australian continent. For example, spinifex hummock grass (Triodia spp.), woodlands and agricultural land (pasture, crops) are all treated as grassland, even though fire behaviour in these fuel types is very different to that in continuous grasslands (Kenny et al. 2024; Cheney et al. 1998; Burrows et al. 2018). While fire behaviour models for fuel types other than forest and grassland have been developed over the past ~25 years (Cruz et al. 2015a), these had not been used to define fire danger ratings in Australia prior to 2022.
With a growing population, and more people living in the wildland–urban or rural–urban interface, Australian communities are at a greater risk to be affected by fire (Gill and Stephens 2009). Also, with more extreme weather events due to climate change, extreme and unseasonal fires are more likely to occur (Williams et al. 2001; Lucas et al. 2007; Pitman et al. 2007; Fox-Hughes et al. 2014). The devastating fires in Queensland, New South Wales, Victoria and the ACT during the 2019/2020 season confirmed this. Therefore, it is of utmost importance (‘national priority’, Cube Group 2015) to be able to forecast the fire danger as accurately as possible.
Prior to 2022, the use of the McArthur fire danger rating system differed slightly from state to state, and the public was mostly unaware of the meaning of the different ratings (Metrix 2019a, 2019b). Consistency in both the use of terminology and the practice of setting the fire danger ratings and declaring total fire bans could improve the efficacy of fire danger ratings as part of a community warning system.
In July 2014, senior fire service officers and the Commonwealth government agreed to develop a new Australian Fire Danger Rating System (AFDRS) that is based on the most current science available. This would benefit a wide range of land managers and fire practitioners, and overall should lead to improved public warning messages (Cube Group 2015). After some previous groundwork, a AFDRSRP was built between April and October 2017 (Kenny et al. 2024). The purpose of the AFDRSRP was to demonstrate that it is feasible to develop a fire danger rating system that is national, modular and open to continual improvement. Please refer to fig. 3 in Hollis et al. (2024b) for the components of the AFDRSRP. More broadly, the architecture of the AFDRS was intended to comprise four modules, as noted in articles accompanying this one: (1) a fuel state editor, allowing fire and land managers to input current fuel information for use in subsequent fire danger calculation; (2) a calculation engine, in which fire danger calculations are made based on forecast weather and fuel state; (3) a fire danger viewer, enabling interactive visualisation of weather and fire danger forecasts, including red flags; and (4) a seasonal outlook module, presenting maps of sub-seasonal to seasonal fire danger forecast information.
Here, we discuss a live trial to assess AFDRSRP performance and reliability during the southern Australian 2017–2018 fire season (October–March), noting that project resource limitations did not permit an extension of the assessment period to include the subsequent northern Australian fire season (generally between May and November). The approach taken was to engage operational staff and volunteers from across Australia to collect observations on real bush fire incidents and prescribed burns. The observed ratings (expert opinion) were then compared to the forecast Fire Behaviour Index (FBI) and ratings as calculated by the AFDRSRP. The dataset was supplemented with data from case study fires. This approach ensured that predictions from the AFDRSRP could be unambiguously compared to expert observation of fire behaviour characteristics. These characteristics in turn could be matched to fire danger ratings from the ratings tables developed earlier. In the absence of widespread objective data on fire behaviour (such as direct radiative measurements of fireline intensity or rate of spread), this was judged to be the best way of clearly and transparently linking model predictions to fire behaviour observations.
As well as providing insight into the performance of the AFDRSRP for significant historical events, the case studies also expanded the number of observations available for extreme fire events – thereby providing a full gamut of events across multiple intensities and fire danger. The performance of the AFDRSRP was subsequently compared to the performance of the McArthur system, based on FFDI and GFDI.
This article is the fourth of a series of five outlining the development and prototype implementation of the Australian Fire Danger Rating System.
Materials and methods
Research prototype specifications
Eight operationally available fire spread models were selected as the core for the AFDRSRP, as described in detail by Kenny et al. (2024). For convenience, they are listed here: (1) Forest; (2) Grassland; (3) Northern Grassland (Savanna); (4) Spinifex; (5) Mallee-Heath; (6) Shrubland; (7) Buttongrass; and (8) Pine. In addition, a non-combustible land cover type was included to account for water bodies, for example. The selection was made based on consultation with experts from the Australian fire community at a science workshop (Sydney, 7 June 2018). Local vegetation types from the Australian mainland and Tasmania were grouped into eight broad fuel types to align with the fire spread models. Since many vegetation types do not have a fire spread model specifically designed for them (e.g. rainforests, arid shrublands, wetlands, rural and urban areas), these vegetation types were assigned to the model with the most similar fuel structure (as per Cruz et al. 2018). Fire Behaviour Indices were derived from each of the fire behaviour models above, mostly based on modelled fire intensity. The exception to this approach was Buttongrass, for which no intensity calculation was available. Instead, a Fire Behaviour Index for Buttongrass was obtained from fire rate of spread calculations.
Taking fire behaviour, operational response and potential consequences into account, thresholds (transition points) for fire danger categories were identified. This is outlined in detail in (Hollis et al. 2024a). For Forest, Grassland, Buttongrass and Pine, six categories of fire danger were identified. Mallee-Heath and Shrubland were assigned five categories, while Northern Grassland (savannah) and Spinifex had four. The categories ranged from ‘mostly self-extinguishing, trouble-free fires’ (Category 1), to fires with ‘rapid fire growth; a high probability of loss of life and property; initial attack success is critical to prevent large fire development; and elevated risk to firefighter safety’ (Category 6). Please note that the descriptions of these categories are, as is fire behaviour, specific to fuel types.
Fire danger rating definition tables were developed that define the characteristics of the fire danger categories (Hollis et al. 2024a). Importantly, these tables were used for the live trial assessment (further discussed below), rather than estimates of other fire characteristics such as fireline intensity or flame length. Calculations were computed daily by the Bureau of Meteorology using gridded forecast weather at hourly intervals at a 1.5-km resolution across Australia. Each grid cell was assigned to one of the eight broad fuel types (Kenny et al. 2024) and with the weather forecast and fuel characteristics combined, fire behaviour metrics such as rate of spread, intensity, flame height and spotting distance were calculated by using the corresponding fire behaviour model. These metrics were subsequently classified into the rating categories as defined by Hollis et al. (2024a). Ratings were then displayed on (1) a static website, updated daily, showing maps and tables of the daily maximum ratings for each jurisdiction and Fire Weather District, and (2) an interactive website that displayed the ratings and fire behaviour metrics as hourly outputs on maps, and as time series plots.
Participants and training
To assess the performance of the AFDRSRP, 71 experts from fire and land management agencies across Australia (Appendix 1) were nominated to take part in the live trial. The intention was to have the experts evaluate the fire danger rating of fire incidents during the 2017–2018 southern fire season and compare their observed ratings with the forecast ratings as calculated by the system. The ratings used in this assessment were those employed more broadly in the AFDRSRP, repeated here for convenience: Category 1–6 for the AFDRSRP, and Low-Moderate, High, Very High, Severe, Extreme and Catastrophic for the FFDI/GFDI. Four members of the AFDRS project team processed the observations received from participants.
Prior to the commencement of the live trial, participants were introduced to the project through a webinar or face-to-face training session that (1) explained the purpose of the project and the design of the AFDRSRP, and (2) demonstrated the use of the interactive visualisation systems. The evaluation of the fire incidents, and the performance assessment of the AFDRSRP, relied critically on the expert opinion of the live trial participants. Because of this, there was potential for bias or variation in opinion depending on the participant’s role, background, experience and training (Plucinski et al. 2012). To quantify the extent of any such variation in expert opinion of fire activity and of AFDRSRP performance, live trial participants were asked to complete evaluations for two fire event scenarios. Details of these two scenarios can be found in Appendix 2. For convenience, the two scenarios are summarised as: (1) an easily managed grass fire with a Category 2 or Low-Moderate rating (as assessed by the project team); and (2) a more complex forest fire with limited available background information with a Category 4 or High fire danger rating. No attempt was made to calibrate or modify expert ratings in light of the results of this scenario exercise, as this was felt likely to introduce the potential for greater error.
Data collection
Live trial participants were asked to put their incident evaluations in a data collection spreadsheet (Appendix 3), which helped to streamline the collation of an observation database. The evaluations included information on the incident such as location, time and date, fire size, fuel type and fire behaviour, but also information such as suppression strategies and potential consequences. Ideally, evaluations were completed within 24 h of a fire incident; otherwise, participants could download the forecast for the current and previous day, which allowed the completion of evaluations at a later time.
In addition to characterising the incident, participants were asked to estimate the performance of the rating systems by comparing the forecast rating with what they observed. Participants provided rating assessments for both the AFDRSRP (Category 1–6) and the McArthur system (Low-Moderate, High, Very High, etc.). This was subsequently used for the performance assessment.
Rating forecasts were used in the evaluation process, rather than observations, because gridded forecast ratings were available at 1.5 km horizontal and hourly temporal resolution across Australia. Observational data, such as from the BARRA reanalysis (Sauvage et al. 2024), was only generally available at a lower horizontal resolution of 12 km. It was felt by the project team that the forecast data in general would provide a better estimate of actual conditions at fire observation locations.
Since limited numbers of observations in Categories 5 and 6 were expected, the database was supplemented with well-documented case studies from significant historical fires. This included incidents at the higher end of fire danger, as required to assess the rating systems at the full potential spectrum of fire danger.
Case studies were chosen from as wide a variety of fuel types as possible, noting the requirement for good documentation of fire, fuel and weather conditions. Thus, in addition to forest and grass fires, case studies included, for example, pine, mallee-heath and heathland fires (Matthews et al. 2018, ch. 9). Case study fires were characterised by both over- and under-prediction at times. The latter occurred especially in periods of extreme fire behaviour outside the bounds of predictability by fire behaviour models (e.g. Waroona fire, Peace et al. (2022)). There is a bias in case selection, which should be noted. Well-documented cases were generally those where fire activity caused significant impacts, whether on communities or assets, and which therefore justified the considerable efforts required for data collection, analysis and documentation.
Data analysis
Database entries were audited to ensure that each incident was consistently reported and that the recorded locations and fuel types were accurate. Where errors were identified, corrections were made where possible or entries were excluded from the analysis.
Multi-category contingency tables were produced to summarise system performance. Apart from the raw numbers in tables, the following five statistical measurements were calculated to evaluate the forecasts:
percentage correct (value between 0 and 100%, higher is better), when the forecast and observed rating were equally matched;
percentage over-predicted (value between 0 and 100%, lower is better), when forecast ratings were higher than the observed ratings, regardless of the extent of the over-prediction (e.g. one or several categories);
percentage under-predicted (value between 0 and 100%, lower is better), when forecast ratings were lower than the observed ratings, regardless of the extent of the under-prediction; and
Peirce skill score* (value between −1 and +1, higher is better). Peirce skill scores represent the fraction of correct forecasts after eliminating those that would be correct due to random change (Peirce 1884).
where K = the number of categories
N = the total number of forecasts
n(Fi, Oj) = the number of forecasts in category i that had observations in category j
N(Fi) = the total number of forecasts in category i
N(Oj) = the total number of observations in category j
(5) Gerrity skill score* (value between −1 and +1, higher is better). Gerrity skill scores reward the ability of a model to correctly forecast rare events, and large forecast errors are penalised more than small forecast errors (Gerrity 1992).
where sij are elements of a scoring matrix given by
(for i = j, diagonal)
(for i ≠ j, off-diagonal)
with the sample probabilities given by
*For a multi-category contingency table:
Observed category | |||||||
---|---|---|---|---|---|---|---|
Forecast category | i, j | 1 | 2 | … | K | Total | |
1 | n(F1, O1) | n(F1, O2) | … | n(F1, O K) | N(F1) | ||
2 | n(F2, O1) | n(F2, O2) | … | n(F2, O K) | N(F2) | ||
… | … | … | … | … | … | ||
K | n(F K, O1) | n(F K, O2) | … | n(F K, O K) | N(F3) | ||
Total | N(O1) | N(O2) | … | N(O K) | N |
To assist with the calculations of these measurements, the ‘verification’ package in R was used (Weather Forecast Verification Utilities, R package ver. 1.42. 2015, R Core Team (2018)).
The analysis, as described above, was used to evaluate overall system performance on different versions of the datasets (e.g. raw data, audited data, with/without case studies). Also, we evaluated the system performance per fuel type, and examined fires with initial attack success. A selection of the results is shown in this paper, the full analysis can be found in Matthews et al. (2019).
Results
Potential observation bias (participants)
Of the 71 registered live trial participants, 21 participants completed scenario 1, and 22 completed scenario 2. Details on these two scenarios are in Appendix 2. There was very little variation in the response to scenario 1. With the exception of two observations, all participants rated the AFDRSRP rating as a Category 2 (Fig. 1a), in agreement with the forecast. Similarly, participants consistently rated the fire as Low-Moderate using the FFDI/GFDI system (Fig. 1b).
Boxplots showing the variation in participant response for two scenarios, (a) for the AFDRSRP, (b) for the FFDI/GFDI system. The boxes represent the inter-quartile range around the median (black bar), the whiskers indicate the range of scores within the first (0–25%) and fourth (75–100%) quartile. Points indicate outliers. For the FFDI/GFDI system: L-M, Low-Moderate; H, High; VH, Very High; S, Severe; E, Extreme; C, Catastrophic.
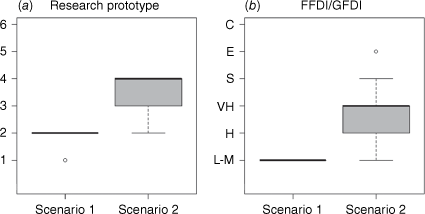
In contrast, there was considerable variation in response to scenario 2 (Fig. 1). While the median response for the AFDRSRP was Category 4 (in agreement with the rating forecast), the observations ranged between 2 and 4 (Fig. 1a). This suggests that participants described the fire of scenario 2 as anything between ‘typical prescribed burning conditions, fires generally easy to suppress’ to a fire where ‘initial attack success is critical to prevent large fire development and defensive strategies are used’ (following Hollis et al. (2024a)). There was even more variation in response to scenario 2 when using the FFDI/GFDI system. Observations varied from Low-Moderate to Extreme, with the median being Very-High, which was a category higher than forecast.
The evaluation of these two scenarios suggests that fairly simple fires, supported with sufficient background information, are likely to have provided consistent observed ratings of the AFDRSRP and FFDI/GFDI system across the dataset. However, more complex fires or fires with minimal supporting information are likely to have resulted in considerable variation in observed ratings, particularly for the FFDI/GFDI system. The clearly defined descriptors of the AFDRSRP, taking fire behaviour, suppression/containment and consequences into account (Hollis et al. 2024a), are likely to have reduced the amount of variation that can be attributed to subjective interpretation.
Summary statistics
During the 2017–2018 southern Australian fire season, observations were collected covering all jurisdictions, all broad fuel types, and all rating categories (Table 1). A total of 264 live trial observations were collected between October and March, most of which came from New South Wales, Western Australia and Victoria (Fig. 2). There were fewer observations from northern Australia (i.e. Northern Territory and Queensland) because the period between October and March spans mostly the wet season there.
Live trial (n = 264) | Case studies (n = 72) | Total (n = 336) | |||
---|---|---|---|---|---|
Jurisdiction | Australian Capital Territory | 6 | 0 | 6 | |
New South Wales | 142 | 14 | 156 | ||
Northern Territory | 1 | 0 | 1 | ||
Queensland | 6 | 9 | 15 | ||
South Australia | 15 | 2 | 17 | ||
Tasmania | 1 | 15 | 16 | ||
Victoria | 42 | 10 | 52 | ||
Western Australia | 51 | 22 | 73 | ||
Broad fuel type (based on fire behaviour models) | Forest | 139 | 48 | 187 | |
Northern Grassland | 18 | 0 | 18 | ||
Grassland | 63 | 14 | 77 | ||
Spinifex | 10 | 0 | 10 | ||
Mallee-Heath | 15 | 0 | 15 | ||
Shrubland | 14 | 4 | 18 | ||
Buttongrass | 0 | 6 | 6 | ||
Pine | 5 | 0 | 5 | ||
Observed rating | Category 1 | 21 | 1 | 22 | |
Category 2 | 88 | 6 | 94 | ||
Category 3 | 75 | 17 | 92 | ||
Category 4 | 64 | 9 | 73 | ||
Category 5 | 15 | 26 | 41 | ||
Category 6 | 1 | 13 | 14 |
Map of observations that were used in the performance assessment. The dataset included 264 live trial observations (black) and 72 case study observations (grey).
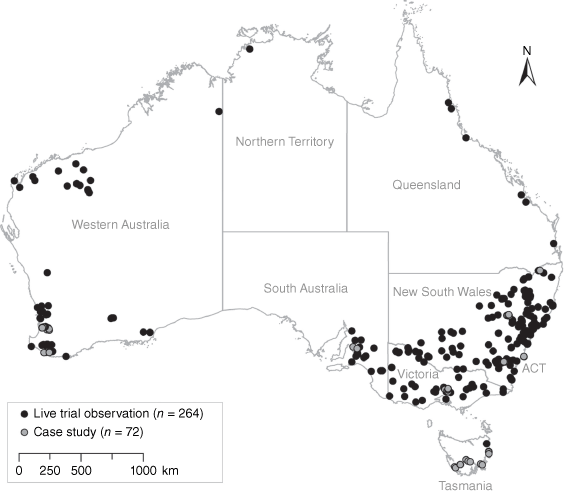
One of the aims of the AFDRSRP was to assess the fire danger across its full categorical spectrum. Therefore, evaluations from nine well-documented and 11 other high impact fires outside the live trial period were added. This provided a larger number of fire danger ratings at the higher end (e.g. Categories 5 and 6). Overall, this supplemented the database with 72 case study observations, leading to a total number of 336 data points for the AFDRSRP performance assessment (Table 1 and Fig. 2).
Many observations came from Forests (n, 1878) and Grasslands (n, 77); while Pine, Buttongrass and Spinifex were less well represented (n ≤ 10, Table 1). As was expected, most fires had an observed fire danger rating of Category 2, 3, or 4, with a lower number of observations at the lower and higher end.
Performance assessment
The overall performance of the AFDRSRP was superior to the FFDI/GFDI system, with 56% of the observations correctly predicted by the AFDRSRP vs 43% for the FFDI/GFDI system (Fig. 3). The AFDRSRP over-predicted more (35 vs 16%), but under-predicted less (9 vs 41%) than the FFDI/GFDI system. While the observations were fairly evenly distributed across the categories (and diagonal axis) for the AFDRSRP (Fig. 3a), in contrast, most of the observations were grouped in the top left corner of the contingency table for the FFDI/GFDI system (Fig. 3b). This is also reflected in the Peirce and Gerrity skill scores, which take into account the distribution of observations, which were higher for the AFDRSRP (0.45 vs 0.23) than the FFDI/GFDI system (0.66 vs 0.42).
Forecast vs observed ratings for all observations (n = 336) for (a) the Research Prototype, and (b) the FFDI/GFDI. Cell values represent the number of observations in each category. Values along the diagonal line (green) represent cases where forecast values matched the observed ratings, and the system was considered to perform correctly. Values under the diagonal represent under-predictions (in purple), and values above the diagonal represent over-predicted fire danger (in orange). L-M, low-moderate; H, high; VH, very high; S, severe; E, extreme; C, catastrophic.
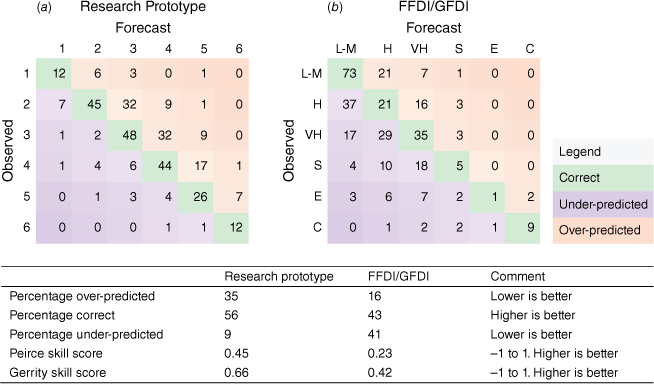
Broad fuel type specific performance
Most of the observations came from forest fires, with 187 observations in total. The percentage of correctly predicted fire danger ratings was higher for the AFDRSRP than for the FFDI/GFDI system (54 vs 46%, medium grey colour in Fig. 4). The AFDRSRP had a higher Peirce skill score as well, 0.43 vs 0.28. Whilst the AFDRSRP tends to over-predict, which may lead to unnecessary total fire bans or crew preparedness, the FFDI/GFDI system under-predicted 35% of the time. This may lead to dangerous conditions, under-preparedness and can put life and properties at risk.
System comparison per fuel type. Percentage under-predicted, correct and over-predicted are given as stacked bar graphs for (a) = AFDRSRP (left bar of the cluster) and (b) = FFDI/GFDI system (right bar of the cluster). Black diamonds represent the Peirce skill score. Number of observations per fuel type are given in brackets.
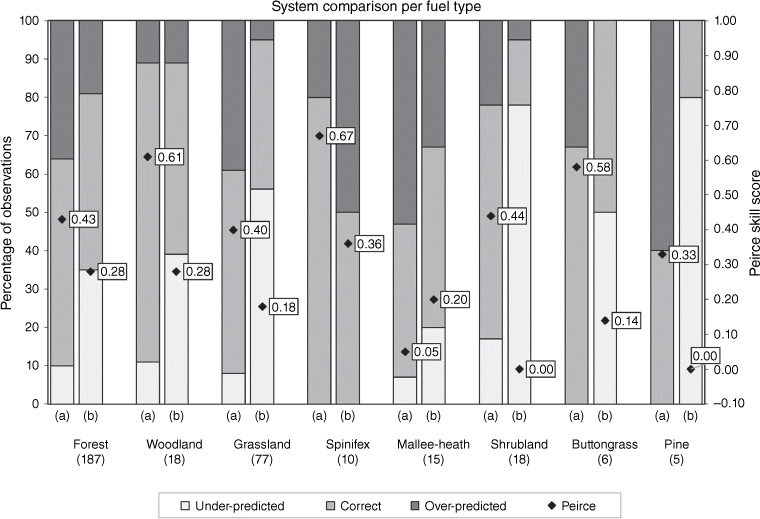
For the fuel types that were grouped to use the northern grassland model (e.g. grassy woodlands, arid woodlands, rural areas), 18 observations were received. The AFDRSRP outperformed the FFDI/GFDI system with 78% of the ratings correctly predicted vs 50% for the FFDI/GFDI system. The Peirce skill score was more than twice as high for the AFDRSRP; i.e. 0.61 vs 0.28, which is a very promising result. Both of the systems over-predicted 11% of the time (darkest grey in Fig. 4), but the FFDI/GFDI system under-predicted more (39%) compared to the AFDRSRP (11%).
A total of 77 observations were assessed for the Grassland fuel type. The percentage of correctly predicted fire danger ratings was higher for the AFDRSRP than for the FFDI/GFDI system (53 vs 39%). The Peirce skill score was more than twice as high for the AFDRSRP, i.e. 0.40 vs 0.18. The AFDRSRP over-predicted in 39% of the observations. This is in contrast with the FFDI/GFDI system that under-predicted the majority of observations (i.e. 56%, Fig. 4). Of note, in the AFDRSRP, the grassland fire spread model was used not just for natural grasslands, but also for crops and pasture, as well as arid areas with ephemeral grasses (e.g. chenopod shrublands). Future improvements may be made when additional fire spread models are developed such as that of Cruz et al. (2020) for cropland fuels.
In contrast with the results of the other fuel types, the AFDRSRP performed slightly worse for Mallee-Heath, with 40% of the fire danger ratings correctly predicted vs 47% for the FFDI/GFDI system. The AFDRSRP over-predicted 53% of the time, and this was reflected in a low Peirce skill score (0.05). The use of the mallee-heath model is further discussed in (Kenny et al. 2024).
The biggest improvement in correctly forecasting fire danger occurred for the Shrubland fuel type; from 17% with the FFDI/GFDI system to 61% with the AFDRSRP. The FFDI/GFDI system under-predicted 78% of the time and this was reduced to 17% with the AFDRSRP (Fig. 4). The skill score confirmed that the FFDI/GFDI system is not appropriate for predicting fire danger rating in Shrubland; the Peirce skill score of 0.00 is the lowest skill score observed in the live trial.
For the other fuel types (Spinifex, Buttongrass and Pine), too few observations were made to achieve any statistically meaningful evaluation (n = 10, 6 and 5, respectively). For completeness, the values are in Fig. 4 (and detailed numbers in Appendix 4). To assess the system performance in these fuel types properly, further observations are needed. Ongoing evaluation is therefore a necessary and ongoing feature of the AFDRS program.
Case studies only
Case studies were included in our performance assessment, next to the observations made by the trial participants, to capture the higher end of the rating system. Eighteen case studies were evaluated and this led to 72 fire danger rating observations. The performance assessment showed that the AFDRSRP is better at predicting the fire danger rating at higher ends of the scale, with correct rating predictions in 61% of the observations compared to 33% for the FFDI/GFDI system (medium grey colour in Fig. 5). This was also reflected in a higher Peirce skill score for the AFDRSRP, 0.48 vs 0.22. The FFDI/GFDI system under-predicted the fire danger rating in 58% of the observations.
Discussion
Improved performance
The AFDRSRP was critically assessed using results from the live trial with observations from all jurisdictions for the duration of the 2017/2018 southern Australian fire season. This demonstrated that the aim of a national system was achieved and that the build of a fire danger rating system based on multiple fire behaviour models for various fuel types, coupled with fuel type mapping and high resolution weather forecasting, is a feasible approach. Small computational changes were made throughout the live trial without affecting the system performance, thereby confirming its modular approach and allowing for continuous improvement – an important design criterion for system development (Jones et al. 2001).
Assessed against expert opinion, the AFDRSRP outperformed the McArthur system overall, for the case study observations, and for most broad fuel types. The observations from the case studies included fire danger ratings at the higher end of the classification; e.g. Category 5 and 6. The assessment of fires with rapid fire growth is crucial because under these conditions the risks of loss and damage to life and property are greatest (Blanchi et al. 2010, 2012). As the AFDRSRP correctly predicted 61% of the case studies, compared to 33% with the FFDI/GFDI system, we affirm the framework and use of the system as a viable replacement.
Despite that the FFDI was specifically designed for forests (McArthur and Dwyer 1958; McArthur 1967) and has been in use for decades, the AFDRSRP more accurately characterised fire danger with the majority of the live trial observations coming from this fuel type (187 out of 336 observations). One of the reasons for this is that the FFDI system assumes a standard fuel load of 1.25 kg m−2, while the AFDRSRP allows for regional variation in fuel inputs. However, there is still room for improvement, given that 36% of the observations of Forest fuels were over-predicted. This could partly be explained by the use of the DEFFM (Vesta) forest fire spread model (Cheney et al. 2012) in conditions it was not designed for, such as the initial build-up phase of fires, or for vegetation types classified as wet sclerophyll forests (Kenny et al. 2024). Ongoing research into improving fire spread models for Australian forests is promising in this regard (e.g. Cruz et al. 2021). The DEFFM/Vesta model does not include fuel availability or rainfall effects, therefore, a drought factor modification was used for the AFDRSRP (Matthews et al. 2019). Including a detailed forest fuel moisture model (e.g. Matthews 2006) could also improve the accuracy of the AFDRS in these fuel types.
The AFDRSRP also outperformed the GFDI, again as judged by expert participants in the project, reflecting the improvements in applying a newer fire spread model (Cheney et al. 1998) as well as an updated curing function (Cruz et al. 2015b). This also demonstrates the value of a modular system that can be adapted to incorporate new research.
Limitations
The live trial relied on the expert opinion of the live trial participants. The accuracy of the rating observations therefore depends on the live trial participant’s experience and area of expertise (Plucinski et al. 2012). While this might lead to participant bias and may affect the reliability of the results (Hirsch and Martell 1996), subjective judgement is an approach commonly used in fire research (Hirsch et al. 1998; Hirsch et al. 2004) and was considered the best option available. During the live trial, everything possible to limit the subjective interpretation was done, such as standardising the available responses, and assessing the potential observation bias by asking the participants to complete two training scenarios. The training scenarios showed that simple fires with adequate information were assessed in a consistent manner while more complex fires, or fires with less information available, led to more variation in observed ratings. The observed ratings for the case studies, based on extensive and detailed information, are probably more accurate and we support the development of well-documented case studies for future important fire events.
The AFDRSRP evaluated the fire danger rating by including fire behaviour, suppression efforts and consequences. This approach is more comprehensive than what has been done in previous studies; e.g. studies that evaluated the predictive ability of fire danger rating systems by using measures such as fire occurrence (Vasilakos et al. 2007; Sebastián-López et al. 2008; Padilla and Vega-García 2011; Eastaugh et al. 2012; Plucinski et al. 2014) or fire area (Viegas et al. 1999; Andrews et al. 2003; Walding et al. 2018). This complexity provided a challenge to critically assess the system as data collection for the AFDRSRP was labour intensive, and data analysis was multi-faceted. However, a comprehensive database focusing on various aspects of fire danger was created that can also be used for future research. We strongly encourage further research into fire behaviour models which may be used to improve the inputs and outputs of the AFDRS.
An additional complexity of the AFDRSRP is the inclusion of high spatial resolution weather forecast data. No attempt was made to disentangle errors in weather forecast information from possible errors in either observations or modelling of fire activity. Difficulties in isolating such sources of error further highlight the value of programs of comprehensive observation of fire events, including accompanying environmental conditions.
Not all broad fuel types were given six fire danger rating categories (Hollis et al. 2024a). Specifically, Spinifex and Northern Grassland did not have a Category 3 nor 6; Mallee-Heath and Shrubland did not have a Category 6. While the thresholds of these categories were based on the best operational and scientific information available (Hollis et al., 2024a), they made statistical analysis difficult especially for ratings around the Category 3 mark because forecast ratings were either correct or out by at least two classes. The absence of Category 6 was less of a problem for the live trial performance assessment, but consistency in the rating categories might be desirable for agencies and public messaging. Also, observed fire danger was not always easy to define as there were several occasions where the subjective nature of the assessment meant that the observed rating would fall in between categories (pers. obs. from trial participants). However, assignment of half-categories was not permitted. Refining the category thresholds, based on the live trial results and ongoing evaluations, is therefore an ongoing objective of the project team.
Time periods chosen for evaluations depended on available information (e.g. fire behaviour observations, linescans, and suppression activities) and was fully determined by the live trial participant. Within the life of an incident one or more time periods could be included for evaluation. Since the case studies used were well documented, up to 10 time periods were included for the same event. We acknowledge the use of multiple time periods from a single fire may introduce bias and pseudo replication (Hurlbert 1984), however these issues were not addressed in detail in this study.
Initial attack (Hirsch and Martell 1996; Plucinski 2013) affected the live trial results (results not shown here, see Matthews et al. (2019) for more information). For example, on a day with elevated fire danger, fire crews may have been on standby and their quick and efficient response could have prevented the development of large fires. The resulting fire behaviour, fire perimeter/area and socio-economic consequences (i.e. asset loss, firefighter safety) would indicate a lower rating (following the definition tables in Hollis et al. (2024a)) even though the potential of these fires could have been much higher (i.e. a higher fire danger rating). Due to this, suppression will be of particular focus for future research by the AFDRS project team.
Recommendations
The build of the AFDRSRP and the results of the live trial identified areas for future improvement. Apart from the suggestions mentioned above, the live trial period was extended so that the dry season in northern Australia could be included. Also, evaluations of the underrepresented fuels (especially Spinifex, Buttongrass and Pine) were targeted during the extension of the live trial throughout the 2018–2019 southern Australian fire season. Evaluation of the AFDRS is an ongoing process and the findings will be used to fine-tune the AFDRS. This work provides a basis for the documentation of these and subsequent upgrades.
To address the over-prediction bias, it will be necessary to revisit the application of some fire behaviour models. Extra attention should be given to the fire build-up phase and initial attack response, topographic effects such as slope and aspect, the role of spotting and application of the fire spread models and AFDRSRP definition tables for less common fuel types. This is further discussed in (Kenny et al. 2024). Any potential biases related to weather inputs are closely monitored by fire weather meteorologists who, as noted above, curate AFDRS forecast output.
The AFDRSRP is intrinsically linked to fire spread models for specific fuel types with each model requiring, to varying degrees of sensitivity (Matthews et al. 2018), a range of fuel input parameters. Consequently, the dependence on consistent and accurate spatial representation of fuel type and characteristics means that continued investment is needed to ensure correct fire danger forecasting. To date, sub-seasonal to seasonal updates of fuel state have occurred to maintain currency of the system and to correct any errors detected.
The AFDRSRP live trial demonstrated promising results. Subsequent developments of the AFDRSRP have also showed improvements for forecasting fire danger across Australia, whether as part of the project work or as new science such as fire behaviour models or system input availability. The AFDRS project has thereby helped, and continues to help, to create a safer environment for the Australian community.
Data availability statement
Fuel data that support this study were obtained from Australian fire agencies by permission/licence. The live trail data that support this study will be shared upon reasonable request to the corresponding author with permission from the Australasian Fire and Emergency Service Authorities Council.
Declaration of funding
The AFDRSRP was funded by grants from the Australian Government’s National Emergency Management Projects programme and the NSW Rural Fire Service’s Bush Fire Risk Mitigation and Resilience Programme. The project also received considerable in-kind support from the Bureau of Meteorology.
Acknowledgements
The development of the AFDRSRP relied on the expertise and participation of many fire managers and researchers and we thank those that were part of early project planning, development and support, particularly from the AFAC Project Office. Special thanks goes out to all operational staff and researchers that helped us design the AFDRSRP, and to the live trial participants for collecting data. A full list of the live trial participants are in Appendix 1.
Author contributions
S. Grootemaat: live trail planning and development, data collation and analysis, original draft. S. Matthews: live trail oversight, planning and development, participant training, data collation and analysis. B. J. Kenny: live trail planning and development, data collation and analysis. J. J. Hollis: live trail planning and development, participant training, data collation and analysis, coordinated manuscript revision, submission process. S. Sauvage: development and support of computer systems, live trail planning and development. P. Fox-Hughes: live trail planning and development, manuscript revision, submission process. All authors contributed to continued AFDRS data collection, writing, review and editing of this paper.
References
Andrews PL, Loftsgaarden DO, Bradshaw LS (2003) Evaluation of fire danger rating indexes using logistic regression and percentile analysis. International Journal of Wildland Fire 12, 213-226.
| Crossref | Google Scholar |
Blanchi R, Lucas C, Leonard J, Finkele K (2010) Meteorological conditions and wildfire-related houseloss in Australia. International Journal of Wildland Fire 19, 914-926.
| Crossref | Google Scholar |
Burrows N, Gill M, Sharples J (2018) Development and validation of a model for predicting fire behaviour in spinifex grasslands of arid Australia. International Journal of Wildland Fire 27, 271-279.
| Crossref | Google Scholar |
Cheney NP, Gould JS, Catchpole WR (1998) Prediction of Fire Spread in Grasslands. International Journal of Wildland Fire 8, 1-13.
| Crossref | Google Scholar |
Cheney NP, Gould JS, McCaw WL, Anderson WR (2012) Predicting fire behaviour in dry eucalypt forest in southern Australia. Forest Ecology and Management 280, 120-131.
| Crossref | Google Scholar |
Cromer DAN (1946) Hygrographic fire danger rating and forecasting. Australian Forestry 10, 52-71.
| Crossref | Google Scholar |
Cruz MG, Gould JS, Alexander ME, Sullivan AL, McCaw WL, Matthews S (2015a) Empirical-based models for predicting head-fire rate of spread in Australian fuel types. Australian Forestry 78, 118-158.
| Google Scholar |
Cruz MG, Gould JS, Kidnie S, Bessell R, Nichols D, Slijepcevic A (2015b) Effects of curing on grassfires: II. Effect of grass senescence on the rate of fire spread. International Journal of Wildland Fire 24, 838-848.
| Crossref | Google Scholar |
Cruz M, Gould J, Hollis J, McCaw W (2018) A Hierarchical Classification of Wildland Fire Fuels for Australian Vegetation Types. Fire 1, 13.
| Crossref | Google Scholar |
Cruz MG, Hurley RJ, Bessell R, Sullivan AL (2020) Fire behaviour in wheat crops – effect of fuel structure on rate of fire spread. International Journal of Wildland Fire 29, 258-271.
| Crossref | Google Scholar |
Eastaugh CS, Arpaci A, Vacik H (2012) A cautionary note regarding comparisons of fire danger indices. Nat. Hazards Earth Syst. Sci. 12, 927-934.
| Crossref | Google Scholar |
Fox-Hughes P, Harris R, Lee G, Grose M, Bindoff N (2014) Future fire danger climatology for Tasmania, Australia, using a dynamically downscaled regional climate model. International Journal of Wildland Fire 23, 309-321.
| Crossref | Google Scholar |
Gerrity Jr JP (1992) A note on Gandin and Murphy’s equitable skill score. Monthly Weather Review 120, 2709-2712.
| Crossref | Google Scholar |
Gill AM, Stephens SL (2009) Scientific and social challenges for the management of fire-prone wildland–urban interfaces. Environmental Research Letters 4, 034014.
| Crossref | Google Scholar |
Gisborne HT (1936b) The principles of measuring forest fire danger. Journal of Forestry 34, 786-793.
| Crossref | Google Scholar |
Hirsch KG, Martell DL (1996) A review of initial attack fire crew productivity and effectiveness. International Journal of Wildland Fire 6, 199-215.
| Crossref | Google Scholar |
Hirsch KG, Corey PN, Martell DL (1998) Using expert judgment to model initial attack fire crew effectiveness. Forest Science 44, 539-549.
| Google Scholar |
Hirsch KG, Podur JJ, Janser RF, McAlpine RS, Martell DL (2004) Productivity of Ontario initial-attack fire crews: results of an expert-judgement elicitation study. Canadian Journal of Forest Research 34, 705-715.
| Crossref | Google Scholar |
Hollis JJ, Matthews S, Anderson WR, Cruz MG, Fox-Hughes P, Grootemaat S, Kenny BJ, Sauvage S (2024a) A framework for defining fire danger rating to support fire management operations in Australia. International Journal of Wildland Fire 33, In press.
| Crossref | Google Scholar |
Hollis JJ, Matthews S, Cruz MG, Fox-Hughes P, Grootemaat S, Heemstra S, Kenny BJ, Sauvage S (2024b) Introduction to the Australian Fire Danger Rating System. International Journal of Wildland Fire 33, In press.
| Crossref | Google Scholar |
Hurlbert SH (1984) Pseudoreplication and the Design of Ecological Field Experiments. Ecological Monographs 54, 187-211.
| Crossref | Google Scholar |
Jones J, Keating B, Porter C (2001) Approaches to modular model development. Agricultural Systems 70, 421-443.
| Crossref | Google Scholar |
Kenny BJ, Matthews S, Sauvage S, Grootemaat S, Hollis JJ, Fox-Hughes P (2024) Australian Fire Danger Rating System: implementing fire behaviour calculations to forecast fire danger in a research prototype. International Journal of Wildland Fire 33, In press.
| Crossref | Google Scholar |
Matthews S (2006) A process-based model of fine fuel moisture. International Journal of Wildland Fire 15, 155-168.
| Crossref | Google Scholar |
Matthews S, Fox-Hughes P, Grootemaat S, Hollis JJ, Kenny BJ, Sauvage S (2018) ‘National Fire Danger Rating System: Research Prototype.’ (NSW Rural Fire Service: Lidcombe, NSW, Australia) Available at https://www.afac.com.au/docs/default-source/afdrs/afdrs_research_prototype_report_2019.pdf?sfvrsn=6&download=false
Metrix (2019b) ‘National Fire Danger Rating System Social Research: Summary NFDRS Research Report Stages 1 to 3.’ (Metrix, Perth, WA). Available at https://www.afac.com.au/docs/default-source/afdrs/afdrs-social-research-report-v1-0.pdf [Verified 08 March 2024]
Padilla M, Vega-García C (2011) On the comparative importance of fire danger rating indices and their integration with spatial and temporal variables for predicting daily human-caused fire occurrences in Spain. International Journal of Wildland Fire 20, 46-58.
| Crossref | Google Scholar |
Peace M, Greenslade J, Ye H, Kepert JD (2022) Simulations of the Waroona fire using the coupled atmosphere–fire model ACCESS-Fire. Journal of Southern Hemisphere Earth Systems Science 72, 126-138.
| Crossref | Google Scholar |
Peirce CS (1884) The numerical measure of the success of predictions. Science 4, 453-454.
| Crossref | Google Scholar | PubMed |
Pitman A, Narisma G, McAneney J (2007) The impact of climate change on the risk of forest and grassland fires in Australia. Climatic Change 84, 383-401.
| Crossref | Google Scholar |
Plucinski MP (2013) Modelling the probability of Australian grassfires escaping initial attack to aid deployment decisions. International Journal of Wildland Fire 22, 459-468.
| Crossref | Google Scholar |
Plucinski MP, McCarthy GJ, Hollis JJ, Gould JS (2012) The effect of aerial suppression on the containment time of Australian wildfires estimated by fire management personnel. International Journal of Wildland Fire 21, 219-229.
| Crossref | Google Scholar |
Plucinski MP, McCaw WL, Gould JS, Wotton BM (2014) Predicting the number of daily human-caused bushfires to assist suppression planning in south-west Western Australia. International Journal of Wildland Fire 23, 520-531.
| Crossref | Google Scholar |
R Core Team (2018) ‘A language and environment for statistical computing.’ (R Foundation for Statistical Computing: Vienna, Austria) Available at https://www.R-project.org/
Sebastián-López A, Salvador-Civil R, Gonzalo-Jiménez J, SanMiguel-Ayanz J (2008) Integration of socio-economic and environmental variables for modelling long-term fire danger in Southern Europe. European Journal of Forest Research 127, 149-163.
| Crossref | Google Scholar |
Sauvage S, Fox-Hughes P, Matthews S, Kenny BJ, Hollis JJ, Grootemaat S, Runcie JW, Holmes A, Harris RMB, Love PT, Williamson G (2024) Australian Fire Danger Rating System research prototype: a climatology. International Journal of Wildland Fire 33, In press.
| Crossref | Google Scholar |
Shank HM (1935) A Measure of Forest Fire Hazard in Central Idaho. Journal of Forestry 33, 389-391.
| Google Scholar |
Taylor SW, Alexander ME (2006) Science, technology, and human factors in fire danger rating: the Canadian experience. International Journal of Wildland Fire 15, 121-135.
| Crossref | Google Scholar |
Vasilakos C, Kalabokidis K, Hatzopoulos J, Kallos G, Matsinos Y (2007) Integrating new methods and tools in fire danger rating. International Journal of Wildland Fire 16, 306-316.
| Crossref | Google Scholar |
Viegas DX, Bovio G, Ferreira A, Nosenzo A, Sol B (1999) Comparative study of various methods of fire danger evaluation in southern Europe. International Journal of Wildland Fire 9, 235-246.
| Crossref | Google Scholar |
Walding NG, Williams HTP, McGarvie S, Belcher CM (2018) A comparison of the US National Fire Danger Rating System (NFDRS) with recorded fire occurrence and final fire size. International Journal of Wildland Fire 27, 99-113.
| Crossref | Google Scholar |
Wallace WR (1936) Forest fire wather research in Western Australia. Australian Forestry 1, 17-24.
| Crossref | Google Scholar |
Williams AAJ, Karoly DJ, Tapper N (2001) The sensitivity of Australian fire danger to climate change. Climatic Change 49, 171-191.
| Crossref | Google Scholar |
Appendix 1.Live trial participants 2017–2018.
Name | Agency | Jurisdiction | |
---|---|---|---|
Chris Condon | ACT Rural Fire Service | ACT | |
Rohan Scott | ACT Rural Fire Service | ACT | |
Brian Levine | Parks and Conservation Service | ACT | |
Belinda Kenny | NSW Rural Fire Service | NSW | |
Jen Hollis | NSW Rural Fire Service | NSW | |
Saskia Grootemaat | NSW Rural Fire Service | NSW | |
Stuart Matthews | NSW Rural Fire Service | NSW | |
Maggie Towers | Bushfires NT | NT | |
Mark Gardener | Bushfires NT | NT | |
Andrew Sturgess | Queensland Fire and Emergency Services | QLD | |
Ben Twomey | Queensland Fire and Emergency Services | QLD | |
Casey Scholten | Queensland Fire and Emergency Services | QLD | |
Russell Stephens-Peacock | Queensland Fire and Emergency Services | QLD | |
Chris White | Queensland National Parks, Sports and Racing | QLD | |
Ian Holloway | Queensland National Parks, Sports and Racing | QLD | |
Jack Hargreaves | Queensland National Parks, Sports and Racing | QLD | |
Anne Mclean | Department of Environment, Water and Natural Resources | SA | |
Damon Ezis | Department of Environment, Water and Natural Resources | SA | |
Simeon Telfer | Department of Environment, Water and Natural Resources | SA | |
Daniel Hoar | Tasmania Fire Service | TAS | |
Jon Marsden-Smedley | University of Tasmania | TAS | |
Musa Kilinc | Country Fire Authority | VIC | |
Tim McKern | Country Fire Authority | VIC | |
Darcy Prior | Department of Environment, Land, Water and Planning | VIC | |
Greg McCarthy | Department of Environment, Land, Water and Planning | VIC | |
Dave Atkins | Department of Biodiversity Conservation and Attractions | WA | |
Dave Turnbull | Department of Biodiversity Conservation and Attractions | WA | |
Glen Daniel | Department of Biodiversity Conservation and Attractions | WA | |
Lachie McCaw | Department of Biodiversity Conservation and Attractions | WA | |
Lance Jackson | Department of Biodiversity Conservation and Attractions | WA | |
Pedro Palheiro | Department of Biodiversity Conservation and Attractions | WA | |
Rob Towers | Department of Biodiversity Conservation and Attractions | WA | |
Ryan Butler | Department of Biodiversity Conservation and Attractions | WA | |
Tammy-Ann Cole | Department of Biodiversity Conservation and Attractions | WA | |
Trevor Howard | Department of Biodiversity Conservation and Attractions | WA | |
Agnes Kristina | Department of Fire and Emergency Services | WA | |
Jackson Parker | Department of Fire and Emergency Services | WA | |
Jonathon Palmer | Department of Fire and Emergency Services | WA | |
Rachael Parkes | Department of Fire and Emergency Services | WA | |
Adrian Allen | Landgate | WA | |
Tim McNaught | Office of Bushfire Risk Management | WA |
Appendix 2.Scenario characteristics for live trial participant’s training exercise. The first scenario represents a simple fire with adequate background information to support decision making. Scenario two represents a more complex fire, with limited supporting information.
Characteristics | Scenario 1 | Scenario 2 | |
---|---|---|---|
Fire type | Wildfire | Wildfire | |
Forecast AFDRS rating (index) | 2 (2) | 4 (89) | |
Forecast FFDI/GFDI (index) | Low-Moderate (GFDI: 1) | High (FFDI: 19) | |
Final fire area | 0.1 ha | 123 ha | |
Broad fuel type | Grassland | Forest | |
Resourcing | 4 personnel (1 tanker) | 44 personnel (13 tankers, 1 aircraft, 1 dozer) | |
Suppression strategies | Direct attack, blacking out | Direct and parallel attack, property protection | |
Consequences | Nil | Infrastructure and private assets threatened |
Appendix 4.Performance table.
AFDRS research prototype | FFDI/GFDI system | ||||||||||
---|---|---|---|---|---|---|---|---|---|---|---|
% over-predicted | % correct | % under-predicted | Peirce skill score | Gerrity skill score | % over-predicted | % correct | % under-predicted | Peirce skill score | Gerrity skill score | ||
Overall | 35 | 56 | 9 | 0.45 | 0.66 | 16 | 43 | 41 | 0.23 | 0.42 | |
Case studies only | 32 | 61 | 7 | 0.48 | 0.46 | 8 | 33 | 58 | 0.22 | 0.48 | |
Forest | 36 | 54 | 10 | 0.43 | 0.66 | 19 | 46 | 35 | 0.28 | 0.45 | |
Northern Grassland | 11 | 78 | 11 | 0.61 | N/A | 11 | 50 | 39 | 0.28 | 0.22 | |
Grassland | 39 | 53 | 8 | 0.40 | 0.60 | 5 | 39 | 56 | 0.18 | 0.36 | |
Spinifex | 20 | 80 | 0 | 0.67 | N/A | 50 | 50 | 0 | 0.36 | N/A | |
Mallee-Heath | 53 | 40 | 7 | 0.05 | N/A | 33 | 47 | 20 | 0.20 | N/A | |
Shrubland | 22 | 61 | 17 | 0.44 | N/A | 6 | 17 | 78 | 0.00 | −0.08 | |
Buttongrass | 33 | 67 | 0 | 0.58 | N/A | 0 | 50 | 50 | 0.14 | N/A | |
Pine | 60 | 40 | 0 | 0.33 | N/A | 0 | 20 | 80 | −0.00 | N/A |