Ingesting GOES-16 fire radiative power retrievals into Warn-on-Forecast System for Smoke (WoFS-Smoke)
Thomas Jones
A
B
C
D
E
F
G
Abstract
The record number of wildfires in the United States in recent years has led to an increased focus on developing tools to accurately forecast their impacts at high spatial and temporal resolutions.
The Warn-on-Forecast System for Smoke (WoFS-Smoke) was developed to improve these forecasts using wildfire properties retrieved from satellites to generate smoke plumes in the system.
The WoFS is a regional domain ensemble data assimilation and forecasting system built around the concept of creating short-term (0–6 h) forecasts of high impact weather. This work extends WoFS-Smoke by ingesting data from the GOES-16 satellite at 15-min intervals to sample the rapidly changing conditions associated with wildfires.
Comparison of experiments with and without GOES-16 data show that ingesting high temporal frequency data allows for wildfires to be initiated in the model earlier, leading to improved smoke forecasts during their early phases. Decreasing smoke plume intensity associated with weakening fires was also better forecast.
The results were consistent for a large fire near Boulder, Colorado and a multi-fire event in Texas, Oklahoma, and Arkansas, indicating a broad applicability of this system.
The development of WoFS-Smoke using geostationary satellite data allows for a significant advancement in smoke forecasting and its downstream impacts such as reductions in air quality, visibility, and potentially properties of severe convection.
Keywords: ensemble data assimilation, fire weather, GOES-R, NWP, probabilistic forecasting, smoke forecasting, weather radar, wildfire.
Introduction
In the past decade, the frequency of high impact wildfires in the western United States has increased significantly due to changes in climate coupled with an increasing population in fire prone regions (e.g. Holden et al. 2018; Halofsky et al. 2020). Examples of recent high impact wildfires include the 2018 Camp Fire in California, the 2021 Marshall Fire in Colorado, and the 2021 Caldor Fire in California, each burning thousands of acres of land, causing millions of dollars in damages and leading to the injury and deaths of multiple people (Chow et al. 2022; Troy et al. 2022). Wildfires also loft large amounts of pollution into the atmosphere, which negatively impacts downstream air quality that further risks human health (e.g. Jaffe et al. 2020; Xu et al. 2020). Lofted smoke aerosols can reach into the mid and upper troposphere and be transported hundreds of kilometres from their source (Lareau et al. 2015, 2018; Lareau and Clements 2016). The presence of these aerosols in the atmosphere, which include large amounts of black carbon, impacts the surrounding environment through blocking solar radiation reaching the surface (e.g. Robock 1988, 1991) and acting as cloud condensation nuclei (CCN) (e.g. Twomey 1974; Fromm et al. 2005, 2006, 2010, 2016). These impacts can occur over timescales from minutes to days. Many forecast systems have been developed that focus on forecasting smoke on daily time scales, but few systems exist that focus on the 0–6 h period. However, this is being remedied with the development of convection allowing models (CAMs) that include the necessary modifications to inject and transport smoke with forecasting its impact on the surrounding environment.
The High-Resolution Rapid Refresh for Smoke (HRRR-Smoke) is a CAM with a 3-km grid spacing and is cycled hourly over a Continental United States (CONUS) domain that includes a smoke aerosol forecasting ability (Benjamin et al. 2016; Ahmadov et al. 2017; Dowell et al. 2022; James et al. 2022). While HRRR-Smoke has been an important smoke forecasting tool, it does have several limitations. Since it currently only uses fire information retrieved from polar orbiting satellites, wildfires that develop and evolve quickly may not be sampled until several hours after the fact (O’Neill et al. 2021; Chow et al. 2022). The next generation HRRR model known as the Rapid Refresh Forecast System (RRFS) is planned to include fire information from geostationary satellites, but the cycling interval remains only hourly. The HRRR and RRFS are both deterministic systems that do not take into account the uncertainties involved in retrieving wildfire properties from satellites and plume injection algorithms. To address these limitations, this work develops an ensemble-based smoke forecasting system that ingests wildfire information at sub-hourly intervals and produces a set of potential forecasts accounting for the measurement and model uncertainties present in the system.
The development of the Warn-on-Forecast System for Smoke (WoFS-Smoke) created a system focused on the 0–6 h forecast period with an emphasis on smoke forecasts from new and rapid evolving wildfires (Jones et al. 2022a, 2022b). WoFS-Smoke is an ensemble data assimilation and forecasting system based on WoFS, which was originally designed to generate probabilistic forecasts of severe convection (Wheatley et al. 2015; Jones et al. 2016, 2020; Skinner et al. 2018). Unique features include the assimilation of radar, geostationary satellite, and conventional observations at 15 min intervals over a limited-area model domain with a grid spacing of 3 km. Probabilistic forecast output is generated in real-time at 5 min intervals out to a period of 3 h or 6 h. The initial version of WoFS-Smoke was an extension of HRRR-Smoke and retained the low-temporal resolution fire radiative power (FRP) retrievals from polar orbiting satellites used to initiate smoke plumes (Jones et al. 2022a). However, the ensemble approach allowed for uncertainties in these retrievals to be taken into account through random perturbations applied to FRP for each member. New probabilistic forecast products such as the probability of total column smoke greater than a specific value and synthetic satellite imagery of smoke were created for the system. However, the limitation of the low-temporal resolution FRP retrievals remained.
This research further extends WoFS-Smoke by replacing the FRP retrievals from polar orbiting satellites with those from the GOES-16 satellite. Retrievals from GOES-16 are available every 5 min allowing for the sampling of newly initiated fires and those whose characteristics change rapidly as a function of time. This new version of WoFS-Smoke uses these data to update fire characteristics in the model at each 15-min cycling interval. This work will compare smoke forecasts from the original version of WoFS-Smoke with the new version that includes the geostationary data to determine how including these data improve overall smoke aerosol forecasts.
Fire power retrievals and smoke plume injection
Fire properties are retrieved from GOES-16 data using a modified version of the Wildfire Automated Biomass Burning Algorithm (WF_ABBA) first developed for polar orbiting and the previous generation of geostationary instruments and updated to use data from the Advanced Baseline Imager (ABI) (Prins and Menzel 1992, 1994; Prins et al. 1998; Csiszar et al. 2014; Giglio et al. 2018) onboard GOES-16. Wildfires generate intense heat plumes that are detectable as elevated brightness temperatures in the shortwave 3.9-μm channel included in several recent polar and geostationary orbiting imagers (Dozier 1981; Weaver et al. 1995, 2004). The WF_ABBA primarily uses the 3.9-μm with the 11.2-μm longwave infra-red channel to identify active fires and determine their sub-pixel fire properties using a dynamic multi-spectral thresholding contextual algorithm. Day time visible (0.64 μm) channel data are used to aid in cloud classification. Sub-pixel wildfire characteristics are retrieved using a two-step process. The first step analyses all pixels and subsets those that satisfy 3.9 and 11.2 μm channel thresholds for potential fires. Initial quality control screening for false and cloud contaminated retrievals is also conducted in this step. For pixels that meet these requirements, sub-pixel estimates of fire size, temperature, and power are derived using the Dozier (1981) technique. The second step loops through the potential fire pixels and classifies the fire type and retrieval confidence level. Finally, a temporal filter is applied at this stage to check for temporal continuity in the retrieval compared to previous times. The final retrieved properties written into the level 2 (L2) Fire Detection and Characterisation (FDC) product include FRP, sub-pixel fire size (FS) and fire temperature (FT) for each full-size pixel. Retrieval uncertainties for these products can be on the order of 50% (Giglio and Kendall 2001) and are also impacted by cloud cover and thick smoke plumes over active fires.
Retrievals over a CONUS domain are generated at 5 min intervals with a nominal resolution of 2 km at nadir with a 4 km2 area. However, pixel size increases as a function of viewing zenith angle (VZA) resulting in a pixel area of ~10 km2 when VZA exceeds 65°. The larger pixels may contain more wildfires than corresponding nadir pixels resulting in a high bias in the FRP retrieval (Li et al. 2019). Li et al. (2019) suggested that an adjustment to FRP would be necessary when VZA > 50° when comparing previous generation GOES retrievals. However, their comparisons using more recent ABI data indicates that the adjustment is smaller than previously estimated (Li et al. 2022). Tests with and without this adjustment were performed in this work, and no significant difference in smoke forecasts was observed for these cases. Thus, it is not used for the forecast output discussed below. The MODIS instruments onboard the Terra and Aqua polar orbiting satellites and the VIIRS instruments onboard the NOAA-20 and Suomi NPP polar orbiting satellites generally have a finer resolution due to observing at much smaller distances (~800 km vs 36,500 km). They are also more sensitive to smaller, cooler wildfires that are undetectable using the ABI. Overall, GOES-ABI FRP retrievals have been shown to have a low bias compared to corresponding MODIS and VIIRS data (Li et al. 2019, 2022). To account for this bias, the adjustment scheme described by Li et al. (2019, 2022) is applied to the raw GOES-ABI FRP retrievals. The adjustment is made using a linear function with correction coefficients previously created using co-located data that are a function of land-surface type. This will reduce the impact of observation biases when comparing WoFS-Smoke forecasts that use polar orbiting or geostationary retrievals.
FRP can be directly linked to the amount of material being burned, which in turn is related to the amount of aerosols injected into the atmosphere (Kaufman et al. 1998a, 1998b; Wooster et al. 2005; Roberts et al. 2005; Freitas et al. 2006, 2007, 2010). GOES-R FRP retrievals are mapped onto the 3-km WoFS grid with biomass burning emissions at each grid point estimated using the parametrisation scheme developed by Freitas et al. (2007). The Freitas method uses a 1D entrainment plume model that ingests model data and fire radiative energy calculated from FRP retrievals at each grid point. Smoke emission parameters are a function of land-surface type including forest, woody savanna, or grasslands. Using these data, vertical velocity within the 1D column is estimated. This estimate takes into account latent heat release from condensed moisture and the entrainment of the pre-existing environment in the upward moving parcel. The derived vertical velocity profile is used to define the plume injection heights, which are used to place smoke aerosols in the model analysis. Once smoke is present in the system, it is allowed to evolve with the surrounding atmospheric environment that is forecast. In this system, the buoyant updraft estimated within the 1D column is not transitioned into WRF. Thus, the vertical transport of aerosols near the fire and the potential development of pyro-cumulus (pyro-Cu) is not well forecast.
WoFS-Smoke
The WoFS is an ensemble-based data assimilation and forecasting system that generates frequent probabilistic forecasts of high impact weather events (Wheatley et al. 2015; Jones et al. 2016; Skinner et al. 2018; Yussouf and Knopfmeier 2019; Jones et al. 2020, 2022a, 2022b). The version of the system used here has a regional domain approximately 900 × 900 km in size centred around the area where high impact weather is expected for a particular day. In real time operation, WoFS is initialised in the mid-morning from initial conditions provided by the 36-member HRRR ensemble system generated by the Global Systems Laboratory (GSL). Hourly boundary conditions are derived from a subset of HRRR ensemble forecasts prior to 2022 and from perturbed Global Ensemble Forecast System (GEFS) forecasts starting in 2022. Horizontal grid-spacing for the WoFS is 3 km using 51 vertical levels and with a model top of ~20 hPa.
Conventional (temperature, humidity, wind, pressure), Doppler radar radial velocity, Multi Radar–Multi Sensor (MRMS) reflectivity, and satellite (GOES-R cloud water path and water vapour channel radiance) data are assimilated at 15 min intervals using the ensemble Kalman filter (EnKF) approach combined with the forward operators within the community Gridpoint Statistical Interpolation (GSI) software (Whitaker et al. 2008; Kleist et al. 2009; Hu et al. 2018). Currently (2023), WoFS uses the Advanced Weather Research and Forecasting model (WRF-ARW) ver. 3.9.1, similar to the version used by GSL for the HRRR ensemble (Skamarock et al. 2008; Powers et al. 2017). WoFS-Smoke extends WoFS by including 2.5 μm diameter particulate matter (PM2.5) as a prognostic variable. Hereafter, PM2.5 aerosols are referred to as ‘smoke’ and are assumed to be only generated from wildfires detected by satellite data. No chemical interactions are allowed and other aerosol types are not included in this system. Radiation feedback is included as part of the Rapid Radiative Transfer Model for Global Climate Models (GCMs) (Iacono et al. 2004) allowing the environmental impacts from solar radiation being blocked by smoke to be taken into account. However, the cloud microphysics scheme (NSSL double-moment) is not linked to smoke aerosol concentrations at this time (Mansell et al. 2010).
At each 15 min data assimilation cycle, the average FRP from the previous 15 min (three retrieval intervals) is calculated and ingested into the new version of WoFS-Smoke. For active wildfires, the new FRP updates the previous FRP at a particular grid point. If the wildfire decreases in intensity such that FRP retrievals are not made over a 15 min period, then the previous FRP is updated with a value of zero. Thus, no additional smoke aerosols are injected into the atmosphere from dead wildfires. This feature is made possible by the consistent time-series of data generated from the GOES satellites. When using only data from polar-orbiting instruments, wildfires may not be sampled for a period of hours, making fire decay rely on empirical functions related to diurnal cycles and precipitation. The new system configuration also has the important advantage of injecting smoke into the model from fires that are only 15 min old. In the existing system, it might take several hours before a wildfire is first sampled by a polar-orbiting instrument. We do not combine retrievals from the GOES ABI and polar-orbiting instruments in the same system. When a wildfire is sampled simultaneously by both platforms, the resulting grid-point FRP estimate could be over-sampled to ones ±15 min around it. Blended products are possible, as shown by Li et al. (2022), and such a product is being utilised in the latest version of the RRFS. However, these products currently lack the temporal resolution and latency requirements for a WoFS-like system. The minimum and maximum thresholds used for FRP retrievals in the system are 10 and 5000 MW, respectively. The former represents the value below which significant amounts of smoke would not be generated and the latter is the maximum value allowed in the plume height model being used here. Corresponding retrieved areal coverages range from <0.01 to >2 km2.
Uncertainties in the FRP retrievals are partially taken into account through applying random perturbations on the order of ±10% to FRP prior to it being ingested into each ensemble member. As a result, each member will contain somewhat different wildfire characteristics that leads to different smoke plume properties. Further ensemble spread is generated by applying random perturbations on the order of 5% to the deterministic HRRR-Smoke forecasts used to generate the smoke initial and boundary conditions for each ensemble member. These perturbations are a layer of random noise that is applied to allow somewhat different smoke conditions to be present in each ensemble member at each cycling interval. If the deterministic HRRR-Smoke output was used as-is, it would significantly inhibit the ensemble spread of later smoke forecasts. Overall, these features allow for the probabilistic smoke forecasts to encompass a range of potential solutions that lie within the realistic envelope of uncertainties.
Two sets of experiments are created for each case. One contains only FRP retrievals from polar orbiting satellites as in the HRRR model and the initial version of WoFS-Smoke (Jones et al. 2022a), which is labelled ‘PSMK’. The second set replaces these retrievals with GOES-16 FRP retrievals and is labelled ‘GSMK’. Available conventional, radar, and satellite observations are assimilated. Smoke and dust plumes are masked in the satellite data and are not assimilated. Radar reflectivity associated with smoke and debris plumes are also not assimilated due to the lack of a suitable forward operator. However, corresponding radial velocity data is being assimilated since particle velocities are still a valid measurement no matter the type of particle being sampled. Verification will be conducted by comparing forecast model output with satellite and radar observations as described by Jones et al. (2022b).
Case study examples
Marshall fire, 30 December 2021
One example of a rapidly evolving extreme wildfire event occurred on 30–31 December 2021 near Boulder, Colorado (CO) known as the Marshall Fire. Existing drought conditions resulting from a long period of above normal temperatures and lower than normal precipitation led to anomalously dry grasslands in the area. On this day, observed relative humidity was ~15% in this area, which was coupled with very strong downslope flows with winds gusting in excess of 50 m s−1 producing the ideal environment for rapid wildfire spread (Fovell et al. 2022). The fire was first reported around 18:00 hours UTC and rapidly intensified with thick smoke blown eastward thereafter. By 20:00 hours UTC, the fire was already causing widespread property damage in the Boulder area. The fire continued into the night time hours and slowly weakened due to a decrease in the downslope winds and fire mitigation efforts. The first evidence of this fire from satellite data occurred at ~18:45 hours UTC with FRP retrievals from GOES-16 being made and smoke becoming evident in the corresponding visible imagery (Fig. 1). Fig. 1 shows GOES-16 visible (0.47 μm) imagery at 30 min intervals from 18:45 hours UTC until 22:30 hours UTC over the area of the fire and corresponding smoke plume. The first evidence of the smoke plume is evident at 18:45 hours UTC, but no FRP retrievals had been made (Fig. 1a). By 19:00 hours UTC, FRP retrievals are present with the initial area of smoke and perhaps a small area of pyro-Cu (Fig. 1b). Smoke spreads rapidly eastward in the next hour and is evident ~50 km downstream from the wildfire by 20:00 hours UTC (Fig. 1d). The fire continues to inject smoke into the atmosphere for the next several hours, resulting in the smoke plume expanding further eastward and growing wider. Throughout this period, several wave-like features are evident in the smoke plume, potentially due to mountain waves in the downslope winds. By 22:30 hours UTC, the smoke plume extends over 150 km downstream of the fire, which is still ongoing (Fig. 1i). Smoke continues to be transported further downstream after 22:30 hours UTC reaching into western Kansas (KS) and Nebraska (NE) by 00:00 hours UTC (not shown).
GOES-16 visible (0.47 μm) imagery between 18:45 hours and 22:30 hours UTC 30 December 2021 showing the evolution of the fire and smoke plume between 1845 UTC to 2230 UTC (a–i). Coloured circles indicate the location of GOES-16 FRP retrievals at each time. Circle size is a representation of the fire size retrieval, though does not correspond to actual fire size.
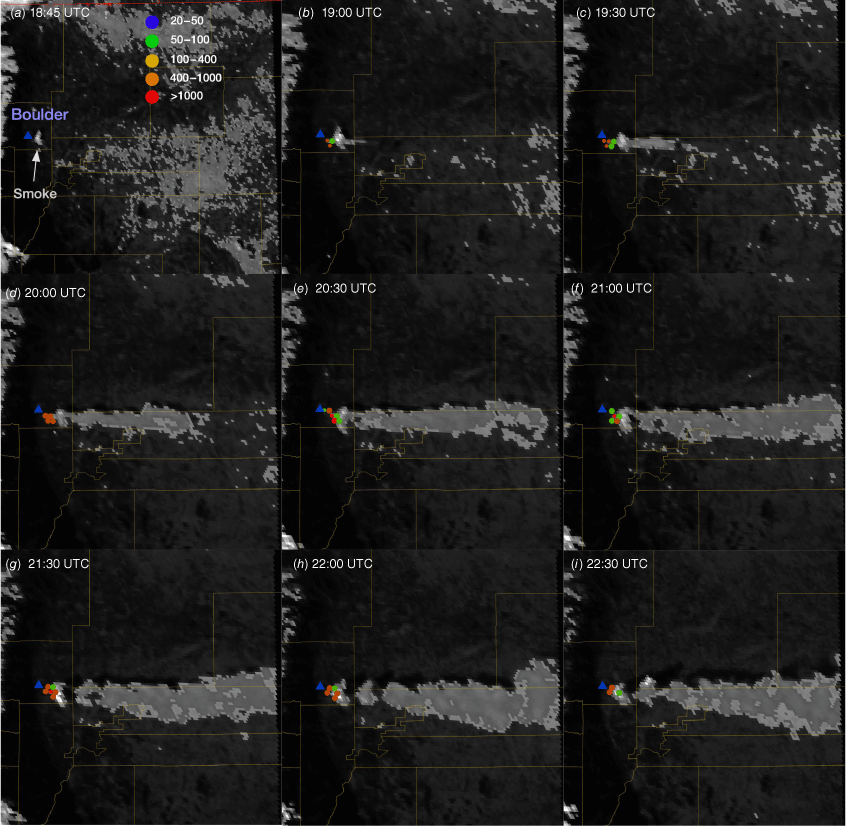
During this period, the characteristics of the fire and the amount of smoke injected at any particular time varies significantly. Fig. 2a shows the spatial distribution of FRP retrievals from GOES-16, Suomi NPP, NOAA-20, and Aqua satellites between 18:00 hours and 03:00 hours UTC on 30–31 December. The MODIS and VIIRS retrievals are essentially co-located, but also cover a limited time window (19:20–21:20 hours UTC). Also evident is the higher resolution of the polar-orbiting instruments compared to the ABI onboard GOES-16. GOES-16 FRP retrievals also appear to extend further north-west of the other retrievals. The observed location of these retrievals may be due to spatial errors introduced from satellite viewing angles >50° as is the case here. Further evidence for this is that the opposite location bias was observed for GOES-17 data, which indicates that future work might need to include a viewing angle adjustment in geolocation that would differ from the intensity adjustment described by (Li et al. 2019, 2022). Otherwise, observed smoke and fire properties from GOES-17 data were very similar to those from GOES-16, so the latter was selected for testing in WoFS-Smoke.
Fire radiative power retrievals from the Terra, Aqua, S-NPP, NOAA-20 and GOES-16 satellites between 18:00 hours and 03:00 hours UTC on 30–31 December 2021. GOES-16 retrievals are plotted at their native resolution of 2 km, with data from the other sensors having a finer resolution (a). The right column shows the sum (in GW) for all retrievals within a 5 min window from each satellite in this domain over the same time period (b).
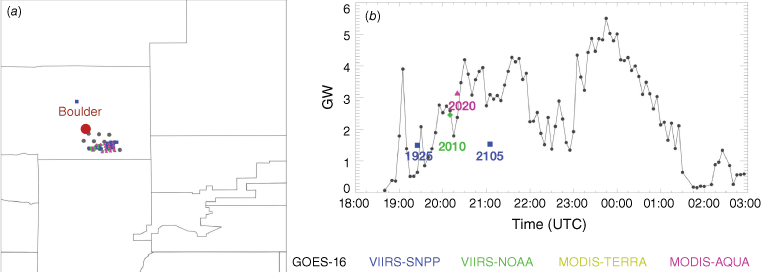
The time series of accumulated FRP at 5 min intervals for these retrievals show the rapid increase in fire intensity from its first detection at 18:45 hours UTC through approximately 21:00 hours UTC (Fig. 2b). FRP retrievals decrease in intensity between 22:00 hours and 23:00 hours UTC before increasing again between 23:00 hours and 00:00 hours UTC. After 00:00 hours UTC, overall fire intensity decreases substantially by 01:30 hours UTC and continues at minimal levels thereafter. The intensity anomaly between 22:00 hours and 23:00 hours UTC is also present on GOES-17 data, so it is likely not a viewing angle artefact or terrain. However, blockage of the fire by thick smoke and/or pyro-Cu in this period cannot be ruled out. The fire was only sampled four times from polar orbiting sensors in this period and no temporal trends in fire characteristics can be inferred from them.
Ensemble mean total column smoke forecasts from both WoFS-Smoke experiments valid at 22:00 hours UTC show the large impact of increasing the frequency of ingesting FRP data (Fig. 3). Forecast initiation times from 19:00, hours 19:30 hours, 20:00 hours, and 21:00 hours UTC are compared to show how the forecast changes as more FRP data are used. For PSMK, ensemble mean smoke >5 mg m−2 was not forecast prior to initiation times of 20:00 hours UTC as no FRP retrievals were present in the system. For the 20:00 hours and 21:00 hours UTC initiation times, the smoke plume is much better forecast by PSMK, primarily due to the retrievals from the S-NPP overpass at 19:25 hours UTC and NOAA-20 and Aqua overpasses at 20:10 hours and 20:20 hours UTC (Fig. 2b).
Forecast ensemble mean total-column smoke from PSMK and GSMK experiments valid at 22:00 hours UTC from forecasts initiated at 19:00 hours (a, b), 19:30 hours (c, d), 20:00 hours (e, f), and 21:00 hours UTC (g, h).
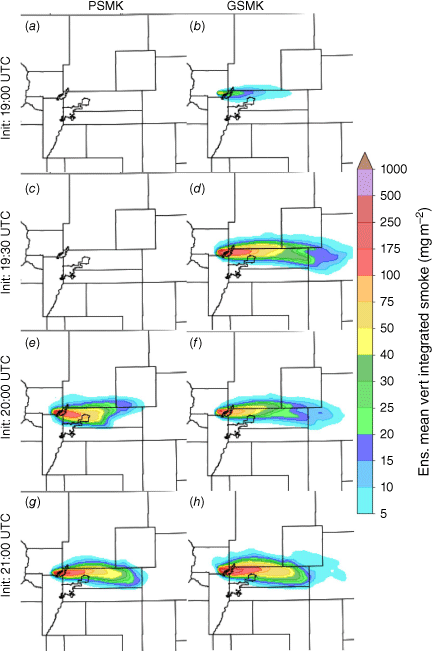
The advantage of ingesting GOES-16 FRP retrievals is clearly evident with GSMK forecasting the 22:00 hours UTC smoke plume from forecast initiation times as early as 19:00 hours UTC. The fire at 19:00 hours UTC is still small; thus, the forecast smoke is under-forecasted (Fig. 3b). By 19:30 hours UTC, the fire has grown in intensity and an additional two cycles of ingesting FRP results in a very large smoke plume being forecast by 22:00 hours UTC, which is broadly consistent with visible imagery at this time (Figs 1h, 3d). The 20:00 hours and 21:00 hours UTC forecasts from GSMK are generally similar to those from PSMK, with a couple of exceptions (Fig. 3e–h). The smoke plume in GSMK extends a little further east, which is consistent with observations. The width of the ensemble smoke plume is somewhat greater, which is a reflection of additional spread in GSMK due to more variations for ingested FRP and the surrounding environment. Overall, the similarity of the forecasts after 20:00 hours UTC indicates that the FRP retrievals at similar times from polar-orbiting satellites and GOES-16 are consistent and being treated equally by the model. Recall that GSMK does not include the polar-orbiting retrievals since they would duplicate the GOES-16 data. For forecasts initiated after 00:00 hours UTC, differences increase again as the FRP data in PSMK becomes less representative of observations (not shown).
Quantitative comparison of these forecasts is difficult, but radar data can provide some information on the 3D plume characteristics from its observations of lofted debris which are large enough to be detected from weather radars. Melnikov et al. (2009), Jones and Christopher (2009); Jones et al. (2009, 2010a, 2010b); Zrnic et al. (2020) provide an in-depth analysis of the radar characteristics of wildfire related debris plumes. In summary, precipitation radars are sensitive to particles of debris lofted into the atmosphere with the smoke aerosols. Since debris particles are larger and heavier than aerosols, they should fall out of the atmosphere quicker. Thus, radar observations can provide a lower bound for the expected smoke aerosol distribution and maximum height. Fig. 4 shows a west-east cross-section of MRMS radar reflectivity 1 km above ground level (AGL) along the plume at 22:00 hours. Reflectivity from debris is evident over 4 km above the surface (6 km above sea level) and extends >100 km downstream from the source fire. The greatest reflectivity (>30 dBZ) occurs nearest the fire and in the lowest 1 km above the surface (Fig. 4b). As debris fall out of the atmosphere, reflectivity decreases significantly further east. One interesting feature apparent from reflectivity observations is the wave-like nature of the plume also evident in the visible imagery. Corresponding cross-sections of forecast smoke valid at 22:00 hours from an initiation time of 21:00 hours UTC show the presence of smoke within much of the same region as radar detected debris, but the details are somewhat different (Fig. 4c–f). Maximum smoke concentrations are located at the surface, and at ~0.5 km above the surface. The latter corresponds to the injection height derived from the Freitas algorithm at this time. Overall forecast smoke heights are actually lower than the corresponding debris detections from radar. Since debris is much larger and heavier than smoke aerosols, it would be expected that smoke would be lofted higher into the atmosphere. The under-estimation of smoke height has been observed in previous WoFS-Smoke studies (Jones et al. 2022a, 2022b), and is due in part to the lack of buoyant updrafts originating from the fires being included in the model analysis. Work is ongoing to add this capability to future versions of the system as the underlying code base does support this capability to some extent. The wave features also present in observations do not appear to be forecast. Differences between PSMK and GSMK forecast smoke are relatively small at this time with only small variations evident since fire information in both is relatively current.
MRMS Reflectivity at 1 km (AGL) from lofted debris at 22:00 hours UTC on 30 December (a). West-to-east cross-section of reflectivity along the debris plume with distance in km from the origin provided (b). Heights are given in km above sea-level with terrain masked. Ensemble mean 1-h forecast of total column smoke valid at 22:00 hours UTC from PSMK and GSMK (c, e). Corresponding cross-sections of forecast smoke along the same path at the radar data (d, f).
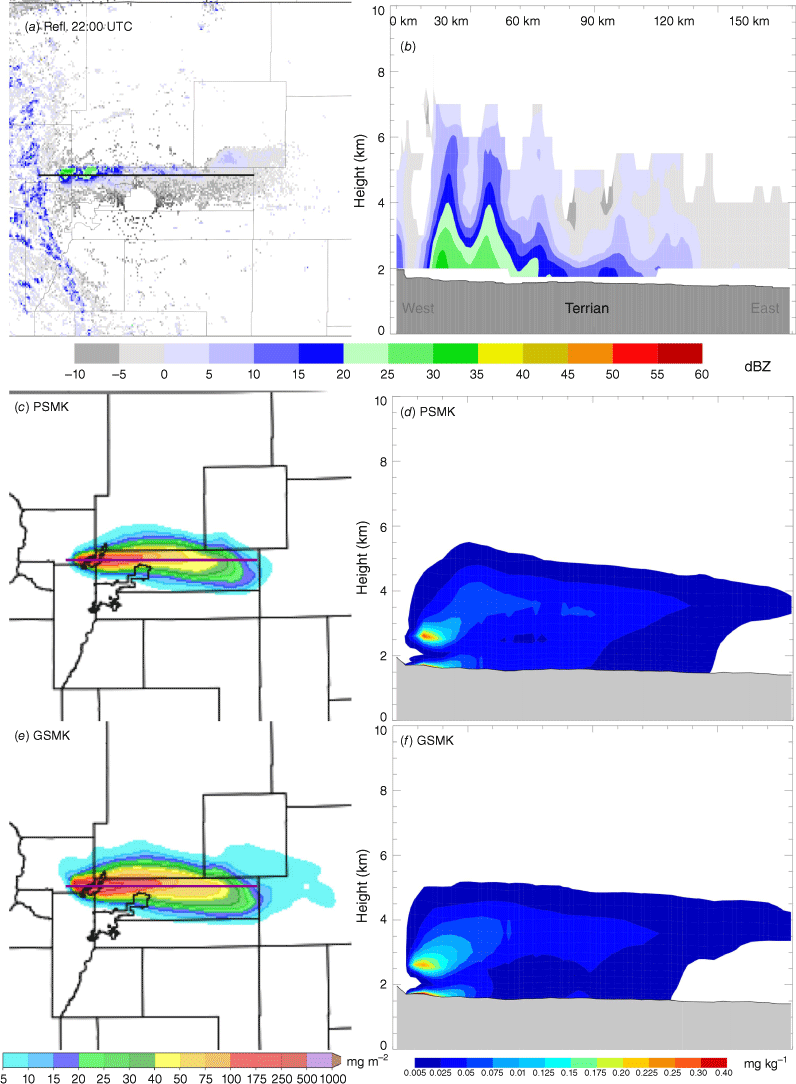
Texas, Oklahoma, and Arkansas fires, 17 March 2022
The second case selected for testing occurred on 17 March 2022 and consisted of many fires in Texas (TX), Oklahoma (OK), and Arkansas (AR) in a complex meteorological environment. During the day, an eastward moving dryline was present in southern OK and throughout TX behind, which were strong westerly winds and ahead of which thunderstorm development was occurring (Fig. 5). Further east, strong southerly winds were present over AR, providing an opportunity for fire growth despite being in a moist environment. GOES-16 visible (0.47 μm) imagery at 19:00 hours UTC shows several smoke plumes in eastern OK and western AR associated with multiple FRP retrievals (‘A’, Fig. 6a). These plumes spread from south to north corresponding to the southerly low-level flow ahead of the approaching storm system. Western OK is covered by a large area of mid-to-upper level clouds with portions of central TX. Behind the dryline, only one major fire is present at the western edge of the forecast domain (‘B’). This fire was not detected at 18:30 hours UTC (not shown) and has yet to generate a large smoke plume at 19:00 UTC. By 20:00 hours UTC, the number of wildfires has increased in both western AR and west-central TX (Fig. 6b). The smoke plume associated with the southern central TX fire is now evident with an additional fire initiating further north in the past hour. Wildfire development continues to occur in TX, with more FRP detections by 21:00 hours UTC (Fig. 6c). One fire complex in particular is located in Eastland County, corresponding to many FRP retrievals >400 MW (‘C’, Fig. 6d). The smoke plumes from the existing fires are also spreading rapidly eastward with the prevailing wind and mixed with a large area of dust. Development of cumulus clouds ahead of the dryline is also occurring at this time. In contrast to the increasing wildfire trend in TX, fires in AR appear to decrease in intensity in this period. Fewer FRP retrievals are being made and several orphan smoke plumes are evident in northern AR (yellow ovals, Fig. 6c, d). By 22:00 hours UTC, the Eastland County wildfire is the dominant feature in the domain, with a smoke plume that extends northeastward until reaching the dryline, where it mixes with the developing thunderstorms. These trends continue at 23:00 hours and 00:00 hours UTC on 18 March, with the Eastland County wildfire remaining large and its smoke plume interacting with storms to the north and east. The wildfires in AR and their smoke plumes are much less evident by 00:00 hours UTC (Fig. 6f).
WoFS-Smoke (GSMK) ensemble mean analysis of 2-m dewpoint (a) and 850 hPa wind speed and direction (b) valid at 23:00 hours UTC on 17 March 2022.
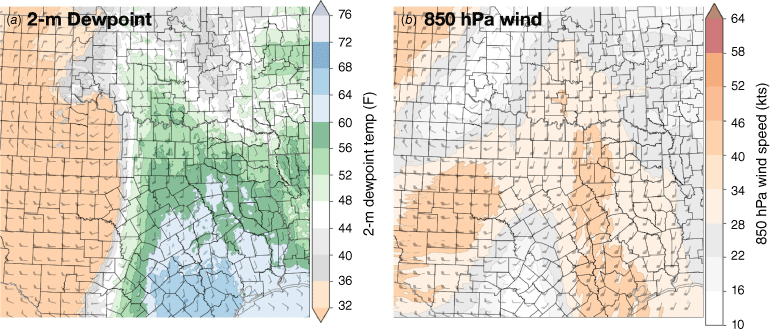
GOES-16 visible (0.47 μm) imagery between 19:00 hours and 00:00 hours UTC on 17–18 March 2022 (a–f) showing the evolution of fires in TX, OK, and AR at hourly intervals. Coloured dots indicate the location of GOES-16 FRP retrievals at each time. Yellow ovals indicate the location of an orphan smoke plume in AR. Areas of clouds, storms, and dust are present in the domain in addition to smoke.
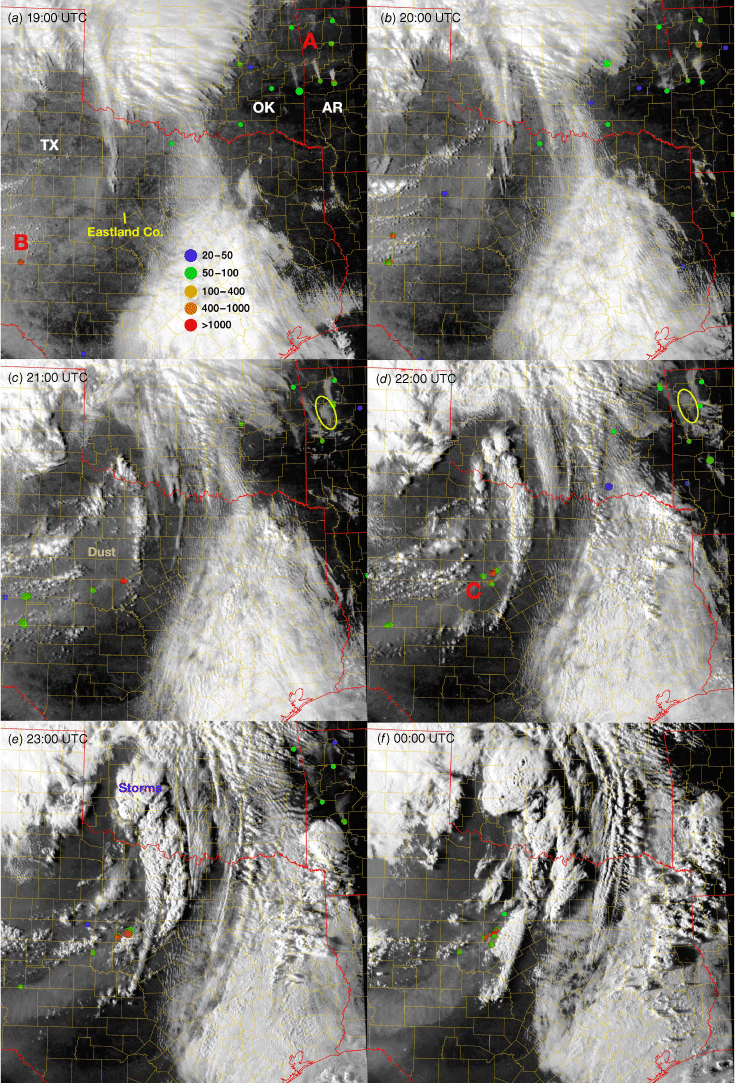
Locations of all FRP retrievals between 18:00 hours and 03:00 hours UTC on 17–18 March 2022 show many fires occurring in eastern OK and western AR in this period (Fig. 7a). Several detected fires do not generate obvious smoke plumes on the satellite imagery, which could be a combination of small fire size, cloud interference, and geo-location uncertainties. Fewer, but overall larger, fires are present in several locations in west-central TX with the highest concentration of retrievals occurring in Eastland County. In this figure, all MODIS and VIIRS retrievals are plotted over GOES-16 retrievals, which indicates the Eastland County fire is not detected from the polar orbiting instruments. The time series of FRP retrievals show overpasses between 18:00 hours and 18:45 hours UTC (Terra and SNPP) and between 19:30 hours and 20:30 hours UTC (Aqua, SNPP, and NOAA-20) (Fig. 7b). The first retrievals associated with the Eastland County fire were made at 20:45 hours UTC after the all the polar-orbiting overpasses of the afternoon had occurred. Thus, this fire is poorly analysed in the baseline HRRR or WoFS-Smoke system, potentially resulting in large smoke forecast errors. A very weak smoke plume was forecast in the baseline system from a FRP retrieval present the previous afternoon. Overall FRP increases from 18:00 hours to 20:30 hours UTC, and varies thereafter as fires increase and decrease in intensity within various parts of the domain. The overall trend is positive, which is primarily driven by the Eastland County fire after 22:00 hours UTC.
Fire radiative power retrievals from the Terra, Aqua, S-NPP, NOAA-20 and GOES-16 satellites between 18:00 hours and 03:00 hours UTC on 17–18 March 2022. GOES-16 retrievals are plotted at their native resolution of 2 km, with data from the other sensors having a finer resolution (a). The right column shows the sum (in GW) for all retrievals within a 5-min window from each satellite in this domain over the same time period (b).
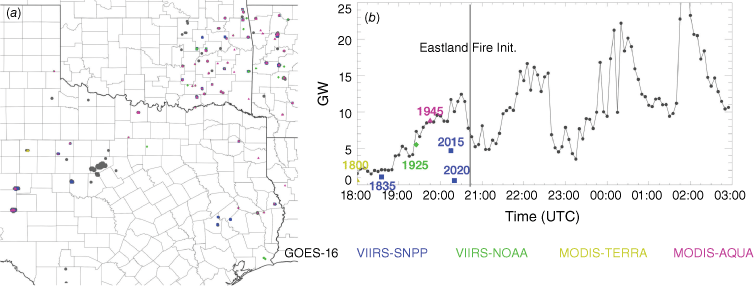
WoFS-Smoke forecasts initiated at 18:00 hours, 19:00 hours, 20:00 hours, and 21:00 hours UTC and valid at 22:00 hours UTC highlight several large differences between PSMK and GSMK. At 18:00 hours UTC, polar orbiting data had yet to fully sample the ongoing AR fires, and could not be ingested into the system at this time (Fig. 8a). Thus, little smoke is forecast in AR by 22:00 hours UTC. Conversely, several small smoke plumes are forecast in central OK, some of which are initiated from FRP retrievals many hours old. A few smoke plumes were forecast in central TX with the largest corresponding to the MODIS retrievals from the Terra satellite at 18:00 hours UTC. However, this fire also dissipates quickly and is no longer detected at 19:00 hours UTC (Fig. 6a). A small smoke plume is also forecast in Eastland County, but this is a remnant from a fire occurring the previous day. Many of the smoke plumes from the 18:00 hours UTC PSMK forecasts were not observed on corresponding visible imagery indicating significant potential for improvement. The corresponding 18:00 hours UTC forecast from GSMK valid at 22:00 hours UTC generates more smoke in AR, and less overall in TX. A very small smoke plume in west-central TX is also present, but not as strong as in the PSMK experiment. At 19:00 hours UTC, additional VIIRS and MODIS data allowed PSMK to generate smoke in AR and for a new large fire in west-central TX (Fig. 8c). GSMK also forecasts smoke from these fires well, though there are differences in the plume characteristics (Fig. 8d). However, both experiments fail to forecast smoke plumes from several TX fires that are ongoing by 22:00 hours UTC. This is not unexpected since most of them had not initiated at the time of forecast initiation between 18:00 hours and 19:00 hours UTC.
Forecast ensemble mean total-column smoke from PSMK and GSMK experiments valid at 22:00 hours UTC from forecasts initiated at 18:00 hours (a, b), 19:00 hours (c, d), 20:00 hours (e, f), and 21:00 hours UTC (g, h).
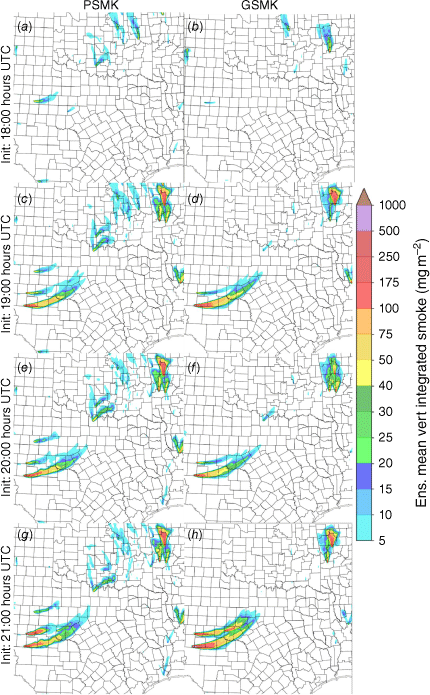
By 20:00 hours UTC, the number of fires in TX has increased and both polar orbiting and GOES-16 satellites sample these fires well (Fig. 8e, f). PSMK also still forecasts the AR plumes. In OK, PSMK also forecasts smoke associated with many small fires. The higher resolution of the VIIRS instrument allows for some of the smaller fires to be detected, which were not seen from GOES-16. GSMK does forecast some very small smoke plumes in eastern OK, the coverage of which does seem more consistent with observations at 22:00 hours UTC. However, significant cloud cover does complicate assessing overall model skill for the OK smoke plumes. In TX, both experiments forecast large smoke plumes associated with two fires that initiated after 19:00 hours UTC in west-central TX. The initial TX plume from the 18:00-hours and 19:00 hours UTC PSMK forecasts remains despite the fire weakening and the smoke dispersing by 20:00 hours UTC. Forecasts initiated at 21:00 hours UTC show similar results. The excess smoke in OK is still being forecast by PSMK with the extra fire in TX (Fig. 8g). GSMK forecasts larger smoke plumes associated with the TX fires as they increase in intensity after 20:00 hours UTC (Fig. 8h).
Similar results are present for forecasts valid at 23:00 hours UTC (Fig. 9a–h). Smoke plumes generally extend further north and east by 23:00 hours UTC, which is consistent with observations. PSMK still forecasts several areas of smoke over OK at 23:00 hours UTC, which are not present in GSMK. The 1-h forecast initialised at 22:00 hours UTC shows the impact of ingesting FRP retrievals associated with the Eastland County fire occurring after 21:00 hours UTC (Fig. 9g, h). Note the new forecast smoke plumes associated with this fire in GSMK, which becomes the largest source of smoke at later times. Additionally, fire intensity has decreased with one of the central TX fires, resulting in its forecast smoke plume decreasing in size and amount. Another difference is the weakening of the AR smoke plumes, also reflection of the decreasing intensity of the fires as seen by GOES-16. The use of GOES-16 data for this event allowed for wildfires to be initiated where none were observed with polar-orbiting sensors and correctly analysed their changing properties, lowering the amount of injected smoke as fires weaken.
As in Fig. 8, but for forecasts valid at 23:00 hours UTC initiated at 19:00 hours (a, b), 20:00 hours (c, d), 21:00 hours (e, f), and 22:00 hours UTC (g, h).
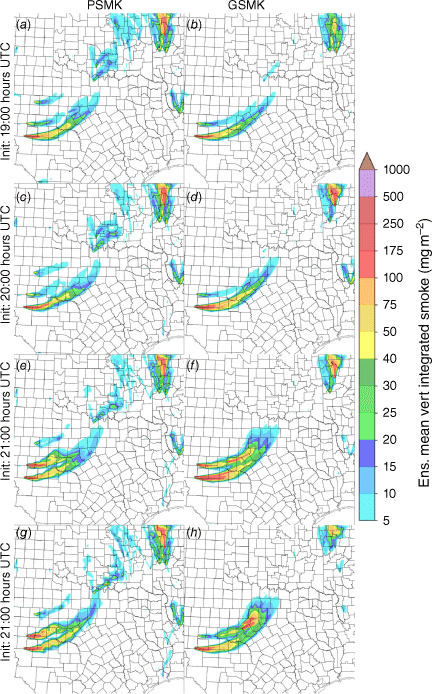
Characteristics of the Eastland County wildfire are further analysed by generating cross-sections of radar and forecast smoke through the smoke plume. The goal is to assess the differences between PSMK and GSMK and whether or not the latter correctly forecasts the 3D structure of the smoke plume. At 23:30 hours UTC, a large debris plume is evident in reflectivity extending from the main (X) fire to the north-east (Fig. 10a). The south-north cross section of reflectivity shows a deep column of lofted debris extending over 7 km AGL and ~15 km in width (Fig. 10b). Remaining debris associated with a decaying fire further south (Y) are also observed. The corresponding smoke forecasts valid at 23:30 hours UTC and initialised at 23:00 hours UTC show that PSMK did not analyse either of these fires (Fig. 10f). Only the smoke layer ~5 km above the surface from existing fire further west is present (Fig. 10d). Conversely, GSMK does analyse these fires and forecasts their smoke plumes (Fig. 10e). The cross-section of forecast smoke predicts the maximum concentration near the surface, which decreases as a function of height (Fig. 10b). Overall, smoke height is still being under-estimated when compared to the radar observations. However, the south-north tilt in the vertical profile that was observed is reasonably well forecast by GSMK.
MRMS Reflectivity at 1 km (AGL) from lofted debris at 23:30 hours UTC on 17 March 2022 (a). South-to-north cross-section of reflectivity along the debris plume with distance in km from the origin provided (b). Heights are given in km above sea-level with terrain masked. Ensemble mean 30 min forecast of total column smoke valid at 23:30 hours UTC from PSMK and GSMK (c, e). Corresponding cross-sections of forecast smoke along the same path as the radar data (d, f).
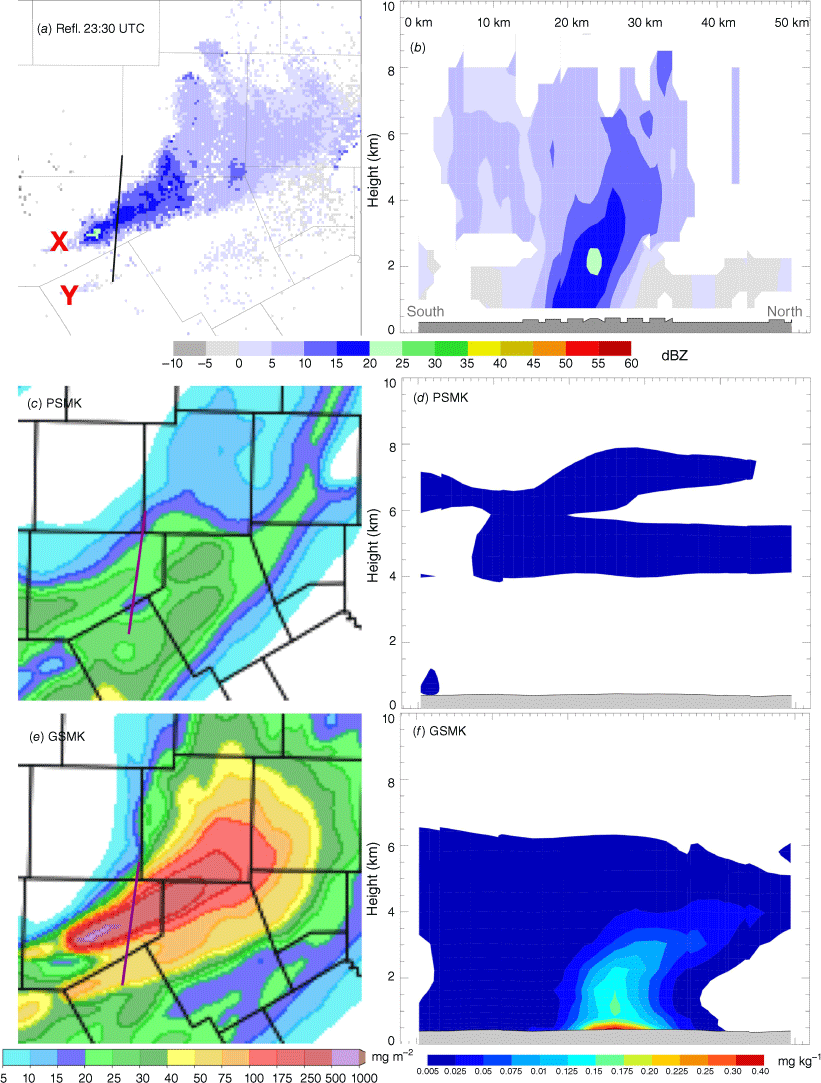
Conclusions
This research has shown that ingesting GOES-16 FRP retrievals into WoFS-Smoke provides several opportunities for the improvement of short-term wildfire smoke forecasts. The previous version of WoFS-Smoke as well as the currently (2023) operational HRRR model only ingest data from polar-orbiting satellites, limiting the opportunities to sample rapidly evolving wildfires. Comparing WoFS-Smoke experiments with only polar orbiting (PSMK) and GOES-16 (GSMK) data showed that the latter was able to initiate fires sooner in the model, leading to better early forecasts of the resulting smoke plumes. For example, the GSMK was able to analyse and forecast smoke associated with the Marshall Fire in CO on 30 December 2021, 1 h before PSMK. For the 17 March 2022 event, many differences were observed. First, GSMK initiated ongoing fires in AR sooner and better captured their weakening later in the period. Second, only GSMK was able to forecast smoke associated with a large fire in Eastland County, TX since it formed just after the overpasses of the polar-orbiting sensors. For forecast times when both polar-orbiting and GOES-16 satellite retrievals were less than 1 hourold, the forecasts were similar. This provides confidence that the observation pre-processing and bias adjustments applied to the GOES-16 data are performing well. Future work will further assess data quality assumptions and revisit the use of merged satellite products as they continue to develop. The higher spatial resolution data from MODIS and VIIRS still provides an ability to sample the very small, but still intense fires not always observable from the GOES ABI.
While ingesting GOES-16 data into WoFS-Smoke results in many improvements to smoke forecasts, further work is required to fully take advantage of these data. In particular, the next step for WoFS-Smoke is to couple the fire properties to the model dynamics in order to analyse and forecast buoyant updrafts associated with strong wildfires. Both PSMK and GSMK had difficulty in lofting smoke high enough in the atmosphere when compared against radar observations of co-located debris. Adding this information to the system is likely to significantly improve smoke plume height forecasts. Finally, a full coupling between the cloud microphysics scheme and smoke aerosol concentrations is desired. With these, it would be possible to forecast features such as pyro-Cu. With high temporal resolution data available from geostationary orbiting satellites, it will be possible in the near future to fully explore the potential of the WoFS-Smoke model.
Data availability
The WoFS-Smoke output images used from these experiments are available at: https://wof.nssl.noaa.gov/research and L2 satellite data as well as WSR-88D radar data are available from Amazon Web Services. VIIRS and MODIS data are available from class.noaa.gov. Additional model data and system configuration information are available upon request.
Acknowledgements
This research was funded in part by the NOAA Warn-on-Forecast project. Additional funding was provided under the NOAA-University of Oklahoma Cooperative Agreement NA21OAR4320204, U.S. Department of Commerce. We thank NOAA’s Joint Polar Satellite System Proving Ground and Risk Reduction program for funding and the rest of the HRRR-Smoke team and collaborators for helping with the model development.
References
Ahmadov R, Grell G, James E, et al. (2017) Using VIIRS Fire Radiative Power data to simulate biomass burning emissions, plume rise and smoke transport in a real-time air quality modeling system. In ‘IEEE International Symposium on Geoscience and Remote Sensing IGARSS’. pp. 2806–2808. (IEEE: New York, NY, USA)
Benjamin SG, Weygandt SS, Brown JM, Hu M, Alexander CR, Smirnova TG, Olson JB, James EP, Dowell DC, Grell GA, Lin H, Peckham SE, Smith TL, Moninger WR, Kenyon JS, Manikin GS (2016) A North American hourly assimilation and model forecast cycle: the rapid refresh. Monthly Weather Review 144, 1669-1694.
| Crossref | Google Scholar |
Chow FK, Yu KA, Young A, James E, Grell GA, Csiszar I, Tsidulko M, Freitas S, Pereira G, Giglio L, Friberg MD, Ahmadov R (2022) High-resolution smoke forecasting for the 2018 Camp Fire in California. Bulletin of the American Meteorological Society 103(6), E1531-E1552.
| Crossref | Google Scholar |
Csiszar I, Schroeder W, Giglio L, Ellicott E, Vadrevu KP, Justice CO, et al. (2014) Active fires from the Suomi NPP Visible Infrared Imaging Radiometer Suite: product status and first evaluation results. Journal of Geophysical Research: Atmospheres 119(2), 803-816.
| Crossref | Google Scholar |
Dowell DC, Alexander CR, James EP, Weygandt SS, Benjamin SG, Manikin GS, Blake BT, Brown JM, Olson JB, Hu M, Smirnova TG, Ladwig T, Kenyon JS, Ahmadov R, Turner DD, Duda JD, Alcott TI (2022) The High-Resolution Rapid Refresh (HRRR): an hourly updating convection-allowing forecast model. Part I: Motivation and system description. Weather and Forecasting 37(8), 1371-1395.
| Crossref | Google Scholar |
Dozier J (1981) A method for satellite identification of surface temperature fields of subpixel resolution. Remote Sensing of Environment 11, 221-229.
| Crossref | Google Scholar |
Freitas SR, Longo KM, Andreae MO (2006) Impact of including the plume rise of vegetation fires in numerical simulations of associated atmospheric pollutants. Geophysical Research Letters 33, L17808.
| Crossref | Google Scholar |
Freitas SR, Longo KM, Chatfield R, Latham D, Silva Dias MAF, Andreae MO, Prins E, Santos JC, Gielow R, Carvalho Jr JA (2007) Including the sub-grid scale plume rise of vegetation fires in low resolution atmospheric transport models. Atmospheric Chemistry and Physics 7(13), 3385-3398.
| Crossref | Google Scholar |
Freitas SR, Longo KM, Trentmann J, Latham D (2010) Technical note: Sensitivity of 1‐D smoke plume rise models to the inclusion of environmental wind drag. Atmospheric Chemistry and Physics 10(2), 585-594.
| Crossref | Google Scholar |
Fromm M, Bevilacqua R, Servranckx R, Rosen J, Thayer JP, Herman J, Larko D (2005) Pyro-cumulonimbus injection of smoke to the stratosphere: observations and impact of a super blowup in northwestern Canada on 3–4 August 1998. Journal of Geophysical Research 110, D08205.
| Crossref | Google Scholar |
Fromm M, Tupper A, Rosenfeld D, Servranckx R, McRae R (2006) Violent pyro-convective storm devastates Australia’s capital and pollutes the stratosphere. Geophysical Research Letters 33, L05815.
| Crossref | Google Scholar |
Fromm M, Lindsey DT, Servranckx R, Yue G, Trickl T, Sica R, Doucet P, Godin-Beekmann S (2010) The untold story of pyrocumulonimbus. Bulletin of the American Meteorological Society 91, 1193-1210.
| Crossref | Google Scholar |
Fromm M, Kablick III G, Caffrey P (2016) Dust-infused baroclinic cyclone storm clouds: the evidence, meteorology, and some implications. Geophysical Research Letters 43, 12643-12650.
| Crossref | Google Scholar |
Fovell RG, Brewer MJ, Garmong RJ (2022) The December 2021 Marshall Fire: predictability and gust forecasts from operational models. Atmosphere 13(5), 765.
| Crossref | Google Scholar |
Giglio L, Kendall JD (2001) Application of the dozier retrieval to wildfire characterization: a sensitivity analysis. Remote Sensing of Environment 77, 34-49.
| Crossref | Google Scholar |
Giglio L, Boschetti L, Roy DP, Humber ML, Justice CO (2018) The Collection 6 MODIS burned area mapping algorithm and product. Remote Sensing of the Environment 217, 72-85.
| Crossref | Google Scholar | PubMed |
Halofsky JE, Peterson DL, Harvey BJ (2020) Changing wildfire, changing forests: the effects of climate change on fire regimes and vegetation in the Pacific Northwest, USA. Fire Ecology 16, 4.
| Crossref | Google Scholar |
Holden ZA, Swanson A, Luce CH, Jolly WM, Maneta M, Oyler JW, Warren DA, Parsons R, Affleck D (2018) Decreasing fire season precipitation increased recent western US forest wildfire activity. Proceedings of the National Academy of Sciences of the United States of America 115(36), E8349-E8357.
| Crossref | Google Scholar | PubMed |
Hu M, Ge G, Zhou C, Stark D, Shao H, Newman K, Beck J, Zhang X (2018) ‘Grid-point Statistical Interpolation (GSI) User’s Guide Version 3.7.’ 149 pp. (Developmental Testbed Center) Available at http://www.dtcenter.org/com-GSI/users/docs/index.php
Iacono MJ, Delamere JS, Mlawer EJ, Clough SA, Morcrette J-J, Hou Y-T (2004) Development and evaluation of RRTMG_SW, a shortwave radiative transfer model for general circulation model applications. In ‘Proceedings 14th Atmospheric Radiation Measurement (ARM) Science Team Meeting’, Albuquerque, NM, DOE. 10 pp.
Jaffe DA, O’Neill SM, Larkin NK, et al. (2020) Wildfire and prescribed burning impacts on air quality in the United States. Journal of the Air & Waste Management Association 70, 583-615.
| Crossref | Google Scholar |
James EP, Alexander CR, Dowell DC, Weygandt SS, Benjamin SG, Manikin GS, Brown JM, Olson JB, Hu M, Smirnova TG, Ladwig T, Kenyon JS, Turner DD (2022) The High-Resolution Rapid Refresh (HRRR): an hourly updating convection-allowing forecast model. Part II: Forecast performance. Weather and Forecasting 37(8), 1397-1417.
| Crossref | Google Scholar |
Jones TA, Christopher SA (2009) Injection heights of biomass burning debris estimated from WSR-88D radar observations. IEEE Trans Geoscience Remote Sensing 47(8), 2599-2605.
| Crossref | Google Scholar |
Jones TA, Christopher SA, Petersen W (2009) Dual-polarization radar characteristics of an apartment fire. Journal of Atmospheric and Oceanic Technology 26, 2257-2269.
| Crossref | Google Scholar |
Jones TA, Christopher SA (2010a) Satellite and radar observations of the 9 April 2009 Texas and Oklahoma grassfires. Bulletin of the American Meteorological Society 91(4), 455-460.
| Crossref | Google Scholar |
Jones TA, Christopher SA (2010b) Satellite and radar remote sensing of Southern Plains grassfires: a case study. Journal of Applied Meteorology and Climatology 49, 2133-2146.
| Crossref | Google Scholar |
Jones TA, Knopfmeier K, Wheatley D, Creager G, Minnis P, Palikonda R (2016) Storm-scale data assimilation and ensemble forecasting with the NSSL experimental Warn-on-Forecast system. Part II: Combined radar and satellite data experiments. Weather and Forecasting 31, 297-327.
| Crossref | Google Scholar |
Jones TA, Skinner P, Yussouf N, Knopfmeier K, Reinhart A, Wang X, Bedka K, Smith Jr W, Palikonda R (2020) Assimilation of GOES-16 radiances and retrievals into the Warn-on-Forecast System. Monthly Weather Review 148, 1829-1859.
| Crossref | Google Scholar |
Jones TA, Ahmadov R, James E, Periria G, Freitas S, Grell G (2022a) Prototype of a Warn-on-Forecast System for Smoke (WoFS-Smoke). Weather and Forecasting 37, 1191-1209.
| Crossref | Google Scholar |
Jones TA, Ahmadov R, James E (2022b) Assimilation of aerosol optical depth into the Warn-on-Forecast System for Smoke (WoFS-Smoke). Journal of Geophysical Research: Atmospheres 127(24), e2022JD037454.
| Crossref | Google Scholar |
Kaufman YJ, Hobbs PV, Kirchhoff VWJH, et al. (1998a) Smoke, Clouds, and Radiation‐Brazil (SCAR‐B) experiment. Journal of Geophysical Research 103, 31,783-31,805.
| Crossref | Google Scholar |
Kaufman YJ, Kleidman R, King MD (1998b) SCAR-B fires in the tropics: Properties and remote sensing from EOS-MODIS. Journal of Geophysical Research 103, 31,955-31,968.
| Crossref | Google Scholar |
Kleist DT, Parrish DF, Derber JC, Treadon R, Wu W-S, Lord S (2009) Introduction of the GSI into the NCEP Global Data Assimilation System. Weather and Forecasting 24, 1691-1705.
| Crossref | Google Scholar |
Lareau NP, Clements CB (2015) Cold Smoke: smoke-induced density currents cause unexpected smoke transport near large wildfires. Atmospheric Chemistry and Physics 15, 11513-11520.
| Crossref | Google Scholar |
Lareau NP, Clements CB (2016) Environmental controls on pyrocumulus and pyrocumulonimbus initiation and development. Atmospheric Chemistry and Physics 16, 4005-4022.
| Crossref | Google Scholar |
Lareau NP, Nauslar NJ, Abatzoglou JT (2018) The Carr Fire vortex: a case of pyrotornadogenesis? Geophysical Research Letters 45, 13107-13115.
| Crossref | Google Scholar |
Li F, Zhang X, Roy DP, Kondragunta S (2019) Estimation of biomass-burning emissions by fusing the fire radiative power retrievals from polar-orbiting and geostationary satellites across the conterminous United States. Atmospheric Environment 211, 274-287.
| Crossref | Google Scholar |
Li F, Zhang X, Kondragunta S, Lu X, Csiszar I, Schmidt CC (2022) Hourly biomass burning emissions product from blended geostationary and polar-orbiting satellites for air quality forecasting applications. Remote Sensing of Environment 281, 113237.
| Crossref | Google Scholar |
Mansell ER, Ziegler CL, Bruning EC (2010) Simulated electrification of a small thunderstorm with two-moment bulk microphysics. Journal of the Atmospheric Sciences 67, 171-194.
| Crossref | Google Scholar |
Melnikov VM, Zrnic DS, Rabin RM (2009) Polarimetric radar properties of smoke plumes: a model. Journal of Geophysical Research 114, D21204.
| Crossref | Google Scholar |
O’Neill SM, Diao M, Raffuse S, Al-Hamdan M, Barik M, Jia Y, et al. (2021) A multi-analysis approach for estimating regional health impacts from the 2017 Northern California wildfires. Journal of the Air & Waste Management Association 71(7), 791-814.
| Crossref | Google Scholar | PubMed |
Powers JG, Klemp JB, Skamarock WC, Davis CA, Dudhia J, Gill DO, et al. (2017) The Weather Research and Forecasting (WRF) model: Overview, system efforts, and future directions. Bulletin of the American Meteorological Society 98(8), 1717-1737.
| Crossref | Google Scholar |
Prins EM, Menzel WP (1992) Geostationary satellite detection of biomass burning in South America. International Journal of Remote Sensing 13, 2783-2799.
| Crossref | Google Scholar |
Prins EM, Menzel WP (1994) Trends in South American biomass burning detected with the GOES visible infrared spin scan radiometer atmospheric sounder from 1983 to 1991. Journal of Geophysical Research 99(D8), 16,719-16,735.
| Crossref | Google Scholar |
Prins EM, Feltz JM, Menzel WP, Ward DE (1998) An overview of GOES-8 diurnal fire and smoke results for SCAR-B and 1995 fire season in South America. Journal of Geophysical Research 103(D24), 31821-31835.
| Crossref | Google Scholar |
Roberts G, Wooster MJ, Perry GLW, Drake N, Rebelo L-M, Dipotso F (2005) Retrieval of biomass combustion rates and totals from fire radiative power observations: application to southern Africa using geostationary SEVIRI imagery. Journal of Geophysical Research 110, D21111.
| Crossref | Google Scholar |
Robock A (1988) Enhancement of surface cooling due to forest fire smoke. Science 242(4880), 911-913.
| Crossref | Google Scholar |
Robock A (1991) Surface cooling due to forest fire smoke. Journal of Geophysical Research 96(D11), 20869-20878.
| Crossref | Google Scholar |
Skamarock WC, et al. (2008) A description of the Advanced Research WRF version 3. NCAR Tech. Note NCAR/TN-4751STR. 113 pp. University Corporation for Atmospheric Research. 10.5065/D68S4MVH
Skinner PS, Wheatley DM, Knopfmeier KH, et al. (2018) Object-based verification of a prototype Warn-on-Forecast system. Weather and Forecasting 33, 1225-1250.
| Crossref | Google Scholar | PubMed |
Troy A, Moghaddas J, Schmidt D, Romsos JS, Sapsis DB, Brewer W, Moody T (2022) An analysis of factors influencing structure loss resulting from the 2018 Camp Fire. International Journal of Wildland Fire 31(6), 586-598.
| Crossref | Google Scholar |
Twomey S (1974) Pollution and the planetary albedo. Atmospheric Environment 8, 1251-1256.
| Crossref | Google Scholar |
Weaver JF, Purdom JFW, Schneider TL (1995) Observing forest fires with the GOES-8, 3.9-μm imaging channel. Weather and Forecasting 10, 803-808.
| Crossref | Google Scholar |
Weaver JF, Lindsey D, Bikos D, Schmidt CC, Prins E (2004) Fire detection using GOES rapid scan imagery. Weather and Forecasting 19, 496-510.
| Crossref | Google Scholar |
Wheatley DM, Knopfmeier KH, Jones TA, Creager GJ (2015) Storm-scale data assimilation and ensemble forecasting with the NSSL Experimental Warn-on-Forecast System. Part I: Radar data experiments. Weather and Forecasting 30, 1795-1817.
| Crossref | Google Scholar |
Whitaker JS, Hamill TM, Wei X, Song Y, Toth Z (2008) Ensemble data assimilation with the NCEP Global Forecast System. Monthly Weather Review 136, 463-482.
| Crossref | Google Scholar |
Wooster MJ, Roberts G, Perry GLW, Kaufman YJ (2005) Retrieval of biomass combustion rates and totals from fire radiative power observations: FRP derivation and calibration relationships between biomass consumption and fire radiative energy release. Journal of Geophysical Research 110, D24311.
| Crossref | Google Scholar |
Xu X, Jia G, Zhang X, et al. (2020) Climate regime shift and forest loss amplify fire in Amazonian forests. Global Change Biology 26, 5874-5885.
| Crossref | Google Scholar | PubMed |
Yussouf N, Knopfmeier KH (2019) Application of the Warn-on-Forecast System for Flash-Flood-Producing heavy convective rainfall events. Quarterly Journal of the Royal Meteorological Society 145, 2385-2403.
| Crossref | Google Scholar |
Zrnic D, Zhang P, Melnikov V, Mirkovic D (2020) Of fire and smoke plumes, polarimetric radar characteristics. Atmosphere 11, 363.
| Crossref | Google Scholar |