Predicting burn severity for integration with post-fire debris-flow hazard assessment: a case study from the Upper Colorado River Basin, USA
Adam G. Wells





A U.S. Geological Survey, Southwest Biological Science Center, Flagstaff, AZ 86001, USA.
B U.S. Geological Survey, Geosciences and Environmental Change Science Center, Denver Federal Center, MS980, Denver, CO 80225, USA.
C U.S. Geological Survey, Ecosystems Mission Area, USGS National Center, 12201 Sunrise Valley Drive, Reston, VA 20192, USA.
D U.S. Geological Survey, Geologic Hazards Science Center, Golden, CO 80401, USA.
E U.S. Geological Survey, Alaska Science Center, Anchorage, AK 99508, USA.
F Present address: Texas A&M Natural Resources Institute, Texas A&M University, 1747 Pennsylvania Avenue, N.W., Suite 400, Washington, D.C. 20006, USA.
International Journal of Wildland Fire 32(9) 1315-1331 https://doi.org/10.1071/WF22200
Submitted: 1 October 2022 Accepted: 24 July 2023 Published: 14 August 2023
© 2023 The Author(s) (or their employer(s)). Published by CSIRO Publishing on behalf of IAWF. This is an open access article distributed under the Creative Commons Attribution-NonCommercial-NoDerivatives 4.0 International License (CC BY-NC-ND)
Abstract
Background: Burn severity significantly increases the likelihood and volume of post-wildfire debris flows. Pre-fire severity predictions can expedite mitigation efforts because precipitation contributing to these hazards often occurs shortly after wildfires, leaving little time for post-fire planning and management.
Aim: The aim of this study was to predict burn severity using pre-fire conditions of individual wildfire events and estimate potential post-fire debris flow to unburned areas.
Methods: We used random forests to model dNBR from pre-fire weather, fuels, topography, and remotely sensed data. We validated our model predictions against post-fire observations and potential post-fire debris-flow hazard estimates.
Key results: Fuels, pre-fire weather, and topography were important predictors of burn severity, although predictor importance varied between fires. Post-fire debris-flow hazard rankings from predicted burn severity (pre-fire) were similar to hazard assessments based on observed burn severity (post-fire).
Conclusion: Predicted burn severity can serve as an input to post-fire debris-flow models before wildfires occur, antecedent to standard post-fire burn severity products. Assessing a larger set of fires under disparate conditions and landscapes will be needed to refine predictive models.
Implications: Burn severity models based on pre-fire conditions enable the prediction of fire effects and identification of potential hazards to prioritise response and mitigation.
Keywords: burn severity, Burned Area Reflectance Classification (BARC), debris flow, differenced Normalised Burn Ratio (dNBR), fuels, Landsat 8, machine learning, Monitoring Trends in Burn Severity (MTBS), pre-fire, Sentinel-2, Wildland fire.
Introduction
Post-wildfire debris-flow hazards are a major concern for natural resource managers (Johansen et al. 2001; Cannon et al. 2008; Kean et al. 2011; Staley et al. 2016). These events can have catastrophic, irreversible consequences, although there are pre-fire management strategies that can mitigate environmental, economic, and societal damage (Robichaud et al. 2008; Parson et al. 2010; Prats et al. 2012). The likelihood and magnitude of post-fire debris flows can be assessed using empirical and physics-based surface hydrology models. These models require a suite of pre- and post-fire environmental predictors describing topography, soil, weather, and burn severity pattern, and magnitude (Gartner et al. 2014; Staley et al. 2016; McGuire et al. 2017; Ebel et al. 2018; Murphy et al. 2020). Variability in burn severity influences ecosystem response to wildfire and is an important predictor of post-fire hazards due to changes in runoff and erosion via removal of vegetation and litter cover, as well as heating-induced changes in soil aggregate stability and water repellency (Vieira et al. 2015; Thomas et al. 2021). Because risk of post-wildfire debris flows and fire-induced changes in surface hydrology are highest in the year immediately following a wildfire (Cannon et al. 2008; Parise and Cannon 2012), pre-fire and rapid post-fire burn severity assessments are critical for mitigating post-fire hazards. Even though methods are well-developed for predicting post-fire debris flows using observed burn severity data, there remains a need for methods to predict burn severity before fires happen so they can inform post-fire debris-flow models.
Maps of soil burn severity are primary inputs for assessment of post-fire hazards, including flooding, erosion, debris flow, and decreased water quality (Parsons et al. 2010; Moody et al. 2013). Models are applied operationally by state and federal post-fire emergency response teams (USGS [United States Geological Survey] 2023) immediately after wildfires to identify post-fire risks, develop emergency response plans, and design mitigation strategies. These models include probabilistic and physically based models to estimate enhanced runoff and erosion following wildfire (Foltz et al. 2009; Goodrich et al. 2012; Robichaud et al. 2016), as well as empirical models to estimate post-fire debris-flow likelihood and volume (Gartner et al. 2014; Staley et al. 2016). Similarly, metrics of burn severity underpin research to understand fire effects on soil-hydrologic properties (Moody et al. 2016; Ebel et al. 2018), water quality (Rust et al. 2018; Murphy et al. 2020), and long-term sediment yields (Pelletier and Orem 2014; Robichaud et al. 2020). In addition to predictions of post-fire hazards, there is growing interest in using projections of burn severity from wildfire scenarios to evaluate potential consequences of future wildfires (Sankey et al. 2017; Staley et al. 2018). Such studies allow for proactive, pre-fire planning and risk mitigation across management units instead of reactive post-fire response to individual events.
Burn severity describes fire effects on soil and vegetation (Eidenshink et al. 2007; Keeley 2009) and is often used interchangeably with the National Wildfire Coordinating Group’s (NWCG [National Wildfire Coordinating Group] 2023) definition of ‘fire severity’, which is the ‘degree to which a site has been altered or disrupted by fire; loosely a product of fire intensity and residence time.’ Currently, various approaches exist to characterise burn severity after wildfires. Field assessments of burn severity are assessed using the composite burn index (CBI), which integrates plot-level measures of severity for individual ecosystem components (e.g. vegetation mortality, soil char; Key and Benson 2006). Remote sensing methods use spectral indices such as the Normalised Burn Ratio (NBR), differenced NBR (dNBR), Relativized dNBR (RdNBR), and Relativized Burn Ratio (RBR), to map burn severity, based on decreases in near infrared reflectance (0.76–0.90 µm) and increases in shortwave infrared reflectance (2.08–2.35 µm) that occur with consumption of vegetation and deposition of charcoal and ash (Lentile et al. 2006; French et al. 2008; Fassnacht et al. 2021). Other studies have related image-derived and field-based severity measures to directly predict CBI scores from imagery (Picotte et al. 2021) and ancillary information, producing estimates that account for climate and ecosystem effects (Parks et al. 2018a; Harvey et al. 2019). In the USA, the Monitoring Trends in Burn Severity (MTBS) program produces dNBR and categorical severity maps based on satellite imagery within 2 years of wildfires (Eidenshink et al. 2007; MTBS 2023). For more rapid assessments, used to address emergency stabilisation after wildfires, the Burned Area Emergency Response (BAER) program provides Burned Area Reflectance Classification (BARC) maps within a week of fires if suitable satellite imagery is available (BAER 2023). Predictive models of burn severity can help identify when, where, and why undesired fire impacts will be greatest, yet no definitive evaluation of pre-fire conditions exists to predict potential burn severity and subsequent ecosystem response, in particular, post-fire debris flow – the underpinning of this study.
Pre-fire estimation of burn severity offers the possibility of understanding how the effects of wildfire can most effectively be mitigated within a management unit, particularly if severity models include predictor variables related to fuel characteristics that can be manipulated through treatments (Parks et al. 2018a). Modifying fuels through mechanical treatments and prescribed fire is one of the main mechanisms managers use to mitigate risk of post-fire hazards and other unwanted effects of wildfires (Cochrane et al. 2012; Loudermilk et al. 2014; Parks et al. 2018a). Previous studies modelled burn severity in relation to pre-fire environmental conditions including weather, vegetation, fuels, and topographic variables (Parks et al. 2018a; Dillon et al. 2020), with the aim of producing regional, generalised models for planning and assessment purposes. Regional-scale models may identify predictors that capture burn severity patterns across many fires and provide burn severity predictions. However, they provide limited insight into how each predictor influenced burn severity patterns in any individual fire. Better insight could be helpful for understanding where pre-fire treatments may be most appropriate and effective (e.g. fuels were important), or where patterns related more to other predictors are more difficult to manipulate through management (e.g. weather conditions). Understanding how different predictors influence burn severity across different fires may explain why patterns of burn severity differ and how relationships among pre-fire environmental conditions vary among individual fires, and across regions and ecosystem types. Fitting, assessing, and comparing burn severity of individual fires could identify divergent ecological pathways driving patterns of severity, which in turn could uniquely inform how fires impact ecosystems and post-fire hazards. This offers promise for catering fuel mitigation to a specific hazard of concern and also prioritising fuels treatments where a hazard is most probable, and where the greatest reduction of risk to lives and infrastructure is achievable.
Therefore, our objectives in this study were twofold. Our first objective was to develop models for predicting burn severity by relating dNBR to pre-fire conditions, specific to individual fires, and to compare our predictions with real-world wildfire incidents. Our second objective was to predict, across both time and space, post-fire debris-flow hazards based on pre-fire potential burn severity and again to compare our predictions to real-world observations. Taken together, we seek to use individually observed wildfire phenomena to model burn severity and post-fire debris flows as a case study for estimating wildfire outcomes based on pre-fire conditions.
Methods
Study area
We modelled dNBR for two wildfires that occurred in 2020 in north central Colorado, USA: the East Troublesome Fire (ETF; burned 14 October 2020 through 30 November 2020) and the Grizzly Creek Fire (GCF; burned 10 August 2020 through 18 December 2020) (Fig. 1). Both fires burned through mountainous terrain at relatively high elevations of 2409–3677 m (ETF) and 1794–3149 m (GCF). Land administration or ownership was a mix of federal (USDA Forest Service, Bureau of Land Management, and National Park Service), state, and private lands. Land cover in the ETF was primarily evergreen forest and shrub/scrub (73 and 16%, respectively; Homer et al. 2020), with forest dominated by lodgepole pine (Pinus contorta), fir/spruce (Picea spp., Abies spp.), and aspen/birch (Populus spp., Betula spp.) communities (Ruefenacht et al. 2008). Lodgepole pine forests in the ETF were heavily impacted by a mountain pine beetle outbreak that peaked in 2008 (Caldwell et al. 2013; Bright et al. 2017). Land cover in the GCF was primarily deciduous forest (44%), shrub/scrub (25%), evergreen forest (24%), and mixed forest (5%) (Homer et al. 2020). Forest land cover in the GCF was primarily aspen/birch, fir/spruce, ponderosa pine (P. ponderosa), and pinyon/juniper (P. edulis, Juniperus spp.) forest-type groups (Ruefenacht et al. 2008). There was no record in the MTBS data base (1984–2019) of previous large fires in the areas burned by either fire (Eidenshink et al. 2007). When it occurred, the ETF was the second largest wildfire in Colorado’s history, burning almost 80 000 ha in total, including a rapid spread of 35 000 ha in 24 h (Meldrum et al. 2022). The GCF burned around 13 000 ha, and heavy rainfall storms the following summer of 2021 resulted in large debris-flow events and associated depositions of material that heavily impacted the USA Interstate-70 travel corridor; economic costs were estimated in the millions of USD (Palmer 2022). Smaller debris flows occurred and closed roads in the ETF burned area. The similarity in geographic location, both located in the Upper Colorado River Basin (Fig. 1), the scale (>10 000 ha), and the realised and potential impacts of post-fire hazards made both fires suitable candidates for analysis.
Random forests modelling overview
A variety of statistical and machine learning (ML) approaches are useful for modelling and predicting wildfire characteristics, including occurrence, fire size, severity, and effect (Taylor et al. 2013; Xi et al. 2019; Jain et al. 2020). Generally, ML is, ‘a set of methods’ (Jain et al. 2020; p. 480) used to ‘detect patterns in data’, and ‘predict future data or other outcomes of interest’ (Murphy 2012). The ML approaches can handle large data volumes without making assumptions about the shape of relationships among response and predictor variables or the interactions among predictor variables (Breiman 2001; Tomaschek et al. 2018). Random forests models are a particularly popular ML method, which use an ensemble of many individual decision trees for supervised learning (Jain et al. 2020). Here, we used a random forests algorithm to model the relationships among burn severity and a suite of static (i.e. topography) and dynamic (i.e. weather) predictor variables. We used Anaconda Python 3.10.0 and the scikit-learn library for model fitting and predictions (Pedregosa et al. 2011; Anaconda Software Distribution 2023).
Response variables
For modelling, we used imagery (Table 1) from the Sentinel-2A MultiSpectral Instruments (MSI) and the Landsat 8 Operational Land Imager (OLI) instrument to calculate observed dNBR as a measure of burn severity. We used individual image pairs instead of temporal composites (Parks et al. 2018b) to simplify processing and allow for more direct comparison with the BARC and MTBS data. Pre-fire and post-fire imagery dates (Table 1) were separated by 1 year; images from Sentinel-2A, Sentinel-2B MSI, and Landsat 8 OLI were considered during searching to find images with low cloud cover. The Sentinel-2A MSI imagery used for analysis of the ETF was collected 3 months pre-fire (15 July 2020) and 1-year post-fire, on nearly the same date (10 July 2021). Imagery used for analysis of the GCF came from Landsat 8 OLI collected 1 month pre-fire (9 July 2020) and 1-year post-fire (14 August 2021). We intentionally selected unique image data from the MTBS and BARC products (Table 1) to ensure independence of our data for comparison, and to ensure it was temporally coincident with the image lag of MTBS.
Grizzly Creek fire | East Troublesome fire | |
---|---|---|
Ignition date | 10 August 2020 | 14 October 2020 |
Response pre-fire | Landsat 8 OLI: 9 July 2020 | Sentinel-2A MSI: 15 July 2020 |
Response post-fire | Landsat 8 OLI: 14 August 2021 | Sentinel-2A MSI: 10 July 2021 |
BARC pre-fire | Sentinel-2A MSI: 7 August 2020 | Landsat 8 OLI: 6 October 2020 |
BARC post-fire | Sentinel-2A MSI: 27 August 2020 | Landsat 8 OLI: 22 October 2020 |
MTBS pre-fire | Sentinel-2B MSI: 10 July 2020 | Landsat 8 OLI: 4 September 2020 |
MTBS post-fire | Sentinel-2A MSI: 10 July 2021 | Landsat 8 OLI: 7 September 2021 |
Predictor variables
We compiled predictors representing pre-fire conditions, including topography, fuel condition and amount, short-term and seasonal weather, and biophysical and spectral factors (Table 2) based on surface reflectance values (Table 3). Topography influences vegetation type, productivity, fuel moisture, and fire behaviour (Dillon et al. 2011; Parks et al. 2018a). Our topographic predictors, including elevation, slope, terrain ruggedness index, surface roughness, flow direction of water, sine of aspect (east-west), and cosine of aspect (north-south), were derived from the Shuttle Radar Topography Mission (SRTM; Farr et al. 2007). Patterns of burn severity are also related to spatial variability in fuel loads (Ferguson et al. 2002). To represent pre-fire fuel loads, we used biomass calculated from the FastFuels fuel density model (Altintas et al. 2015; WIFIRE Commons 2022). The FastFuels data product uses the TreeMap plot identifiers (Riley et al. 2021) to approximate tree locations and characteristics to produce a fully 3-dimensional high-resolution mapping of fuel density and structure (Pimont et al. 2016; Parsons et al. 2018). We summed biomass values over all 1-m FastFuels voxels to produce total biomass at a 10-m resolution.
Category | Time period | Variable name |
---|---|---|
Topography (~90 m) | 2000 | Elevation |
2000 | Flow direction of water | |
2000 | Roughness | |
2000 | Slope | |
2000 | Terrain position index | |
2000 | Terrain ruggedness index | |
Fire Weather (4 km) | Year & 1-month prior | Total precipitation accumulation |
Year & 1-month prior | Maximum & minimum temperature | |
Year & 1-month prior | 100-h fuel moisture | |
Year & 1-month prior | 1000-h fuel moisture | |
Year & 1-month prior | Wind speed & direction (cosine & sine) | |
Year & 1-month prior | Specific humidity | |
Year & 1-month prior | Maximum and minimum relative humidity | |
Year & 1-month prior | Vapour pressure deficit | |
Year & 1-month prior | Burning index model-g | |
Year & 1-month prior | Energy release component model-g | |
Year & 1-month prior | Surface downward shortwave radiation | |
Year & 1-month prior | Alfalfa reference evapotranspiration | |
Year & 1-month prior | Reference grass evapotranspiration | |
Biophysical (10 m) | Leaf-on & leaf-off | Canopy water content |
Leaf-on & leaf-off | Chlorophyll content in the leaf | |
Leaf-on & leaf-off | Fraction absorbed photosynthetically active radiation | |
Leaf-on & leaf-off | Fraction of vegetation cover | |
Leaf-on & leaf-off | Leaf-area index | |
Spectral (10 m) | Leaf-on & leaf-off | Sentinel-2 band 1 |
Leaf-on & leaf-off | Sentinel-2 band 2 | |
Leaf-on & leaf-off | Sentinel-2 band 3 | |
Leaf-on & leaf-off | Sentinel-2 band 4 | |
Leaf-on & leaf-off | Sentinel-2 band 5 | |
Leaf-on & leaf-off | Sentinel-2 band 6 | |
Leaf-on & leaf-off | Sentinel-2 band 7 | |
Leaf-on & leaf-off | Sentinel-2 band 8 | |
Leaf-on & leaf-off | Sentinel-2 band 8a | |
Leaf-on & leaf-off | Sentinel-2 band 9 | |
Leaf-on & leaf-off | Sentinel-2 band 11 | |
Leaf-on & leaf-off | Sentinel-2 band 12 | |
Fuel Amount (10 m) | 2016 | FastFuels |
Fuel Condition (30 m) | 1 & 4-months prior & day after | Landsat provisional actual evapotranspiration |
Band name | Landsat 8 OLI band number | Sentinel 2 MSI band number | Wavelength (micrometres) |
---|---|---|---|
Coastal Aerosol | 1 | 1 | 0.43–0.45 |
Blue | 2 | 2 | 0.45–0.51 |
Green | 3 | 3 | 0.53–0.59 |
Red | 4 | 4 | 0.64–0.67 |
Red-edge 1 | 5 | 0.69–0.71 | |
Red-edge 2 | 6 | 0.73–0.75 | |
Red-edge 3 | 7 | 0.77–0.79 | |
Near infrared broad | 8 | 0.78–0.88 | |
Near infrared narrow | 5 | 8A | 0.85–0.88 |
Shortwave infrared 1 | 6 | 11 | 1.57–1.65 |
Shortwave infrared 2 | 7 | 12 | 2.11–2.29 |
Water vapour | 9 | 0.93–0.95 | |
Cirrus | 9 | 10 | 1.36–1.38 |
Fire weather is an important driver of fire behaviour and patterns in many fire-prone ecosystems (Abatzoglou 2013; Yebra et al. 2013). To account for fire weather patterns leading up to ignition, we included daily values of meteorological conditions, fuel moistures, and fire behaviour indices averaged over the month and annual calendar year prior to ignition date to represent pre-fire fuel weather conditions (Table 2) and longer-term fuel productivity (Holden and Jolly 2011; Flannigan et al. 2016). These predictors were acquired from the 4-km resolution GridMET data (Abatzoglou 2013) and included: 100- and 1000-h dead fuel moisture; maximum temperature; minimum temperature; precipitation accumulation; downward surface shortwave radiation; wind speed; wind direction; maximum relative humidity; minimum relative humidity; specific humidity; reference evapotranspiration; energy release component; burning index; and vapour pressure deficit (Table 2). We also used the 30-m resolution provisional Landsat actual evapotranspiration data products from image dates captured 1 year, 4 months, and 1 month prior to the fire (Senay 2018) for an additional representation of fuel moisture conditions.
We included Sentinel-2 bands as predictors because previous studies demonstrated relationships between Landsat and ASTER bands with spectral canopy fuels and past bark beetle mortality (Jakubauskas 1996; Song et al. 2002; Hall et al. 2006; van der Meer et al. 2014; Bright et al. 2017, 2020; Ye et al. 2021). We incorporated seasonal (leaf-on growing season and leaf-off dormant season) remotely sensed predictors to represent pre-fire vegetation and fuel conditions not captured by the weather-related predictors. For the ETF, we used Sentinel-2B MSI images from 10 July 2020, corresponding to leaf-on season, and Sentinel-2A MSI images from the day prior to fire ignition, 13 October 2020, for leaf-off. For the GCF, we used Sentinel-2A MSI spectral data from 7 August 2020, for leaf-on, and excluded leaf-off because the timing of the ignition was before leaf senescence. Both sets of images were acquired before fire ignition but independent from the images used to calculate the dNBR response. Using the European Space Agency’s Sentinel Application Platform (SNAP; http://step.esa.int), we applied corrections (atmospheric, topographic, cirrus (Table 3; band 10), and bidirectional reflectance distribution functions) to each set of images and retained for analysis bands 1–9, 11, and 12 (Table 3) (Drusch et al. 2012). Additionally, we generated biophysical variables with SNAP for each corresponding leaf-on and leaf-off image representing leaf area index, fraction of absorbed photosynthetically active radiation, fraction of vegetation cover, chlorophyll content in the leaf, and canopy water content (European Space Agency 2022).
Model training and evaluation
We randomly sampled the data for each fire, 24 866 points for ETF and 1741 points for GCF, or roughly 0.5% of total pixels, enforcing a minimum of 100-m separation between points. At each point, we averaged pixel values within a 14-m radius. We split the sampled data into training (80%) and validation (20%) partitions. We then used the training samples to fit two random forests models using samples from each fire. Even though random forests models can handle many predictors, correlated predictors, and even unimportant predictors, including unimportant and correlated predictors can make model interpretation challenging. Therefore, we used a model-fitting routine that reduced the number of predictors through an iterative forward stepwise selection routine following the approach used by Hawbaker et al. (2020). The forward stepwise selection routine includes an additional test to exclude remaining predictors with 0.7 or greater correlation with selected predictors at each step. We set hyperparameter values for number of estimators at 500 and minimum samples per leaf at 10. We used 10-fold cross-validation (CV) throughout to evaluate model performance with root mean squared errors calculated on CV test partitions to limit overfitting. We calculated RMSE and R2 for the CV-test folds and validation data. We also calculated RMSE and R2 on the withheld validation data and compiled predictor importance values for evaluation.
After fitting random forests models to each fire, we used regression kriging to determine if the model residuals were spatially autocorrelated, which would violate the assumption of independence among model errors, could distort predictions (Dormann et al. 2013), and bias variable importance values (Strobl et al. 2007). Regression kriging combines a regression between a response variable and predictor variables (like our random forests models) but adds simple kriging of the residuals to account for any remaining spatial autocorrelation (Hengl et al. 2007). We fitted a kriged versions of the random forests model for each fire using the PyKrige 1.7.0 library (https://pypi.org/project/PyKrige/) and then calculated RMSE and R2 for the CV-test folds and validation data. We assumed no significant spatial dependence in the residuals if the CV-test RMSE values from the regression and regression kriging models were within 1 standard deviation. If spatial dependence was significant, we used the regression kriging model for predictions.
Comparing burn severity predictions with existing burn severity products
We used random forests models to spatially predict dNBR for fires from which training data were collected (henceforth ‘predictions’) and for the other fire not in the training data (henceforth ‘pairwise predictions’). In both cases, we compared the predicted dNBR values with independent observations of dNBR from the MTBS and BARC data products (Table 1). Debris-flow hazards models require categorical classification of burn severity, so we used the Jenks natural breaks algorithm (Jenks and Caspall 1971) to translate predicted dNBR into four severity categories (unburned to low, low, moderate, and high), following the MTBS labels, as well as two simple severity classes, combining the unburned to low and low categories (unburned-low), and also combining the moderate and high categories (moderate-high). We compared the count of pixels in each category with BARC and MTBS using overall accuracy, user’s and producer’s errors, and Kappa. The purpose of this comparison was not validation, but to compare with well-known datasets to assess the differences in severity produced by our modelling approach.
Evaluating burn severity predictions in debris-flow models
Finally, we incorporated our burn severity predictions into the debris-flow likelihood and volume models (Gartner et al. 2014; Staley et al. 2018). The U.S. Geological Survey (USGS) uses these models for emergency post-fire debris-flow hazard assessments for real-world wildfires. The post-fire debris-flow hazards analysis produces basin-level hazard rankings from combined rankings of debris-flow likelihood and volume using burn severity, elevation range, rainfall accumulation, slope, and soil erodibility as predictors. Basins with high debris-flow likelihood and large estimated volumes have high rankings (USGS [United States Geological Survey] 2023). We used this analysis framework with the categorised pre-fire pairwise predictions of dNBR (predictions from the random forests model trained on the ETF and applied to the GCF). We estimated combined hazards at increasing 15-min peak rainfall intensities from 16 to 40 mm h−1 by 8 mm h−1 increments. We overlaid locations of observed debris-flow depositions, and associated uphill catchment basins, from debris flows that impacted the Interstate-70 corridor. We compared the predicted hazard rankings with the original hazard rankings completed in 2020 (USGS [United States Geological Survey] 2023), looking for congruency of high hazard predictions associated with real-world observed debris-flow events.
Results
Model fit and predictors
Observed dNBR values averaged 0.4817 and 0.1681 for the ETF and GCF, respectively, and ranged between −0.9400 and 1.2540 for ETF and −0.2633 and 0.6140 for GCF. Negative dNBR values typically indicated enhanced post-fire vegetation growth, and values between −0.1 and 0.099 indicated unburned pixels. Random forests R2 values calculated with the validation data explained 48–64% of the observed variation in dNBR (Table 4), and RMSE values were 51 and 34% of the mean observed dNBR for GCF and ETF, respectively (Table 4). Regression kriging did not significantly reduce CV-test RMSE values for either fire (Table 4), indicating the model residuals were not spatially autocorrelated.
Fire and model type | RMSE | Adjusted R2 | ||
---|---|---|---|---|
CV test folds | Validation | CV test folds | Validation | |
East Troublesome fire | ||||
Regression | 0.1687 ± 0.0296 | 0.1616 | 0.5775 ± 0.1136 | 0.6369 |
Regression kriging | 0.1587 ± 0.0308 | 0.6288 ± 0.1069 | ||
Grizzly Creek fire | ||||
Regression | 0.0847 ± 0.0050 | 0.0849 | 0.4516 ± 0.0524 | 0.4792 |
Regression kriging | 0.0811 ± 0.0057 | 0.4981 ± 0.0565 |
T-test P-values between the regression and regression kriging models were 0.47 for East Troublesome Fire and 0.15 for Grizzly Creek Fire, indicating no difference in the RMSE values (i.e. no evidence for spatial autocorrelation). Cross-validation (CV) metrics represent the mean ± standard deviations. RMSE, root mean squared error; R2, coefficient of determination.
The predictor variables (Figs 2 and 3) and their importance for predicting dNBR (Fig. 4) varied between fires. We grouped the selected predictors into five general categories: (1) fuels; (2) topography; (3) weather 1 day after the fire started; (4) weather 1 month before the fire started; and (5) weather 1 year before the fire started. The most important predictor for the ETF was leaf-off Sentinel-2 band 12 (shortwave infrared 2; Table 3), with other substantially lower importance for the other selected predictors. Predictor importance summed by predictor categories for the ETF indicated that weather 1 month before the fire started was the most important (summed importance = 0.2078), followed by fuel-related predictors (0.1838), then weather 1 year before the fire started (0.0538), then topography (0.0329), and finally, weather 4 months before the fire started (0.0108). For the GCF (Fig. 3), the most important predictor was related to remotely sensed fuel loads and conditions: leaf-on chlorophyll content. Grouping the predictors into categories and ranking them indicated that burn severity patterns were primarily related to fuels (summed importance = 0.0533), topography (0.0343), then weather 1 year before the fire started (0.0136), weather 1 month before the fire started (0.0119), and finally, weather 1 day after the fire started (0.0074).
Selected variables and their importance from the random forests model used to predict differenced Normalised Burn Ratio (dNBR) for the East Troublesome Fire, in the Upper Colorado River Basin, 2020. Variables are arranged from bottom to top in the order they were selected in the forward stepwise selection routine.
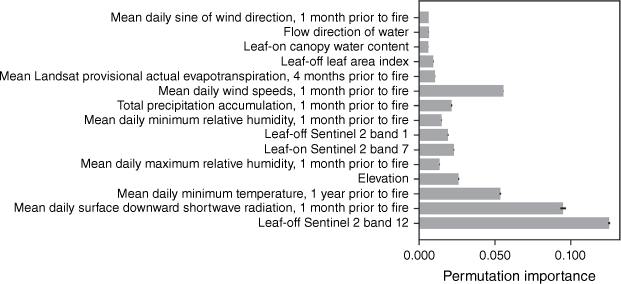
Selected variables and their importance from the random forests model used to predict differenced Normalised Burn Ratio (dNBR) for the Grizzly Creek Fire in the Upper Colorado River Basin, 2020. Variables are arranged from bottom to top in the order they were selected in the forward stepwise selection routine.
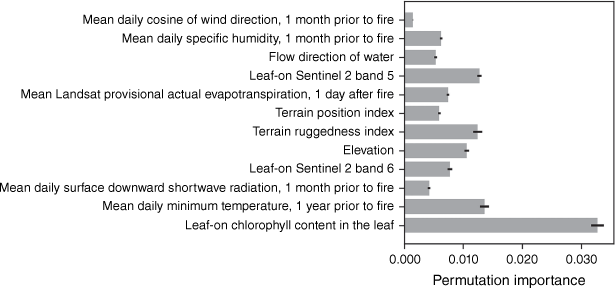
Observed differenced Normalised Burn Ratio (dNBR) for (a) the East Troublesome Fire and (b) the Grizzly Creek Fire. (c, d) Predicted dNBR using the random forests model trained with data from each individual fire. (e, f) Predicted dNBR using the random forests model trained with data from the other fire.
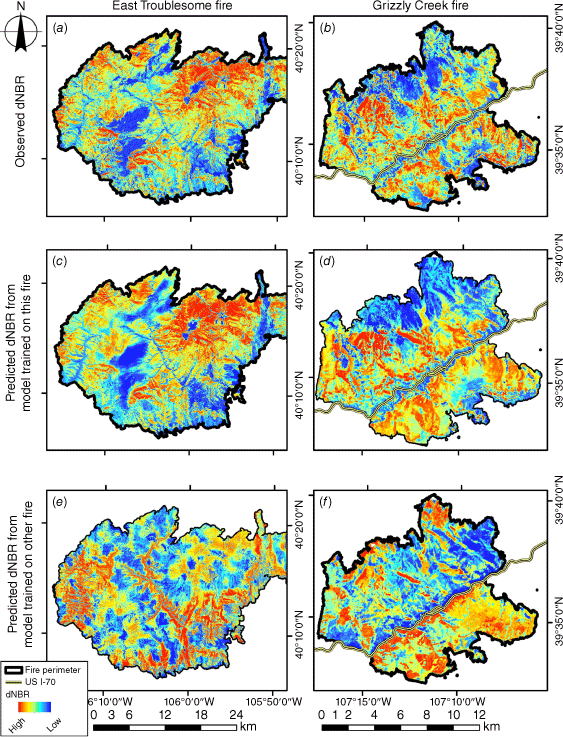
Comparing burn severity predictions with existing burn severity products
Observed dNBR for both the ETF and the GCF showed distinctive patterns where severity was low in riparian areas and valley bottoms, as well as along transportation routes and near developed areas (Fig. 4a, b). The spatial patterns of dNBR predicted by models trained to each fire generally matched the observed patterns for both the ETF (Fig. 4a, c) and GCF (Fig. 4b, d), although fine-scale variability in severity was often smoothed out in the predictions. Spatial patterns of dNBR in the pairwise predictions (Fig. 4e, f) were less similar to the observed patterns (Fig. 4a, b).
Spatial patterns of observed burn severity categories were visibly different between the BARC maps and MTBS data for both the ETF and GCF (Fig. 5). The BARC maps (Fig. 5a, b) tended to have less granularity and were generally lower in estimating severity than the MTBS maps (Fig. 5c, d). For the GCF, the BARC map had more moderate severity areas than MTBS and some overlap in areas with high severity (Fig. 5b, d). Predictions of dNBR from models trained on their respective fires translated to burn severity categories (Fig. 5e, f) tended to match the MTBS observed severity categories (Fig. 5c, d) better than the BARC severity categories (Fig. 5a, b). However, some of the differences between our predictions and the MTBS and BARC data may be related to the pre- and post-fire images we selected to calculate observed dNBR, which were captured closer to the MTBS dates than the BARC image dates. Our predictions may have better matched the BARC data if we had used the BARC image selection process for pre- (1 year before fire) and post-fire (immediately after fire). As expected, pairwise predictions of dNBR, from the model trained on the ETF and predicted on the GCF (Fig. 5g) and the model trained on the GCF and predicted on the ETF (Fig. 5h), showed less congruency.
Observed burn severity from the Burned Area Reflectance Classification (BARC) maps for (a) the East Troublesome Fire and (b) the Grizzly Creek Fire and (c, d) Monitoring Trends in Burn Severity (MTBS) data. Burn severity categories were based on predicted differenced Normalised Burn Ratio (dNBR) from (e, f) models trained with data from each fire and from (g, h) models trained with data from the other fire.
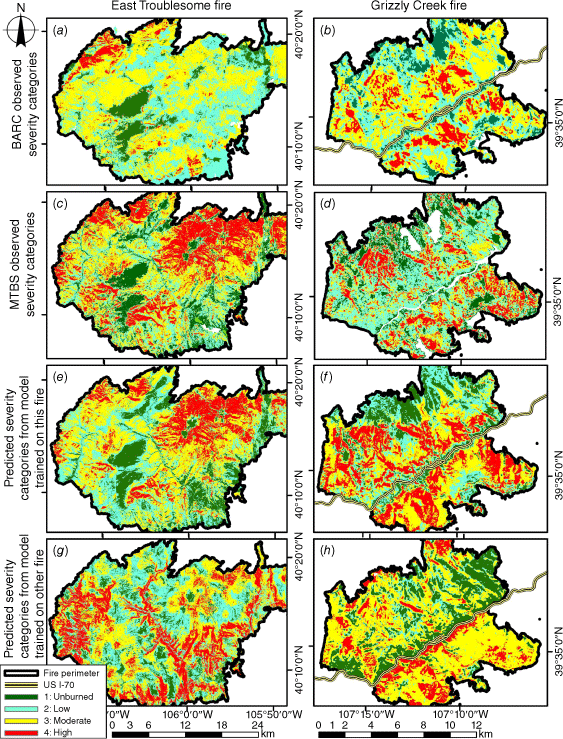
We used confusion matrices (Table 5) to characterise congruency amongst our categorical severity maps (Fig. 5e–h) and the MTBS severity maps (Fig. 5c, d). The ETF prediction and pairwise prediction had lower Kappa statistic and overall accuracy than the GCF model predictions. Accuracy was 0.58 and 0.41 for the ETF and GCF model predictions, respectively. However, accuracy and Kappa values decreased for the pairwise predictions. Omission and commission errors decreased substantially when severity categories were combined (unburned-low and moderate-high), which is how these variables are received by the post-fire debris-flow equations.
Severity category | |||||||||
---|---|---|---|---|---|---|---|---|---|
Trained with | Predicted on | Metric | Unburned | Low | Moderate | High | Unburned & low | Moderate & high | All |
(a) MTBS compared with ETF predicted by ETF model shown in Fig. 5e | |||||||||
ETF | ETF | Commission | 0.41 | 0.55 | 0.44 | 0.18 | 0.27 | 0.15 | |
Omission | 0.39 | 0.49 | 0.39 | 0.42 | 0.20 | 0.21 | |||
PCC | 0.61 | 0.51 | 0.61 | 0.58 | 0.80 | 0.79 | |||
Overall accuracy | 0.58 | ||||||||
Kappa accuracy | 0.42 | ||||||||
(b) MTBS compared with GCF predicted by GCF model shown in Fig. 5f | |||||||||
GCF | GCF | Commission | 0.48 | 0.42 | 0.73 | 0.61 | 0.14 | 0.46 | |
Omission | 0.57 | 0.68 | 0.56 | 0.39 | 0.46 | 0.14 | |||
PCC | 0.43 | 0.32 | 0.44 | 0.61 | 0.54 | 0.86 | |||
Overall accuracy | 0.41 | ||||||||
Kappa accuracy | 0.21 | ||||||||
(c) MTBS compared with ETF predicted by GCF model shown in Fig. 5g | |||||||||
GCF | ETF | Commission | 0.74 | 0.75 | 0.71 | 0.77 | 0.53 | 0.36 | |
Omission | 0.82 | 0.68 | 0.71 | 0.78 | 0.51 | 0.38 | |||
PCC | 0.18 | 0.32 | 0.29 | 0.22 | 0.49 | 0.62 | |||
Overall accuracy | 0.26 | ||||||||
Kappa accuracy | −0.012 | ||||||||
(d) MTBS compared with GCF predicted by ETF model shown in Fig. 5h | |||||||||
ETF | GCF | Commission | 0.72 | 0.47 | 0.73 | 0.67 | 0.15 | 0.50 | |
Omission | 0.63 | 0.89 | 0.34 | 0.70 | 0.56 | 0.12 | |||
PCC | 0.37 | 0.11 | 0.66 | 0.30 | 0.44 | 0.88 | |||
Overall accuracy | 0.31 | ||||||||
Kappa accuracy | 0.11 |
The Monitoring Trends in Burn Severity (MTBS) data were used for observations to compare against predicted severity categories. ETF, East Troublesome Fire; GCF, Grizzly Creek Fire. Commission = 1 – user’s accuracy; Omission = 1 – producer’s accuracy; PCC, proportion correctly classified.
Evaluating burn severity predictions in debris-flow models
In 2021, the year following the GCF, there were 17 records of post-fire debris-flow depositions along Interstate-70 (Fig. 6). These recorded events represented the largest and most destructive events that impacted transportation infrastructure because of post-fire debris flow from the GCF. An untold number of unrecorded debris-flow events originated with the burn perimeter but did not impact Interstate-70 and were not recorded. USGS operational estimates from the Emergency Assessment of Post-fire Debris Flow Hazards (USGS [United States Geological Survey] 2023) showed combined hazard steadily increased as rainfall intensities increased (Fig. 6a). At 40 mm h−1, 13 of 17 basins with observed depositions were ranked in the highest category of combined likelihood and volume hazard, with 4 of 17 basins ranked moderate. Actual precipitation intensities prior to initiation of observed debris flows ranged between 50 and 70 mm h−1, greater than model predictions shown in Fig. 6a.
Combined (likelihood and volume) debris-flow hazard classification based on USGS emergency assessment of post-fire debris-flow hazards of (a) Burned Area Emergency Response (BAER) program Burned Area Reflectance Classification (BARC) maps, (b) based on severity categories from differenced Normalised Burn Ratio (dNBR) predictions from a random forests model trained with data from the East Troublesome Fire (ETF) and applied to the Grizzly Creek Fire (GCF; Fig. 5h), and (c) GCF burn severity model applied to GCF. Note: flow basins are the watershed boundary associated with observed deposition sites from post-fire debris flow, which heavily damaged Interstate-70.
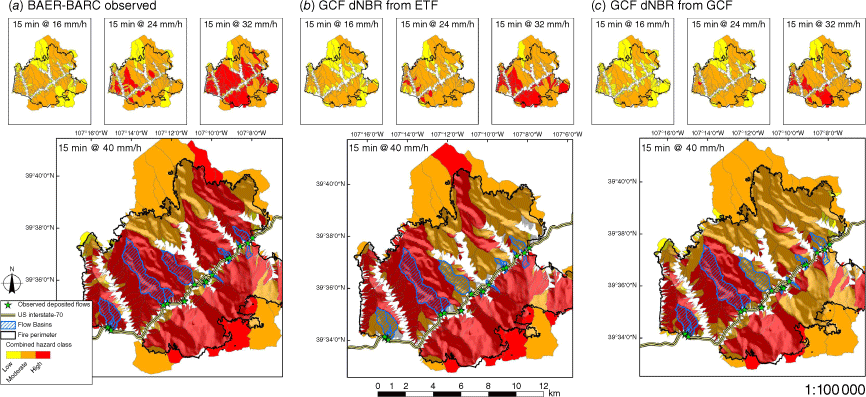
Combined hazard rankings generated using our pre-fire predictions of categorised dNBR across space and time (Fig. 6b; model trained on ETF and applied to GCF) and just time (Fig. 6c; model trained on GCF and applied to GCF) had a similar but not identical progression and patterning as the USGS operational hazard rankings. Using the model trained on ETF and applied to GCF, five of 17 observed debris flows occurred in basins with high combined hazard classification, seven were in basins with moderate combined hazard classification, and five were not classified (Fig. 6b). Using the model trained on GCF and applied to GCF, seven of 17 occurred in high, seven in moderate, two in low combined hazard classification, and one was not classified (Fig. 6c). Both predictions diverged from the operational products in the smaller subbasins but matched the largest basin that produced the most damaging observed debris flows. Differences in predicted versus observed debris flow were in basins with differences between the predicted (Fig. 4f) and observed dNBR (Fig. 4b) and classifications of unburned/low or medium/high burn categorisation shown in (Fig. 5d, h). Many additional post-fire debris flows occurred through the area, but not all deposition sites were recorded because these areas were inaccessible and did not impact the travel corridor.
Discussion
In this case study, we illustrated opportunities for ML to predict burn severity based on pre-fire landscape and weather conditions and incorporate those estimates into post-fire debris flow hazard classifications. Our ML models predicted continuous and discrete thematic burn severity estimates based on pre-fire conditions and have distinct advantages over typical post-fire assessments based on remote sensing: mainly post-fire images are not required, and severity can be estimated in advance of fires. We demonstrated that predictions of burn severity based on pre-fire conditions were similar to post-fire observations of burn severity. Additionally, post-fire debris-flow hazard rankings were similar in their progression of increasing hazard classifications as precipitation intensity increased. Our findings highlight the opportunities for using ML to build a priori assessments of burn severity where detailed spatial resolution or rapid timelines are required by managers to plan for and mitigate post-fire hazards before wildfire ignition.
Accurate predictions of burn severity produced before fires occur can inform proactive planning and development of mitigating strategies before fires and associated post-fire hazards occur. The accuracy of our predictions compared favourably with previous work (Birch et al. 2015; Kane et al. 2015; Picotte et al. 2021). Picotte et al. (2021) reported a wide range of R2 values (range = 0.26–0.60) for models predicting dNBR based on Landsat imagery for a variety of spatial scales. Birch et al. (2015) modelled dNBR for 42 fires in Idaho and Montana and reported R2 values between 0.42 and 0.49, and Kane et al. (2015) predicted the relativized dNBR for the 2013 Rim Fire in California and reported R2 values between 0.41 and 0.63, depending on predictors used and sample spacing. The R2 value for the GCF (0.48) was similar to those results; however, R2 for ETF (0.64) was higher than those previously published metrics. We also evaluated the accuracy of our categorical severity estimates with a variety of metrics for comparison with other studies (Dillon et al. 2011; Howe et al. 2022), which both reported Kappa values ≥0.44, comparable to our results. More recent work predicting high severity by ecoregion reported percentage correctly classified values between 0.65 and 0.87 (Dillon et al. 2020), somewhat higher than our results but based on a larger sample of fires. Refining our approach to thresholding dNBR values may improve the accuracy of severity classes, for example by using MTBS cut points rather than Jenks natural breaks to define cut-points. These comparisons suggest that our fire-specific severity models perform as well as some regional models trained with a large number of fires (Dillon et al. 2011; Birch et al. 2015; Kane et al. 2015). However, the reduced range of variability in the predictor values used for single-fire models may explain why their performance was lower than more recent regional-scale modelling efforts (Dillon et al. 2020). Furthermore, there were large differences in accuracy between models trained and applied to the same fire compared with models trained on one fire and applied to the other. Fitting models to individual fires constrains their application to other fires because the range of variability in predictors varied across the two fires considered here. This is a shortcoming of statistical and ML models and highlights the benefit of considering a larger set of fires to better characterise burn severity across the full range of predictor variability (Howe et al. 2022).
Pre-fire weather and fuel conditions impact fire temperatures, intensity and residency times, key factors behind variability in ecological change and burn severity, and ultimately, post-fire debris flows (Keeley 2009; Heward et al. 2013; Morgan et al. 2014). Similar to previous studies (Dillon et al. 2011; Birch et al. 2015; Kane et al. 2015; Whitman et al. 2018; Parks et al. 2018a), we found that variables representing the factors of fuel, topography, and weather all contributed to predictions of burn severity. However, our models did not select variables directly measuring fuels (FastFuels) and instead selected remotely sensing variables related to fuels, perhaps due to the inherent challenge mapping fuels in the field and over large spatial extents (Keane et al. 2012; Keane and Gray 2013; Duff et al. 2017). The relative contributions of the selected variables differed, however, between fires, because the individual variables important for predicting severity varied between the two large fires in this case study; each fire had a unique set of drivers controlling severity patterns. Of all the predictors considered, fuel-related predictors were the most important in GCF, with 44% of total relative variable importance, and second-most important in ETF, with 38% of total relative importance. The importance of the Sentinel-2 band 12 (Shortwave infrared 2) and band 7 (Red-edge 3) in ETF and leaf-on chlorophyll content, and bands 5 and 6 (Red-edge 1 and 2) in GCF, indicate that pre-fire vegetation was an important source of variability in burn severity in both fires. Previous studies have shown that shortwave infrared and near infrared bands capture variability in forest structure and age classes, especially younger forests (Jakubauskas 1996; Song et al. 2002; Hall et al. 2006), and can be used to distinguish between live and dead biomass and soil (van der Meer et al. 2014), areas affected by bark beetle mortality and post-mortality canopy fuels (Bright et al. 2017; Ye et al. 2021), and burned and unburned areas (Amos et al. 2019).
The area burned by ETF experienced extensive beetle-caused forest mortality, with nearly 80% of basal area killed (Caldwell et al. 2013). The fuel-related predictors in ETF also relate to patterns of forest mortality in that ecosystem (Vogelmann et al. 2009; Liang et al. 2014; Ye et al. 2021). DeCastro et al. (2022) demonstrated improved fire spread predictions for the ETF after updating fuels to represent past beetle kill. The importance of fuel-related predictors in our models indicates the potential to mitigate post-fire hazards and risk through fuels management (Ager et al. 2010; Prichard and Kennedy 2014; Prichard et al. 2020). However, simulation models indicate that a wide range of potential post-fire effects are possible (Burke et al. 2021), that treatments maybe short lived (Finney et al. 2007; Stephens et al. 2012; Vaillant et al. 2015), and that extensive areas may need to be treated before benefits are observed (Finney et al. 2007; Syphard et al. 2011). Accurately estimating burn severity before fires can inform pre-fire mitigation planning. Because fuel-related variables and their interactions with other predictors influence severity predictions, models like ours can help identify priority locations to consider for mitigation actions.
Predictors related to topography were important for both fires, second-most for GCF (28% of total relative variable importance) and third-most for ETF (7%). These predictors included elevation and the flow direction of water in both fires, but also the terrain ruggedness index and topographic position index in GCF. These predictors relate to fuel moisture, fire spread, and edaphic controls on vegetation. For example, aspect and slope affect the rate at which fuels dry and fires spread (Rothermel 1972; Bradshaw et al. 1984), as well as the development and depth of soil. Topographic predictors may also capture gradients in vegetation composition and productivity and fine-scale heterogeneity in temperature and moisture that were not well represented in other predictors (Peet 1981; Bassman et al. 2003). The importance of topography suggests considering interactions between fuels and topography will be important for effective pre-fire mitigation actions.
The most important predictors for ETF were related to weather conditions; combined across time scales (year prior, 4 months prior, and 1 month prior) variables representing weather factor accounted for 56% of total relative variable importance. The time scale of 1 month before the fire showed the greatest contribution to weather and included downward shortwave radiation (cloud cover), wind speed, precipitation, humidity, and wind direction. Predictors related to weather conditions 1 month before the fire were less important for GCF, but also included downward shortwave radiation and wind direction. Predictors characterising weather 1 year before the fire was the third-most important group of predictors for ETF, and GCF included only the mean of daily minimum temperatures for 2019. Radiation, precipitation, humidity, and temperature all relate to fuel moisture and, along with wind speed and direction, can influence fire behaviour. Past studies have demonstrated that weather conditions can be as or more important than fuel loads for predicting fire occurrence and behaviour (Bessie and Johnson 1995; Cary et al. 2009; Dennison and Moritz 2009). Priority locations for mitigation actions may change depending on weather conditions, and the inclusion of weather variables in our models provides an opportunity to evaluate how sensitive potential locations for mitigation actions are to different weather conditions.
There are other variables influencing patterns of burn severity not considered in our models. We excluded them for practical reasons (e.g. lack of data), but considering how they could be incorporated in future efforts might improve modelling results. For example, past fires (Stevens-Rumann et al. 2016; Harris and Taylor 2017), pre-fire fuel treatments, and firefighting activities may have influenced fire behaviour and spread, and ultimately severity. However, although some data are available for pre-fire fuel treatments, they are not routinely geared for use in national-scale modelling approaches. Furthermore, information about the location and types of firefighting activities are difficult to acquire, and it is not clear how to represent those activities in statistical and machine learning models. Additionally, our modelling approach may benefit from using ecologically based measures of burn severity. For example, CBI values could be used to identify thresholds in predicted dNBR values; however, CBI values are often not available. Alternatively, approaches do exist to create CBI severity outputs from Landsat imagery (Picotte et al. 2021). However, using CBI severity predictions would require retraining post-fire debris flow models to use CBI as an input instead of dNBR or MTBS-defined categorical severity values. The benefit of taking that extra step would be an integrated modelling approach for burn severity and debris flow hazards that is more readily verified with in situ information (Kolden et al. 2015; Howe et al. 2022).
Overall, the two fires we analysed were in close proximity and had similar vegetation types, but each had unique and different sets of predictors explaining patterns of burn severity. This phenomenon is most evident as shown by the topographic influence of the model of the GCF (Fig. 4d) (an extreme high burn severity along high topographic relief) predicted to the ETF (Figs 4e and 5g). Based on the outcome and topography of the GCF, the model painted onto the ETF is characteristic of the GCF site conditions showing higher expected burn severity in canyons. Future modelling endeavours may benefit by matching topography for comparative fire analysis and considering predictors that capture both dead and live fuel loads and conditions, as representative of the extensive beetle-kill at ETF. Furthermore, expanding our analysis to additional fires may reveal common modes of wildfires in relation to burn severity. These individualised effects may be overlooked when modelling severity at regional scales due to data pooling, thereby smoothing important variability and relationships shown to be distinct amongst fires in this case study (Howe et al. 2022). Treating fires as independent events in future modelling efforts may help identify variable divergence leading to spatial variability in burn severity; however, the effect of spatial autocorrelation among predictor variables, multi-collinearity over fitting predictions of burn severity, and assessing variable importance may increase at smaller scales of analysis. Broadening the spatial extent and increasing the sample size in our approach may allow for a comprehensive analysis of fires within geographic regions of interest, temporally coincident with recent remotely sensed imagery.
Data availability
The data that support the findings of this study are publicly available at Wells et al. (2023).
Declaration of funding
This research was funded by the U.S. Department of the Interior, U.S. Geological Survey, Colorado River Basin: Actionable and Strategic Integrated Science and Technology program, the Land Change Science Program in the Core Science Systems Mission Area, and the Wildland Fire Science program in the Ecosystems Mission Area.
Acknowledgements
We thank Dr. Russ Parsons from U.S. Forest Service Missoula Fire Lab and Daniel Rosales-Giron from WIFIRE Commons at U.C. San Diego, for providing FastFuel inputs. We thank Jaime Kostelnik for applying the U.S. Geological Survey debris-flow models. We also greatly appreciate comments from the three anonymous International Journal of Wildland Fire reviewers.
References
Abatzoglou JT (2013) Development of gridded surface meteorological data for ecological applications and modelling. International Journal of Climatology 33, 121-131.
| Crossref | Google Scholar |
Ager AA, Vaillant NM, Finney MA (2010) A comparison of landscape fuel treatment strategies to mitigate wildland fire risk in the urban interface and preserve old forest structure. Forest Ecology and Management 259, 1556-1570.
| Crossref | Google Scholar |
Altintas I, Block J, de Callafon R, Crawl D, Cowart C, Gupta A, Nguyen M, Braun H-W, Schulze J, Gollner M, Trouve A, Smarr L (2015) Towards an Integrated Cyberinfrastructure for Scalable Data-driven Monitoring, Dynamic Prediction and Resilience of Wildfires. Procedia Computer Science 51, 1633-1642.
| Crossref | Google Scholar |
Amos C, Petropoulos GP, Ferentinos KP (2019) Determining the use of Sentinel-2A MSI for wildfire burning & severity detection. International Journal of Remote Sensing 40, 905-930.
| Crossref | Google Scholar |
Anaconda Software Distribution (2023) Anaconda version 3.10.0. Available at https://www.anaconda.com/ [verified 21 March 2023]
BAER (2023) Burned Area Emergency Response program. Available at https://burnseverity.cr.usgs.gov/baer/ [verified 16 May 2023]
Bassman JH, Johnson JD, Fins L, Dobrowolski JP (2003) Rocky Mountain ecosystems: diversity, complexity and interactions. Tree Physiology 23, 1081-1089.
| Crossref | Google Scholar |
Bessie WC, Johnson EA (1995) The relative importance of fuels and weather on fire behavior in sub-alpine forests. Ecology 76, 747-762.
| Crossref | Google Scholar |
Birch DS, Morgan P, Kolden CA, Abatzoglou JT, Dillon GK, Hudak AT, Smith AMS (2015) Vegetation, topography and daily weather influenced burn severity in central Idaho and western Montana forests. Ecosphere 6, 1-23.
| Crossref | Google Scholar |
Bradshaw LS, Deeming JE, Burgan RE, Cohen JD (1984) The 1978 National Fire-Danger Rating System: technical documentation. General Technical Report INT-169. 44 p. (U.S. Department of Agriculture, Forest Service, Intermountain Forest and Range Experiment Station: Ogden, UT) 10.2737/INT-GTR-169
Breiman L (2001) Random Forests. Machine Learning 45, 5-32.
| Crossref | Google Scholar |
Bright BC, Hudak AT, Meddens AJH, Hawbaker TJ, Briggs JS, Kennedy RE (2017) Prediction of forest canopy and surface fuels from lidar and satellite time series data in a bark beetle-affected forest. Forests 8, 322.
| Crossref | Google Scholar |
Bright BC, Hudak AT, Meddens AJH, Egan JM, Jorgensen CL (2020) Mapping Multiple Insect Outbreaks across Large Regions Annually Using Landsat Time Series Data. Remote Sensing 12, 1655.
| Crossref | Google Scholar |
Burke WD, Tague C, Kennedy MC, Moritz MA (2021) Understanding how fuel treatments interact with climate and biophysical setting to affect fire, water, and forest health: a process-based modeling approach. Frontiers in Forests and Global Change 3, 591162.
| Crossref | Google Scholar |
Caldwell MK, Hawbaker TJ, Briggs JS, Cigan PW, Stitt S (2013) Simulated impacts of mountain pine beetle and wildfire disturbances on forest vegetation composition and carbon stocks in the Southern Rocky Mountains. Biogeosciences 10, 8203-8222.
| Crossref | Google Scholar |
Cannon SH, Gartner JE, Wilson RC, Bowers JC, Laber JL (2008) Storm rainfall conditions for floods and debris flows from recently burned areas in southwestern Colorado and southern California. Geomorphology 96, 250-269.
| Crossref | Google Scholar |
Cary GJ, Flannigan MD, Keane RE, Bradstock RA, Davies ID, Lenihan JM, Li C, Logan KA, Parsons RA (2009) Relative importance of fuel management, ignition management and weather for area burned: evidence from five landscape–fire–succession models. International Journal of Wildland Fire 18, 147-156.
| Crossref | Google Scholar |
Cochrane MA, Moran CJ, Wimberly MC, Baer AD, Finney MA, Beckendorf KL, Eidenshink J, Zhu Z (2012) Estimation of wildfire size and risk changes due to fuels treatments. International Journal of Wildland Fire 21, 357-367.
| Crossref | Google Scholar |
DeCastro AL, Juliano TW, Kosović B, Ebrahimian H, Balch JK (2022) A computationally efficient method for updating fuel inputs for wildfire behavior models using Sentinel imagery and random forest classification. Remote Sensing 14, 1447.
| Crossref | Google Scholar |
Dennison PE, Moritz MA (2009) Critical live fuel moisture in chaparral ecosystems: a threshold for fire activity and its relationship to antecedent precipitation. International Journal of Wildland Fire 18, 1021-1027.
| Crossref | Google Scholar |
Dillon GK, Holden ZA, Morgan P, Crimmins MA, Heyerdahl EK, Luce CH (2011) Both topography and climate affected forest and woodland burn severity in two regions of the western US, 1984 to 2006. Ecosphere 2, 1-33.
| Crossref | Google Scholar |
Dillon GK, Panunto MH, Davis B, Morgan P, Birch DS, Jolly WM (2020) Development of a severe fire potential map for the contiguous United States. General Technical Report RMRS-GTR-415. 107 p. (U.S. Department of Agriculture, Forest Service, Rocky Mountain Research Station: Fort Collins, CO) Available at https://www.fs.usda.gov/treesearch/pubs/60733
Dormann CF, Elith J, Bacher S, Buchmann C, Carl G, Carré G, Marquéz JRG, Gruber B, Lafourcade B, Leitão PJ, Münkemüller T, McClean C, Osborne PE, Reineking B, Schröder B, Skidmore AK, Zurell D, Lautenbach S (2013) Collinearity: a review of methods to deal with it and a simulation study evaluating their performance. Ecography 36, 27-46.
| Crossref | Google Scholar |
Drusch M, del Bello U, Carlier S, Colin O, Fernandez V, Gascon F, Hoersch B, Isola C, Laberinti P, Martimort P, Meygret A, Spoto F, Sy O, Marchese F, Bargellini P (2012) Sentinel-2: ESA’s optical high-resolution mission for GMES operational services. Remote Sensing of Environment 120, 25-36.
| Crossref | Google Scholar |
Duff TJ, Keane RE, Penman TD, Tolhurst KG (2017) Revisiting wildland fire fuel quantification methods: the challenge of understanding a dynamic, biotic entity. Forests 8, 351.
| Crossref | Google Scholar |
Ebel BA, Romero OC, Martin DA (2018) Thresholds and relations for soil-hydraulic and soil-physical properties as a function of burn severity 4 years after the 2011 Las Conchas Fire, New Mexico, USA. Hydrological Processes 32, 2263-2278.
| Crossref | Google Scholar |
Eidenshink J, Schwind B, Brewer K, Zhu Z-L, Quayle B, Howard S (2007) A project for monitoring trends in burn severity. Fire Ecology 3, 3-21.
| Crossref | Google Scholar |
European Space Agency (2022) STEP – Science Toolbox Exploitation Platform. Available at http://step.esa.int/main/
Farr TG, Rosen PA, Caro E, Crippen R, Duren R, Hensley S, Kobrick M, Paller M, Rodriguez E, Roth L, Seal D, Shaffer S, Shimada J, Umland J, Werner M, Oskin M, Burbank D, Alsdorf D (2007) The Shuttle Radar Topography Mission. Reviews of Geophysics 45, RG2004.
| Crossref | Google Scholar |
Fassnacht FE, Schmidt-Riese E, Kattenborn T, Hernández J (2021) Explaining Sentinel 2-based dNBR and RdNBR variability with reference data from the bird’s eye (UAS) perspective. International Journal of Applied Earth Observation and Geoinformation 95, 102262.
| Crossref | Google Scholar |
Ferguson SA, Ruthford JE, McKay SJ, Wright D, Wright C, Ottmar R (2002) Measuring moisture dynamics to predict fire severity in longleaf pine forests. International Journal of Wildland Fire 11, 267-279.
| Crossref | Google Scholar |
Finney MA, Seli RC, McHugh CW, Ager AA, Bahro B, Agee JK (2007) Simulation of long-term landscape level fuel treatment effects on large wildfires. International Journal of Wildland Fire 16, 712-727.
| Crossref | Google Scholar |
Flannigan MD, Wotton BM, Marshall GA, de Groot WJ, Johnston J, Jurko N, Cantin AS (2016) Fuel moisture sensitivity to temperature and precipitation: climate change implications. Climatic Change 134, 59-71.
| Crossref | Google Scholar |
Foltz RB, Robichaud PR, Rhee H (2009) A synthesis of postfire road treatments for BAER teams: methods, treatment effectiveness, and decision-making tools for rehabilitation. General Technical Report. RMRS-GTR-228. 152 p. (U.S. Department of Agriculture, Forest Service, Rocky Mountain Research Station: Fort Collins, CO)
French NHF, Kasischke ES, Hall RJ, Murphy KA, Verbyla DL, Hoy EE, Allen JL (2008) Using Landsat data to assess fire and burn severity in the North American boreal forest region: an overview and summary of results. International Journal of Wildland Fire 17, 443-462.
| Crossref | Google Scholar |
Gartner JE, Cannon SH, Santi PM (2014) Empirical models for predicting volumes of sediment deposited by debris flows and sediment-laden floods in the transverse ranges of southern California. Engineering Geology 176, 45-56.
| Crossref | Google Scholar |
Goodrich DC, Burns IS, Unkrich CL, Semmens DJ, Guertin DP, Hernandez H, Yatheendradas S, Kennedy JR, Levick LR (2012) KINEROS2/AGWA: Model use, calibration, and validation. Transactions of the ASABE 55, 1561-1574.
| Crossref | Google Scholar |
Hall RJ, Skakun RS, Arsenault EJ, Case BS (2006) Modeling forest stand structure attributes using Landsat ETM+ data: Application to mapping of aboveground biomass and stand volume. Forest Ecology and Management 225, 378-390.
| Crossref | Google Scholar |
Harris L, Taylor AH (2017) Previous burns and topography limit and reinforce fire severity in a large wildfire. Ecosphere 8, e02019.
| Crossref | Google Scholar |
Harvey BJ, Andrus RA, Anderson SC (2019) Incorporating biophysical gradients and uncertainty into burn severity maps in a temperate fire-prone forested region. Ecosphere 10, e02600.
| Crossref | Google Scholar |
Hawbaker TJ, Vanderhoof MK, Schmidt GL, Beal Y-J, Picotte JJ, Takacs JD, Falgout JT, Dwyer JL (2020) The Landsat Burned Area algorithm and products for the conterminous United States. Remote Sensing of Environment 244, 111801.
| Crossref | Google Scholar |
Hengl T, Heuvelink GBM, Rossiter DG (2007) About regression-kriging: From equations to case studies. Computers & Geosciences 33, 1301-1315.
| Crossref | Google Scholar |
Heward H, Smith AMS, Roy DP, Tinkham WT, Hoffman CM, Morgan P, Lannom KO (2013) Is burn severity related to fire intensity? Observations from landscape scale remote sensing. International Journal of Wildland Fire 22, 910-918.
| Crossref | Google Scholar |
Holden ZA, Jolly WM (2011) Modeling topographic influences on fuel moisture and fire danger in complex terrain to improve wildland fire management decision support. Forest Ecology and Management 262, 2133-2141.
| Crossref | Google Scholar |
Homer C, Dewitz J, Jin S, Xian G, Costello C, Danielson P, Gass L, Funk M, Wickham J, Stehman S, Auch R, Riitters K (2020) Conterminous United States land cover change patterns 2001–2016 from the 2016 National Land Cover Database. ISPRS Journal of Photogrammetry and Remote Sensing 162, 184-199.
| Crossref | Google Scholar |
Howe AA, Parks SA, Harvey BJ, Saberi SJ, Lutz JA, Yocom LL (2022) Comparing Sentinel-2 and Landsat 8 for Burn Severity Mapping in Western North America. Remote Sensing 14, 5249.
| Crossref | Google Scholar |
Jain P, Coogan SCP, Subramanian SG, Crowley M, Taylor S, Flannigan MD (2020) A review of machine learning applications in wildfire science and management. Environmental Reviews 28, 478-505.
| Crossref | Google Scholar |
Jakubauskas ME (1996) Thematic Mapper characterization of lodgepole pine seral stages in Yellowstone National Park, USA. Remote Sensing of Environment 56, 118-132.
| Crossref | Google Scholar |
Jenks GF, Caspall FC (1971) Error on choroplethic maps: definition, measurement, reduction. Annals of the Association of American Geographers 61, 217-244.
| Crossref | Google Scholar |
Johansen MP, Hakonson TE, Breshears DD (2001) Post-fire runoff and erosion from rainfall simulation: contrasting forests with shrublands and grasslands. Hydrological Processes 15, 2953-2965.
| Crossref | Google Scholar |
Keane RE, Gray K (2013) Comparing three sampling techniques for estimating fine woody down dead biomass. International Journal of Wildland Fire 22, 1093-1107.
| Crossref | Google Scholar |
Kean JW, Staley DM, Cannon SH (2011) In situ measurements of post-fire debris flows in southern California: Comparisons of the timing and magnitude of 24 debris-flow events with rainfall and soil moisture conditions. Journal of Geophysical Research 116, F04019.
| Crossref | Google Scholar |
Keane RE, Gray K, Bacciu V, Leirfallom S (2012) Spatial scaling of wildland fuels for six forest and rangeland ecosystems of the northern Rocky Mountains, USA. Landscape Ecology 27, 1213-1234.
| Crossref | Google Scholar |
Kane VR, Cansler CA, Povak NA, Kane JT, McGaughey RJ, Lutz JA, Churchill DJ, North MP (2015) Mixed severity fire effects within the Rim fire: Relative importance of local climate, fire weather, topography, and forest structure. Forest Ecology and Management 358, 62-79.
| Crossref | Google Scholar |
Keeley JE (2009) Fire intensity, fire severity and burn severity: a brief review and suggested usage. International Journal of Wildland Fire 18, 116-126.
| Crossref | Google Scholar |
Key CH, Benson NC (2006) Landscape assessment: remote sensing of severity, the Normalized Burn Ratio. In ‘FIREMON: Fire Effects Monitoring and Inventory System’. (Eds DC Lutes, RE Keane, JF Caratti, et al.) General Technical Report, RMRS-GTR-164-CD:LA1-LA51. (USDA Forest Service, Rocky Mountain Research Station: Ogden, UT) Available at http://www.fs.usda.gov/treesearch/pubs/24066
Kolden CA, Smith AM-S, Abatzoglou JT (2015) Limitations and utilisation of Monitoring Trends in Burn Severity products for assessing wildfire severity in the USA. International Journal of Wildland Fire 24, 1023-1028.
| Crossref | Google Scholar |
Lentile LB, Smith FW, Shepperd WD (2006) Influence of topography and forest structure on patterns of mixed severity fire in ponderosa pine forests of the South Dakota Black Hills, USA. International Journal of Wildland Fire 15, 557-566.
| Crossref | Google Scholar |
Liang L, Chen Y, Hawbaker TJ, Zhu Z, Gong P (2014) Mapping Mountain Pine Beetle Mortality through Growth Trend Analysis of Time-Series Landsat Data. Remote Sensing 6, 5696-5716.
| Crossref | Google Scholar |
Loudermilk EL, Stanton A, Scheller RM, Dilts TE, Weisberg PJ, Skinner C, Yang J (2014) Effectiveness of fuel treatments for mitigating wildfire risk and sequestering forest carbon: A case study in the Lake Tahoe Basin. Forest Ecology and Management 323, 114-125.
| Crossref | Google Scholar |
McGuire LA, Rengers FK, Kean JW, Staley DM (2017) Debris flow initiation by runoff in a recently burned basin: Is grain-by-grain sediment bulking or en masse failure to blame? Geophysical Research Letters 44, 7310-7319.
| Crossref | Google Scholar |
Meldrum JR, Barth CM, Goolsby JB, Olson SK, Gosey AC, White J, Brenkert-Smith H, Champ PA, Gomez J (2022) Parcel-Level Risk Affects Wildfire Outcomes: Insights from Pre-Fire Rapid Assessment Data for Homes Destroyed in 2020 East Troublesome Fire. Fire 5, 24.
| Crossref | Google Scholar |
Moody JA, Shakesby RA, Robichaud PR, Cannon SH, Martin DA (2013) Current research issues related to post-wildfire runoff and erosion processes. Earth-Science Reviews 122, 10-37.
| Crossref | Google Scholar |
Moody JA, Ebel BA, Nyman P, Martin DA, Stoof C, McKinley R (2016) Relations between soil hydraulic properties and burn severity. International Journal of Wildland Fire 25, 279-293.
| Crossref | Google Scholar |
Morgan P, Keane RE, Dillon GK, Jain TB, Hudak AT, Karau EC, Sikkink PG, Holden ZA, Strand EK (2014) Challenges of assessing fire and burn severity using field measures, remote sensing and modelling. International Journal of Wildland Fire 23, 1045-1060.
| Crossref | Google Scholar |
MTBS (2023) Monitoring Trends in Burn Severity program. Available at https://www.mtbs.gov/ [verified 15 May 2023]
Murphy SF, McCleskey RB, Martin DA, Holloway JM, Writer JH (2020) Wildfire-driven changes in hydrology mobilize arsenic and metals from legacy mine waste. Science of the Total Environment 743, 140635.
| Crossref | Google Scholar |
NWCG [National Wildfire Coordinating Group] (2023) ‘NWCG Glossary of Wildland Fire, PMS 205.’ (National Interagency Fire Center: Boise, ID) Available at https://www.nwcg.gov/publications/pms205 [verified 3 August 2023]
Palmer J (2022) The devastating mudslides that follow forest fires. Nature 601, 7892 Available at https://www.nature.com/articles/d41586-022-00028-3.
| Google Scholar |
Parise M, Cannon SH (2012) Wildfire impacts on the processes that generate debris flows in burned watersheds. Natural Hazards 61, 217-227.
| Crossref | Google Scholar |
Parks SA, Holsinger LM, Panunto MH, Jolly WM, Dobrowski SZ, Dillon GK (2018a) High-severity fire: evaluating its key drivers and mapping its probability across western U.S. forests. Environmental Research Letters 13, 044037.
| Crossref | Google Scholar |
Parks SA, Holsinger LM, Voss MA, Loehman RA, Robinson NP (2018b) Mean composite fire severity metrics computed with Google Earth Engine offer improved accuracy and expanded mapping potential. Remote Sensing 10, 879.
| Crossref | Google Scholar |
Parson A, Robichaud PR, Lewis SA, Napper C, Clark JT (2010) Field guide for mapping post-fire soil burn severity. General Technical Report. RMRS-GTR-243. p. 24. (U.S. Department of Agriculture, Forest Service, Rocky Mountain Research Station 49: Fort Collins, CO) 10.2737/RMRS-GTR-243
Parsons RA, Pimont F, Wells L, Cohn G, Jolly WM, de Coligny F, Rigolot E, Dupuy J-L, Mell W, Linn RR (2018) Modeling thinning effects on fire behavior with STANDFIRE. Annals of Forest Science 75, 7.
| Crossref | Google Scholar |
Pedregosa F, Varoquaux G, Gramfort A, Michel V, Thirion B, Grisel O, Blondel M, Prettenhofer P, Weiss R, Dubourg V, Vanderplas J, Passos A, Cournapeau D, Brucher M, Perrot M, Duchesnay E (2011) Scikit-learn: Machine learning in Python. Journal of Machine Learning Research 12, 2825-2830.
| Google Scholar |
Peet RK (1981) Forest vegetation of the Colorado Front Range. Vegetatio 45, 3-75.
| Crossref | Google Scholar |
Pelletier JD, Orem CA (2014) How do sediment yields from post-wildfire debris-laden flows depend on terrain slope, soil burn severity class, and drainage basin area? Insights from airborne-LiDAR change detection. Earth Surface Processes and Landforms 39, 1822-1832.
| Crossref | Google Scholar |
Picotte JJ, Cansler CA, Kolden CA, Lutz JA, Key C, Benson NC, Robertson KM (2021) Determination of burn severity models ranging from regional to national scales for the conterminous United States. Remote Sensing of Environment 263, 112569.
| Crossref | Google Scholar |
Pimont F, Parsons R, Rigolot E, de Coligny F, Dupuy JL, Dreyfus P, Linn RR (2016) Modeling fuels and fire effects in 3D: Model description and applications. Environmental Modelling & Software 80, 225-244.
| Crossref | Google Scholar |
Prats SA, MacDonald LH, Monteiro M, Ferreira AJD, Coelho COA, Keizer JJ (2012) Effectiveness of forest residue mulching in reducing post-fire runoff and erosion in a pine and a eucalypt plantation in north-central Portugal. Geoderma 191, 115-124.
| Crossref | Google Scholar |
Prichard SJ, Kennedy MC (2014) Fuel treatments and landform modify landscape patterns of burn severity in an extreme fire event. Ecological Applications 24, 571-590.
| Crossref | Google Scholar |
Prichard SJ, Povak NA, Kennedy MC, Peterson DW (2020) Fuel treatment effectiveness in the context of landform, vegetation, and large, wind-driven wildfires. Ecological Applications 30, e02104.
| Crossref | Google Scholar |
Riley KL, Grenfell IC, Finney MA, Wiener JM (2021) TreeMap, a tree-level model of conterminous U.S. forests circa 2014 produced by imputation of FIA plot data. Scientific Data 8, 11.
| Crossref | Google Scholar |
Robichaud PR, Wagenbrenner JW, Brown RE, Wohlgemuth PM, Beyers JL (2008) Evaluating the effectiveness of contour-felled log erosion barriers as a post-fire runoff and erosion mitigation treatment in the western United States. International Journal of Wildland Fire 17, 255-273.
| Crossref | Google Scholar |
Robichaud PR, Elliot WJ, Lewis SA, Miller ME (2016) Validation of a probabilistic post-fire erosion model. International Journal of Wildland Fire 25, 337-350.
| Crossref | Google Scholar |
Robichaud PR, Lewis SA, Wagenbrenner JW, Brown RE, Pierson FB (2020) Quantifying long-term post-fire sediment delivery and erosion mitigation effectiveness. Earth Surface Processes and Landforms 45, 771-782.
| Crossref | Google Scholar |
Rothermel RC (1972) A mathematical model for predicting fire spread in wildland fuels. Research Paper INT-115. p. 115. (U.S. Department of Agriculture, Intermountain Forest and Range Experiment Station 40: Ogden, UT) Available at https://www.fs.usda.gov/treesearch/pubs/32533
Ruefenacht B, Finco MV, Nelson MD, Czaplewski R, Helmer EH, Blackard JA, Holden GR, Lister AJ, Salajanu D, Weyermann D, Winterberger K (2008) Conterminous U.S. and Alaska forest type mapping using forest inventory and analysis data. Photogrammetric Engineering & Remote Sensing 74, 1379-1388.
| Crossref | Google Scholar |
Rust AJ, Hogue TS, Saxe S, McCray J (2018) Post-fire water-quality response in the western United States. International Journal of Wildland Fire 27, 203-216.
| Crossref | Google Scholar |
Sankey JB, Kreitler J, Hawbaker TJ, McVay JL, Miller ME, Mueller ER, Vaillant NM, Lowe SE, Sankey TT (2017) Climate, wildfire, and erosion ensemble foretells more sediment in western USA watersheds. Geophysical Research Letters 44, 8884-8892.
| Crossref | Google Scholar |
Senay GB (2018) Satellite psychrometric formulation of the operational simplified surface energy balance (SSEBop) model for quantifying and mapping evapotranspiration. Applied Engineering in Agriculture 34, 555-566.
| Crossref | Google Scholar |
Song C, Woodcock CE, Li X (2002) The spectral/temporal manifestation of forest succession in optical imagery: The potential of multitemporal imagery. Remote Sensing of Environment 82, 285-302.
| Crossref | Google Scholar |
Staley DM, Tillery AC, Kean JW, McGuire LA, Pauling HE, Rengers FK, Smith JB (2018) Estimating post-fire debris-flow hazards prior to wildfire using a statistical analysis of historical distributions of fire severity from remote sensing data. International Journal of Wildland Fire 27, 595-608.
| Crossref | Google Scholar |
Stephens SL, Collins BM, Roller G (2012) Fuel treatment longevity in a Sierra Nevada mixed conifer forest. Forest Ecology and Management 285, 204-212.
| Crossref | Google Scholar |
Stevens-Rumann CS, Prichard SJ, Strand EK, Morgan P (2016) Prior wildfires influence burn severity of subsequent large fires. Canadian Journal of Forest Research 46, 1375-1385.
| Crossref | Google Scholar |
Strobl C, Boulesteix A-L, Zeileis A, Hothorn T (2007) Bias in random forest variable importance measures: Illustrations, sources and a solution. BMC Bioinformatics 8, 25.
| Crossref | Google Scholar |
Syphard AD, Scheller RM, Ward BC, Spencer WD, Strittholt JR (2011) Simulating landscape-scale effects of fuels treatments in the Sierra Nevada, California, USA. International Journal of Wildland Fire 20, 364-383.
| Crossref | Google Scholar |
Taylor SW, Woolford DG, Dean CB, Martell DL (2013) Wildfire prediction to inform fire management: Statistical science challenges. Statistical Science 28, 586-615.
| Crossref | Google Scholar |
Thomas MA, Rengers FK, Kean JW, McGuire LA, Staley DM, Barnhart KR, Ebel BA (2021) Postwildfire soil-hydraulic recovery and the persistence of debris flow hazards. Journal of Geophysical Research: Earth Surface 126, e2021JF006091.
| Crossref | Google Scholar |
Tomaschek F, Hendrix P, Baayen RH (2018) Strategies for addressing collinearity in multivariate linguistic data. Journal of Phonetics 71, 249-267.
| Crossref | Google Scholar |
USGS [United States Geological Survey] (2023) Emergency Assessment of Post-fire Debris-flow Hazards. Available at https://landslides.usgs.gov/hazards/postfire_debrisflow/ [verified 21 March 2023]
Vaillant NM, Noonan-Wright EK, Reiner AL, Ewell CM, Rau BM, Fites-Kaufman JA, Dailey SN (2015) Fuel accumulation and forest structure change following hazardous fuel reduction treatments throughout California. International Journal of Wildland Fire 24, 361-371.
| Crossref | Google Scholar |
van der Meer FD, van der Werff HMA, van Ruitenbeek FJA (2014) Potential of ESA’s Sentinel-2 for geological applications. Remote Sensing of Environment 148, 124-133.
| Crossref | Google Scholar |
Vieira DCS, Fernández C, Vega JA, Keizer JJ (2015) Does soil burn severity affect the post-fire runoff and interrill erosion response? A review based on meta-analysis of field rainfall simulation data. Journal of Hydrology 523, 452-464.
| Crossref | Google Scholar |
Vogelmann JE, Tolk B, Zhu Z (2009) Monitoring forest changes in the southwestern United States using multitemporal Landsat data. Remote Sensing of Environment 113, 1739-1748.
| Crossref | Google Scholar |
Wells AG, Hawbaker, TJ, Steblein PF, Hiers KJ, Loehman RA, Kean JW (2023) Predicted burn severity of the East Troublesome and Grizzly Creek wildfires for integration with post-fire debris flow in the upper Colorado River basin, USA. U.S. Geological Survey data release. 10.5066/P9T077XK
Whitman E, Parisien M-A, Thompson DK, Hall RJ, Skakun RS, Flannigan MD (2018) Variability and drivers of burn severity in the northwestern Canadian boreal forest. Ecosphere 9, e02128.
| Crossref | Google Scholar |
WIFIRE Commons (2022) FastFuels. Available at https://wifire.ucsd.edu/commons[verified 10 February 2022]
Xi DDZ, Taylor SW, Woolford DG, Dean CB (2019) Statistical models of key components of wildfire risk. Annual Review of Statistics and Its Application 6, 197-222.
| Crossref | Google Scholar |
Ye S, Rogan J, Zhu Z, Hawbaker TJ, Hart SJ, Andrus RA, Meddens AJH, Hicke JA, Eastman JR, Kulakowski D (2021) Detecting subtle change from dense Landsat time series: Case studies of mountain pine beetle and spruce beetle disturbance. Remote Sensing of Environment 263, 112560.
| Crossref | Google Scholar |
Yebra M, Dennison PE, Chuvieco E, Riaño D, Zylstra P, Hunt ER, Danson FM, Qi Y, Jurdao S (2013) A global review of remote sensing of live fuel moisture content for fire danger assessment: Moving towards operational products. Remote Sensing of Environment 136, 455-468.
| Crossref | Google Scholar |