Quantifying burned area of wildfires in the western United States from polar-orbiting and geostationary satellite active-fire detections
Melinda T. Berman
A Department of Atmospheric and Oceanic Sciences, University of California, Los Angeles, Los Angeles, CA, USA.
B Marine Meteorology Division, US Naval Research Laboratory, Monterey, CA, USA.
C National Institute of Aerospace, Hampton, VA, USA.
D NASA Langley Research Center, Hampton, VA, USA.
E NOAA/NESDIS Center for Satellite Applications and Research, College Park, MD, USA.
F Cooperative Institute for Meteorological Satellites Studies, Space and Science Engineering Center, University of Wisconsin-Madison, Madison, WI, USA.
G Institute of the Environment and Sustainability, University of California, Los Angeles, Los Angeles, CA, USA.
International Journal of Wildland Fire 32(5) 665-678 https://doi.org/10.1071/WF22022
Submitted: 1 March 2022 Accepted: 15 March 2023 Published: 26 April 2023
© 2023 The Author(s) (or their employer(s)). Published by CSIRO Publishing on behalf of IAWF. This is an open access article distributed under the Creative Commons Attribution-NonCommercial-NoDerivatives 4.0 International License (CC BY-NC-ND)
Abstract
Background: Accurately estimating burned area from satellites is key to improving biomass burning emission models, studying fire evolution and assessing environmental impacts. Previous studies have found that current methods for estimating burned area of fires from satellite active-fire data do not always provide an accurate estimate.
Aims and methods: In this work, we develop a novel algorithm to estimate hourly accumulated burned area based on the area from boundaries of non-convex polygons containing the accumulated Visible Infrared Imaging Radiometer Suite (VIIRS) active-fire detections. Hourly time series are created by combining VIIRS estimates with Fire Radiative Power (FRP) estimates from GOES-17 (Geostationary Operational Environmental Satellite) data.
Conclusions, key results and implication: We evaluate the performance of the algorithm for both accumulated and change in burned area between airborne observations, and specifically examine sensitivity to the choice of the parameter controlling how much the boundary can shrink towards the interior of the area polygon. Results of the hourly accumulation of burned area for multiple fires from 2019 to 2020 generally correlate strongly with airborne infrared (IR) observations collected by the United States Forest Service National Infrared Operations (NIROPS), exhibiting correlation coefficient values usually greater than 0.95 and errors <20%.
Keywords: active-fire detections, burned area, fire radiative power, GOES-ABI, NIROPS, NOAA-20, satellites, Suomi-NPP, VIIRS, wildfire.
Introduction
In addition to the destruction that wildfires can cause to infrastructure and homes, they also emit large amounts of smoke that contain substances harmful to human health like particulate matter 2.5 (PM2.5) (Wegesser et al. 2009; Munoz-Alpizar et al. 2017). Accurately predicting and estimating wildfire emissions has become critical as more people move into the wildland–urban interface and wildfire activity in the western United States continues to increase (Westerling et al. 2006; Radeloff et al. 2018). Longer fire seasons, and earlier snowmelts and springs contribute to increasing fire activity (Westerling et al. 2006).
Improving quantification of burned area of wildfires is essential to improving biomass burning emissions predictions. The commonly used ‘bottom–up’ methodology requires a combination of fuel information, estimated burned area and emission rates of chemical species (Seiler and Crutzen 1980; French et al. 2011; Paton-Walsh et al. 2012). Fuel availability and other bottom–up components may better predict carbon emissions and changes in fire size (Fernandes et al. 2016; Walker et al. 2020). Improved burned area estimates may therefore facilitate improved predictions of biomass burning emissions for a variety of modelling applications. Recent work in biomass burning emissions predictions has used a fusion of polar-orbiting and geostationary sources to enhance hourly estimates (Li et al. 2022).
Satellite remote sensing provides the only pathway to quantify fire activity and biomass burning emissions worldwide. These active-fire detection data have the capacity to estimate burned area and emissions using instruments on board polar-orbiting satellites such as the Moderate Resolution Imaging Spectroradiometer (MODIS) or the Advanced Very High Resolution Radiometer (AVHRR) (Soja et al. 2004; Sukhinin et al. 2004; Wiedinmyer et al. 2010). However, the resolution of the fire products for AVHRR and MODIS (Csiszar et al. 2003; Giglio et al. 2006), which at nadir are 1.1 and 1 km respectively (Belward and Lambin 1990; Giglio et al. 2016), is not sufficient to capture details of individual fire fronts located within a given pixel (Peterson and Wang 2013; Peterson et al. 2013; Schmidt 2019).
Fire detections from the Visible Infrared Imaging Radiometer Suite (VIIRS) I-Band sensor have an enhanced nadir spatial resolution of 375 m, thus the capacity to detect smaller fires and increase overall accuracy of burned area estimations (Schroeder et al. 2014; Oliva and Schroeder 2015). This imagery provides the higher resolution necessary to approximate a solution for the Gauss Circle Problem (Berndt et al. 2018), which constrains the number of integer lattice points needed to define the area of a polygon, which is the basis for our method. The higher-resolution VIIRS data enable an increased number of active-fire detections in a fire perimeter compared with MODIS or AVHRR (Goldberg et al. 2013; Wolfe et al. 2013; Schroeder et al. 2014). Gaps still remain, however, in assessing how well VIIRS sensors can be used to estimate burned area (Briones-Herrera et al. 2020), especially utilising the VIIRS sensor launched aboard the National Oceanic and Atmospheric Administration (NOAA) NOAA-20 satellite launched in 2017.
In addition to active-fire detections from polar-orbiting satellites, active-fire detections and fire radiative power (FRP) derived from geostationary satellite sensors, such as the Geostationary Operational Environmental Satellite (GOES)-R Series, can also be used to characterise fire behaviour (Schmidt 2019). The GOES-17 Advanced Baseline Imager produces fire information on a fairly coarse spatial footprint covering 5–8 km2 over the contiguous United States based on Wildfire Automated Biomass Burning Algorithm (WFABBA) outputs. However, when compared with twice-daily observations from polar-orbiting satellite sensors, geostationary sensors provide a much higher temporal resolution, with scans every 5 min over the continental United States (CONUS) (Schmit and Gunshor 2019). This finer temporal resolution provides a detailed representation of fire behaviour over time, which is critical for estimating growth between overpasses of polar-orbiting sensors.
Large incidents in the western United States are routinely observed by the National Infrared Operations (NIROPS) program run by the United States Forest Service (USFS) using airborne infrared (IR) sensors (Page et al. 2019). Verification of satellite-based burned area with aircraft observations can be challenging owing to the temporal offset between polar-orbiting satellite overpasses and these aircraft observations (Oliva and Schroeder 2015). If a satellite overpass occurs during a time of major fire growth after aircraft have already observed the fire, it may appear that the satellite is overestimating true fire size, when in reality it may be accurately estimating fire size at the time of the overpass.
This study develops a new method to estimate burned area using a combination of polar-orbiting and geostationary satellite sensors. Incorporating near-continuous data from geostationary satellite sensors based on FRP variations observed with burned area estimates from polar-orbiting satellite sensors, hourly time series of fire burned area can be obtained. This method can be used to reduce the impact of time offsets between airborne and polar-orbiting satellite overpasses. The main improvement this method provides is a way to achieve highly accurate temporal burned area estimates without sacrificing high spatial resolution. This can be helpful in multiple applications, such as calculations of hourly emissions for bottom–up approaches without having to apply fixed diurnal cycles (Ye et al. 2021) assisting with evaluation of methods to predict fire spread at hourly time resolution that are generally evaluated at coarser time resolutions such as those from VIIRS, NIROPS or with final perimeters (Muñoz-Esparza et al. 2018; Coen et al. 2020).
Study region and test fires
This study focuses on the wildfires in the western United States (final fire sizes from 4200 to >100 000 ha) shown in Fig. 1. The western United States has robust spatial and temporal coverage from both geostationary satellites, like GOES-17 (occupying the GOES-West position during the study period), and polar-orbiting satellites. Additionally, the western United States has frequent IR observations of large fires from NIROPS.
![]() |
Williams Flats
The Williams Flats Fire was selected for detailed examination in this study. It burned on the Colville Indian Reservation in Washington State from 2 August 2019 until it was fully contained on 25 August 2019 with a final size of 17 986 ha, according to the Incident Command System ICS-209 report. The Williams Flats Fire was heavily observed and exhibited a range of fire growth patterns. The fire was monitored by NIROPS, multiple satellites and NASA’s ER-2 and DC-8 aircraft during the Fire Influence on Regional to Global Environments Experiments-Air Quality (FIREX-AQ) field campaign (Warneke et al. 2022).
The Williams Flats Fire exhibited unique patterns of diurnal fire growth. During the first days after ignition, the fire followed a typical diurnal pattern of fire growth (Mu et al. 2011; Andela et al. 2015) with the largest growth occurring during the afternoon and the fire becoming less active at night. As the fire continued to grow, however, it actively burned overnight. This behaviour has been repeatedly observed in large western wildfires during periods of extreme fire growth (Peterson et al. 2015; Saide et al. 2015). Large active periods of fire growth were detected by satellites overnight, especially on 7 and 8 August 2019 UTC (Coordinated Universal Time). The Williams Flats Fire exhibited extreme fire behaviour on 8 August 2019 UTC when the fire produced multiple pyrocumulonimbus (National Aeronautics and Space Administration (NASA) 2019).
Other 2019 fires
In addition to the Williams Flats Fire, other fires sampled during the FIREX-AQ field campaign and notable incidents from 2019 were used (Table 1). Additional fires were chosen to diversify the location, fire behaviour, size and topography, among other features, to provide rigorous testing of the algorithm across a variety of conditions. Of the 2019 fires, the 204 Cow and Walker Fires are also discussed in the text. Detailed statistics and maps for the other 2019 fires are in the supplement.
![]() |
2020 fires
2020 was a record-breaking fire season with some of the largest fires in state history for multiple states in the United States. In total, more than 4 million ha burned in the United States (National Interagency Fire Center n.d.). Table 1 shows the fires chosen from the 2020 fire season. All fires studied are single incident fires; none are complexes. Complexes are two or more incidents in a general area managed by the same incident commander or a unified command (United States Forest Service n.d.). Complexes are an area of future research to continue to explore the performance of the algorithm. Of the 2020 fires, the Dolan, Lake and Riverside Fires are analysed here, with the statistics and maps for the remaining fires found in the supplement.
Observational datasets
VIIRS 375 m data
The NASA-generated VIIRS Active Fire 375 m VNP14IMG and VJ114IMGTDL Collection 1 data products and compatible NOAA-generated products are available from both the Suomi National Polar-orbiting Partnership (SNPP) (2019 and 2020) and NOAA-20 (2020) satellites of the Joint Polar Satellite System (Schroeder and Giglio 2017). SNPP flies in a sun-synchronous orbit, crossing the equator at ~1330 hours and ~0130 hourslocally for ascending and descending nodes, respectively, while NOAA-20 also has a local equatorial crossing time of ~0130/1330 hours and has ~50.5 min of separation from SNPP (Wolfe et al. 2013; Schroeder et al. 2014; Cao et al. 2018).
GOES data
The GOES-17 ABI (referred to as ABI hereafter) FRP data from the WFABBA Versions 6_5_012g and 6_6_001g hotspot detection algorithm were used (Schmidt 2019). Most 2019 data are available on the FIREX-AQ archive, while some 2019 and all 2020 ABI FRP data were obtained directly from University of Wisconsin Space Science and Engineering Center (SSEC). Although the GOES-W ABI spatial resolution is coarser than the VIIRS spatial resolution (5–8 km2 for the CONUS based on WFABBA outputs), the ABI data have a much higher temporal resolution at 5 min over CONUS. The relatively large size of the detections makes the area estimates much larger than reality using an accumulation method. FRP is an instantaneous estimate of the power released by a fire and has been extensively tied to various measurements of fire behaviour and intensity (Li et al. 2018). Additionally, geostationary FRP has been shown to be well correlated with fire behaviour and aerosol and gas emissions from wildfires (Wiggins et al. 2020). As a result, ABI FRP data were used to describe the temporal evolution of burned area and are expected to result in a more realistic evolution than linearly interpolating VIIRS estimates.
NIROPS data
To evaluate the estimated burned area from the satellite detections, fire size was also estimated by the USFS’ NIROPS program, which maps large incidents in the United States using both dedicated USFS airborne IR sensors (Greenfield et al. 2003) and privately owned sensors flown under contract. Both USFS and contractor flights were used in the present study, collectively referred to as NIROPS, but are denoted separately in figures. Area estimates included in daily fire perimeter maps from NIROPS consist of the outer NIROPS polygon, which does not include interior areas like unburned islands. NIROPS data are the best available data for detecting ‘daily’ burn perimeters, when available. Even though ICS-209 reports and GeoMAC perimeters are the best estimates of the total burned area of the fire scars, the daily data can be vastly under- and overestimated. Further details about all datasets can be found in the supplementary material.
Fire burned area algorithm
Identification of fire perimeter and selection of satellite pixels
VIIRS data for a fire were filtered within a bounding box based on the latitude/longitude range of the final map from NIROPS (Fig. 2). This range was chosen to ensure that the entire area of the fire was included in our estimation, as well as providing a consistent framework to evaluate across datasets. Some fires required further geographic filtering using a polygon bounding box. This secondary filtering was needed when there were other incidents or spot fires within the initial bounding box to prevent their inclusion in the area estimates. Spot fires within 0.1° of the fire and included on the NIROPS perimeter map were not filtered out, as they are reasonably close to the main body of the fire. This further filtering increases accuracy of the area estimate by removing close active-fire detections that are not from the main incident. This smaller bounding box was not applied to all fires, but only when needed, and was applied to both VIIRS and ABI detections. The filter was applied to the Cameron Peak, Creek, Holiday Farm and Riverside fires.
![]() |
Once spatially filtered, active-fire detections from both SNPP and NOAA-20 are accumulated beginning from 00 UTC on the day the fire began to the end of the day of the last NIROPS flight used for the fire. There are cases where fires continued to have active-fire detections after the last NIROPS flight, but we chose to end our estimations when the NIROPS flights ended. There are also cases with NIROPS flight when there were no new active-fire detections since the previous NIROPS flight. Those NIROPS flights were removed when evaluating the algorithm but are included in time series plots.
Calculation of fire area from VIIRS active-fire detections
Area is calculated for every overpass by drawing a polygon around the accumulated detections. This polygon is drawn using MATLAB’s boundary function. The algorithm consists of constructing an alpha shape (Edelsbrunner et al. 1983) from the specified points and then determining which points lie on the boundary. The convexity of the hull derived from the accumulated detections is changed by modifying the shrink factor, an input parameter to the boundary function that controls the radius used to build the alpha shape. The shrink factor ranges from 0 to 1, with 0 resulting in a convex hull and 1 providing the most compact single polygon around the detections, which is generally non-convex (The MathWorks, Inc 2022). Non-convexity allows the exclusion of unburned area around the generally irregular fire perimeters.
Application of ABI FRP data to refine temporal evolution
Once the VIIRS detections have been processed, and area decreases have been filtered out and combined by overpass time, a continuous, hourly time series can be created with hourly ABI FRP data. Averaged ABI FRP estimates, with units of megawatts, are integrated over the entire life of the fire to create a cumulative FRP estimate, also known as Fire Radiative Energy (FRE). The FRE is then used to interpolate between VIIRS area estimates using:
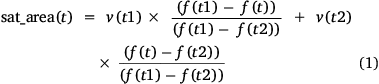
where sat_area corresponds to the combined burned area estimates in hectares, v corresponds to the VIIRS area estimates in hectares, and f corresponds to the ABI FRE in megajoules. The times t, t1 and t2 are the current time, closest overpass before the current time and closest overpass after the current time, respectively. The equation is run for each hour during the life of the fire and for 10 shrink factor values ranging from 0.1 to 1. When FRP is constant with time or there are no FRP measurements, a linear interpolation is used to estimate area between the overpasses. To examine how well the model predicts both accumulated burned area and change in burned area, the normalised mean bias (NMB), normalised mean error (NME), mean absolute error (MAE), root mean square error (RMSE) and mean bias (MB) were calculated (Willmott and Matsuura 2005; Eder and Yu 2006).
Results
The combined time series from VIIRS and ABI were evaluated, using NIROPS as a reference, in two ways: by total accumulated burned area and by change in burned area between NIROPS flights. Flight times were converted from local time to UTC, and rounded to the nearest hour, for easier comparison with accumulated burned area. The latter approximately corresponds to daily burned area where NIROPS flew on consecutive days. Obtaining strong agreement for both metrics ensures that the algorithm is not only estimating true fire size well, but that it is accurately capturing changes in fire behaviour, which may improve bottom–up emissions estimates as they generally use daily changes in burned area.
Spatial agreement
Fig. 2 shows accumulated fire detections against the final NIROPS heat perimeter for the Williams Flats Fires, as well as the boundary with a shrink factor of 1 (the most compact polygon). The fire shows good spatial agreement between the active-fire detections and NIROPS perimeter. There are some interior areas of another large 2019 fire, the Walker Fire, surrounded by VIIRS detections and they are included in our burned area estimations, but did not burn according to NIROPS (Supplementary Fig. S14). Despite these unburned ‘islands’, which are a known problem for all burned area estimations (Kolden et al. 2012; Hall et al. 2020) that worsens with coarser-resolution data, the outer VIIRS perimeter for the Walker Fire has good spatial agreement with the final NIROPS heat perimeter. Spatial agreement assesses how well the filters work to retain only detections from the incident, a critical component for accurate burned area estimates.
For the 204 Cow Fire, a relatively small 2019 fire (3912 ha), initial examination of the satellite perimeter against the NIROPS perimeter indicated further geographic filtering would be necessary (Fig. 3). The NOAA-20 pass on 29 August at 0900 UTC contains a number of detections in the vicinity of the fires deemed to be false, resulting in a large overestimation in burned area and an incorrect perimeter. To filter out these false detections, detections and boundaries from both VIIRS sensors were used to find a common set of points. Once detections were accumulated for both SNPP and NOAA-20, boundaries were created for both sets. The boundary from each satellite was then applied to the other set of detections; the SNPP boundary was applied to the set of NOAA-20 detections and vice versa, as shown in Fig. 3. With this additional filtering, agreement between the satellite and NIROPS perimeter was greatly improved.
![]() |
The accumulated fire detections with the final NIROPS perimeter and most compact shrink factor for three of the 2020 fires, the Dolan, Lake and Riverside Fires, are shown in Supplementary Figs S29, S38, S41. Like 2019, there are fires where the algorithm has limitations. The 2020 Lake Fire that occurred in the Angeles National Forest is a prime example of cloud cover affecting the detection of active burning (Incident Information Web Services 2020). Owing to persistent cloud cover early in the fire, some active-fire detections were missed, leading to large underestimations (~3800 ha max.) in burned area that affected the subsequent area estimations (Fig. S39). The accumulated burned area is persistently low-biased compared with NIROPS, and there are small (R < 0.3) to negative R values for the change in burned area (Fig. S40). We note that although clouds decrease algorithm skill for the Lake Fire, the algorithm is capable of overcoming cloud cover, such as in the 204 Cow Fire.
Accumulated burned area
Figs 4, 5 show the combined NOAA-20 and SNPP VIIRS time series (multicoloured symbols for different shrink factors from 0.5 to 0.8), the accumulated FRP (green line) and the burned area estimate for the 0.5 shrink factor (black dashed line) for the 204 Cow, Walker and Williams Flats (Fig. 4) and Dolan, Lake and Riverside (Fig. 5) Fires. Area estimations from SNPP and NOAA-20, multicoloured symbols for four shrink factors (S = 0.5 to 0.8) shown, visually agree well with the values and trend of the NIROPS (black circles) estimates for the Williams Flats and 204 Cow Fires. The estimations mainly agree with the trend for the Walker Fire but overestimate the final NIROPS area by 14–32% (~3000–7800 ha), depending on the shrink factor, owing to unburned islands being included in the area estimated. Errors in burned area for the 204 Cow and Williams Flats Fires range from −2.2 to 9.6% (−87 to ~400 ha) and −3.5 to 18% (~−600 to ~3300 ha).
![]() |
The top of Supplementary Figs S3, S16, S19, S31, S40, S43 compares the NIROPS area estimations with the estimated accumulated burned area at the same time and shows the correlation coefficient for four of the mid-range shrink factors (0.5, 0.6, 0.7 and 0.8). All of the fires show high R values (>0.98) for accumulated burned area for those shrink factors. Having very high positive correlation coefficient values for all of the fires makes sense, as a strong relationship between satellite estimated accumulated burned area and NIROPS perimeter areas over time is expected, regardless of high or low biases that may arise from detection mapping issues. The high and low biases for fires like the Walker and Lake Fires become evident when looking at the correlation plots.
Error metrics for all fires with linear interpolations between the VIIRS overpasses only can be found in the supplement (Supplementary Tables S1–S3). For most fires, there is minimal change between the calculated error metrics without the inclusion of the ABI data (R = 0.52 vs 0.50 for Pedro Mountain). NIROPS flights and VIIRS night-time overpasses tend to occur at similar times of typically decreased fire activity. This will yield similar results between the methods with and without the inclusion of ABI FRP when NIROPS and VIIRS area estimates are compared.
Change in burned area
The bottom of Supplementary Figs S3, S16, S19, S31, S40, S43 compares change in burned area estimates for all six fires, with a variety of results. The Dolan, Riverside, Walker and Williams Flats Fires all have high correlation coefficients (R ≥ 0.96), whereas the 204 Cow and Lake Fires have much lower, and even negative, correlation coefficients (R < 0.5). The 204 Cow and Lake Fires are both <13 000 ha in size compared with the other four being >22 000 ha, indicating a potential dependence on fire size for accuracy of the change in burned area estimates. These values do not necessarily mean that the algorithm does a poor job at predicting change in burned area as many factors can impact correlation values (Aggarwal and Ranganathan 2016).
Additional error metrics
Accumulated burned area
Table 2 compares error metrics for the Williams Flats Fire across all shrink factors from 0.1 to 1. Although the range in the errors across the shrink factors is small, there is not one shrink factor that is universally better than the others. However, shrink factors in the range of 0.7–1.0 (the most compact shrink factors) tend to produce the smallest errors, <6% for NMB and <11% for NME for the Williams Flats Fire. This trend applies with the other error metrics calculated as well, with the smallest MB, RMSE and MAE values in the S = 0.7 to 1.0 range, with most being the smallest at S = 0.8.
![]() |
The algorithm performs similarly across a range of fire sizes, and sensitivity to the shrink factor is small relative to other errors. Table 3 compares all 2019 fires at the 0.8 shrink factor, more compact than the default setting of 0.5. The NMB and NME for accumulated burned area range from −23.7 to 19.4% and 6.5 to 23.7% respectively. Excluding the Granite Gulch and Walker Fires, the range of NMB and NME drops to ~±6 and <12%, respectively, with an overall slight underprediction. The NMB and NME values are similar to other error values from previous studies (Oliva and Schroeder 2015), with the exception of the Granite Gulch and Walker Fires, which have slightly larger errors, (−4.1 to 1.4% for NMB and 6.5–11.9% for NME) but within the error range (<~50%) seen in Oliva and Schroeder (2015).
![]() |
Statistics for 2020 fires are slightly worse than for 2019. Excluding the Lake Fire, the range of NMB and NME is −10 to +13% and 2 to <14%, respectively for the S = 0.8 shrink factor. Three of these fires (Riverside, Holiday Farm and Creek) show NBM values larger than 10%. The Riverside Fire shows an overestimation due to spot fires near the fire (Table 4), but the change in burned area error metrics shows an underestimation for most shrink factors. Holiday Farm presents overestimations due to spotting as well (Fig. S24), while the Creek Fire has overestimations due to large irregularities in the fire perimeter and large unburned islands. The Lake Fire has larger errors than the other 2020 fires (~40% NME), due to previously described complications with cloud cover. Despite some large errors, the consistency across fire size shows the algorithm can handle both very large and small fires well.
![]() |
Change in burned area error metrics
There is less of an identifiable trend in the 2019 change in burned area error metrics (Table 3). Larger spread is expected as uncertainty and error are introduced when taking the difference between times, but the range of values for NMB (from approximately −18 to +50%) and NME (approximately 30–73%) is large. These large spreads show the error ranges widely and appears to be independent of fire size. For instance, while the two smallest fires have the smallest NMB values, the Walker Fire has a smaller NMB than the Williams Flats or Pedro Mountain Fires (which are smaller in size) for change in burned area. Although the Walker Fire has the worst skill for accumulated burned area, we note that fire growth estimates can still have skill even when the algorithm overpredicts accumulated burned area.
Excluding the Creek Fire, the 2020 fires have similar error values to 2019 fires (NMB, −22 to 8%, NME 33 to 64%, Table 4). In the case of the Creek Fire, previously described irregularities in fire perimeter led to large overestimations of true fire size that also impacted the change in burned area errors. There is also a much larger range (−0.14 to 0.99) of correlation coefficients between the algorithm estimated change in burned area and the NIROPS change in burned area (Table 4). This is much larger than the range of accumulated burned area correlation coefficients for the 2020 fires (0.85–0.99), but the NMB and NME ranges are comparable with the 2019 ranges. The algorithm has a tendency to slightly underpredict at this shrink factor (S = 0.8), with negative NMB values for all fires except for Cameron Peak and Holiday Farm.
Comparison with other datasets
The results of the algorithm for Williams Flats can be evaluated against the burned area estimates the FIREX-AQ Fuel2Fire team performed (https://www-air.larc.nasa.gov/cgi-bin/ArcView/firexaq?ANALYSIS=1#SOJA.AMBER/). MODIS and VIIRS active-fire detections were used to estimate daily burned area by assuming an instrument resolution footprint of 1 km and 375 m respectively, and then removing overlapping areas, similarly to the methods of Oliva and Schroeder (2015) and allowing comparison between different methods with similar inputs. Area is accumulated over every local day, and time is then converted to UTC for comparison. For the Williams Flats Fire, there is a strong correlation (R = 0.96) between the accumulated algorithm burned area estimates and the Fuel2Fire estimates (Fig. 6). The strong correlation is seen across the shrink factors shown from S = 0.5 to 0.8. The final burned area estimates for all fires for the 0.8 shrink factor were also compared with the final burned area from ICS-209 reports (Fig. 6). There is good agreement (R = 0.99) between the algorithm and the ICS-209 reports burned area estimates. These results are encouraging as they prove the applicability of this method and show that when compared with different data, the algorithm-estimated final burned area is usually close to that measured in official reports.
Discussion and conclusions
We developed a novel algorithm to estimate burned area of wildfires from satellite active-fire detections. Using active-fire detections from NOAA-20 and SNPP VIIRS data and FRP estimates from GOES ABI data, we can generate generally accurate hourly burned area estimates of wildfires. Once geographically filtered, acccumulated active-fire detections visually compare well with the USFS NIROPS airborne-derived perimeters. Using polygons of different convexity around accumulated fire detections provides a measure of uncertainty in the algorithm. Inclusion of unburned islands in fire interiors remains an issue for accumulated burned area estimates, however. Although there are some manual components to this method, in the future, it could be the basis for automated techniques and be applied to other regions.
Larger shrink factors, i.e. more compact polygons, typically provide better results as they minimise the inclusion of unburned islands and irregular perimeters. Some smaller fires, however, have better results with smaller shrink factors and less compact polygons, indicating a potential size dependence on shrink factor. There is not one shrink factor that minimises all errors universally, but rather the choice of shrink factor is driven by the type of error that should be minimised. The inclusion of ABI FRP data does not significantly improve the algorithm, but does better capture the pronounced diurnal cycle of fires, making the estimates more realistic (Mu et al. 2011; Wiggins et al. 2020; Li et al. 2022).
Errors (NME) in accumulated burned area for most fires are below 14%. Larger underpredictions are found when clouds obscure detections at the edge of the final perimeter of the fire, whereas large overpredictions occur when the fire has unburned islands, spotting or highly irregular fire perimeters. Change in burned area results sees a wider spread in errors, typically between 30 and 73%, with one outlier over 200% owing to irregular fire perimeters impacting accumulated burned area estimates. Smaller fires, relative to other fires in the same season, tend to have smaller NMB and NME values. Many of the patterns with fire size and corresponding trends in error metrics seen in 2019 are also seen in 2020. Correlation coefficients are usually >0.95 for accumulated burned area, but more variable for change in burned area, with R typically >0.89, but with some R values <0.5. When compared with other burned area datasets, both the accumulated burned area and final estimated burned area perform well, with correlation coefficients >0.96.
Realistic burned area estimates can improve emissions estimations for air quality forecasts, potentially in near-real time. Many air quality and emissions models currently rely on persistence to forecast burned area, which can lead to drastic over- or underestimations in emissions predictions (Ye et al. 2021). Burned area estimates from the present algorithm can be used to inform better predictions of burned area; using methods such as machine learning shows tremendous potential for forecasting fire spread and emissions, especially if trained with fire weather and fuels, variables that control fire growth and spread (Reid et al. 2015; Jain et al. 2020). Recent work shows the uses of ABI FRP to predict hourly biomass burning estimates (Wiggins et al. 2020). When used with near-real time burned area, following a similar approach with hourly ABI FRP estimates, emissions and air quality forecasts are expected to be improved owing to the strong correlation between ABI FRP and smoke concentrations (Wiggins et al. 2020).
Supplementary material
Supplementary material is available online.
Data availability
Suomi NPP and NOAA-20 VIIRS I-band fire data for 2019 were provided to FIREX-AQ by NOAA/NESDIS Center for Satellite Applications and Research (DOI: 10.5067/SUBORBITAL/FIREXAQ2019/DATA001). Some 2019 and all 2020 SNPP and NOAA-20 data were downloaded from the NASA FIRMS archive (VJ114IMGTDL_NRT; DOI: 10.5067/FIRMS/VIIRS/VJ114IMGT_NRT.002, VNP14IMGTDL_NRT; DOI: 10.5067/FIRMS/VIIRS/VNP14IMGT_NRT.002). GOES-16 and GOES-17 ABI data for 2019 were provided to FIREX-AQ by the University of Wisconsin SSEC. Some 2019 and all 2020 data for GOES-17 were acquired directly from the University of Wisconsin SSEC. Fuel2Fire burned area estimates are from communication with the Fuel2Fire team; Fuel2Fire emissions estimates can be found at https://www-air.larc.nasa.gov/cgi-bin/ArcView/firexaq?ANALYSIS=1#SOJA.AMBER/. In addition to the public sources described, all satellite data used can be found at the following DOI: https://ezid.cdlib.org/id/doi:10.15144/S4CC7K. All information for fires can be found in the online supplementary material. Incident-specific NIROPS data are from the NIFC NIROPS file repository (https://ftp.wildfire.gov/public/incident_specific_data/).
Conflicts of interest
The authors declare no conflicts of interest.
Declaration of funding
The research and preparation of this article was supported by the following grants: NASA: 80NSSC18K0629, 80NSSC18K0685, 80NSSC20K1650, 80HQTR18T0063; NOAA: NA18OAR4310107; NSF: 2013461.
Acknowledgements
We acknowledge the use of data and/or imagery from NASA’s Fire Information for Resource Management System (FIRMS) (https://earthdata.nasa.gov/firms), part of NASA’s Earth Observing System Data and Information System (EOSDIS). We acknowledge the use of data and/or imagery from NASA’s Land, Atmosphere Near real-time Capability for EOS (LANCE) system (https://earthdata.nasa.gov/lance), part of NASA’s EOSDIS. Code snippet to flatten some NIROPS shapefiles from 3D to 2D can be found at https://gist.github.com/rmania/8c88377a5c902dfbc134795a7af538d8. The authors would like to thank the entire FIREX-AQ science team including the leadership team: James Crawford, Jack Dibb, Joshua Schwarz and Carsten Warneke.
References
Aggarwal R, Ranganathan P (2016) Common pitfalls in statistical analysis: the use of correlation techniques. Perspectives in Clinical Research 7, 187–190.| Common pitfalls in statistical analysis: the use of correlation techniques.Crossref | GoogleScholarGoogle Scholar |
Andela N, Kaiser JW, van der Werf GR, Wooster MJ (2015) New fire diurnal cycle characterizations to improve fire radiative energy assessments made from MODIS observations. Atmospheric Chemistry and Physics 15, 8831–8846.
| New fire diurnal cycle characterizations to improve fire radiative energy assessments made from MODIS observations.Crossref | GoogleScholarGoogle Scholar |
Belward AS, Lambin E (1990) Limitations to the identification of spatial structures from AVHRR data. International Journal of Remote Sensing 11, 921–927.
| Limitations to the identification of spatial structures from AVHRR data.Crossref | GoogleScholarGoogle Scholar |
Berndt BC, Kim S, Zaharescu A (2018) The circle problem of Gauss and the divisor problem of Dirichlet – still unsolved. The American Mathematical Monthly 125, 99–114.
| The circle problem of Gauss and the divisor problem of Dirichlet – still unsolved.Crossref | GoogleScholarGoogle Scholar |
Briones-Herrera CI, Vega-Nieva DJ, Monjarás-Vega NA, Briseño-Reyes J, López-Serrano PM, Corral-Rivas JJ, Alvarado-Celestino E, Arellano-Pérez S, Álvarez-González JG, Ruiz-González AD, Jolly WM, Parks SA (2020) Near real-time automated early mapping of the perimeter of large forest fires from the aggregation of VIIRS and MODIS active fires in Mexico. Remote Sensing 12, 2061
| Near real-time automated early mapping of the perimeter of large forest fires from the aggregation of VIIRS and MODIS active fires in Mexico.Crossref | GoogleScholarGoogle Scholar |
Cao C, Blonski S, Wang W, Uprety S, Shao X, Choi J, Lynch E, Kalluri S (2018) NOAA-20 VIIRS on-orbit performance, data quality, and operational Cal/Val support. In ‘Earth observing missions and sensors: development, implementation, and characterization V’. (Eds X Xiong, T Kimura) p. 107810K. (SPIE)
| Crossref |
Coen JL, Schroeder W, Conway S, Tarnay L (2020) Computational modeling of extreme wildland fire events: a synthesis of scientific understanding with applications to forecasting, land management, and firefighter safety. Journal of Computational Science 45, 101152
| Computational modeling of extreme wildland fire events: a synthesis of scientific understanding with applications to forecasting, land management, and firefighter safety.Crossref | GoogleScholarGoogle Scholar |
Csiszar I, Abuelgasim A, Li Z, Jin J, Fraser R, Hao W-M (2003) Interannual changes of active fire detectability in North America from long-term records of the advanced very high resolution radiometer. Journal of Geophysical Research: Atmospheres 108, 4075
| Interannual changes of active fire detectability in North America from long-term records of the advanced very high resolution radiometer.Crossref | GoogleScholarGoogle Scholar |
Edelsbrunner H, Kirkpatrick D, Seidel R (1983) On the shape of a set of points in the plane. IEEE Transactions on Information Theory 29, 551–559.
| On the shape of a set of points in the plane.Crossref | GoogleScholarGoogle Scholar |
Eder B, Yu S (2006) A performance evaluation of the 2004 release of Models-3 CMAQ. Atmospheric Environment 40, 4811–4824.
| A performance evaluation of the 2004 release of Models-3 CMAQ.Crossref | GoogleScholarGoogle Scholar |
Fernandes PM, Monteiro-Henriques T, Guiomar N, Loureiro C, Barros AMG (2016) Bottom–up variables govern large-fire size in Portugal. Ecosystems 19, 1362–1375.
| Bottom–up variables govern large-fire size in Portugal.Crossref | GoogleScholarGoogle Scholar |
French NHF, de Groot WJ, Jenkins LK, Rogers BM, Alvarado E, Amiro B, de Jong B, Goetz S, Hoy E, Hyer E, Keane R, Law BE, McKenzie D, McNulty SG, Ottmar R, Pérez-Salicrup DR, Randerson J, Robertson KM, Turetsky M (2011) Model comparisons for estimating carbon emissions from North American wildland fire. Journal of Geophysical Research: Biogeosciences 116, G00K05
| Model comparisons for estimating carbon emissions from North American wildland fire.Crossref | GoogleScholarGoogle Scholar |
Giglio L, van der Werf GR, Randerson JT, Collatz GJ, Kasibhatla P (2006) Global estimation of burned area using MODIS active fire observations. Atmospheric Chemistry and Physics 6, 957–974.
| Global estimation of burned area using MODIS active fire observations.Crossref | GoogleScholarGoogle Scholar |
Giglio L, Schroeder W, Justice CO (2016) The Collection 6 MODIS active fire detection algorithm and fire products. Remote Sensing of Environment 178, 31–41.
| The Collection 6 MODIS active fire detection algorithm and fire products.Crossref | GoogleScholarGoogle Scholar |
Goldberg MD, Kilcoyne H, Cikanek H, Mehta A (2013) Joint Polar Satellite System: the United States next generation civilian polar-orbiting environmental satellite system. Journal of Geophysical Research: Atmospheres 118, 13,463–13,475.
| Joint Polar Satellite System: the United States next generation civilian polar-orbiting environmental satellite system.Crossref | GoogleScholarGoogle Scholar |
Greenfield PH, Smith W, Chamberlain DC (2003) Phoenix – the new Forest Service airborne infrared fire detection and mapping system. In ‘2nd International Wildland Fire Ecology and Management Congress and 5th Symposium on Fire and Forest Meteorology’, 16–20 November 2003, Orlando, FL, J1G.3. Available at https://ams.confex.com/ams/FIRE2003/techprogram/paper_66675.htm
Hall RJ, Skakun RS, Metsaranta JM, Landry R, Fraser RH, Raymond D, Gartrell M, Decker V, Little J (2020) Generating annual estimates of forest fire disturbance in Canada: the National Burned Area Composite. International Journal of Wildland Fire 29, 878–891.
| Generating annual estimates of forest fire disturbance in Canada: the National Burned Area Composite.Crossref | GoogleScholarGoogle Scholar |
Inciweb Incident Information Services (2020) Lake Fire. Available at https://inciweb.nwcg.gov/incident/6953/ [verified May 2021]
Jain P, Coogan SCP, Subramanian SG, Crowley M, Taylor S, Flannigan MD (2020) A review of machine learning applications in wildfire science and management. Environmental Reviews 28, 478–505.
| A review of machine learning applications in wildfire science and management.Crossref | GoogleScholarGoogle Scholar |
Kolden CA, Lutz JA, Key CH, Kane JT, van Wagtendonk JW (2012) Mapped versus actual burned area within wildfire perimeters: characterizing the unburned. Forest Ecology and Management 286, 38–47.
| Mapped versus actual burned area within wildfire perimeters: characterizing the unburned.Crossref | GoogleScholarGoogle Scholar |
Li F, Zhang X, Kondragunta S, Csiszar I (2018) Comparison of fire radiative power estimates from VIIRS and MODIS observations. Journal of Geophysical Research: Atmospheres 123, 4545–4563.
| Comparison of fire radiative power estimates from VIIRS and MODIS observations.Crossref | GoogleScholarGoogle Scholar |
Li F, Zhang X, Kondragunta S, Lu X, Csiszar I, Schmidt CC (2022) Hourly biomass burning emissions product from blended geostationary and polar-orbiting satellites for air quality forecasting applications. Remote Sensing of Environment 281, 113237
| Hourly biomass burning emissions product from blended geostationary and polar-orbiting satellites for air quality forecasting applications.Crossref | GoogleScholarGoogle Scholar |
Mu M, Randerson JT, van der Werf GR, Giglio L, Kasibhatla P, Morton D, Collatz GJ, Defries RS, Hyer EJ, Prins EM, Griffith DWT, Wunch D, Toon GC, Sherlock V, Wennberg PO (2011) Daily and 3-hourly variability in global fire emissions and consequences for atmospheric model predictions of carbon monoxide. Journal of Geophysical Research: Atmospheres 116, D24303
| Daily and 3-hourly variability in global fire emissions and consequences for atmospheric model predictions of carbon monoxide.Crossref | GoogleScholarGoogle Scholar |
Munoz-Alpizar R, Pavlovic R, Moran MD, Chen J, Gravel S, Henderson SB, Ménard S, Racine J, Duhamel A, Gilbert S, Beaulieu PA, Landry H, Davignon D, Cousineau S, Bouchet V (2017) Multi-year (2013–2016) PM2.5 wildfire pollution exposure over North America as determined from operational air quality forecasts. Atmosphere 8, 179
| Multi-year (2013–2016) PM2.5 wildfire pollution exposure over North America as determined from operational air quality forecasts.Crossref | GoogleScholarGoogle Scholar |
Muñoz-Esparza D, Kosović B, Jiménez PA, Coen JL (2018) An accurate fire-spread algorithm in the weather research and forecasting model using the level-set method. Journal of Advances in Modeling Earth Systems 10, 908–926.
| An accurate fire-spread algorithm in the weather research and forecasting model using the level-set method.Crossref | GoogleScholarGoogle Scholar |
National Aeronautics and Space Administration (2019) Flying through a Fire Cloud. Available at https://earthobservatory.nasa.gov/images/145446/flying-through-a-fire-cloud [verified August 2021]
National Interagency Fire Center (n.d.) Wildfires and Acres: Total Wildland Fires and Acres (1983–2020). Available at https://www.nifc.gov/fire-information/statistics/wildfires [verified May 2021]
Oliva P, Schroeder W (2015) Assessment of VIIRS 375 m active fire detection product for direct burned area mapping. Remote Sensing of Environment 160, 144–155.
| Assessment of VIIRS 375 m active fire detection product for direct burned area mapping.Crossref | GoogleScholarGoogle Scholar |
Page WG, Wagenbrenner NS, Butler BW, Blunck DL (2019) An analysis of spotting distances during the 2017 fire season in the Northern Rockies, USA. Canadian Journal of Forest Research 49, 317–325.
| An analysis of spotting distances during the 2017 fire season in the Northern Rockies, USA.Crossref | GoogleScholarGoogle Scholar |
Paton-Walsh C, Emmons LK, Wiedinmyer C (2012) Australia’s Black Saturday fires – comparison of techniques for estimating emissions from vegetation fires. Atmospheric Environment 60, 262–270.
| Australia’s Black Saturday fires – comparison of techniques for estimating emissions from vegetation fires.Crossref | GoogleScholarGoogle Scholar |
Peterson D, Wang J (2013) A sub-pixel-based calculation of Fire Radiative Power from MODIS observations: 2. Sensitivity analysis and potential fire weather application. Remote Sensing of Environment 129, 231–249.
| A sub-pixel-based calculation of Fire Radiative Power from MODIS observations: 2. Sensitivity analysis and potential fire weather application.Crossref | GoogleScholarGoogle Scholar |
Peterson D, Wang J, Ichoku C, Hyer E, Ambrosia V (2013) A sub-pixel-based calculation of Fire Radiative Power from MODIS observations: 1: Algorithm development and initial assessment. Remote Sensing of Environment 129, 262–279.
| A sub-pixel-based calculation of Fire Radiative Power from MODIS observations: 1: Algorithm development and initial assessment.Crossref | GoogleScholarGoogle Scholar |
Peterson DA, Hyer EJ, Campbell JR, Fromm MD, Hair JW, Butler CF, Fenn MA (2015) The 2013 Rim Fire: implications for predicting extreme fire spread, pyroconvection, and smoke emissions. Bulletin of the American Meteorological Society 96, 229–247.
| The 2013 Rim Fire: implications for predicting extreme fire spread, pyroconvection, and smoke emissions.Crossref | GoogleScholarGoogle Scholar |
Radeloff VC, Helmers DP, Kramer HA, Mockrin MH, Alexandre PM, Bar-Massada A, Butsic V, Hawbaker TJ, Martinuzzi S, Syphard AD, Stewart SI (2018) Rapid growth of the US wildland–urban interface raises wildfire risk. Proceedings of the National Academy of Sciences 115, 3314–3319.
| Rapid growth of the US wildland–urban interface raises wildfire risk.Crossref | GoogleScholarGoogle Scholar |
Reid CE, Jerrett M, Petersen ML, Pfister GG, Morefield PE, Tager IB, Raffuse SM, Balmes JR (2015) Spatiotemporal prediction of fine particulate matter during the 2008 Northern California wildfires using machine learning. Environmental Science & Technology 49, 3887–3896.
| Spatiotemporal prediction of fine particulate matter during the 2008 Northern California wildfires using machine learning.Crossref | GoogleScholarGoogle Scholar |
Saide PE, Peterson DA, da Silva A, Anderson B, Ziemba LD, Diskin G, Sachse G, Hair J, Butler C, Fenn M, Jimenez JL, Campuzano-Jost P, Perring AE, Schwarz JP, Markovic MZ, Russell P, Redemann J, Shinozuka Y, Streets DG, Yan F, Dibb J, Yokelson R, Toon OB, Hyer E, Carmichael GR (2015) Revealing important nocturnal and day-to-day variations in fire smoke emissions through a multiplatform inversion. Geophysical Research Letters 42, 3609–3618.
| Revealing important nocturnal and day-to-day variations in fire smoke emissions through a multiplatform inversion.Crossref | GoogleScholarGoogle Scholar |
Schmidt C (2019) Monitoring Fires with the GOES-R Series. In ‘GOES-R Series. A new generation of geostationary environmental satellites’. (Eds S Goodman, T Schmit, J Daniels, R Redmon) pp. 145–163. (Elsevier Inc.)
| Crossref |
Schmit TJ, Gunshor MM (2019) ABI Imagery from the GOES-R Series. In ‘GOES-R Series. A new generation of geostationary environmental satellites’. (Eds S Goodman, T Schmit, J Daniels, R Redmon) pp. 23–34. (Elsevier Inc.)
| Crossref |
Schroeder W, Giglio L (2017) Visible Infrared Imaging Radiometer Suite (VIIRS) 375 m & 750 m Active Fire Detection Data Sets Based on NASA VIIRS Land Science Investigator Processing System {(SIPS)} Reprocessed Data-Version 1 Product User’s Guide Version 1.2. (National Aeronautics and Space Administration (NASA)).
Schroeder W, Oliva P, Giglio L, Csiszar IA (2014) The New VIIRS 375 m active fire detection data product: algorithm description and initial assessment. Remote Sensing of Environment 143, 85–96.
| The New VIIRS 375 m active fire detection data product: algorithm description and initial assessment.Crossref | GoogleScholarGoogle Scholar |
Seiler W, Crutzen PJ (1980) Estimates of gross and net fluxes of carbon between the biosphere and the atmosphere from biomass burning. Climatic Change 2, 207–247.
| Estimates of gross and net fluxes of carbon between the biosphere and the atmosphere from biomass burning.Crossref | GoogleScholarGoogle Scholar |
Soja AJ, Cofer WR, Shugart HH, Sukhinin AI, Stackhouse PW, McRae DJ, Conard SG (2004) Estimating fire emissions and disparities in boreal Siberia (1998–2002). Journal of Geophysical Research: Atmospheres 109, D14S06
| Estimating fire emissions and disparities in boreal Siberia (1998–2002).Crossref | GoogleScholarGoogle Scholar |
Sukhinin AI, French NHF, Kasischke ES, Hewson JH, Soja AJ, Csiszar IA, Hyer EJ, Loboda T, Conrad SG, Romasko VI, Pavlichenko EA, Miskiv SI, Slinkina OA (2004) AVHRR-based mapping of fires in Russia: new products for fire management and carbon cycle studies. Remote Sensing of Environment 93, 546–564.
| AVHRR-based mapping of fires in Russia: new products for fire management and carbon cycle studies.Crossref | GoogleScholarGoogle Scholar |
The Mathworks Inc. (2022) Boundary. Available at https://www.mathworks.com/help/matlab/ref/boundary.html [verified January 2022]
United States Forest Service (n.d.) Fire terminology. Available at https://www.fs.usda.gov/nwacfire/home/terminology.html [verified August 2021]
Walker XJ, Rogers BM, Veraverbeke S, Johnstone JF, Baltzer JL, Barrett K, Bourgeau-Chavez L, Day NJ, de Groot WJ, Dieleman CM, Goetz S, Hoy E, Jenkins LK, Kane ES, Parisien MA, Potter S, Schuur EAG, Turetsky M, Whitman E, Mack MC (2020) Fuel availability not fire weather controls boreal wildfire severity and carbon emissions. Nature Climate Change 10, 1130–1136.
| Fuel availability not fire weather controls boreal wildfire severity and carbon emissions.Crossref | GoogleScholarGoogle Scholar |
Warneke C, Schwarz JP, Dibb J, Kalashnikova O, Frost G, Al-Saad J, Brown SS, Brewer WA, Soja A, Seidel FC, Washenfelder RA, Wiggins EB, Moore RH, Anderson BE, Jordan C, Yacovitch TI, Herndon SC, Liu S, Kuwayama TI, Jaffe D, Johnston N, Selimovic V, Yokelson R, Giles DM, Holben BN, Goloub P, Popovici I, Trainer M, Kumar A, Pierce RB, Fahey D, Roberts J, Gargulinski EM, Peterson DA, Ye X, Thapa LH, Saide PE, Fite CH, Holmes CD, Wang S, Coggon MM, Decker ZCJ, Stockwell CE, Xu L, Gkatzelis G, Aikin K, Lefer B, Kaspari J, Griffin D, Zeng L, Weber R, Hastings M, Chai J, Wolfe GM, Hanisco TF, Liao J, Campuzano Jost P, Guo H, Jimenez JL, Crawford J (2022) Fire Influence on Regional to Global Environments and Air Quality (FIREX-AQ). Journal of Geophysical Research: Atmospheres 128, e2022JD037758
| Fire Influence on Regional to Global Environments and Air Quality (FIREX-AQ).Crossref | GoogleScholarGoogle Scholar |
Wegesser TC, Pinkerton KE, Last JA (2009) California wildfires of 2008: coarse and fine particulate matter toxicity. Environmental Health Perspectives 117, 893–897.
| California wildfires of 2008: coarse and fine particulate matter toxicity.Crossref | GoogleScholarGoogle Scholar |
Westerling AL, Hidalgo HG, Cayan DR, Swetnam TW (2006) Warming and earlier spring increase western US forest wildfire activity. Science 313, 940–943.
| Warming and earlier spring increase western US forest wildfire activity.Crossref | GoogleScholarGoogle Scholar |
Wiedinmyer C, Akagi SK, Yokelson RJ, Emmons LK, Al-Saadi JA, Orlando JJ, Soja AJ (2010) The Fire INventory from NCAR (FINN) – a high-resolution global model to estimate the emissions from open burning. Geoscientific Model Development Discussions 3, 2439–2476.
| The Fire INventory from NCAR (FINN) – a high-resolution global model to estimate the emissions from open burning.Crossref | GoogleScholarGoogle Scholar |
Wiggins EB, Soja AJ, Gargulinski E, Halliday HS, Pierce RB, Schmidt CC, Nowak JB, DiGangi JP, Diskin GS, Katich JM, Perring AE, Schwarz JP, Anderson BE, Chen G, Crosbie EC, Jordan C, Robinson CE, Sanchez KJ, Shingler TJ, Shook M, Thornhill KL, Winstead EL, Ziemba LD, Moore RH (2020) High temporal resolution satellite observations of Fire Radiative Power reveal link between fire behavior and aerosol and gas emissions. Geophysical Research Letters 47, e2020GL090707
| High temporal resolution satellite observations of Fire Radiative Power reveal link between fire behavior and aerosol and gas emissions.Crossref | GoogleScholarGoogle Scholar |
Willmott CJ, Matsuura K (2005) Advantages of the mean absolute error (MAE) over the root mean square error (RMSE) in assessing average model performance. Climate Research 30, 79–82.
| Advantages of the mean absolute error (MAE) over the root mean square error (RMSE) in assessing average model performance.Crossref | GoogleScholarGoogle Scholar |
Wolfe RE, Lin G, Nishihama M, Tewari KP, Tilton JC, Isaacman AR (2013) Suomi NPP VIIRS prelaunch and on-orbit geometric calibration and characterization. Journal of Geophysical Research: Atmospheres 118, 11,508–11,521.
| Suomi NPP VIIRS prelaunch and on-orbit geometric calibration and characterization.Crossref | GoogleScholarGoogle Scholar |
Ye X, Arab P, Ahmadov R, James E, Grell GA, Pierce B, Kumar A, Makar P, Chen J, Davignon D, Carmichael GR, Ferrada G, McQueen J, Huang J, Kumar R, Emmons L, Herron-Thorpe FL, Parrington M, Engelen R, Peuch VH, da Silva A, Soja A, Gargulinski E, Wiggins E, Hair JW, Fenn M, Shingler T, Kondragunta S, Lyapustin A, Wang Y, Holben B, Giles DM, Saide PE (2021) Evaluation and intercomparison of wildfire smoke forecasts from multiple modeling systems for the 2019 Williams Flats fire. Atmospheric Chemistry and Physics 21, 14427–14469.
| Evaluation and intercomparison of wildfire smoke forecasts from multiple modeling systems for the 2019 Williams Flats fire.Crossref | GoogleScholarGoogle Scholar |
§ Melinda T. Berman has since moved to the Department of Atmospheric Sciences at the University of Illinois at Urbana-Champaign, USA.