Fire severity and plant productivity recovery in a mixed grass prairie wildfire driven by extreme winds
Sera A Grover A , Roy Vera-Velez A , Xulin Guo B and Eric G. Lamb
A
B
Abstract
Wildfire on rangelands in the mixed grassland can severely disrupt livestock operations. Understanding how fire severity impacts post-fire production recovery is important for grazing management.
We examined how topography and other environmental factors influence wildfire severity, or the consumption of biomass and exposure of soil, under extreme (>120 km h−1) wind conditions in native mixed grass prairie in western Canada. We also examined how variation in fire severity impacts grassland production recovery.
Fire severity and production recovery were measured using the bare soil index (BSI) and normalised difference vegetation index (NDVI). Impacts of topography, wind exposure, and site capability on fire severity and production recovery were assessed using generalised additive models.
Fire severity varied as a function of slope, wind exposure and fuel load. Severity peaked at NDVI between 0 and 0.4, values associated with high litter content and minimal green vegetation. Interactions between slope and aspect with respect to dominant wind direction generated very high fire severity on slopes greater than 15° that faced into the wind. Production recovery increased moderately with higher fire severity and recovery was generally higher on sites with lower potential productivity.
Post-fire production recovery was rapid; fire severity and site capability had only modest impacts on recovery rates demonstrating the resilience of grassland ecosystems to even severe wildfire.
Keywords: Alberta, bare soil index, Canada, fire severity, grassland wildfire, NDVI, rangeland productivity recovery, Saskatchewan.
Introduction
Fire is a common disturbance in the mixed grasslands of North America. Historically, fires were naturally occurring due to weather or purposeful ignitions by Indigenous peoples (Pyne 1983; Higgins 1986; Pyne 1997; Anderson 2006; Whealan 2009; Scasta et al. 2016). Following colonisation, fire was largely removed from Canadian grasslands with negative consequences including excessive amounts of litter in under-grazed areas, expansion of woody vegetation, and a surge in noxious and invasive species (Gonzalez et al. 2015; Letts et al. 2015; Ohrtman et al. 2015; Martin and Hamman 2016; Gross 2023). Despite aggressive fire suppression, periodic wildfires occur on lands managed for livestock production in the mixed grassland. Wildfire can severely disrupt livestock operations, yet grassland wildfire remains understudied relative to prescribed fire. Understanding how topography and other environmental factors influence wildfire severity, as measured by biomass consumption and soil exposure, and burn severity, or the long-term effects of fire including post-fire biomass production recovery (Key and Benson 2006), is important for post-fire grazing management.
Livestock operations typically defer grazing post-wildfire (Bureau of Land Management 2007). While post-fire vegetation has increased nutritional value, forage volumes can be substantially reduced (Bischoff et al. 2022; Vera-Velez et al. 2023). Recent studies of grazing after wildfire in native mixed grasslands recommend a 1 to 2-year period of rest before grazing can return (Gates et al. 2017; Arterburn et al. 2018). In Alberta, Canada, grazing is recommended to be postponed for 1 year, while in the United States it is prohibited to graze for 2 years following fire (Bureau of Land Management 2007; Government of Alberta 2018). Post-fire deferment is economically challenging for livestock producers, particularly if a wildfire has affected the majority of lands used by a producer. Developing tools to measure and understand grassland recovery patterns is critical to providing ecologically realistic deferment recommendations to livestock producers.
Vegetation recovery is influenced by the intensity and the severity of the wildfire. Fire intensity is characterised by flame length, residency time, and amount of energy released (Lentile et al. 2006; Keeley 2009). Severity has two components, fire severity, which captures the immediate effects of fire, and burn severity which captures the long-term effects of fire (Key and Benson 2006). Fire severity is measured by the removal of standing biomass and exposure of bare soil, and is generally a function of wind speed and direction, topography, and fuel availability (Lentile et al. 2006; French et al. 2008; Keeley 2009). Burn severity is determined by factors including plant mortality, germination and regrowth, post-burn precipitation, and temperature (solar heat load) (Neary et al. 1999; Morgan et al. 2001; Key and Benson 2006; Lentile et al. 2006; French et al. 2008; García-Llamas et al. 2019).
Here we use remote sensing to capture this variability in fire severity and examine how burn severity impacts grassland recovery. Remote sensing is an increasingly useful tool to study grasslands over a broader spatial and temporal scale than is possible with ground-based studies (Shuman and Ambrose 2003; Xu and Guo 2015). Remote sensing has been used for many years to assess forest fires (Trigg and Flasse 2001; Schepers et al. 2014). Applying the same approach to grassland fires would allow a better understanding of the effects of fire and recovery across a whole landscape. Our objectives were therefore to use remote sensing to study two large grassland wildfires to (1) determine how grassland fire severity is affected by environmental gradients such as wind, topography, and available vegetation across a large spatial scale, and (2) to determine how variation in fire severity affects grassland production recovery.
Methods
Study area
The study area is located near the provincial border of Saskatchewan and Alberta in the Mixed Prairie of the Northern Great Plains (Coupland 1961). On 17 October 2017, two large fires ignited in southeast Alberta near Acadia Valley (Empress Fire; 51.114 N, −110.121 W) and Hilda (Hilda Fire; 50.535 N, −109.992 W). Both fires crossed the provincial border into southwest Saskatchewan, with approximately 28,000 ha of land burned, 8000 of which was pastureland. High wind speeds and a moderate drought contributed to extreme fire behaviour (Briere 2017). Peak winds on the day of ignition exceeded 120 km h−1 (Glen and Briere 2017); the dominant wind direction up to approximately 5 pm was 224° followed by a shift to approximately 277° (Environment and Climate Change Canada; https://climate.weather.gc.ca/climate_data/hourly_data_e.html) causing a distinct ‘finger’ pattern to the fire footprint.
The Alberta sites are classified as Dry Mixedgrass Natural Subregion, while Saskatchewan sites are classified as Dry Mixed Grass Ecoregion (Coupland 1961; Thorpe 2014). In the Dry Mixed Grass Ecoregion of Saskatchewan, mean annual precipitation and temperature are 250–350 mm and 3.5°C, respectively (Acton et al. 1998). In the Dry Mixedgrass Natural Subregion of Alberta, mean annual precipitation and temperature is 333 mm and 4.2°C, respectively (Natural Regions Committee 2006). Vegetation at the study sites is characterised by xeric graminoid species, such as Bouteloua gracilis, Pascopyrum smithii, Koeleria macrantha, and Hesperostipa comata, forbs such as Selaginella densa, Artemisia ludoviciana, and Phlox hoodii, and shrubs such as Rosa arkansana (Natural Regions Committee 2006; Thorpe 2014). The topography of the study area is composed of knolls, gentle and steep slopes, as well as bordering a steep river valley of the South Saskatchewan River. The elevation of the study area ranges from 590 m to 880 m above sea level.
Satellite images and image processing
Satellite imagery was retrieved from the United States Geological Survey Earth Explorer Database for Sentinel-2 Level 1C. Sentinel-2 Level 1C was chosen for its high spatial resolution and for already being atmospherically and radiometrically corrected. Criteria for image selection included: less than 30% cloud cover with burn scars not covered by cloud, both fires needed to be within one image, image capture date needed to occur as close as possible to peak biomass production (mid-July) and as close as possible to before and after the fires. Five images were chosen for use (Table 1). Images include three pre-fire images. Mid-July is the typical peak in growth in this ecosystem, therefore the 15 July 2017 image captures peak green biomass pre-fire. Senescence is normally well advanced by late September in this ecosystem, therefore 28 September 2017 image captures cured biomass. The immediate post-fire image was collected approximately 11 days after ignition when all active fire had been suppressed.
Image name | Acronym | Date of image acquisition | Cloud cover (%) | Days before (−) or after (+) fire | |
---|---|---|---|---|---|
Spring 2017 | S17 | 11 May 2017 | 0.15 | −159 | |
Pre-Fire 2017 | PrF17 | 15 July 2017 | 22.04 | −98 | |
Fall 2017 | F17 | 28 September 2017 | 3.52 | −25 | |
Immediate Post-Fire | F | 23 October 2017 | 0.02 | +6 | |
Post-Fire 2018 | PF18 | 15 June 2018 | 1.76 | +272 | |
Post-Fire 2020 | PF20 | 19 June 2020 | 27.25 | +976 |
Upon image selection, bands 2–4, 8, 10, and 11 for each image were transferred into one file in Geomatica (PCI Geomatics 2018). The immediate post-fire (F) image was assessed to determine the bands with the highest visual contrast between burned and unburned areas (RGB: SWIR2, SWIR1, and Red) (Fig. 1). Visual interpretation of these bands was used to draw two irregular polygons outlining the Hilda and Empress fires (Fig. 1). These irregular polygons were combined and used for clipping all five Sentinel-2 images and ancillary data down to manageable file sizes.
False-colour (RGB: SWIR2, SWIR1, and Red) composite of both the Hilda and Empress wildfires on 23 October 2017. The original image was cut into an irregular polygon to remove any unnecessary image. The two pink irregular polygons were then used later in the study when the burn scars were undetectable. The northern burn scar (yellow) is the Empress fire, while the southern burn scar is the Hilda fire. Fires originated near the westernmost point of each burn scar.
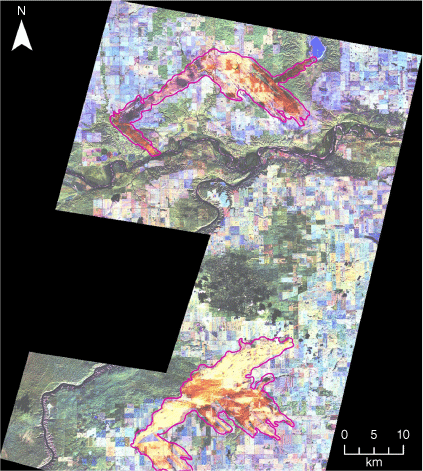
After each image was cut down to a manageable size the SWIR 1 and SWIR 2 bands were resampled to 10 m by 10 m from their original 20 m by 20 m resolution. This was done to ensure that when spectral indices were calculated, the resolution of all pixels matched. Two spectral indices - the normalised differenced vegetation index (NDVI) and the bare soil index (BSI) - were calculated. NDVI measures the greenness of vegetation through the absorption of red light by the vegetation chlorophyll content while simultaneously reflecting near infrared light off of turgid cell walls (Tucker 1979; Smith et al. 2015). BSI measures the amount of bare ground through the absorption of infrared and red light, and the reflectance of near infrared and blue light (Rikimaru et al. 2002; Diek et al. 2017; Nguyen et al. 2021).
Environmental data
Four types of ancillary environmental data were used. Soil Landscapes of Canada (SLC) version 3.2 polygons of a scale of 1:1,000,000 contained information such as soil name, soil texture components, and soil chemical composition. The Canadian Land Inventory (CLI) maps at a scale of 1:1,000,000 describe the agricultural capability of the landscape based on soil classification, texture, presence of stones, and topography. The Digital Elevation Map (DEM) of Canada at a scale of 1:50,000 described topography. A calculation of solar heat load was made to estimate the amount of heat a slope receives relative to aspect (McCune and Keon 2002). Aspect with respect to the wind (whether a location was on a slope facing into the fire, flanking a fire, or on a reverse slope away from the fire) was calculated based on dominant wind direction. Fire movement prior to 5 pm of the day of fire was assumed to be driven by winds from 224° while the fingers extending to the southwest were assumed to driven by winds from 277°.
Both the SLC polygons and the CLI maps were georeferenced and projected to World Geodetic System (WGS) 1984 (QGIS Development Team 2015). To ensure the CLI maps and the SLC polygons were correctly georeferenced, a preloaded QGIS map of Canada was used to ensure the border and corners of the maps and polygons aligned correctly with Alberta and Saskatchewan. The SLC polygons were clipped to the study area. Upon georeferencing, the CLI maps had individual polygons drawn to reflect the different land use areas (QGIS Development Team 2015). Lastly, the Canada wide DEM was projected to WGS 1984 and clipped to the study area prior to computing aspect and slope.
Both fires covered just over 1 million pixels. To ensure the most efficient exportation of all data, the irregular polygon that outlined each fire was split along the provincial border. The four polygons were auto-populated with points (a maximum of 1 million points 10 m apart) to ensure every part of the polygon was sampled and that each pixel was only sampled once. Following auto population, the points were linked to the three layers of ancillary data using the join function. Lastly, both NDVI and BSI for all five Sentinel-2 images were linked to the points using Extract Multi-Values to Points. Once linking was complete the four attribute tables were exported.
Following data exportation, the fire severity index (FSI) and the recovery index (RI) were calculated. The FSI measured the change in Bare Soil Index (BSI) between fall 2017 (F17) and immediate post-fire (F) images. Before FSI calculation, negative BSI values for both F17 and F images were truncated to zero to ensure that only the effects of the fires were captured. Following the creation of FSI, it was found that some negative values FSI were produced. It was assumed that these negative values were caused by random error due to the large data set, thus a second truncation was performed to change negative FSI values to zero to ensure misinterpretation of FSI was avoided. RI was produced by assessing the overall change in NDVI between post-burn (PF) and pre-burn (PrF17) values. The log response ratio of NDVI (log(PFx + 1)/(PrF17 + 1)), was taken to ensure only the recovery (increased greenness) of the specific PF year being evaluated could be determined. Positive values created from the log response ratio indicate greater biomass than pre-fire conditions. Negative values form the log response ratio indicate a production deficit compared to pre-fire conditions.
Statistical analysis
The role of environment in determining fire severity index (FSI) were assessed using models with slope, aspect with respect to wind, PrB17 NDVI, and F17 NDVI as predictors. The role of environment and fire severity in determining grassland recovery index (RI) were assessed using models with soil order, land capability, solar heat load, and fire severity as predictors. Prior to analysis, correlations between FSI and RI and the environmental predictors were examined using R (Simko 2021; R Development Core Team 2022). Any variables including predictor variables with correlations greater than |0.6| were selected for subsequent analysis. Environmental variables that could not logically affect fire behaviour were excluded from analysis.
Generalised Additive Models (GAM) were used to examine how fire severity and recovery varied with respect to environmental gradients (Wood 2011; R Development Core Team 2022). GAM models were used because they permit complex non-linear relationships between predictor and response variables to be assessed. To test how slope aspect with respect to wind direction (categorical variable with three levels) impacted fire severity, a series of four GAM models were fit. These models included (1) the slope alone model, (2) aspect as a categorical fixed effect, (3) aspect as a categorical fixed effect and the smoothed slope term as separate effects, and (4) the continuous smoothed term with the categorical variables broken down into by = terms (effectively fitting a separate smoothed line for each aspect) (Table 2). Recovery was modelled using a similar approach with separate GAM models using Fire Severity and Heat Load index as continuous predictors, and models combining the continuous and categorical predictors with Soil Order and Land Class as categorical predictors (Table 3). In all cases, function gam from package mgcv was used to fit gam models (Wood 2006). Smoothed relationships with continuous predictor variables were fit using thin-plate regression splines; by = arguments were used when separate smoothed relationships were desired for each level of a categorical variable.
Model name | Response variable | Explanatory variable(s) | Model code | AIC | R2 | |
---|---|---|---|---|---|---|
Slope alone | Fire severity | Slope (continuous; 0–23°) | Fire severity ~s(Slope) | −2,651,446 | 0.003 | |
July 2017 NDVI | Fire severity | July 2017 NDVI (continuous; 0–1) | Fire severity ~s(July NDVI) | −2,738,044 | 0.082 | |
Fall 2017 NDVI | Fire severity | September 2017 NDVI (continuous; 0–1) | Fire severity ~s(September NDVI) | −2,768,809 | 0.109 | |
Aspect 2 | Fire severity | Aspect with respect to wind (categorical with three terms, facing upwind into fire, facing downwind away from fire, and oblique) and slope (continuous variable; 0–23°) | Fire severity ~(Aspect) + s(Slope) | −2,658,095 | 0.010 | |
Aspect 3 | Fire severity | As for model Aspect 2, but with separate curve shapes for each Aspect category | Fire Severity ~ Aspect + s(Slope), by = Face + s(Slope), by = Away + s(Slope), by = Oblique | −2,660,419 | 0.012 | |
Aspect 4 | Fire severity | Aspect with respect to wind (categorical with three levels) | Fire Severity ~(Aspect) | −2,652,375 | 0.004 | |
Fuel – Aspect 1 | Fire severity | September 2017 NDVI (continuous; 0–1) and aspect with respect to wind as for model Aspect 3 | Fire Severity ~ Aspect + s(September NDVI) + s(Slope), by = Face + s(Slope), by = Away + s(Slope), by = Oblique | −2,746,082 | 0.089 | |
Fuel – Aspect 2 | Fire severity | September 2017 NDVI (continuous; 0–1) and aspect with respect to wind with separate interactions between slope and NDVI for each aspect category | Fire Severity ~ Aspect + s(Slope + September NDVI), by = Face + s(Slope + September NDVI), by = Away + s(Slope + September NDVI), by = Oblique | −2,747,960 | 0.091 |
Model terms fitting nonlinear continuous relationships are indicated by s(variable). Categorical variables are indicated by just the variable name. by = terms indicate that a separate nonlinear relationship is being fit for each level of the categorical variable. Akaike’s Information Criterion (AIC) is provided for each model. Bolded values represent the model selected that best predicts fire severity.
Model name | Response variable | Explanatory variable(s) | Example code | AIC | R2 | |
---|---|---|---|---|---|---|
Fire severity | Recovery year 1 | Fire severity (continuous; ranging from 0 to 0.30) | Recovery ~s(fire severity) | −1,622,372 | 0.042 | |
Recovery years 3 | −1,341,850 | 0.057 | ||||
Heat load index | Recovery year 1 | Heat load index (continuous; 0–1) | Recovery ~s(heat load INDEX) | −1,582,838 | 0.005 | |
Recovery years 3 | −1,287,182 | 0.007 | ||||
Soil order 2 | Recovery year 1 | Soil order (categorical) & Fire severity (continuous; 0–0.30) | Recovery ~(soil order) + s(fire severity) | −1,642,749 | 0.060 | |
Recovery years 3 | −1,353,254 | 0.068 | ||||
Soil order 3 | Recovery year 1 | Soil order (categorical; CH, GL, RG, and VE) & fire severity (continuous; 0–0.30) | Recovery ~ soil order + s(fire severity), by = CH + s(fire severity), by = GL + s(fire severity), by = RG + s(fire severity), by = VE | −1,671,599 | 0.085 | |
Recovery years 3 | ||||||
−1,371,517 | 0.084 | |||||
Soil order 4 | Recovery year 1 | Soil order (categorical) | Recovery ~(Soil order) | −1,597,988 | 0.019 | |
Recovery years 3 | −1,293,235 | 0.017 | ||||
Land class 2 | Recovery year 1 | Land class (categorical) & Fire severity (continuous; 0–0.30) | Recovery ~(land class) + s(fire severity) | −1,630,806 | 0.049 | |
Recovery years 3 | ||||||
−1,370,909 | 0.083 | |||||
Land class 3 | Recovery year 1 | Land Class (categorical; Class 1, Class 2, Class 3, and Class 4) & Fire Severity (continuous; 0–0.30) | Recovery ~ Land Class + s(fire severity), by = Class 1 + s(fire severity), by = Class 2 + s(fire severity), by = Class 3 + s(fire severity), by = Class 4 | −1,684,058 | 0.096 | |
Recovery years 3 | −1,393,386 | 0.102 | ||||
Land class 4 | Recovery year 1 | Land Class (categorical) | Recovery ~(land class) | −1,601,057 | 0.022 | |
Recovery years 3 | ||||||
−1,316,382 | 0.034 |
Akaike’s Information Criterion (AIC) for each model, to aid in best fit model selection. Upper value in AIC and R2 columns relates to year one of recovery models, and lower value relates to year three of recovery. Bolded values represent the model selected that best predicts recovery for both the continuous variable and the multiple categorical variable models.
Results
Fire severity
Fire severity, a measure of how much bare ground was exposed, was best predicted by pre-fire (September 2017) NDVI. Fire severity increased rapidly with pre-fire (F17 and PrB17) NDVI (Fig. 2a; Table 2). Peak fire severity occurred between 0 and 0.2 NDVI with a gradual decline to an NDVI of ~0.6 where a secondary peak of fire severity occurred. Similar patterns were observed with July 2017 (PrB17) NDVI, where a rapid increase in fire severity to an NDVI of ~0.35 was followed by a plateau in fire severity at higher NDVI.
Relationships between fire severity and (a) NDVI, (b) hill slope, and hill slope divided by aspect with respect to the wind. Fitted lines from GAM models are shown with shaded areas around the lines indicating the standard error of the fitted values.
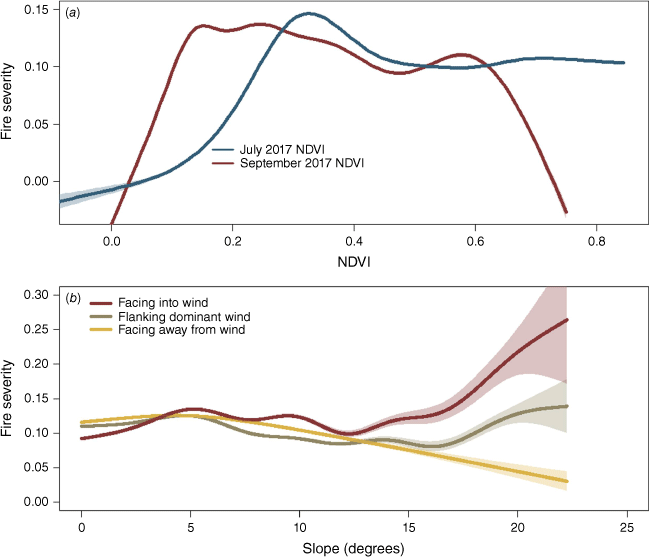
Aspect had smaller effects on fire severity than NDVI. The relationships between slope and wind direction (aspect) created complex effects on fire severity (Fig. 2b; Table 2). Aspect with respect to wind was divided into three categories: facing upwind into fire ±45° of the dominant wind direction, ±45° downwind away from fire, and flanks. Aspect with respect to wind direction had limited impact on fire severity on slopes less than 10°. On slopes greater than 10° higher fire severity occurred on slopes facing into the wind, and lower severity on slopes facing away from the wind. There was little impact of slope on severity on the flanks.
Recovery
Recovery following fire, a measure of how much green regrowth occurred relative to the amount of pre-fire green biomass, was rapid; Year 1 NDVI was similar to pre-fire greenness (Fig. 3a; Table 3). Recovery was much lower in Year 3. Relationships between recovery and fire severity were complex with greater recovery detected above a fire severity of 0.2. Recovery was similar across heat load values indicating similar recovery rates on both hot south facing slopes and more mesic locations (Fig. 3b; Table 3). More fertile sites (Chernozemic and Vertisolic soils, and Land Class 3) had generally lower recovery than less fertile sites; this trend was most pronounced on sites with lower fire severity and in year 3 (Fig. 4; Table 3).
Relationships between (a) fire severity and (b) solar heat load for year one (blue line) and year three (red line) post-fire. Recovery is the log response relationship between ratio of NDVI for the year of recovery divided by pre-burn values; values greater than zero exceed pre-fire greenness and values less than zero have less greenness than pre-fire. Solar heat was calculated using McCune and Keon (2002) method which uses slope and aspect to derive sun exposure. Shaded areas indicate the standard error around the fitted values.
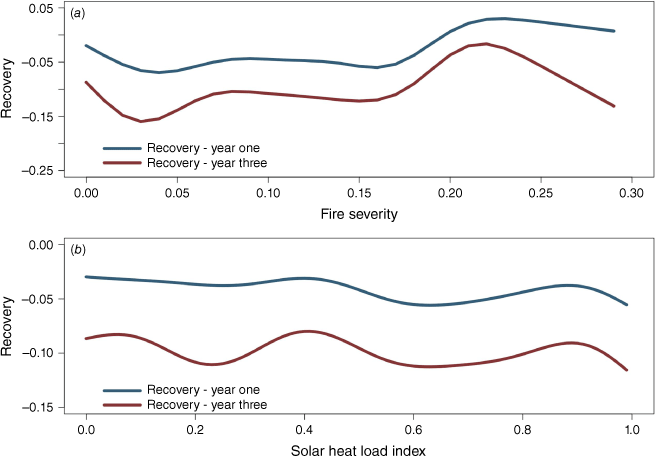
Relationships between fire severity and recovery for year one and year three post-fire as a function of site soil order (a, b) and agricultural land class (c, d). Recovery is the log response ratio of NDVI for in the year of recovery divided by pre-fire values greater than zero exceed pre-fire greenness, values less than zero have less greenness than pre-fire. Soil order and land class are proxies for site potential productivity.
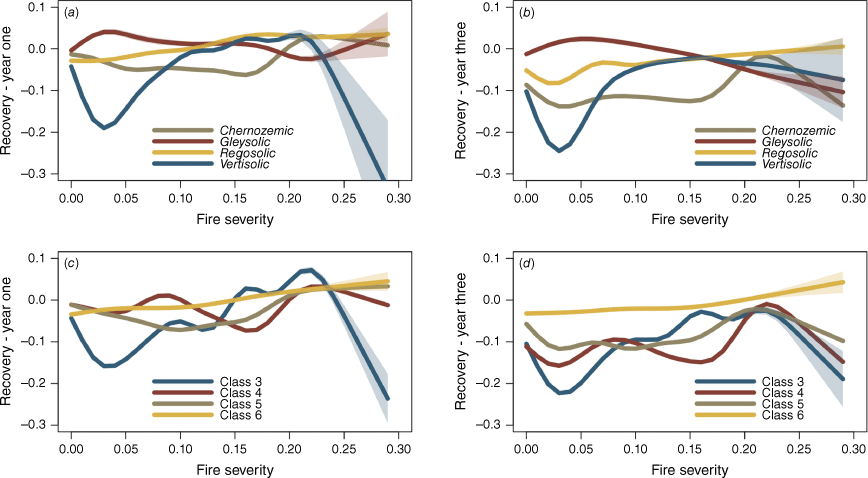
Discussion
Fire severity
This study is one of the first to examine the landscape factors that drive grassland fire severity under extreme (>120 km h−1) wind conditions. Fire severity, or the amount of bare soil exposed by the fire, varied as a function of topographic and environmental conditions including pre-fire NDVI, slope, and aspect with respect to wind with NDVI as the dominant factor. We interpret the peak in fire severity at an NDVI of 0–0.2 and 0.2–0.4, to be driven by fuel loading as these NDVI values in grasslands are associated with areas of high litter content and minimal green vegetation, respectively (Xu et al. 2014). In September, senescence is normally well advanced in these grasslands, thus September NDVI should provide a reliable measure of available cured fuels. A peak in fire severity in areas of high fuel is consistent with other studies (Cruz et al. 2017; Kraaij et al. 2018; Wragg et al. 2018; García-Llamas et al. 2019).
We observed important interactions between slope and slope aspect with respect to wind direction that generated very high fire severity on slopes greater than 15° that faced into the wind (±45° of the dominant wind direction). Slope was unimportant, however, on sites oblique to the wind. We suspect that slopes facing into the wind had high severity due to both the extreme wind directly feeding oxygen to the flames causing a hotter fire (Thomas et al. 2017; Evers et al. 2022), and flames being forced parallel to the ground. When uphill slopes are greater than 24° the tip of the flame to the base of the flame lay parallel to the ground (McArthur 1967). There are no studies assessing the fire severity generated by parallel flames, though it can be hypothesised parallel flames would have a higher fire severity due to longer residence time as the entire flame length (tip to base) would expose a single spot to heat for longer. Parallel flames are typically associated with slopes greater than 24°, but when compounded with the extreme winds, parallel flames could occur at slopes as low as 15°. Many slopes greater than 15° are found in the study area.
The interactions between aspect with respect to wind and slope driving fire severity are novel. Studies that assess aspect and slope are commonly done theoretically or within controlled environments, such as prescribed fire, where fire intensity (energy released) is typically quantified rather than fire severity (McArthur 1967; Weise and Biging 1996; Nelson 2002; Thomas et al. 2017; Hall 2019). Fire severity is used in wildfire studies where direct measures of intensity are challenging to take (Evers et al. 2022). Both measures are typically assessed as independent factors, but when aspect with respect to wind and slope are considered simultaneously, wind either overrides the effects of slope, or is additive to the effects of slope (Weise and Biging 1996; Nelson 2002; Hall 2019; Evers et al. 2022). The importance of aspect with respect to wind in our study demonstrates the importance of studying fire behaviour under both moderate and extreme conditions.
Recovery
Recovery varied as a function of fire severity and of environmental conditions including potential site productivity (soil order and land classification) and heat load. The heat load index showed the highest recovery on the coolest and warmest aspects. Overall recovery was lower in year three than in year one.
The fire severity–recovery relationship was similar for both soil orders and land classes. The four dominant soil orders and land use classifications in the study area vary in potential productivity (Regosols < Chernozems < Vertisols < Gleysols; Class 3 < Class 4 < Class 5 < Class 6) (Bedard-Haughn 2010; VandenBygaart 2010; Brierley et al. 2011; Pennock et al. 2011). Faster recovery might be expected on more fertile sites where soil moisture is less limiting and greater growth rates are possible, but such a pattern was not observed here. The lack of a site potential effect on recovery rate may be because each site type has its own characteristic native plant communities. These communities differ in species composition with more xeric tolerant species on less productive sites; all of these species however have extensive belowground structures that are well adapted to resprouting post-fire, particularly a dormant season fire (Coupland and Johnson 1965; Comfort and Lamb 2023).
Year one of recovery was similar to pre-fire greenness, congruent with the findings of Steiner et al. (2020). Both fires occurred in the late fall, which can cause lower productivity (recovery) the year following when compared to spring fires (Jardine et al. 2017). Recovery could also have been underestimated in year one because the bright green regrowth contrasted with dark soil. NDVI measures the total greenness of vegetation but can be disrupted by large amounts of bare ground, which can create a lower NDVI value (Smith et al. 2015; Steiner et al. 2020). Finally, lower precipitation occurred in June 2018 compared to June 2017. The lower average precipitation for June could have been an additional contributing factor to the lower-than-expected recovery rates for year one. Normal temperature and precipitation trends were seen in 2019, but in 2020 a season-long severe drought drastically reduced productivity (Grover 2024). Biomass production in grassland ecosystems is closely tied to precipitation (Sala et al. 1988; Huxman et al. 2004; Knapp et al. 2015; Kreyling et al. 2017), and while average productivity declined due to drought, the relationships between recovery and fire severity and site heat load did not change substantially. This lack of interactions demonstrates that variation in fire severity does not change the vulnerability of these grasslands to drought. This is a surprising finding given that litter is very important for soil moisture retention (Deutsch et al. 2010; Hilger and Lamb 2017), and increased fire severity implies reduced biomass carryover. In this case, the lack of interaction can likely be explained by the nearly complete consumption of litter by the fire observed at all sites surveyed on the ground (Grover 2024).
Recovery of vegetation was highest in both areas with a moderate heat load and areas with a high heat load. Recovery peaking under a high heat load could be attributed to a community composition of xeric species that have robust belowground perennial organs and can tolerate drought conditions (Natural Regions Committee 2006; Thorpe 2014). Recovery peaking under a moderate heat load could be attributed to the increased soil moisture content in those areas.
Post-fire grazing management decisions can be challenging for agricultural producers. Regulations limiting grazing post-fire (Bureau of Land Management 2007; Government of Alberta 2018) perpetuate the idea that fire within native grasslands ecosystems are not a historically naturally occurring process; yet grasslands evolved under the co-disturbance of fire and grazing (Anderson 2006). Given the landscape scale variation in severity and recovery demonstrated here, one-size-fits-all grazing prescriptions are not appropriate. Grazing animals are attracted to the higher nutritional content of recovering burned vegetation (Fuhlendorf et al. 2009; Vera-Velez et al. 2023), but areas with limited vegetation recovery may be damaged. Understanding the relationships between fire severity slope, and aspect can enable agricultural producers to manage their lands using techniques such as cross fencing or virtual fencing to reduce grazing pressure on areas with higher fire severity while taking advantage of nutritious recovering vegetation in areas of lower severity.
Data availability
A complete copy of the data used in this paper will be archived and available electronically in the University of Saskatchewan thesis repository (harvest.usask.ca) when first author Grover’s MSc thesis is finalised.
Declaration of funding
Funding for this research came from the Saskatchewan Cattlemen’s Association, Saskatchewan Agriculture Development Fund, and Environment and Climate Change Canada. Funding agencies had no role in the preparation of the data or manuscript.
Acknowledgements
Funding for this research came from the Saskatchewan Cattlemen’s Association, Saskatchewan Agriculture Development Fund, and Environment and Climate Change Canada. We thank Irini Soubry for advice on image processing and analysis.
References
Anderson RC (2006) Evolution and origin of the Central Grassland of North America: climate, fire, and mammalian grazers. The Journal of the Torrey Botanical Society 133, 626-647.
| Crossref | Google Scholar |
Arterburn JR, Twidwell D, Schacht WH, Wonkka CL, Wedin DA (2018) Resilience of sandhills grassland to wildfire during drought. Rangeland Ecology & Management 71, 53-57.
| Crossref | Google Scholar |
Bedard-Haughn A (2010) Gleysolic soils of Canada: genesis, distribution, and classification. Canadian Journal of Soil Science 91, 763-779.
| Crossref | Google Scholar |
Bischoff BK, Bork EW, Carlyle CN (2022) Forage productivity is resilient to timing of post-wildfire defoliation in mixed-grass Prairie. Canadian Journal of Plant Science 102, 926-930.
| Crossref | Google Scholar |
Briere K (2017) Wildfire damage “catastrophic”. The Western Producer, 2017/10/26. Available at https://www.producer.com/2017/10/wildfire-damage-catastrophic/ [accessed 26 October 2017]
Brierley JA, Stonehouse HB, Mermut AR (2011) Vertisolic soils of Canada: genesis, distribution, and classification. Canadian Journal of Soil Science 91, 903-916.
| Crossref | Google Scholar |
Comfort M, Lamb EG (2023) Silver Sagebrush (Artemisia cana) survival and regrowth after fire in the mixed grass prairie. Western North American Naturalist 83, 3.
| Crossref | Google Scholar |
Coupland RT (1961) A Reconsideration of grassland classification in the Northern Great Plains of North America. Journal of Ecology 49, 135-167.
| Crossref | Google Scholar |
Coupland RT, Johnson RE (1965) Rooting characteristics of grassland species in Saskatchewan. Journal of Ecology 53, 475-507.
| Crossref | Google Scholar |
Deutsch E, Bork E, Willms WD (2010) Separation of grassland litter and ecosite influences on seasonal soil moisture and plant growth dynamics. Plant Ecology 209, 135-145.
| Crossref | Google Scholar |
Diek S, Fornallaz F, Schaepman ME, Rogier De J (2017) Barest pixel composite for agricultural areas using landsat time series. Remote Sensing 9, 1245.
| Crossref | Google Scholar |
Evers C, Holz A, Busby S, Nielsen-Pincus M (2022) Extreme winds alter influence of fuels and topography on megafire burn severity in seasonal temperate rainforests under record fuel aridity. Fire 5, 41.
| Crossref | Google Scholar |
French NHF, Kasischke ES, Hall RJ, Murphy KA, Verbyla DL, Hoy EE, Allen JL (2008) Using Landsat data to assess fire and burn severity in the North American boreal forest region: an overview and summary of results. International Journal of Wildland Fire 17, 443.
| Crossref | Google Scholar |
Fuhlendorf SD, Engle DM, Kerby JAY, Hamilton R (2009) Pyric herbivory: rewilding landscapes through the recoupling of fire and grazing. Conservation Biology 23, 588-598.
| Crossref | Google Scholar | PubMed |
García-Llamas P, Suárez-Seoane S, Taboada A, Fernández-García V, Fernández-Guisuraga JM, Fernández-Manso A, Quintano C, Marcos E, Calvo L (2019) Assessment of the influence of biophysical properties related to fuel conditions on fire severity using remote sensing techniques: a case study on a large fire in NW Spain. International Journal of Wildland Fire 28, 512.
| Crossref | Google Scholar |
Gates EA, Vermeire LT, Marlow CB, Waterman RC (2017) Fire and season of postfire defoliation effects on biomass, composition, and cover in mixed-grass prairie. Rangeland Ecology & Management 70, 430-436.
| Crossref | Google Scholar |
Glen B, Briere K (2017) Windstorm, grass fires wreak havoc in Saskatchewan, Alberta. The Western Producer, 18 October 2017. Available at https://www.producer.com/farmliving/windstorm-grassfire-wreak-havoc-in-saskatchewan/ [accessed 18 October 2017]
Gonzalez SL, Ghermandi L, Peláez DV (2015) Growth and reproductive post-fire responses of two shrubs in semiarid Patagonian grasslands. International Journal of Wildland Fire 24, 809.
| Crossref | Google Scholar |
Hilger H, Lamb EG (2017) Quantifying optimal rates of litter retention to maximize annual net primary productivity on Mixed-Grass prairie. Rangeland Ecology & Management 70, 219-224.
| Crossref | Google Scholar |
Huxman TE, Smith MD, Fay PA, Knapp AK, Shaw MR, Loik ME, Smith SD, Tissue DT, Zak JC, Weltzin JF, Pockman WT, Sala OE, Haddad BM, Harte J, Koch GW, Schwinning S, Small EE, Williams DG (2004) Convergence across biomes to a common rain-use efficiency. Nature 429, 651-654.
| Crossref | Google Scholar | PubMed |
Jardine LE, Ryburn AK, Stancampiano AJ (2017) Effects of fire severity on habitat recovery in a mixed grass prairie ecosystem. Oklahoma Native Plant Record 16, 64-78.
| Crossref | Google Scholar |
Keeley JE (2009) Fire intensity, fire severity and burn severity: a brief review and suggested usage. International Journal of Wildland Fire 18, 116.
| Crossref | Google Scholar |
Knapp AK, Carroll CJW, Denton EM, La Pierre KJ, Collins SL, Smith MD (2015) Differential sensitivity to regional-scale drought in six central US grasslands. Oecologia 177, 949-957.
| Crossref | Google Scholar | PubMed |
Kraaij T, Baard JA, Arndt J, Vhengani L, van Wilgen BW (2018) An assessment of climate, weather, and fuel factors influencing a large, destructive wildfire in the Knysna region, South Africa. Fire Ecology 14, 4.
| Crossref | Google Scholar |
Kreyling J, Dengler J, Walter J, Velev N, Ugurlu E, Sopotlieva D, Ransijn J, Picon-Cochard C, Nijs I, Hernandez P, Güler B, von Gillhaussen P, De Boeck HJ, Bloor JMG, Berwaers S, Beierkuhnlein C, Arfin Khan MAS, Apostolova I, Altan Y, Zeiter M, Wellstein C, Sternberg M, Stampfli A, Campetella G, Bartha S, Bahn M, Jentsch A (2017) Species richness effects on grassland recovery from drought depend on community productivity in a multisite experiment. Ecology Letters 20, 1405-1413.
| Crossref | Google Scholar | PubMed |
Lentile LB, Holden ZA, Smith AMS, Falkowski MJ, Hudak AT, Morgan P, Lewis SA, Gessler PE, Benson NC (2006) Remote sensing techniques to assess active fire characteristics and post-fire effects. International Journal of Wildland Fire 15, 319-345.
| Crossref | Google Scholar |
Letts B, Lamb EG, Mischkolz JM, Romo JT (2015) Litter accumulation drives grassland plant community composition and functional diversity via leaf traits. Plant Ecology 216, 357-370.
| Crossref | Google Scholar |
Martin RA, Hamman ST (2016) Ignition patterns influence fire severity and plant communities in Pacific Northwest, USA, prairies. Fire Ecology 12, 88-102.
| Crossref | Google Scholar |
McCune B, Keon D (2002) Equations for potential annual direct incident radiation and heat load. Journal of Vegetation Science 13, 603-606.
| Crossref | Google Scholar |
Morgan P, Hardy CC, Swetnam TW, Rollins MG, Long DG (2001) Mapping fire regimes across time and space: understanding coarse and fine-scale fire patterns. International Journal of Wildland Fire 10, 329.
| Crossref | Google Scholar |
Neary DG, Klopatek CC, DeBano LF, Ffolliott PF (1999) Fire effects on belowground sustainability: a review and synthesis. Forest Ecology and Management 122, 51-71.
| Crossref | Google Scholar |
Nelson JRM (2002) An effective wind speed for models of fire spread. International Journal of Wildland Fire 11, 153.
| Crossref | Google Scholar |
Nguyen CT, Chidthaisong A, Kieu Diem P, Huo L-Z (2021) A modified bare soil index to identify bare land features during agricultural fallow-period in Southeast Asia using Landsat 8. Land 10, 231.
| Crossref | Google Scholar |
Ohrtman MK, Clay SA, Smart AJ (2015) Surface temperatures and durations associated with spring prescribed fires in eastern South Dakota tallgrass prairies. The American Midland Naturalist 173, 88-98.
| Crossref | Google Scholar |
Pennock D, Bedard-Haughn A, Viaud V (2011) Chernozemic soils of Canada: genesis, distribution, and classification. Canadian Journal of Soil Science 91, 719-747.
| Crossref | Google Scholar |
Pyne SJ (1983) Indian fires. Natural History (USA) 2, 6-11.
| Google Scholar |
Rikimaru A, Roy PS, Miyatake S (2002) Tropical forest cover density mapping. International Society for Tropical Ecology 43, 39-47.
| Google Scholar |
Sala OE, Parton WJ, Joyce LA, Lauenroth WK (1988) Primary production of the central grassland region of the United States. Ecology 69, 40-45.
| Crossref | Google Scholar |
Scasta JD, Weir JR, Stambaugh MC (2016) Droughts and wildfires in western U.S. rangelands. Rangelands 38, 197-203.
| Crossref | Google Scholar |
Schepers L, Haest B, Veraverbeke S, Spanhove T, Vanden Borre J, Goossens R (2014) Burned area detection and burn severity assessment of a heathland fire in Belgium using airborne imaging spectroscopy (APEX). Remote Sensing 6, 1803-1826.
| Crossref | Google Scholar |
Shuman CS, Ambrose RF (2003) A comparison of remote sensing and ground-based methods for monitoring wetland restoration success. Restoration Ecology 11, 325-333.
| Crossref | Google Scholar |
Wei T, Simko WT (2024) R package ‘corrplot’: Visualization of a Correlation Matrix (Version 0.94). Available at https://github.com/taiyun/corrplot.
Smith AM, Hill MJ, Zhang Y (2015) Estimating ground cover in the mixed prairie grassland of southern Alberta using vegetation indices related to physiological function. Canadian Journal of Remote Sensing 41, 51-66.
| Crossref | Google Scholar |
Steiner JL, Wetter J, Robertson S, Teet S, Wang J, Wu X, Zhou Y, Brown D, Xiao X (2020) Grassland wildfires in the southern Great Plains: monitoring ecological impacts and recovery. Remote Sensing 12, 619.
| Crossref | Google Scholar |
Thomas JC, Hadden RM, Simeoni A (2017) Experimental investigation of the impact of oxygen flux on the burning dynamics of forest fuel beds. Fire Safety Journal 91, 855-863.
| Crossref | Google Scholar |
Trigg S, Flasse S (2001) An evaluation of different bi-spectral spaces for discriminating burned shrub-savannah. International Journal of Remote Sensing 22, 2641-2647.
| Crossref | Google Scholar |
Tucker CJ (1979) Red and photographic infrared linear combinations for monitoring vegetation. Remote Sensing of Environment 8, 127-150.
| Crossref | Google Scholar |
VandenBygaart AJ (2010) Regosolic soils of Canada: genesis, distribution and classification. Canadian Journal of Soil Science 91, 881-887.
| Crossref | Google Scholar |
Vera-Velez R, Grover S, Bischoff BK, Carlyle CN, Lamb EG (2023) Wildfire-grazing impact on forage quality assessed with near-infrared spectroscopy and generalized partial least squares regression. Rangeland Ecology & Management 87, 132-140.
| Crossref | Google Scholar |
Weise D, Biging G (1996) Effects of wind velocity and slope on flame properties. Canadian Journal of Forest Research 26, 1849-1858.
| Crossref | Google Scholar |
Whealan RJ (2009) The ecology of fire – developments since 1995 and outstanding questions. Proceedings of the Royal Society of Queensland 115, 59-68.
| Crossref | Google Scholar |
Wood SN (2011) Fast stable restricted maximum likelihood and marginal likelihood estimation of semiparametric generalized linear models. Journal of the Royal Statistical Society: Series B (Statistical Methodology) 73, 3-36.
| Crossref | Google Scholar |
Wragg PD, Mielke T, Tilman D (2018) Forbs, grasses, and grassland fire behaviour. Journal of Ecology 106, 1983-2001.
| Crossref | Google Scholar |
Xu D, Guo X (2015) Some insights on grassland health assessment based on remote sensing. Sensors 15, 3070-3089.
| Crossref | Google Scholar | PubMed |
Xu D, Guo X, Li Z, Yang X, Yin H (2014) Measuring the dead component of mixed grassland with Landsat imagery. Remote Sensing of Environment 142, 33-43.
| Crossref | Google Scholar |