Influence of fuel data assumptions on wildfire exposure assessment of the built environment
Air M. Forbes

A
Abstract
Land cover information is routinely used to represent fuel conditions in wildfire hazard, risk and exposure assessments. Readily available land cover data options that vary in resolution, extent, cost and purpose of collection have become increasingly accessible in recent years.
This study investigates the sensitivity of community-scale wildfire exposure assessments to different land cover information products used to identify hazardous fuel.
Ten versions of a community wildfire exposure assessment were conducted for each of five case study locations in Alberta, Canada, by varying the input land cover data. Proportional and spatial distribution of hazardous fuels and classified exposure are compared across datasets and communities.
We found proportional and spatial variation of exposure values between datasets within each community, but the nature of this variation differed between communities. Land cover classification definitions and scale were important factors that led to inconsistencies in assessment results.
Readily available land cover information products may not be suitable for exposure assessments at a localised scale without consideration of unique context and local knowledge of the assessment area.
Results may inform fuel data selection considerations for improved results in various wildfire applications at localised scales.
Keywords: Alberta, Canada, community protection, fuel management, fuel mapping, land cover, spatial data, wildland fire, risk assessment.
Introduction
Data representing wildland fuels (i.e. flammable vegetation) are essential for assessing fire danger and modelling fire behaviour (Keane et al. 2001; Allgӧwer et al. 2003). As a component of the fire environment, fuel characteristics interact with weather and topography to drive fire behaviour (Countryman 1972). Fuel data are inherently spatial, tied directly to a real-world geographic location. Fuel data can range in complexity from a simple classification of dominant land cover (e.g. Forestry Canada Fire Danger Group 1992; Nadeau et al. 2005; Latifovic et al. 2017) to detailed inventories of fuel characteristics within a stand (e.g. Phelps et al. 2022) or from advanced remote sensing techniques like LiDAR (light detection and ranging) to map the vertical structure of a forest (Chuvieco et al. 2020; Abdollahi and Yebra 2023). Fuel data are frequently integrated with weather information to predict rates of spread and intensity (e.g. Forestry Canada Fire Danger Group 1992; Scott and Burgan 2005), estimate fire growth (e.g. Finney 2006; Tymstra et al. 2010), or map burn probability when ignition patterns are also incorporated (e.g. Pariesien et al. 2005; Finney et al. 2011). Wildfire management decisions are driven by models that utilise fuel data as an input, so it is imperative that these data are representative of the real-world conditions. Parisien et al. (2011) found that fuel input data contributed the most to spatial pattern of burn probability model outputs, Parks et al. (2011) found fuel influential for burn probability at multiple scales, and Beverly et al. (2009) found the likelihood of high and extreme Fire Susceptibility Index (FSI) values in a given location was strongly associated with the surrounding proportion of conifer fuels. Existing defined fuel types in the Canadian Forest Fire Behaviour Prediction (FBP) System (Forestry Canada Fire Danger Group 1992), a foundational system that has supported decades of wildfire research in Canada, have also been shown to misrepresent fuels in southern British Columbia (Baron et al. 2024) and ignores within-type variability, as demonstrated in forest ecosystems in Alberta by Phelps and Beverly (2022) and Cameron et al. (2021).
The wildfire exposure metric was developed as a method to assess fuel independently from other fire controls that may exhibit comparatively greater temporal and spatial variability (Beverly et al. 2021). The metric is a numerical value that indicates the potential for fire to transmit to a location irrespective of weather or ignition patterns. Addressing the components of wildfire risk (i.e. fuel, weather, ignitions) separately allows customised assessments that match the temporal variability in wildfire decision making. Operational decision horizons can vary from days to minutes, whereas mitigation decision horizons can span months to years. Decoupling and quantifying components individually allows relatively static elements like fuel and values at risk to be assessed well in advance of a wildfire for mitigation planning decisions (McFayden et al. 2019; Beverly et al. 2021; Macauley et al. 2022; Beverly and Forbes 2023). Elements that are more variable, like weather, can then be integrated and updated as conditions change over shorter time periods.
The concept of wildfire exposure was first proposed by Beverly et al. (2010) to evaluate potential ignition sources from wildland fuels to the built environment for four communities in Alberta, Canada. This analysis identified three wildfire ignition processes that could result in transmission from vegetated fuel to another location: radiant heat, short-range ember spotting, and long-range ember spotting. Those methods were then adapted and validated for landscape-scale assessments in the province of Alberta (Beverly et al. 2021). When validated against real-world fire events, the landscape exposure metric performed well across Alberta in all but 1 of 11 ecological units (Beverly et al. 2021) and has recently been shown to preform well in Alaska (Schmidt et al. 2024). Recent applications of the exposure metric include directional wildfire pathway identification (Beverly and Forbes 2023) and transportation network vulnerability assessments (Kim et al. 2024). Community-scale wildfire exposure assessments are advertised by FireSmart Canada as a planning tool for prioritising mitigation efforts (FireSmart Canada 2018). Community exposure assessments identify areas and values that are exposed to potential ignition sources (i.e. fire entry points) within the built environment, and where surrounding fuels are contributing to that increased exposure.
Fuel data are the only input required to compute wildfire exposure. The first step of the analysis is to reclassify the fuel into a binary hazardous layer for the transmission method being considered (i.e. short- or long-range ember transport, radiant heat); for example, conifer fuels alone are deemed hazardous for long-range ember spotting in Alberta. Exposure assessment data requirements are unique as they can accommodate a variety of pre-existing land cover information products, unlike most wildfire applications that typically demand narrowly defined fuel characteristics. Land cover information is a spatially explicit representation of dominant land cover types on the Earth’s surface using a defined classification scheme (Wulder et al. 2018). FBP System fuel type maps are one of many examples of land cover information products. Land cover maps are often derived from remotely sensed imagery with classification schemes defined to meet a specific objective. Availability of land cover information products has increased in recent years as technological advances have made production and distribution faster, easier and more accessible (Wulder et al. 2018).
Although many options are available, some data products may not be suitable for the intended purpose. This suitability is often referred to as the fitness for use, which can be assessed based on data source, scale, cost, format and more. Land cover information for a given location can vary by source depending on the classification methods applied or the purpose it was created for, which can result in markedly different yet equally correct information in the same location (Comber et al. 2004). For example, an area that is dominated by mature white spruce (Picea glauca (Moench) Voss) trees could be classified as ‘White Spruce’, ‘Spruce’, ‘Conifer Forest’, ‘Merchantable Timber’, ‘Forested’, or ‘Natural’, which are all valid classifications though some are less helpful for indicating hazardous fuels. When conducting an exposure assessment, the required scale (i.e. spatial, temporal and operational) will also depend on the scale of the wildfire transmission mechanisms being considered (i.e. short- or long-range ember transport, radiant heat). The scale of data is introduced at the observation process (Frank 2010).
Spatial scale pertains to the resolution and extent of the data. Spatial resolution denotes the smallest captured area or object in the data, expressed as a linear unit for raster data or a minimum mappable unit (MMU) for vector data. Spatial scale can also describe the extent or total area that is represented by a dataset. Temporal scale relates to the history of a dataset (i.e. how long it has been maintained), the currency of a dataset (i.e. when it was captured), or the consistency of the data over time (i.e. the duration the data remain accurate). Generally, as spatial resolution becomes finer, the temporal resolution will become coarser, so compromises are often made in favour of one or the other.
Operational scale can be described as the scale at which the process or phenomenon operates (Sayre 2005). The operational scale of the exposure transmission process of interest should match the operational scale of the data being used. For example, an exposure assessment addressing long-range ember transport occurring over distances of hundreds of metres can be computed with a coarser-resolution hazardous fuel layer (i.e. 100 m) than an exposure assessment of radiant heat transmission that occurs over distances of metres to tens of metres. Clearly, a process governed by variation within a 100-m pixel in a coarse-resolution land cover grid cannot be informed by the uniform, homogeneous classification of that pixel at that resolution. Further, a hazardous fuel layer with a resolution of 3 m would be appropriate for a local assessment of radiant heat transmission, but that level of detail would be unnecessary for a landscape-scale process.
Previous research has independently applied the exposure metric with a single unique input fuel layer which differ in scale and source between studies (see Beverly et al. 2010, 2021, Schmidt et al. 2024). In the present study, we investigate the sensitivity of community-scale wildfire exposure assessments by varying the land cover information product used to identify hazardous fuels. Ten land cover datasets were used to generate exposure assessments within the built environment of five communities in Alberta, Canada. These datasets were selected and modified to represent readily available options that a community may access. Each of the selected datasets exhibits variation in purpose of collection, scale and cost, with unique compromises in relation to the fitness for use. The selected datasets have notable differences in land cover classifiers and spatial resolution. Each community also differed, with unique surrounding land cover, quantity of values present and morphology of the built environment. We compare the proportional exposure distribution and spatial agreement of hazard and exposure classifications derived from the different input land cover datasets within each community and by exposure transmission process.
Methods
Study area
We included five communities within Alberta, Canada (Fig. 1, Table 1): High Level (58°30′N, 117°08′W), Hinton (53°24′N, 117°35′W), Whitecourt (54°08′N, 115°41′W), Nordegg (52°29′N, 116°04′W) and Crowsnest Pass – an amalgamation of Bellevue, Blairmore, Coleman, Frank and properties within Improvement District No. 5 (49°36′N, 114°26′W). All five communities are considered part of the wildland–urban interface (WUI), a transition zone between wildland fuels and human development (USDA and USDI 2001). The selected communities are also located within Alberta’s Forest Protection Area, the administrative boundary that delineates land managed by the province in respect to the prevention and control of wildfire and other forest disturbances. Selected communities had pre-existing local contacts and data-sharing agreements established from earlier projects. All five communities also demonstrated a history of action and growing interest in wildfire planning and preparedness (e.g. Montane Forest Management Ltd 2016). Wildfire occurrence and evacuations are not uncommon for Albertan communities: during the 2023 fire season, 38,000 residents were evacuated across the province (Government of Alberta 2023); the 2016 Horse River Fire had the largest wildfire evacuation in Canadian history, displacing over 88,000 people (McGee 2019), and in 2019, over 3,000 residents were evacuated from the included case-study community of High Level during the Chuckegg Creek Fire (MNP 2020).
Location of the five communities in Alberta, Canada, with natural regions from the provincial ecological land classification system (Downing and Pettapiece 2006).
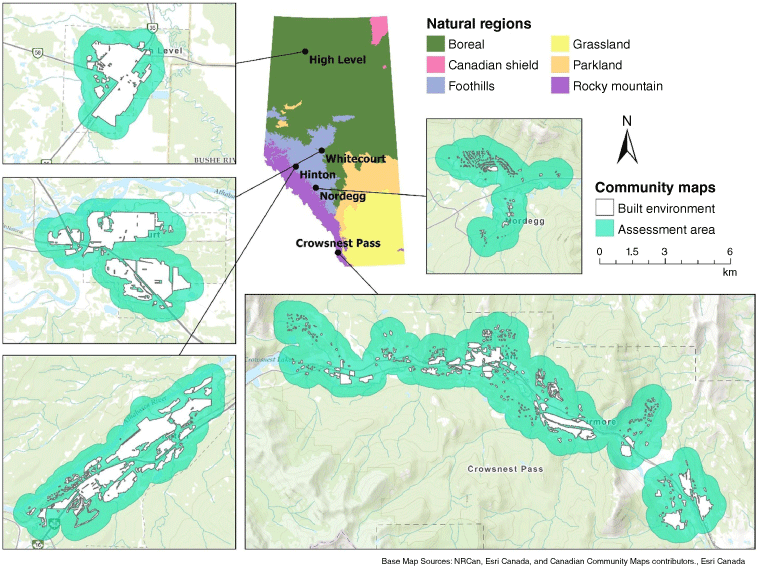
Area (km2) | |||||||
---|---|---|---|---|---|---|---|
Community | Population | Natural Region (Subregion) A | Common tree species A | Number of structures | Built environment | Assessment area | |
Crowsnest Pass | 5642 | Rocky Mountain (Montane) | Pinus contorta Dougl. var. latifolia Engelm. | 5065 | 7.9 | 67.4 | |
Pseudotsuga menziesii (Mirb.) Franco Populus tremuloides Michx. | |||||||
High Level | 3293 | Boreal Forest (Dry Mixedwood) | Populus tremuloides Michx. | 2256 | 5.9 | 15.9 | |
Picea glauca (Moench) Voss | |||||||
Hinton | 10,087 | Rocky Mountain (Montane) | Pinus contorta Dougl. var. latifolia Engelm. | 4421 | 9.5 | 33.1 | |
Pseudotsuga menziesii (Mirb.) Franco | |||||||
Populus tremuloides Michx. | |||||||
Nordegg | 200 | Foothills (Upper Foothills) | Pinus contorta Dougl. var. latifolia Engelm. | 775 | 0.6 | 15.5 | |
Picea mariana (Mill.) B.S.P. | |||||||
Picea glauca (Moench) Voss | |||||||
Whitecourt | 9926 | Boreal Forest (Central Mixedwood) | Populus tremuloides Michx. | 4457 | 9.6 | 27.6 | |
Picea glauca (Moench) Voss | |||||||
Pinus banksiana Lamb. |
The vegetation surrounding each community is variable owing to Alberta’s diverse land cover; common tree species as defined by the Natural Subregions (NSR) are listed in Table 1 (Downing and Pettapiece 2006; Fig. 1). Wildfire frequency and area burned are highest in the Central Mixedwood NSR, where Whitecourt is located, but it also is the largest NSR in the province (Tymstra et al. 2005; Ahmed and Hassan 2023). The Montane NSR, where Crowsnest Pass and Hinton are located, has the highest wildfire occurrence when compared per unit area (Tymstra et al. 2005). The Montane NSR is also densely populated and contains many transportation corridors within valley bottoms (Tymstra et al. 2005). The Dry Mixedwood NSR, where High Level is located, has a higher density of agricultural land and settlements than the Central Mixedwood NSR, which allows rapid wildfire detection and suppression in this area (Tymstra et al. 2005). Provincially, 55% of ignitions are caused by humans but lightning ignitions account for most of the area burned (Ahmed and Hassan 2023). The proportion of ignition cause varies by NSR. The Lower Foothills and Central Mixedwood are similar to the provincial numbers but ignitions in the Montane and Dry Mixedwood NSRs are most often caused by humans; however, human ignitions are observed most often near anthropogenic features across the province as a whole (Tymstra et al. 2005; Ahmed and Hassan 2023).
Data
The boundary of the built environment was delineated manually for each community following Beverly et al. (2010) using 0.5 m resolution Pleiades satellite imagery and other sources (i.e. Microsoft building footprints (Microsoft 2019), and Google Maps). Structures and any immediately adjacent developed features including lawns, streets, parking lots and managed vegetation were included in the built environment polygon, whereas patches of unmanaged vegetation and major roads were omitted. A 600-m buffer was applied to the built environment polygons to generate an assessment area for each community (Fig. 1). The assessment area and built environment sizes and a count of identified structures for each community are shown in Table 1. The extent of the built environment, assessment area and number of structures varied by community. Nordegg had the smallest extent and fewest structures. In contrast, the attributes of the built environment were all similar when comparing Hinton and Whitecourt. Crowsnest Pass had a notably large assessment area due to the unique morphology of the built environment (Fig. 1, Table 1).
Six land cover datasets were obtained in raster format to serve as inputs to wildfire exposure assessments from different sources (Table 2). We selected datasets that vary in classification scheme, extent, resolution and cost to represent available options that could be acquired by agencies, communities, or individuals undertaking exposure assessments. Labels are used to identify each dataset by the classification scheme used and the raster resolution. For example, the label FBP30 specifies a dataset that has been classified using FBP System fuel types and has a resolution of 30 m (LCCS, Land Cover Classification System; COM, commissioned).
Transmission method | ||||||||||
---|---|---|---|---|---|---|---|---|---|---|
ID | Dataset description | Resolution (m) | Year | Extent | Source | Availability | Long-range ember transport | Short-range ember transport | Radiant heat | |
FBP100 | Provincial Fuel Type Classification | 100 | 2022 | Alberta | Alberta Wildfire | By request, with data use agreement | √ | Insufficient resolution | ||
LCCS30 | National Land Cover Classification | 30 | 2020 | Canada | NRCan | Open data | √ | √ | ||
FBP30a | National Fuel Type Classification | 30 | 2020 | Canada | NRCan | Future open data | √ | √ | ||
FBP30b | Modified Version of FBP30a | – | √ | |||||||
COM30a | Commissioned Classified LANDSAT Imagery Product | 30 | 2021 | Community | Independent consultant | Purchased | √ | √ | ||
COM30b | Modified Version of COM30a | – | √ | |||||||
COM10a | Commissioned Classified Sentinel 2 Imagery Product | 10 | 2021 | Community | Independent consultant | Purchased | √ | √ | √ | |
COM10b | Modified Version of COM10a | – | √ | √ | ||||||
COM3a | Commissioned Classified Planetscope Imagery Product | 3 | 2021 | Community | Independent consultant | Purchased | √ | √ | √ | |
COM3b | Modified Version of COM3a | – | √ | √ |
Note: modifications do not affect the computation of long-range exposure.
Land cover classifications for the entire Canadian land base are captured in two datasets, labelled LCCS30 and FBP30, which were both sourced from Natural Resources Canada (NRCan). The national extent of these datasets offers the unique advantage of standardising input data across the largest area. Both products have a spatial resolution of 30 m, which allows both long- and short-range ember exposure to be assessed. The Land Cover Classification System (LCCS) dataset, labelled LCCS30, is derived from LANDSAT imagery for the purpose of tracking land cover changes across North America (Commission for Environmental Cooperation 2023). The LCCS is well documented and globally recognised (Di Gregorio 2005; Commission for Environmental Cooperation 2023), and the detailed descriptions of each cover type in this documentation are helpful for differentiating between hazardous and non-hazardous fuels for the purpose of an exposure assessment. This product is updated every 5 years, with availability typically lagging the reference year by several years. For example, the 2020 product was not available to publicly download until 2022, and the full continental extent was not available until 2023. The methods used to produce these data are extensively documented in Latifovic et al. (2017), and Latifovic and Pouliot (2005). We obtained the most recent available version (2020), which is freely available from the Government of Canada’s open data portal. The national FBP System fuel grid (FBP30) used is an early version of a forthcoming product that is in development to replace the 250 m resolution national fuel grid that has been in use since 2005 (Nadeau et al. 2005). Contrasting the LCCS classifiers, the FBP System fuel types are based on the fuel characteristics that influence fire behaviour, which makes them well suited for wildfire-related assessments. It is expected that this data product will be publicly available along with well-documented metadata when it is complete. We acknowledge that there is increased uncertainty associated with a dataset that is a work in progress.
In addition to FBP30, a second dataset using the FBP System fuel type classifications is also included. We obtained FBP100, the 2022 provincial fuel grid, from the Alberta Wildfire Management Branch. Fire management in Canada is a provincial or territorial responsibility and associated government agencies generate fuel map layers to inform a wide range of fire management decisions and activities. This data product is not publicly available and metadata records are limited, though some documentation about the initial development does exist (e.g. Frederick 2012). The provincial fuel grid was developed with data from the Alberta Vegetation Inventory and other sources. Areas that have been disturbed by wildfire and forest harvesting are updated annually using detailed cut block reports from forest management companies and wildfire perimeters. Land cover changes that evolve gradually (e.g. a successional transition from mixedwood to conifer) may not be reflected in annual updates. Of all included datasets, FBP100 is updated most frequently. However, the 100 m spatial resolution of these data limits exposure assessments to long-range ember transmission.
Three additional datasets, labelled with COM, were commissioned from an independent consultant to represent typical data products that would be obtained from a commercial supplier for wildfire risk assessments. Commissioned data products are typically generated using proprietary methods that are not openly shared owing to their perceived commercial value. These data are therefore presented without any formal documentation of the methods used to produce them. Datasets for each community were produced independently at three resolutions (3, 10 and 30 m) covering the extent of the assessment areas. A machine learning algorithm was used to categorise land cover into predetermined classes from three satellite imagery sources: Planet Scope (3 m resolution, COM3), Sentinel 2 (10 m resolution, COM10) and LANDSAT8 (30 m resolution, COM30). The COM datasets were the only included options that have a financial cost, but they are available at higher spatial resolutions than the other data options included. The COM3 and COM10 datasets are the only products that allow for exposure assessments of all three scales of wildfire transmission (i.e. long- and short-range embers and radiant heat).
A visual assessment of each dataset was conducted prior to any analysis to evaluate the fitness for use in calculating community-scale exposure. Differences in the classification of grass-dominated land cover were an obvious issue. Natural grass land cover is an important fuel type in Alberta, where grass fires are a common occurrence during spring conditions. Grass land cover that is anthropogenically influenced, such as lawns and agricultural crops, do not fit the description of a grass fuel type because they are maintained and altered in an often manicured (i.e. mowed) or irrigated state. Community-scale exposure assessments address fire transmission processes associated with grass type fuels (i.e. short-range ember transport, radiant heat) and are therefore sensitive to how those fuels are classified. This observation prompted development of four modified versions of the original datasets, where labels are paired with a suffix of a for unmodified or b for modified, for a total of 10 land cover datasets overall (Table 2).
The datasets were modified by adjusting classifications where grass type land cover is observed. Observations of grass type land cover in the COMa datasets revealed presence of grass in two classes: grassland and cropland. Depending on the community, agricultural areas are included within the grassland class or are classified independently as croplands. Grasses that would be considered fuel and non-fuel are both present within the grassland class. The COMb datasets were produced by a GIS (geographic information system) technician who manually delineated areas with grass type land cover in COMa datasets into three new classes: maintained grass, cropland and natural grass. Grass type land cover in FBP30 was present in two classes: grasslands and non-fuel. Most of the maintained grasses are present in the non-fuel class. The grassland class had natural grasses and all of the agricultural areas with minimal presence of maintained grasses present. Grass type land cover in LCCS30 was observed in three classes: grassland, cropland and urban/built up. Maintained grasses are primarily included in the urban and built up areas but some are also present in the grassland class. The cropland class captures agricultural areas within the study area well. The inclusion of agricultural areas in the FBP30 grassland class was concerning and prompted the production of a modified FBP30b dataset by updating all areas originally classified as grasslands with the intersecting classifications from LCCS30. Grass classification modifications were not made to LCCS30 because no major discrepancies were observed. Adjustments for grass classifications were also not required for FBP100, which is only used in computations of long-range ember exposure.
Key characteristics for all 10 datasets are summarised in Table 2. A full table of areal proportions for the unique land cover classes used by each dataset is available in the Supplementary Material S1 (see Supplementary Table S1).
Assessment
Each land cover dataset was used to compute wildfire exposure for each of the transmission scales the resolution allowed for (Table 2). First, each dataset was reclassified into binary hazardous fuel layers for the study area. In Alberta, fuels capable of transmitting long-range embers have been defined as conifer and mixedwood stands with greater than 25% conifer composition (Beverly et al. 2010, 2021). Short-range ember and radiant heat transmission entail shorter assessment distances and specify conifer stands, all mixedwood stands, deciduous stands, wildland grasses and shrubs as hazardous fuels (Beverly et al. 2010). Reclassification decisions were unique for each dataset and transmission scale because of the variation in land cover classifiers used – a full table of these decisions is available in the Supplementary Material S1 for all datasets (see Supplementary Tables S1 and S2). All land cover datasets were reclassified into short-range and radiant heat hazardous fuel layers except for FBP100 owing to its coarse resolution. Long-range hazardous fuel layers were derived from FBP100, LCCS and the unmodified (suffix a) landcover datasets. The modifications to the suffix b datasets only affect the grass type land cover classes so the long-range hazardous fuel reclassifications produce the same output.
The exposure metric is calculated by evaluating each pixel of the hazardous fuel layer using a moving window, sized according to the transmission scale, to assess the proportion of surrounding hazard (Beverly et al. 2021). Calculated exposure values range from 0 to 1. A pixel with an exposure value of 0 (0%) indicates that there is no hazardous fuel within the specified transmission distance. Therefore, none of the surrounding pixels are capable of transmitting fire to that location. Conversely, a fire in that location has no surrounding pixels to spread to. A pixel with an exposure value of 1 (100%) indicates that hazardous fuel occupies all areas within the specified transmission distance, so that pixel could transmit or receive fire to or from any cell within the transmission distance.
Nineteen exposure layers were created for each community, namely: six long-range ember exposure layers, nine short-range ember exposure layers and four radiant heat exposure layers (Table 2). Exposure values within the built environment were also classified as extreme (>45%), high (30–45%), moderate (15–30%), low (>0–15%) or nil (0%) following Beverly et al. (2010).
Hazardous fuel and exposure results for each dataset are compared by community and by wildfire transmission method. Comparing area-based and spatial-based consistencies is a common practice to assess agreement between land cover datasets (Gao et al. 2020; Liu et al. 2021, 2022). We computed the areal proportion of pixels within the assessment area classified as hazardous fuel and compared these proportions across datasets. An empirical cumulative distribution function (eCDF) of the continuous unclassified exposure values within the built environment is compared graphically.
To compare datasets spatially, a systematic 10-m point sampling grid was used to extract the underlying classification computed by each dataset across the community assessment areas. We opted for a sampling grid instead of a pixel-based resampling approach in similar comparative studies with variable resolutions (e.g. Liu et al. 2022) because we also wanted to capture the fuel input spatial resolution influences at the operational scale of community exposure assessments. In practice, values within the built environment are often object-based and mapped at a much finer spatial scale than the fuel data inputs used to assess them. Beyond a simple understanding of where the datasets agreed most frequently, we also sought to quantify any patterns of disagreement. The ordinality of exposure classes means that disagreements could be higher or lower than a reference dataset. Having no validated reference dataset to compare with, we opted to find the mode at each sampling location to serve as the majority agreement classification. This majority class does not serve as a way to assess the accuracy of any dataset, so results should only be interpreted as a measure of consensus between datasets. Any modal ties for exposure were broken by selecting the higher of the two exposure values. Datasets were then scored based on their disagreement with the majority classification. Additionally, the magnitude of difference from the exposure class majority was explored to identify if datasets exhibited patterns of higher or lower exposure values across communities and wildfire transmission method.
Results
Hazardous fuels within assessment area
If the input land cover datasets are each representing the fuel landscape effectively for the purpose of exposure assessments, we would expect to see similar proportions of hazardous fuels within each community despite the mixed resolutions and classifiers. The proportion of hazardous fuels within the 600 m assessment area varied by community and land cover dataset (Fig. 2). Nordegg exhibited the highest proportion of hazardous fuels, with an average of 71% hazard across the six datasets for long-range ember hazard and an average of 91% hazard across nine datasets for short-range ember hazard. High Level exhibited the lowest proportion, with an average of 11% hazard for long-range and 50% hazard for short-range. Crowsnest Pass and Hinton had similar proportions of hazardous long-range fuels; however, there was less variability across datasets in Hinton compared with Crowsnest Pass (Fig. 2a). This pattern was reversed for short-range hazard, where Hinton had more variability between datasets than Crowsnest Pass (Fig. 2b). The grass modifications reduced the proportion of short-range hazardous fuels between the a/b paired datasets for all communities with the exception of FBP30a/b in Nordegg and Hinton where there were no changes (Fig. 2b). The reduction of short-range hazard between the paired COM30 and COM3a datasets had more dramatic reductions in proportional exposure than FBP30 and COM10 (Fig. 2b).
Areal proportion of hazardous fuels in community assessment areas by dataset that are capable of (a) long-range ember transmission, and (b) short-range ember and radiant heat transmission.
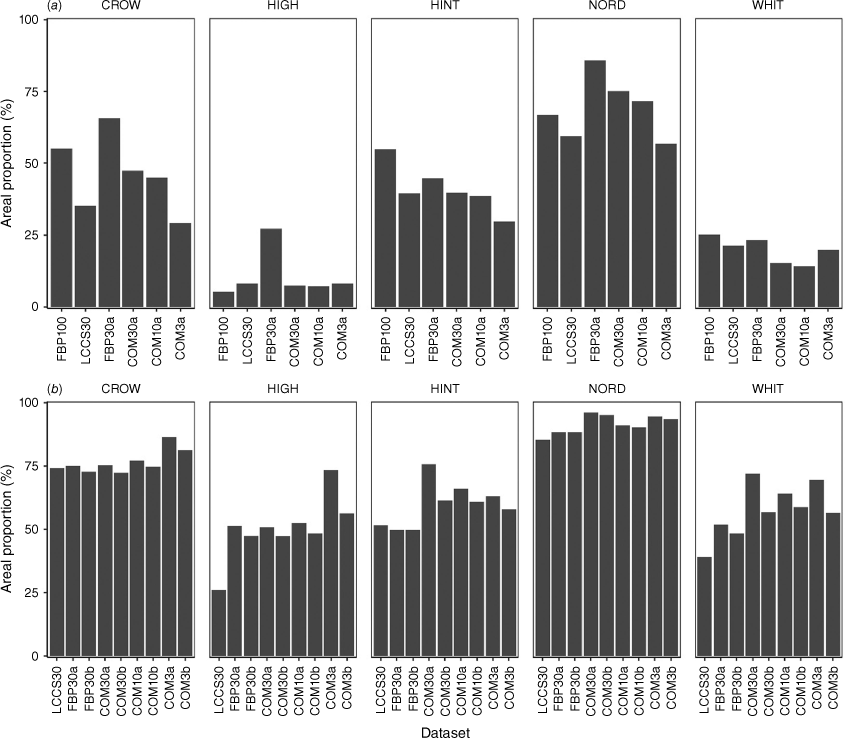
The pattern of proportional hazard variation between datasets was expected to remain consistent between communities because the production methods for each dataset were the same for all communities. For example, FBP30a had the highest proportion of long-range hazardous fuels between datasets in Crowsnest Pass, High Level and Nordegg, but this pattern did not continue in Hinton or Whitecourt (Fig. 2a). We found some patterns of similarity between datasets by community (Fig. 3). The general pattern of Nordegg having the highest proportion of hazardous fuels, Crowsnest Pass and Hinton having moderate proportions, and High Level and Whitecourt having the lowest proportions is clearly illustrated in Fig. 3. COM10a and COM30a were very consistent with each other between communities for proportional long-range hazard; however, COM3a from the same producer did not maintain this consistency (Fig. 3a). The same pattern of similarity was also observed for short-range hazard between COM10b and COM30b; interestingly, the patterns were least similar between the unmodified COM10a and COM30a overall (Fig. 3b). The effect of grass modifications to short-range and radiant heat hazard fuel proportions appears to have had the largest effect for COM30 and COM3 (Fig. 3b).
Heat maps illustrating Euclidean distances between areal proportion of hazardous fuels in community assessment areas by dataset that are capable of (a) long-range ember transmission, and (b) short-range and radiant heat transmission. The colour gradient represents the magnitude of difference between lower (light) and higher proportions (dark). Hierarchical clustering is used to show similarities between communities and datasets.
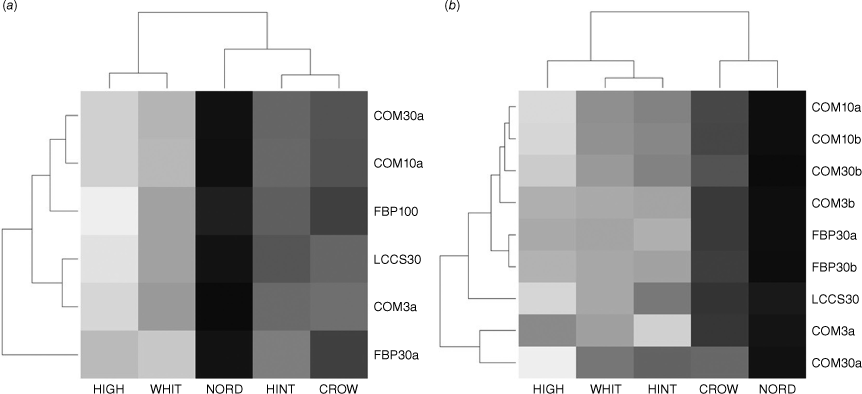
Classified exposure within the built environment
The distribution of exposure values and spatial agreement of exposure classes varied by dataset within each community and by transmission method. A full table of proportional distributions of classified exposure and high-resolution maps are available in the Supplementary Material S1. Fig. 4 illustrates the proportional and spatial variability of short-range ember exposure between four input fuel datasets for the community of Hinton, Alberta.
Spatial visualisation of differences in short-range ember hazardous fuels and classified exposure locations for the community of Hinton, Alberta, produced using four alternative land cover information sources (FBP30a, LCCS30, COM30a and COM30b). Land cover classifiers have been standardised to simplified categorical land cover classes for comparative purposes.
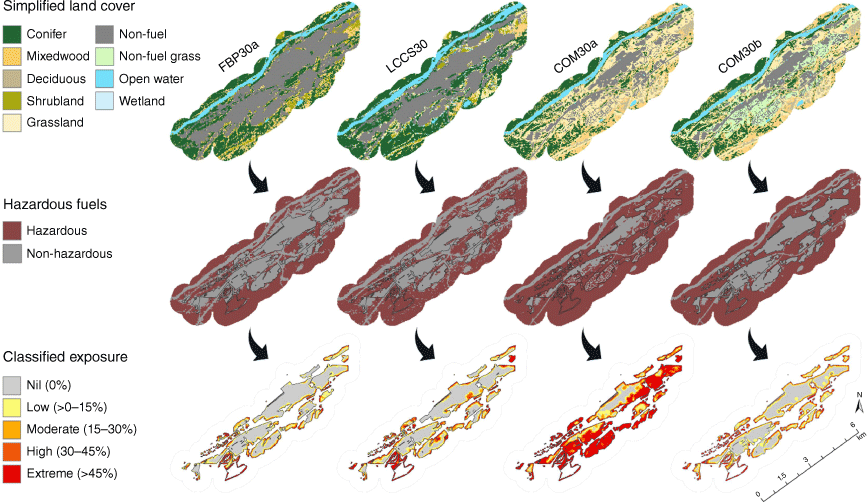
Proportional distribution
If the input datasets are effectively representing the same fuel landscape, we would expect the cumulative proportion of exposure values to follow the same distribution within each group. Empirical cumulative distribution functions (eCDFs) of the exposure values reveal that there are observable differences between datasets (Fig. 5). The eCDF represents the cumulative area of a certain exposure value. For example, the FBP30a (solid green) curve for short-range ember exposure in Hinton (Fig. 5, centre) has an eCDF of 0.75 for an exposure value of 0.15; this can be interpreted as 75% of the total area has an exposure value equal to or below 0.15. Comparatively, only 20% of the area is equal to or below 0.15 for COM30a (solid light blue) (Fig. 5).
Cumulative distribution functions of exposure values for input fuel datasets, by community and exposure transmission distance.
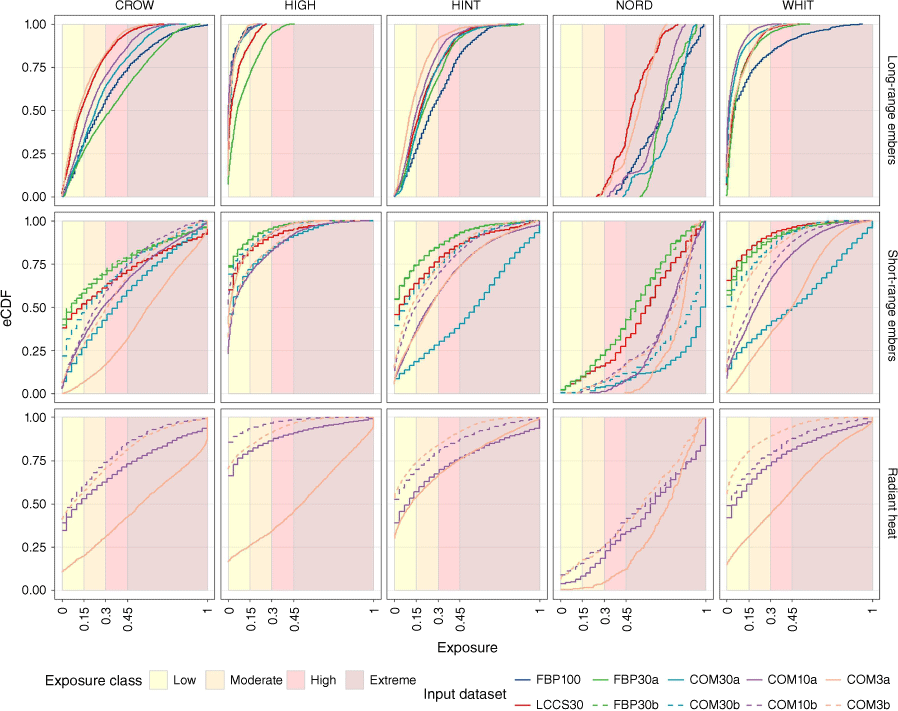
There are observable differences in the overall exposure distributions between communities (Fig. 5). For example, all the curves in Nordegg have lower eCDF intersections with extreme exposure when compared with other communities. Curves for long-range exposure in High Level and Whitecourt are positioned steeply in low exposure values, whereas curves in Crowsnest Pass and Hinton are more linear (Fig. 5). Differences in curve shape and position can be observed between datasets within each group. For long-range exposure, the dataset curves follow a similar shape within each community; however, differences are still apparent. Although the Nordegg curves are similar in shape, they are positioned apart from each other, and the start and end of each curve are farther apart (Fig. 5). The long-range exposure curves in the other communities have similar starting points, but there is more distance between the ends of the curves. Differences in shape between the short-range curves are more apparent. For example, the COM30a (solid light blue) and COM3a (solid orange) curves are much less steep than the others in all communities except High Level.
If each of the independent input data sources is produced equally across all study areas, we would expect the variation of the eCDF curves between datasets to remain consistent between communities. The order of the curves can be interpreted as the top- (or left-)most curve having lower exposure than the bottom- (or right-)most curve. There are some observable patterns, like LCCS30 (red) and FBP30 (green) curves remaining towards the top for short-range exposure (Fig. 5). For long-range exposure, the curve order shifts from community to community. For example, LCCS30 curve (red) is toward the top in Crowsnest Pass and Nordegg, but second from the bottom in High Level.
The reduction of exposure from grass type land cover modifications can be seen in the paired eCDF curves. The dashed modified dataset curves fall above the paired solid unmodified dataset curves (Fig. 5). The distance between them reveals the magnitude of impact of these modifications. For example, the radiant heat curves for COM3a/b are much farther apart than the COM10a/b curves. Kolmogorov–Smirnov test statistics did not indicate significant differences in the cumulative short-range exposure distributions for FBP30a/b in all communities except Crowsnest Pass (P-value 0.019). Kolmogorov–Smirnov test statistics indicated significant differences between all of the paired COM datasets except for the COM10 short-range exposure curve in Nordegg (P-value 0.053). This suggests the grass type land cover modifications to FBP30 did not have a significant impact on computing wildfire exposure in our study area.
Spatial agreement
The proportional distribution of exposure values does not indicate where they are located within the communities. The computed exposure values were classified and mapped to visualise differences in the spatial distribution of exposure (see Figs 4, 7, 8, and Supplementary Material S1).
Disagreement with the majority exposure class at sampling points for long-range ember transmission shows an inconsistent pattern from community to community (Fig. 6a). Overall, COM30a had the lowest disagreement between datasets, indicated by the smallest bar heights (Fig. 6a). FBP100 had the highest disagreement across datasets in most communities owing to the comparatively coarse resolution with the exception of Nordegg, which had homogeneous extreme exposure across all datasets; however, exposure values were more frequently above majority in Crowsnest Pass and Hinton, below majority in High Level and more evenly split in Whitecourt (Fig. 6a). FBP30a had a consistent pattern of above majority exposure values across all communities; over 30% of sampling points in Crowsnest Pass and High Level were classified higher than the majority (Fig. 6a). COM3a and LCCS30 exhibited a pattern of below majority exposure, especially in Crowsnest Pass (Fig. 6a).
Relative frequency and magnitude of exposure class disagreement from the majority in the built environment by dataset and community for (a) long-range ember transmission, (b) short-range ember transmission for original versions, and (c) short-range ember transmission for datasets with grass modifications. Bars above the black line are instances of disagreements above the majority classification, bars below the black line are instances of disagreements below the majority classification, and colour saturation indicates the difference in classes from the majority classification. The sum of both bars represents the total disagreement.
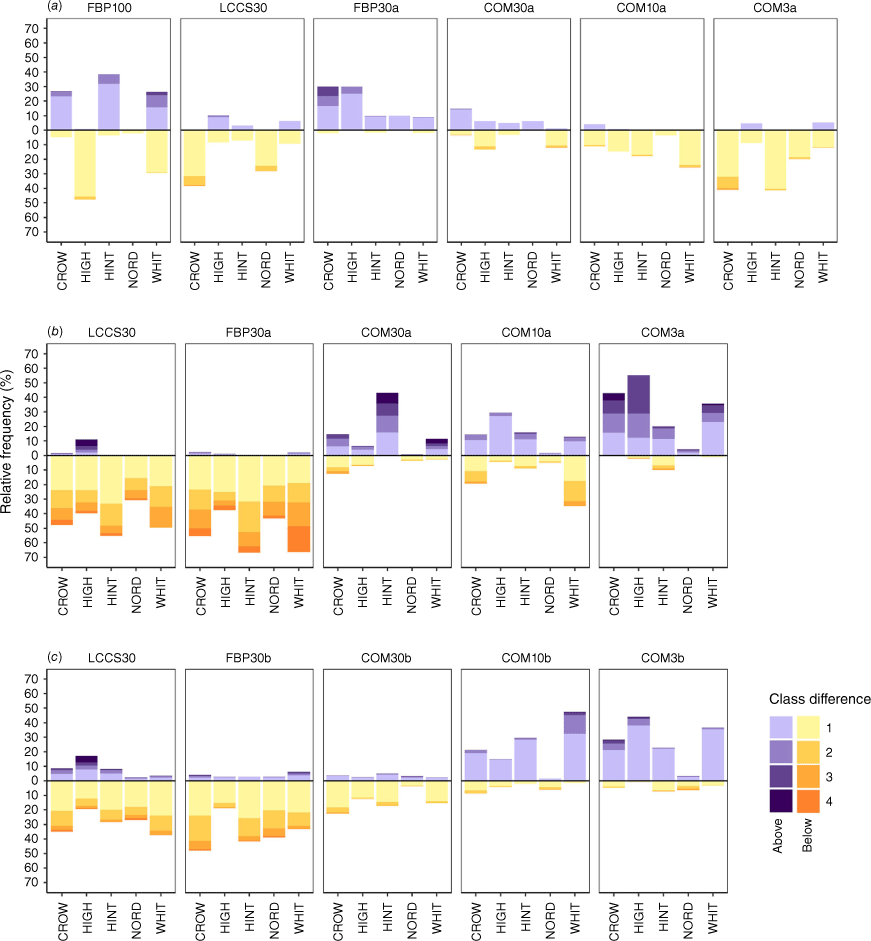
Disagreement with the short-range exposure majority classification was assessed separately for the original datasets (suffix a, Fig. 6b) and grass modified datasets (suffix b, Fig. 6c); LCCS30 is included in both sets. Total disagreement with the majority exposure class was much greater for the original datasets, with some datasets disagreeing by as much as 70% (Fig. 6b). Dataset majority disagreement for short-range exposure deviated upwards and downwards by as much as four exposure classes within the built environment with and without modifications (Fig. 6b, c). Patterns of dataset disagreement are not consistent between communities. For example, COM30a had the lowest disagreement with the majority between datasets in Nordegg, but the highest disagreement in Hinton. Overall, Nordegg had the least disagreement across all datasets owing to the high proportion of exposure values being classified as extreme (Fig. 5).
Pairwise spatial disagreement comparisons were also used to assess the impact of grass modifications on classified exposure. Overall, FBP30 had the lowest disagreement between datasets, with an average of 18% across communities, and the disagreement remained consistent between communities, with a difference of 4% between the maximum and minimum (Table 3). COM3 disagreements were the highest for both transmission methods, disagreeing with each other by as much as 84% in High Level for short-range exposure. Between all of the COM datasets, COM10 had the lowest disagreement for both transmission distances and those disagreements were more consistent from community to community (Table 3). The largest cross-community disagreement variability was for COM3, where the 20% disagreement in Nordegg was 64% lower than High Level (Table 3).
Radiant heat | Short-range embers | ||||||
---|---|---|---|---|---|---|---|
COM3 (%) | COM10 (%) | COM3 (%) | COM10 (%) | COM30 (%) | FBP30 (%) | ||
CROW | 67 | 42 | 59 | 27 | 49 | 18 | |
HIGH | 80 | 28 | 84 | 45 | 36 | 15 | |
HINT | 50 | 38 | 51 | 34 | 71 | 19 | |
NORD | 39 | 34 | 20 | 17 | 10 | 19 | |
WHIT | 72 | 37 | 78 | 36 | 70 | 18 | |
Range | 41 | 14 | 64 | 28 | 61 | 4 | |
Mean | 62 | 36 | 58 | 32 | 47 | 18 |
Discussion
We conducted community-scale exposure assessments for five communities in Alberta using 10 different land cover input data sources to represent hazardous fuels and compared the output wildfire exposure values. Results of our community-scale exposure assessments were highly sensitive to the choice of input fuel data. There were observable differences between the proportion of identified hazardous fuels, and the proportional and spatial distribution of exposure between datasets for a given community and transmission distance. Further, the differences between datasets did not remain consistent between communities. Despite this inconsistency, general patterns of proportional hazardous fuels and exposure between communities could be observed. The mismatched scales and production goals of the input data likely influenced the observable differences in land cover and resulting exposure within the study area. The unique land cover classifiers also produced variation in the amount and location of hazardous fuel and resulting exposure, especially the classification of anthropogenically influenced grasses. Comparisons were drawn to quantify differences between the input data sources; therefore, results do not indicate the accuracy or performance of the exposure assessments themselves. Further, the accuracy or performance of these data sources as an input to community-scale exposure assessments cannot be interpreted from the relative differences. The inclusion of a referenced and validated land cover product was beyond the scope of this project; however, the variation identified highlights that it is an important next step to assess the fitness for use of readily available land cover products for inputs to wildfire exposure assessments at this scale.
At spatial scales, there were differences between both the extent and resolution of the input datasets. The spatial extent of the input datasets covered national (FBP30 and LCCS30), provincial (FBP100) and local (COM datasets) areas. Validating the accuracy and precision of a dataset becomes more involved and more expensive as the extent broadens. It is not feasible, or expected, for data covering broad extents to be validated with the same methods that can be employed for a finer extent. For example, validating derived land cover classes for a data product that covers a single community could be achieved within a reasonable time frame with ground truthing, site visits, and/or local consultation and can offer a higher confidence in the accuracy. In contrast, validation of a dataset with a regional or national extent cannot be conducted to the same standard because of the scale. These datasets employ various algorithms to classify pixels into land cover classes, and because they cover large geographic areas, it is unlikely that a human has verified the classification decisions across the entire extent. Therefore, there is added data uncertainty when the extent is broad. Data products with broad extents do offer an advantage – the opportunity to standardise data, and the accompanying level of uncertainty, across many smaller study areas. In the context of data selection for exposure assessments, this can be particularly advantageous if the exposure of multiple communities within a larger management unit (e.g. a municipal district or administrative boundary) is to be summarised or compared (e.g. Beverly and Forbes 2023). The spatial resolution of input data determined which wildfire transmission mechanisms could be assessed (i.e. long-range ember transport, short-range ember transport and radiant heat). The products with fine resolution (i.e. COM10, COM3) capture more detail of land cover changes than the 30- and 100-m alternatives in this study. A single pixel of FBP100, equivalent to 1 ha, can only represent one dominant land cover in that area, whereas that same location is represented by 100 different pixels in COM10, allowing more variation in land cover to be captured.
Data selection for assessing exposure should be informed by the operational scale of the most refined wildfire transmission mechanism of interest. This includes the spatial and temporal scales but it should also include other factors pertaining to fitness for use. Community exposure assessments are conducted at a localised scale, but the operational scale of the three wildfire transmission mechanisms considered do vary. Long-range ember transport occurs over much larger distances (i.e. landscapes) than short-range ember transport and radiant heat. We found more consistency in the proportional and spatial distribution of exposure values for long-range ember exposure, indicating that the finer operational scale of short-range and radiant heat transmission assessments are more sensitive to differences in the input fuel data source. For example, the approach used to classify grass type land cover figured prominently in exposure differences between datasets for short-range ember and radiant heat exposure. Assessments of exposure to long-range embers were not sensitive to grass fuel classification approaches because grass fuel is not considered a hazardous fuel for ember transport over those longer distances, which highlights that data fitness for use must be assessed within the context of the intended application.
Differences in land cover classifiers were revealed during an initial visual assessment of the source data, a recommended best practice of any remotely sensed product (Olofsson et al. 2014), before any analysis was done. This led to the modification of classifiers in some source data to better represent grasses within the context of wildfire exposure assessments. We found that the COM datasets were more sensitive to the grass classification modifications than FBP30. This was likely influenced by where modifications occurred within the assessment areas. The grass type land cover that was adjusted in the COM datasets was located primarily within the built environment of the communities because lawns were being included in the original grassland class. The adjustments to classifiers in FBP30 primarily occurred within the assessment area buffer surrounding the built environment because corrections were made to exclude agricultural areas from the original grassland class. The impact of differences in land cover classifications to wildfire exposure assessments is not limited to grass; this was just the only issue we identified in our selected datasets and within our selected communities. Land cover classifiers were also adjusted by Schmidt et al. (2024) to better represent conifer fuels in their study area.
To illustrate how the grass type land cover classification differences we identified could potentially impact management decisions, an example is shown for Whitecourt. Fuels considered hazardous for exposure to short-range embers derived from three datasets are shown within an inset-area in the northeast section of Whitecourt in Fig. 7. For two of the datasets (LLCS30, FBP30), there are minimal hazardous fuels present within the built environment, but for COM30a, the inset area is almost completely covered with hazardous fuel. Unsurprisingly, corresponding exposure levels are markedly different, with COM30a producing a large core area of high exposure across the built environment. High exposure areas generated by the other two datasets (LLCS30, FBP30a) are limited to the fringe or edges of the built environment. Inspection of aerial imagery, also shown in Fig. 7, reveals hazardous fuel classification discrepancies within the built environment are associated with maintained lawns, sports fields and agriculture.
Whitecourt 3 m Planetscope aerial imagery (right) and hazardous fuels and short-range exposure maps made using LCCS30, FBP30a and COM30a. The extent of the hazard and exposure maps is shown in the red box.
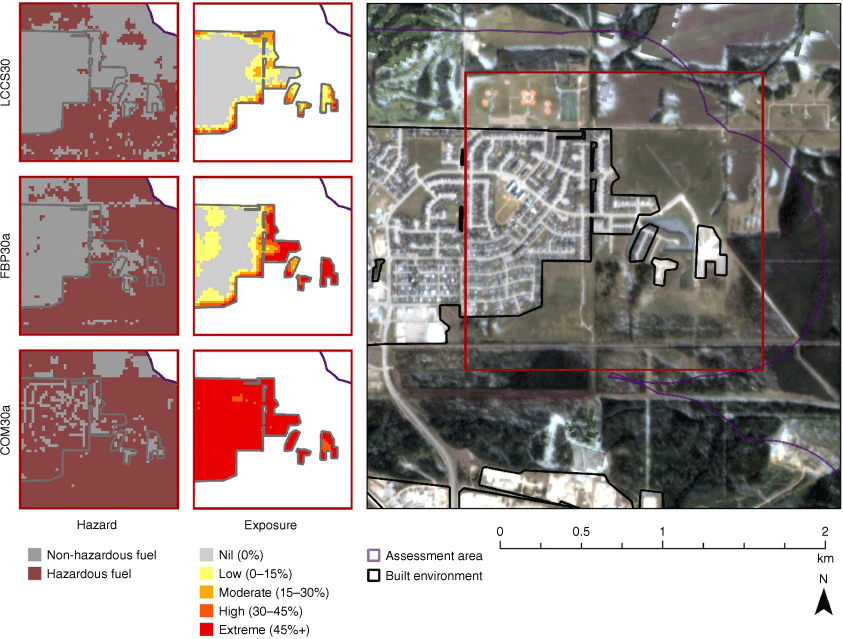
Maps are powerful tools for communicating spatially explicit information in an easily understandable format. A well-made map can appear authoritative regardless of the underlying data, which can lead to unintentional misinterpretations by map readers, or to future misuse of the information in subsequent geospatial analysis (Kraak and Ormeling 2010; Heywood et al. 2011). Maps that communicate information quickly and effectively are pivotal for wildfire decision making, and any uncertainty can have consequences (Boychuk et al. 2021). If a manager was tasked with identifying priority areas for fuel reduction treatments to mitigate short-range ember exposure in the neighbourhood mapped in Fig. 7, the decision could be very different depending on the exposure map that is presented.
The operational scale and small extent of community exposure assessments are conducive to verification efforts to assess the accuracy of fuel classifications through desktop interpretation of imagery, field-based ground-truthing and/or documentation of local knowledge and expertise. In addition to the insight gained about grass classification issues from inspection of aerial imagery in Whitecourt (Fig. 7), differences in long-range exposure were revealed in High Level. Hazardous fuel reclassifications and resulting exposure maps of the built environment using FBP100, FBP30a and LCCS30 reveal some obvious differences (Fig. 8). A log stockyard can be seen in the aerial imagery in the south of the built environment; both of the FBP System-based datasets classify this area as a non-hazardous fuel, but it is represented as a hazardous fuel type by the LCCS product. The difference is due to the source land cover classifications where the FBP System source datasets classify this area as non-fuel because it is part of the built environment, whereas the LCCS production methods classified some pixels in this area as forest.
High Level 3 m Planetscope aerial imagery (right) and hazardous fuels and short-range exposure maps made using LCCS30, FBP30a and COM30a. The extent of the hazard and exposure maps is shown in the red box. The area impacted by the Chuckegg Creek fire is overlaid in orange.
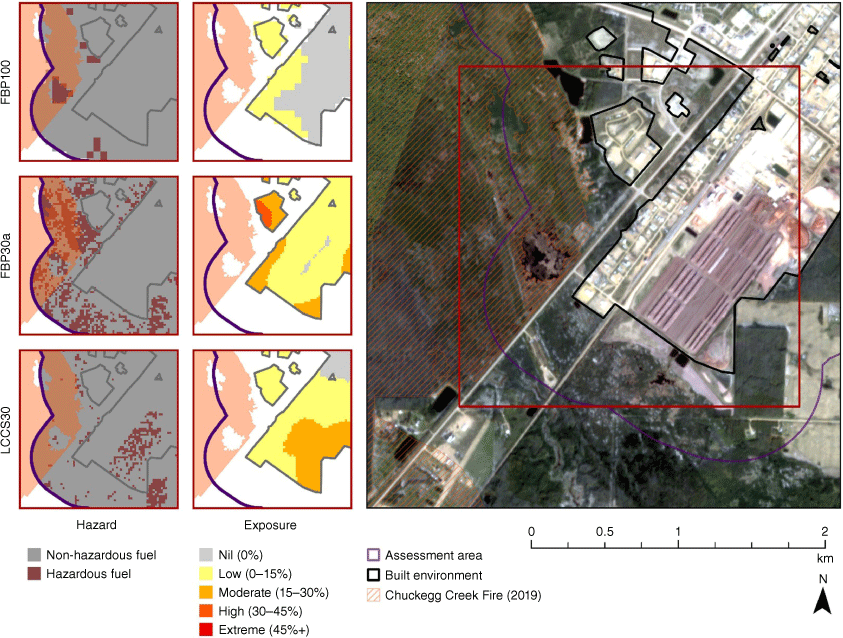
Another area of interest in Fig. 8 is the boundary of the 2019 Chuckegg Creek Fire, which reached a final size of 350,135 ha (MNP 2020). This fire prompted the evacuation of over 3000 residents in the area, and although firefighting efforts kept the fire outside the core community, some rural properties were lost in the surrounding area (MNP 2020). Hazardous fuel reclassification within the post-fire boundary also differs by dataset (Fig. 8). FBP100 produces no hazardous fuels within the boundary of the Chuckegg Creek Fire and aligns well with the unburned island within the assessment area. The wildfire boundary does not appear to have an effect on the FBP30a hazardous fuel reclassifications despite the 2020 temporal currency, whereas the LCCS30 reclassifications show little hazardous fuels both inside and outside of the fire boundary, so it is uncertain if this disturbance was accounted for.
When large datasets are clipped to a smaller study area, they can be more closely inspected to assess the fitness for use for the application, and potentially altered to better fit the requirements. Meeting with local knowledge holders and land managers can also help inform decisions around which fuel types and geographic areas are hazardous or non-hazardous fuels in the context of the study area. The classification of hazardous fuels can also be unique from community to community. For example, local knowledge could aid in classifying the timber storage yard shown in Fig. 8; past experiences in High Level may determine this area as hazardous fuel based on a fire at a different nearby log-deck that prompted the evacuation of 74 rural residents in 2016 (Barton 2016), whereas other communities might consider similar land cover as non-hazardous because forestry mills have fire suppression systems and plans in place to protect against the loss of valuable merchantable timber (Refai 2022).
Time and financial costs must also be considered for fuel data selection (Abdollahi and Yebra 2023). The commissioned datasets used in this case study were costly to purchase and did not perform well for calculating short-range ember and radiant heat exposure values when used without corrective modifications. Once modified, results improved but time and financial costs were increased further. In contrast, when a technician was employed to delineate the fuels surrounding four case study communities by photo interpretation for Beverly et al. (2010) the process took approximately 1 week, and then data were further verified and updated with field visits and local knowledge acquisition. Though more time is invested, the certainty of data quality is vastly increased when the collection, verification and application of the data are done by the same individual or organisation. Both options are ill-advised for large geographical areas as the time and financial costs could be prohibitive. Our results suggest that using readily available datasets can save time and money, but comes with the additional cost of uncertainty. If constrained financially, time can be invested to utilise free datasets and references to refine and verify regional fuel classifications. If constrained by time, proprietary data may be appropriate but data requesters should emphasise the importance of metadata and validation to be completed and supplied by the data producer to decrease uncertainties.
Fuel data are an integral part of many wildfire assessment and modelling methods and the use of remote sensing has increased available options (Chuvieco et al. 2020). Though a crucial component, there is little discussion about the sensitivity of such assessments to the input data used. This may be owing to fuel data options historically being limited, by availability and modelling requirements, that modellers simply used the best (and often only) available option. Standardisation across large management areas and organisations can also be a limiting factor in fuel data selection decisions. Many wildfire modelling applications in Canada rely on the FBP System for fuel type information (e.g. Parisien et al. 2005; Tymstra et al. 2010) so options for readily available datasets are extremely limited. Challenges with FBP System fuel types are known and options for improvements (e.g. Baron et al. 2024) and alternatives (e.g. Phelps and Beverly 2022) have been presented in literature. Drivers of landscape-scale wildfire processes can be modelled using comparatively coarser scales and broadly defined fuel classifications than local assessments. Fuel complexes within WUI zones and communities are atypical relative to landscape scale fuels. Community-scale fuel complexes are often anthropogenically influenced and may not fit within standardised fuel type definitions. A refined spatial resolution is also required to capture the distribution of these atypical fuel complexes because changes in land cover are occurring over shorter distances.
Wildfire exposure methodologies allow for alternative land cover information to be used to represent fuel, which can not only increase options for sourcing data but also for making corrections and improvements. Schmidt et al. (2024) used a machine learning model to improve the chosen remotely sensed land cover information resource to better differentiate conifer species diversity in their study area. In this study, we made manual modifications to add classifiers to the COM datasets. We also updated FBP30 with information from LCCS30 to create a custom integrated dataset. Like all spatial data, land cover information is a simplification of an infinitely complex reality, making error inevitable and unavoidable (Heuvelink 1998; Fisher et al. 2010; Olofsson et al. 2014). Chaotic real-world land cover must be abstracted, generalised and aggregated to fit into pre-defined classification schemes and scales. An understanding of how and why a product was developed can guide decisions on the suitability and potential improvements that can be made to limit uncertainty. There is also value in assessing the agreement between more than one data source to identify areas that could be improved. A logical next step to comparing fuel input layers for localised assessments is the inclusion of a reference dataset that has been validated with local knowledge and ground truthing. Future research into how different input source data affect results for other wildfire assessment methods and geographic areas would be prudent and insightful.
Conclusion
In this study, we sought to evaluate the sensitivity of community-scale wildfire exposure assessments by varying the input land cover products used to represent hazardous fuels. Six land cover products that varied in scale and source were selected to be used as readily made products, four of which were also modified to adjust the classification of grass type land cover within the context of wildfire exposure for an overall total of 10 datasets to compare. We found variation in the distribution and spatial agreement of exposure between datasets and between communities over three different wildfire transmission distances. A large contributing factor was the difference in how land cover was classified between input data, especially the classification of grasses for assessing exposure to short-range ember and radiant heat transmission. The commissioned datasets, the only inputs with an associated financial cost, required further modification to these classifiers. Differences in scale were also a factor. The operational scale of community assessments is more sensitive to input fuel data differences. This study highlights the importance of assessing the fitness for use of any data product before using it for a specific application and within the context of the geographic area. At the operational scale of community assessments, data should be optimised for the area and include unique context and local knowledge of surrounding hazard and values in the community.
For future wildfire exposure assessments, the authors make the following recommendations to reduce uncertainty. A thorough review of the available land cover information products for the area of interest is a good starting point. Consultation with local knowledge holders and wildland fuel experts in the area is also recommended to establish which land cover types are considered hazardous for different scales of wildfire exposure transmission – this will determine what land cover classification schemes can provide effective differentiation. If readily available options are found to be unsuitable, consider modifying or combining them to improve any identified deficiencies. Validation of land cover classification accuracy is also recommended if time and resources permit. There may be further value in assessing exposure with multiple fuel input layers for comparison.
Conflicts of interest
J. L. Beverly is an Associate Editor of International Journal of Wildland Fire. To mitigate this potential conflict of interest, she didn’t have any editor-level access to this paper during peer review. The authors declare no other conflicts of interest.
Declaration of funding
This research was funded by the Forest Resource Improvement Association of Alberta and National Research Council of Canada.
Acknowledgements
We would like to thank Patrick Mahler for their assistance with data compilation and aerial imagery interpretation.
References
Abdollahi A, Yebra M (2023) Forest fuel type classification: review of remote sensing techniques, constraints and future trends. Journal of Environmental Management 342, 118315.
| Crossref | Google Scholar | PubMed |
Ahmed MR, Hassan QK (2023) Occurrence, area burned, and seasonality trends of forest fires in the natural subregions of Alberta over 1959–2021. Fire 6, 96.
| Crossref | Google Scholar |
Allgӧwer B, Carlson JD, van Wagtendonk JW (2003) Introduction to fire danger rating and remote sensing - will remote sensing enhance wildland fire danger rating? In ‘Wildland fire danger estimation and mapping: the role of remote sensing data. Series in remote sensing Vol. 4’. (Ed. E Chuvieco) pp. 1–19. (World Scientific)
Baron JN, Hessburg PF, Parisien M-A, Greene GA, Gergel SE, Daniels LD (2024) Fuel types misrepresent forest structure and composition in interior British Columbia: a way forward. Fire Ecology 20, 15.
| Crossref | Google Scholar | PubMed |
Barton K (2016) Fire at lumber mill near High Level contained, evacuation order lifted. CBC News, 5 May 2016. Available at https://www.cbc.ca/news/canada/north/fire-mill-high-level-evacuation-1.3568131 [verified 15 January 2024]
Beverly JL, Forbes AM (2023) Assessing directional vulnerability to wildfire. Natural Hazards 117, 831-849.
| Crossref | Google Scholar |
Beverly JL, Herd EPK, Conner JCR (2009) Modeling fire susceptibility in west central Alberta, Canada. Forest Ecology and Management 258, 1465-1478.
| Crossref | Google Scholar |
Beverly JL, Bothwell P, Conner JCR, Herd EPK (2010) Assessing the exposure of the built environment to potential ignition sources generated from vegetative fuel. International Journal of Wildland Fire 19, 299-313.
| Crossref | Google Scholar |
Beverly JL, McLoughlin N, Chapman E (2021) A simple metric of landscape fire exposure. Landscape Ecology 36, 785-801.
| Crossref | Google Scholar |
Boychuk D, McFayden CB, Woolford DG, Wotton M, Stacey A, Evens J, Hanes CC, Wheatley M (2021) Considerations for categorizing and visualizing numerical information: a case study of fire occurrence prediction models in the province of Ontario, Canada. Fire 4(3), 50.
| Crossref | Google Scholar |
Cameron HA, Schroeder D, Beverly JL (2021) Predicting black spruce fuel characteristics with Airborne Laser Scanning (ALS). International Journal of Wildland Fire 31, 124-135.
| Crossref | Google Scholar |
Chuvieco E, Aguado I, Salas J, García M, Yebra M, Oliva P (2020) Satellite remote sensing contributions to wildland fire science and management. Current Forestry Reports 6, 81-96.
| Crossref | Google Scholar |
Comber A, Fisher P, Wadsworth R (2004) Integrating land-cover data with different ontologies: identifying change from inconsistency. International Journal of Geographical Information Science 18(7), 691-708.
| Crossref | Google Scholar |
Commission for Environmental Cooperation (2023) 2020 Land Cover of North America at 30 meters metadata. Available at http://www.cec.org/north-american-environmental-atlas/land-cover-30m-2020/ [verified 15 January 2024]
Countryman CM (1972) The fire environment concept. 15 p. (USDA Forest Service, Pacific Southwest Forest and Range Experiment Station: Berkeley, CA). Available at https://www.frames.gov/catalog/8189 [verified 15 January 2024]
Finney MA (2006) An overview of FlamMap fire modeling capabilities. In ‘2006 Fuels Management – How to Measure Success: Conference Proceedings’, 28–30 March 2006, Portland, OR. (Eds PL Andrews, BW Butler) RMRS-P-41, pp. 213–220. (USDA Forest Service, Rocky Mountain Research Station: Fort Collins, CO)
Finney MA, McHugh CW, Grenfell IC, Riley KL, Short KC (2011) A simulation of probabilistic wildfire risk components for the continental United States. Stochastic Environmental Research and Risk Assessment 25, 973-1000.
| Crossref | Google Scholar |
FireSmart Canada (2018) Wildfire exposure assessment. Available at https://firesmartcanada.ca/wp-content/uploads/2022/01/FS_ExposureAssessment_Sept2018-1.pdf [verified 1 July 2024]
Gao Y, Liu L, Zhang X, Chen X, Mi J, Xie S (2020) Consistency analysis and accuracy assessment of three global 30-m land-cover products over the European Union using the LUCAS dataset. Remote Sensing 12(21), 3479.
| Crossref | Google Scholar |
Government of Alberta (2023) Alberta’s wildfire season has come to an end [Press release]. Available at https://www.alberta.ca/release.cfm?xID=89225D8B6B03D-0395-830B-7D29C9D64E07E94D [verified 15 January 2024]
Keane RE, Burgan R, van Wagtendonk J (2001) Mapping wildland fuels for fire management across multiple scales: Integrating remote sensing, GIS, and biophysical modeling. International Journal of Wildland Fire 10, 301-319.
| Crossref | Google Scholar |
Kim AM, Beverly JL, Al Zahid A (2024) Directional analysis of community wildfire evacuation capabilities. Safety Science 171, 106378.
| Crossref | Google Scholar |
Latifovic R, Pouliot D (2005) Multitemporal land cover mapping for Canada: methodology and products. Canadian Journal of Remote Sensing 31(5), 347-363.
| Crossref | Google Scholar |
Latifovic R, Pouliot D, Olthof I (2017) Circa 2010 land cover of Canada: local optimization methodology and product development. Remote Sensing 9(11), 1098.
| Crossref | Google Scholar |
Liu L, Zhang X, Gao Y, Chen X, Shuai X, Mi J (2021) Finer-resolution mapping of global land cover: recent developments, consistency analysis, and prospects. Journal of Remote Sensing 2021, 2589697.
| Crossref | Google Scholar |
Liu P, Pei J, Guo H, Tian H, Fang H, Wang L (2022) Evaluating the accuracy and spatial agreement of five global land cover datasets in the ecologically vulnerable south China karst. Remote Sensing 14(13), 3090.
| Crossref | Google Scholar |
Macauley KAP, Beverly JL, McLoughlin N (2022) Modelling fire perimeter formation in the Canadian Rocky Mountains. Forest Ecology and Management 506, 119958.
| Crossref | Google Scholar |
McFayden CB, Boychuk D, Woolford DG, Wheatley MJ, Johnston L (2019) Impacts of wildland fire effects on resources and assets through expert elicitation to support fire response decisions. International Journal of Wildland Fire 28(11), 885-900.
| Crossref | Google Scholar |
McGee TK (2019) Preparedness and experiences of evacuees from the 2016 Fort McMurray Horse River Wildfire. Fire 2, 13.
| Crossref | Google Scholar |
Microsoft (2019) Microsoft/Canadianbuildingfootprints: Computer generated building footprints for Canada. GitHub. Available at https://github.com/Microsoft/CanadianBuildingFootprints [verified 15 January 2024]
MNP (2020) Spring 2019 Wildfire Review – Final Report. (Edmonton, AB, Canada: MNP LLP) Available at https://open.alberta.ca/publications/spring-2019-wildfire-review [verified 30 October 2024]
Montane Forest Management Ltd. (2016) Town of Hinton Wildfire Mitigation Strategy. Available at https://www.hinton.ca/DocumentCenter/View/612/Town-of-Hinton-FireSmart-Mitigation-Strategy?bidId= [verified 15 January 2024]
Nadeau LB, McRae DJ, Jin J-Z (2005) Development of a national fuel-type map for Canada using fuzzy logic. Information Report NOR-X-406. 26 p. (Natural Resources Canada, Canadian Forest Service, Northern Forestry Centre: Edmonton, AB, Canada) Available at publications.gc.ca/pub?id=9.617622&sl=0 [verified 15 January 2024]
Olofsson P, Foody GM, Herold M, Stehman SV, Woodcock CE, Wulder MA (2014) Good practices for estimating area and assessing accuracy of land change. Remote Sensing of Environment 148, 42-57.
| Crossref | Google Scholar |
Parisien M-A, Parks SA, Miller C, Krawchuk MA, Heathcott M, Moritz MA (2011) Contributions of ignitions, fuels, and weather to the spatial patterns of burn probability of a boreal landscape. Ecosystems 14, 1141-1155.
| Crossref | Google Scholar |
Parks SA, Parisien M-A, Miller C (2011) Multi-scale evaluation of the environmental controls on burn probability in a southern Sierra Nevada landscape. International Journal of Wildland Fire 20, 815-828.
| Crossref | Google Scholar |
Phelps N, Beverly JL (2022) Classification of forest fuels in selected fire-prone ecosystems of Alberta, Canada – implications for crown fire behaviour prediction and fuel management. Annals of Forest Science 79, 40.
| Crossref | Google Scholar |
Phelps N, Cameron H, Forbes AM, Schiks T, Schroeder D, Beverly JL (2022) The Alberta Wildland Fuels Inventory Program (AWFIP): data description and reference tables. Annals of Forest Science 79, 28.
| Crossref | Google Scholar |
Refai R (2022) Log-deck fire suppression using high volume water delivery systems. FPInnovations. Project Number 301014968. Available at https://library.fpinnovations.ca/media/FOP/TR2022N3.PDF [verified 15 January 2024]
Sayre NF (2005) Ecological and geographical scale: parallels and potential for integration. Progress in Human Geography 29(3), 276-290.
| Crossref | Google Scholar |
Schmidt JI, Ziel RH, Calef MP, Varvak A (2024) Spatial distribution of wildfire threat in the far north: exposure assessment in boreal communities. Natural Hazards 120, 4901-4924.
| Crossref | Google Scholar |
Scott JH, Burgan RE (2005) Standard fire behavior fuel models: a comprehensive set for use with Rothermel’s surface fire spread model. RMRS-GTR-153. (USDA Forest Service, Rocky Mountain Research Station: Ft Collins, CO) 10.2737/RMRS-GTR-153
Tymstra C, Wang D, Rogeau M-P (2005) ‘Alberta wildfire regime analysis.’ (Alberta Sustainable Resource Development, Forest Protection Division, Wildfire Policy and Business Planning Branch: Edmonton, AB) Available at http://archive.org/details/albertawildfirer00tyms_0
USDA USDI (2001) Notices. Federal Register. 751–777 pp. Available at https://www.federalregister.gov/articles/2001/01/04/01-52/urban-wildland-interface-communities-within-the-vicinity-of-federal-lands-that-are-at-high-risk-from [verified 15 January 2024]
Wulder MA, Coops NC, Roy DP, White JC, Hermosilla T (2018) Land Cover 2.0. International Journal of Remote Sensing 39, 4254-4284.
| Crossref | Google Scholar |