Australian Fire Danger Rating System: implementing fire behaviour calculations to forecast fire danger in a research prototype†
B. J. Kenny A C , S. Matthews A D , S. Sauvage B , S. Grootemaat A E , J. J. Hollis A F * and P. Fox-Hughes
A
B
C Present address:
D Present address:
E Present address:
F Present address:
Abstract
The Australian Fire Danger Rating System (AFDRS) was implemented operationally throughout Australia in September 2022, providing calculation of fire danger forecasts based on peer-reviewed fire behaviour models. The system is modular and allows for ongoing incorporation of new scientific research and improved datasets.
Prior to operational implementation of the AFDRS, a Research Prototype (AFDRSRP), described here, was built to test the input data and systems and evaluate the performance and potential outputs.
Fire spread models were selected and aligned with fuel types in a process that captured bioregional variation in fuel characteristics. National spatial datasets were created to identify fuel types and fire history in alignment with existing spatial weather forecast layers.
The AFDRSRP demonstrated improvements over the McArthur Forest and Grass Fire Danger systems due to its use of improved fire behaviour models, as well as more accurately reflecting the variation in fuels.
The system design was robust and allowed for the incorporation of updates to the models and datasets prior to implementation of the AFDRS.
Keywords: AFDRS, Australian Fire Danger Rating System, fire behaviour calculations, fire behaviour models, fuel attributes, fuel classification, fuel type map, interactive forecast display, research prototype.
Introduction
The five articles that comprise this Special Section of the International Journal of Wildland Fire introduce the Australian Fire Danger Rating System (AFDRS):
Article 1 (Hollis et al. 2024b) introduces the AFDRS and provides a short history of fire danger in Australia together with requirements to make advancements;
Article 2 (Hollis et al. 2024a) identifies and presents a framework for identification and categorisation of fire danger;
Article 3 (this paper, Kenny et al. 2024) describes a system for implementing fire danger calculations to forecast fire danger;
Article 4 (Grootemaat et al. 2024) evaluate the performance of the AFDRS Research Prototype during a live trial in the 2017–18 southern Australian fire season; and
Article 5 (Sauvage et al. 2024) presents a climatology of the AFDRS Research Prototype.
Background
Fire danger ‘is an expression of probable fire behaviour in relation to a particular set of fuel and weather conditions’ (McArthur 1977). Fire danger rating (FDR) expresses fire danger in descriptive categories (e.g. low to extreme) indicating levels for appropriate actions. FDR is an important tool for fire management, driving fire and land management agency preparedness (including suppression, ignition management and resource allocation) and communicating risk to the community (Taylor and Alexander 2006). The AFDRS program included the development of a Research Prototype platform to demonstrate the feasibility of developing a fire danger forecasting system based on contemporary fire behaviour models that was national, modular and open to continuous improvement.
Prior to 2022, the operational fire danger forecasting system within Australia used the Forest and Grass Fire Danger Indexes (FFDI and GFDI) derived from the fire behaviour models of McArthur and Dwyer (1958), McArthur (1960). Identified limitations in the McArthur system, such as the inability to incorporate contemporary fire behaviour knowledge (e.g. Cruz et al. 2015a) without changing the way users interacted with the system, complex weather effects such as stability (e.g. Dowdy and Pepler 2018) and local fuel variation (e.g. Duff et al. 2013) led to the development of the Australian Fire Danger Rating System: Research Prototype (AFDRSRP) (Hollis et al. 2024b). This formed part of a broader program to introduce the AFDRS as the operational fire danger rating system nationally in Australia. Within this system, individual fire behaviour models could be implemented then retired as scientific progress occurred.
Since the pioneering work of McArthur in fire behaviour and fire danger, new fire spread models have been developed for both grassland (Cheney et al. 1998) and dry sclerophyll forests (Cheney et al. 2012). Additional models have been developed for other vegetation types: temperate shrublands (Anderson et al. 2015); semi-arid mallee-heath shrublands (Cruz et al. 2013); spinifex hummock grasslands (Burrows et al. 2018); buttongrass moorlands (Marsden-Smedley and Catchpole 1995a); and pine plantations (Cruz et al. 2008). These models cover the major vegetation types in Australia, but gaps remain in the knowledge of fire behaviour in less fire-prone vegetation types (e.g. rainforests, arid shrublands, wetlands and alpine herblands) and vegetation in disturbed and developed areas (e.g. crops, invasive weeds, agricultural/rural landscapes, urban areas) (Cruz et al. 2015a; Plucinski et al. 2017; Cruz et al. 2018).
Implementing spatially explicit fire management systems (e.g. fire danger ratings, fire spread simulation) requires full spatial coverage of all elements (e.g. fuel types, fire behaviour calculations, environmental data, weather forecasts) (Loveland 2001; Taylor and Alexander 2006). The lack of fire behaviour models for some vegetation types needs to be accounted for, e.g. by the application or modification of an existing model based on similarity of fuel types (Plucinski et al. 2017).
Fuel types are used to group and map vegetation in a manner relevant to fire management (Keane et al. 2001; Keane 2013; Cruz et al. 2018). In Australia, operational fuel type mapping is usually based on existing vegetation and land use mapping (Tolhurst et al. 2013; Lynch et al. 2015; Kenny and Roberts 2016); however, there is a lack of consistency in fuel type classification and the level of fuel attribute detail documented between jurisdictions. The Australian Bushfire Fuel Classification (BFC) was proposed to provide this consistency at a national scale (Hollis et al. 2015; Cruz et al. 2018) but had not been implemented at the time of AFDRSRP development.
Vegetation classification and mapping itself has variable purposes and focus, e.g. structure, floristics, climatic zone, bioregion or conservation status (Groves 1981; Specht and Specht 1999). From a fuels and fire behaviour perspective, the vegetation stratum that will carry the fire (such as surface litter, grasses or understorey plants) is the most important factor (Sullivan et al. 2012); therefore, structural vegetation classification is the most useful for broad fuel type classification (Lynch et al. 2015). However, additional information can be gained from other aspects of vegetation mapping. For example, climatic and bioregional variation influence ecosystem productivity (and hence fuel condition and dynamics) within broad vegetation types (Walker 1981; Watson 2009; Duff et al. 2013). Additionally, floristic information can provide data on some specific fuel attributes, e.g. bark type (Horsey and Watson 2012). These details help to define local fuel types that have the same broad fuel structure (i.e. use the same fire behaviour model) but will have different fuel attributes (e.g. height, bark, fuel load) that drive specific fire behaviour (e.g. flame height, spotting distance, intensity).
Aims
A Fire Behaviour Index (FBI – see description below in the ‘Fire Behaviour Outputs’ section), together with fire danger ratings, were computed daily by the Bureau of Meteorology (the Bureau) at hourly intervals on a 1.5 × 1.5 km resolution grid across Australia using gridded forecast weather. The 6 × 6 km gridded weather data were interpolated to the 1.5 km FBI grid. Each grid cell was assigned a fuel type that allowed the selection of the appropriate fire behaviour model and provided fuel attribute details. Weather and fuel inputs were then used to calculate fire behaviour metrics (rate of spread, intensity, flame height and spotting distance) that were classified into rating categories (Hollis et al. 2024a).
A live trial using data collected between October 2017 and March 2018 was conducted to test the performance of the system (Grootemaat et al. 2024). Live trial output was made available to fire agency staff via a specifically developed website for assessment. Given that the output was developmental during the live trial, it was not made available to the public at that time, to avoid any potential for confusion.
Methods
We describe the construction of the AFDRS Research Prototype following the system architecture as implemented in the prototype, i.e. fuel state, fire danger calculations, then visualisation.
Fuel types
For the purpose of the AFDRSRP, fuel type classification was driven by the need to select an appropriate fire behaviour model and to capture the range of variation in fuel characteristics that provide the required fuel attributes and parameters for the fire behaviour models. Because the BFC had not been implemented when developing the AFDRSRP, the lack of commonality between jurisdictional fuel classifications drove development of an AFDRSRP fuel type classification that would fit this purpose, using three tiers of increasing detail in fuel information within the AFDRSRP:
Broad fuel type | Fire behaviour model | AFDRSRP fuel type | Fuel type description | Limitations | Model application | |
---|---|---|---|---|---|---|
Forest | Dry Eucalypt Forest Fire Model (DEFFM); Cheney et al. (2012) | Forest | Dry eucalypt forest and temperate woodland with a shrubby understorey and litter surface fuel | n/a | Fuel availability function applied (Eqn 1) | |
Wet forest | Forests with high moisture content due to structure (closed forest cover >70%, tall forest >30 m), topography or inundation, e.g. rainforest, wet sclerophyll forest, swamp forest | Fuel availability limited by moisture content | Fuel availability function applied (Eqn 2) | |||
Grassland | CSIRO Grassland fire spread meter; Cheney et al. (1998) | Grass | Continuous and tussock grasslands | n/a | Cruz et al. (2015b) curing function; fuel condition from reported grass fuel load | |
Pasture | Modified or native pasture where primary land use is grazing | Fuel availability variable with management | ||||
Crop | Non-irrigated cropping land (cereals, hay, sugar, etc.) | |||||
Low wetland | Wetland with low or no overstorey, e.g. low swamp heath, sedgeland, rushland | Fuel availability limited by moisture content | Cruz et al. (2015b) curing function; fuel condition set to eaten-out | |||
Chenopod shrubland | Low arid shrublands dominated by chenopod (saltbush) species, or similar non-arid vegetation with samphire species. Limited flammability except when high cover of ephemeral grasses | Fuel connectivity limited and variable with ephemeral grass growth | ||||
Northern grassland (savanna) | CSIRO Grassland northern Australia; Cheney et al. (1998), Cheney and Sullivan (2008) | Woodland | Woodland and shrubland with a continuous grass understorey (minimal shrub or litter component), e.g. tropical savanna woodland, temperate grassy woodlands, semi-arid woodlands or shrublands with a perennial grass understorey | n/a | Cruz et al. (2015b) curing function; fuel condition from reported grass fuel load | |
Gamba | Grassland, woodland or rural area invaded by gamba grass (Andropogon gayanus Kunth.) or similar high fuel load invasive grass | Higher than standard grass fuel loads | Cruz et al. (2015b) curing function; fuel load applied via attribute table | |||
Arid woodland | Arid/semi-arid woodland or shrubland with an ephemeral grass understorey; fuel connectivity only when grass cover occurs after sufficient rain. Mostly Acacia dominated (e.g. Mulga) but also includes Eucalyptus, Casuarina, Callitris, etc. | Fuel connectivity limited and variable with ephemeral grass growth | Cruz et al. (2015b) curing function; fuel condition set to eaten-out | |||
Woody horticulture | Perennial woody horticulture, likely managed (mown, irrigated) grass understorey, e.g. orchards, vineyards | Fuel availability variable with management | ||||
Rural | Rural residential areas. Typically continuous grass with variable tree cover. Note fuel management (e.g. grazing, mowing) may be highly variable | Fuel availability variable with management | Cruz et al. (2015b) curing function; fuel condition set to grazed | |||
Urban | Urban residential areas with grass or garden and variable tree cover. Includes suburbs with tree cover, recreation areas within urban areas (e.g. parks, golf courses). Note fuel cover and management may be highly variable | Fuel availability variable with management | Cruz et al. (2015b) curing function; fuel condition set to eaten-out | |||
Spinifex | Spinifex grassland model; Burrows et al. (2018) | Spinifex | Spinifex hummock grassland | n/a | Modelled soil moisture (AWRA) | |
Spinifex woodland | Woodland and shrubland with a hummock grass (spinifex) understorey. Note: includes vegetation described as mallee if the understorey is spinifex | Overstorey presence reduces wind penetration | Modelled soil moisture (AWRA); wind factor applied via attribute table | |||
Mallee-heath | Semi-arid mallee-heath model; Cruz et al. (2013) | Mallee-heath | Semi-arid mallee-heath woodland and shrubland, specifically with a shrubby understorey. Note that mallee with a spinifex understorey is included in Spinifex woodland; mallee with a chenopod or ephemeral grass understorey is included in Arid woodland | n/a | Fuel availability function applied Marsden-Smedley et al. (1999) | |
Shrubland | Temperate shrubland model; Anderson et al. (2015) | Heath | Temperate shrublands. Primarily heathland; may also include tall closed shrubland, low closed forest or open woodland with heath understorey where the structure is dominated by a single shrub layer (cf. multiple strata of forest). Note that arid and semi-arid shrublands are included in other fuel types (e.g. mallee-heath, arid woodland, spinifex woodland, chenopod shrubland) | n/a | Fuel availability function applied Marsden-Smedley et al. (1999) | |
Wet heath | Wetlands with a medium to tall shrubland structure, e.g. swamp heath, melaleuca shrubland. Note that buttongrass moorlands of Tasmania have a separate fuel type | Fuel availability limited by moisture content | ||||
Buttongrass | Buttongrass moorlands model; Marsden-Smedley and Catchpole (1995a) | Buttongrass | Buttongrass moorland of Tasmania: largely treeless sedgeland/low heath containing the hummock-forming tussock sedge Gymnoschoenus sphaerocephalus (R.Br.) Hook.f. | n/a | Fuel availability function applied Marsden-Smedley et al. (1999) | |
Pine | Adjusted Cruz et al. (2008) Pine model; M. Cruz, pers. comm. | Pine | Pine plantation | n/a | Fuel availability function applied (Eqns 3 and 4) | |
Non-combustible | n/a | Horticulture | Seasonal horticulture, very low flammability, e.g. vegetables, herbs and irrigated crops | Minimal flammable material | Nil fire behaviour calculations | |
Built up | Non-combustible urban areas and intensive land use, e.g. business districts, industrial areas, infrastructure, mining | |||||
Non-combustible | Non-combustible areas of water, sand, rock, etc. Includes saline wetlands |
Broad fuel types are defined by fire spread models (Table 1; Cruz et al. 2015a). At this level, fuel types reflect broad vegetation structure in a way that indicates the primary fuel strata for fire propagation and closely resembles the fuel types implemented in the CSIRO-developed fire behaviour decision support system Amicus (Plucinski et al. 2017).
Many vegetation types don’t have a specifically developed fire behaviour model (e.g. rainforests, arid shrublands, wetlands, rural and urban areas); therefore, these have been allocated to the fire spread model with the most similar fuel structure. However, there are often factors (broadly represented by climatic variation or human management) limiting the flammability, fuel availability or fuel connectivity in these vegetation types (Plucinski et al. 2017). Thus, some modifications (e.g. fuel availability function, wind factor, grass condition) must be made to the fire behaviour calculations to reflect these limitations. These are documented in the ‘Model Application’ column in Table 1 and described in the ‘Fire Spread Models’ subsection above.
At the most detailed level, the definition of local fuel types describes the spatial variation in fuel attributes and parameters (e.g. bioregional and floristic influences) and allows for the use and adaptation of existing agency fuel maps and fuel datasets.
Fuel characteristics and temporal fuel state variation
The fundamental physical characteristics of fuel particles and fuelbeds (fuel attribute; Hollis et al. 2015), as well as the characteristics required as direct input into existing fire behaviour models (fuel parameter; Hollis et al. 2015), were collated to enable calculation of rate of spread, flame height and intensity in the various fire behaviour models (Supplementary Table S2). Examples of the fuel attributes include fuel hazard score and fuel height. Most fuel attributes were recorded per local fuel type in a look up table, and a few were applied directly in the model code (e.g. grass condition, fuel load equations for spinifex and buttongrass).
Fuel attribute tables were primarily collated from operational fuel tables for each jurisdiction, as well as additional published values. Where there was no known value for a required fuel attribute, data were converted from another format (e.g. from fuel hazard rating (McCarthy et al. 1999; Hines et al. 2010) to DEFFM fuel hazard score (Gould et al. 2007b) or to fuel load), or generic values were applied (e.g. values from the published fire behaviour models).
Temporal fuel state data were acquired from additional spatial layers (grass curing, reported grass fuel load and time since last fire). Grass curing and grass fuel load are existing ADFD layers (provided by agencies to the Bureau) used in GFDI calculation and were used in the AFDRSRP to vary fuel availability, grass condition and fuel load (Table 1). A time since fire spatial layer was created from jurisdictional fire history data to allocate certain fuel attributes (fuel load, fuel hazard scores and near-surface height) in recently burnt areas. Fuel load was estimated using the time since fire layer and the fuel accumulation curve of Olson (1963), with fuel curve parameters (maximum and constant) either in the fuel attribute table (forest, mallee-heath, shrubland) or built into the model code (spinifex and buttongrass).
Fire spread models
Eight models of rate of forward spread were used, as recommended by Cruz et al. (2015a), including those specific to forest (Cheney et al. 2012), grassland (McArthur 1973), spinifex (Burrows et al. 2017), pine (Cruz et al. 2008), northern grassland (savanna woodland) (Cheney and Sullivan 2008), mallee-heath (Cruz et al. 2013), shrubland (Anderson et al. 2015) and buttongrass (Marsden-Smedley and Catchpole 1995a) fuel types. Table 1 lists the fire spread models used within the AFDRSRP. Most models were implemented as recommended by (Cruz et al. 2015a), with the addition of fuel availability modifiers where these were lacking, as described below and in Table 1.
Dead fuel moisture is an important determinant of the potential for fires to start and spread (Matthews 2014). Two main approaches have been used for including the effect of rainfall on operational fire spread models: (1) increasing moisture content above the fibre saturation point, e.g. Marsden-Smedley and Catchpole (1995b); or (2) reducing the amount of fuel available to burn, e.g. the McArthur drought factor (Noble et al. 1980). Below, we describe the approaches used to evaluate fuel availability within the models used in the AFDRSRP. Note that observed values of grass curing were used in grassland calculations, as occurred in operational fire weather forecasts.
The Dry Eucalypt Forest Fire Model (DEFFM: Cheney et al. 2012) assumes dry summer conditions and as such, does not include fuel availability or rainfall effects in its fuel moisture models. The DEFFM does not specify recommendations for treatment of these effects on either fuel moisture or fuel quantity. The process-based fuel moisture model of Matthews (2006) was considered too complex to implement within the AFDRSRP. Instead, simple fuel availability curves were implemented as functions of drought factor, loosely based on fire occurrence observations presented by (Cawson et al. 2017). Fuel quantity values (fuel hazard scores (Gould et al. 2007b) and fuel loads) were multiplied by the fuel availability factor
For dry forests:
For wet forests, a logistic function was used:
where Fuel_availability is the fraction of fuel available for combustion and DF is the drought factor, calculated by the Bureau of Meteorology at the same resolution as the weather parameters (6 × 6 km, downscaled to 1.5 × 1.5 km), using the method of Griffiths (1999). A different fuel availability function was developed for wet forests in order to account for the more limited fuel availability in these forest types compared with the dry forests for which the DEFFM model had been developed (Matthews et al. 2018).
Similar to the DEFFM, fuel availability and rainfall effects are not accounted for in the Cruz et al. (2013) mallee-heath and Anderson et al. (2015) shrubland models. Because these fuel types are expected to become flammable more rapidly after antecedent rainfall than a forest fuel type, we applied the Marsden-Smedley et al. (1999) fuel moisture modifier function, originally developed for buttongrass fuels, to derive fuel availability for the mallee-heath and shrublands models. Although these fuel types are structurally dissimilar and occur in different climatological zones, the buttongrass fuel moisture modification function has a response time of 1–2 days, making it suitable for fuel types with a large near-surface and elevated fuel component. We note that very little rainfall is required for fire to be extinguished in buttongrass moorland fuel, a characteristic which may not be ideally suited to mallee-heath and shrubland fuels.
For pine plantation, the recommended Cruz et al. (2008) fire behaviour model and fine fuel moisture code (Van Wagner 1987) were considered too complex to implement in the AFDRSRP. Instead, we used a simplified fire behaviour model originally developed for use with the Commonwealth Scientific and Industrial Research Organisation (CSIRO)-developed SPARK fire spread simulation software (Hilton et al. 2015; Miller et al. 2015), with the following functions to modify dead fuel amount and foliar moisture content (M. Cruz pers. comm.):
where DF is the drought factor.
Fuel type modifications to fire behaviour model application
The CSIRO grassland models (Olson 1963; Cheney et al. 1998) have separate rate of spread equations depending on grass condition (natural/undisturbed, grazed/cut, eaten-out). Australian grass fuel reporting protocols record fuel load and curing only, so reported fuel load was used as a proxy for condition. Ideally, information on grass condition would be used in these calculations. The use of load instead is a pragmatic decision, and does not suggest that the two quantities are coupled in the model. For the standard grassland fuel types (grass, woodland), the reported grass fuel load layer was used to select the grass condition (where fuel load of 3 t ha−1 or less was set to eaten-out; between 3 and 6 t ha−1 was set to grazed; 6 t ha−1 and above was set to natural). For fuel types where the grass fuel is limited by ephemeral grass growth (chenopod shrubland, arid woodland), inundation (low wetland) or human management (pasture, crop, rural, urban, woody horticulture), the grass condition was set by fuel type (reported grass fuel for pasture and crop; grazed for rural; eaten out for all others; see Table 1). For all grassland and northern grassland fuel types, fireline intensity was calculated using the reported grass fuel load.
For the spinifex model, a wind factor was added as per the northern Australia grassland model (Cheney and Sullivan 2008), to allow for the effect of overstorey cover on the 2 m wind speed. This was implemented using the fuel attribute tables, where the wind factor for spinifex was set to 1, whereas the wind factor for spinifex woodland was a value less than 1 depending on the level of overstorey cover. Note that as specified in Table 1, spinifex woodland is a distinct fuel type from other woodland types or mallee-heath.
Weather data
Weather parameters (such as wind speed, air temperature and relative humidity) are primary inputs to all of the fire behaviour models. Most weather parameters (Supplementary Table S1) were acquired from the Australian Digital Forecast Database (ADFD: Bureau of Meteorology 2023b).
The Wind Change Danger Index was calculated from ADFD layers (surface wind gust strength, wind direction) using the algorithm of Huang and Mills (2006).
The soil moisture input required to calculate spinifex fuel availability came from the Australian Water Resource Assessment (AWRA) (Frost et al. 2018) data available through the Bureau.
Spatial data processing
For the purpose of fire behaviour calculations, the ~6 km resolution of the ADFD weather forecast grids was considered too broad to capture the spatial variability in fuel type and fire history data, and the native resolution of the original data (30–250 m) was too fine for efficiently processing in the forecast calculation system. Therefore, a ~1.5 km grid was built to nest within the ADFD grids. The fuel type and fire history spatial data were post-processed to this AFDRSRP resolution. Spatial analyses were performed on the input data to produce layers of dominant fuel type (the local fuel type with the maximum area coverage within a grid cell) and average time since fire.
Forecast calculation system
A calculation system was built and run by the Bureau to take the fuel and daily weather forecast inputs and run the various fire behaviour model calculations to produce a range of fire danger outputs. Calculations ran within the Bureau’s development environment, entirely separate from operational forecasting systems. The FDR was calculated per grid cell and summarised at the Fire Weather District (Bureau of Meteorology 2023a) scale as the highest rating with at least 10% coverage of the Fire Weather District (i.e. 90th percentile). Calculations were primarily performed using the Python programming language using the NumPy (van der Walt et al. 2011), Pandas (McKinney 2011) and xarray (Hoyer and Hamman 2017) libraries to efficiently perform scientific calculations using the large meteorological datasets required. A Jenkins (https://jenkins.io) automation server was used to control data processing tasks.
To support the ability of the system to include continuous improvement by allowing the safe inclusion of changes to the model code, Git (Blischak et al. 2016) was used for version control, and all code updates were tested using the pytest framework (Okken 2017) through GitLab’s (https://gitlab.com/) continuous integration tools. These tests were conducted prior to deployment.
The system collected ADFD forecast data and AWRA soil moisture data at 0200 hours Australian Eastern Daylight Time (AEDT) daily. The data for each jurisdiction were unified by transforming them onto a common grid at hourly intervals. At 0500 hours AEDT, the unified data were then passed through the fire behaviour model calculations for the relevant fuel types for each jurisdiction. Computations were performed in parallel, with a maximum calculation time of 15 min. Results were stored and published as netCDF grids (Rew and Davis 1990) through existing Bureau data delivery infrastructure. Summary products such as area rating tables and images of daily maximum rating values were also produced.
Red flag warnings
Not all weather (or weather-related) quantities that might affect the occurrence or spread of a fire were amenable to direct inclusion in fire spread model calculation (and therefore of fire danger in the AFDRSRP). For this reason, fire agency managers wished to have additional red flag warnings calculated, to identify conditions where fire danger was likely to be increased by a wind change, atmospheric instability or long-distance spotting. A Daily Wind Change Danger Index (Huang and Mills 2006) was included together with a value for the 90th percentile daily maximum spotting distance (Gould et al. 2007a) and maximum CHaines (Dowdy and Pepler 2018) above the 95th percentile climatological value displayed in the output websites. These red flags are described in more detail by Hollis et al. (2024b). The Red flag warnings did not modify the rating categories, but are noted here because they formed part of the implemented AFDRSRP.
Fire behaviour outputs
All of the fire spread models used within the AFDRSRP use a combination of weather parameters and fuel characteristics, including fuel moisture to calculate rate of spread. Some models also produce flame height/length and spotting outputs.
Fireline intensity was calculated from rate of spread and fuel load using the Byram (1959) fireline intensity equation, assuming a heat yield of 18 600 kJ kg−1 (Albini 1976) for all fuel types except buttongrass and spinifex, where 19 900 kJ kg−1 (Marsden-Smedley and Catchpole 1995b) and 16 700 kJ kg−1 (M. Possell, pers. comm.) were used respectively. Total fuel load was modified by the fuel availability (e.g. as in Eqn 3) and was then used to calculate fireline intensity for most fuel types, but fuel load of different strata was added incrementally for forest (based on flame height) and mallee-heath (based on probability of crown fire).
A Fire Behaviour Index (FBI) was determined using a linear interpolation based on the fire behaviour metric (e.g. rate of spread, fireline intensity) and the table of Fire Behaviour Index values and intensity thresholds (Hollis et al. 2024a). From this, a forecast was produced that included a Fire Behaviour Index as well as Fire Danger Rating categories (FDR) (Hollis et al. 2024a).
Website outputs
An interactive website was produced to display the hourly forecasts of the Fire Behaviour Index and Fire Danger Rating, including the input weather forecasts and the output fire behaviour calculations (Supplementary Table S3). The main features of the website include: an interactive map display of FBI (Supplementary Fig. S1a) and other variables for the current and previous days at hourly intervals; the ability to view and download time series graphs for all variables at any location; and incident markers and information from agency incident feeds.
All jurisdictions in Australia publish a live, web-based feed of active, going fire incidents. These data were displayed on the interactive website and stored in a database for analysis of reported incidents during the AFDRSRP live trial.
A daily summary website was produced to display the daily FBI calculations and associated FDR and delivered using the Bureau’s registered-user’s websites. A national map of daily maximum FDR by Fire Weather District was generated (Supplementary Fig. S1b), as well as state-based maps of daily maximum fire danger rating and index displayed on both the AFDRSRP grid and by Fire Weather District. Tables were generated summarising daily maximum FDR per Fire Weather District and displaying the red flag warnings for wind change, instability and spotting. Additional weather demonstration products (weather products not yet fully operational, but with potential for future incorporation into the AFDRS) were presented, including a link to JASMIN (Dharssi and Vinodkumar 2017) soil moisture data and images of pyrocumulonimbus and dry lightning potential (produced by the Bureau Extreme Weather Desk).
Re-calculation system
The forecast calculation system was built in a modular structure to allow changes as the underlying scientific knowledge improves. However, each module or node was sufficiently complex that the effect of changes to model code on model performance may not have been immediately obvious. To ensure the effect of changes are clear and can be reproduced in a transparent way, we developed a system for re-calculating and evaluating the performance of the AFDRSRP models. The re-calculation system was built as a self-contained virtual machine using an Amazon Web Services EC2 instance (https://aws.amazon.com/ec2/). This allowed the system to be duplicated if required and allowed snapshots of the system at various points in time to be archived. The same Python modules that are used in the forecast calculation system are also used in the re-calculation system, ensuring consistency between both systems (with code changes tracked using the Git version control system). The system included a set of interactive Jupyter (Kluyver et al. 2016) notebooks for comparing the performance of the AFDRSRP among versions to ensure that changes improve predictive skill before they are incorporated into the operational forecast system.
Results and discussion
The AFDRSRP successfully demonstrated that it is possible to build a fire danger rating system that is national, modular and open to continuous improvement. The requirements for an improved fire danger rating system are outlined by Hollis et al. (2024b). Some principles relating to these requirements are discussed here, with further details covered by Grootemaat et al. (2024) and Hollis et al. (2024a).
System performance
The forecast calculation system and the two output websites were fully functional and available to users around Australia during the period of the live trial and remained functional for ongoing evaluation up to implementation in September 2022. Minor outages occurred, but automated error checking and alerts enabled rapid identification of calculation or display errors. As detailed in the accompanying ‘Live Trial’ article in this series describing the AFDRSRP, the overall performance of the AFDRSRP was superior to the McArthur system, with a tendency to over-predict rather than under-predict fire danger potential (Grootemaat et al. 2024).
An example of an hourly AFDRSRP rating for a day with elevated fire danger (4 January 2019 at 1400 hours) on the AFDRSRP forecast grid (interactive website output) is included in Supplementary Fig. S1a. The daily maximum FDR for the same day by Fire Weather District (summary website output) is also provided in Supplementary Fig. S1b.
Fuels
A major improvement that the AFDRSRP introduced was the use of fire behaviour models for a wider range of fuel types and the use of detailed fuel attributes allowing for regional variation. The McArthur derived Forest Fire Danger Index (FFDI; McArthur and Dwyer 1958) and Grass Fire Danger Index (GFDI; McArthur 1960) were used to determine fire danger ratings within Australia, so all vegetation was classified as either forest or grass. The calculation of GFDI incorporates variation in grass fuel state through reported grass curing and grass fuel load, but FFDI calculation assumes forest fuels to be static both spatially and temporally.
The mapping of forest fuel types between the McArthur systems and the AFDRSRP is roughly equivalent (Fig. 1 and Supplementary Fig. S2). Although forest only covers a small portion of Australia, it coincides with the areas of greatest population (including around Australia’s three biggest cities: Sydney, Melbourne and Brisbane), and forest is the fuel type most likely to impact on residential areas. This human influence is seen in the discrepancy between overall area covered (6%), reported incidents (20%) and the distribution of forest within Fire Weather Districts (Table 2). Forest is the dominant fuel type (greatest area coverage) in 18% of Fire Weather Districts, and significant (at least 10% area coverage, i.e. enough to set the fire danger rating) in 38% of Fire Weather Districts. Note that Fire Weather Districts are variable in size, generally reflecting population density.
Comparison of fuel type coverage between AFDRSRP and GFDI/FFDI, shown as both percentage of national area covered and percentage of Fire Weather Districts with dominant fuel type. Black, grassland; diagonal lines, northern grassland (AFDRSRP only); grey, forest; horizontal lines, other AFDRSRP fuel types (see Table 2 for detailed breakdown); light grey, combined (where both GFDI and FFDI are calculated).
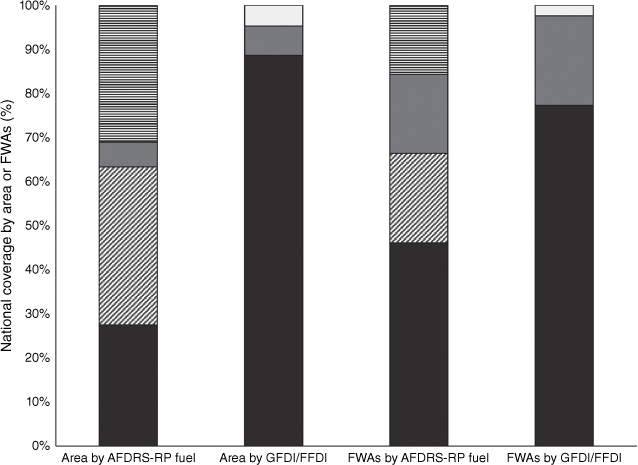
Broad fuel type | Area covered (% national) | Dominates FWD (% national) | Significant in FWD (% national) | Reported incidents (%) | Evaluations live trial + case studies (%) | |
---|---|---|---|---|---|---|
Forest | 5.5 | 18.0 | 38.3 | 20.2 | 55.7 | |
Grassland | 27.5 | 46.1 | 75.8 | 33.2 | 22.9 | |
Northern grassland (savanna) | 35.9 | 20.3 | 50.8 | 37.0 | 5.4 | |
Spinifex | 25.7 | 12.5 | 28.9 | 1.7 | 3.0 | |
Mallee-heath | 2.2 | 1.6 | 14.1 | 0.6 | 4.5 | |
Shrubland | 1.0 | 1.6 | 7.8 | 0.9 | 5.4 | |
Buttongrass (% Tasmania) | 0.1 (10) | 0.0 (0.0) | 0.8 (9.1) | 0.01 (0.3) | 1.8 | |
Pine | 0.2 | 0.0 | 0.0 | 0.6 | 1.5 | |
Non-combustible | 1.8 | 0.0 | 0.0 | 5.7 | 0.0 |
Fuel type coverage is given as a percentage of the total national area (calculated from the AFDRSRP 1.5 km grid) and by Fire Weather District. Dominates Fire Weather District (FWD) means the fuel type with greatest area coverage of an FWD; 'significant' in an FWD means that a fuel type has at least 10% coverage within an FWD (i.e. could set the fire danger rating) (note that multiple fuel types may meet this criterion per FWD, hence the total of this column exceeding 100). Incidents reported through fire agency incident feeds (n = 31 945) and evaluations received (n = 336) (Grootemaat et al. 2024) during the live trial (October 2017–March 2018) are shown by percentage per fuel type.
Bioregional variation and disturbance (e.g. fire history), which have a significant influence on the distribution and condition of forest fuels (Duff et al. 2013), are not accounted for in the FFDI. The influence of variable fuel attributes was seen in the AFDRSRP calculated fire behaviour (and hence forecast FBI and FDR), both between different forest fuel types and within a fuel type as fire history changed between sites (example shown in Fig. 2).
Variation in fuel load and AFDRSRP calculated fire intensity for Forest fuel types. Circle = calculated at a time since fire of 5 years; crosses = calculated at a time since fire of 25 years. The x-axis shows maximum total fuel load for each local level forest fuel type (n = 82); note that all other fuel attributes used to calculate intensity also vary per fuel type. Weather parameters used: wind speed 35 km h−1; temperature 30°C; relative humidity 20%; drought factor 9. Equivalent FFDI = 35 (very high).
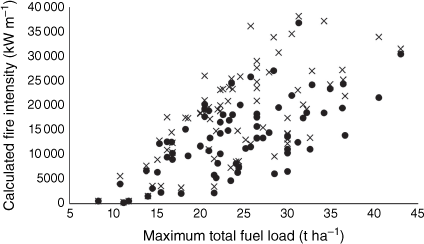
Although grass-like fuels cover the largest area of Australia (Cheney and Sullivan 2008; Sullivan et al. 2012), this includes a variety of fuel types: tussock grassland; pasture and crop land (all included in AFDRSRP grassland broad fuel type); tropical grasslands (AFDRSRP northern grassland) and hummock grass (AFDRSRP spinifex).
The classification of grass fuels is where the difference between the McArthur based fire danger forecasts and the AFDRSRP becomes most apparent (Fig. 1 and Supplementary Fig. S2). McArthur fire danger ratings are primarily set by GFDI, with 89% area coverage and 77% of Fire Weather Districts dominated by fuel classified as grass (Fig. 1). Under the AFDRSRP classification, Grassland and Northern Grassland (allowing for a tree canopy over a grassy understorey) are still the primary fuels in terms of area covered (28 and 36% respectively), Fire Weather Districts influenced (46 and 20%), and reported incidents (33 and 37%) (Table 2). Between Grassland and Northern Grassland, the latter covers a greater physical area but in less populated areas, hence Grassland influences more Fire Weather Districts.
The other fire behaviour models (i.e. spinifex, mallee-heath, shrubland, buttongrass and pine), which are not considered when calculating fire danger in the McArthur System, collectively cover a substantial portion (29%) of Australia (Table 2).
Spinifex covers a large area of inland Australia, but coincides with the most arid, remote and sparsely populated areas. In the FFDI/GFDI forecast system, this is all treated as grass. Fire behaviour in spinifex hummock grasslands is very different to that in continuous grasslands, due to the different structure, continuity and life cycle of spinifex (Cruz et al. 2015a; Burrows et al. 2018). The influence of recent fire on the calculation of probability and rate of fire spread in the spinifex model was observed as obvious patterns in forecast fire danger (Supplementary Fig. S1c). This is in contrast with the GFDI in continuous grasslands, which is driven by weather-dependent grass curing and condition (Cheney et al. 1998; Cruz et al. 2015a).
The other broad fuel types (mallee-heath, shrubland, buttongrass and pine) are generally represented by FFDI calculations in the McArthur system. Spatially, these fuel types have a small national area coverage and do not influence many Fire Weather Districts (Table 2, Supplementary Fig. S2). However, these fuel types all have unique characteristics influencing their fire behaviour (Marsden-Smedley and Catchpole 1995a; Cruz and Fernandes 2008; Cruz et al. 2013; Anderson et al. 2015). For example, buttongrass moorlands are known to burn rapidly with high fuel moisture levels and low–moderate forecast FFDI (Marsden-Smedley and Catchpole 1995a), when surrounding rainforest and wet eucalypt forest are unlikely to be flammable under those conditions (Marsden-Smedley et al. 1999). Such differences became apparent in the AFDRSRP where these fuel types occur in proximity to each other, resulting in considerably different forecast FBI in adjacent forecast grid cells (Supplementary Fig. S1d). An evaluation of the performance of the AFDRSRP across the range of fuel types is presented by Grootemaat et al. (2024).
System improvements
Various improvements were made to the system during the live trial period, demonstrating the importance of the modular nature of the system and its ability to support continual improvement.
Updates to the spinifex model provide an example of successful updates to the AFDRSRP. During development of the AFDRSRP model code, the spinifex model was under revision (released as Burrows et al. (2018)). A draft version was initially implemented in discussion with the authors, then updated once the latest version was published. This update occurred successfully during the live trial period. Equations for accumulation of spinifex fuel load and cover were implemented in the spinifex module via code rather than the fuel attribute tables. The equations used describe spinifex growth in the arid and semi-arid zones (N. Burrows, pers. comm.). Spinifex growth and cover follow a rainfall gradient from arid to tropical zones (Allan and Southgate 2002); therefore, more appropriate fuel values (the late dry season tables from the Carbon Farming Initiative methodology (Australian Government 2015)) have now been applied, with a productivity code in the fuel attribute table to determine which fuel values to use.
Limitations
During the development of the AFDRSRP, a list of knowledge gaps, limitations and potential improvements was compiled (Matthews et al. 2018). Many of these items were addressed and revisions made prior to implementation of the AFDRS. The major issues are discussed below.
The fire spread models adopted in the AFDRSRP were designed for calculating the forward rate of spread of fully developed fires. Using them at a broader scale to predict fire danger (i.e. potential fire behaviour if an ignition were to occur) creates some limitations. The lack of incorporating a build-up phase may lead the system to over-predict fire potential (Cruz et al. 2015a; Plucinski et al. 2017). Local terrain effects (e.g. slope, alignment with wind direction), which can have a significant impact on potential rate of spread (McArthur 1967; Sullivan et al. 2014), are not accounted for due to the scale of calculations.
The simple fuel availability function applied for wet forests was identified as requiring revision (Grootemaat et al. 2024). This is a topic of ongoing research (Cawson et al. 2017; Duff et al. 2018), so improvements are expected when this research can be collated into an operational solution. Similar limitations of fuel availability apply to other fuel types, for example mallee-heath and shrublands, for which the buttongrass moorland fuel availability calculation has been used in this work.
A limitation seen in other applications of fuel classification (Plucinski et al. 2017; Cruz et al. 2018) is that some vegetation types (e.g. rainforests, arid shrublands, wetlands) do not have an established fire spread model, nor do disturbed and developed areas. When building a spatial system with full national coverage, these must be accounted for. The approach taken here was to use the fire spread model most likely to represent the fuel structure and make modifications based on fuel characteristics (as per Plucinski et al. 2017). Some of these modified models worked well, but most have not been adequately tested through the evaluations conducted to date. Although fire spread models may be developed for some of these fuel types in the future (e.g. crops, Cruz et al. 2020), most are of low priority due to the low flammability (Cruz et al. 2018) and/or remote distribution. Of these, the ephemeral grass fuel types (arid woodland and chenopod shrublands) cover the greatest area. Several solutions could be implemented to more accurately reflect ephemeral grass condition, including remote sensing (Tindall et al. 2015), rainfall monitoring (Bastin 2014), grass growth modelling (Stone et al. 2010) or improved jurisdictional reporting.
Implementation of the AFDRS
The full operational AFDRS was implemented nationally in September 2022, based on the foundation for calculating fire danger developed in the AFDRSRP. The system architecture for the AFDRS is based on four modules: (1) a fuel state editor (an interactive tool for capture, upload and approval of the fuel inputs); (2) fire danger calculations (implementation of the fire behaviour models and forecast calculations); (3) a fire danger viewer (interactive visualisation of the weather and fire danger forecasts, including red flags); and (4) seasonal outlooks (visualisation products of seasonal forecasts of fire danger).
Data availability
Fuel data that support this study were obtained from Australian fire agencies by permission/licence. Data will be shared upon reasonable request to the corresponding author with permission from the Australasian Fire and Emergency Service Authorities Council.
Declaration of funding
The AFDRS was funded by grants from the Australian Government’s National Emergency Management Projects programme and the NSW Rural Fire Service’s Bush Fire Risk Mitigation and Resilience Programme. The project also received considerable in-kind support from the Bureau of Meteorology.
Acknowledgements
The development of the AFDRSRP relied on the expertise and participation of many fire managers and researchers, and we thank those that were part of early project planning, development and support, particularly from the AFAC Project Office. Special thanks goes out to all operational staff and researchers that helped us design the AFDRSRP and provided input fuel and fire history data (Tables S4). We also thank the International Journal of Wildland Fire’s Associate Editor for the Special Section and anonymous reviewers for their contributions towards improving our manuscript.
Author contributions
B. J. Kenny: development of AFDRSRP fuel type classification and national spatial datasets, original draft. S. Matthews: development oversight, data analysis. S. Sauvage: system software build. S. Grootemaat: selection and integration of fire spread models. J. J. Hollis and P. Fox-Hughes: coordinated manuscript revision, submission process. All authors contributed to the system development, data analysis, writing, review and editing of this paper.
References
Anderson WR, Cruz MG, Fernandes PM, McCaw L, Vega JA, Bradstock RA, Fogarty L, Gould J, McCarthy G, Marsden-Smedley JB, Matthews S, Mattingley G, Pearce HG, van Wilgen BW (2015) A generic, empirical-based model for predicting rate of fire spread in shrublands. International Journal of Wildland Fire 24, 443-460.
| Crossref | Google Scholar |
Blischak JD, Davenport ER, Wilson G (2016) A Quick Introduction to Version Control with Git and GitHub. PLOS Computational Biology 12, e1004668.
| Crossref | Google Scholar |
Bureau of Meteorology (2023a) ‘Fire Weather Service Level Specification, Season 2022-2023, Severe Weather Environmental Prediction Services.’ (Commonwealth of Australia) Available at http://www.bom.gov.au/weather-services/fire-weather-centre/fire-weather-services/documents/Fire_Weather_Service_Level_Specification.pdf
Bureau of Meteorology (2023b) User Guide – Australian Digital Forecast Database (ADFD), Version 21 (Updated 19 May 2023). (Australian Government, Bureau of Meteorology) Available at http://www.bom.gov.au/catalogue/adfdUserGuide.pdf, chrome-extension://efaidnbmnnnibpcajpcglclefindmkaj/http://www.bom.gov.au/catalogue/adfdUserGuide.pdf
Burrows N, Gill M, Sharples J (2018) Development and validation of a model for predicting fire behaviour in spinifex grasslands of arid Australia. International Journal of Wildland Fire 27, 271-279.
| Crossref | Google Scholar |
Cawson JG, Duff TJ, Tolhurst KG, Baillie CC, Penman TD (2017) Fuel moisture in Mountain Ash forests with contrasting fire histories. Forest Ecology and Management 400, 568-577.
| Crossref | Google Scholar |
Cheney NP, Gould JS, Catchpole WR (1998) Prediction of fire spread in grasslands. International Journal of Wildland Fire 8, 1-13.
| Crossref | Google Scholar |
Cheney NP, Gould JS, McCaw WL, Anderson WR (2012) Predicting fire behaviour in dry eucalypt forest in southern Australia. Forest Ecology and Management 280, 120-131.
| Crossref | Google Scholar |
Cruz MG, Fernandes PM (2008) Development of fuel models for fire behaviour prediction in maritime pine (Pinus pinaster Ait.) stands. International Journal of Wildland Fire 17, 194-204.
| Crossref | Google Scholar |
Cruz MG, Alexander ME, Fernandes PAM (2008) Development of a model system to predict wildfire behaviour in pine plantations. Australian Forestry 71, 113-121.
| Crossref | Google Scholar |
Cruz MG, McCaw WL, Anderson WR, Gould JS (2013) Fire behaviour modelling in semi-arid mallee-heath shrublands of southern Australia. Environmental Modelling & Software 40, 21-34.
| Crossref | Google Scholar |
Cruz MG, Gould JS, Kidnie S, Bessell R, Nichols D, Slijepcevic A (2015b) Effects of curing on grassfires: II. Effect of grass senescence on the rate of fire spread. International Journal of Wildland Fire 24, 838-848.
| Crossref | Google Scholar |
Cruz M, Gould J, Hollis J, McCaw W (2018) A hierarchical classification of wildland fire fuels for Australian vegetation types. Fire 1, 13.
| Crossref | Google Scholar |
Cruz MG, Hurley RJ, Bessell R, Sullivan AL (2020) Fire behaviour in wheat crops – effect of fuel structure on rate of fire spread. International Journal of Wildland Fire 29, 258-271.
| Crossref | Google Scholar |
Dharssi I, Vinodkumar (2017) ‘JASMIN: A prototype high resolution soil moisture analysis system for Australia.’ (Bureau of Meteorology Research Report No. 26) Available at http://www.bom.gov.au/research/publications/researchreports/BRR-026.pdf
Dowdy AJ, Pepler A (2018) Pyroconvection risk in Australia: Climatological changes in atmospheric stability and surface fire weather conditions. Geophysical Research Letters 45, 2005-2013.
| Crossref | Google Scholar |
Duff TJ, Bell TL, York A (2013) Predicting continuous variation in forest fuel load using biophysical models: a case study in south-eastern Australia. International Journal of Wildland Fire 22, 318-332.
| Crossref | Google Scholar |
Duff TJ, Cawson JG, Harris S (2018) Dryness thresholds for fire occurrence vary by forest type along an aridity gradient: evidence from Southern Australia. Landscape Ecology 33, 1369-1383.
| Crossref | Google Scholar |
Griffiths D (1999) Improved Formula for the Drought Factor in McArthur’s Forest Fire Danger Meter. Australian Forestry 62, 202-206.
| Crossref | Google Scholar |
Grootemaat S, Matthews S, Kenny BJ, Runcie J, Hollis JJ, Sauvage S, Fox-Hughes P, Holmes A (2024) Live trial performance of the Australian Fire Danger Rating System - Research Prototype. International Journal of Wildland Fire 33,.
| Crossref | Google Scholar |
Hilton JE, Miller C, Sullivan AL, Rucinski C (2015) Effects of spatial and temporal variation in environmental conditions on simulation of wildfire spread. Environmental Modelling & Software 67, 118-127.
| Google Scholar |
Hollis JJ, Matthews S, Anderson WR, Cruz MG, Fox-Hughes P, Grootemaat S, Kenny BJ, Sauvage S (2024a) A framework for defining fire danger rating to support fire management operations in Australia. International Journal of Wildland Fire 33,.
| Crossref | Google Scholar |
Hollis JJ, SM, Cruz MG, Fox-Hughes P, Grootemaat S, Heemstra S, Kenny BJ, Savage S (2024b) Intruduction to the Australian Fire Danger Rating System. International Journal of Wildland Fire 33,.
| Crossref | Google Scholar |
Hollis JJ, Gould JS, Cruz MG, Lachlan McCaw W (2015) Framework for an Australian fuel classification to support bushfire management. Australian Forestry 78, 1-17.
| Crossref | Google Scholar |
Hoyer S, Hamman J (2017) xarray: N-D labeled Arrays and Datasets in Python. Journal of Open Research Software 5, 10.
| Crossref | Google Scholar |
Keane RE (2013) Describing wildland surface fuel loading for fire management: a review of approaches, methods and systems. International Journal of Wildland Fire 22, 51-62.
| Crossref | Google Scholar |
Keane RE, Burgan R, van Wagtendonk J (2001) Mapping wildland fuels for fire management across multiple scales: integrating remote sensing, GIS, and biophysical modeling. International Journal of Wildland Fire 10, 301-319.
| Crossref | Google Scholar |
Kluyver T, Ragan-Kelley B, Perez F, Granger B, Bussonnier M, Frederic J, Kelley K, Hamrick J, Grout J, Corlay S, Ivanov P, Avila D, Abdalla S, Willing C (2016) Jupyter Notebooks – a publishing format for reproducible computational workflows. In ‘Positioning and Power in Academic Publishing: Players, Agents and Agendas’ (Eds F Loizides, B Scmidt) pp. 87–90. (IOS Press: Amsterdam, Netherlands)
Loveland TR (2001) Toward a national fuels mapping strategy: lessons from selected mapping programs. International Journal of Wildland Fire 10, 289-299.
| Crossref | Google Scholar |
Marsden-Smedley J, Catchpole WR (1995a) Fire behaviour modelling in tasmanian buttongrass moorlands. I. Fuel characteristics. International Journal of Wildland Fire 5, 215-228.
| Crossref | Google Scholar |
Marsden-Smedley JB, Catchpole WR (1995b) Fire Behaviour modelling in tasmanian buttongrass moorlands. II. Fire behaviour. International Journal of Wildland Fire 5, 203-214.
| Crossref | Google Scholar |
Marsden-Smedley JB, Rudman T, Catchpole WR, Pyrke A (1999) Buttongrass moorland fire behaviour prediction and management. Tasforests 11, 87-107.
| Google Scholar |
Matthews S (2006) A process-based model of fine fuel moisture. International Journal of Wildland Fire 15, 155-168.
| Crossref | Google Scholar |
Matthews S (2014) Dead fuel moisture research: 1991–2012. International Journal of Wildland Fire 23, 78-92.
| Crossref | Google Scholar |
Matthews S, Fox-Hughes P, Grootemaat S, Hollis JJ, Kenny BJ, Sauvage S (2018) ‘National Fire Danger Rating System: Research Prototype.’ (NSW Rural Fire Service: Lidcombe, NSW, Australia) Available at https://www.afac.com.au/docs/default-source/afdrs/afdrs_research_prototype_report_2019.pdf?sfvrsn=6&download=false
McKinney W (2011) Pandas: A Foundational Python Library for Data Analysis and Statistics. PyHPC2011. Available at https://www.dlr.de/sc/en/Portaldata/15/Resources/dokumente/pyhpc2011/submissions/pyhpc2011_submission_9.pdf
Noble IR, Gill AM, Bary GAV (1980) McArthur’s fire-danger meters expressed as equations. Australian Journal of Ecology 5, 201-203.
| Crossref | Google Scholar |
Olson JS (1963) Energy storage and the balance of producers and decomposers in ecological systems. Ecology 44, 322-331.
| Crossref | Google Scholar |
Plucinski MP, Sullivan AL, Rucinski CJ, Prakash M (2017) Improving the reliability and utility of operational bushfire behaviour predictions in Australian vegetation. Environmental Modelling & Software 91, 1-12.
| Crossref | Google Scholar |
Rew R, Davis G (1990) NetCDF: an interface for scientific data access. IEEE Computer Graphics and Applications 10, 76-82.
| Crossref | Google Scholar |
Sauvage S, Fox-Hughes P, Matthews S, Kenny B, Hollis J, Grootemaat S, Runcie J, Holmes A, Harris R, Love P, Williamson G (2024) Australian Fire Danger Rating System Research Prototype: a climatology. International Journal of Wildland Fire 33,.
| Crossref | Google Scholar |
Stone GS, Day KA, Carter JO, Bruget DN, Panjkov AA (2010) The AussieGRASS Environmental Calculator: its application in Australian grasslands. In ‘16th Biennial Conference of the Australian Rangeland Society’, Perth, Australia. (Eds DJ Eldridge, C Waters) (Australian Rangeland Society, Perth, Australia)
Sullivan AL, McCaw WL, Cruz MG, Matthews S, Ellis PF (2012) Fuel, fire weather and fire behaviour in Australian ecosystems. In ‘Flammable Australia: Fire regimes, biodiversity and ecosystems in a changing world’. (Eds RA Bradstock, AM Gill, RJ Williams) pp. 51–77. (CSIRO Publishing: Vic., Australia)
Sullivan AL, Sharples JJ, Matthews S, Plucinski MP (2014) A downslope fire spread correction factor based on landscape-scale fire behaviour. Environmental Modelling & Software 62, 153-163.
| Crossref | Google Scholar |
Taylor SW, Alexander ME (2006) Science, technology, and human factors in fire danger rating: the Canadian experience. International Journal of Wildland Fire 15, 121-135.
| Crossref | Google Scholar |
Tolhurst KG, Duff TJ, Chong DM (2013) “Wildland–Urban Interface” to “Wildfire Interface Zone” using dynamic fire modelling. In ‘MODSIM2013 20th International Congress on Modelling and Simulation’. (Eds J Piantadosi RS Anderssen, J Boland) pp. 290–296. (Modelling and Simulation Society of Australia and New Zealand, Adelaide)
van der Walt S, Colbert SC, Varoquaux G (2011) The NumPy Array: A Structure for Efficient Numerical Computation. Computing in Science & Engineering 13, 22-30.
| Crossref | Google Scholar |