A national accounting framework for fire and carbon dynamics in Australian savannas
Keryn I. Paul A * and Stephen H. Roxburgh AA
Abstract
Tropical savannas represent a large proportion of the area burnt each year globally, with growing evidence that management to curtail fire frequency and intensity in some of these regions can contribute to mitigation of climate change. Approximately 25% of Australia’s fire-prone tropical savanna region is currently managed for carbon projects, contributing significantly to Australia’s National Greenhouse Gas Inventory.
To improve the accuracy of Australia’s national carbon accounting model (FullCAM) for reporting of fire emissions and sequestration of carbon in savanna ecosystems.
Field data from Australian savannas were collated and used to calibrate FullCAM parameters for the prediction of living biomass, standing dead biomass and debris within seven broad vegetation types.
Revised parameter sets and improved predictions of carbon stocks and fluxes across Australia’s savanna ecosystems in response to wildfire and planned fire were obtained.
The FullCAM model was successfully calibrated to include fire impacts and post-fire recovery in savanna ecosystems.
This study has expanded the capability of FullCAM to simulate both reduced emissions and increased sequestration of carbon in response to management of fire in tropical savanna regions of Australia, with implications for carbon accounting at national and project scales.
Keywords: carbon, carbon accounting, emissions, fire, fuel, FullCAM, litterfall, mortality, savannas, standing dead.
Introduction
Tropical savannas currently account for most of the global area burnt (van der Werf et al. 2017; Giglio et al. 2018). In Australia, tropical savanna represents 26% of the land area (Edwards et al. 2021), burning at frequencies of 0.158–0.362 year−1 (Whitehead et al. 2014; Cook et al. 2020). Traditional Indigenous use of fire as a land management tool is again becoming common practice in these regions (Cook et al. 2012; Edwards et al. 2021). This entails igniting cool and patchy fires in the early dry season (EDS) to decrease prevalence of large high intensity late dry season (LDS) wildfires. This management decreases greenhouse gas emissions (Russell-Smith et al. 2013) and may also lead to increased storage of carbon in pools of dead (Cook et al. 2016, 2020) and live (Ryan and Williams 2011; Murphy et al. 2023) biomass.
This change in land management is having a significant impact on Australia’s National Inventory Reporting (NIR) and greenhouse gas emissions (expressed in units of carbon dioxide equivalents: CO2-e). Australia’s savanna region was initially (1990–2015) a major source of emissions (~+20 Mt CO2-e year−1), but more recently (2016–2020) has become a net sink (~−5.5 Mt CO2-e year−1), coinciding with an increased proportion of fires in the EDS, and hence accounting for both a decrease in total emissions from fire and increased storage of carbon in dead and live biomass (NIR, Commonwealth of Australia 2022).
To inform Australia’s annual NIR for savanna regions, remote sensing of fire scars provides inputs to the spatial-temporal carbon accounting model, FullCAM (Paul and Roxburgh 2019). FullCAM is an empirical carbon tracking model, and therefore, its accuracy directly relates to the quality and quantity of underlying calibration datasets. The aim of the present study was to improve the accuracy of FullCAM for reporting fire emissions and sequestration of carbon in savanna ecosystems through collating extensive field data to calibrate model parameters for the prediction of living biomass, standing dead biomass and debris. Through more accurate simulations of both fire emissions and sequestration of carbon, the primary outcome of this work was enhanced accuracy of Australia’s NIR (as recently implemented in Commonwealth of Australia 2022). A secondary outcome of this study was to provide options to enhance the accuracy in accounting for net abatement from savanna fire management projects. A comprehensive methodology encompassing the impacts of different fire types on both avoided emissions and sequestration of carbon is required given current methodologies available either only account for avoided emissions (Commonwealth of Australia 2015), account for sequestration only in dead pools rather than both live and dead pools (Commonwealth of Australia 2018), or do not distinguish between effects of fires of different intensities (Voluntary Carbon Standard 2015).
Methods
Description of the FullCAM model and its simulation of fire events
For any given pixel (approximately 25 × 25 m area of application of the model, as applied in the NIR) or ‘site’ (0.02–2 ha transects or plots measured in field assessments, as applied during model calibration), FullCAM simulates carbon dynamics of live and dead pools of both woody and grass components in response to regular mortality and turnover (and corresponding recruitment and recovery), as well as stochastic disturbance events such as fires (Fig. 1 and Supplementary Material A). Because FullCAM is a pool-based model and does not simulate populations of individuals, mortality, recruitment and recovery are simulated via increases and reductions in standing biomass. The model includes: (i) live biomass of trees and shrubs, simulated as woody Above-Ground Biomass (AGB); (ii) heavy woody fuel, including stags (elevated dead trees or shrubs) and Coarse Woody Debris (CWD, on-ground components of debris ≥5 cm diameter), simulated together in the model as the ‘standing dead’ pool; (iii) coarse woody fuel, including on-ground branch and bark litter of 0.6–5 cm diameter, simulated as 60% of branch and bark debris; (iv) fine woody fuel, including foliage litter and some of the ≤0.6 cm diameter branch and bark litter that is partially decomposed or broken down, simulated as foliage debris plus the remaining 40% of branch and bark debris; and (v) fine grass fuel, simulated as grass leaf biomass and grass litter.
Flow of carbon in a typical savanna woodland system as simulated by FullCAM. Flows of carbon associated with fire events are indicated in red.
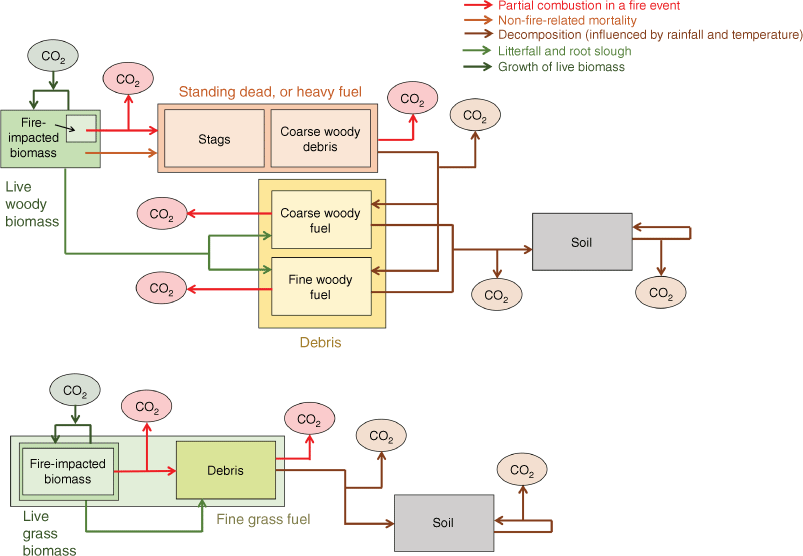
A key spatially explicit input influencing productivity, and therefore predicted rates of post-fire recovery, is the maximum above-ground woody biomass, M, (Roxburgh et al. 2019) that a pixel or ‘site’ can support given long-term average site resources (e.g. rainfall), which for savanna regions is well validated (Supplementary Fig. S2; although this could still be further improved; Supplementary Material F). In the current implementation of the model, M is therefore constant, and thus FullCAM is insensitive to potential changes in M driven by changes in resource availability, such as a change to the hydrological regime leading to increases (or decreases) in soil water availability (Supplementary Material B). To further account for spatially explicit differences in sensitivity of biomass to fire, the model also accounts for seven different types of savanna vegetation (Supplementary Material A), as fire impacts these differently, largely because of their differing wood-to-grass composition (Table 1). Subsuming groundcover types (tussock, hummock, mixed grassed) into the vegetation structural classes outlined in Table 1 is a simplification of previous vegetation categories that accounted for differences in recovery rates of these groundcovers as it was assumed that over the extent of fire-prone savannas, such differences might be negligible.
Rainfall zone | Vegetation category | Sub-division | Woody canopy cover (%) A | Previous vegetation types | |
---|---|---|---|---|---|
H | WH B | WH0.6 | 60 | hOFM G | |
WH0.3 | 30 | hWMi H, hWHu I | |||
SH C | 15 | hSHH J | |||
L | WL D | WL0.2 | 20 | lWHu K, lWMi L, lWTu M | |
WL0.1 | 10 | lOWM N | |||
SL E | 10 | lSHH O | |||
PL F | 30 | Not previously applied |
Also indicated are the previous definitions of savanna vegetation applicable to these categories (Meyer et al. 2015). Further explanation of these different categories of vegetation is given in Supplementary Material A.
Different types of fire are simulated, depending on the season (EDS, April–July inclusive; or LDS, August–December inclusive) and intensity (with subscripts of 1, 2 or 3 indicating fire intensity of <1, 1–2 and >2 MW m−1, respectively, e.g. EDS1 indicating early dry season fire of low intensity) (Russell-Smith and Edwards 2006). In FullCAM, the fire type influences combustion factors (CF = CO2-C released per unit of dry fuel C consumed, expressed on a dry weight basis and represented below as a percentage C loss term), and in the case of live pools of biomass, fire type also influences an additional loss of carbon from live pools of biomass accounted for via live-to-dead biomass pool transfer factors (TF = proportion of carbon in live biomass pools transferred to standing dead pools in response to fire, expressed on a dry weight basis). To account for emissions of methane (CH4) and nitrous oxide (N2O) in response to changed fire management (IPCC 2006), the simplifying assumption was made that FullCAM-predicted CO2-C emissions (Mg C ha−1) are multiplied by the emission factors (EF) listed in Supplementary Table S1 (Surawski et al. 2016). Differences in emissions between vegetation types were partly attributed to local variability in fire intensity and flame height (Meyer and Cook 2015).
The capacity for vegetative regeneration post-fire is well developed in savannas (e.g. Lacey 1974), and so although trees or shrubs may experience partial mortality due to fire, they typically survive and recover (e.g. Lonsdale and Braithwaite 1991; Williams et al. 1999). This is attributable to a combination of resprouting from epicormic buds and/or regrowth of saplings (Bond et al. 2012; Lawes et al. 2022). Hence, FullCAM simulations ensure fire-induced changes in AGB inversely impact standing dead mass (via the TF), consistent with the creation of heavy fuel when trees die (Cook et al. 2015a) (Supplementary Fig. S3).
When high-intensity fires are frequent, predicted AGB does not fully recover to M between fire events, with a fire-induced suppression of AGB (e.g. as occurs in 1972–1980 in Supplementary Fig. S3), ensuring simulations produce negative correlations between predicted AGB and fire frequency where fires are frequent and severe (e.g. Murphy et al. 2013). Hence, fire simulation in FullCAM temporarily decreases AGB below M, the climatically determined upper bound (e.g. Liedloff and Cook 2007; Murphy et al. 2015) (Supplementary Material B). Consistent with findings of Cook et al. (2017), a modelled assumption was that increased fire intensity results in increased fire impact on AGB (which in FullCAM is CF + TF), and hence, time to recover. Given Cook et al. (2017) reported recovery times of savanna biomass of 2–17 years, as fire impact on AGB increases from 2.5 to 15%, predicted recovery time increases from 1 to 5 years, with a maximum time to recover of 20 years (Supplementary Fig. S4).
As FullCAM can be applied spatially across the landscape, patchiness of fire is accounted for by simulating the fire event across only a proportion of the pixels within the total fire scar area (Paul and Roxburgh 2019). For EDS fires, this was 70.9 and 79.0% of the fire scar in high and low rainfall zones, respectively, whereas for LDS fires, it was 88.9 and 97.0% in high and low rainfall zones, respectively (Russell-Smith and Yates 2007; Russell-Smith et al. 2009a; Yates et al. 2015).
In summary, the type and frequency of fire event has an impact on carbon stocks in live and dead pools (both in total mass and in vegetative composition), which, in turn, impacts subsequent fire emissions owing to the availability of volatile components of live and dead biomass. Hence, the FullCAM structure allows for interactions and feedbacks between fire emissions and carbon stocks.
Model calibration
Calibration of FullCAM to savanna fires was a two-step process: firstly, constraining parameter values based on review of the collated datasets, and secondly, optimising remaining ‘unknown’ parameters by ensuring predicted pools of AGB and heavy, coarse, fine and grass fuel matched observations. Across these steps, calculations were required to estimate observed AGB and fuel pools from the available field data (details provided in Supplementary Material C). It was beyond the scope of this work to calibrate FullCAM for fire impacts on soil carbon.
Parameters constrained to observed data: data collected and assumptions made
To derive stand-level biomass allocation (AGB components of stem, branch, bark, foliage, and below-ground biomass components of coarse roots (BGBC) and fine roots (BGBF), data were collated from: (a) biomass sampling studies demonstrating proportions of AGB allocated to components for the different Plant Functional Types (PFTs, Supplementary Tables S2 and S11), and (b) 1091 stand inventories across Australian savannas (Supplementary Tables S3 and S4) that indicated the relative contribution of each PFT to total stand AGB (Supplementary Fig. S11a), and the BGBC:AGB ratio (Supplementary Fig. S11b). Given the paucity of data, contribution of BGBF (<2 mm diameter) to total root biomass (BGBF:BGBTotal) was calculated via application of the empirical relationship derived by Mokany and Raison (2004).
To calibrate FullCAM biomass turnover parameters (Fig. 1 and Supplementary Fig. S1), litterfall datasets were collated from 61 stands across Australia’s savannas (Supplementary Table S12). As outlined in Supplementary Material C, additional calculations were required to (a) estimate total litterfall attributable to foliage, branch and bark; and (b) determine a multiplier to convert litterfall measured from litterfall traps into total litterfall, given these traps often fail to capture spatially heterogeneous litterfall arising from large branches (and pieces of bark, and any twigs or foliage attached to these larger branches).
FullCAM parameters for decomposition of standing dead (stags), deadwood debris (components of heavy and coarse fuels) and bark debris (also components of heavy and coarse fuels) pools were calibrated to be constrained within the range reported by Cook et al. (2020).
Previous CF estimates (Fensham 2005; Russell-Smith et al. 2009a; Yates et al. 2015) provided only a guide in constraining the FullCAM CF parameters as they were estimates for pools as a whole, whereas FullCAM separately simulates different components of each of these broad categories (Fig. 1 and Supplementary Fig. S1).
Parameters calibrated via model optimisation and testing
Three parameter sets required fitting via optimisation given the paucity and/or high uncertainty of available data. The first was mortality, defined here as the regular annual rate of death of live stems not attributable to fire, and assumed to occur in November of each year (consistent with the seasonal observations of coarse litter production), but with resulting AGB loss being replaced within 1 year via regrowth that maintains the assumed steady-state condition. The other two were fire event parameters that determine, for different types of fire, CF and TF of different components of AGB. These parameters were calibrated together to ensure their combination resulted in dynamic predictions of AGB that matched those observed across the monitored transects (Supplementary Table S3 and Fig. 2).
Location of the transect-based surveys used to assess live biomass and heavy fuel in the different types of tropical savanna vegetation. Data source: Lynch et al. (2018), Cook et al. (2020), Murphy et al. (2023), S. Bray, pers. comm. (2020). WH, Woodland – High rainfall; WL, Woodland – Low rainfall; SH, Shrubland – High rainfall; SL, Shrubland – Low rainfall; PL, Pindan. Full vegetation type definitions are provided in Table 1.
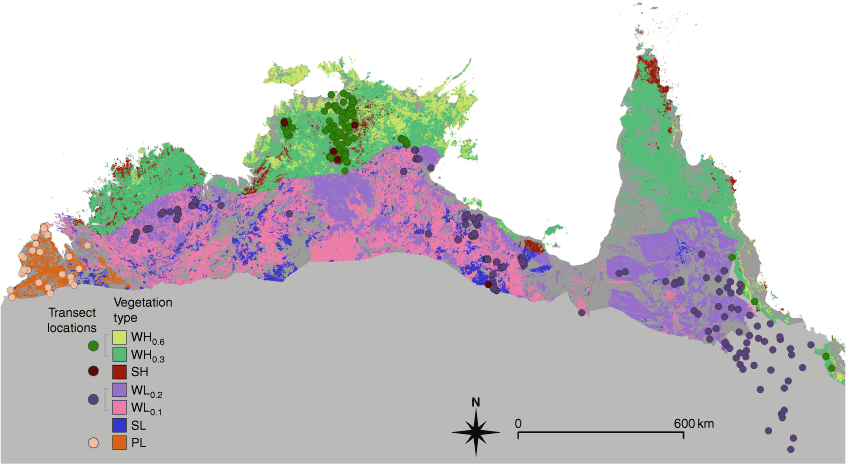
Calibration stands were simulated to replicate field plot or transect areas (averaging 0.24 ha, range 0.03–2.00 ha) where: (i) measurements were made across a range of savanna vegetation types based on field assessments of tree stem density, cover and growth forms of dominant grasses, or when not available, vegetation maps of Thackway et al. (2014) and Lynch et al. (2018); (ii) recent (~5 years) fire history for each of these calibration stands was derived from aerial photos with on-site observations, or when not available and for earlier time-periods, time series of satellite-based observations ( 1-km resolution) as described by Meyer et al. (2015); and (iii) climatic conditions (monthly rainfall, temperature, evaporation over the period of simulation) for each of these calibration stands were derived from Commonwealth of Australia (2022).
As outlined in Supplementary Material B, the model input M is important in providing the upper limit for AGB increases following savanna fire management. The M applied to each calibration site was the maximum observed AGB at that site (i.e. maximum observed AGB in a time-series of observations for a given site, Supplementary Material B). A 500-year model spin-up period was applied (where satellite-derived fire histories for that site or region were assumed to also apply prior to 1988), such that by the time of interest (when predictions were compared with observations), predicted stand biomass and fuel pools were in a state of equilibrium with respect to the fire regime simulated, with simulated fire events at this time only influencing interannual variations in predicted stocks and fluxes. Where the fire intensity was unknown, it was assumed to be moderate, EDS2 or LDS2.
In addition to M, the predicted AGB of a stand is also influenced by the assumed rates of mortality. Annual rates of total mortality (fire- and non-fire-related) in stands of Australian savannas have been estimated to range from <0.1 to 4% per year (e.g. Lonsdale and Braithwaite 1991; Prior et al. 2009; Cook et al. 2016, 2020). There is a paucity of data to indicate how fire- and non-fire-related mortality interact, but an assumption was made that fire-related mortality would be between ~50 and 75% of these estimates of total mortality (Cook et al. 2020), but declining to a negligible contribution of total mortality under conditions of low fire intensities (Murphy et al. 2023). Based on these estimates, when calibrating typical rates at which AGB declines (and subsequently regrows) owing to non-fire-related mortality, values of 2.24% year−1 (or 2.70% year−1) for categories of vegetation from high (or low) rainfall zones were assumed to be the upper limit, and were implemented within FullCAM as percentage transfers from the above- and below-ground standing biomass pools to the standing dead pool.
When calibrating CF and TF parameters for AGB, it was ensured that the total impact of fire on AGB (= CF + TF) was consistent with that previously observed. Previous workers (Russell-Smith et al. 2009a; Yates et al. 2015) have estimated that total fire impact on AGB (previously defined as the ‘shrub fuel’ pool comprising individuals <2 m tall) in high (or low) rainfall zones was 0.29 (or 0.10) and 0.39 (or 0.11) in EDS and LDS fire respectively. These previous estimates were for the AGB as a whole, whereas FullCAM simulates a different CF and TF for stem, branch, bark and foliage components of AGB. Therefore, again, previous estimates provided only broad constraints on the calibrations.
Different combinations of parameter values for mortality and the CF and TF of components of AGB were tested to determine which provided the highest model efficiency (EF; Soares et al. 1995) and least bias of prediction of AGB for each category of vegetation while also ensuring that, across the different categories of vegetation, the extent of fire impact on AGB increased with the observed average proportion of total woody biomass that was heavy fuel:
where is the mean square deviation of each observation from the mean of the observations and is the mean squared residual. An EF of 100% indicates perfect match between observations and predictions; 0% indicates the predictions are no better than simply using the mean of the observations, and <0% indicates that residual variation is greater than the variation in the data.
In addition to testing the efficiency of prediction of AGB, performance of the calibrated model was also tested by comparing the predicted biomass with that observed for each of the various pools of fuel: heavy, coarse, fine and grass, including their rates of recovery from time since last burn.
Scenarios of fire management: implications
To demonstrate how FullCAM can be applied to predict the impacts of fire management on avoided emissions and sequestration of carbon, hypothetical savanna burning scenarios were simulated for each of the different savanna vegetation types (Table 1, Supplementary Material D). Project areas were assumed to be 25 ha, comprising 25 × 1 ha strata. Each stratum had a unique hypothetical fire history and was therefore separately simulated using a FullCAM plot file with a unique sequence of fire events to replicate the assumed fire histories, as indicated in Supplementary Fig. S12. For simplicity, only EDS2 and LDS2 fire types were simulated.
After a 500-year model spin-up period to bring carbon pools to equilibrium, the average frequencies of EDS2 and LDS2 fires across the project area were assumed to change between a 25-year early baseline period, and subsequent 5-year intermediate period (where fire management was assumed to commence, and pools of carbon begin to re-equilibrate) and followed by a 25-year project period. The strata 25-year average differences (and standard deviation) between baseline and project were calculated for: (a) carbon stocks, and (b) fire emissions using the vegetation types in Table 1: Woodland – High rainfall (WH0.6, WH0.3), Shrubland – High rainfall (SH) and Pindan (PL) scenarios (assumed to be in localities of relatively high M, and where the baseline fire frequency was relatively high), and Woodland – Low rainfall (WL0.2, WL0.1) and Shrubland – Low rainfall (SL) scenarios (assumed to be in localities of relatively low M, and where the baseline fire frequency was relatively low) (Supplementary Table S13).
Results and discussion
Parameters constrained to observed data
Allocation parameters among the four vegetation types were broadly similar, with allocation to stem ranging from 0.337 to 0.420; allocation to branch ranging from 0.158 to 0.178; allocation to bark from 0.093 to 0.121; allocation to leaf from 0.044 to 0.099; allocation to coarse roots from 0.219 to 0.230; and allocation to fine roots from 0.051 to 0.064 (Table 2).
Stem | Branch | Bark | Leaf | BGBC | BGBF | |||
---|---|---|---|---|---|---|---|---|
Live biomass | ||||||||
Allocation | WHA | 0.376 | 0.158 | 0.121 | 0.064 | 0.230 | 0.051 | |
WLB | 0.420 | 0.158 | 0.108 | 0.044 | 0.219 | 0.051 | ||
SHC or SLD | 0.371 | 0.175 | 0.098 | 0.083 | 0.221 | 0.054 | ||
PLE | 0.337 | 0.178 | 0.093 | 0.099 | 0.229 | 0.064 | ||
Turnover (half-life, years)F, G | H | – | 9 | 10 | 1.2 | 6.56 | 0.431 | |
L | – | 9 | 10 | 1.5 | 6.56 | 0.431 | ||
Standing dead | ||||||||
Decomposition (half-life, years)F | All | 8.00 | 4.00 | 2.00 | 0.50 | – | – | |
C F (%) | H-EDS | 20 | 30 | 70 | 80 | – | – | |
H-LDS | 30 | 40 | 80 | 90 | – | – | ||
L-EDS | 10 | 20 | 70 | 80 | – | – | ||
L-LDS | 10 | 30 | 80 | 90 | – | – |
Dead wood | Bark | Leaf | BGBC | BGBF | |||
---|---|---|---|---|---|---|---|
Debris biomass | |||||||
Decomposition (half-life, years) | All | 2.00 | 1.00 | 0.866H | 4.00A | 0.0001A | |
C F (%) | H-EDS | 20I | 75J | 80 | – | – | |
H-LDS | 40I | 85J | 90 | – | – | ||
L-EDS | 10I | 75J | 80 | – | – | ||
L-LDS | 20I | 85J | 90 | – | – | ||
Grass | |||||||
C F (and T F) (%, live) | All | – | – | 93 (2.0) | – | – | |
C F (%, dead or litter) | All | – | – | 99 | – | – |
H, High rainfall zone; L, Low rainfall zone; EDS, Early Dry Season; LDS, Late Dry Season; CF, Combustion Factor; TF, Transfer Factor; BGBC, coarse roots; BGBF, fine roots.
When the resulting data-constrained turnover rates were applied in the model (Table 2), the model-predicted turnover-to-AGB relationship matched that observed (Fig. 3). Moreover, the FullCAM-predicted contributions of branch and bark to total litterfall were 25 and 16% respectively, which was similar to those expected (Supplementary Material C).
FullCAM-predicted and observed relationships between total turnover (Mg C ha−1 year−1) and the above-ground biomass carbon (AGB, Mg C ha−1) of a stand. Source of observed data is given in Supplementary Table S12, but the observed litterfall data have been multiplied by a factor of 1.33 to account for additional turnover typically attributable to larger litter components not generally captured in traditional litter traps monitoring. FullCAM-predicted turnover and AGB are the average across years for which fire history data were available (1988–2018). EF, model efficiency.
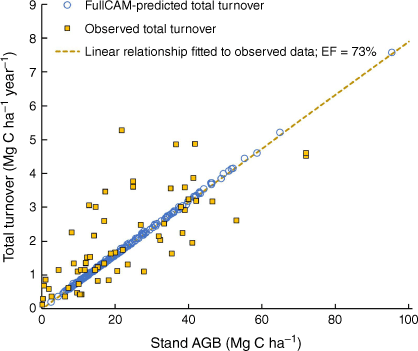
Seasonality of turnover is also important when accounting for the impact of fire management on fuel dynamics, with higher peaks of total standing litter in LDS cf. EDS, contributing to LDS fires being of relatively high intensity (Yates et al. 2020). Therefore, monthly turnover parameters were also constrained by datasets on seasonality of turnover collated from 11 stands within the high-rainfall zone (Williams et al. 1997; Cook 2003; Cuff and Brocklehurst 2015; Yates et al. 2020), and in four stands within the low-rainfall zone (McIvor 2001; Cuff and Brocklehurst 2015; Yates et al. 2020). These datasets showed foliage litterfall was typically highest in the months May to September in high-rainfall zones (Fig. 4b), but slightly later (August to November) in low-rainfall zones (Fig. 4c). In contrast, there was large variability in the seasonality of branch and bark litterfall (relatively large error bars in Fig. 4a cf. Fig. 4b, c), probably because a majority of branch and bark litterfall tends to be associated with stochastic severe wet season storms (i.e. October to December) (Yates et al. 2020).
Seasonal turnover (proportion of total annual turnover that occurs each month) for: (a) branch and bark litter as observed in either high or low rainfall zones; (b) foliage litter as observed in the high rainfall zone; (c) foliage litter as observed in the low rainfall zone, and (d) grass die-back as assumed in the FullCAM application in the NIR (Commonwealth of Australia 2022). Error bars represent the standard deviation across: (a–c) observations, or (d) years 1970–2018 in FullCAM inputs of monthly estimates of monsoonal perennial grass die-back. Source of data: Williams et al. (1997), McIvor (2001), Cook (2003), Cuff and Brocklehurst (2015), Yates et al. (2020), Commonwealth of Australia (2022).
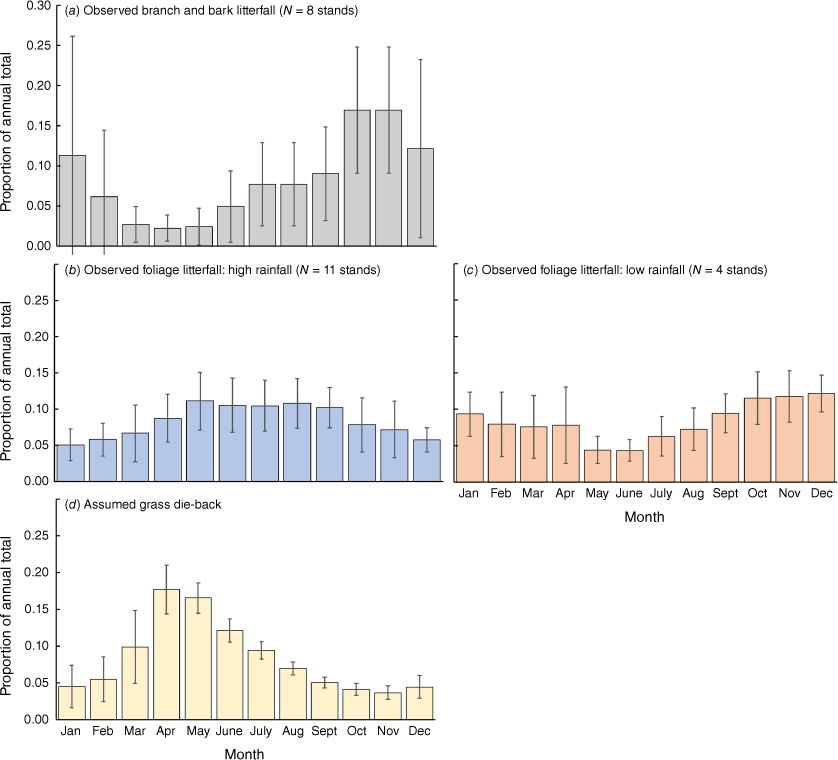
Although most of the grass curing in Australian savannas occurs in April and June (Meyer et al. 2012), it was beyond the scope of the present study to collate datasets on seasonality of turnover of grass, with default monthly parameters and seasonality for grass die-back being applied (Fig. 4d, Commonwealth of Australia 2022). Similarly, default values were applied for turnover rates of grass roots (Commonwealth of Australia 2022).
FullCAM parameters for decomposition of standing dead (stags), deadwood debris (components of heavy and coarse fuels) and bark debris (also components of heavy and coarse fuels) were 8, 2 and 1 years, respectively (Table 2). The slower decomposition for larger standing dead cf. debris deadwood was attributable to the finding that decomposition rates appear to be functions of both the density and size of the deadwood (O’Connell 1997; Mackensen et al. 2003). Half-life of wood samples of 2.2–6.5 cm diameter ranged between 0.49 and 26.7 years over a 2-year period at a savanna stand in the high-rainfall zone (Cook et al. 2020). Similarly, studies from non-savanna regions of Australia indicate decomposition rates of eucalypt deadwood ranging from 8 to 15 cm diameter had a half-life that varies from 4 to 32 years (Brown et al. 1996; O’Connell 1997). In Australian savannas, attack from termites is another important factor influencing decomposition of deadwood (e.g. Dawes 2010), but was beyond the scope of the present study to explicitly consider, with termite impacts on decomposition assumed to be subsumed into the available observations of decomposition rates.
When considering the fine fuel decomposition rates in Australian savannas, observations were also varied. Dawes (2010) reported a half-life of between 0.58 and 1.61 years (average 0.79 years) for decomposing ‘dry straw mulch’, withdecomposition rates varying with the type of litter bag and whether or not termites were present. Studying a species of native grass, Rossiter-Rachor et al. (2017) reported a half-life of only 0.33 years. Cook (2003) reported a half-life of 0.866 years for decomposing savanna ‘tree litter and grass’ within litter bags. This value was applied to calibrate the decomposition parameter for the resistant fraction of foliage litter (Table 2). Relatively high rates of decomposition were assumed for the small proportion (23%) of foliage litter assumed to be highly decomposable (see footnote H in Table 2).
Estimates of CF for heavy fuel in high (or low) rainfall zones were 0.17 (or 0.07) and 0.31 (or 0.12) in EDS and LDS fires, respectively (Fensham 2005; Russell-Smith et al. 2009a; Yates et al. 2015). Previous workers have estimated that CF for coarse fuel in high (and low) rainfall zones was 0.15 (or 0.11) and 0.36 (or 0.20) following EDS and LDS fire, respectively (Russell-Smith et al. 2009a; Yates et al. 2015). These workers also estimated CF for fine fuel as 0.74–0.80 and 0.83–0.86 following EDS and LDS fire, respectively, with little differences between rainfall zones.
These CF estimates provided a guide to constraining the FullCAM CF parameters (Table 2) as they were estimates for pools as a whole, whereas FullCAM separately simulates different components of each of these broad categories (Fig. 1 and Supplementary Fig. S1). For example, ‘fine fuel’ in FullCAM includes foliage litter, grass foliage, grass litter and also twigs and bark litter of <0.6 cm diameter, with grass-based pools expected to have relatively high CF compared with the other ‘fine fuel’ components because fire generally occurs when grasses are cured (senesced and dry, particularly in the high rainfall zone), with only the green base of the grass likely to remain unburnt (Meyer and Cook 2015).
Given the percentage of total fine fuel biomass that was attributable to foliage litter was predicted to be 63% ± 6% (average ± s.d.) (Supplementary Material C), it was ensured foliage litter CF was within 5% of previous estimates for fine fuel, with branch and bark (or grass) components of fine fuel having a lower (or higher) CF (Table 2). Similarly, given the percentage of total coarse fuel attributable to branch litter was predicted to be 80% ± 3% (average ± s.d.) (Supplementary Material C), it was ensured that CF for branch litter was within 5% of previous estimates for coarse fuel, but with a higher CF for bark litter applied (Table 2).
Model optimisation and testing
The highest model efficiency for prediction of AGB was found with mortality rates between 1.120 and 2.025% year−1, total fire impacts (=CF + TF) on AGB that were either very low or low for woodland or open forest vegetation types, but moderate to high for shrubland vegetation types, and TF that varied between components of AGB, increasing in the order: stem, branch, bark and foliage (Table 3). Applying these calibrations gave model efficiencies of AGB prediction between 82 and 93%, demonstrating goodaccuracy (Fig. 5).
Vegetation category | Non-fire deaths (% year−1) | Fire impact level | Fire type | Total H impact (%) | CF (and TF), % impact on the live AGB pool | ||||
---|---|---|---|---|---|---|---|---|---|
Stem | Branch | Bark | Leaf | ||||||
WH A | 2.025 F | Low | EDS1 | 0.00 | 0.00 (0.00) | 0.00 (0.00) | 0.00 (0.00) | 0.00 (0.00) | |
EDS2 | 2.50 | 0.50 (2.00) | 0.75 (1.75) | 1.50 (1.00) | 1.75 (0.75) | ||||
EDS3 | 5.00 | 1.00 (4.00) | 1.50 (3.50) | 3.00 (2.00) | 3.50 (1.50) | ||||
LDS1 | 5.00 | 1.50 (3.50) | 2.00 (3.00) | 3.50 (1.50) | 4.00 (1.00) | ||||
LDS2 | 10.0 | 3.00 (7.00) | 4.00 (6.00) | 7.00 (3.00) | 8.00 (2.00) | ||||
LDS3 | 15.0 | 4.50 (10.5) | 6.00 (9.00) | 10.5 (4.50) | 12.0 (3.00) | ||||
WL B | 1.120 G | Very low | EDS1 | 0.00 | 0.00 (0.00) | 0.00 (0.00) | 0.00 (0.00) | 0.00 (0.00) | |
EDS2 | 0.00 | 0.00 (0.00) | 0.00 (0.00) | 0.00 (0.00) | 0.00 (0.00) | ||||
EDS3 | 2.50 | 0.25 (2.25) | 0.50 (2.00) | 1.50 (1.00) | 1.75 (0.75) | ||||
LDS1 | 0.00 | 0.00 (0.00) | 0.00 (0.00) | 0.00 (0.00) | 0.00 (0.00) | ||||
LDS2 | 5.00 | 0.55 (4.45) | 1.50 (3.50) | 3.50 (1.50) | 4.00 (1.00) | ||||
LDS3 | 10.0 | 1.10 (8.90) | 3.00 (7.00) | 7.00 (3.00) | 8.00 (2.00) | ||||
SH C | 2.025 F | High | EDS1 | 5.00 | 1.00 (4.00) | 1.50 (3.50) | 3.00 (2.00) | 3.50 (1.50) | |
EDS2 | 7.50 | 1.50 (6.00) | 2.25 (5.25) | 4.50 (3.00) | 5.25 (2.25) | ||||
EDS3 | 10.0 | 2.00 (8.00) | 3.00 (7.00) | 6.00 (4.00) | 7.00 (3.00) | ||||
LDS1 | 15.0 | 4.5 (10.5) | 6.00 (9.00) | 10.5 (4.50) | 12.0 (3.00) | ||||
LDS2 | 20.0 | 6.00 (14.0) | 8.00 (12.0) | 14.0 (6.00) | 16.0 (4.00) | ||||
LDS3 | 25.0 | 7.50 (17.5) | 10.0 (15.0) | 17.5 (7.50) | 20.0 (5.00) | ||||
SL D | 1.680 F | Moderate | EDS1 | 2.50 | 0.25 (2.25) | 0.50 (2.00) | 1.50 (1.00) | 1.75 (7.50) | |
EDS2 | 5.00 | 0.50 (4.50) | 1.00 (4.00) | 3.00 (2.00) | 3.50 (1.50) | ||||
EDS3 | 7.50 | 0.75 (6.75) | 1.50 (6.00) | 4.50 (3.00) | 5.25 (2.25) | ||||
LDS1 | 10.0 | 1.10 (8.90) | 3.00 (7.00) | 7.00 (3.00) | 8.00 (2.00) | ||||
LDS2 | 15.0 | 1.65 (13.4) | 4.50 (10.5) | 10.5 (4.50) | 12.0 (3.00) | ||||
LDS3 | 20.0 | 2.20 (17.8) | 6.00 (14.0) | 14.0 (6.00) | 16.0 (4.00) | ||||
PL E | 1.680 F | Moderate | EDS2 | 5.00 | 0.50 (4.50) | 1.00 (4.00) | 3.00 (2.00) | 3.50 (1.50) | |
LDS2 | 15.0 | 1.65 (13.4) | 4.50 (10.5) | 10.5 (4.50) | 12.0 (3.00) |
Relationship between observed and predicted AGB that represented the woodland and open forest vegetation types in: (a) Woodland – high rainfall zones (WH); (b) Woodland – low rainfall zones (WL), and (c) shrubland vegetation types, either in high rainfall zones (circle symbols, SH) or low rainfall zones (square symbols, SL). Predictions of AGB were based on the fire intensity assumptions that provided the highest model efficiency (EF) (Table 3). Dashed line represents the one-to-one line. Axis units are Mg of dry mass (DM) per hectare.
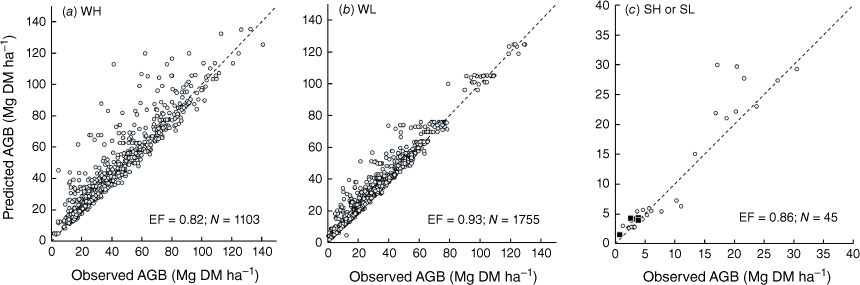
Consistent with Cook et al. (2020), mortality of AGB was higher in the vegetation from high cf. low rainfall zones (Table 3). Within the low rainfall zones, this non-fire related death rate was predicted to be higher in vegetation types dominated by relatively short-lived species (e.g. Acacia and Grevillea), consistent with calibrated mortality rates being higher in stands of Shrubland – Low rainfall (SL) and Pindan (PL) cf. Woodland – Low rainfall (WL).
When CF and TF parameters of AGB (Table 3) were applied in FullCAM, the model predicted that a total (= CF + TF) of between 0 and 25% of AGB is lost through either combustion or transfer to non-living pools, which was within the order of the 1–23% estimate for Australian savannas, albeit for fires of fairly high intensity (Cook et al. 2015a). Using Woodland – High rainfall (WH) calibration sites as a case study, it was demonstrated that the fire impact parameter values were appropriately optimised, as decreasing CF + TF below the calibrated value resulted in an increasing positive bias in predicted AGB (Supplementary Fig. S15).
The relative sensitivity of different vegetation types to fire (Table 3) was generally consistent with the observed average proportion of total above-ground woody biomass that was heavy fuel (Fig. 6). There was evidence that the average proportion of total above-ground woody biomass that is heavy fuel was in turn related to the proportion of AGB that was attributable to fire-susceptible small trees and shrubs (Supplementary Material E). The relatively high total fire impact on AGB for Shrubland – High rainfall (SH) and Pindan (PL) (and presumably also Shrubland – Low rainfall (SL)) vegetation was expected given they have a much greater proportion of shrubs or small multi-stemmed acacias (e.g. Acacia, Calytrix, etc.) compared with the other vegetation types (Supplementary Fig. S11). Shrubs have relatively small diameters, heights and bark thicknesses, which renders them more fire-sensitive than trees (Williams et al. 1999; Lawes et al. 2011; Bond et al. 2012). For example, in plots of SH vegetation, 94% of live stems had a stem diameter at 130cm height (D130) < 20 cm cf. only 67–89% for other vegetation types (data not shown).
Relationship between the relative impact of fire on above-ground biomass (AGB) (Table 3) and the observed proportion of total woody biomass that was heavy fuel (Fig. 7). WH, Woodland – High rainfall; SH, Shrubland – High rainfall; WL, Woodland – Low rainfall; SL, Shrubland – Low rainfall; PL, Pindan.
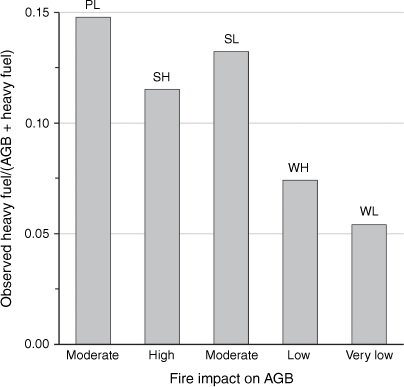
The calibrated total fire impact on AGB was higher for vegetation from high cf. low rainfall zones (Table 3), perhaps for two reasons. First, high rainfall zone vegetation had quite high proportions of stems that were relatively large. Across the calibration dataset (Fig. 5), the high rainfall zone vegetation category had 0.3–2.8% of live stems with D130 > 50 cm, whereas the percentage of stems of this size was ≤0.1% for the other categories of vegetation. Larger trees in Australian savannas are often fire-sensitive as they can burn from the inside when hollow owing to damage from termites or previous fire (Williams et al. 1999; Cook et al. 2005). Secondly, high rainfall zone vegetation has relatively high proportions of fire-sensitive non-eucalypt species relative to the less fire-sensitive eucalypt species (Bond et al. 2012). Although calibration sites used in the present study were not a random sample, across the calibration dataset, stands from high rainfall zones had relatively high proportions of the more fire-sensitive non-eucalypt trees such as Callitris intratropica (e.g. 16% of species found in WH0.6) and Erythrophleum chlorostachys (e.g. 17% of species found in WH0.3) (Supplementary Fig. S11).
The final test of model performance was the assessment of how well predicted pools of fuel matched those observed, with results again indicating model performance was good (Fig. 7). Moreover, with the calibrated parameters given in Table 3, FullCAM-predicted recovery (Supplementary Fig. S4) was consistent with observations (Russell-Smith et al. 2009a; Yates et al. 2015, 2020; Lynch et al. 2018), with increases in fuel with time since last burn being least pronounced with the coarser components (e.g. deadwood, Supplementary Fig. S17a) and most pronounced with the relatively fine components of litter (e.g. foliage litter, Supplementary Fig. S17b, c).
Comparison between observed and predicted fuel biomass (in Mg C ha−1) in: (a) heavy (stags and CWD), (b) coarse, (c) fine, and (d) grass pools, within differing categories of vegetation. Error bars represent standard errors. Numbers and percentages above the bar represent the sample size (N), and proportion of ‘observed’ heavy fuel calculated to be stags. Bars with the same letters were not statistically different according to the Tukey test at 95% confidence interval. Data source is provided in Supplementary Tables S4–S8. Vegetation type definitions are given in Table 1.
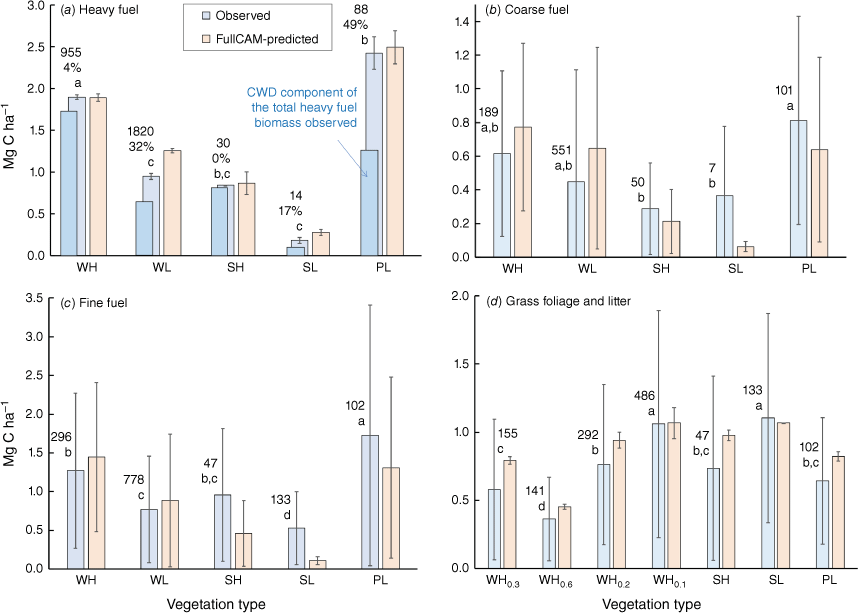
Scenarios of fire management: implications
Land managers can use planned EDS fires to reduce the frequency of high-intensity LDS fires and, to a lesser extent, overall fire frequencies (e.g. Russell-Smith et al. 2013). When such fire management scenarios were simulated with FullCAM using the calibrated parameters (Tables 2 and 3), on average across all vegetation types, 65% of total abatement (range 45–87%) was attributable to sequestration of CO2-C, with the remainder being attributable to avoided emissions (Fig. 8). In regions of high (or low) rainfall, net abatement over a 25-year simulation period was between 0.06 and 0.43 (or 0.02 and 0.05) Mg CO2-e ha−1 year−1 (Fig. 8). But even within a region, variation in this abatement was evident among vegetation types owing to differences in CF of AGB (Table 3) and the productivities of the woody and grass components of the stand (i.e. M and grass cover).
Summary of the impact of changed fire regime (project compared with baseline) on annual average rates (over the 25-year baseline and project periods) of: (a) sequestration of CO2-e and annual average rates (over the 25-year baseline- and project-periods) and (b) avoided fire-induced CO2-e emissions, with these being attributable to five pools: total biomass of woody vegetation, namely above- and below-ground pools, heavy fuel, coarse fuel, fine fuel and grass fuel. Vegetation type definitions are given in Table 1.
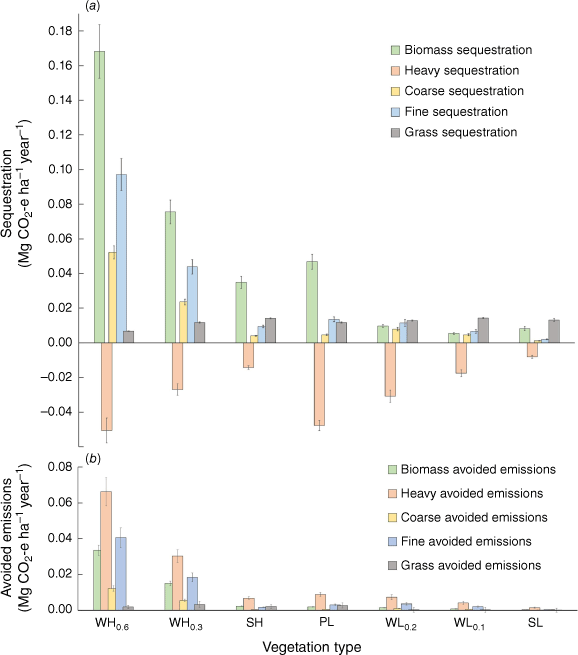
The results from these analyses illustrate potential outcomes across a range of scenarios with differing baseline fire frequencies, and with assumed impacts of management reflected as changes in the relative frequencies of EDS and LDS fire. It is important to note these scenarios are hypothetical and are thus not validated, and do not relate to specific locations or situations. Cook et al. (2015b), using the individual-based FLAMES model, also investigated the impacts of changed fire management on total above-ground carbon stocks and rates of sequestration, with model parameters based on the ‘Three Parks’ monitoring study (Russell-Smith et al. 2009b). Although the simulations of Cook et al. (2015b) explored a greater range of fire frequencies and seasonal fire timings than those considered here, changes in carbon stocks and sequestration rates were broadly similar to our results in terms of both direction and magnitude.
Consistent with the other recent studies (Levick et al. 2019; Werner and Peacock 2019; Murphy et al. 2023), our results indicate that sequestration of carbon in live biomass is a key driver of abatement following savanna fire management, noting that predictions are highly sensitive to the assumed upper limit of AGB, or the M input layer. Although verified for savanna vegetation (Supplementary Fig. S2), the M input layer remains a key source of uncertainty for any given stand, given M may be inaccurate depending on fine-scale spatial variability associated with position within the landscape, and hence, soil nutrients and depth (and thus, water holding capacity) (Supplementary Fig. S6). Also, not all categories of savanna vegetation were represented in the calibration of this input layer (e.g. Pindan). As outlined in Supplementary Material F, in addition to improving predictions of AGB, there are opportunities to collate additional datasets to further constrain calibration of model parameters that are currently highly uncertain owing to the paucity of available data, e.g. rates of decomposition of pools of standing dead and debris. Parameters that could not be constrained owing to negligible available data (mortality, CF and TF of AGB) were optimised in this study, and further work is required to verify these, and where required, constrain them to collected datasets.
As a result of this work, modelling capability has been developed to facilitate the development of a comprehensive methodology encompassing the impacts of different fire types on both avoided emissions and sequestration of carbon, thereby overcoming limitations of existing methods that only account for avoided emissions (Commonwealth of Australia 2015), only account for sequestration in dead (rather than also live) pools (Commonwealth of Australia 2018), or do not distinguish between effects of fires of different intensities (Voluntary Carbon Standard 2015). However, the scenario analysis (Fig. 8) provides only simplistic hypothetical results on abatement (both sequestration and avoided emissions) from implementation of savanna fire management. Work is currently under way to facilitate the spatial application of FullCAM at a project scale (as currently, spatial FullCAM application is limited to national-scale application via the NIR; Commonwealth of Australia 2022). This capacity will provide opportunities to work with project proponents to test FullCAM-predicted abatement implications of actual savanna fire management projects, and compare results with existing methodologies (Commonwealth of Australia 2015, 2018).
Conclusions
The compilation of vast amounts of field datasets for FullCAM calibrations undertaken here provides confidence in predicted dynamics of the composition and quantity of fuel pools in response to different types of savanna fires, resulting in substantially improved accuracy and capability of Australia’s NIR (Commonwealth of Australia 2022), and hence, project-level accounting (Commonwealth of Australia 2015, 2018). The improved capability for accounting for AGB and fuel dynamics and the mosaic patterns associated with patchiness within observed fire scars has enabled biomass in these pools at the time of a fire event to be accurately estimated, thereby increasing the accuracy of emissions estimates. To further improve FullCAM and develop confidence in FullCAM predictions, it will be necessary to compare project‐scale emissions derived under current and proposed methods and, over time, model-predicted sequestration with independently validated measurements. Opportunities for further progressing improvements in FullCAM-predicted fire emissions and sequestration of carbon in savanna ecosystems are outlined in Supplementary Material F.
Data availability
Calibration data were sourced from the published literature, with data summaries and references provided in the Supplementary Tables S3–S9, S11 and S12.
Declaration of funding
The work was funded by the Australian Government’s Department of Climate Change, Energy, the Environment and Water, Canberra.
Acknowledgements
For their guidance and provision of data, we thank Peter Whitehead, Stephen Bray, Cameron Yates, Garry Cook, Mick Meyer, Brett Murphy, Dominique Lynch, Jay Evans and Jeremy Russell-Smith. Max Collett and David Stock are thanked for reviewing an earlier draft.
References
Bond WJ, Cook GD, Williams RJ (2012) Which trees dominate in savannas? The escape hypothesis and eucalypts in northern Australia. Austral Ecology 37, 678-685.
| Crossref | Google Scholar |
Brown S, Mo J, McPherson JK, Bell DT (1996) Decomposition of woody debris in Western Australian forests. Canadian Journal of Forest Research 26, 954-966.
| Crossref | Google Scholar |
Commonwealth of Australia (2015) ‘Carbon Credits (Carbon Farming Initiative – Emissions Abatement through Savanna Fire Management) Methodology Determination 2015.’ (ComLaw, Australian Government: Canberra) Available at https://www.legislation.gov.au/Series/F2015L00344 [verified 13 November 2023]
Commonwealth of Australia (2018) ‘Carbon Credits (Carbon Farming Initiative – Savanna Fire Management – Sequestration and Emissions Avoidance) Methodology Determination 2018.’ (ComLaw, Australian Government: Canberra) Available at https://www.legislation.gov.au/Series/F2018L00562 [verified 13 November 2023]
Commonwealth of Australia (2022) ‘National Greenhouse Gas Inventory Report: 2021. Australian Government submission to the United Nations Framework Convention on Climate Change and its first report under the Paris Agreement. (Australia’s National Greenhouse Accounts. Department of Climate Change, Energy, the Environment and Water: Canberra, Australia) Available at https://www.dcceew.gov.au/climate-change/publications/national-inventory-report-2021 [verified 13 November 2023]
Cook GD, Liedloff AC, Eager RW, Chen X, Williams RJ, O’Grady AP, Hutley LB (2005) The estimation of carbon budgets of frequently burnt tree stands in savannas of northern Australia, using allometric analysis and isotopic discrimination. Australian Journal of Botany 53, 621-630.
| Crossref | Google Scholar |
Cook GD, Jackson S, Williams RJ (2012) A revolution in northern Australia fire management: recognition of Indigenous knowledge, practice and management. In ‘Flammable Australia: fire regimes, biodiversity and ecosystems in a changing word’. (Eds RA Bradstock, AM Gill, RJ Williams) pp. 293–306. (CSIRO Publishing: Melbourne, Australia)
Cook GD, Liedloff AC, Murphy BP (2015a) Towards a methodology for increased carbon sequestration in dead fuels through implementation of less severe fire regions in savannas. In ‘Carbon Accounting and Savanna Fire Management’. (Eds BP Murphy, AC Edwards, M Meyer, J Russell-Smith) pp. 321–328. (CSIRO Publishing: Melbourne, Australia)
Cook GD, Liedloff AC, Murphy BP (2015b) Predicting the effects of fire management on carbon stock dynamics using statistical and process-based modelling. In ‘Carbon accounting and savanna fire management’. (Eds BP Murphy, AC Edwards, M Meyer, J Russell-Smith) pp. 295–320. (CSIRO Publishing: Melbourne, Australia)
Cook GD, Meyer CP, Muepu M, Liedloff AC (2016) Dead organic matter and the dynamics of carbon and greenhouse gas emissions in frequently burnt savannas. International Journal of Wildland Fire 25, 1252-1263.
| Crossref | Google Scholar |
Cook GD, Liedloff AC, Meyer CP, Richards AE, Bray SG (2020) Standing dead trees contribute significantly to carbon budgets in Australian savannas. International Journal of Wildland Fire 29, 215-228.
| Crossref | Google Scholar |
Cuff N, Brocklehurst P (2015) Leaf and coarse fuel accumulation and relationships with vegetation attributes in ‘evergreen’ tropical eucalypt savannas. In ‘Carbon accounting and savanna fire management’. (Eds B Murphy, A Edwards, M Meyer, J Russell-Smith) pp. 169–181. (CSIRO Publishing: Melbourne, Australia)
Dawes TZ (2010) Impacts of habitat disturbance on termites and soil water storage in a tropical Australian savanna. Pedobiologia 53, 241-246.
| Crossref | Google Scholar |
Edwards A, Archer R, De Bruyn P, Evans J, Lewis B, Vigilante T, Whyte S, Russell-Smith J (2021) Transforming fire management in northern Australia through successful implementation of savanna burning emissions reductions projects. Journal of Environmental Management 290, 112568.
| Crossref | Google Scholar | PubMed |
Fensham RJ (2005) Monitoring standing dead wood for carbon accounting in tropical savanna. Australian Journal of Botany 53, 631-638.
| Crossref | Google Scholar |
Giglio L, Boschetti L, Roy DP, Humber ML, Justice CO (2018) The Collection 6 MODIS burned area mapping algorithm and product. Remote Sensing of Environment 217, 72-85.
| Crossref | Google Scholar | PubMed |
Lacey CJ (1974) Rhizomes in tropical eucalypts and their role in recovery from fire damage. Australian Journal of Botany 22, 29-38.
| Crossref | Google Scholar |
Lawes MJ, Adie H, Russell-Smith J, Murphy B, Midgley JJ (2011) How do small savanna trees avoid stem mortality by fire? The roles of stem diameter, height and bark thickness. Ecosphere 2, art42.
| Crossref | Google Scholar |
Lawes MJ, Crisp MD, Clarke PJ, Murphy BP, Midgley JJ, Russell-Smith J, Nano CEM, Bradstock RA, Enright NJ, Fontaine JB, Gosper CR, Woolley LA (2022) Appraising widespread resprouting but variable levels of post-fire seeding in Australian ecosystems: the effect of phylogeny, fire regime and productivity. Australian Journal of Botany 70, 114-130.
| Crossref | Google Scholar |
Levick SR, Richards AE, Cook GD, Schatz J, Guderle M, Williams RJ, Subedi P, Trumbore SE, Andersen AN (2019) Rapid response of habitat structure and above-ground carbon storage to altered fire regimes in tropical savanna. Biogeosciences 16, 1493-503.
| Crossref | Google Scholar |
Liedloff AC, Cook GD (2007) Modelling the effects of rainfall variability and fire on tree populations in an Australian tropical savanna with the Flames simulation model. Ecological Modelling 201, 269-282.
| Crossref | Google Scholar |
Lonsdale WM, Braithwaite RW (1991) Assessing the effects of fire on vegetation in tropical savannas. Australian Journal of Ecology 16, 363-374.
| Crossref | Google Scholar |
Lynch D, Russell‐Smith J, Edwards AC, Evans J, Yates C (2018) Incentivising fire management in pindan (Acacia shrubland): a proposed fuel type for Australia’s savanna burning greenhouse gas emissions abatement methodology. Ecological Management & Restoration 19, 230-238.
| Crossref | Google Scholar |
Mackensen J, Bauhus J, Webber E (2003) Decomposition rates of coarse woody debris – A review with particular emphasis on Australian tree species. Australian Journal of Botany 51, 27-37.
| Crossref | Google Scholar |
McIvor JG (2001) Litterfall from trees in semiarid woodlands of north-east Queensland. Austral Ecology 26, 150-155.
| Crossref | Google Scholar |
Meyer CP, Cook GD, Reisen F, Smith TEL, Tattaris M, Russell‐Smith J, Maier SW, Yates CP, Wooster MJ (2012) Direct measurements of the seasonality of emission factors from savanna fires in northern Australia. Journal of Geophysical Research: Atmospheres 117, 1-14.
| Crossref | Google Scholar |
Murphy BP, Lehmann CER, Russell‐Smith J, Lawes MJ (2013) Fire regimes and woody biomass dynamics in Australian savannas. Journal of Biogeography 41, 133-144.
| Crossref | Google Scholar |
Murphy BP, Whitehead PJ, Evans J, Yates CP, Edwards AC, MacDermott HJ, Lynch DC, Russell‐Smith J (2023) Using a demographic model to project the long-term effects of fire management on tree biomass in Australian savannas. Ecological Monographs 93, e1564.
| Crossref | Google Scholar |
O’Connell AM (1997) Decomposition of slash residues in thinned regrowth eucalpt forest in Western Australia. Journal of Applied Ecology 34, 111-122.
| Crossref | Google Scholar |
Prior LD, Murphy BP, Russell-Smith J (2009) Environmental and demographic correlates of tree recruitment and mortality in north Australian savannas. Forest Ecology and Management 257, 66-74.
| Crossref | Google Scholar |
Rossiter-Rachor NA, Setterfield SA, Hutley LB, McMaster D, Schmidt S, Douglas MM (2017) Invasive Andropogon gayanus (Gamba grass) alters litter decomposition and nitrogen fluxes in an Australian tropical savanna. Scientific Reports 7, 11705.
| Crossref | Google Scholar | PubMed |
Roxburgh SH, Karunaratne SB, Paul KI, Lucas RM, Armston JD, Sun J (2019) A revised above-ground maximum biomass layer for the Australian continent. Forest Ecology and Management 432, 264-275.
| Crossref | Google Scholar |
Russell-Smith J, Edwards AC (2006) Seasonality and fire severity in savanna landscapes of monsoonal northern Australia. International Journal of Wildland Fire 15, 541-550.
| Crossref | Google Scholar |
Russell-Smith J, Yates CP (2007) Australian savanna fire regimes: context, scales, patchiness. Fire Ecology 3, 48-63.
| Crossref | Google Scholar |
Russell-Smith J, Murphy BP, Meyer CP, Cook GD, Maier S, Edwards AC, Brocklehurst P (2009a) Improving estimates of savanna burning emissions for greenhouse accounting in northern Australia: limitations, challenges, applications. International Journal of Wildland Fire 18, 1-18.
| Crossref | Google Scholar |
Russell-Smith J, Edwards AC, Woinarski JCZ, McCartney J, Kerin S, Winderlich S, Murphy BP, Watt FA (2009b) Fire and biodiversity monitoring for conservation managers: a 10-year assessment of the ‘Three Parks’ (Kakadu, Litchfield and Nitmiluk) program. In ‘Culture, ecology and economy of fire management in north Australian savannas: rekindling the Wurrk tradition’. (Eds J Russell-Smith, PJ Whitehead, PM Cooke) pp. 257–285. (CSIRO Publishing: Melbourne, Australia)
Russell-Smith J, Cook GD, Cooke PM, Edwards AC, Lendrum M, Meyer C, Whitehead P J (2013) Managing fire regimes in north Australian savannas: applying Aboriginal approaches to contemporary global problems. Frontiers in Ecology and the Environment 11, e55-e63.
| Crossref | Google Scholar |
Ryan CM, Williams M (2011) How does fire intensity and frequency affect miombo woodland tree populations and biomass? Ecological Applications 21, 48-60.
| Crossref | Google Scholar | PubMed |
Soares P, Tomé M, Skovsgaard JP, Vanclay JK (1995) Evaluating a growth model for forest management using continuous forest inventory data. Forest Ecology and Management 71, 251-265.
| Crossref | Google Scholar |
Surawski NC, Sullivan AL, Roxburgh SH, Meyer CP, Polglase PJ (2016) Incorrect interpretation of carbon mass balance biases global vegetation fire emission estimates. Nature Communications 7, 11536.
| Crossref | Google Scholar | PubMed |
van der Werf GR, Randerson JT, Giglio L, van Leeuwen TT, Chen Y, Rogers BM, Mu M, van Marle M J E, Morton DC, Collatz GJ, Yokelson RJ, Kasibhatla PS (2017) Global fire emissions estimates during 1997–2016. Earth System Science Data 9, 697-720.
| Crossref | Google Scholar |
Voluntary Carbon Standard (2015) VM0029 methodology for avoided forest degradation through fire management. Available at https://verra.org/wp-content/uploads/2018/03/VM0029-Methodology-for-Avoided-Degradation-through-Fire-Management-v1.0.pdf [verified 13 November 2023]
Werner PA, Peacock SJ (2019) Savanna canopy trees under fire: long-term persistence and transient dynamics from a stage-based matrix population model. Ecosphere 10, e02706.
| Crossref | Google Scholar |
Whitehead PJ, Russell-Smith J, Yates C (2014) Fire patterns in north Australian savannas: extending the reach of incentives for savanna fire emissions abatement. The Rangeland Journal 36, 371-388.
| Crossref | Google Scholar |
Williams RJ, Myers BA, Muller WJ, Duff GA, Eamus D (1997) Leaf phenology of woody species in a North Australian tropical savanna. Ecology 78, 2542-2558.
| Crossref | Google Scholar |
Williams RJ, Cook GD, Gill AM, Moore PHR (1999) Fire regime, fire intensity and tree survival in a tropical savanna in Northern Australia. Australian Journal of Ecology 24, 50-59.
| Crossref | Google Scholar |
Yates CP, Russell-Smith J, Murphy BP, Desailly M, Evans J, Legge S, Lewis F, Lynch D, Edwards AC (2015) Fuel accumulation, consumption and fire patchiness in the lower rainfall savanna region, In ‘Carbon Accounting and Savanna Fire Management’. (Eds BP Murphy, AC Edwards, M Meyer, J Russell-Smith) pp. 115–132. (CSIRO Publishing: Melbourne, Australia)
Yates C, MacDermott H, Evans J, Murphy BP, Russell-Smith J (2020) Seasonal fine fuel and coarse woody debris dynamics in north Australian savannas. International Journal of Wildland Fire 29, 1109-1119.
| Crossref | Google Scholar |