SoCal EcoServe: an online mapping tool to estimate wildfire impacts in southern California
Emma C. Underwood


A
B
Abstract
Wildfires in Mediterranean-type climate regions have numerous impacts on the ecosystem services provided by native shrublands, however, quantifying these impacts is challenging.
We developed a reproducible method to quantify fire impacts on ecosystem services and created a tool for resource managers in southern California.
The SoCal EcoServe tool consists of two components: a desktop tool and an online mapping tool. We used the Alisal Fire of 2021 as a case study and quantified: aboveground live carbon storage using pre- and post-fire biomass data; water runoff, groundwater recharge and sediment erosion retention by integrating data on burn severity into hydrological and sediment erosion models; and estimated recreation services and biodiversity using pre-fire data.
We estimated the Alisal Fire resulted in an immediate post-fire reduction in carbon storage of 25%, of which 20% was estimated to be permanently lost. Water runoff increased by 21%, groundwater recharge 7-fold, and sediment erosion increased 24-fold.
The EcoServe tool provides an initial approximation of wildfire impacts that can support damage assessments post-fire, track carbon storage and help identify priorities for post-fire restoration.
We intend the tool to be used by USDA Forest Service resource managers of shurblands in southern California. However, it can provide the framework for future work in shrublands throughout the western USA.
Keywords: Biodiversity, carbon storage, groundwater recharge, recreation, resource management, sediment erosion, shrublands, water runoff.
Introduction
Wildfire is a characteristic of the world’s Mediterranean-type climate regions. In California, the extent of shrubland burned and the frequency of wildfires are of ecological and socio-economic concern, especially given the proximity of shrublands to heavily populated coastal regions (Keeley and Safford 2016; Safford et al. 2022). The environmental impacts of wildfires vary depending on fire characteristics such as intensity, size and frequency (Roces-Díaz et al. 2022). The combustion of shrublands leads to both negative and positive impacts. Most notably, combustion reduces biomass and fuels and the ability of landscapes to sequester carbon from greenhouse gases (Pausas and Keeley 2019). With the removal of vegetation, soil erosion, water runoff and groundwater recharge increase, especially in the first year or two post-fire (Wohlgemuth and Lilley 2018; Flint et al. 2019). In addition, other services such as recreational opportunities on public lands or habitat for wildlife and biodiversity can be temporarily lost.
Understanding and quantifying fire impacts on ecosystem services is increasingly important given potential impacts of future climates on wildfire. Shrublands in southern California experience burning under two scenarios: spring/summer fires where higher fuel moisture and moderate onshore winds restrict the area burned, whereas autumn/winter fires occur when ignitions are influenced by hot strong winds, like the Santa Ana, and the areas burned are extensive (Safford et al. 2022). Warming climates are increasing drought and, as a consequence, are contributing to the expansion of the fire season year-round in California (Diffenbaugh et al. 2015). More drought is increasing shrub mortality and fuel loads, while increasing short-interval fire results in vegetation type conversion and expansion of annual (largely non-native) grasslands providing highly combustible fuels (Safford et al. 2021; Pratt 2022). Moreover, post-fire drought negatively influences seedling survival and growth of resprouting shrubs (Pratt et al. 2014) and thus shrubland recovery.
For resource managers of shrubland-dominated national forests, being able to quantify the impacts of wildfire is important for numerous reasons, including tracking carbon storage and estimating the impacts of wildfire on carbon storage, understanding fuel loads to inform fire models and prescriptive fuel treatments, and identifying sites for post-fire restoration. Furthermore, generating data on wildfire impacts is necessary for post-fire Natural Resource Damage Assessments frequently conducted for fires on public lands, which are used to estimate and acquire cost-recovery funds to complete much needed post-fire restoration.
To date, there has been no systematic method for estimating fire impacts on shrubland ecosystem services: impacts have been estimated with little standardisation between fires. In addition, there has been no tool for resource managers to readily access relevant spatial data associated with ecosystem services. To fill this critical need of resource managers, we developed an online mapping tool that allows users to view, query and download pre- and post-fire data on ecosystem services. The SoCal EcoServe tool (https://manzanita.forestry.oregonstate.edu/EcoServeHome/) provides the first transparent, reproducible and scientifically sound tool for resource managers of shrublands in southern California. It provides an important contribution by packaging and summarising data on ecosystem services and wildfires that have been previously published (e.g. Underwood et al. 2018; Schrader-Patton and Underwood 2021; Schrader-Patton et al. 2022, 2023; Underwood and Hollander 2023). The six ecosystem services included in the tool were identified by resource managers at the beginning of the project as those most valuable to understand for management of southern California shrublands, as well as practical to model within the timeframe of the project. The ecosystem services included are: carbon storage, water runoff, groundwater recharge, sediment erosion, recreation services and biodiversity.
Although other studies have compiled and modelled data on ecosystem services in California (e.g. Chan et al. 2006; Chang and Bonnette 2016) as well as estimated fire impacts on services (e.g. Kinoshita and Hogue 2011; Flint et al. 2019), this is the only tool we are aware of for resource managers to access ecosystem services data associated with impacts of specific wildfires across an ecoregion. SoCal EcoServe currently includes large fires since 2017 that have occurred on public lands and have burn severity data available. To date, the tool has been tested on a limited number of fires and has received positive review by resource managers, and we are now rolling it out to USDA Forest Service (USFS) resource managers more widely across southern California.
In this paper, we describe the methods used to quantify wildfire impacts on ecosystem services and illustrate the tool and data outputs using a case study of the Alisal Fire, which burned on the Los Padres National Forest in 2021. We selected the Alisal Fire as it covered a large spatial area, is dominated by shrub vegetation and the majority of the fire burned at high severity.
Methods
SoCal EcoServe tool
The SoCal EcoServe tool consists of two components: a behind-the-scenes desktop tool, currently managed by select USFS staff responsible for maintaining EcoServe, that prepares the ecosystem services raster data, and an online mapping tool that allows users to query these outputs. The goal of the desktop tool is to process all fires on USFS National Forest lands within the southern California ecoregion (Bailey 2016) (Fig. 1). It requires two inputs: the ecosystem services data in pre-fire condition and burn severity data. More specifically, it uses the fire-induced canopy cover loss produced by the USDA Forest Service’s Rapid Assessment of Vegetation Condition after Wildfire (RAVG), which is produced for all fires over 1000 acres (405 ha) on public lands. In a small number of instances, RAVG data may not be available, e.g. in fires <405 ha (1000 acres) or if running the tool on non-USDA Forest Service lands. As an alternative, the canopy cover loss data can be generated through the use of a Google Earth Engine application (Parks et al. 2018).
Map of southern California displaying the area within which the EcoServe tool can currently be applied. The boundary consists of the Level 4 ecoregions surrounding the four National Forests in southern California.
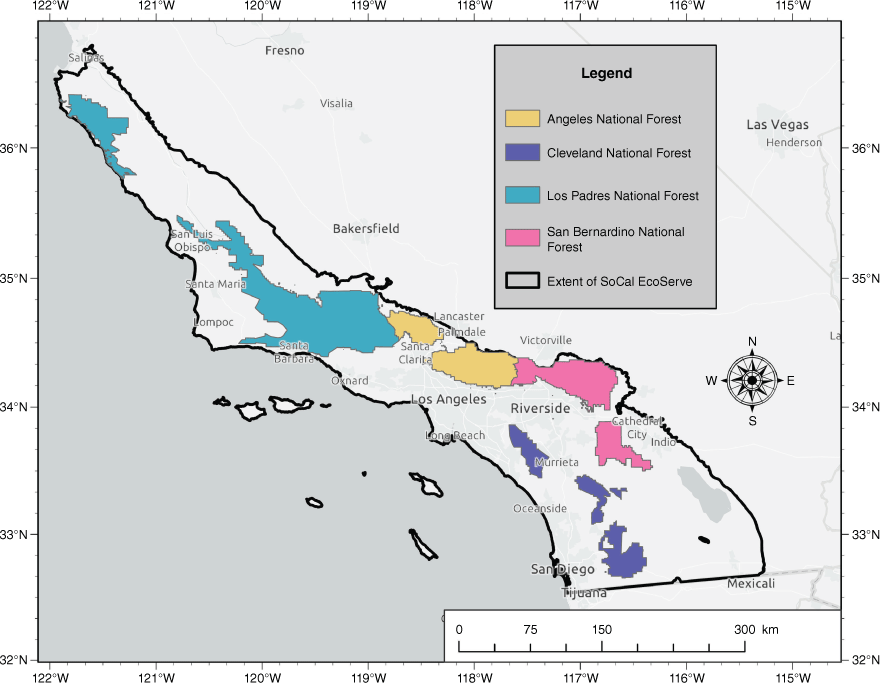
Using a Python script, the desktop tool clips the input ecosystem services raster files to the fire boundary and also to the portion of the fire that is within USFS lands, performs the raster calculations and prepares the tabular outputs as described for each ecosystem service below. Outputs from the desktop tool are compiled into a zip file that is then uploaded by Forest Service staff to the server hosting the online mapping tool.
The EcoServe online mapping tool provides the interface for users to query the ecosystem services data prepared in the desktop tool, view outputs and download data as tables and rasters to include in reports or to conduct further spatial analysis. The tool accounts for all vegetation types within the fire perimeter; however, additional data relating to carbon storage are available for shrublands. The online mapping tool is an ASP.NET Core Model-View-Controller (MVC) application and built using Microsoft Visual Studio 2019 software using C# (C-sharp) programming language along with hypertext markup language (HTML), Cascading Style Sheets (CSS) and Javascript. It is hosted on a Dell PowerEdge R440 server running Windows 2016 Standard, build 10.0.14393. Below, we summarise the ecosystem services data developed for southern California that are used in the tool and provide citations to previous uses of these data.
We illustrate the EcoServe tool using the Alisal Fire, 6868 ha (16 970 acres). The fire was started by lightning on 11 October 2021, and occurred on the Los Padres National Forest in Santa Barbara County.
Carbon storage services
For the user-selected fire, SoCal EcoServe reports carbon storage which has been converted from estimates of aboveground live biomass (AGLBM, 30 m resolution, dataset extends from 2000 to 2021). We developed AGLBM data for southern California as existing biomass estimates for shrublands were limited to one-time data acquisitions and, in some datasets, underestimated shrub biomass values. We used a Random Forest model to analyse a variety of environmental and climatic variables (e.g. elevation, aspect, precipitation) for 766 field plots, primarily from the USDA Forest Service Forest Inventory and Assessment and the Landfire Reference DataBase (Schrader-Patton and Underwood 2021; Schrader-Patton et al. 2022, 2023). Cross-validation using reserved plot data showed our model had an R2 of 0.51 and an uncertainty of 905 g m−2 (Schrader-Patton and Underwood 2021; Schrader-Patton et al. 2023). Two of the model input variables were generated on an annual basis, the Normalized Difference Vegetation Index (NDVI) and annual precipitation, thereby allowing the biomass model to be rerun every year to provide a stack of biomass data from 2000 to 2022. Using the AGLBM estimates, we also developed a methodology to estimate other shrub biomass pools including standing dead, litter and belowground biomass based on field data reported in the literature and other spatial datasets (full details in Schrader-Patton et al. 2022). To estimate carbon storage, we converted vegetative biomass measurements to carbon by multiplying by 0.47 (McGroddy et al. 2004).
To report on carbon storage in the tool for a specific fire, we cross-referenced the start month of the selected fire with the biomass dataset. For fires that burned between 1 January and 31 June, we used the July-August AGLBM from the previous year, given that the primary shrubland growing season is March through June, the previous year better represents pre-fire conditions. For fires that burned between 1 September and 31 December, we used the July-August AGLBM of the current year. In addition to reporting the pre- and post-fire carbon storage for the year of the specific fire, we also report an average carbon storage using biomass data from 2001 to 2007, which captures a range of drought and non-drought climate years in southern California (see Schrader-Patton and Underwood 2021 for details).
To report the post-fire change in carbon storage for a specific fire, we use the biomass raster (same source) for the year following the fire. However, as shrubland biomass recovers quickly post-fire under normal conditions, with estimates ranging from 8 to 12 years (Black 1987; Bohlman et al. 2018), we also calculated the amount of biomass that is ‘permanently lost’, i.e. unlikely to recover. We identified pixels with low regeneration potential and, therefore, unlikely to recover pre-fire shrub biomass given their fire history (Underwood and Hollander 2023). We did this by querying each pixel using two criteria: either three or more fires in the last 40 years or less than 10 years since the last fire, based on findings in the literature (Zedler et al. 1983; Syphard et al. 2019). We assumed the biomass of the qualifying pixels would be ‘permanently lost’ (as documented in the referenced studies) and consequently replaced by annual (non-native) grasses with a lower biomass value. To determine this grassland biomass value, we queried the AGLBM data from 2000 to 2022 in areas classified as annual grasslands in a statewide vegetation map (FRAP 2015) to provide an average AGLBM value of annual grasslands of 0.14 kg m−2. We calculated the amount of aboveground carbon storage permanently lost post-fire as the difference between the pre-fire shrubland carbon storage value and the post-fire annual grassland carbon storage value.
Water runoff and groundwater recharge services
We used the Basin Characterisation Model (BCM) (Flint et al. 2013) to provide data on water runoff and groundwater recharge. The BCM is a regional water-balance model that generates gridded runoff and recharge rasters using vegetation-specific actual evapotranspiration, soil characteristics, and precipitation and temperature specific to each water year. The BCM is unique in its ability to partition available water into recharge and runoff based on spatial variations in bedrock permeability and to be run for large spatial areas, providing the opportunity to study the impacts of disturbance – such as wildfire – and climate (Flint et al. 2013). The BCM has been used in a wide range of studies in California including evaluating water resources, biodiversity, wildfire and forest health, and is accepted to be the most appropriate model at the watershed scale, with outputs validated using streamflow measurements from 159 largely unimpaired watersheds in California (CalWater 1999; Flint et al. 2013).
Wildfires exert a strong influence on the hydrological conditions of a watershed, particularly when occurring at high severity (which characterise shrubland fires in California) and can produce hydrological changes in a watershed beyond the range of historic variation (DeBano et al. 1998; Neary et al. 2005). With fire, removal of vegetation reduces transpiration and evaporation, which increases water runoff and groundwater recharge (Flint et al. 2013, 2019). In addition, the partial combustion of litter and redeposition of vaporised waxy substances from the leaves into cooler soil below, can create hydrophobic soils that reduce infiltration (DeBano 1981; Wohlgemuth and Lilley 2018). Previous studies have used burn severity to estimate the impacts of fire on hydrological services (Neary et al. 2011).
In addition to wildfire, climatic conditions can also have a substantial influence on recharge and runoff: when drought is severe, runoff and recharge can decrease instead of increase post-fire (Flint et al. 2019). To compensate for this, we averaged the BCM outputs over 12 years that are considered to be representative of the climate from 2000 to 2022 in terms of their drought conditions as indicated by the Palmer Drought Severity Index for the south coast ecoregion of California (2000, 2003, 2005, 2006, 2008, 2009, 2014, 2015, 2016, 2017, 2020 and 2021).
Normalised pre-fire estimates of surface runoff and groundwater recharge, assuming undisturbed vegetation conditions, were calculated under the average climatic conditions described above. To estimate the impacts of fire, we reran the BCM for each year from 2000 to 2021, integrating data on fire-induced canopy cover loss from the RAVG data. The five RAVG canopy cover classes were cross-walked to a single value for each class (0, 20, 40, 60 and 80%) and the BCM rerun to integrate this value as the amount of vegetation lost post-fire, e.g. if canopy cover loss was 75–100% change (midpoint 80%), we reran the BCM with 20% canopy cover. We then calculated the average of each canopy cover class run over the 12 years (specified above). Based on the fire-induced canopy cover loss data for a target fire, the normalised post-fire runoff and recharge quantities reported were taken from these 12-year average outputs.
Sediment erosion services
Estimates of sediment erosion used InVEST’s sediment erosion model (Hamel et al. 2015), which can be parameterised in a straightforward way by integrating data on landform, climate, soil and vegetation. The sediment erosion model uses the Universal Soil Loss Equation (Wischmeier and Smith 1978) to predict erosion, sedimentation and retention. It can simulate how changes in vegetation associated with landscape disturbances such as fire can affect sediment yield. It has been widely used for modelling soil loss and sediment yield in a variety of countries and ecosystems (e.g. Zhou et al. 2019; Gashaw et al. 2021) and has been calibrated to predict post-fire sediment yield at the watershed scale in the western US using sediment erosion measurements compiled from the literature (Moody and Martin 2009; Sankey et al. 2015). Sankey et al. (2015) found the model could accurately predict post-fire sediment yield by adjusting vegetation cover and the erodibility factor in the model.
To apply the sediment erosion module in southern California, we integrated input data for southern California including a soil erodibility data layer from county-scale soil maps (USDA Natural Resources Conservation Service Soil Service Geographic Database [SSURGO]) and vegetation cover data (which influences soil erosion) from NDVI data (2014–2015). Pre-fire estimates of sediment erosion reported in the tool use the average InVEST outputs calculated over the 12 years listed in the previous section for hydrological services. Similarly to water runoff and groundwater recharge, post-fire values were generated by rerunning the sediment erosion module and integrating data on percentage canopy cover loss from the RAVG data. Again, we calculated the average of each canopy cover class run over the 12 years and report these according to the fire-induced canopy cover loss for each target fire.
Recreation services
The EcoServe tool is also able to report on recreation services using data for recreation sites from a national scale recreation database that contains spatial data on recreational sites, areas, activities and facilities on USFS public lands (USFS Geodata Clearinghouse; https://data.fs.usda.gov/geodata/help.php), as well as recreation sites surveyed by the USDA Forest Service National Visitor Use Monitoring Survey (NVUM; USDA Forest Service 2015; English et al. 2002). For the subset of recreation sites that were NVUM sites, the tool reports the predicted total number of visits per year to each site developed using a recreation demand model (Garnache and Lupi 2018). The assumption is that wildfire will result in the closure of all recreation sites within the target fire during containment; however, the length of closure time is undetermined and may be more or less than 1 year depending on the degree of fire-related damage and conditions.
Biodiversity services
Given the challenges of understanding the impacts of wildfire on biodiversity, SoCal EcoServe only presents this service in its pre-fire condition. We created an overall biodiversity index using Marxan conservation planning software (Ball et al. 2009). Marxan has been used to support reserve design in many types of ecosystems around the world and is the most widely used conservation planning software. The software uses a simulated annealing algorithm to explore configurations of planning units to identify a solution that meets the input conservation objectives in low-cost ways. For the purposes of generating an output for the EcoServe tool, we identified a list of conservation targets in discussion with USFS resource managers, including: sensitive species identified in national forest plans, wildlife habitat linkages and watersheds with high-value aquatic biota (see details in Underwood et al. 2018). For each target, we assigned a conservation goal. The Marxan output is an irreplaceability index for each planning unit that comprises the most suitable solutions to meet the specified conservation objectives. The EcoServe tool presents tabular results for the target fire as well as for each of the four southern National Forests (Angeles, Cleveland, Los Padres and San Bernardino) to allow comparisons with the broader landscape.
Hotspots of change in ecosystem services
To assist in identifying areas of potential interest for post-fire activities such as restoration, the tool quantifies the amount of change in carbon storage, water runoff, groundwater recharge and sediment erosion services. For the latter three services, the change raster is generated by using the 12-year average pre-fire and the 12-year average post-fire rasters:
The resulting change raster is then rendered into the following classes: ≤0, 0–20, 20–40, 40–60, 60–80, 80–90, 90–95, 95–100 and >100%. A table in the tool reports the area of fire that experienced >80% increase in runoff, recharge, or sediment erosion. For carbon storage, hotspots of change are calculated as the area of pixels in the fire perimeter identified with low regeneration potential (see Carbon storage services above).
Results
We obtained the Alisal Fire perimeter and burn severity raster data from the Burn Severity Portal (https://burnseverity.cr.usgs.gov/) and created the pre- and post-fire ecosystem services data using the desktop tool, and uploaded outputs to the EcoServe server. A resource manager accesses spatial data and estimates of fire impacts by opening the online mapping EcoServe tool (https://manzanita.forestry.oregonstate.edu/EcoServeHome/). On starting the tool, the user is presented with a map of the southern California ecoregion and options to add viewable layers, e.g. National Forest boundaries, hydrological unit boundaries (Fig. 2). A user then selects the year, fire (either entire perimeter or portion of fire on National Forest land), and one of six ecosystem services to quantify or ‘hotspots of change’ (Fig. 2). In the map display for carbon storage, surface runoff, groundwater recharge and sediment erosion, the user can toggle between viewing spatial data in pre-fire and post-fire condition, RAVG burn severity data and (for carbon storage) pixels identified with low regeneration potential.
The initial page of the SoCal EcoServe tool, showing three steps for selecting year, fire and service to query, along with options for changing map background layers. Red boundary shows the Alisal Fire (2021).
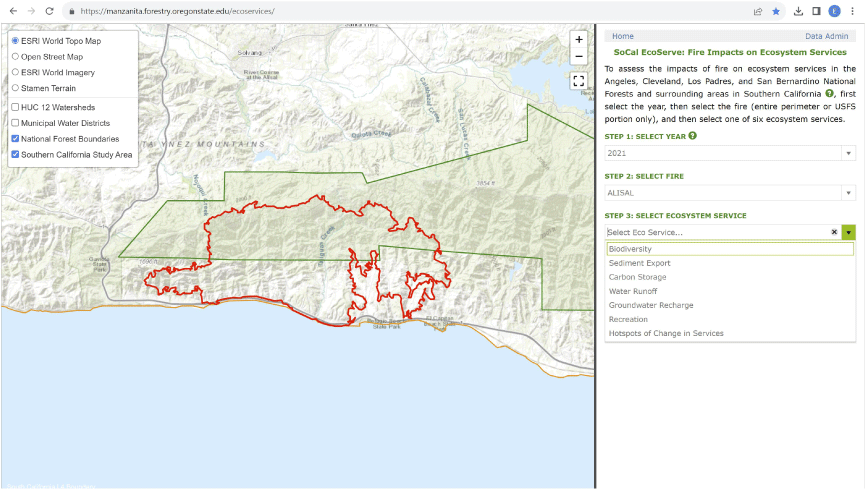
The outputs for carbon storage services in the EcoServe tool include a table summarising data of pre- and post-fire carbon storage for five different biomass pools (Table 1) and corresponding bar chart; summary of major shrub communities in the fire; and the amount of carbon ‘permanently lost’ post-fire. The majority of the Alisal Fire consisted of shrub (69%), with mixed chaparral (54%) dominating, followed by coastal sage scrub (14%).
Carbon pool | Carbon pre-fire (metric tons) | 1 year post-fire (metric tons) | |
---|---|---|---|
Aboveground live | 26 920 | 15 870 | |
Standing dead | 26 924 | 25 318 | |
Litter | 24 803 | 20 163 | |
Belowground | 24 298 | 15 590 | |
Total All vegetative pools | 102 944 | 76 941 | |
Total All vegetative pools average (2001–2007) | 99 130 | – |
The carbon stored in vegetative carbon pools in the Alisal Fire pre-fire totalled 102 944 metric tons, which was slightly higher than the 7-year average (99 130 metric tons). The aboveground live biomass and standing dead pools contributed relatively more than the belowground and litter (Table 1, Fig. 3). Using biomass data for the post-fire year (2022), carbon storage decreased by 25% across all vegetative pools to 76 941 metric tons (Table 1). Recognising that shrubland that experiences high-frequency short-interval fire is unlikely to recover and is vulnerable to conversion to annual grassland (Zedler et al. 1983; Syphard et al. 2019), we estimated 13 831 metric tons or 20% of the pre-fire total vegetative biomass could be permanently lost based on the identification of pixels with low regeneration potential according to fire history. The Alisal Fire had 1344 ha (3322 acres or 20% of the fire) with low regeneration pixels owing to the occurrence of the Sherpa Fire (2016) that occurred in the eastern portion of the Alisal burn perimeter.
Graphical outputs of EcoServe tool for the Alisal Fire showing different vegetative carbon pools pre- and post-fire.
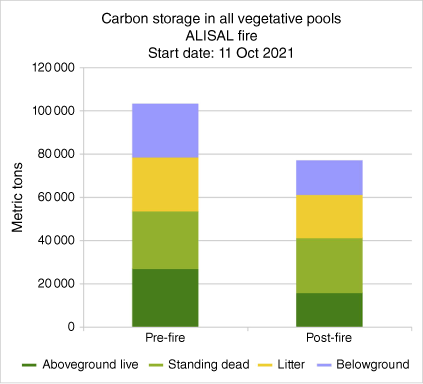
For runoff, recharge and sediment erosion services that integrated the RAVG burn severity data, the tabular outputs reported in the EcoServe tool include the area within each canopy cover loss class. For the Alisal Fire, 57% experienced 75–100% canopy cover loss; 11% of the fire experienced 1–25% canopy cover loss; and 6% of the fire experienced 25–50 and 50–75% loss each (Fig. 4).
EcoServe tool showing outputs for water runoff pre- and post-fire for the Alisal Fire and reported in table by different canopy cover loss classes in the RAVG burn severity data. Map image also shows display options including pre-fire, post-fire water runoff and burn severity data.
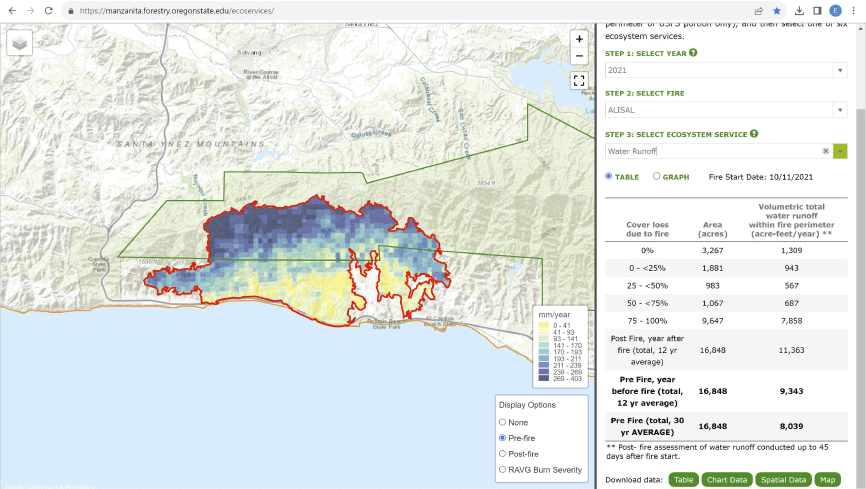
The difference between pre-fire and post-fire (normalised) change in water runoff for the Alisal Fire (calculated based on the 12-year average) showed an increase of 21%, from 1154 to 1401 ha metres year−1 (Table 2, note the tool reports runoff and recharge in acre feet per year but here we report in SI units of ha metres a year). The increase in groundwater recharge was greater, increasing seven times from 73 to 560 ha metres year−1 (Table 2). Sediment erosion increased by 24-fold from an average pre-fire estimate of 5499 m3 year−1 to an average post-fire estimate of 131 407 m3 year−1 (Table 2). The difference in hydrological and sediment erosion services can also be viewed in graphical format in the tool (Fig. 5). In the case of the Alisal Fire, there were no recreation sites contained in the two data sources integrated into the EcoServe tool. However, in other shrubland fires currently integrated in the tool, the number of recreation sites ranges from 1 to 17.
Canopy cover loss (%) | Area (ha) | Runoff (ha metres year−1) | Recharge (ha metres year−1) | Sediment erosion (m3 year−1) | |
---|---|---|---|---|---|
0 | 1308 | 161 | 26 | 1431 | |
0–<25 | 757 | 116 | 19 | 12 770 | |
25–<50 | 397 | 70 | 16 | 7958 | |
50–<75 | 431 | 85 | 31 | 9410 | |
75–100 | 3900 | 969 | 468 | 99 773 | |
Post-fire (average) | 1402 | 560 | 131 407 | ||
Pre-fire (average) | 1154 | 73 | 5499 |
Graphical outputs of EcoServe tool for the Alisal Fire showing water runoff pre- and post-fire including average pre-fire for comparison.
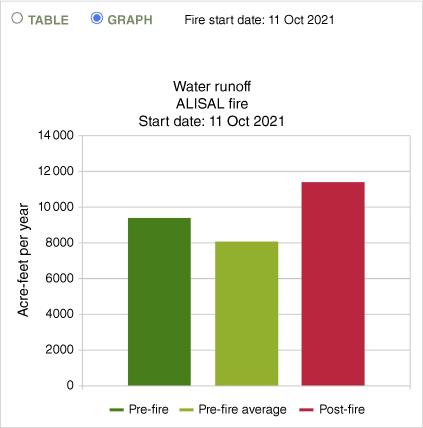
The mean Marxan biodiversity score for the Alisal Fire was 27 out of a maximum irreplaceability score of 100. Highest biodiversity scores were mapped along the southern edge of the fire perimeter (Fig. 6). The table output compares biodiversity information for the Alisal Fire with the four southern National Forests (Fig. 6): the mean Marxan score ranked third after the San Bernardino and Cleveland National Forests. The Alisal Fire had rare and native species richness levels similar to the four National Forests (California Department of Fish and Wildlife’s Areas of Conservation Emphasis Map data 2010, https://map.dfg.ca.gov/ace/) (table in Fig. 6).
Biodiversity output from Marxan showing irreplaceability of planning units within the Alisal Fire. Tabular results compare the Alisal Fire with the four southern National Forests.
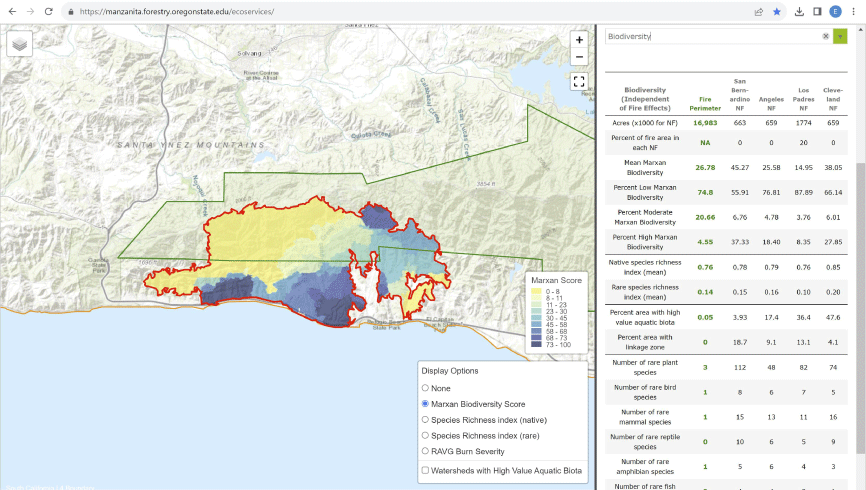
The ‘hotspots of change’ calculation showed the majority of the Alisal Fire was impacted by high change (defined as >80% change) in groundwater recharge and sediment erosion (78% and 74% respectively, Fig. 7), whereas only 1% of the fire experienced high change in water runoff. As reported previously under post-fire carbon storage, the EcoServe tool identified 20% of the fire (1 344 ha) with low regeneration potential. These data appear in tabular format in the tool and the change rasters can be viewed in the map window (Fig. 7).
Table (right) reporting change in ecosystem services in Alisal Fire. Map (left) showsoptions for map display for the hotspots of change selection.
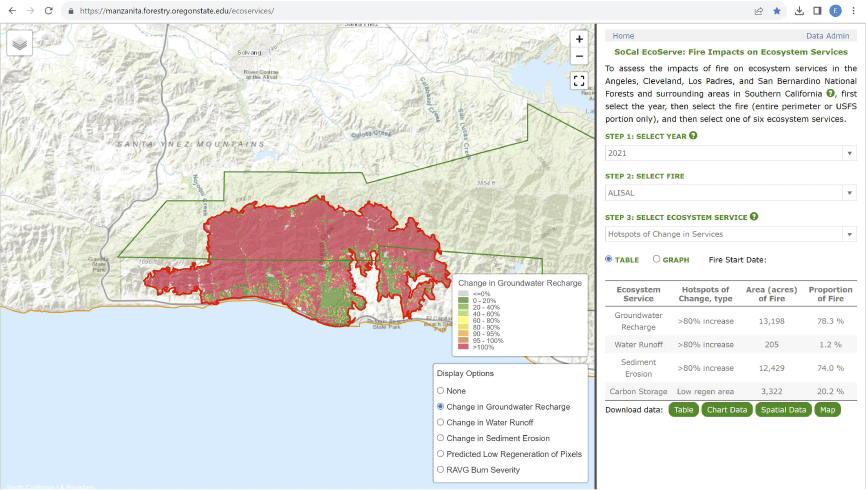
Finally, a range of data presented in the EcoServe online mapping tool can be downloaded for reports or conducting additional spatial analyses. Data include: pre- and post-fire raster spatial data and change data for carbon storage, runoff, recharge and sediment erosion, and chart and tabular data can be downloaded for each service. For biodiversity, the downloadable table includes additional information such as a list of rare species by taxonomic groups (California Natural Diversity Database, https://www.wildlife.ca.gov/Data/CNDDB).
Discussion and conclusion
We have described the input data on ecosystem services and the methodology used in the SoCal EcoServe tool to quantify the impacts of fire in shrublands in southern California and report outputs using the Alisal Fire as a case study. The outputs generated by EcoServe provide important data for resource managers to quantify fire impacts for assessing damages to natural resources on National Forest lands. This was identified as a need by resource managers and will become increasingly important given that future climates will result in higher fuel loads from drought-related shrub mortality and vegetation type conversion associated with drought, fire and nitrogen deposition (Allen et al. 2018; Safford et al. 2021; Pratt 2022).
Outputs from the EcoServe tool showed the Alisal Fire reduced carbon storage by 25% across all vegetative biomass pools (Table 1) and approximately one-fifth of this (1344 metric tons) is estimated not to recover and be permanently lost. When interpreting the change in carbon storage post-fire, it is important to note the time between the fire occurrence (in this case 11 October 2021; note ‘Fire start date’ in Fig. 3) and the date of the post-fire AGLBM data (July-August 2021). For the Alisal Fire, there were 9 months for shrub vegetation to recover post-fire. This should be noted when comparing change in carbon storage between different fires; for example, a spring fire will have fewer months for post-fire recovery before the July-August date of the post-fire biomass data.
In applying the EcoServe tool to the Alisal Fire, there are a number of discussion points associated with outputs of the different ecosystem services. First, the Alisal Fire had a notable increase in water runoff of 21%, which is expected with the reduction in evaporation and transpiration as vegetation is removed. To provide context for this output, other shrub-dominated fires in southern California have experienced water runoff increases between 8 and 46% post-fire (E. Underwood, unpubl. data). However, for fires occurring in years with severe or extreme drought conditions, runoff (and recharge) have been found to decline (versus increase) post-fire. This was a key motivation for reporting the average runoff and recharge over 12 years, which capture a range of climatic conditions. For groundwater recharge, the 12-year pre-fire average for the Alisal Fire is similar on a per hectare basis to other shrub-dominated fires in the tool such as the Willow (2021), Thomas (2017), Whitter (2017) and Valley (2020) fires. Groundwater recharge increased seven times post-fire which, in part, is explained by it occurring on excessively drained soils (Maymen series) that will have relatively more recharge when the transpiring plant cover is removed.
Second, the substantial increase in sediment erosion after the Alisal Fire is a pattern found in other shrub-dominated fires both in 2021 and in other years. One caveat associated with the InVEST model is that it only considers annual precipitation and does not account for the intensity of individual winter storm events that characterise southern California and cause substantial erosion. Furthermore, the loss of soil also reduces ecosystem productivity upslope as well as impacts infrastructure downstream, which are not reflected in this estimate of impact.
Third, there were no recreation sites reported for the Alisal Fire using the two datasets currently in the EcoServe tool which, in part, reflects the fairly remote location of the fire. However, there may well be recreation facilities affected but just not contained in these datasets. Fourth, in interpreting the summary of the biodiversity data, it is important to note that the output is dependent on the individual biodiversity layers entered into the Marxan software. Different conservation targets and associated goals would result in different solutions. Furthermore, the input biodiversity data originated in different years.
Finally, in relation to areas identified as ‘hotspots of change’ post-fire, there is variation in the amount of area identified for each ecosystem service. This is, in part, due to the services being generated by different models and approaches (i.e. water runoff and groundwater recharge from the Basin Characterisation Model and sediment erosion retention from InVEST). In addition, it is important to note that this is only one way to define hotspots; other approaches could include integrating ecosystem services data with fire frequency, climatic water deficit data, or non-native species, which could be incorporated into future versions of the tool.
Currently, the outputs from the EcoServe tool report post-fire impacts over the short term, which is often sufficient for quantifying some immediate environmental damages to resources. However, we also recognise that understanding longer-term impacts is equally important for managing shrublands. We have taken steps to do this for carbon storage in two ways. First, we calculate the permanent loss of carbon storage over the long-term given fire history data and second, we include in the tool a link to a graph showing the trajectory of biomass recovery for mixed chaparral shrubland. One challenge in projecting recovery is the difference in temporal scales for each service. For example, in the spring following shrubland fires, a flush of wildflowers can attract visitors (Safford et al. 2018), even though recreation facilities and infrastructure may take much longer to be usable; similarly, hydrological services are estimated to recover 2–4 years post-fire however have been found to be more sensitive to climate (Flint et al. 2019).
Climate, particularly drought conditions, is a major challenge for developing pre- and post-fire data relating to carbon storage, hydrology and sediment erosion. We address the year-to-year variations in these services by reporting the pre-and-post-fire values for these services using a 12-year average climatic condition. This approach has the advantage of facilitating comparisons of fires occurring in different years, increasing the influence of burn severity (RAVG) data over climate on determining fire impacts, and allowing fire impacts to be calculated as soon as burn severity data are available.
SoCal EcoServe is primarily a compilation and aggregation tool for existing ecosystem services data that have been previously published. The use of burn severity data to capture fire impacts on biomass, hydrology and sediment erosion has appeared in previous studies (e.g. Neary et al. 2011; Sankey et al. 2015). Ideally, a quantitative landscape-level assessment of the ecosystem services in pre- and post- fire condition generated by the EcoServe tool is required to conduct a full validation of outputs; however, this would be challenging in practice (e.g. it would require a priori knowledge of a fire perimeters or fires located in the identical geographies burning at different severities to measure). However, we welcome the opportunity to fully validate EcoServe results should appropriate data become available. Development of spatial ecosystem services data is an active area of research and we are open to future datasets and methods that could contribute to capturing pre- and post-fire conditions. That said, we believe the data and methods used in EcoServe are currently the best available for generating data on fire impacts to shrub-dominated ecosystems in southern California.
The spatial data on ecosystem services within the current EcoServe tool are limited to the southern California ecoregion (Fig. 1). However, the same tool framework can be utilised if data on ecosystem services inputs are generated for shrublands in different areas of California or the western US, including non-National Forest lands if burn severity data are generated through alternative means (e.g., Park). The EcoServe tool outputs can be used to determine the loss of ecosystem services to inform natural resource damage assessments, help prioritise restoration activities post-fire (e.g. focus on high fire impactareas or hotspots of change), identify areas vulnerable to type-conversion to non-native grasses (using the data on low biomass regeneration potential), and, ultimately, contribute to supporting resource managers to ensure the provision of ecosystem services over the long term.
Data availability
The SoCal EcoServe tool and associated ecosystem services data are available for selected fires at: https://manzanita.forestry.oregonstate.edu/EcoServeHome/, along with methods and caveats for the approach.
Acknowledgements
We thank Lorrie Flint and Alan Flint for providing BCM data and Chloe Garnache and Frank Lupi for contributions to the recreation component. We are grateful to Hugh Safford, Nicole Molinari and Ken Bunzel for assistance in developing the SoCal EcoServe tool.
References
Bailey RG (2016) ‘Bailey’s ecoregions and subregions of the United States, Puerto Rico, and the US Virgin Islands.’ (US Forest Service Research Data Archive: Fort Collins, CO, USA) doi:10.2737/RDS-2016-0003
Bohlman GN, Underwood EC, Safford HD (2018) Estimating biomass in California’s chaparral and coastal sage scrub shrublands. Madroño 65, 28-46.
| Crossref | Google Scholar |
Chan KMA, Shaw MR, Cameron DR, Underwood EC, Daily GC (2006) Conservation planning for ecosystem services. PLoS Biology 4(11), e379.
| Crossref | Google Scholar | PubMed |
Chang H, Bonnette MR (2016) Climate change and water-related ecosystem services: impacts of drought in California, USA. Ecosystem Health and Sustainability 2(12), e01254.
| Crossref | Google Scholar |
Diffenbaugh NS, Swain DL, Touma D (2015) Anthropogenic warming has increased drought risk in California. Proceedings of the National Academy of Sciences 112(13), 3931-3936.
| Crossref | Google Scholar | PubMed |
Flint LE, Flint AL, Thorne JH, Boynton R (2013) Fine-scale hydrologic modeling for regional landscape applications: the California Basin Characterization Model development and performance. Ecological Processes 2, 25.
| Crossref | Google Scholar |
Flint LE, Underwood EC, Flint AL, Hollander AD (2019) Characterizing the influence of fire on hydrology in southern California. Natural Areas Journal 39(1), 108-121.
| Crossref | Google Scholar |
FRAP (Fire and Resource Assessment Program) (2015) California Department of Forestry and Fire Protection’s CALFIRE Fire and Resource Assessment Program (FRAP). Fveg15_1 vegetation data. Available at http://frap.fire.ca.gov/data/frapgisdata-sw-fveg_download
Garnache C, Lupi F (2018) The Thomas Fire and the effect of wildfires on the value of recreation services in Southern California. In ‘Agricultural and Applied Economics Association Annual Meeting’, 5–7 August 2018. (Washington, DC, USA). https://ideas.repec.org/p/ags/aaea18/274028.html
Gashaw T, Bantider A, Zeleke G, Alamirew T, Jemberu W, Worqlul AW, et al. (2021) Evaluating InVEST model for estimating soil loss and sediment export in data scarce regions of the Abbay (Upper Blue Nile) Basin: implications for land managers. Environmental Challenges 5, 100381.
| Crossref | Google Scholar |
Hamel P, Chaplin-Kramer R, Sim S, Mueller C (2015) A new approach to modeling the sediment retention service (InVEST 3.0): case study of the Cape Fear catchment, North Carolina, USA. Science of the Total Environment 524–525, 166-177.
| Crossref | Google Scholar | PubMed |
Kinoshita AM, Hogue TS (2011) Spatial and temporal controls on post-fire hydrologic recovery in southern California watersheds. Catena 87(2), 240-252.
| Crossref | Google Scholar |
McGroddy ME, Daufresne T, Hedin LO (2004) Scaling of C:N:P stoichiometry in forests worldwide: implications of terrestrial Redfield‐type ratios. Ecology 85, 2390-2401.
| Crossref | Google Scholar |
Moody JA, Martin DA (2009) Synthesis of sediment yields after wildland fire in different rainfall regimes in the western United States. International Journal of Wildland Fire 18, 96-115.
| Crossref | Google Scholar |
Neary DG, Koestner KA, Youberg A (2011) Hydrologic impacts of high severity wildfire: learning from the past and preparing for the future. In ‘24th Annual Symposium of the Arizona Hydrological Society; Watersheds near and far: Response to changes in climate and landscape’, 18–20 September 2010. (Flagstaff, AZ, USA) https://www.fs.usda.gov/research/treesearch/40608#:~:text=Basins%20with%20high%2Dburn%20severity,greater%20than%20pre%2Dfire%20conditions
Parks SA, Holsinger LM, Voss MA, Loehman RA, Robinson NP (2018) Mean composite fire severity metrics computed with Google Earth Engine offer improved accuracy and expanded mapping potential. Remote Sensing 10, 879.
| Crossref | Google Scholar |
Pausas JG, Keeley JE (2019) Wildfires as an ecosystem service. Frontiers in Ecology and the Environment 17, 289-295.
| Crossref | Google Scholar |
Pratt RB (2022) Vegetation‐type conversion of evergreen chaparral shrublands to savannahs dominated by exotic annual herbs: causes and consequences for ecosystem function. American Journal of Botany 109, 9-28.
| Crossref | Google Scholar | PubMed |
Pratt RB, Jacobsen AL, Ramirez AR, Helms AM, Traugh CA, Tobin MF, Heffner MS, Davis SD (2014) Mortality of resprouting chaparral shrubs after a fire and during a record drought: physiological mechanisms and demographic consequences. Global Change Biology 20, 893-907.
| Crossref | Google Scholar | PubMed |
Roces-Díaz JV, Santín C, Martínez‐Vilalta J, Doerr SH (2022) A global synthesis of fire effects on ecosystem services of forests and woodlands. Frontiers in Ecology and the Environment 20(3), 170-178.
| Crossref | Google Scholar |
Safford HD, Butz RJ, Bohlman GN, Coppoletta M, Estes BL, Gross SE, Merriam KE, Wuenschel A (2021) Fire ecology of the North American Mediterranean-climate zone. chapter 7. In ‘Fire ecology and management: past, present, and future of US forested ecosystems’. (Eds B Collins, CH Greenberg) pp. 337–392. (Springer: Cham, Switzerland).
Safford HD, Paulson AK, Steel ZL, Young DJN, Wayman RB (2022) The 2020 California fire season: a year like no other, a return to the past, or a harbinger of the future? Global Ecology and Biogeography 31, 2005-25.
| Crossref | Google Scholar |
Sankey JB, McVay J, Kreitler J, Hawbaker T, Vaillant N, Lowe S (2015) Predicting watershed post-fire sediment yield with the InVEST sediment retention model: accuracy and uncertainties. In ‘Conference paper: 5th Federal Interagency Hydrologic Modeling Conference and the 10th Federal Interagency Sedimentation Conference’, Reno, NV, USA. (Published by the Joint Federal Interagency Conference, Reno, NV, USA) acwi.gov/sos/pubs/3rdJFIC/Proceedings.pdf
Schrader-Patton CC, Underwood EC (2021) New biomass estimates for chaparral-dominated southern California landscapes. Remote Sensing 13, 1581.
| Crossref | Google Scholar |
Schrader-Patton CC, Underwood EC, Sorenson QM (2022) Annual biomass data (2001-2021) for southern California: above- and below-ground, standing dead, and litter. Dryad Dataset. 10.5061/dryad.qz612jmjt
Schrader-Patton CC, Underwood EC, Sorenson QM (2023) Annual biomass spatial data for southern California (2001-2021): above- and belowground, standing dead, and litter. Ecology 104, e4031.
| Crossref | Google Scholar | PubMed |
Syphard AD, Brennan TJ, Keeley JE (2019) Drivers of chaparral type conversion to herbaceous vegetation in coastal southern California. Diversity and Distributions 25, 90-101.
| Crossref | Google Scholar |
Underwood EC, Hollander AD (2023) Areas of low natural regeneration potential post-fire in shrublands of southern California (selected years between 2008 and 2020). [Dataset]. Dryad. https://datadryad.org/stash/dataset/doi:10.25338/B8CH2T
Underwood EC, Hollander AD, Huber PR, Schrader-Patton C (2018) Mapping the value of national forest landscapes for ecosystem service provision. In ‘Valuing chaparral: ecological, socio-economic, and management perspectives’. (Eds EC Underwood, HD Safford, NA Molinari, JE Keeley) pp. 245–270. (Springer: Cham, Switzerland)
USDA Forest Service (2015) National visitor use monitoring: visitor use report. Database queried for: Angeles National Forest (2006, 2011), Cleveland National Forest (2009, 2014), Los Padres National Forest (2009, 2014), and San Bernardino National Forest (2009, 2014). Available at https://apps.fs.usda.gov/nfs/nrm/nvum/results
Wohlgemuth PM, Lilley KA (2018) Sediment delivery, flood control, and physical ecosystem services in southern California chaparral landscapes. In ‘Valuing chaparral: ecological, socio-economic, and management perspectives’. (Eds EC Underwood, HD Safford, NA Molinari, JE Keeley) pp. 181–205. (Springer: Cham, Switzerland)
Zedler PH, Gautier CR, McMaster GS (1983) Vegetation change in response to extreme events – the effect of a short interval between fires in California chaparral and coastal scrub. Ecology 64(4), 809-818.
| Crossref | Google Scholar |
Zhou M, Deng J, Lin Y, Belete M, Wang K, et al. (2019) Identifying the effects of land use change on sediment export: integrating sediment source and sediment delivery in the Qiantang River Basin, China. Science of the Total Environment 686, 38-49.
| Crossref | Google Scholar | PubMed |