Net reductions or spatiotemporal displacement of intentional wildfires in response to arrests? Evidence from Spain
Jeffrey P. Prestemon A E , David T. Butry B , María L. Chas-Amil C and Julia M. Touza DA Forest Policy and Economics Research, Southern Research Station, USDA Forest Service, Forestry Sciences Laboratory, PO Box 12254, Research Triangle Park, NC 27709, USA.
B Applied Economics Office, Engineering Laboratory, National Institute of Standards and Technology, 100 Bureau Drive, Mailstop 8603, Gaithersburg, MD 20899-8603, USA.
C Departamento de Economía Cuantitativa, Universidade de Santiago de Compostela, Avenida Xoán XXIII s/n, 15782, Santiago de Compostela, Spain.
D Department of Environment and Geography, University of York, Wentworth Way, Heslington, York, YO10 5NG, UK.
E Corresponding author. Email: jeff.prestemon@usda.gov
International Journal of Wildland Fire 28(6) 397-411 https://doi.org/10.1071/WF18234
Submitted: 13 June 2018 Accepted: 16 April 2019 Published: 31 May 2019
Journal compilation © IAWF 2019 Open Access CC BY-NC-ND
Abstract
Research to date has not examined how the impacts of arrests manifest across space and time in environmental crimes. We evaluate whether arrests reduce or merely spatiotemporally displace intentional illegal outdoor firesetting. Using municipality-level daily wildfire count data from Galicia, Spain, from 1999 to 2014, we develop daily spatiotemporal ignition count models of agricultural, non-agricultural and total intentional illegal wildfires as functions of spatiotemporally lagged arrests, the election cycle, seasonal and day indicators, meteorological factors and socioeconomic variables. We find evidence that arrests reduce future intentional illegal fires across space in subsequent time periods.
Additional keywords: arson, autoregression, elections, negative binomial, panel data, spatiotemporal, zero inflation.
Introduction
Natural resource managers worldwide face a challenge of how to limit the occurrence of accidentally and intentionally ignited wildfires that destroy property, damage resources and harm people. A changing climate is projected to increase wildfire potential, severity and extent (e.g. Krawchuk et al. 2009; Liu et al. 2010; Jolly et al. 2015), potentially exacerbating the damages and raising the urgency of addressing human-ignited wildfires (Balch et al. 2017; Bowman et al. 2017). Among the strategies and tactics available to policy-makers in response to heightened wildfire risks is to boost law enforcement efforts (e.g. Donoghue and Main 1985; Butry and Prestemon 2005; Prestemon and Butry 2005; Prestemon and Butry 2010; Abt et al. 2015) aimed at apprehending and deterring intentional firesetters.1 With only a few exceptions (e.g. Thomas et al. 2011; Prestemon et al. 2012), research has not evaluated how and whether arrests, a discrete measure of law enforcement efforts, are linked to reductions in the occurrence of intentional fires or whether such efforts have broader impacts across space and time.
The effects of law enforcement efforts are potentially complex, considering motivated offenders’ dynamic responses. Criminologists have long recognised that actions by law enforcement or other groups intended to reduce crime in one time or place or of one type might be at least partially offset by compensating shifts in behaviours of prospective offenders in response to those efforts (e.g. Cornish and Clarke 1987; Barr and Pease 1990; Eck 1993; Clarke and Weisburd 1994; Guerette 2009). Although spatiotemporal concentrations in arson are evident in maps of wildfires (e.g. Mothershead 2012), no study has sought to characterise the effects of arrests on these fires at fine spatial scales or over longer time scales than detected by Prestemon et al. (2012). Understanding how law enforcement efforts affect the timing and locations of future offending, including illegal firesetting, is critical to enacting effective policies and in the design of more effective law enforcement strategies (e.g. Weisburd and Eck 2004) aimed at reducing the occurrence of unwanted wildfires.
The primary objective of our study is to assess whether there is evidence of spatiotemporal displacement effects of law enforcement in intentional outdoor firesetting. To do this, we develop statistical models that control for hypothesised driving factors in addition to arrests. Among the driving factors we include are variables describing the seasonality of intentional firesetting, which likely stems from regular variations in fuel flammability and ignition attempts related to agricultural or other seasonal activities (e.g. hunting) and daily human routines (days of the week and holidays). Additionally, we include variables explaining firesetting variation at longer time scales, which capture associations with slowly changing aggregate wildland fuels and demographic and economic conditions. Finally, we include a set of indicators of election seasons, which have been shown in other research to be criminogenic for firesetting in Europe (e.g. Seijo 2005; Álvarez-Díaz et al. 2015; Ramos and Sanz 2018).2 With the inclusion of election season indicators, we are able to quantify the daily time scale imprint of one form of politically based protest actions (Pyne 1995), extending existing research and providing additional policy-relevant insights. Their inclusion also helps to explain variation in observed intentional wildfires, increasing the power of our statistical inferences regarding law enforcement spatiotemporal effects.
This study advances the earlier work by Prestemon et al. (2012) by including spatiotemporal lags of arrests in the equation specifications. Although those authors allowed for effects of arrests at long temporal scales, their models did not include arrests lagged as long as in the present study. Furthermore, their models were not specified in a way that quantified how intentional fires in one location could be associated with arrests in nearby locations in previous time periods. Finally, Prestemon et al. (2012) ignored the potentially differing firesetting processes across reported motivations, which we find are distinct in their responses to arrests, a potentially important distinction with implications for law enforcement resource allocation decisions. The study also advances research into the role of elections on wildfires in Spain conducted by Álvarez-Díaz et al. (2015), who used reduced-form vector error correction modelling methods to identify the association between the total numbers of forest fires during ‘intense’ electoral years. Our study, in contrast, estimates count data structural equations of intentional wildfires in Spain, controlling for the many factors that influence wildfire ignition processes, including the motivations of firesetters, arrests and elections. Our equations are estimated at fine temporal (daily) and spatial (municipality) scales, measuring how specific phases of elections affect the daily pattern of firesetting in the run-up to elections.
Theory and methods
Theory of intentional firesetting
Cohen and Felson (1979) describe a Routine Activities (RA) theory of crime that forms the foundation on which we build our empirical model of the numbers of intentional wildfires. These authors contend that criminal activities vary over time and space according to variations in the simultaneous overlap of three essential elements of crime occurrence: (1) a motivated offender, (2) a suitable target, and (3) the absence of capable guardians against a violation; if one of these elements is missing, then a crime will not occur. It is straightforward to design an empirical modelling framework around RA theory because motivated offenders and suitable targets are simple to define.
Geographers, philosophers and criminologists dating back several centuries have recognised that persistent criminal activity has evident spatial components related to societal and landscape features (see Cohen 1941 and Cohen and Felson 1979 for informative syntheses). As Cohen and Felson (1979) argue, defining RA theory, persistent spatial and temporal concentrations of offending may be attributed to fine- and coarse-scale spatial and temporal (hourly, daily, seasonal) features of landscapes and routine human behaviours as well as to temporally trending variations in the relative abundances of motivated offenders, suitable targets and capable guardians. Here, we propose that RA theory is an amenable framework for understanding these observed spatial and temporal patterns. In the development of an empirical version of an RA theory of intentional firesetting, we identify candidate physical, biological and societal variables that vary over space and time that can be mapped to one (or more) of the three elements of RA theory. With this empirical framework, we are able to test for spatiotemporal displacement and uncover the effects of other policy-relevant phenomena on intentional firesetting.
We summarise the variables connected to each of these three elements of RA theory as it relates to intentional firesetting:
Motivated offenders
Variations in the numbers of motivated firesetters in a location are likely related to several demographic, social and economic factors, including the size of the human population, historical efforts to remove known offenders from the location and factors that influence motivations to offend. Such motivational factors can be broadly defined as incentives to commit a crime (e.g. utility gained or pecuniary benefits acquired). In the case of intentional wildland firesetting, greater incentives might exist during times of political discord (Dyke 2007), when firesetters protest by igniting wildfires (e.g. Pyne 1995; Kull 2002; Seijo 2005, 2009; Hovardas 2014, 2015; Skouras and Christodoulakis 2014; Álvarez-Díaz et al. 2015; Ramos and Sanz 2018); when agricultural areas may be prepared for planting by (illegally) burning them; and when the opportunity costs of time for firesetters are low (e.g. in times and places where they are unemployed) (e.g. Prestemon and Butry 2005; Sebastián-López et al. 2008), which reduces the pecuniary cost of spending time trying to ignite fires and the expected costs of being arrested and imprisoned for igniting a fire.
Suitable targets
Variations in suitable targets for intentional wildfires can be explained by variations in the availability of flammable wildland fuels and in the weather suitable for successful ignition and spread.
Capable guardians
Variations over time and space of capable guardians could be indexed by law enforcement efforts: the number of patrol officers or the effectiveness of law enforcement actions (e.g. arrests). Measures of political discord, which can induce reallocations of law enforcement resources across space and time, could further be proxies for short-run changes in capable guardianship.
Our introduction of arrests into an RA-based theory of intentional firesetting bears further exploration. Because offenders who are caught are often sanctioned by imprisonment, an arrest actually affects two elements of RA theory. First, the arrest can shrink the pool of motivated offenders by removing spatially stationary serial criminals from the landscape (e.g. Canela-Cacho et al. 1997; Ratcliffe and Rengert 2008). Second, an arrest, if observed widely across space, potentially raises the perceived strength of capable guardianship.3 Guardianship adjustments may be more likely to occur close to the location of the arrest (e.g. Levitt 1998; Pogarsky et al. 2004), we hypothesise, because arrest information may be more readily communicated locally to motivated offenders, achieving a local crime reduction subsequent to the arrest. If the arrest information is more broadly disseminated across space, then this adjustment could result in a deterrent effect across a larger spatial domain, revealed, in the case of intentional firesetting, as a reduction in the number of fires in subsequent time periods in surrounding locations. The motivated offender may also be induced by an arrest to change their ‘domestic base’ of operation in order to avoid localities where capable guardianship has increased (e.g. Telep et al. 2014). The result of this dynamic response by motivated offenders may be higher overall crime rates farther from the arrest location in subsequent time periods, revealing how law enforcement actions could displace crime in space–time (e.g. Eck 1993; Bowers and Johnson 2003; Guerette and Bowers 2009; Bowers et al. 2011). Displacement caused by an arrest would be revealed by, all other things being equal, higher numbers of intentional fires in subsequent time periods in areas more distant from the arrest location.
Application of RA theory to intentional firesetting should comprehend existing evidence of positive temporal autocorrelation in intentional firesetting (e.g. Butry and Prestemon 2005; Prestemon et al. 2012). The cited studies attribute positive autocorrelation to a combination of serial and copycat firesetting. Kocsis and Irwin (1997) find that serial arsonists (along with rapists and burglars) tend to commit their crimes close to their domestic base but also exhibit an occasional ‘commuter pattern’, ranging farther from the domestic base to carry out some of their offending. Such spatiotemporal clustering of firesetting is consistent with the near repeat hypothesis in crime victimisation tested by many analysts of certain property crimes (e.g. Townsley et al. 2003; Bowers and Johnson 2005; Bernasco 2008; Ratcliffe and Rengert 2008; Bernasco et al. 2015) and identified as well for non-wildland arson (Prestemon et al. 2013; Grubb and Nobles 2016).
Empirical specifications
The study location chosen is the region of Galicia, Spain, where intentional wildfires are the dominant cause attribution (Ponte Pintor and Bandín Buján 2008). Galicia is also a region with a large enough number of intentional wildfires (half of Spain’s) and associated arrests to permit statistical identification of spatiotemporal effects of arrests.
Accurate quantification of the spatiotemporal displacement of intentional firesetting due to arrests might depend on the type of motivated offender who is being modelled. Therefore, we test for the spatiotemporal effects of arrests and the effects of elections and other driving variables by partitioning our data according to whether a fire is classified as agriculturally or non-agriculturally motivated. Separate evaluation of these two broad categories of intentional firesetting could reveal the potential biases of modelling that ignores their different nature and uncovers their distinct spatiotemporal dynamics. Also, separate modelling by motivation could potentially yield more accurate assessments of the overall effects of changes in law enforcement efforts and elections on firesetting in the region and reveal how prevention efforts might be differently focused on these two categories of intentional fires.
More formally, we express the probability of I intentional wildfires started for motivation m in location j in period t as a function of a vector of variables affecting the number of motivated offenders in period t (Yj,t), a vector of variables measuring the availability of suitable targets in period t (Wj,t), and vectors of measures (Aj,t) of the presence of (effective) capable guardians in location j and nearby locations k over l lagged time periods as:

Vector Yj,t, the number of motivated offenders in our study, comprises the human population in the location (Pj,t); income-earning (Gj,t) potential for residents of location j; the unemployment rate (Uj,t); dummies measuring holidays (Ht) and each of two weekend days (), when the opportunity costs of firesetting are lower (and motivations higher) (Gill et al. 1987; Prestemon and Butry 2005; Prestemon et al. 2012); and a subvector of variables describing the occurrence of an election (Ej,t),
, where
is a dummy variable = 1 during the next 15 days (also called campaign days) in advance of an election, 0 otherwise;
is a dummy variable = 1 during the single silence day before an election, 0 otherwise; and
is = 1 for the single day of the election. Note that the t + 15, t + 30 and t + 45 leads of the election campaign days dummy variable are intended to capture the potentially long criminogenic temporal footprint of elections. Also, note that the last lead only occurs to 52 days, which covers only the last week of the 52-day statutorily required number of days from the announcement of an election and the election’s actual occurrence. The motivated offender vector is therefore
.
The vector Wj,t, the number of suitable targets in location j in period t, is described as a function of available flammable fuels, which can be quantified by a vector of meteorological variables (), consisting of minimum daily relative humidity (
), total daily precipitation (
), the Keetch–Byram Drought Index (Keetch and Byram 1968) (
), the Fire Weather Index (Fosberg 1978) (
), daily maximum hourly wind speed (
) and maximum daily temperature (
) (e.g. Prestemon et al. 2012), so that
. It also includes seasonal variation in firesetting (e.g. Fuller 1996), as a set of 11 month dummy variables,
, with the effect of December included in the intercept. The suitable targets vector is
.
Finally, vectors of variables indexing capable guardianship in location j in period t are measured by successive lags of arrests for intentional firesetting (of either motivation, because we lack specific information about the motivations of arrestees). We include running sums of the count of arrests lagged t − 1 to t − 365 and t − 366 to t − 545 in location j, so that . Recognising the recommendations about choice of buffer distances (Phillips 2011), we use a proportionate size criterion, with buffer radii at 10, 15 and 25 km. These radii are also justified by observed firesetting behaviour by serial arsonists in Spain (Sotoca Plaza 2016). Variables on the neighbouring arrests were also generated using running sums; these occurring in three successively more distant ‘doughnuts’ around the municipality: 10, 15 and 25 km, with vector
,
,
,
.
The number of intentional wildfires is hypothesised to follow a count process, distributed as Poisson but with a variance σ2 that is a function of its expected value (µj) and a scale parameter, α, i.e. a negative binomial model (Cameron and Trivedi 1998, p. 70), . Adding the time subscript (t), indicating the motivation m and consolidating the independent variables shown in Eqn 1 into a single vector (Xj,t) and a conforming vector of parameters (βm), we have:

Eqn 2 assumes that all spatial units have independently and identically distributed errors, a situation not likely to be met. Therefore, a fixed-effects process is considered, allowing for variations across municipalities in the average rates of intentional wildfires due to time-invariant unobservable factors. Therefore, Eqn 2 is augmented to include indicator parameters representing locations, a j-dimensional vector Dm:

The likelihood function for Eqn 3 is:
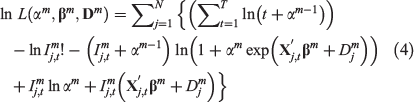
A random-effects version of this same general model for cross-sectional data is also available, but in the interest of brevity, we do not show its specification or likelihood equation.
To account for temporal autoregressivity in intentional wildfires, we adopt a method recommended by Zeger and Qaqish (1988) and elaborated in Cameron and Trivedi (1998, p. 239–240). Their approach entails specifying two variables, and
, constructed from lags of the dependent variable:

Lags of the variables specified in Eqn 5 accommodate higher orders of autoregression in the count process. In estimation, can be augmented to include r-dimensional subvectors
and
, where r is the order of autoregression. Coefficients on the elements of
are estimates of autoregressive components, and those in
rescale the effect of the lagged dependent variable in cases in which
.
The presence of significant autoregression in the dependent variable implies that there are short-run and long-run effects of a change in the elements of Xj,t besides and
. The long-run effect of any non-autoregressive variable i in Xj,t on
is calculated as
, where
is the estimated autoregressive component u (u = 1,…, r), quantified as the coefficient of
from the estimate of Eqn 3 or Eqn 4, augmented by
and
.
Following Leamer (1983), and with examples of the importance of his recommendations (e.g. Levine and Renelt 1992), it has now become standard practice in many economics journals to test inferences across multiple specifications in order to evaluate whether these inferences are robust to specification. We follow this practice by testing for the effects of arrests, elections and other hypothesised variables using several specifications that carry with them different assumptions about the intentional wildfire data generation process. In addition to a fixed-effects negative binomial and a random-effects negative binomial, we model intentional wildfires using four alternative estimators, which each make differing assumptions about the intentional wildfire data-generating process. These include (i) a pooled (across municipalities) negative binomial model that controls for municipality-level differences in error variance; (ii) a pooled negative binomial (NB) model with municipality indicators that also controls for municipality-level differences in error variance; and two specifications that recognise potential zero inflation in the count of intentional wildfires: (iii) a pooled zero-inflated negative binomial (ZINB) model that controls for municipality-level differences in error variance, and (iv) a pooled ZINB with municipality indicators that also controls for municipality-level differences in error variance.
Evaluation of the magnitudes of the effects of the variables of interest – arrests and elections – was done with a counterfactual analysis. In the case of arrests, we can quantify the long-run average effects of an arrest by, say, simulating an increase in the number of arrests by 1% and observing how the predicted counts of intentional wildfires changes, or . Arrest effects are measured with an elasticity. To evaluate the non-marginal effects of elections, we compare the predicted counts of intentional wildfires with the counts predicted with all election dummy variables set to zero,
. Election effects are measured as changes in total numbers and percentage.
Given that our data are spatially arranged, maximum likelihood estimation of any of our model specifications could produce spatially autocorrelated residuals deriving from omitted spatially correlated factors (e.g. Dormann et al. 2007), which would have the effect of attenuating standard errors. Although more complex methods of bounding of our model coefficient estimates do exist (e.g. Hall 1985; Liu and Singh 1992), and these could be tried in future studies to uncover any residual spatial autocorrelation remaining, Monte Carlo bootstrapped generation of the effects size confidence limits provides a view of the statistical significances of the overall effects of arrests and elections, regardless of any possible standard error attenuation in model estimates.
Data and estimation
We assembled data on total daily counts of reported ignitions of agricultural () and non-agricultural intentional wildfires (
) in Galicia, covering a 16-year period between 1 January 1999 and 31 December 2014. The spatial unit of observation is the municipality (Fig. 1), of which there are 313. The wildfire database compiled by the Spanish Forest Service contains observations on 108 527 fires over the 16-year span, burning 431 956 ha. Data on each wildfire include a general cause attribution (lightning, negligence and accidents, intentional, reignition and unknown), the majority of which (81%) are classified as intentional. Intentional wildfires are further subcategorised by ‘motivation’, i.e. as either agriculturally related (i.e. started by farmers to eliminate brush and agricultural debris) or non-agricultural. In our econometric estimates of the fixed effects, random effects and ZINB models, separate equations are reported for each motivation (m = agricultural, non-agricultural) as well as their sum, as mentioned.
![]() |
Population data were obtained from municipal administrative records. The population figure was divided by the area of the municipality (Pj,t), with daily changes computed by interpolation. As a proxy of income level (Gj,t), we used the change in the average income declared in annual income tax per year in the municipality, which was provided by the Spanish Tax Agency, and deflated with the annual average of the Consumer Price Index by province (base year 2016); daily values were found by interpolation. The unemployment rate (Uj,t) was the change in the average 30-day centred average rate calculated from monthly municipality unemployment data, with daily changes computed by interpolation. Collected from the Public Employment Service, it was the seasonally adjusted number of unemployed persons aged 16 to 64 years, divided by the total population; daily values were found by interpolation.
Holidays (Ht) were official days recognised across Spain and those official days recognised across Galicia only. Data on the meteorological variables for each municipality (Zj,t) were based on a network of weather stations distributed across Galicia; the centre of each municipality was used as the reference location for computing distances to each weather station, and values were generated with an inverse-distance-weighted spatial averaging process.
Daily data on the numbers of arrests, , were employed as daily sums of arrests made by the Guardia Civil (gendarmerie) and local police service. Neighbourhood arrest data,
and their temporal lags were generated for three circular buffers around the centroid of the municipality. Centroid distance was determined using a 1 : 25 000 map of municipalities obtained from the Galician Territorial Information System. Note that buffers for municipalities on the edges of the region can extend into the Atlantic Ocean, where there are no arrests, and into Portugal and neighbouring regions of Spain, where no consistent daily data are available on arrests. To avoid potential biases, the sample of municipalities used was limited to those that did not have a regional border with the rest of Spain or an international border with Portugal within the outermost ring of neighbourhood arrests of the municipality. Municipalities with buffers that reached into the ocean were kept in the sample.
Finally, elections variables are defined as representing the various phases of the election cycle of any of four possible levels: local (council), regional, national or European parliamentary. Owing to their individual infrequency, identification of the effects of the different levels of elections was usually not possible (likelihood maximisation failed) if separately modelled, compelling their aggregation.
In all models, we attempted to specify autoregressive orders as high as five, but it was clear on estimation that high orders prevented many longer-order autoregressive specifications from converging. Given the convergence problems, we limited our models to control for only first-order autocorrelation.
The long lags of arrest data (back to t – 545) effectively shortened the usable dataset by 1.5 years, meaning that parameter estimates cover mid-2000 through December 2014. Effects are reported for the short run, which ignores the autoregressive effect of wildfires within a municipality, and the long run, which incorporates the autoregressive effect. When estimating separate agricultural and non-agricultural intentional fire models, we included the lagged count and lagged zero count indicators (Eqn 5) of both categories. When calculating the long-run effects of elections and arrests, we used only the lagged count of the own type (i.e. the coefficient on agricultural intentional lagged fires was used to make the long-run adjustment, and the same for the non-agricultural category).
Maximum likelihood estimation of some of the alternative specifications of the intentional fire count models failed when the sample of municipalities included those with fewer than 200 intentional wildfires, probably a result of lack of model parameter identification. To allow for greater comparability in model results and effect size estimates, models were estimated only for the 113 municipalities with at least 200 total intentional wildfires over the time span. Data sources and descriptions of variables included in models are shown in Table 1.
![]() |
Results
Equation estimates
Equation estimates for the fixed-effects NB models and the random-effects NB models are shown in Tables 2 and 3 respectively. Estimates with alternative estimators are available from the authors. Summary estimation statistics indicate that the model specifications are significant compared with constant-only model estimates, as measured by the Wald Statistic, distributed chi-squared(i). For total, agricultural and non-agricultural fires, the random-effects NB specification (allowing for differences in variances across municipalities) is preferred over a pooled NB specification with municipality-level heteroscedasticity, according to a chi-squared statistic and according to the significance of the dispersion parameters, which were all significant at α ≤ 0.01.
Model estimates indicate that nearly all included variables are statistically significant at α ≤ 0.01. Nearly all variables intended to account for suitable targets are highly statistically significant in all model estimates, including relative humidity, precipitation, wind speed, maximum daily temperature, the Keetch–Byram Drought Index and the Fire Weather Index. Forest area, however, a measure of aggregate fuels available as ignition targets, is positively related to intentional fires, in total and then also for agricultural intentional wildfires, though not significant for non-agricultural intentional fires.
Variables intended to account for temporal variations in the number of motivated offenders are typically also highly statistically significant in all models. Month indicators quantify a seasonal pattern in firesetting for both rural agricultural (possibly related to field preparation) and non-agricultural activities (possibly related to hunting), and they are nearly all highly statistically significantly different from the reference month of firesetting (December), with peak rates observed in February, March and April, an ebb in June and July, more fires in August, and then somewhat more in autumn. In all model estimates, variables controlling for lower opportunity costs for carrying out firesetting at the daily time scale on non-work days, indicators of Saturdays, Sundays and holidays, are statistically significant at α ≤ 0.01 and positively signed, as expected (e.g. Prestemon and Butry 2005; Prestemon et al. 2012). Population density generally is associated with more intentional firesetting, as expected (more motivated firesetters per unit area) in most of the model estimates. Unemployment, modelled as the change in rate, meant to capture how the opportunity cost of carrying out and getting caught setting intentional fires affects the number of motivated offenders, is usually not statistically significant. The rate of change in real personal income, expected to be negatively related to the numbers of motivated offenders owing to the higher opportunity costs of being caught intentionally igniting wildfires, demonstrates unexpected sign differences between intentional agricultural and non-agricultural firesetting. In the fixed-effects NB specifications (Table 2), agricultural intentional fires respond negatively to income changes, as expected, while non-agricultural respond positively, counter to expectations (and also positively for total intentional fires). In the random-effects NB specification (Table 3), agricultural fires have the expected negative sign on income changes, while not significant for non-agricultural fires and having an unexpectedly positive association with the total number of intentional fires.
Variables intended to model spatial and temporal variations in capable guardianship are nearly all highly significant explainers of variation in intentional firesetting. Arrests at all lag orders evaluated are strongly statistically significant (α ≤ 0.01) and negatively signed. These results are found for within the municipality and at progressively greater spatiotemporal lags around the municipality, and they are found for both the fixed-effects and random-effects NB specifications. The negative signs on the spatiotemporal lags of arrests support a hypothesis that arrests do not simply displace intentional fires in space or time; rather, they work to decrease occurrence of such fires for long periods and across broad geographical areas near the arrests, presumably by increasing perceived capable guardianship both within the municipality and in surrounding municipalities.
The modelled effects of elections, hypothesised to measure both the numbers of motivated offenders (because elections encourage political protest fires) and the numbers of capable guardians (shifting policing intensities), are consistently associated with intentional fire counts across all motivations and model specifications. Results reveal the temporal pattern of election-associated firesetting. First, election days either do not have greater numbers of intentional fires (agricultural in particular) or have fewer such fires (non-agricultural). Second, silence day has a pronounced higher rate of intentional firesetting for total, agricultural and non-agricultural intentional fires. Third, campaign day periods have generally no effects or positive effects on intentional firesetting. For both the fixed-effects NB (Table 2) and the random-effects NB, higher firesetting rates are found in the 32 to 46 days and the 47 to 52 days before the election day but not in the month just before the election.
Finally, we find that for total, agricultural and non-agricultural intentional wildfires, intentional wildfires are positively first-order autocorrelated at the daily time step. Findings are similar for all model specifications, with all parameter estimates significant at α ≤ 0.01. For total intentional fires, the coefficient on the intentional fire count from day t − 1 is 0.22 in both the fixed- and random-effects specifications. For agricultural intentional fires, the coefficient is 0.25 on its own type in both specifications and 0.045 and 0.046 on the non-agricultural fire count day t − 1 in the fixed-effects and random-effects NB specifications respectively. For non-agricultural intentional fires, the coefficient is 0.21 on its own type and 0.12 on the agricultural fire count from day t − 1, and the values are the same in both the fixed-effects and random-effects NB specifications. In all specifications and fire types, coefficients controlling for zero fires in the previous day are negative, as expected, which shows that a count of zero fires in day t − 1 leads to fewer intentional fires in day t.
Effect size estimates
Summary effects of elections and arrests on total, agricultural and non-agricultural intentional wildfires are shown in Tables 4–6. The results are arranged according to the fixed-effects NB, random-effects NB and the four alternative estimators. The intentional wildfires in the 113 municipalities analysed, over the time span of our sample, represented ~77% of all intentional, 84% of all agricultural intentional and 74% of all non-agricultural intentional fires that occurred in the municipalities whose 25-km arrest buffers did not cross into Portugal or the rest of Spain. If our equations were to be applied as well to the municipalities in Galicia meeting the buffer restrictions but having fewer than 200 intentional fires (1999–2014), the simulated changes in total fire counts would be larger and the percentage changes would be different than those shown in Tables 4–6.
![]() |
Effect sizes of arrests and of elections for the modelled 113 municipalities are statistically different from zero at α ≤ 0.01 in all specifications except for one alternative estimator, the pooled ZINB with municipality indicators and municipality-level heteroscedasticity for non-agricultural intentional wildfires, for which election effect sizes are significant at α ≤ 0.05.
Across all specifications and model versions, arrests are associated with a reduction in the counts of intentional wildfires in the municipality. Arrests in the previous 545 days before day t reduce wildfire occurrences in the municipality in day t. For the total of intentional wildfires (Table 4), the short-run elasticity of an arrest – i.e. the percentage change in the number of intentional fires given a 1% increase in the number of arrests – is −0.93 and −0.92 in the fixed-effects and random-effects NB specifications respectively and ranges from −0.29 to −0.51 among the four alternative estimators. Long-run effects, which account for the autoregressive component in the intentional wildfire count process, are larger, at −1.20 and −1.18 for the fixed-effects and random-effects NB specifications respectively, and range from −0.46 to −0.73 among the alternative estimators. For agricultural intentional fires (Table 5), the effects of arrests are substantially larger. Short-run effects are −1.68 and −1.67 and long-run effects are −2.25 and −2.24 in the fixed-effects and random-effects NB specifications respectively. For the alternative estimators, short-run effects range from an elasticity of −0.24 to −0.43 and long-run effects −0.43 to −0.66. Non-agricultural intentional fires are more inelastically related to arrests (Table 6). Arrest elasticities in the short run range from −0.94 and −1.13 and in the long run from −1.19 to −1.43 in the fixed-effects and random-effects NB specifications respectively. Alternative estimators produce elasticity estimates from −0.32 to −0.54 in the short run and −0.48 to −0.78 in the long run.
Across all types of intentional fires and all specifications, the net effect of an election in Galicia is to increase the number of intentional wildfires. As shown in Tables 4–6, and as we did for arrests, we assessed the overall impacts of elections on firesetting in both the short run and the long run. Tables 4–6 report the simulated effects of setting all election indicator variables to zero and quantifying the simulated counterfactual of no elections during the duration of our estimation dataset (mid-2000 to December 2014).
Consistent with the positive signs on many of the election indicator variables (Tables 2 and 3), the effect of simulating no elections is to reduce the total number and also the number of agricultural and non-agricultural intentional wildfires. For the sum of agricultural and non-agricultural models (Table 4), short-run effects are −1330 and −1328 in the short run for the fixed-effects and random-effects NB specifications, and −1649 in the long run for both specifications. Short-run effects among the alternative estimators range from −498 to −796 and long-run effects from −687 to −1240. In percentage terms, the simulated effect of not having elections would be to reduce the total number of intentional fires by −3.33% in the long run according to the fixed-effects and random-effects NB specifications, and from −1.35 to −2.44% among the alternative estimators.
Effects of elections on agricultural fires (Table 5) are similar in magnitude but larger in percentage terms, compared with those quantified by the total intentional fire models. This finding indicates that aggregating both agricultural and non-agricultural intentional fires in a single model likely produces downwardly biased parameter estimates – i.e. aggregation bias. As shown in Table 5, both the fixed-effects and random-effects NB specifications produce nearly identical short- and long-run simulated reductions in the expected fires. In the long run, the change in the number of intentional agricultural fires is approximately −1630, corresponding to −10.16% for the fixed-effects NB. Alternative estimators had long-run effects ranging from −327 to −623 and percentage changes from −1.99 to −3.74%.
For non-agricultural intentional fires, effects of simulated no-elections counterfactuals (Table 6) are also larger in percentage terms than found in the total intentional fire count changes (Table 4). For the fixed-effects NB specification, the long-run effect is −1986, or −5.78%. For the random-effects NB specification, the corresponding values are −934 and −2.79%. For the alternative estimators, the long-run effects range from −386 to −778, changes corresponding to −1.12 to −2.25%.
Combining the effects reported in Tables 5 and 6, we can quantify the total effect more accurately than by using the total intentional fire model effects shown in Table 4. For example, if we sum the fixed-effects NB specification long-run effect of −1630 for the agricultural fires and −1986 for the non-agricultural fires, we have −3616, a change of approximately −7.18% in the number of total intentional wildfires, calculated over mid-2000 through the end of 2014. For the random-effects NB specifications in Tables 5 and 6, the summed effects are −2565 and −5.19%. These long-run values can be compared with the corresponding values shown in Table 4, which are −1649 and −3.33%, which are produced by both the fixed-effects and random-effects NB specification effects.
Discussion and conclusions
This study set out to evaluate whether arrests affect the spatiotemporal distribution of intentionally set future wildfires, which can provide insights regarding the overall effectiveness of law enforcement efforts. We used geographically and chronologically precise data on fire occurrence and arrests in Galicia to examine, based on the theoretical framework of RA theory (Cohen and Felson 1979), whether wildfire numbers changed locally and in nearby locations following intentional wildfire-related arrests.
Statistical model estimates indicate that variables connected to the three elements of RA theory were related to intentional firesetting, generally, in the ways expected. The numbers of motivated offenders were measured by population density, indicators of election periods, indicators of seasons when agricultural areas are being prepared for planting, and variables quantifying the opportunity costs of carrying out or potentially being arrested for intentional firesetting. The only motivated offender variables that did not have a sign in the direction expected or significant in our empirical specifications were unemployment and income. For suitable targets, quantified by variables measuring aggregate fuels quantities (forest area) and amenable weather conditions (fire weather indices and several direct meteorological variables), the RA framing was supported by the signs and the significances of parameter estimates. Primary measures of the presence or absence of capable guardians were the temporal and spatiotemporal lags of recent arrests for intentional firesetting and election variables. Arrests were hypothesised to communicate to motivated intentional firesetters that their likelihood of arrest, and hence capable guardianship, has increased. We found that all temporal and spatiotemporal lags of arrests were highly statistically significantly and negatively related to counts of intentional wildfires at all spatiotemporal lags tested, a finding expected given either perceived broad-scale increases in capable guardianship or overall decreases in motivated offenders. Election variables were also generally statistically significantly related to intentional firesetting, adding additional weight to the usefulness of the RA-based theoretical framework for this crime process.
Our study demonstrated that our hypotheses regarding potential spatiotemporal displacement of the effects of arrests and the effects of elections were robust to assumptions about the form of the wildfire data generation process. The fixed-effects and random-effects NB specifications generated broadly similar effect sizes and strong statistical significances. Although also strongly statistically significant and signed in the same way, estimates generated by arguably less-well-fitting specifications, including the pooled NB models and the zero-inflated pooled NB models, produced effect sizes that were somewhat smaller.
The separate estimation of agricultural and non-agricultural intentional fire models highlighted the importance of separately modelling intentional fires by motivation of the firesetter. One benefit of the disaggregation was to reveal that the effects of arrests and of elections were larger than when all motivations were combined and modelled together. Separate estimation produced summed total effect sizes of arrests and of elections that were more than twice as large as the effect sizes produced by models that combined them. In future research, analysts would be counselled to model intentional fires at as fine a level of motivational aggregation as can be supported by the data.
Another benefit of the separate modelling was to reveal the differences in the magnitudes of the effects of driving variables on intentional firesetting of different motivations. A comparison of the results reveals that agricultural fires are more elastically related to an arrest. The greater sensitivity of agricultural intentional firesetting to arrests could be considered in the context of the profit-maximising behaviour of an agriculturist, who decides when and whether to use fire as a land-clearing method, including in times when such fire use is forbidden. Because agriculturists depend on agriculture for their livelihoods, it makes sense that they would be particularly responsive to conditions under which their expected incomes would be reduced by criminal sanctions. The differential arrest sensitivity of these two classes of motivation also implies that attention to their relative prevalence in a landscape could be considered when making decisions on how to optimally deploy law enforcement resources across an agricultural–wildland gradient: in agricultural intentional wildfire-dominated landscapes, arrests would yield larger overall wildfire reductions than in places where non-agriculturally motivated firesetting predominates.
Although law enforcement resource allocations are guided by a variety of trade-off considerations, including attention to non-wildfire crimes and reducing fear of crime (Weisburd and Eck 2004), our results could aid policy-makers in assessing the consequences of police resource trade-offs. And although not explicitly designed to forecast future intentional wildfire locations in the form modelled by Prestemon et al. (2012), our results are potentially useful for implementing problem-oriented policing (e.g. Goldstein 1979; Weisburd et al. 2010) and intelligence-led policing (e.g. Ratcliffe 2016) strategies that focus on anticipating spatiotemporal crime concentrations and on identifying and incapacitating repeat offenders (e.g. serial arsonists). In the particular case of Galicia, by characterising how wildfires are concentrated in space–time and how the effects of arrests (and elections) alter such concentrations, our findings could help police organisations identify proactive steps to reducing intentional wildfire occurrences.
Our results do not provide support for the existence of spatial displacement caused by one measure of law enforcement effort, the number of arrests (e.g. Eck 1993), a finding consistent with other research on the effects of stepped-up policing (Bowers et al. 2011; Telep et al. 2014). Further study would be required to detect the effects of more distant (>25 km) and longer-lasting (>1.5 years) spatiotemporal lags of arrests.
The arrest findings are possibly related to altered adjustments in perceptions of increased capable guardianship in response to local arrests. In Spain, fewer than 10% of intentional wildfire cases result in a conviction and sanction, a relatively low rate of clearance compared with other crime types, due to evidentiary difficulties (Fiscalía General del Estado Medio Ambiente y Urbanismo 2016). Furthermore, most convictions typically lead to fines, not imprisonment, the latter outcome primarily reserved for repeat offenders (e.g. González et al. 2017). Given the low probability of imprisonment for firesetting, we conclude that arrests primarily result in a perceived increase in capable guardianship rather than a reduction in motivated offenders. Although we could identify no published research on how arrests for intentional firesetting are communicated across a population of motivated firesetters, such as through traditional and social media, the precise mechanisms of information diffusion responsible for changes in perceived capable guardianship are an area worthy of additional study.
Our analyses also offer evidence that political activity is associated with increased overall rates of intentional firesetting. The signs and significances of the various election indicator variables highlight the potentially complex behavioural patterns among political protest actors in Galicia. Furthermore, the identified temporal pattern of the effects of elections on intentional firesetting provides a roadmap for new strategies for allocating law enforcement resources during the election cycle. Four to seven weeks before an election, intentional firesetting is elevated. This is followed by a 4-week window approaching silence day and election day of normal rates of firesetting, a silence day spike in intentional fires, and then low to normal rates on election day. The measured overall (net) effect of the entire election cycle, however, is to boost intentional firesetting. The fixed-effects and random-effects NB specifications implied that a no-election counterfactual had up to 10% fewer agriculturally based intentional fires and 6% fewer non-agriculturally based intentional fires, with smaller impacts measured by the alternative estimators. Consistent with the conclusions of Kull (2002) and Skouras and Christodoulakis (2014), the larger agricultural effect of election cycles may occur because farmers perceive that capable guardianship is lower – i.e. the likelihood of being caught and sanctioned is lower – during (the earlier phase of) campaigns, when law enforcement may direct more resources towards addressing spikes in other forms of social disruption. Likewise, election periods might increase the numbers of prospective offenders who are motivated to focus politicians’ and the media’s attention to ongoing public–government disagreements about how forests are managed (Hovardas 2014). Ramos and Sanz (2018) provide statistical evidence that large accidental wildfires may affect election outcomes, favouring the incumbent party in municipal elections in Spain. If the conflict hypothesis is a partial explanation for the statistical evidence that our models provide, then policy-makers could use our results to help evaluate the potential benefits of efforts to reduce such conflicts.
Conflicts of interest
The authors declare that they have no conflicts of interest.
Acknowledgements
This research was partly funded by Project ECO2017–89274-R MINECO/AEI/FEDER, UE. The authors thank the Spanish Ministry of Agriculture and Fisheries, Food and Environment (MAPAMA) and AEMet (Agencia Estatal de Meteorología) for providing the forest fires and climatic databases respectively. We are grateful to the Laboratorio de Criminalística – Zona Guardia Civil de Galicia for facilitating arrest information. We thank Juan Fung and Marcos Álvarez-Díaz for helpful comments on an earlier draft, and Scott Goodrick for preparation of the meteorological data.
References
Abt KL, Butry DT, Prestemon JP, Scranton S (2015) Effect of fire prevention programs on accidental and incendiary wildfires on tribal lands in the United States. International Journal of Wildland Fire 24, 749–762.| Effect of fire prevention programs on accidental and incendiary wildfires on tribal lands in the United States.Crossref | GoogleScholarGoogle Scholar |
Álvarez-Díaz M, González-Gómez M, Otero-Giraldez MS (2015) Detecting the socioeconomic driving forces of the fire catastrophe in NW Spain. European Journal of Forest Research 134, 1087–1094.
| Detecting the socioeconomic driving forces of the fire catastrophe in NW Spain.Crossref | GoogleScholarGoogle Scholar |
Balch JK, Bradley BA, Abatzoglou JT, Nagy RC, Fusco EJ, Mahood AL (2017) Human-started wildfires expand the fire niche across the United States. Proceedings of the National Academy of Sciences of the United States of America 114, 2946–2951.
| Human-started wildfires expand the fire niche across the United States.Crossref | GoogleScholarGoogle Scholar | 28242690PubMed |
Barr R, Pease K (1990) Crime placement, displacement and deflection. In ‘Crime and justice: a review of research’. (Eds M Tonry, N Morris) Vol. 12, pp. 277–318. (University of Chicago Press: Chicago, IL, USA)
Becker GS (1968) Crime and punishment: an economic approach. Journal of Political Economy 76, 169–217.
| Crime and punishment: an economic approach.Crossref | GoogleScholarGoogle Scholar |
Berdejó C, Yuchtman N (2013) Crime, punishment, and politics: an analysis of political cycles in criminal sentencing. The Review of Economics and Statistics 95, 741–756.
| Crime, punishment, and politics: an analysis of political cycles in criminal sentencing.Crossref | GoogleScholarGoogle Scholar |
Bernasco W (2008) Them again? Same offender involvement in repeat and near repeat burglaries. European Journal of Criminology 5, 411–431.
| Them again? Same offender involvement in repeat and near repeat burglaries.Crossref | GoogleScholarGoogle Scholar |
Bernasco W, Johnson SD, Ruiter S (2015) Learning where to offend: effects of past on future burglary locations. Applied Geography 60, 120–129.
| Learning where to offend: effects of past on future burglary locations.Crossref | GoogleScholarGoogle Scholar |
Bowers KJ, Johnson SD (2003) Measuring the geographical displacement and diffusion of benefit effects of crime prevention activity. Journal of Quantitative Criminology 19, 275–301.
| Measuring the geographical displacement and diffusion of benefit effects of crime prevention activity.Crossref | GoogleScholarGoogle Scholar |
Bowers KJ, Johnson SD (2005) Domestic burglary repeats and space–time clusters: the dimensions of risk. European Journal of Criminology 2, 67–92.
| Domestic burglary repeats and space–time clusters: the dimensions of risk.Crossref | GoogleScholarGoogle Scholar |
Bowers KJ, Johnson SD, Guerette RT, Summers L, Paynton S (2011) Spatial displacement and diffusion of benefits among geographically focused policing initiatives: a meta-analytical review. Journal of Experimental Criminology 7, 347–374.
| Spatial displacement and diffusion of benefits among geographically focused policing initiatives: a meta-analytical review.Crossref | GoogleScholarGoogle Scholar |
Bowman DMJS, Williamson GJ, Abatzoglou JT, Kolden CA, Cochrane MA, Smith AMS (2017) Human exposure and sensitivity to globally extreme wildfire events. Nature Ecology & Evolution 1, 0058
| Human exposure and sensitivity to globally extreme wildfire events.Crossref | GoogleScholarGoogle Scholar |
Butry DT, Prestemon JP (2005) Spatiotemporal wildland arson crime functions. Paper presented at the Annual Meeting of the American Agricultural Economics Association, Providence, RI, 26–29 July 2005. (Agricultural and Applied Economics Association: Milwaukee, WI). Available at http://ageconsearch.umn.edu/record/19197 [Verified 20 December 2017]
Cameron AC, Trivedi PK (1998) ‘Regression analysis of count data.’ (Cambridge University Press: Cambridge, MA, USA)
Canela-Cacho JA, Blumstein A, Cohen J (1997) Relationship between the offending frequency of imprisoned and free offenders. Criminology 35, 133–176.
| Relationship between the offending frequency of imprisoned and free offenders.Crossref | GoogleScholarGoogle Scholar |
Clarke RV, Weisburd D (1994) Diffusion of crime control benefits: observations on the reverse of displacement. In ‘Crime prevention studies’. (Ed. RV Clarke) Vol. 2, pp. 165–183 (Criminal Justice Press: Monsey, NY, USA)
Cohen J (1941) The geography of crime. The Annals of the American Academy of Political and Social Science 217, 29–37.
Cohen LE, Felson M (1979) Social change and crime rate trends: a Routine Activity approach. American Sociological Review 44, 588–605.
| Social change and crime rate trends: a Routine Activity approach.Crossref | GoogleScholarGoogle Scholar |
Cornish D, Clarke RV (1987) Understanding crime displacement: an application of Rational Choice Theory. Criminology 25, 933–948.
| Understanding crime displacement: an application of Rational Choice Theory.Crossref | GoogleScholarGoogle Scholar |
Donoghue LR, Main WA (1985) Some factors influencing wildfire occurrence and measurement of fire prevention effectiveness. Journal of Environmental Management 20, 87–96.
Dormann CF, McPherson JM, Araújo MB, Bivand R, Bolliger J, Carl G, Davies RG, Hirzel A, Jetz W, Kissling WD, Kühn I, Ohlemüller R, Peres-Neto PR, Reineking B, Schröder B, Schurr FM, Wilson R (2007) Methods to account for spatial autocorrelation in the analysis of species distributional data: a review. Ecography 30, 609–628.
| Methods to account for spatial autocorrelation in the analysis of species distributional data: a review.Crossref | GoogleScholarGoogle Scholar |
Dyke A (2007) Electoral cycles in the administration of criminal justice. Public Choice 133, 417–437.
| Electoral cycles in the administration of criminal justice.Crossref | GoogleScholarGoogle Scholar |
Eck JE (1993) The threat of crime displacement. Criminal Justice Abstracts 253, 527–546.
Efthyvoulou G (2012) Political budget cycles in the European Union and the impact of political pressures. Public Choice 153, 295–327.
| Political budget cycles in the European Union and the impact of political pressures.Crossref | GoogleScholarGoogle Scholar |
Fiscalía General del Estado Medio Ambiente y Urbanismo (2016) Memoria 2015: Medio Ambiente y Urbanismo. (Fiscalía General del Estado, Medio Ambiente y Urbanismo: Madrid, Spain) Available at: http://www.fiscal.es/fiscal/PA_WebApp_SGNTJ_NFIS/descarga/Memoria%202015%20Fiscal%C3%ADa%20de%20Medio%20Ambiente.pdf?idFile=0a07ddd4-c77a-472e-9f38-a003b571ca5e [Verified 4 June 2018]
Fosberg MA (1978) Weather in wildland fire management: the fire weather index. Conference on Sierra Nevada Meteorology, 19–21 June Lake Tahoe, CA, pp. 1–4 (American Meteorological Society and USDA Forest Service: Boston, MA, USA).
Fuller WA (1996) ‘Introduction to statistical time series, 2nd edn.’ (Wiley: New York, NY, USA)
Gill AM, Christian KR, Moore PHR, Forrester RI (1987) Bushfire incidence, fire hazard and fuel reduction burning. Australian Journal of Ecology 12, 299–306.
| Bushfire incidence, fire hazard and fuel reduction burning.Crossref | GoogleScholarGoogle Scholar |
Goldstein H (1979) Improving policing: a Problem-Oriented Approach. Crime and Delinquency 254, 236–258.
| Improving policing: a Problem-Oriented Approach.Crossref | GoogleScholarGoogle Scholar |
González JL, Muñoz V, Calcerrada ML, Sotoca A (2017) Perfil psicosocial del incendiario forestal español privado de libertad. Behavior & Law Journal 3, 26–34.
Grubb JA, Nobles R (2016) A spatiotemporal analysis of arson. Journal of Research in Crime and Delinquency 53, 66–92.
| A spatiotemporal analysis of arson.Crossref | GoogleScholarGoogle Scholar |
Guerette RT (2009) Analyzing crime displacement and diffusion. ‘Problem-Oriented Guides for Police Problem-Solving Tools Series No. 10’ (US Department of Justice: Washington, DC, USA) Available at https://ric-zai-inc.com/Publications/cops-p167-pub.pdf [Verified 7 May 2019]
Guerette RT, Bowers KJ (2009) Assessing the extent of crime displacement and diffusion of benefits: a review of situational crime prevention evaluations. Criminology 47, 1331–1368.
| Assessing the extent of crime displacement and diffusion of benefits: a review of situational crime prevention evaluations.Crossref | GoogleScholarGoogle Scholar |
Hall P (1985) Resampling a coverage pattern. Stochastic Processes and their Applications 20, 231–246.
| Resampling a coverage pattern.Crossref | GoogleScholarGoogle Scholar |
Hovardas T (2014) ‘Playing with fire’ in a pre-election period: newspaper coverage of 2007 wildfires in Greece. Society & Natural Resources 27, 689–705.
| ‘Playing with fire’ in a pre-election period: newspaper coverage of 2007 wildfires in Greece.Crossref | GoogleScholarGoogle Scholar |
Hovardas T (2015) An ‘asymmetric threat’ that should have been anticipated: political discourse on 2007 wildfires in Greece. Environmental Communication 9, 409–427.
| An ‘asymmetric threat’ that should have been anticipated: political discourse on 2007 wildfires in Greece.Crossref | GoogleScholarGoogle Scholar |
Jolly WM, Cochrane MA, Freeborn PH, Holden ZA, Brown TJ, Williamson GJ, Bowman DMJS (2015) Climate-induced variations in global wildfire danger from 1979 to 2013. Nature Communications 6, 7537
| Climate-induced variations in global wildfire danger from 1979 to 2013.Crossref | GoogleScholarGoogle Scholar | 26172867PubMed |
Keetch JJ, Byram GM (1968) A drought index for forest fire control. USDA Forest Service, Research Paper SE-38. (Southeastern Forest Experiment Station: Asheville, NC, USA).
Kocsis RN, Irwin HJ (1997) An analysis of spatial patterns in serial rape, arson, and burglary: the utility of the circle theory of environmental range for psychological profiling. Psychiatry, Psychology and Law 4, 195–206.
| An analysis of spatial patterns in serial rape, arson, and burglary: the utility of the circle theory of environmental range for psychological profiling.Crossref | GoogleScholarGoogle Scholar |
Krawchuk MA, Moritz MA, Parisien M-A, Van Dorn J, Hayhoe K (2009) Global pyrogeography: the current and future distribution of wildfire. PLoS One 4, e5102
| Global pyrogeography: the current and future distribution of wildfire.Crossref | GoogleScholarGoogle Scholar | 19352494PubMed |
Kull CA (2002) Madagascar aflame: landscape burning as peasant protest, resistance, or resource management tool? Political Geography 21, 927–953.
| Madagascar aflame: landscape burning as peasant protest, resistance, or resource management tool?Crossref | GoogleScholarGoogle Scholar |
Leamer EE (1983) Let’s take the con out of econometrics. The American Economic Review 77, 31–43.
Levine R, Renelt D (1992) A sensitivity analysis of cross-country growth regressions. The American Economic Review 82, 942–963.
Levitt SD (1997) Using electoral cycles in police hiring to estimate the effect of police on crime. The American Economic Review 87, 270–290.
Levitt SD (1998) Why do increased arrest rates appear to reduce crime? Deterrence, incapacitation, or measurement error? Economic Inquiry 36, 353–372.
| Why do increased arrest rates appear to reduce crime? Deterrence, incapacitation, or measurement error?Crossref | GoogleScholarGoogle Scholar |
Liu RY, Singh K (1992) Moving-blocks jacknife and bootstrap capture weak dependence. In ‘Exploring the limits of the bootstrap’. (Eds R Lesage, L Billard) pp. 225–248 (Wiley: New York, NY, USA).
Liu Y, Stanturf J, Goodrick S (2010) Trends in global wildfire potential in a changing climate. Forest Ecology and Management 259, 685–697.
| Trends in global wildfire potential in a changing climate.Crossref | GoogleScholarGoogle Scholar |
Mothershead PT (2012) Geospatial analysis of socioeconomic risk factors affecting wildfire arson occurrence in the south-eastern United States. MS thesis, North Carolina State University, Raleigh, NC, USA.
Phillips C (2011) Situational crime prevention and crime displacement: myths and miracles? Internet Journal of Criminology. Available at https://docs.wixstatic.com/ugd/b93dd4_d84b686ba20d4058941fc90e4b2439cd.pdf [Verified 7 May 2019]
Pogarsky G, Piquero AR, Paternoster R (2004) Modeling change in perceptions about sanction threats: the neglected linkage in deterrence theory. Journal of Quantitative Criminology 20, 343–369.
| Modeling change in perceptions about sanction threats: the neglected linkage in deterrence theory.Crossref | GoogleScholarGoogle Scholar |
Ponte Pintor JM, Bandín Buján C (2008) Los incendios forestales en Galicia y su investigación. Estudios Penales y Criminológicos XXVIII, 317–341.
Prestemon JP, Butry DT (2005) Time to burn: modeling wildland arson as an autoregressive crime function. American Journal of Agricultural Economics 87, 756–770.
| Time to burn: modeling wildland arson as an autoregressive crime function.Crossref | GoogleScholarGoogle Scholar |
Prestemon JP, Butry DT (2010) Wildland arson: a research assessment. In ‘Advances in threat assessment and their application to forest and rangeland management’. (Eds JM Pye, HM Rauscher, Y Sands, DC Lee, JS Beatty) USDA Forest Service, General Technical Report PNW-802, pp. 271–283. (Pacific Northwest Research Station: Portland, OR, USA)
Prestemon JP, Chas-Amil ML, Touza Montero J, Goodrick SJ (2012) Forecasting intentional wildfires using temporal and spatiotemporal autocorrelations. International Journal of Wildland Fire 21, 743–754.
| Forecasting intentional wildfires using temporal and spatiotemporal autocorrelations.Crossref | GoogleScholarGoogle Scholar |
Prestemon JP, Butry DT, Thomas DS (2013) Exploiting autoregressive properties to develop prospective urban arson forecasts by target. Applied Geography 44, 143–153.
| Exploiting autoregressive properties to develop prospective urban arson forecasts by target.Crossref | GoogleScholarGoogle Scholar |
Pyne SJ (1995) ‘World fire.’ (Henry Holt: New York, NY, USA)
Ramos R, Sanz C (2018) Backing the incumbent in difficult times: the electoral impact of wildfires. Working paper no. 1810. (Banco de España) Available at www.bde.es/f/webbde/SES/Secciones/Publicaciones/PublicacionesSeriadas/DocumentosTrabajo/18/Files/dt1810e.pdf [Verified 6 June 2018]
Ratcliffe JH (2016) ‘Intelligence-led policing.’ (Routledge: London, UK)
Ratcliffe JH, Rengert GF (2008) Near repeat patterns in Philadelphia shootings. Security Journal 21, 58–76.
| Near repeat patterns in Philadelphia shootings.Crossref | GoogleScholarGoogle Scholar |
Sebastián-López A, Salvador-Civil R, Gonzalo-Jiménez J, San-Miguel-Ayanz J (2008) Integration of socio-economic and environmental variables for modelling long-term fire danger in southern Europe. European Journal of Forest Research 127, 149–163.
| Integration of socio-economic and environmental variables for modelling long-term fire danger in southern Europe.Crossref | GoogleScholarGoogle Scholar |
Seijo F (2005) The politics of fire: Spanish forest policy and ritual resistance in Galicia, Spain. Environmental Policy 14, 380–402.
Seijo F (2009) Who framed the forest fire? State framing and peasant counter-framing of anthropogenic forest fires in Spain since 1940. Journal of Environmental Policy and Planning 11, 103–128.
| Who framed the forest fire? State framing and peasant counter-framing of anthropogenic forest fires in Spain since 1940.Crossref | GoogleScholarGoogle Scholar |
Skouras S, Christodoulakis N (2014) Electoral misgovernance cycles: evidence from wildfires and tax evasion in Greece. Public Choice 159, 533–559.
| Electoral misgovernance cycles: evidence from wildfires and tax evasion in Greece.Crossref | GoogleScholarGoogle Scholar |
Smith KB (2004) The politics of punishment: evaluating political explanations of incarceration rates. The Journal of Politics 66, 925–938.
| The politics of punishment: evaluating political explanations of incarceration rates.Crossref | GoogleScholarGoogle Scholar |
Sotoca Plaza A (2016) Perfil criminológico del incendiario forestal: estudio empírico basado en la evidencia. PhD dissertation, Universidad Complutense de Madrid, Spain.
Telep CW, Weisburd D, Gill CE, Vitter Z, Teichman D (2014) Displacement of crime and diffusion of crime control benefits in large-scale geographic areas: a systematic review. Journal of Experimental Criminology 10, 515–548.
| Displacement of crime and diffusion of crime control benefits in large-scale geographic areas: a systematic review.Crossref | GoogleScholarGoogle Scholar |
Thomas DS, Butry DT, Prestemon JP (2011) Enticing arsonists with broken windows and social disorder. Fire Technology 47, 255–273.
| Enticing arsonists with broken windows and social disorder.Crossref | GoogleScholarGoogle Scholar |
Townsley MT, Homel R, Chaseling J (2003) Infectious burglaries: a test of the near repeat hypothesis. British Journal of Criminology 43, 615–633.
| Infectious burglaries: a test of the near repeat hypothesis.Crossref | GoogleScholarGoogle Scholar |
Weisburd D, Eck JE (2004) What can police do to reduce crime, disorder, and fear? The Annals of the American Academy of Political and Social Science 593, 42–65.
| What can police do to reduce crime, disorder, and fear?Crossref | GoogleScholarGoogle Scholar |
Weisburd D, Telep C, Hinkle J, Eck J (2010) Is Problem-Oriented Policing effective in reducing crime and disorder? Findings from a Campbell systematic review. Criminology & Public Policy 9, 139–172.
| Is Problem-Oriented Policing effective in reducing crime and disorder? Findings from a Campbell systematic review.Crossref | GoogleScholarGoogle Scholar |
Zeger SL, Qaqish B (1988) Markov regression models for time series: a quasi-likelihood approach. Biometrics 44, 1019–1031.
| Markov regression models for time series: a quasi-likelihood approach.Crossref | GoogleScholarGoogle Scholar | 3148334PubMed |
1 In this study, we define all intentional firesetting as illegal and so refer to intentional illegal firesetting as ‘intentional firesetting’.
2 Mechanisms proposed or identified for how electoral cycles could influence intentional firesetting include election-related adjustments in law enforcement effectiveness – law enforcement budgets (Efthyvoulou 2012) and police force levels (Levitt 1997) – and the scope or severity of criminal sanctions (Smith 2004; Dyke 2007; Berdejó and Yuchtman 2013).
3 Another way to view ‘motivation’ is in terms of the prospective offender’s expected net psychic or monetary benefits of crime commission, in the form advanced by Becker (1968). Hence, an increased probability of arrest would be expected to lower the expected net benefits of crime commission.