Evaluating a simulation-based wildfire burn probability map for the conterminous US
Amanda R. Carlson

A
B
C
D
E
F
Abstract
Wildfire simulation models are used to derive maps of burn probability (BP) based on fuels, weather, topography and ignition locations, and BP maps are key components of wildfire risk assessments.
Few studies have compared BP maps with real-world fires to evaluate their suitability for near-future risk assessment. Here, we evaluated a BP map for the conterminous US based on the large fire simulation model FSim.
We compared BP with observed wildfires from 2016 to 2022 across 128 regions representing similar fire regimes (‘pyromes’). We evaluated the distribution of burned areas across BP values, and compared burned area distributions among fire size classes.
Across all pyromes, mean BP was moderately correlated with observed burned area. An average of 71% of burned area occurred in higher-BP classes, vs 79% expected. BP underpredicted burned area in the Mountain West, especially for extremely large fires.
The FSim BP map was useful for estimating subsequent wildfire hazard, but may have underestimated burned areas where input data did not reflect recent climate change, vegetation change or human ignition patterns.
Our evaluations indicate that caution is needed when relying on simulation-based BP maps to inform management decisions. Our results also highlight potential opportunities to improve model estimates.
Keywords: burn probability, climate change, fire simulation models, FSim, model evaluation, pyromes, risk assessment, wildfire hazard, wildland fire.
Introduction
Wildfires pose many challenges to human health and safety, economic values, biodiversity and ecosystem services. Although wildfire is a natural component of many ecosystems and may be ecologically beneficial, extreme events threaten lives, cause billions of dollars in property loss, create the potential for post-fire hazards such as flooding and debris flows, and lead to widespread adverse public health effects from smoke exposure (Raymond et al. 2020; Vardoulakis et al. 2020; Bayham et al. 2022). Many parts of the world are experiencing increasingly large and destructive wildfires, including the unprecedented events of the 2019–2020 season in eastern Australia (Boer et al. 2020), the 2020 and 2021 seasons in western North America (Higuera and Abatzoglou 2021) and the 2022 season in Mediterranean Europe (Rodrigues et al. 2023). These extreme events are expected to become more frequent and destructive as a result of climate change and expansion of the wildland–urban interface (Senande-Rivera et al. 2022; Schug et al. 2023). Given these challenges, government agencies, conservation organisations and communities need information and tools to assess and manage wildfire risk (Calkin et al. 2014).
Wildfire risk assessments are valuable tools for determining where communities and other values are most likely to be exposed to wildfire, and for planning adaptations accordingly (Haas et al. 2013; Calkin et al. 2014). According to the risk assessment framework developed by the US Forest Service, risk is defined quantitatively by combining wildfire hazard (likelihood of fire occurrence and likely intensity) and resource vulnerability, using response functions to link wildfire of a given intensity to change in value for a particular resource (Scott et al. 2013). Landscape-scale risk maps are then used to prioritise mitigation actions at local to national scales, including allocating suppression resources, implementing fuel treatments and developing community response plans (Schoennagel et al. 2017; Murray et al. 2023). Risk maps also inform communities’ perceptions of wildfire danger and can thus motivate homeowners to invest in home-hardening actions that can reduce building loss (McFarlane et al. 2011).
Simulation models are commonly used to estimate the hazard components of wildfire risk (Oliveira et al. 2021). Models such as FlamMap (Finney 2006), FSim (Finney et al. 2011), or Burn-P3 (Parisien et al. 2005) are based on empirical or semi-empirical fire spread models such as FARSITE (Finney 1998) and the Minimum Travel Time algorithm (MTT; Finney 2002). These algorithms predict the growth of individual wildfire perimeters based on ignition locations, fuels, topography and weather conditions, including wind speed and direction. Simulation models are used to generate burn probability (BP) maps using a Monte Carlo approach in which individual wildfire perimeters are generated across landscapes over several thousands of iterations, representing hypothetical ‘fire years’ with varying ignition locations and weather scenarios. BP is derived by dividing the number of times a pixel burns in the simulation by the total number of iterations. BP is therefore interpreted as the probability that a pixel will burn in any given year. This simulation modelling approach has been used in several national-scale risk assessments, including for the US (using FSim; US Department of Agriculture et al. 2020), Israel (using FSim; Carmel et al. 2009), southern Europe (using MTT; Alcasena et al. 2021) and Canada (using Burn-P3; Parisien et al. 2005).
Despite increasing reliance on simulation models in wildfire risk assessments, there have been few efforts to determine whether their outputs align with observed wildfire perimeters at spatial and temporal scales suitable for informing management actions. Past studies have found that observed burned areas and fire size distributions match simulations well when aggregated at fairly coarse scales in the conterminous US (Finney et al. 2011; Ager et al. 2021). Additional studies have evaluated BP maps at small regional scales by comparing perimeters for individual fires with simulation-derived BP values, and determined that burned areas corresponded well to high-BP areas (Paz et al. 2011; Alcasena et al. 2015; Thompson et al. 2016). In contrast, Beverly and McLoughlin (2019) compared BP maps generated with the Burn-P3 model with 138 observed fires over several years in several regions of Alberta, Canada, and found that a high proportion of burned area occurred in lower-BP areas. However, Parisien et al. (2020) argued that the analysis by Beverly and McLoughlin (2019) was misleading because observed fires over a period of only a few years cannot capture the full range of variability represented by BP maps that are based on simulations of thousands of hypothetical fire years. This is indeed an important consideration when evaluating BP maps. Wildfire is a highly stochastic process, and burned areas may vary greatly from year to year depending on weather conditions and ignition locations. It is therefore not possible to reliably evaluate maps at very fine scales (i.e. on a per-pixel basis) using available fire records that only span a few decades or less. However, evaluating patterns in wildfire burned area on an aggregate scale, across large spatial extents, can indicate whether burned areas generally occur in areas mapped as having higher BP or not. Such evaluations are important for determining the usefulness of BP maps in informing actions to mitigate near-term wildfire risk.
As with any model, evaluations of wildfire simulation models can help users understand their strengths and limitations and point to opportunities for improvement. Limitations may lie either with model input data or from their underlying empirical spread equations. Wildfire simulation models have been critiqued for lack of field validation of their predictions of spread rates and crown fire probabilities (Alexander and Cruz 2013), as well as their representations of spread processes in wildland–urban interface fuels (Mell et al. 2010). Furthermore, simulations based on historical weather ranges may have limited ability to capture current and near-future burning patterns in regions of the western US where wildfire activity is rapidly shifting owing to climate change (Adams 2013; Abatzoglou et al. 2021; Iglesias et al. 2022). BP maps based on weather ranges and burn patterns seen in the historical record may therefore have limited utility to guide management under future conditions. Additionally, fuels are changing rapidly in some regions affected by large-scale forest mortality (Anderegg et al. 2015) or spread of invasive grasses (Fusco et al. 2019), and simulations may therefore be limited by out-of-date fuel maps. Finally, simple ignition density surfaces used in simulations may not capture fine-scale human ignition patterns that may be important predictors of wildfire occurrence (Bar Massada et al. 2011). Identifying where observed wildfires most differ from BP maps can help to identify how any these factors may be improved to result in more realistic simulations.
In this study, we evaluated an FSim-based BP map for the conterminous US. We focused on the BP output because BP can be readily compared with mapped wildfire perimeters, whereas there are no readily available data to evaluate FSim’s intensity outputs (i.e. flame length probability). We compared BP values with observed wildfires over a 7-year period after the period of model calibration (a dataset spanning 7 years; n = 3112 fires). We replicated our evaluations across 128 sub-regions representing similar fuel types and fire regimes (‘pyromes’). Our analysis consisted of four components: (1) comparing predicted and observed percentages of mean annual burned area across pyromes; (2) comparing expected with observed proportions of burned area among equal-area BP classes within pyromes; (3) assessing how results for the western US were affected by extremely large fires by assessing differences in proportions of observed burned area per BP class among three fire size classes; and (4) comparing results based on an updated BP map using more recent calibration data.
Methods
Data
The US Department of Agriculture Forest Service provides a publicly available FSim-based BP map for the conterminous US at 270 m resolution (Fig. 1a; Short et al. 2020b). This version of the map was released in 2020 and was based on 2014 LANDFIRE fuels data (Rollins 2009), an ignition density surface based on wildfire point locations >40 ha from 1992 to 2015 from the Fire Program Analysis Fire Occurrence Database (Short 2017) and historical weather from 1972 to 2012 (Abatzoglou 2013). The FSim model used to derive this map generates wildfire perimeters for every pixel across the conterminous US that contains burnable fuels according to the LANDFIRE fuels map. Pixels that do not burn in any simulation runs are assigned nominal values of 0.00001. Pixels with developed, agricultural, water, or other non-vegetated land cover types are considered ‘non-burnable’ in the FSim runs and are assigned values of zero. We set these zero values to null to exclude them from our analyses.
(a) The USDA Forest Service’s FSim burn probability (BP) map for the conterminous US, based on 2014 LANDFIRE fuels, 1972–2012 weather ranges and observed wildfires from 1992 to 2015. (b) Wildfire perimeters from 2016 to 2022 used to evaluate the BP map, with Omernik Level 1 ecoregions and pyrome boundaries indicated. M.W.C.F, Marine West Coast Forest; M.C., Mediterranean California; N.F.M., Northwestern Forested Mountains; N.A.D., North American Deserts; S.S.H., Southern Semi-Arid Highlands; T.S., Temperate Sierras; G.P., Great Plains; N.F., Northern Forests; E.T.F., Eastern Temperate Forests; T.W.F., Tropical Wet Forests. (c) Observed and modelled mean annual burned areas per ecoregion, as a proportion of total LANDFIRE burnable area.
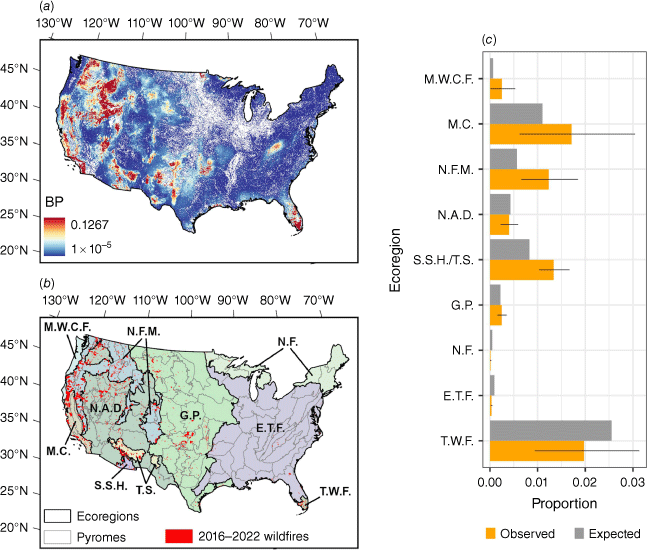
Observed wildfire perimeters from 2016 to 2021 were obtained from the Monitoring Trends in Burn Severity (MTBS) dataset (Eidenshink et al. 2007), and additional perimeters for 2022 wildfires were obtained from the National Interagency Fire Center (NIFC; https://data-nifc.opendata.arcgis.com/maps/wfigs-interagency-fire-perimeters; accessed 9 December 2022). The MTBS data only include fires larger than 404 ha in the western US and 202 ha in the eastern US. We removed fires that were smaller than this threshold from the NIFC data prior to merging the two datasets. We additionally removed prescribed fires from both datasets. For the MTBS data, we also removed wildfires with ‘unknown’ incident type, as many of these may be undocumented prescribed fires. The final combined MTBS and NIFC dataset consisted of 3112 fires and 163,914 km2 burned area (Fig. 1b).
Pyromes are ecologically similar regions with relatively homogeneous fire regimes (Fig. 1b; Short et al. 2020a). These units were developed by the USDA Forest Service to define areas of homogeneous fire activity based on geospatial clustering of observed wildfires, and were derived directly from FSim outputs. We selected these as our units of analysis because they are large enough to encompass a large range of BP values and multiple wildfire perimeters, and to display patterns in model performance in an interpretable way, while also being fine enough to allow for sufficient replication across the entire study area (mean size 60,857 km2, n = 128). We obtained pyrome boundaries from the USDA Forest Service Research Data Archive (https://www.fs.usda.gov/rds/archive/catalog/RDS-2020-0020).
The conterminous US encompasses a wide range of fuels, ignition sources and weather patterns, and we therefore grouped our analyses by Omernik Level 1 ecoregions (Fig. 1b; Omernik 1987). We simplified these ecoregions somewhat by combining the Southern Semi-Arid Highlands with the Temperate Sierras. Pyromes were partially derived from Level 4 ecoregions, but did not perfectly overlap. We assigned each pyrome to the dominant Level 1 ecoregion by determining which ecoregion had the greatest degree of overlap with LANDFIRE burnable area (Supplementary Fig. S1, Supplementary Table S1).
Expected and observed burned areas by pyrome
The first component of our analysis was to evaluate relationships between expected and observed mean annual burned areas by pyrome. We tabulated observed burned area by year to calculate mean annual burned areas for each pyrome. We determined 95% confidence intervals for mean annual burned areas using a sieve bootstrap, a non-parametric method that accounts for temporal autocorrelation and effects of large outlier years (Bühlmann 1997). We then divided these values by the total burnable area (non-zero pixels) to derive proportions of observed burned area. Expected annual burned area proportions were determined by calculating the mean of all burnable BP pixels within each pyrome. We evaluated the significance of expected to observed correlations used a zero-inflated generalised additive model with a beta response distribution. Regressions were split by major geographic region (eastern and western US), wherein the western US was defined as the Marine West Coast Forest, Mediterranean California, Northwestern Forested Mountains, North American Deserts, and Southern Semi-Arid Highlands and Temperate Sierras ecoregions, and the eastern US as the Great Plains, Northern Forests, Eastern Temperate Forests and Tropical Wet Forests ecoregions (Fig. 1b).
Burned area proportions by BP class
We assessed the BP map within individual pyromes by comparing expected with observed proportions of burned area along a gradient of BP classes (Fig. 2). We defined BP classes independently for each pyrome by dividing pixel values (excluding zero values) into equal-area quintiles, resulting in Low, Low–Medium, Medium, Medium–High and High classes of wildfire likelihood. We selected five classes because we determined this to be the best number of classes for differentiating fire likelihood across landscapes while also allowing interpretable results. However, we compared proportions of area in the lower-BP classes with those obtained using three, four and six classes, and determined that they were similar (Supplementary Fig. S2). Our analysis methods are therefore robust to the number of classes selected.
Diagram of methodology used to define burn probability classes and calculate expected proportions of burned area based on FSim burn probabilities (BP). (a) BP map cropped for an example pyrome (Far Southern Rockies). (b) Map of five equal-area classes based on quantile breaks of BP values. (c) Histogram of BP values for the Far Southern Rockies with class breaks (coloured vertical lines). BP values were summed within each class group, then divided by the total to obtain expected proportions of burned area per class (d). Dashed horizontal line in (d) indicates the approximate expected proportion of burned area per BP class if burned area occurred randomly.
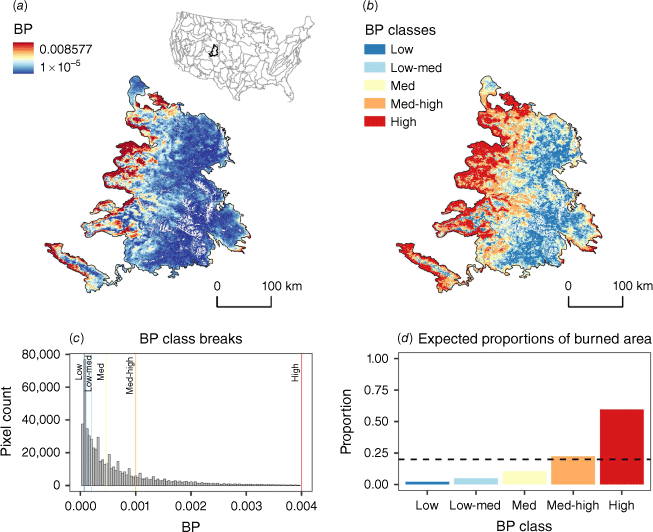
Within each pyrome, we determined the expected proportions of burned area per BP class i (E(Pi)) by summing all BP pixel values within the areas defined by class i, then dividing by the sum of all BP pixels (BPtotal; Fig. 2c, d, Eqn 1). We calculated observed proportions per BP class by tabulating all pixels overlapping 2016–2022 wildfire perimeters by year, thereby accounting for pixels that burned in multiple years. We then calculated means of expected and observed proportions per pyrome by Level 1 ecoregion, for the eastern and western US, and for the entire conterminous US. Because proportional data are constrained so that proportions sum to 1, the data must be transformed to Euclidean space to find the means. We used the additive log-ratio transformation (Aitchison 1986) to find the centre of transformed proportions for all pyromes for each geographic division. We derived mean proportions by taking the inverse additive log-ratio of each centre of transformed proportions.
We used Aitchison’s D metric for proportional data (Aitchison 1992) to assess differences between observed and expected distributions of burned area per BP class for each pyrome. However, this method results in similar D values for pyromes where burned area is greater than expected in higher-BP classes to pyromes where burned area was greater than expected in lower-BP classes. For our evaluations, we were more concerned with identifying areas where more burned area occurred in lower-BP classes, as this would indicate potential for models to underestimate wildfire risk. We therefore split pyromes according to whether more burned area than expected fell into the two lower BP classes (Low and Low-Med), or into the higher-BP classes (Med, Med-High and High).
Fire size class comparison
In the western US (defined here as the Marine West Coast Forest, Mediterranean California, Northwestern Forested Mountains, North American Deserts, Southern Semi-Arid Highlands and Temperate Sierras ecoregions), wildfire burned area is often dominated by extremely large events. We evaluated the effect of extremely large wildfire events on our assessments of burned area proportions per BP class, as we hypothesised that these events are more likely to occur under extreme weather conditions and therefore may result in greater burned area in low-probability areas. We based our fire size classes on the 95th and 99th percentile breaks for all 2016–2022 wildfire perimeters included in our assessment, limited to ecoregions of the western US. We split the burned area perimeters into our three fire size classes (<95th, 95th–99th and >99th percentiles) to determine proportions of burned area per BP class for each pyrome. We then determined mean proportions for all pyromes for each size class.
BP map version comparison
We focused on evaluating the version of the BP map released in 2020 and based on 2014 LANDFIRE fuels because this version has been widely implemented in regional, state and national risk assessment products in the US (Hawbaker et al. 2023), and because the vintage of the fuels map and historical calibration data allowed for 7 years of subsequent observed fires for evaluation. However, an update to the map was released in late 2023 (Dillon et al. 2023). This newer version is based on observed wildfires from 1992 to 2020, a 2020 LANDFIRE fuels map and a more recent window of historical weather (2006–2020; Supplementary Fig. S3). We did not perform a thorough evaluation of the 2023 release of the USDA Forest Service’s BP map, owing to limitations of only 2 years of evaluation data subsequent to the modelling period (2021–2022). Although it is desirable to perform evaluations using data that are independent from the calibration data, it is also impossible to evaluate fires that occurred prior to the 2020 LANDFIRE fuels vintage map because the fuels map reflects vegetation changes from these past burned areas. However, we used these 2 years of observed fire perimeters to compare burned area proportions by BP class between the two map versions in order to evaluate differences resulting from a more recent historical weather range and fuels map. We hereafter refer to the two different versions based on the LANDFIRE fuels vintage as ‘LF2014’ and ‘LF2020’.
Results
Wildfires occurred in 114 of 128 pyromes from 2016 to 2022. Mean annual burned area for this period was 23,416 km2 (±10,586 km2 s.d.) compared with 14,235 km2 (±9158 km2) for the 1992–2015 calibration period. At the ecoregion level, the mean annual percentage of burnable area affected ranged from 0.01% (in the Northern Forests) to 1.97% (in the Tropical Wet Forests). Both expected and observed proportions of burned area from 2016 to 2022 were greatest for the Tropical Wet Forests ecoregion (consisting of only one pyrome, the Southern Florida Coastal Plain/Everglades) and the western ecoregions (Fig. 1c). Observed mean annual burned areas matched expected burned areas well at the ecoregion level, but in both the Northwestern Forested Mountains and the Southern Semi-Arid Highlands, the 95% confidence interval for observed mean annual burned area exceeded the expected value (Fig. 1c). Mean observed burned area was approximately double the expected area in the Northwestern Forested Mountains (1.2% observed vs 0.57% expected).
Expected and observed burned areas by pyrome
In individual pyromes, observed mean annual percentage area burned was 0.56% on average (excluding pyromes with no area burned). The maximum mean annual percentage area burned was 4.85% in the Klamath Mountains. Expected and observed burned area was significantly positively correlated when aggregated by pyrome (R2 = 0.30, P < 0.001 for the western US; R2 = 0.23, P < 0.001 for the eastern US). However, there were notable outliers in the western US in which several pyromes had greater burned area than other pyromes with similar BP values (Fig. 3). These pyromes were all located in the southern Cascade Range, northern California coast and southern Sierra Nevada regions. All of these regions experienced historic wildfire seasons related to extreme heat and drought throughout the 2016–2022 evaluation period, including extreme events such as the 2018 August Complex and Camp fires in northern California, multiple large wildfires in the western Cascade Range during the 2020 season (Rosen et al. 2022), and the 2021 Dixie fire in the northern Sierra Nevada and southern Cascade Range. Outlying pyromes were not as notable in the eastern US as in the western US (Fig. 3). Two pyromes – the Canadian/Cimarron Breaks and High Plains and the Rolling Red Hills and Prairie Tableland, which are both located in the Oklahoma/Texas panhandle region of the southern Great Plains – had slightly greater burned areas than other pyromes with similar BP values. BP slightly overestimated burned area in the Southern Florida Coastal Plain/Everglades, the pyrome with greatest burned area in the eastern US, and in several pyromes in the Great Plains and Northern Forests.
Comparisons between expected and observed mean annual proportion of area burned for 2016–2022, by pyrome. Error bars indicate bootstrapped 95% confidence intervals for annual values. Pyromes are split by major geographic region (Western US = ecoregions west of the Great Plains). Dashed line indicates a 1:1 relationship. Circled and labelled points highlight outlier pyromes where observed burned area greatly exceeded the predicted area. M.W.C.F, Marine West Coast Forest; M.C., Mediterranean California; N.F.M., Northwestern Forested Mountains; N.A.D., North American Deserts; S.S.H./T.S., Southern Semi-Arid Highlands and Temperate Sierras; G.P., Great Plains; N.F., Northern Forests; E.T.F., Eastern Temperate Forests; T.W.F., Tropical Wet Forests.
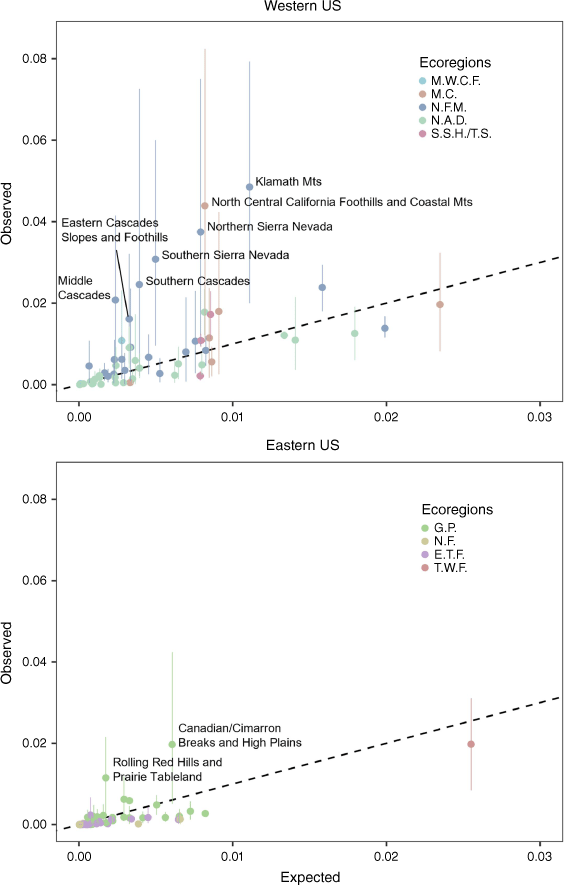
Burned area proportions by BP class
Observed proportions of burned area among our five classes were similar to the expected proportions when averaged across all 114 pyromes in the conterminous US that experienced wildfires from 2016 to 2022 (Fig. 4a, Table 1). The mean proportion of observed burned area in the Med-High or High-BP classes was approximately two-thirds (67.8%), greatly exceeding the ~40% that would be expected if burned area were occurring randomly across BP classes. Burned area proportions across BP classes were highly variable from year to year, but in most pyromes, expected burned areas for all BP classes were within a 95% confidence interval of observed means (Supplementary Fig. S4). However, the mean observed proportion in the High-BP class was substantially lower than expected (40.0% vs 53.0%) across all pyromes, whereas the mean proportion in the lower-BP classes (Low to Med) was greater than expected (32.2% vs 22.6%). Results were similar for the western and eastern US, with greater observed proportions in Low to Med-BP classes than expected (Fig. 4). Observed proportion in the Low to Med-BP classes in the western US was 34.4%, compared with 24.5% expected, and 30.0% in the eastern US compared with 21.0% expected.
(a) Mean proportions of expected and observed burned area in each BP class aggregated across all pyromes in the western US, eastern US, and the entire conterminous US. The number of pyromes per region is indicated by n. Regions are split by Omernik Level 1 ecoregions (Western US, Marine West Coast Forest, Mediterranean California, Northwestern Forested Mountains, North American Deserts, Southern Semi-Arid Highlands, and Temperate Sierras; Eastern US, Great Plains, Northern Forests, Eastern Temperate Forests, and Tropical Wet Forests). (b) Aitchison’s distance (D) comparing observed proportions of burned area per burn probability (BP) class with expected proportions per pyrome. Maps are split by pyromes where observed proportions of burned area exceeded expected in Low and Low-Medium BP classes (left, blue shades) and those where observed proportions exceeded expected in Med-High and High BP classes (right, red shades). Pyromes in white with no values indicate where no wildfire occurred from 2016 to 2022. Omernik Level 1 ecoregion groupings are indicated by bold lines.
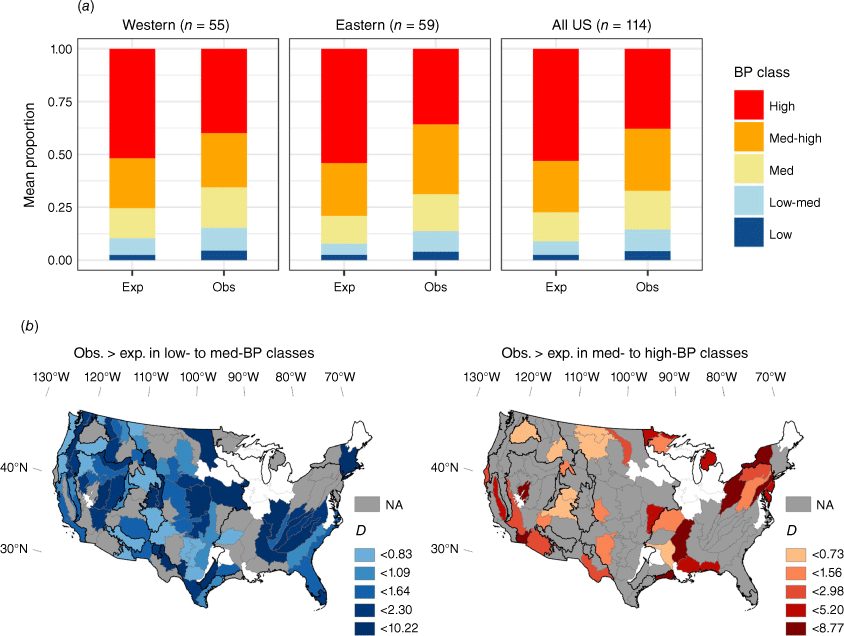
Ecoregion/Region | No. of pyromes A | Low | Low-Med | Med | Med-High | High B | |
---|---|---|---|---|---|---|---|
Marine West Coast Forest | 2 | 0.04 (0.05) | 0.03 (0.06) | 0.08 (0.14) | 0.20 (0.20) | 0.66 (0.55) | |
Mediterranean California | 6 | 0.05 (0.06) | 0.13 (0.12) | 0.19 (0.18) | 0.24 (0.25) | 0.38 (0.39) | |
Northwestern Forested Mountains | 21 | 0.10 (0.03) | 0.16 (0.09) | 0.21 (0.15) | 0.24 (0.24) | 0.28 (0.50) | |
North American Deserts | 23 | 0.02 (0.02) | 0.07 (0.06) | 0.17 (0.12) | 0.26 (0.23) | 0.48 (0.57) | |
Southern Semi-Arid Highlands and Temperate Sierras | 3 | 0.13 (0.05) | 0.14 (0.10) | 0.14 (0.16) | 0.22 (0.24) | 0.36 (0.45) | |
All Western US | 55 | 0.05 (0.03) | 0.11 (0.08) | 0.19 (0.14) | 0.26 (0.24) | 0.40 (0.52) | |
Great Plains | 29 | 0.05 (0.03) | 0.11 (0.09) | 0.17 (0.16) | 0.31 (0.25) | 0.36 (0.48) | |
Northern Forests | 6 | 0.01 (0.02) | 0.09 (0.05) | 0.10 (0.12) | 0.28 (0.24) | 0.52 (0.56) | |
Eastern Temperate Forests | 23 | 0.05 (0.02) | 0.08 (0.03) | 0.19 (0.10) | 0.36 (0.24) | 0.32 (0.61) | |
Tropical Wet Forests | 1 | 0.04 (0.02) | 0.25 (0.09) | 0.31 (0.17) | 0.25 (0.27) | 0.15 (0.46) | |
All Eastern US | 59 | 0.04 (0.03) | 0.10 (0.05) | 0.17 (0.13) | 0.33 (0.25) | 0.36 (0.54) | |
All Conterminous US | 114 | 0.04 (0.03) | 0.10 (0.06) | 0.18 (0.14) | 0.29 (0.24) | 0.38 (0.53) |
When comparing observed burned area proportions with their expected proportions by BP class, 79 of 114 pyromes, or 69.3%, had greater burned area than expected in the Low to Med-BP classes. Pyromes with the greatest difference between observed and expected burned area proportions, as measured by Aitchison’s D, were concentrated in the Cascade Range/northern Sierra Nevada region, the Northern Rockies, Great Basin, southern Rocky Mountains, central and southern Great Plains, southern Appalachian Mountains, coastal northeastern US and Florida Everglades (Fig. 4b). Conversely, pyromes where a greater burned area occurred in the higher-BP classes than expected were concentrated in the desert Southwest, lower Mississippi River valley and Great Lakes region.
Proportions of burned area among BP classes were highly variable across individual pyromes (Figs 5, 6). Seventy-four of 114 pyromes had >50% of their burned area occurring in the two highest BP classes (Med-High or High), and 30 pyromes had >50% of their burned area in the High-BP class. Focusing on the 28 pyromes with the greatest burned areas (top 25%, >0.80% mean annual area burned), fewer than half of these (n = 11) had >50% of burned area in Med-High or High-BP classes. Generally, pyromes with higher amounts of burned area in the lower-BP classes were in the Northwestern Forested Mountains ecoregion (Fig. 5). Notably, the Southern Cascades, Northeast Cascades, Northern Sierran Foothills and Tuscan Flows, Southern Rockies Front Range and Southern Rockies (Upper Colorado River Basin) experienced large wildfire burned areas (>100 km2 burned annually, on average) and had more than 20% of their burned areas occur in the Low-BP class (Fig. 5). Observed burned areas did not necessarily exceed expected values for higher-BP classes in these pyromes, but did for the low-BP classes (including 95% confidence interval ranges; see Supplementary material). Pyromes in Mediterranean California also had relatively high burned areas in lower-BP classes (~40% for most pyromes, Central California Valley excluded; Fig. 5). However, it is important to note that this ecoregion had, on average, lower expected proportions of burned area in the high-BP classes than other ecoregions (Table 1). This is because BP values in these pyromes are high overall and there is therefore less differentiation among BP classes.
Effects of extremely large fires
We split fires in the western US into size classes representing the lower 95th, 95th–99th and upper 99th percentiles, resulting in groups of 1723, 109 and 26 events, respectively. Eleven of the 26 fires in the 99th percentile occurred in the summer and fall (autumn) of 2020. The top 5% of largest fires made up 59.6% of burned area in the western US from 2016 to 2022, whereas the top 1% made up 27.0%. Restricting our analyses to pyromes where all fire size classes occurred resulted in 25 pyromes spanning all five western US ecoregions. Proportions did not differ substantially between the <95th percentile events and the 95th–99th percentile, but the most extreme 99th percentile events had considerably lower proportions of burned area occurring in the High-BP class and greater proportions in the Low-Med BP classes (Fig. 7a). Although approximately three-quarters of the burned area in 0–95th percentile events was in the two High-BP classes, this was less than half of the burned area for 99th percentile events.
(a) Mean proportions of observed burned area from 2016 to 2022 among burn probability (BP) classes based on FSim BP values for typical wildfires (0–95th percentile) compared with extremely large fires (95th–99th and >99th percentiles) among pyromes in the western US. (b) Examples of pyromes where smaller fires burned more High-BP areas while extreme large fires burned more Low-BP areas: (1) Eastern Cascades Slopes and Foothills, (2) Southern Sierra Nevadas, and (3) Southern Rockies (Upper Colorado River Basin).
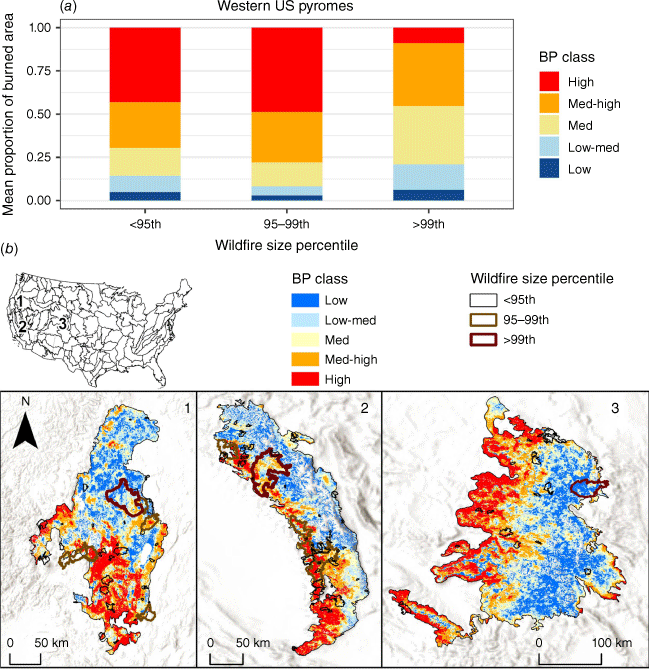
Only a few pyromes had notable differences in the proportions of Low-BP area burned by fires of different size classes (Supplementary Fig. S5). These were primarily located in mountain foothill regions of the Cascade Range, Sierra Nevada and Rocky Mountains (e.g. the Middle Cascades, Eastern Cascades Slopes and Foothills, Idaho Batholith, Northern Sierran Foothills and Tuscan Flows, Southern Sierran and Tehachapi Foothills, Southern Sierra Nevada and Southern Rockies (Upper Colorado River Basin) pyromes). All of these except for the Idaho Batholith were affected by major wildfires in the 2020 and 2021 seasons, including the North Complex, Bootleg, Creek and East Troublesome fires. Three of these example areas are illustrated in Fig. 7b, indicating where smaller <95th percentile fires primarily burned in the higher-BP classes whereas the extreme events burned either primarily in low-BP areas (as in the Southern Rockies pyrome), or a mix of low- and high-BP areas. In most cases, these low-BP areas corresponded to higher-elevation forests. However, some pyromes had high proportions of burned area in low-BP classes for the smaller fire size classes as well (e.g. the Marine Northwest Coast Forest, North Central California Foothills and Coastal Mountains, Klamath Mountains, Southern Cascades and Southern Rockies Front Range; Supplementary Fig. S5).
Differences in BP map versions
Performing evaluations based on only wildfire perimeters from 2021 to 2022 reduced the number of pyromes considered from 114 to 101. When we split pyromes into western and eastern US ecoregions, this resulted in 48 pyromes with burned area in the west and 53 pyromes in the east. For both the western and eastern US, the more recent LF2020 version resulted in greater proportions of burned area falling in the High-BP class (29.1% vs 47.6% in the West; 36.1% vs 44.0% in the East; Fig. 8). Furthermore, substantially lower burned area occurred in the Low-BP class in the western US based on LF2020 classes (7.9% vs 1.8%). Pyromes in Mediterranean California, the Northwestern Forested Mountains, and the Southern Semi-Arid Highlands and Temperate Sierras particularly had much lower proportions of burned area in the lower-BP classes compared with results for the LF2014 version (Supplementary Fig. S6). However, results did not similarly change in the Eastern Temperate Forests ecoregion (Supplementary Fig. S7).
Bar plots comparing mean proportions of observed burned area (2021–2022) by burn probability (BP) class across all pyromes in the conterminous US based on two different versions of the USDA Forest Service’s BP map: LF2014, version based on LANDFIRE 2014 fuels; LF2020, version based on LANDFIRE 2020 fuels. The LF2020 version also uses a more recent range of historical weather (2006–2020) and observed wildfires (1992–2020). Means are split by major geographic region according to Omernik Level 1 ecoregions (Western US, Marine West Coast Forest, Mediterranean California, Northwestern Forested Mountains, North American Deserts, Southern Semi-Arid Highlands and Temperate Sierras; Eastern US, Great Plains, Northern Forests, Eastern Temperate Forests and Tropical Wet Forests).
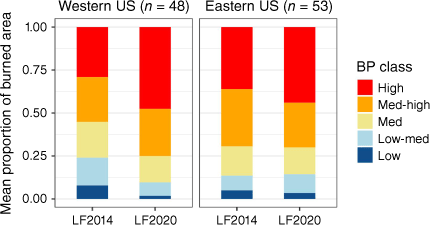
Discussion
Our assessment of an FSim-based BP map for the conterminous US revealed that, for most regions, BP could reliably estimate mean annual burned areas at coarse scales (i.e. pyromes and ecoregions). Furthermore, wildfires burned preferentially in higher-BP classes within most pyromes. However, a large number of pyromes (79 out of 114) had greater burned area in lower-BP classes than expected. Notably, several pyromes in the forested Mountain West burned extensively from 2016 to 2022 and had more than half the burned area occur in Low to Medium-BP classes. Although our results suggest that FSim-derived BP values are generally useful for determining where wildfire is most likely to occur, the fairly high proportions of burned area that occurred in low-BP areas indicate that there are many areas where the FSim-based BP map underestimated burn probability. Our evaluation methods could not determine the causes for these discrepancies, but it is reasonable to assume that areas that historically burned infrequently may not be well-represented in simulations based on recent wildfire activity. This underscores potential limitations of the BP map in estimating areas most at risk from wildfires in the coming years and decades. These limitations are critical to understand when implementing risk assessments with wildfire likelihood estimates based on simulation modelling.
Pyromes where high-BP areas best matched observed burned areas were concentrated in the desert Southwest, lower Mississippi River valley and Great Lakes regions. Generally, these are regions where wildfire activity is limited by the availability of flammable fuels, owing to limited vegetation growth in an arid climate (Mueller et al. 2020), fragmentation of forest fuels by agriculture and development (Poulos 2015), or small remnant patches of fire-prone conifer forests within largely mesic forest types (Nowacki and Abrams 2008). This result suggests that FSim may be best-suited to estimate burn probability in landscapes where there are large variations in fuel availability. However, there were larger differences between expected and observed proportions of burned area among high- and low-BP areas in pyromes of the Mountain West, Great Plains and Southeast. These may indicate that the BP map is most limited in landscapes where fuels are more consistently abundant, and where fire activity is driven more by variations in weather and ignition patterns. Finally, observed burned areas corresponded well with expected areas in Mediterranean California, but it is important to note here that the BP map predicted smaller differences in burned areas among BP classes for these pyromes compared with other ecoregions. This is because BP values in this region are high overall. Burned area occurred in nearly equal proportions among classes in many pyromes in southern California, indicating that here modelled BP was only slightly different than random predictions.
Many pyromes with high burned areas in low-BP classes were in the forested Mountain West, notably in the Cascade Range, northern Sierra Nevada, northern California coast, Sierra Nevada foothills and southern Rocky Mountains. In these pyromes, lower-BP areas often corresponded to higher-elevation forests. Furthermore, much of the burned area in these regions occurred during extreme large events in the summer and fall of 2020, when extreme high temperatures and low atmospheric moisture led to the largest wildfire season on record in the western US (Higuera and Abatzoglou 2021). Although the inclusion of extreme fire years such as 2020 (as well as 2017, 2018 and 2021, which had similarly high burned areas across the US) may be seen as biasing our evaluations, wildfire activity in these years is likely not an anomaly, but indicative of near-future conditions. Increases in wildfire size and mean area burned in the western US have been well-documented since ~2000, and although not every year will be as extreme as 2020, extreme conditions are likely to become more frequent with continued warming (Abatzoglou et al. 2021; Iglesias et al. 2022). Increased warming and drought may particularly increase wildfire likelihood in moist forests where fire has been historically climate-limited (Sibold and Veblen 2006; Halofsky et al. 2020; Alizadeh et al. 2021). Large fires are not unprecedented in many of these regions (e.g. in the western Cascade Range; Reilly et al. 2022), but if these events are not included in calibration records, then FSim will be constrained from reproducing these types of extreme fire seasons with frequency when using weather simulations drawn from historical ranges. Our evaluations therefore indicate that simulation models based on historical weather and calibrated to fires since the 1990s have limited ability to estimate future wildfire patterns under dynamic weather conditions.
Further discrepancies between observed burned areas and FSim BP estimates may result from a fuels layer that does not reflect recent changes. The BP map used in our evaluation was based on 2014 LANDFIRE fuels, and although this is recent regarding our observed period of 2016–2022, it is possible that localised vegetation changes in some areas affected wildfire patterns significantly enough to affect our results. For example, extensive forest mortality occurred in the western US over this period as a result of fires, insect outbreaks and drought (Anderegg et al. 2015). Furthermore, the continued spread of invasive grasses such as cheatgrass (Bromus tectorum) and buffelgrass (Cenchrus ciliaris) have increased wildfire probability throughout the Southwest and Great Basin (Davis et al. 2019; Fusco et al. 2019). Invasive grass spread may explain greater-than-expected burned areas in low-BP classes throughout the Great Basin. The 2020 Dome fire in Joshua Tree National Park, in the Eastern Mojave Basin and Range pyrome, and the 2019 Sheep fire near Idaho National Laboratory, in the Snake River Plain pyrome, both burned largely in lower-BP areas and were fuelled by recent increases in cheatgrass cover (Menser 2020; McDermott 2024). BP maps may therefore be sensitive to land cover changes such as forest mortality, invasive species spread and development in the wildland–urban interface, and frequent updates based on the most recent data can improve their usefulness.
In the eastern US, we observed the greatest burned area in low-BP areas in the southern Great Plains and southern Appalachian Mountains regions. Fire regimes in these regions are strongly driven by human ignitions, in contrast to the western US, where many of the largest fires are started by lightning strikes in remote areas (Prestemon et al. 2002; Balch et al. 2017). Here, a key limitation of the FSim BP map may by its simple weighted density surface estimating ignition probabilities based on historical observations of fires larger than 40.5 ha. This method may not necessarily capture fine-scale landscape features that explain ignition patterns (e.g. roads, powerlines, railroads, presence of the wildland–urban interface; Bar Massada et al. 2013; Radeloff et al. 2018; Chen and Jin 2022). Furthermore, probabilities of ignitions escaping and growing into large fires are influenced by factors such as road accessibility, topography, proximity to development and land management class (Rodrigues et al. 2020). It is also important to note that our evaluations using MTBS data only included observed fires larger than 202 ha, representing only a small number of fires that occur in the eastern US. The coarse scale of FSim modelling at a national scale may therefore present an additional limitation for understanding finer-scale burned area patterns.
We were only able to evaluate the more recent version of the US Forest Service’s BP map using 2 years of wildfire observations, and therefore our confidence in capturing the variability of wildfire occurrence is low. However, our comparisons of observed burned area proportions by BP classes between the two versions indicate improvements in model accuracy. Basing evaluations on the more recent map substantially reduced proportions of burned area that occurred in low-BP classes in the western US, and particularly in the Northwestern Forested Mountains ecoregion. Primary differences between the two versions of the map were (1) weather scenarios drawn from a more recent historical range (2006–2020 vs 1972–2012), and (2) a more recent fuels map (2020 vs 2014). Our analysis methods unfortunately do not allow us to attribute model improvements to either of these factors; that would require a true experimental design matrix. However, it is quite likely that weather ranges for the more recent period more accurately reflect the potential for large wildfire occurrence in places such as the Pacific Northwest and Rocky Mountains, in addition to reflecting more current fuels. This highlights the importance of continuously updating wildfire probability products with information to reflect constantly changing conditions that influence wildfire activity. However, this does not mean that BP maps will necessarily predict changes in burned area patterns that have not yet occurred – for instance, where wildfire activity may rapidly increase as climatic thresholds are crossed. For example, continued warming and increasing variability in atmospheric moisture could potentially trigger much larger fires in the northeastern US in the near future (Barbero et al. 2015; Poulos 2015).
Despite limitations, BP maps reflecting current wildfire activity can be valuable tools for identifying areas at risk of wildfire disasters and planning mitigation actions accordingly. However, robust evaluations are needed for managers to have confidence that these products can reliably estimate real-world wildfire patterns. An ideal method for evaluating models would consist of running a retrospective simulation based on older vintage fuels data, then comparing using a long time series of subsequent observed fires. An experimental approach based on varying ignition surfaces, fuels maps and weather scenarios could further help to identify opportunities for improving model estimates. However, this was not feasible in the current study owing to the high computational demands of running FSim at the scale of the entire conterminous US, and we were limited to evaluating the publicly available BP maps based on 2014 and 2020 LANDFIRE. Given that these products are widely used to assess risk in the US, the evaluations in our study are informative for managers and researchers desiring a better understanding of how these maps can be appropriately used to guide risk mitigation. Our results demonstrate that BP maps should be used with caution, as it should never be assumed that low-BP areas will never experience wildfire. Furthermore, our results suggest that potential limitations in the current approach of deriving BP maps may stem from reliance on historical weather ranges, out-of-date fuel maps, or inadequate representation of fine-scale ignition patterns. Simulations based on future climate projections, or detailed ignition models, as well as more frequent updating of BP maps, are potential avenues for producing maps with more realistic estimates, thus providing managers with more reliable tools to guide risk mitigation.
Conclusions
We compared a BP map for the conterminous US derived from an FSim model based on 2014 LANDFIRE fuels, 1972–2012 weather and 1992–2015 historic fires, with observed burned area from 2016 to 2022. Our results indicate that FSim BP outputs are useful for estimating the likelihood of wildfire exposure, as pyromes across the conterminous US had an average of ~71% of burned area occur in Med-High or High BP classes. However, there were key areas where BP underpredicted major wildfire burned areas, notably in the Cascade Range and southern Rocky Mountains. Although it should not be expected that a model will predict locations of future fires, major discrepancies between BP estimates and observed burned areas highlight the need for caution when using risk assessments based on FSim BP maps to guide risk mitigation. Given the regional patterns of where low-BP areas burned more than expected, it is quite likely that FSim BP estimates calibrated to historical conditions will underestimate BP in areas where fire regimes are rapidly shifting from historical baselines, or where the variability of wildfire occurrence exceeds what is captured in the calibration period. Continuously updating BP models based on recent weather, vegetationd and ignitions data can help to produce more realistic BP estimates. Furthermore, robust evaluations of new BP products are needed to improve their methodologies and test their underlying assumptions. Such evaluations and improvements are crucial to ensure that models are reliable tools for meeting the growing wildfire challenge.
Data availability
All data for this research were derived from publicly available datasets cited in the text. Code and output datasets are available as a USGS ScienceBase data release (https://doi.org/10.5066/P1JWC2TH).
Declaration of funding
This work was funded by the Department of the Interior Office of Wildland Fire, applying funding under Section 40803 of the Bipartisan Infrastructure Law/Infrastructure Investment and Jobs Act through Intra-Departmental Agreement #IDG1000002441.
Acknowledgements
This work was developed with feedback from numerous wildland fire scientists, particularly those involved in the US Geological Survey Wildland Fire Science Community of Practice and the SILVIS Lab at the University of Wisconsin–Madison. We thank Jason Kreitler and two anonymous reviewers for comments on an earlier draft of this manuscript. Any use of trade, firm, or product names is for descriptive purposes only and does not imply endorsement by the US Government.
References
Abatzoglou JT (2013) Development of gridded surface meteorological data for ecological applications and modelling. International Journal of Climatology 33(1), 121-131.
| Crossref | Google Scholar |
Abatzoglou JT, Battisti DS, Williams AP, et al. (2021) Projected increases in western US forest fire despite growing fuel constraints. Communications Earth & Environment 2(1), 227.
| Crossref | Google Scholar |
Adams MA (2013) Mega-fires, tipping points and ecosystem services: Managing forests and woodlands in an uncertain future. The Mega-Fire Reality 294, 250-261.
| Crossref | Google Scholar |
Ager AA, Day MA, Alcasena FJ, et al. (2021) Predicting Paradise: Modeling future wildfire disasters in the western US. Science of The Total Environment 784, 147057.
| Crossref | Google Scholar | PubMed |
Aitchison J (1992) On criteria for measures of compositional difference. Mathematical Geology 24(4), 365-379.
| Crossref | Google Scholar |
Alcasena FJ, Salis M, Ager AA, et al. (2015) Assessing landscape scale wildfire exposure for highly valued resources in a Mediterranean area. Environmental Management 55(5), 1200-1216.
| Crossref | Google Scholar | PubMed |
Alcasena F, Ager A, Le Page Y, et al. (2021) Assessing wildfire exposure to communities and protected areas in Portugal. Fire 4(4), 82.
| Crossref | Google Scholar |
Alexander ME, Cruz MG (2013) Are the applications of wildland fire behaviour models getting ahead of their evaluation again? Environmental Modelling & Software 41, 65-71.
| Crossref | Google Scholar |
Alizadeh MR, Abatzoglou JT, Luce CH, et al. (2021) Warming enabled upslope advance in western US forest fires. Proceedings of the National Academy of Sciences 118(22), e2009717118.
| Crossref | Google Scholar | PubMed |
Anderegg WRL, Hicke JA, Fisher RA, et al. (2015) Tree mortality from drought, insects, and their interactions in a changing climate. New Phytologist 208(3), 674-683.
| Crossref | Google Scholar | PubMed |
Balch JK, Bradley BA, Abatzoglou JT, et al. (2017) Human-started wildfires expand the fire niche across the United States. Proceedings of the National Academy of Sciences 114(11), 2946-2951.
| Crossref | Google Scholar | PubMed |
Barbero R, Abatzoglou JT, Kolden CA, et al. (2015) Multi-scalar influence of weather and climate on very large fires in the eastern United States. International Journal of Climatology 35(8), 2180-2186.
| Crossref | Google Scholar |
Bar Massada A, Syphard AD, Hawbaker TJ, et al. (2011) Effects of ignition location models on the burn patterns of simulated wildfires. Environmental Modelling & Software 26(5), 583-592.
| Crossref | Google Scholar |
Bar Massada A, Syphard AD, Stewart SI, et al. (2013) Wildfire ignition-distribution modelling: a comparative study in the Huron–Manistee National Forest, Michigan, USA. International Journal of Wildland Fire 22(2), 174-183.
| Crossref | Google Scholar |
Bayham J, Yoder JK, Champ PA, et al. (2022) The economics of wildfire in the United States. Annual Review of Resource Economics 14(1), 379-401.
| Crossref | Google Scholar |
Beverly JL, McLoughlin N (2019) Burn probability simulation and subsequent wildland fire activity in Alberta, Canada – Implications for risk assessment and strategic planning. Forest Ecology and Management 451, 117490.
| Crossref | Google Scholar |
Boer MM, Resco de Dios V, Bradstock RA (2020) Unprecedented burn area of Australian mega forest fires. Nature Climate Change 10(3), 171-172.
| Crossref | Google Scholar |
Bühlmann P (1997) Sieve bootstrap for time series. Bernoulli 3(2), 123-148.
| Crossref | Google Scholar |
Calkin DE, Cohen JD, Finney MA, et al. (2014) How risk management can prevent future wildfire disasters in the wildland–urban interface. Proceedings of the National Academy of Sciences 111(2), 746-751.
| Crossref | Google Scholar | PubMed |
Carmel Y, Paz S, Jahashan F, et al. (2009) Assessing fire risk using Monte Carlo simulations of fire spread. Forest Ecology and Management 257(1), 370-377.
| Crossref | Google Scholar |
Chen B, Jin Y (2022) Spatial patterns and drivers for wildfire ignitions in California. Environmental Research Letters 17(5), 055004.
| Crossref | Google Scholar |
Davis KT, Dobrowski SZ, Higuera PE, et al. (2019) Wildfires and climate change push low-elevation forests across a critical climate threshold for tree regeneration. Proceedings of the National Academy of Sciences 116(13), 6193-6198.
| Crossref | Google Scholar | PubMed |
Dillon GK, Scott JH, Jaffe MR, et al. (2023) ‘Spatial datasets of probabilistic wildfire risk components for the United States (270m)’, 3rd edn’. (USDA Forest Service Research Data Archive: Fort Collins, CO) 10.2737/RDS-2016-0034-3
Eidenshink J, Schwind B, Brewer K, et al. (2007) A project for Monitoring Trends in Burn Severity. Fire Ecology 3(1), 3-21.
| Crossref | Google Scholar |
Finney MA (2002) Fire growth using minimum travel time methods. Canadian Journal of Forest Research 32, 1420-1424.
| Crossref | Google Scholar |
Finney MA, McHugh CW, Grenfell IC, et al. (2011) A simulation of probabilistic wildfire risk components for the continental United States. Stochastic Environmental Research and Risk Assessment 25(7), 973-1000.
| Crossref | Google Scholar |
Fusco EJ, Finn JT, Balch JK, et al. (2019) Invasive grasses increase fire occurrence and frequency across US ecoregions. Proceedings of the National Academy of Sciences 116(47), 23594-23599.
| Crossref | Google Scholar | PubMed |
Haas JR, Calkin DE, Thompson MP (2013) A national approach for integrating wildfire simulation modeling into wildland–urban interface risk assessments within the United States. Landscape and Urban Planning 119, 44-53.
| Crossref | Google Scholar |
Halofsky JE, Peterson DL, Harvey BJ (2020) Changing wildfire, changing forests: the effects of climate change on fire regimes and vegetation in the Pacific Northwest, USA. Fire Ecology 16(1), 4.
| Crossref | Google Scholar |
Hawbaker TJ, Challis BL, Carlson AR, et al. (2023) The Wildfire Hazard and Risk Assessment Inventory: US Geological Survey Data Release. 10.5066/P9EZVDUZ.
Higuera PE, Abatzoglou JT (2021) Record-setting climate enabled the extraordinary 2020 fire season in the western United States. Global Change Biology 27(1), 1-2.
| Crossref | Google Scholar | PubMed |
Iglesias V, Balch JK, Travis WR (2022) US fires became larger, more frequent, and more widespread in the 2000s. Science Advances 8(11), eabc0020.
| Crossref | Google Scholar | PubMed |
McDermott A (2024) Fire in the desert. Proceedings of the National Academy of Sciences 121(12), e2402794121.
| Crossref | Google Scholar |
McFarlane BL, McGee TK, Faulkner H (2011) Complexity of homeowner wildfire risk mitigation: an integration of hazard theories. International Journal of Wildland Fire 20(8), 921-931.
| Crossref | Google Scholar |
Mell WE, Manzello SL, Maranghides A, et al. (2010) The wildland–urban interface fire problem – current approaches and research needs. International Journal of Wildland Fire 19(2), 238-251.
| Crossref | Google Scholar |
Menser P (2020) ‘One year after historic Sheep Fire, Idaho Site contractors, agencies eye coming fire season.’ (Idaho National Laboratory) Available at https://inl.gov/community-outreach/after-sheep-fire/
Mueller SE, Thode AE, Margolis EQ, et al. (2020) Climate relationships with increasing wildfire in the southwestern US from 1984 to 2015. Forest Ecology and Management 460, 117861.
| Crossref | Google Scholar |
Murray AT, Baik J, Figueroa VE, et al. (2023) Developing effective wildfire risk mitigation plans for the wildland–urban interface. International Journal of Applied Earth Observation and Geoinformation 124, 103531.
| Crossref | Google Scholar |
Nowacki GJ, Abrams MD (2008) The demise of fire and ‘mesophication’ of forests in the eastern United States. BioScience 58(2), 123-138.
| Crossref | Google Scholar |
Oliveira S, Rocha J, Sá A (2021) Wildfire risk modeling. Current Opinion in Environmental Science & Health 23, 100274.
| Crossref | Google Scholar |
Omernik JM (1987) Ecoregions of the conterminous United States. Annals of the Association of American Geographers 77(1), 118-125.
| Crossref | Google Scholar |
Parisien MA, Ager AA, Barros AM, et al. (2020) Commentary on the article ‘Burn probability simulation and subsequent wildland fire activity in Alberta, Canada – Implications for risk assessment and strategic planning’ by J.L. Beverly and N. McLoughlin. Forest Ecology and Management 460, 117698.
| Crossref | Google Scholar |
Paz S, Carmel Y, Jahshan F, et al. (2011) Post-fire analysis of pre-fire mapping of fire-risk: a recent case study from Mt Carmel (Israel). Forest Ecology and Management 262(7), 1184-1188.
| Crossref | Google Scholar |
Poulos H (2015) Fire in the Northeast: learning from the past, planning for the future. Journal of Sustainable Forestry 34(1–2), 6-29.
| Crossref | Google Scholar |
Prestemon JP, Pye JM, Butry DT, et al. (2002) Understanding broadscale wildfire risks in a human-dominated landscape. Forest Science 48(4), 685-693.
| Crossref | Google Scholar |
Radeloff VC, Helmers DP, Kramer HA, et al. (2018) Rapid growth of the US wildland–urban interface raises wildfire risk. Proceedings of the National Academy of Sciences 115(13), 3314-3319.
| Crossref | Google Scholar | PubMed |
Raymond CA, McGuire LA, Youberg AM, et al. (2020) Thresholds for post-wildfire debris flows: insights from the Pinal Fire, Arizona, USA. Earth Surface Processes and Landforms 45(6), 1349-1360.
| Crossref | Google Scholar |
Reilly MJ, Zuspan A, Halofsky JS, et al. (2022) Cascadia Burning: the historic, but not historically unprecedented, 2020 wildfires in the Pacific Northwest, USA. Ecosphere 13(6), e4070.
| Crossref | Google Scholar |
Rodrigues M, Alcasena F, Gelabert P, et al. (2020) Geospatial modeling of containment probability for escaped wildfires in a Mediterranean region. Risk Analysis 40(9), 1762-1779.
| Crossref | Google Scholar | PubMed |
Rodrigues M, Cunill Camprubí À, Balaguer-Romano R, et al. (2023) Drivers and implications of the extreme 2022 wildfire season in southwest Europe. Science of The Total Environment 859, 160320.
| Crossref | Google Scholar | PubMed |
Rollins MG (2009) LANDFIRE: a nationally consistent vegetation, wildland fire, and fuel assessment. International Journal of Wildland Fire 18(3), 235-249.
| Crossref | Google Scholar |
Rosen Z, Henery G, Slater KD, et al. (2022) A culture of fire: identifying community risk perceptions surrounding prescribed burning in the Flint Hills, Kansas. Journal of Applied Communications 106(4), Art 6.
| Crossref | Google Scholar |
Schoennagel T, Balch JK, Brenkert-Smith H, et al. (2017) Adapt to more wildfire in western North American forests as climate changes. Proceedings of the National Academy of Sciences 114(18), 4582-4590.
| Crossref | Google Scholar | PubMed |
Schug F, Bar-Massada A, Carlson AR, et al. (2023) The global wildland–urban interface. Nature 621(7977), 94-99.
| Crossref | Google Scholar | PubMed |
Scott JH, Thompson MP, Calkin DE (2013) A wildfire risk assessment framework for land and resource management. General Technical Report GTR-315. p. 83. (USDA Forest Service, Rocky Mountain Research Station: Fort Collins, CO) Available at https://www.fs.usda.gov/rm/pubs/rmrs_gtr315.pdf
Senande-Rivera M, Insua-Costa D, Miguez-Macho G (2022) Spatial and temporal expansion of global wildland fire activity in response to climate change. Nature Communications 13(1), 1208.
| Crossref | Google Scholar | PubMed |
Short KC (2017) ‘Spatial wildfire occurrence data for the United States, 1992-2015’, 4th edn. [FPA_FOD_20170508]. (USDA Forest Service Research Data Archive: Fort Collins, CO) 10.2737/RDS-2013-0009.4
Short KC, Grenfell IC, Riley KL, et al. (2020a) Pyromes of the conterminous United States. (USDA Forest Service Research Data Archive: Fort Collins, CO) 10.2737/RDS-2020-0020
Short KC, Finney MA, Scott JH, et al. (2020b) Spatial dataset of probabilistic wildfire risk components for the conterminous United States (270 m), 2nd edn. (USDA Forest Service Research Data Archive: Fort Collins, CO) 10.2737/RDS-2016-0034
Sibold JS, Veblen TT (2006) Relationships of subalpine forest fires in the Colorado Front Range with interannual and multidecadal-scale climatic variation. Journal of Biogeography 33(5), 833-842.
| Crossref | Google Scholar |
Thompson MP, Bowden P, Brough A, et al. (2016) Application of wildfire risk assessment results to wildfire response planning in the southern Sierra Nevada, California, USA. Forests 7(3), 64.
| Crossref | Google Scholar |
US Department of Agriculture Forest Service, Pyrologix and Headwaters Economics (2020) Wildfire risk to communities. Available at wildfirerisk.org
Vardoulakis S, Marks G, Abramson MJ (2020) Lessons Learned from the Australian bushfires: climate change, air pollution, and public health. JAMA Internal Medicine 180(5), 635-636.
| Crossref | Google Scholar | PubMed |