Improved logistic models of crown fire probability in Canadian conifer forests
Daniel D. B. Perrakis

A Natural Resources Canada - Canadian Forest Service, 506 West Burnside Road, Victoria, BC V8P 1Z5, Canada.
B Department of Geography, University of Victoria, Victoria, BC V8P 5C2, Canada.
C CSIRO, GPO Box 1700, Canberra, ACT 2601, Australia.
D Wild Rose Fire Behaviour, 180–50434 Range Road 232, Leduc County, AB T4X 0L1, Canada.
E Natural Resources Canada - Canadian Forest Service, 1219 Queen Street East, Sault Ste. Marie, ON P6A 2E5, Canada.
F B.J. Stocks Wildfire Investigations Ltd., 128 Chambers Avenue, Sault Ste. Marie, ON P6A 4V4, Canada.
International Journal of Wildland Fire 32(10) 1455-1473 https://doi.org/10.1071/WF23074
Submitted: 12 May 2023 Accepted: 8 August 2023 Published: 25 August 2023
Abstract
Crown fires are an ecologically necessary but hazardous process in conifer forests. Prediction of their behaviour in Canada has largely depended on the Canadian Forest Fire Behaviour Prediction System, in which fire weather indices drive primarily fixed fuel type models. The Crown Fire Initiation and Spread (CFIS) system presents a more flexible approach to predicting crown fire occurrence than fixed fuel type models.
Using a multi-decadal database of experimental fires carried out in conifer plots (1960–2019, n = 113), our aim was to develop updated models based on the CFIS system approach, fitting crown fire occurrence models to fire environment variables using logistic regression.
We tested alternative fuel moisture estimates and compared various model forms using repeated cross-validation. In two-storeyed stands, crown fire occurrence was defined as the involvement of lower canopy stratum fuels.
Final models based on wind speed, fuel strata gap, litter moisture and surface fuel consumption predicted crowning events correctly in up to 92% of cases in training data (89% in cross-validation).
These new models offer improved accuracy and flexibility that will help users assess how competing environmental factors interact under different fuel treatments and wildfire scenarios.
Keywords: Canadian Forest Fire Behaviour Prediction (FBP) System, Canadian Forest Fire Weather Index (FWI) System, Crown Fire Initiation and Spread (CFIS) system, crown fire occurrence, experimental burning, modelling, wildfire management, wildland fire behaviour.
Introduction
Crown fires have long been recognised as important ecological phenomena in conifer forests of boreal and temperate regions, including Canada (Bell 1889; Van Wagner 1983; Coogan et al. 2021). As a spreading flame front moves from surface fuels into the forest canopy and accesses crown fuels, it is exposed to higher winds resulting in a 2- to 10-fold increase in rate of spread (ROS) and fireline intensity (Alexander and Cruz 2016). Because most conifer forests will support crown fire under extreme fire weather conditions (Beverly et al. 2020; Cruz et al. 2022), it is critical to understand the necessary conditions for predicting the occurrence of crown fires. Such information has many practical fire and fuel management applications, including wildfire preparedness, response tactics and firefighter safety, as well as planning fuel treatments to mitigate crown fire behaviour potential (Perrakis et al. 2020).
Canadian empirical and semi-empirical crown fire models
Since the late twentieth century, Canadian fire management personnel and fire researchers have primarily used the Canadian Forest Fire Behaviour Prediction (FBP) System (Forestry Canada Fire Danger Group 1992) to forecast quantitative measures of wildfire behaviour. The FBP and Canadian Forest Fire Weather Index (FWI; Van Wagner 1987) Systems are both part of the larger Canadian Forest Fire Danger Rating System (CFFDRS; Stocks et al. 1989; Taylor and Alexander 2006). The present-day CFFDRS is being renewed, with many proposed changes and improvements anticipated (CFS Fire Danger Group 2021). The current FBP System depends on discrete fuel types representing various fire-prone Canadian vegetation complexes. Each of the seven conifer fuel types has empirical functions for predicting ROS and surface fuel consumption (SFC) based on components of the FWI System, along with mostly fixed values for live crown base height (LCBH) and other fuel attributes (Forestry Canada Fire Danger Group 1992). At the time of its release, the fixed-fuel type concept was well-suited to the needs of operational users, who generally lacked detailed forest structure data (Van Wagner 1990).
The Van Wagner (1977) crown initiation model provides a physical and conceptual framework for crown fire in the FBP System and is used to predict the overall type of fire (i.e. surface or crown) and trigger further calculations of fuel consumption and fireline intensity (Forestry Canada Fire Danger Group 1992). According to Van Wagner’s (1977) model, crown fire initiation is deemed to occur when a critical surface fire intensity threshold is exceeded based on the LCBH and foliar moisture content (FMC). In this scheme, LCBH is the critical forest structure variable influencing crown fire occurrence, a readily defined measure in single-storeyed stands. Although researchers have long used Van Wagner’s (1977) equation to study how fuel structure can affect crown fire occurrence (Alexander 1988; Agee et al. 2000), it did not address questions related to more complex canopy structure or the influence of ladder fuels (Keane 2015). It also demanded a separate surface fire model, which was available for the FBP System fuel types but otherwise required a separate means of estimation.
Seeking empirical model solutions
Earlier statistical analyses of the influence of LCBH, weather, fuel moisture and selected FWI System components on crown fire occurrence in the Canadian experimental fire database (Cruz et al. 2003, 2004) are incorporated into the Crown Fire Initiation and Spread (CFIS) system and software package (Alexander et al. 2006). Here, we introduce additional data and several novel analytical procedures and modelling methods that have emerged in the intervening years.
The present analysis involves: (1) a larger dataset than previously considered; (2) a stand-adjusted model of surface litter moisture content (MCSA); (3) a new approach to modelling the probability of crown fire occurrence in complex conifer stands; and (4) a more robust statistical testing framework for comparing different model forms than previously used. We also sought to clarify data flow from forest surveys through to crown fuel characteristics, an often-skipped over step (Affleck et al. 2012). The overall goal of the present study was to harness the findings from six decades of experimental burning research in Canada (Fig. 1) in order to improve the operational capability of crown fire occurrence models.
Photographs of experimental fires in Canadian conifer forests, 1960s–2019. (a) Vigorous surface fire near the crown fire threshold in a red pine plantation at the Petawawa National Forestry Institute, Ontario. (b and c) Line source ignition in overstocked immature jack pine at Sharpsand Creek, Ontario, showing high stem density and rapid crowning. (d) Surface fire in jack pine at Darwin Lake, Alberta. (e) Active crown fire in boreal lowland black spruce at Big Fish Lake, Alberta. (f) Mid-storey black spruce under mature jack pine overstorey at Kenshoe Lake, Ontario. (g) Active crown fire in jack pine–black spruce at the International Crown Fire Modelling Experiment site, Northwest Territories. (h) Active crown fire in thinned and unthinned black spruce, Pelican Mountain, Alberta. (i) Line source ignition and surface fire spread in mature lodgepole pine at Summit Lake (near Prince George), British Columbia. (j) Transition to torching and crowning following ignition in semi-mature jack pine, Sharpsand Creek. All photos from Canadian Forest Service fire research archives. For additional photographs, see Alexander and de Groot (1988), Alexander and Lanoville (1989), Stocks and Hartley (1995), and Hirsch et al. (2000).
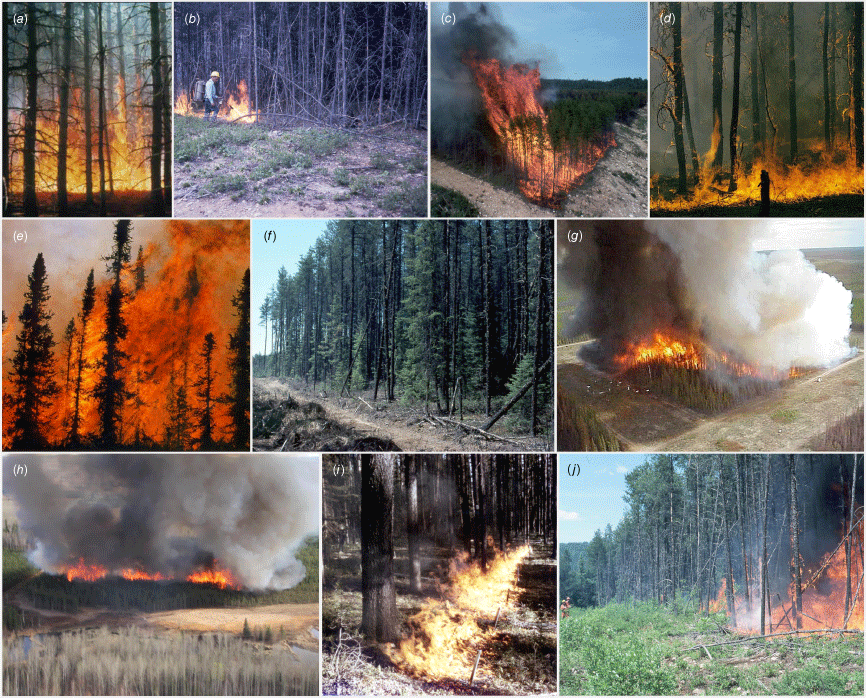
For convenience, a summary list of abbreviations used throughout the paper is provided in Appendix 1 (Table A1).
Material and methods
Experimental fire database: 60 years of experimental burning in Canada
The experimental fire data (Alexander and Quintilio 1990) behind the FBP System (Fig. 1) have been summarised in part in previous publications (e.g. Cruz 1999; de Groot et al. 2022). Our present database (Table 1) includes the majority of conifer experimental fire data between 1960 and 1986, along with 21 more recent observations (the latest being 2019), for a total of 42 more fires than were used in the development of previous crown fire occurrence models (Cruz et al. 2004). The database remains heavily weighted to natural stands of boreal and sub-boreal forest types, particularly jack pine (Pinus banksiana Lamb.) and black spruce (Picea mariana (Mill.) B. S. P.), and to a lesser extent white pine (Pinus strobus L.)–red pine (Pinus resinosa Ait.), lodgepole pine (Pinus contorta var. latifolia Engelm. Dougl. ex Loud.) and red pine plantations. Not included in this study’s database were experimental fires and wildfires in dead insect-killed stands (Stocks 1987b; Perrakis et al. 2014), nor sparsely documented fires in dry forest types of southern interior British Columbia (i.e. FBP System fuel type C-7; Forestry Canada Fire Danger Group 1992). It is also noteworthy that we did not include wildfire observations from the FBP System database, other than two well-documented exceptions, as described below.
Site & overstorey species | Years | Number: SF/CF | WS10 km h−1 | FFMC | DMC | FMCA% | SFC kg m−2 | |
---|---|---|---|---|---|---|---|---|
1. PNFI (WRP) | 1960–1964 | 8/0 | 10.9 (5.0–23.0) | 90.8 (87.8–92.8) | 58.8 (42–76) | 113.7 (100.5–120.0) | 2.1 (0.9–3.1) | |
2. PNFI (JP) | 1962–1965 | 5/2 | 12.6 (5.0–23.0) | 90.6 (88.5–92.8) | 55.6 (28–77) | 107.3 (100.0–118.0) | 1.5 (0.4–2.7) | |
3. PNFI (RP) | 1962–1971 | 8/4 | 13.3 (5.0–23.0) | 90.9 (88.5–92.9) | 47.6 (21–89) | 98.1 (91.0–108.0) | 1.3 (0.6–2.1) | |
4. Prince George (LP): PG | 1970 | 8/0 | 8.2 (6.4–12.9) | 91.6 (90.5–93.0) | 61.0 (33–77) | 102.8 (90.5–116.6) | 0.6 (0.2–1.2) | |
5. Big Fish Lake and others (BS): BFL | 1972–1986 | 3/9 | 11.6 (5.0–18.7) | 87.7 (80.0–91.2) | 21.0 (9–44) | 102.3 (79.0–120.0) | 1.5 (0.8–2.3) | |
6. Kenshoe Lake (JP/BS): KL | 1973–1983 | 6/6 | 12.3 (3.0–29.0) | 89.6 (87.2–91.4) | 31.8 (19–42) | 89.4 (85.0–106.1) | 0.9 (0.4–1.5) | |
7. Darwin Lake (JP): DL | 1974 | 5/2 | 10.3 (6.3–16.9) | 91 (88.7–93.7) | 30.3 (15–43) | 116.3 (109.7–120.0) | 2.0 (1.0–3.2) | |
8. Sharpsand Thinned (JP): SC-TH | 1974–1981 | 2/3 | 12.4 (8.0–15.0) | 92 (89.0–94.1) | 39.2 (29–45) | 107.9 (99.8–115.2) | 1.1 (0.8–1.5) | |
9. Sharpsand Immature (JP): SC-IM | 1975–1981 | 3/11 | 12.4 (6.0–21.0) | 90.5 (87.9–93.3) | 43.0 (25–57) | 108.0 (85.7–117.8) | 1.4 (0.7–2.4) | |
10. Porter Lake (BS): PL | 1982 | 0/7 | 23.5 (14.5–34.6) | 91.5 (89.4–92.8) | 58.7 (51–66) | 86.0 (85.1–86.5) | 1.3 (1.1–1.6) | |
11. Sharpsand Semi-mature (JP): SC-SM | 1988–1991 | 4/4 | 12 (9.0–19.0) | 90.8 (88.6–93.4) | 47.9 (32–70) | 86.8 (85.1–92.6) | 2.5 (1.4–3.6)B | |
12. ICFME (JP/BS) | 1997–2000 | 0/11 | 14.2 (7.9–25.0) | 91.8 (89.4–94.2) | 53.8 (35–84) | 82.2 (74.2–92.1) | 2.6 (1.5–3.8) | |
13. Pelican Mountain (BS): PM | 2019 | 0/2 | 12.0 | 93.6 | 46 | 79.0 | 1.6 (1.4–1.7) | |
Overall | 1960–2019 | 52/61 | 12.7 (3.0–34.6) | 90.6 (80.0–94.2) | 44.6 (9–89) | 99.0 (74.2–120.0) | 1.57 (0.2–3.8) |
Site | MCFFMC% | MCSA% | MCSA: stand | MCSA: season | MCSA: density | LCBHA M | FSG adjust. | Primary sources | |
---|---|---|---|---|---|---|---|---|---|
1. | 10.0 (7.9–13.2) | 9.5 (6.9–11.7) | Pine | 0/0/8 | 2/6/0 | 11.7 (7.4–13.3) | – | Van Wagner (1963), unpublished files | |
2. | 10.2 (7.9–12.4) | 9.3 (7.2–11.9) | Pine | 1/2/4 | 1/6/0 | 8.4 (6.0–12.0) | – | Van Wagner (1977), Hummel (1979), Weber et al. (1987) | |
3. | 9.9 (7.8–12.4) | 10.8 (7.4–17.2) | Pine | 2/2/8 | 0/0/12 | 7.5 (6.0–9.0) | – | Van Wagner (1968), Van Wagner (1986), unpublished files | |
4. | 9.1 (7.7–10.3) | 8.2 (7.3–9.7) | Pine | 0/0/8 | 4/4/0 | 8.2 (6.7–9.8) | – | Lawson (1972) | |
5. | 13.4 (9.6–22.2) | 13.9 (10.9–19.8) | Spruce | 0/0/12 | 9/3/0 | 1.1 (0.4–1.9) | – | Kiil (1975), Newstead and Alexander (1983), Cruz (1999), unpublished files | |
6. | 11.2 (9.4–13.8) | 12 (10.9–14.1) | Pi/Sp | 7/1/4 | 0/0/12 | 2.0 | – | Stocks (1989), unpublished files | |
7. | 9.8 (7.0–12.2) | 10.3 (7.0–14.5) | Pine | 0/0/7 | 4/3/0 | 5.6 (4.8–6.3) | – | Quintilio et al. (1977) | |
8. | 8.7 (6.6–11.9) | 9.3 (7.3–12.2) | Pine | 0/0/5 | 0/5/0 | 4.4 (4.3–4.8) | – | Stocks (1987a), de Groot et al. (2022), unpublished files | |
9. | 10.3 (7.4–13.1) | 11.6 (7.9–15.0) | Pine | 0/1/13 | 0/3/11 | 4.4 (4.1–4.9) | LCBH-2.0 | Stocks (1987a), unpublished files | |
10. | 9.3 (7.9–11.5) | 8 (6.9–9.7) | Spruce | 0/0/7 | 7/0/0 | 0.9 (0.8–1.1) | – | Alexander et al. (1991) | |
11. | 10 (7.3–12.3) | 9.8 (7.3–11.7) | Pine | 2/5/1 | 0/1/7 | 5.3 | LCBH-1.0 | McRae et al. (2017) | |
12. | 9 (6.5–11.5) | 9.8 (6.3–13.5) | Pi/Sp | 0/0/11 | 0/3/8 | 2.6 (0.7–8.2) | LCBH-1.0C | Alexander et al. (2004), Stocks et al. (2004) | |
13. | 7.1 | 6.6 (6.3–6.9) | Spruce | 2/0/0 | 1/1/0 | 3.1 (2.1–4.2) | – | Thompson et al. (2020) | |
Overall | 10.2 (6.5–22.2) | 10.5 (6.3–19.8) | – | 14/11/88 | 28/35/50 | 4.9 (0.4–13.3) | – | – |
ASee Supplementary Material for sources of FMC and LCBH estimates.
BEstimated using reported total fuel consumption values, with crown fuel consumption values from SC-IM and SC-TH plots; see Supplementary Material for details.
CFSG Adjustment at ICFME only applied to plots with LCBH >2.0 m. See text for details.
Most of the experimental fires were carried out in varying sizes of square (Fig. 1g) or rectangular (Fig. 1h) shaped plots (~0.05–5.0 ha) on flat terrain; the fires were generally lit from an ignition line (Fig. 1b, i) along the windward edge of cleared fireguards using hand-held drip torches or pressurised fuel dispensers (Fig. 1b, j). Alternate ignition techniques were used on a small number of plots. These included: ignition as a backing fire, which then became a headfire following a change in wind direction (Van Wagner 1964); paired experiments where one plot’s flame front ignited an adjacent area, as part of a fuel treatment effectiveness trial (Fig. 1h; Thompson et al. 2020) and an escaped experimental fire, monitored independently (Plot 5a in Alexander et al. 1991); point source ignition with allowance for acceleration to equilibrium conditions (Kiil 1975); and cases where single plots were split into two parts due to significant changes in weather conditions, principally wind speed (Plots 4a and 4b in Quintilio et al. 1977; Plots 11a and 11b in Stocks 1987a; and Plots 8a and 8b in Stocks et al. 2004). Finally, two wildfires have long been included among the experimental observations due to their connections with experimental stands: the Porter Lake CR-6-1982 wildfire resulting from the escaped experimental fire (Alexander et al. 1991) and two observations related to distinct fuel patches in the 1964 Gwatkin Lake wildfire (Van Wagner 1965, 1977), based on a detailed wildfire reconstruction within <1.0 km of a pre-existing experimental study area (Hummel 1979). In all cases, we treated these as independent observations following the advice of the original investigators.
Canopy fuel structure: live crown base height, fuel strata gap, and two-storeyed stands
Previous analyses used LCBH or the related fuel strata gap (FSG; Cruz et al. 2004) as the measure of vertical fuel separation, based on field measurements or alternate means of estimation (Table 1). Due to variable sampling methods and limited descriptions in some studies, we made a considerable effort to locate and reanalyse original stand data wherever possible. The LCBH was calculated as the mean value of a single species canopy stratum sampled directly from plots or estimated using models or measurements of crown ratio. See Supplementary Material for details on the various means used to estimate LCBH. Most stands had a simple one-layer canopy (as per Van Wagner 1977), and we assumed that the FSG was simply the LCBH, with two exceptions: multi-layered stands, and stands with a large number of small dead stems that visibly contributed to crowning.
Most plots at the Kenshoe Lake (KL) and International Crown Fire Modelling Experiment (ICFME) sites had two canopy layers, a mid-storey black spruce layer underneath an upper canopy of jack pine (Fig. 1f; Stocks 1989; Alexander et al. 2004). Rather than using a combined LCBH value, we assumed these distinct strata involve separate conditional crowning processes: vertical flame propagation into the mid-canopy spruce component is first required before testing the much higher overstorey pine canopy (Van Wagner 1993). Aside from the species mix and two-storeyed structure, the stands at these two sites, KL and ICFME, differ in terms of age class and vertical structure. However, it is apparent that they are otherwise similar, with black spruce comprising about 11% of live basal area and 30–35% of live tree density at both sites (Walker and Stocks 1975; Alexander et al. 2004, Table 2).
Model | Predictors | Accuracy | MCC | AIC | FSG | MCC Rank | AIC Rank | |
---|---|---|---|---|---|---|---|---|
A | WS10 + FSG | 0.815 | 0.628 | 71.7 | Base | 14 | 14 | |
B | WS10 + FSG + MCFFMC | 0.841 | 0.680 | 56.0 | Base | 12 | 13 | |
1. | WS10 + FSG + SFC + MCFFMC | 0.863 | 0.724 | 50.8 | Base | 8 | 10 | |
2. | WS10 + FSG + SFC + MCSA | 0.839 | 0.676 | 54.7 | Base | 13 | 12 | |
3. | WS10 + FSG1.5 + SFC + MCFFMC | 0.868 | 0.733 | 49.7 | Base | 6 | 7 | |
4. | WS10 + FSG1.5 + SFC + MCSA | 0.849 | 0.696 | 52.4 | Base | 11 | 11 | |
5. | WS10 + FSG1.5 + SFC + MCFFMC x WS10 | 0.873 | 0.744 | 46.5 | Base | 5 | 5 | |
6. | WS10 + FSG1.5 + SFC + MCSA x WS10 | 0.856 | 0.710 | 50.3 | Base | 9 | 9 | |
7. | WS10 + FSG1.5 + ln(SFC) + MCFFMC x WS10 | 0.878 | 0.755 | 46.0 | Base | 3 | 4 | |
8. | WS10 + FSG1.5 + ln(SFC) + MCSA x WS10 | 0.865 | 0.727 | 50.3 | Base | 7 | 8 | |
9. | WS10 + FSG1.5 + SFC.CLS + MCFFMC x WS10 | 0.853 | 0.705 | 47.2 | FSG Adj. | 10 | 6 | |
10. | WS10 + FSG1.5 + ln(SFC) + MCFFMC x WS10 | 0.887 | 0.772 | 42.8 | FSG Adj. | 2 | 2 | |
11. | WS10 + FSG1.5 + ln(SFC) + MCSA x WS10 | 0.890 | 0.779 | 42.5 | FSG Adj. | 1 | 1 | |
12. | WS10 + FSG2 + ln(SFC) + MCSA x WS10 | 0.876 | 0.751 | 44.8 | FSG Adj. | 4 | 3 |
MCC and AIC represent the Matthews Correlation Coefficient and Akaike’s Information Criterion, respectively. ‘FSG’ refers to the form of the fuel strata gap: the Base live crown base height, or adjusted value reflecting standing dead ladder fuels (see Table 1). Model 9 includes a categorical term for SFC; all other inputs are continuous. See Appendix 1 for abbreviation descriptions.
A number of plots at Sharpsand Creek (SC) and at ICFME contained significant quantities of ladder fuels in the form of small (<5.0 cm diameter at breast height (DBH)) dead trees that were observed to be influential in the crowning process (Stocks 1987a; Stocks and Hartley 1995). The abundance of dead ladder fuels evident in the immature jack pine stands at SC (SC-IM: Fig. 1b, c; SC-SM: Fig. 1j) was a result of the stem mortality associated with natural stand development. A separate set of plots at the site had been hand-thinned 15 years prior to their burning (SC-TH) and had much lower overstorey tree density (Stocks 1987a; de Groot et al. 2022). Earlier studies have treated these experimental plots as separate fuel types (Forestry Canada Fire Danger Group 1992; de Groot et al. 2022),1 or estimated that the standing dead trees at SC-IM reduced the FMC (Van Wagner 1993).
For the present analysis, we expanded on the FSG concept introduced by Cruz et al. (2004, 2006) and estimated FSG for the SC-IM (~10 000 dead stems ha−1) and SC-SM (~3800 dead stems ha−1) plots as LCBH–2.0 and LCBH–1.0 m, respectively. For the SC-TH plots, no adjustment was made (i.e. FSG = LCBH), due to the lower dead tree density (<1200 ha−1). At the ICFME site, 31% of the total stem density consisted of small diameter dead jack pine (Alexander et al. 2004); however, there was substantial variation in the amount of black spruce understorey and LCBH. For those plots with LCBH values >2.0 m, we adjusted FSG (FSG = LCBH – 1.0). No adjustment was made (FSG = LCBH) for plots with low LCBH (LCBH < 2.0 m) because we judged that dead stems would not add significantly to the ladder fuel effect. Although these adjustments were subjective, we analysed our database using both (unadjusted) LCBH and (adjusted) FSG to reveal whether use of the FSG adjustments could be justified. No other site descriptions suggested the presence of standing dead trees in comparable amounts, but lower densities were often noted, such as 0–60 dead stems ha−1 at Darwin Lake (DL; Quintilio et al. 1977) and 109–250 ha−1 at Prince George (PG; Lawson 1972).
Fine fuel moisture content estimates
To incorporate the influence of fine dead fuel moisture content (MC), we tested two approaches. The first used the Fine Fuel Moisture Code (FFMC) component of the FWI System (MCFFMC). This code is calibrated to predict MC broadly across conifer forests for a ‘generalised pine stand’ (Van Wagner 1974a). The second approach used a stand-adjusted moisture content estimate (MCSA; Wotton and Beverly 2007) incorporating several stand structure and condition influences on MC in addition to the FFMC and Duff Moisture Code (DMC) components of the FWI System. Calculating the MCSA for the fires assembled in our database required assigning several necessary input variables: FFMC; DMC; and categorical variables for stand type, stand density class and season (Wotton and Beverly 2007).
Although the MCSA model includes five stand type options (Wotton and Beverly 2007), all of our observations were classed as either ‘pine’ or ‘spruce’ (or the mean of both), based on overstorey composition (Table 1). Density class and season variables required some interpretation and judgement. For example, the density class variable has three levels (i.e. ‘light’, ‘moderate’, ‘dense’) based on site descriptions, canopy closure and photographic evidence. We assumed that density affected MC primarily due to variable solar radiation at the forest floor level and the influence of in-stand wind (Whitehead et al. 2008; Ma et al. 2010; Moon et al. 2016). Moderately closed pine stands (~50–60% canopy closure) were assigned to the ‘moderate’ density class, in parallel with the dominant class found in the litter moisture data (Wotton and Beverly 2007). The ‘dense’ class was assigned to sites with estimated canopy closure of >60% or particularly high crown fuel loading values (Forestry Canada Fire Danger Group 1992; Cruz 1999; de Groot et al. 2022). ‘Light’ density class was assigned to sites with obvious low-density structure (Alexander et al. 1991), as well as to individual plots at other sites with <45% canopy closure (Table 1). The ‘dense’ class experimental fires with FFMC ≥ 93.0 were reclassed to ‘moderate’ due to some illogical behaviour of the MCSA model at high FFMC levels; see Supplemental Material for details.
Based on the guidance in Wotton and Beverly (2007), we used simple calendar dates to assign the season factor to each observation. Fires taking place before June were assigned to Spring (SP) and fires from 16 June through 1 September were regarded as summer (SU) fires. To avoid an artificially abrupt transition and to approximate the green up process (Chrosciewicz 1986), we added a ‘transition’ (TR) season for dates from 1 to 15 June, calculated as the mean of spring and summer MCSA estimates (Table 1).
Surface fuel consumption
Recognised as a key influence on surface fire intensity and therefore crown fire initiation (Van Wagner 1977; Agee et al. 2000), SFC was measured or estimated for all experimental fire plots. We used the sum of all consumed forest floor and fine woody fuels (i.e. roundwood diameters <7.0 cm) as the SFC value in our analysis; most studies did not report separate measures for litter and duff, and some did not separate fuels into forest floor and woody fuel portions. In most experimental fires (e.g. Stocks 1989; Alexander et al. 1991), SFC values were dominated by forest floor consumption, with lower contributions (i.e. 1/3 or less of the SFC) from woody fuels. Several fires had particularly high SFC levels (>3.0 kg m−2) caused by the consumption of dense, compact duff layers. To reduce the influence of these observations on regression equations, we tested log-transformations of SFC values during the model-building phase.
Model fitting and evaluation
All final records for each fire in the database included measurements or estimates of fuel structure (i.e. LCBH and FSG), FMC, SFC, 10-m open wind speed (WS10), FWI System components (FFMC, DMC) calculated from an onsite or nearby representative station, fine fuel moisture (MCFFMC and MCSA) and finally, a fire behaviour description including the type of fire – i.e. surface (Fig. 1a, d, i) or crown (Fig. 1c, e, g, h, j). Crown fire was considered to have occurred when crowning-type fire behaviour (i.e. passive or active, as per Van Wagner 1977; or intermittent, as per Forestry Canada Fire Danger Group 1992) was described or could be inferred from the original source publications or file photographs. At the two sites with two-layered canopy fuels (i.e. KL and ICFME), only crown involvement of the mid-storey spruce was tested in our main analysis. Following Cruz et al. (2003, 2004), crown fire occurrence was modelled as the response variable in a generalised linear model (logistic regression). Predicted probability values above and below 0.5 were interpreted as crown fire and surface fire, respectively.
The initial explanatory variables were suggested from previous modelling results (Cruz et al. 2004; Perrakis et al. 2020). A backwards stepwise regression approach was used starting with all interaction effects between predictor variables: WS10, SFC, FSG, FMC and MCFFMC or MCSA, with terms tested at the ⍺ = 0.05 level. For WS10, linear and squared terms were tested. For LCBH and FSG variables, linear, squared and 3/2 exponents were tested, the latter following Van Wagner’s (1977) crown fire initiation model. For the SFC, linear, log-transformed and categorical terms as per Cruz et al. (2004) were tested.
Various model forms were then compared using a Monte Carlo implementation of k-fold cross-validation, with four folds. This analysis, considered highly efficient for comparing alternative model forms with small datasets (Zhang 1993; Lantz 2019), involves splitting the data into four equal but randomly selected ‘folds’, fitting the models to three of them, and evaluating the ensuing models using the fourth (unused) portion of observations. Cross-validation was performed in R v.4.2.2, using the ‘vtreat’ data package (Mount and Zumel 2018), with 1000 repeated iterations of training and testing for each model form. Model ranking was based on comparing mean values of Akaike’s Information Criterion (AIC) and Matthews Correlation Coefficient (MCC) values from the iterations. The MCC is an evaluation method based on calculation of the Pearson correlation coefficient between values of a binary confusion matrix. It has been shown to be less biased than more common evaluation measures (Powers 2011) and particularly effective for unbalanced datasets (Chicco and Jurman 2020), as might occur in random selections of surface and crown fire observations. Initial model tests used the unmodified LCBH. We then retested the best performers using the adjusted FSG values for some sites as previously described.
Following cross-validation, the best models were fitted to the complete dataset and evaluated based on accuracy, AIC and Nagelkerke R2 (Mangiafico 2015). We also tested the dataset on previously published CFIS system models (i.e. LOGIT 1 and 2 models from Cruz et al. 2003) for comparative purposes.
Results
Our final experimental fire database contained 113 observations, consisting of 52 surface fires and 61 crown fires (Table 1). Individual relationships between predictors and crown fire occurrence were generally as expected. Crown fire was clearly associated with low LCBH (and FSG), MCFFMC and FMC values, and high WS10 and SFC values (Fig. 2). Estimated FMC had the only bivariate correlation >0.3 (positive with FSG). We then tested the FMC between low FSG (<4.0 m, 90.8%) and high FSG (≥4.0, 103.6%) and found them significantly different (Welch’s t-test: P < 0.001). This appeared to be caused by a disproportionate number of experimental fires in black spruce stands (with low LCBH) during ‘spring dip’ conditions (Van Wagner 1974b) in June and early July, compared with more evenly timed fires in higher-LCBH pine stands.
Pairwise plots and correlations for surface (S, blue, circles) and crown fire (C, red, triangles) observations. See Table A1 for abbreviation details. Lines in diagonal cells indicate frequency distributions of environmental variables, bivariate scatter diagrams show the actual variable values, and ‘Corr.’ indicates Spearman’s Correlation, at the specified significance level: 0.1 (.), 0.05 (*), 0.01 (**), 0.001 (***).
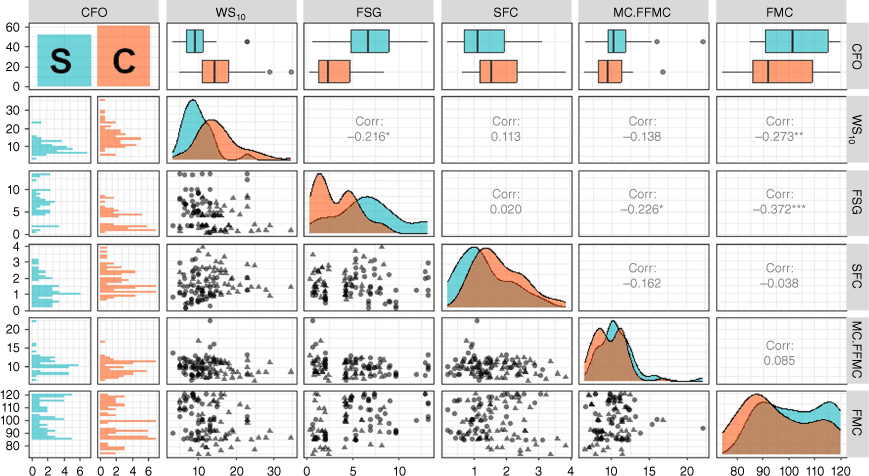
During model building, complex interaction terms were first dropped due to non-significance. Some models with multiple two-way interaction terms initially appeared significant, but further testing revealed illogical behaviour, thereby suggesting overfitting. The next-best model forms involved dropping the FMC term, it being non-significant (P > 0.1) in any tests at this level once insignificant interaction terms were removed. For the fine fuel moisture terms (MCSA or MCFFMC), either the main effect or the interaction term with WS10 (i.e. WS10 × MCSA or WS10 × MCFFMC) was significant when included, but not both; in these cases, the interaction terms all produced better results (i.e. greater accuracy, lower AIC) than the main effects alone. Two simple main effects model forms that excluded the SFC term also showed promise (termed A and B): one used only WS10 and LCBH, with MCFFMC added for the second.
We then proceeded to the cross-validation step comparing model forms. Results comparing predictor sets using various interactions and transformations are shown in Table 2. The two simplest models, A and B, were the weakest performers, but still achieved mean accuracy >80%. Other models used WS10, FSG (initially based on a linear LCBH value), MC (MCFFMC or MCSA) and estimated SFC, starting with simple main-effect predictors (Models 1 and 2) followed by the successive substitution of LCBH3/2, WS10 × MC interaction and log-transformed SFC for their respective linear and main effect terms. The adjusted FSG with ladder fuel influence was used in lieu of LCBH for the later tests, as shown in Table 2. More complex inputs improved model performance, in the form of higher accuracy and MCC, and lower AIC, although overall gains were small among top performers. The best-performing models approached 90% accuracy (Model 10: 88.7%; Model 11; 89.0%). Model 12 had a squared FSG term but was otherwise identical to Model 11, with slightly inferior performance. Models with a squared WS10 term were inferior to all comparable forms with a linear WS10 predictor (not shown). Tests of additional categorical terms for stand type (pine, spruce or a mix) or stand density were non-significant (P > 0.1). Based on these results, we moved ahead with the final analysis using model forms 7–11, these appearing to be the most accurate and practically useful forms to consider.
Table 3 shows the fitted coefficients and performance of the final models based on the full dataset. Model 9, with categorical SFC, predicted crowning occurrence correctly for 99 of 113 observations, as shown. As with the cross-validation results, Models 10 and 11 had the best performance. Model 11 (using MCSA) was slightly more accurate (104/113) than Model 10 (using MCFFMC; 103/113). Model 11 also had better AIC and Nagelkerke pseudo-R2 scores, indicative of lower residual deviance and better overall data representation, respectively. There was a relatively even split between classification errors for both models. Out of 113 possible classifications, Models 11 and 10 miscategorized four and five, and six and four surface and crown fires, respectively.The full list of fitted coefficients for all models in Table 2 is given in the Supplementary Table S1.
Predictor: | – | WS10 | FSG3/2 | WS10 X MC | ln(SFC) | Input Type | ||||||
---|---|---|---|---|---|---|---|---|---|---|---|---|
ModelA | β0 | β1 | β2 | β3 | β4 | Acc. | AIC | N.R2 | MC | FSG | ||
7 | −3.0923* | 1.2611*** | −0.39518*** | −0.06588*** | 2.8324** | 0.903 | 60.1 | 0.813 | MCFFMC | LCBH | ||
8 | −2.4341* | 1.0449*** | −0.37591*** | −0.04869*** | 2.1943** | 0.876 | 65.0 | 0.789 | MCSA | LCBH | ||
10 | −4.0204** | 1.3514*** | −0.43355*** | −0.06881** | 2.8906** | 0.912 | 56.3 | 0.830 | MCFFMC | Adjusted | ||
11 | −3.5550** | 1.4407*** | −0.53211*** | −0.07019*** | 2.4897* | 0.920 | 55.2 | 0.835 | MCSA | Adjusted |
SFC.CLS 2 | SFC.CLS 3 | |||||||||||
---|---|---|---|---|---|---|---|---|---|---|---|---|
β0 | β1 | β2 | β3 | β4 | β5 | |||||||
9 | −4.6830** | 1.3156*** | −0.41448*** | −0.06632** | 1.6792† | 3.2814* | 0.876 | 61.6 | 0.815 | MCFFMC | Adjusted |
Symbols β0–β5 represent intercept and coefficients for predictor terms as indicated (see Appendix I for abbreviations). FSG term refers to either live crown base height (LCBH) or adjusted fuel strata gap, as indicated. Residual degrees of freedom was 108 for all models except 107 for Model 9. Acc. is Accuracy (number correct out of total); AIC is Akaike’s Information Criterion; and N.R2 is Nagelkerke’s R2. Input type indicates the type of MC estimate and type of FSG used, either simple LCBH or adjusted (see Table 1).
AModels are in the form where p(CFO) is probability of crown fire occurrence (0–1), β0–βn are coefficients as shown in the table, n = 4 (Models 7–11) or n = 5 (Model 9), and x1–xn represent estimated values of predictors WS10, FSG3/2, WS10 × MC and ln(SFC), respectively (models 7–11). For Model 9, x4 and x5 are dummy variables used for representing SFC classes 2 (SFC.CLS 2) and 3 (SFC.CLS 3) as follows: SFC class 1: x4 = 0, x5 = 0; class 2: x4 = 1, x5 = 0; class 3: x4 = 0, x5 = 1.
Asterisks represent significance levels: <0.001 (***); <0.01 (**); <0.05 (*); <0.1 (†).
The CFIS LOGIT 1 and 2 models (Cruz et al. 2003), which were fitted from approximately half of the observations in our dataset and based on a slightly different definition of crown fire, both produced mean accuracy values of 81% based on the full dataset (92 of 113 correct observations). Further inspection of the results revealed a bias associated with classification errors, with the LOGIT 1 model producing 15 false negatives, or failure to detect crown fires, compared with six false positives (i.e. prediction of crown fires on actual surface fires). For the LOGIT 2 model, the results were 16 and 5 for missed crown and surface fires, respectively.
Model 11 was selected for further display. The probability distributions of crown fire at different WS10 and FSG values are shown in Fig. 3, based on constant SFC and MC values. Fire observations are shown as either surface or crown, with crown fires further indicated as passive or active (Van Wagner 1977). Predictions from Cruz et al.’s (2003) LOGIT 1 model are also shown for comparative purposes. A more extensive comparison between models 9–11 with Cruz et al.’s (2003) LOGIT 1 and 2 models across input levels is shown in the Supplementary Table S2. The distribution of observations is further illustrated in Fig. 4, in which all fire environment variables are displayed. Iso-lines representing the 50% probability level using Model 11 are shown for MC values of 6.0–15.0%, as indicated; these were calculated at SFC of 1.5 kg m−2, close to the median and mean SFC values from all observations (1.42 and 1.57 kg m−2, respectively).
Probability of crown fire occurrence using Model 11 (p(CFO), black lines) under varying open wind speed (WS10) and Fuel Strata Gap (FSG) values. Calculation constants are surface fuel consumption = 1 kg m−2 and stand- adjusted moisture content = 10.0%. Fire observations (vertically jittered for clarity) are shown by type of fire: surface (blue circles); passive crown (green squares); or active crown (red diamonds). Grey lines show predicted probability values from previously published CFIS system LOGIT 1 model (Cruz et al. 2003; calculated using constant Fine Fuel Moisture Code of 90.8 and Drought Code of 200).
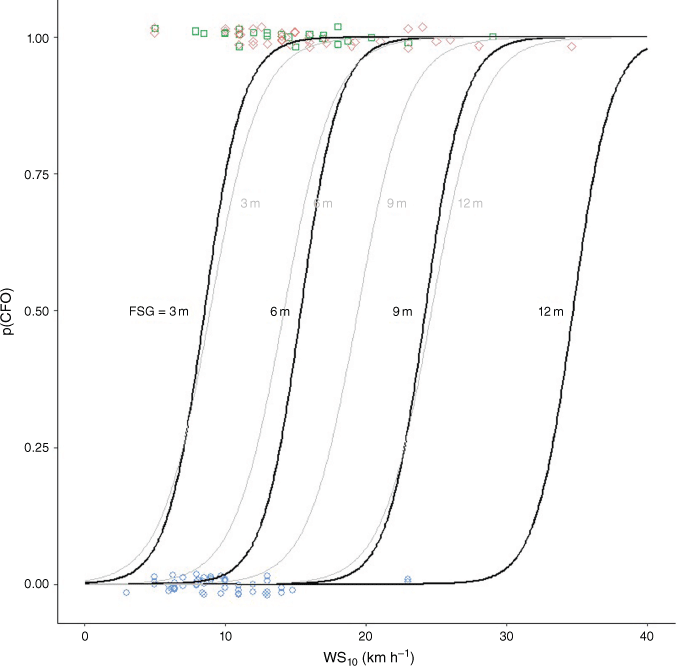
Scatter plot of 113 fire observations by 10 m open wind speed (WS10) and fuel strata gap (FSG, shown unadjusted). As indicated, symbol sizes represent values of surface fuel consumption (SFC, kg m−2), colours indicate estimated dead fine fuel moisture content (MC,%) and shapes depict crown fire occurrence (1, triangles) or absence (i.e. surface fire; 0, circles). Iso-lines represent P = 0.5 for crown fire initiation based on Model 11 at various indicated stand-adjusted MC values (6–15%), calculated at SFC = 1.5 kg m−2. Observation space below and right of isolines represents crown fire behaviour, and observation space above and to the left of the lines represents surface fire behaviour.
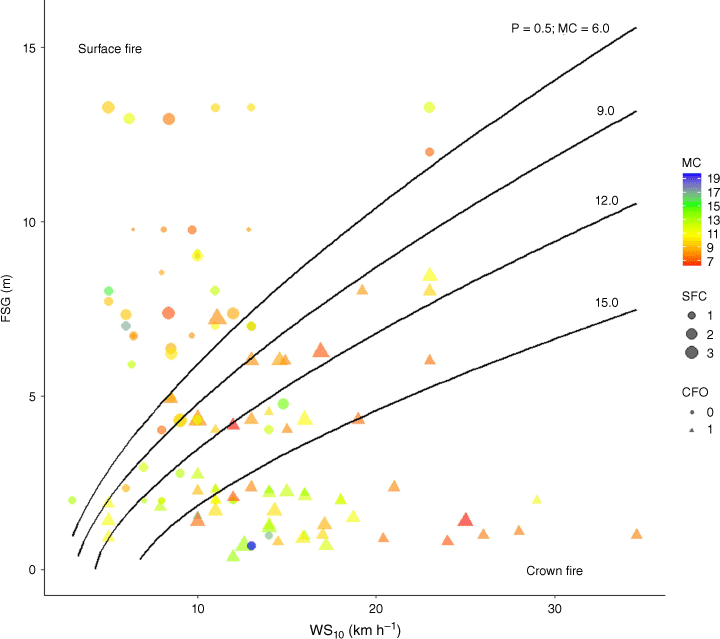
Discussion
Updated and improved probability of crown fire occurrence models
Empirical and quasi-empirical fire behaviour models have typically been simple, aimed at operational use but constrained by small sample sizes (Sullivan 2009). In attempting to improve the flexibility of crown fire occurrence models, we followed the successful approaches taken in the development of the FBP and CFIS system, using physical principles to guide modelling logic while respecting empirically fitted model terms and forms.
Based on our analysis, crown fires in live boreal and sub-boreal conifer forests can be correctly predicted about eight to nine times out of 10 using WS10, MC, FSG and estimated SFC, at least within our described data range (Fig. 4) and assuming the inputs can be reliably estimated. Our final models used two to four of these predictors with various transformations and are applicable to level or gently undulating terrain. The full-featured Model 11 showed the best combination of performance and flexibility, using log-transformed SFC and incorporating ladder fuel influence on the onset of crowning via the logical (albeit subjective) adjustments on FSG values. Model 11 retains the 3/2 power function associated with FSG as originally conceived by Van Wagner (1977), based on physical principles related to flame height (Lee and Emmons 1961; Thomas 1963). It was encouraging to see how the empirical results from our study confirm this relationship despite its simplicity.
When predictors have conflicting influences on fire behaviour, outcomes become more uncertain, such as in the case of high WS10–MC conditions. The interaction term (WS10 × MCSA or WS10 × MCFFMC) in Models 5–12 helps avoid overprediction of crown fire occurrence in such cases. For instance, under WS10 of 30–40 km h−1 and high fine fuel moisture content (MCSA = 20–25%, or FFMC 78–82), a main effects-only model such as Model 3 from this study or the LOGIT 1 model of Cruz et al. (2003) would predict a very high probability of crowning (Model 3: P = 0.9981; LOGIT 1: P = 0.9978 for an FFMC of 82, WS10 of 35 km h−1 and FWI System Drought Code (DC) component of 100) with mid-range values for other predictors (LCBH/FSG = 4.0, SFC = 1.0 kg m−2).2 Using these same predictors with Models 10 or 11 suggests a very low chance of crowning (P < 0.005 for both), a consequence of the interaction factor suppressing the crowning probability as MC values approach extinction levels. Although experimental fire data under such conditions are lacking, the closest comparable fires in our database suggest marginal conditions for ignition and negligible crown fire risk. The highest MC observation in our database (BFL, plot 6: FFMC of 80, MCSA = 19.8%) was associated with a surface fire with a WS10 of 13 km h−1, FSG of 0.7 m and SFC of 1.2 kg m−2 (M. E. Alexander, unpubl. data). Ignition attempts under high moisture conditions failed at several other experimental study sites (e.g. FFMC 84, MCSA = 15.3% at DL; Quintilio et al. 1977; see also Stocks 1987a; Stocks 1989).
Wind speed and canopy influence
The role of wind in fire behaviour is complex, with various interactions and feedbacks between wind and other fire environment elements occurring at multiple scales (Finney et al. 2021). Wind speed has long been known to control ROS (Potter 2012) in surface fire (Rothermel 1972; Nelson and Adkins 1988) and crown fire behaviour models (Rothermel 1991; Alexander and Cruz 2016; Cruz and Alexander 2019). Wind speed remains the most significant variable in our modelling of crown fire occurrence. Our simplest model form (Model A), based only on WS10 and LCBH, correctly predicted crown fire occurrence in over 80% of cases during our cross-validation analysis, along with nearly all cases of upper canopy fuel involvement in two-layered stands (see Appendix 2).
Wind speed in experimental fires was measured with anemometers in a cleared opening at a standard 10-m height (Lawson and Armitage 2008). The windward-exposed edges of experimental plots may have had drier and windier conditions compared with plot interiors (Chen et al. 1999). The line ignition protocol ensured that after the initial 20–30 m, fires were spreading through the plot at a pseudo-equilibrium with environmental conditions. This ensured that the natural fire acceleration process (McAlpine and Wakimoto 1991), where point-source fires have documented delays of 10–90 min or longer to reach equilibrium conditions (Lawson 1972; Kiil 1975; Alexander et al. 1991; McRae et al. 2017), was bypassed. The suggested interpretation is that the present models (as with the FBP or CFIS systems) will therefore be most accurate for predicting the probability of crown fire occurrence from an established equilibrium surface or crown fire ignition source exposed to ambient conditions. These models do not predict crowning during fire acceleration and are not intended for point-source ignition fires in sheltered locations.
Foliar moisture content
FMC was not a significant predictor of crown fire occurrence in our final models, matching the previous findings of Cruz et al. (2004, 2006) and Stocks et al. (2004). FMC appears influential in the ignition of conifer foliage in a physical sense (Fazeli et al. 2022; Melnik et al. 2022; but see Alexander and Cruz 2013). However, in our analysis, FMC was significantly correlated to other variables in our dataset (e.g. WS10, FSG), and as such had minimal explanatory power. Experimental measurement of FMC was also absent in many studies, requiring the use of imprecise regional interpolation curves (Chrosciewicz 1986) or the model in the FBP System for estimating FMC on the basis of calendar date, latitude/longitude and elevation (Forestry Canada Fire Danger Group 1992). Although Van Wagner’s (1977) crown fire initiation model suggests that realistic FMC differences could affect critical FSG values by 2.0 m or more (Keyes 2006), recent studies have noted differences in the seasonality of live conifer tissue flammability compared with FBP System predictions (Melnik et al. 2022) and have called for improved methods of estimation.
Despite the convenience of a pan-specific ‘spring dip’ in foliar moisture causing heightened crown fire hazard (Van Wagner 1974b), the flammability of conifer foliage appears related to multiple physiological variables, including moisture, particle density and phenology (Jolly et al. 2016), as well as species (Russell and Turner 1975; Keyes 2006). The effects of severe drought (Peng et al. 2011) are also not well captured in our dataset and may affect the FMC levels. Although FMC minima in conifers are generally associated with phenology rather than moisture availability (Kozlowski and Clausen 1965; Chrosciewicz 1986; Jolly et al. 2016), severe drought may also impact the timing and amplitude of seasonal FMC variation in unforeseen ways.
Aside from flammability, other factors could affect the FMC signal on the ignition of the foliage above a surface fire. Factors such as the surface fire’s upward heat flux or the contribution of bark fuels (for some species) might confound the FMC effect on crowning. Cruz et al. (2006) found through explicit heat transfer modelling that the increase in time to foliar ignition due to elevated FMC was small compared with the surface fire residence time (Alexander 1998). Therefore, although heightened FMC could delay ignition, canopy fuels will still tend to ignite when high-intensity surface fire passes under them. The binary, in contrast to continuous, response of the process being studied makes it difficult to observe and isolate the effect.
Stand-adjusted fine dead moisture content
The MC of fine forest floor litter has long been known to be a key variable influencing fire behaviour (Van Wagner 1968; Dimitrakopoulos and Papaioannou 2001). Our results showed that the FFMC, an index based on weather conditions alone, remains a significant predictor of crown fire initiation in a variety of conifer forests. Given the large dataset behind the MCSA model (Wotton and Beverly 2007) and that previous studies identified significant overstorey influence on MC and fire behaviour (Rothermel et al. 1986; Tanskanen et al. 2005), we expected that models using the MCSA might perform better than those using FFMC alone. Final results were generally supportive: the best overall performance was Model 11, which used the MCSA along with the adjusted FSG. However, FFMC-based models performed nearly as well, and Model 7 was superior to the MCSA-based Model 8 using the unadjusted LCBH (Table 2). It is logical that an FFMC-based model should outperform one using MCSA at sites using a base LCBH. In dense stands such as SC-IM, incorporating stand density influence on crown fire occurrence (by using the MCSA) but not the effect of ladder fuels creates a bias, where moisture is assumed to be higher due to stand structure but potential ladder fuel effects are ignored. This reduced the accuracy of certain marginal predictions compared with the approach of combining both stand density (via MCSA) and ladder fuel (via adjusted FSG) influences in Model 11. Thus, Wotton and Beverly’s (2007) model for estimating MCSA appears to have some skill in the right context, though a stand-adjusted model with more quantitatively defined predictors would be superior and less subjective to apply. See also Supplementary Material for additional cautions and suggestions for future use of the MCSA model.
Surface fuel consumption
SFC, including contributions from all fine surface fuel load (SFL) elements, is a required input with the present equations. This adds an element of uncertainty, because actual consumption values are never available prior to burning; even the relevant inputs to fuel consumption models, such as SFL, will rarely be on hand in wildfire situations, for instance. A number of tools presently exist for estimating SFC. The simplest of these are probably the FBP System equations that require only the BUI and (or) FFMC and fuel type as inputs, with fixed SFL values (ranging from 1.5 to 5 kg m−2) hard-coded into the fuel consumption equations (Forestry Canada Fire Danger Group 1992). The more comprehensive studies from the FBP System era include site-specific SFC models based on selected FWI System components and SFL (Van Wagner 1972; Stocks 1987a, 1989; Alexander et al. 1991). The forest floor consumption models of de Groot et al. (2009), including data from several sites in the present study (based on the SFL and various FWI System components), offer additional practical and logically consistent options.
It bears mentioning that SFC models will be most accurate when surface fuel conditions match the input data, those being surface fuels characteristic of unmanaged or thinned boreal and sub-boreal conifer forests, featuring moderately compact needle litter, compact duff layers and relatively smaller contributions of dead-down woody fuels. Higher SFC values in our dataset (>~2.5 kg m−2) were typically associated with thicker forest floor loads as well as drier conditions due to elevated BUI levels. Although this fuel consumption likely contributes to surface fire intensity, and thereby crowning (Van Wagner 1977), the relationship between SFC and CFO may not be linear. In our analysis, log-transformed SFC models were slightly more accurate than comparable linear SFC models (e.g. models 5 or 6 vs models 7 or 8; Table 2), effectively muting the influence of a high SFC in certain situations. Significantly different fuelbed types, including those with higher woody debris loads (Agee and Huff 1987) or masticated fuels (Moore et al. 2020) would likely require calibration to produce credible results.
Canopy structure
Van Wagner’s (1977) concept of the canopy fuel layer consisted of ‘a layer of uniform bulk density and height above ground’ (p. 23) and noted in his field experiments that the critical surface intensity for onset of crowning occurred when the surface fire produced a flame front ‘whose tip is just reaching into the crown layer’ (p. 25). Further studies have suggested that flame tips do not need to actually contact the crown base for crown fire initiation to occur (Alexander and Cruz 2012), with time–temperature curves and net energy release being more representative of the canopy ignition process (Xanthopoulos and Wakimoto 1993; Cruz et al. 2006; Melnik et al. 2022). The image of a surface fire flame height reaching the vicinity of the lower live canopy remains useful for visualising crown fire initiation (Alexander and Cruz 2016), although it may exaggerate the critical surface fire intensity needed for crown fire to occur.
For conifer stands with multi-layered canopy fuel structure, our models more explicitly defined the onset of crowning; further vertical propagation may be inferred but is not assured. The biggest difference between the present analysis and previous empirically based crown fire occurrence models (Cruz et al. 2003, 2004) is the use of the 3/2 exponent for the FSG/LCBH term, which leads to higher crowning thresholds as the FSG increases (Fig. 3). This aligns with the findings of McCaffrey (1979), who found the relationship between temperature change and height (z) above a pulsing flame in laboratory studies varied from z−1 to z−5/3 depending on the plume region in question. In all cases, models with the 3/2 exponent performed better than comparable model forms with either linear or squared FSG terms (Table 2). Values of LCBH or FSG used in our analysis represented the mean stratum values at the stand or plot level. Variability in LCBH is clearly important in crown fire initiation and defining crown fire thresholds (Parsons et al. 2011), but the varied nature of the LCBH data precluded studying the effects of LCBH variability in our analyses. Previous studies that combined alternative LCBH definitions with Van Wagner’s (1977) model resulted in significant underprediction bias (Cruz and Alexander 2010).
Ladder fuels remain a significant challenge in the development of accurate crown fire occurrence models. Our use of an adjusted FSG based on dead ladder fuels appears to have improved model performance slightly but still offers uncertain guidance. Users of these models are advised to estimate the FSG as lower than the LCBH when dense fine-textured biomass exists in the sub-canopy space that visibly contributes to vertical fuel connectivity. Future analyses are anticipated to provide additional methods to quantify this process more directly.
Management considerations and conclusion
After six decades of progress, the experimental fire database contains a sufficient number and range of observations to support relatively sophisticated statistical models of crown fire occurrence. Users can select from the various models presented (Table 3) depending on the level of detail of their input data. For instance, for general use in a typical moderately closed conifer stand, FFMC-based models may be adequate, whereas MCSA-based models may be more flexible when considering different stand conditions, such as very open stands or comparisons across seasons. For further details, see Supplementary Table S2 for a comparison of crown fire probability predictions among models under a range of input conditions.
Despite the range of our database (FSG 0.4–13.3 m; MC 6.3–19.8%; SFC 0.2–3.8 kg m−2; WS10 3.0–34.6 km h−1), certain conditions have yet to be captured within the four-dimensional variable space (Fig. 4). Obvious data gaps include fires in high-FSG stands (>6.0 m) under high to extreme fire danger conditions (e.g. WS10 > 15 km h−1, FFMC > 90), observations that will also be relevant to planning effective hazard reduction treatments (Agee and Skinner 2005; Beverly et al. 2020). Fire behaviour observations in extreme conditions will likely only be obtained from detailed wildfire monitoring (Hart et al. 2021), typically through collaboration with fire management organisations. Although there is potential to recover fuel structure for wildfires in areas with LIDAR coverage, obtaining high-quality wind speed and fuel consumption data will continue to be problematic, suggesting that field-scale fire behaviour experiments will still be required.
Changes in fine fuel moisture dynamics and in-stand microclimate caused by fuel treatments (Ma et al. 2010) have often been ignored (Varner and Keyes 2009). This is only partially addressed in our models thanks to the MCSA model. Along with FMC, the influence of conifer species is also unresolved. Although pine and spruce forests comprise most of the Canadian boreal biome, additional tests and evaluation seem warranted in other temperate forests in Canada and worldwide, where other conifer genera are often dominant.
Compared with previous studies using Canadian experimental fire behaviour data (Forestry Canada Fire Danger Group 1992; Cruz et al. 2003, 2004), the present analysis used a greater number of continuous predictors. This may have improved the overall predictive accuracy but requires users to have more detailed inputs. The present models also defined crowning differently from previous studies, at least for multi-layered stands, where crowning is only properly considered for the lowest LCBH or FSG layer. The original CFIS system models (Alexander et al. 2006) will remain useful for more general predictions of crown fire occurrence encompassing full canopy involvement and combined LCBH values in multi-layered stands. As discussed in Appendix 2, the present models and approach may also prove useful for predicting transitions between canopy strata in two-layered stands, but further studies and testing will be required. Our results show continued improvement in crown fire occurrence modelling using experimental data. Such models can be expected to be further refined with time.
Acknowledgements
We are grateful for the efforts of all individuals who led or contributed to the experimental fire database, from the 1960s to the present day. The support of fire management agencies in Alberta, Northwest Territories and Ontario is particularly appreciated. We thank Gregory Greene for reviewing and correcting errors in an earlier draft, as well as the contributions and improvements suggested by two anonymous reviewers.
References
Affleck DLR, Keyes CR, Goodburn JM (2012) Conifer crown fuel modeling: current limits and potential for improvement. Western Journal of Applied Forestry 27, 165-169.
| Crossref | Google Scholar |
Agee JK, Huff MH (1987) Fuel succession in a western hemlock/Douglas-fir forest. Canadian Journal of Forest Research 17, 697-704.
| Crossref | Google Scholar |
Agee JK, Skinner CN (2005) Basic principles of forest fuel reduction treatments. Forest Ecology and Management 211, 83-96.
| Crossref | Google Scholar |
Agee JK, Bahro B, Finney MA, Omi PN, Sapsis DB, Skinner CN, van Wagtendonk JW, Phillip Weatherspoon C (2000) The use of shaded fuelbreaks in landscape fire management. Forest Ecology and Management 127, 55-66.
| Crossref | Google Scholar |
Alexander ME (1988) Help with making crown fire hazard assessments. In ‘People and Homes in the Interior West: Proceedings of the Symposium and Workshop’, 6–8 October 1987, Missoula, MT, USA. General Technical Report INT-251. (Comps WC Fischer, SF Arno) pp. 147–156. (USDA Forest Service, Intermountain Research Station: Ogden, UT, USA)
Alexander ME, Cruz MG (2012) Interdependencies between flame length and fireline intensity in predicting crown fire initiation and crown scorch height. International Journal of Wildland Fire 21, 95-113.
| Crossref | Google Scholar |
Alexander ME, Cruz MG (2013) Assessing the effect of foliar moisture on the spread rate of crown fires. International Journal of Wildland Fire 22, 415-427.
| Crossref | Google Scholar |
Alexander ME, Cruz MG (2016) Crown fire dynamics in conifer forests. In ‘Synthesis of Knowledge of Extreme Fire Behavior: Volume 2 for Fire Behavior Specialists, Researchers, and Meteorologists’. General Technical Report PNW-GTR-891. pp. 163–258. (USDA Forest Service, Pacific Northwest Research Station: Portland, OR, USA)
Alexander ME, Lanoville RA (1989) Predicting fire behavior in the black spruce-lichen woodland fuel type of western and northern Canada. Poster (with text). (Forestry Canada, Northern Forestry Centre and Government of Northwest Territories, Department of Renewable Resources, Territorial Forest Fire Centre: Edmonton, AB and Fort Smith, NT, Canada)
Alexander ME, Quintilio D (1990) Perspectives on experimental fires in Canadian forestry research. Mathematical and Computer Modelling 13, 17-26.
| Crossref | Google Scholar |
Alexander ME, Stefner CN, Mason JA, Stocks BJ, Hartley GR, Maffey, ME, Wotton BM, Taylor SW, Lavoie N, Dalrymple GN (2004) Characterizing the jack pine-black spruce fuel complex of the International Crown Fire Modelling Experiment (ICFME). Information Report NOR-X-393. (Natural Resources Canada, Canadian Forest Service, Northern Forestry Centre: Edmonton, AB, Canada)
Alexander ME, Cruz MG, Lopes AMG (2006) CFIS: a software tool for simulating crown fire initiation and spread. Forest Ecology and Management 234, S133.
| Crossref | Google Scholar |
Bell R (1889) Forest fires in northern Canada. Proceedings of the American Forestry Congress 7, 50-55.
| Google Scholar |
Beverly JL, Leverkus SER, Cameron H, Schroeder D (2020) Stand-level fuel reduction treatments and fire behaviour in Canadian boreal conifer forests. Fire 3, 35.
| Crossref | Google Scholar |
Chen J, Saunders SC, Crow TR, Naiman RJ, Brosofske KD, Mroz GD, Brookshire BL, Franklin JF (1999) Microclimate in forest ecosystem and landscape ecology: variations in local climate can be used to monitor and compare the effects of different management regimes. Bioscience 49, 288-297.
| Crossref | Google Scholar |
Chicco D, Jurman G (2020) The advantages of the Matthews correlation coefficient (MCC) over F1 score and accuracy in binary classification evaluation. BMC Genomics 21, 6.
| Crossref | Google Scholar |
Chrosciewicz Z (1986) Foliar moisture content variations in four coniferous tree species of central Alberta. Canadian Journal of Forest Research 16, 157-162.
| Crossref | Google Scholar |
Coogan SCP, Daniels LD, Boychuk D, Burton PJ, Flannigan MD, Gauthier S, Kafka V, Park JS, Wotton BM (2021) Fifty years of wildland fire science in Canada. Canadian Journal of Forest Research 51, 283-302.
| Crossref | Google Scholar |
Cruz MG, Alexander ME (2010) Assessing crown fire potential in coniferous forests of Western North America: a critique of current approaches and recent simulation studies. International Journal of Wildland Fire 19, 377-398.
| Crossref | Google Scholar |
Cruz MG, Alexander ME (2019) The 10% wind speed rule of thumb for estimating a wildfire’s forward rate of spread in forests and shrublands. Annals of Forest Science 76, 44.
| Crossref | Google Scholar |
Cruz MG, Alexander ME, Wakimoto RH (2003) Assessing the probability of crown fire initiation based on fire danger indices. The Forestry Chronicle 79, 976-983.
| Crossref | Google Scholar |
Cruz MG, Alexander ME, Wakimoto RH (2004) Modeling the likelihood of crown fire occurrence in conifer forest stands. Forest Science 50, 640-658.
| Crossref | Google Scholar |
Cruz MG, Butler BW, Alexander ME, Forthofer JM, Wakimoto RH (2006) Predicting the ignition of crown fuels above a spreading surface fire. Part I: model idealization. International Journal of Wildland Fire 15, 47-60.
| Crossref | Google Scholar |
Cruz MG, Alexander ME, Fernandes PM (2022) Evidence for lack of a fuel effect on forest and shrubland fire rates of spread under elevated fire danger conditions: implications for modelling and management. International Journal of Wildland Fire 31, 471-479.
| Crossref | Google Scholar |
de Groot WJ, Pritchard JM, Lynham TJ (2009) Forest floor fuel consumption and carbon emissions in Canadian boreal forest fires. Canadian Journal of Forest Research 39, 367-382.
| Crossref | Google Scholar |
de Groot WJ, Hanes CC, Wang Y (2022) Crown fuel consumption in Canadian boreal forest fires. International Journal of Wildland Fire 31, 255-276.
| Crossref | Google Scholar |
Dimitrakopoulos AP, Papaioannou KK (2001) Flammability assessment of Mediterranean forest fuels. Fire Technology 37, 143-152.
| Crossref | Google Scholar |
Fazeli H, Jolly WM, Blunck DL (2022) Stages and time-scales of ignition and burning of live fuels for different convective heat fluxes. Fuel 324, 124490.
| Crossref | Google Scholar |
Hart H, Perrakis DDB, Taylor SW, Bone C, Bozzini C (2021) Georeferencing oblique aerial wildfire photographs: an untapped source of fire behaviour data. Fire 4, 81.
| Crossref | Google Scholar |
Jolly WM, Hintz J, Linn RL, Kropp RC, Conrad ET, Parsons RA, Winterkamp J (2016) Seasonal variations in red pine (Pinus resinosa) and jack pine (Pinus banksiana) foliar physio-chemistry and their potential influence on stand-scale wildland fire behavior. Forest Ecology and Management 373, 167-178.
| Crossref | Google Scholar |
Keyes CR (2006) Role of foliar moisture content in the silvicultural management of forest fuels. Western Journal of Applied Forestry 21, 228-231.
| Crossref | Google Scholar |
Kiil AD (1975) Fire spread in a black spruce stand. Canadian Forestry Service Bi-Monthly Research Notes 31, 2-3.
| Google Scholar |
Kozlowski TT, Clausen JJ (1965) Changes in moisture contents and dry weights of buds and leaves of forest trees. Botanical Gazette 126, 20-26.
| Crossref | Google Scholar |
Lee S-L, Emmons HW (1961) A study of natural convection above a line fire. Journal of Fluid Mechanics 11, 353-368.
| Crossref | Google Scholar |
Ma S, Concilio A, Oakley B, North M, Chen J (2010) Spatial variability in microclimate in a mixed-conifer forest before and after thinning and burning treatments. Forest Ecology and Management 259, 904-915.
| Crossref | Google Scholar |
Mangiafico SS (2015) An r companion for the handbook of biological statistics, version 1.09. Available at https://rcompanion.org/rcompanion [accessed 15 December 2022]
McAlpine RS, Wakimoto RH (1991) The acceleration of fire from point source to equilibrium spread. Forest Science 37, 1314-1337.
| Google Scholar |
Melnik OM, Paskaluk SA, Ackerman MY, Melnik KO, Thompson DK, McAllister SS, Flannigan MD (2022) New in-flame flammability testing method applied to monitor seasonal changes in live fuel. Fire 5, 1.
| Crossref | Google Scholar |
Moon K, Duff TJ, Tolhurst KG (2016) Sub-canopy forest winds: understanding wind profiles for fire behaviour simulation. Fire Safety Journal 105, 320-329.
| Crossref | Google Scholar |
Moore B, Thompson DK, Schroeder D, Johnston JM, Hvenegaard S (2020) Using infrared imagery to assess fire behaviour in a mulched fuel bed in black spruce forests. Fire 3, 37.
| Crossref | Google Scholar |
Mount J, Zumel N (2018) The vtreat r package: a statistically sound data processor for predictive modeling. Journal of Open Source Software 3, 584.
| Crossref | Google Scholar |
Nelson Jr RM, Adkins CW (1988) A dimensionless correlation for the spread of wind-driven fires. Canadian Journal of Forest Research 18, 391-397.
| Crossref | Google Scholar |
Parsons RA, Mell WE, McCauley P (2011) Linking 3D spatial models of fuels and fire: effects of spatial heterogeneity on fire behavior. Ecological Modelling 222, 679-691.
| Crossref | Google Scholar |
Peng C, Ma Z, Lei X, Zhu Q, Chen H, Wang W, Liu S, Li W, Fang X, Zhou X (2011) A drought-induced pervasive increase in tree mortality across Canada’s boreal forests. Nature Climate Change 1, 467-471.
| Crossref | Google Scholar |
Perrakis DDB, Lanoville RA, Taylor SW, Hicks D (2014) Modeling wildfire spread in mountain pine beetle-affected forest stands, British Columbia, Canada. Fire Ecology 10(2), 10-35.
| Crossref | Google Scholar |
Perrakis DDB, Cruz MG, Alexander ME, Taylor SW, Beverly JL (2020) Linking dynamic empirical fire spread models: introducing Canadian Conifer Pyrometrics. In ‘Proceedings for the 6th Fuels and Fire Behavior Conference’, 29 April–3 May 2019, Sydney, NSW, Australia - Marseille, France - Albuquerque, NM, USA. pp. 67–72. (International Association of Wildland Fire: Missoula, MT, USA)
Potter BE (2012) Atmospheric interactions with wildland fire behaviour - I. Basic surface interactions, vertical profiles and synoptic structures. International Journal of Wildland Fire 21, 779-801.
| Crossref | Google Scholar |
Powers DMW (2011) Evaluation: from precision, recall and F-measure to ROC, informedness, markedness and correlation. International Journal of Machine Learning Technology 2, 37-63.
| Google Scholar |
Russell RN, Turner JA (1975) Foliar moisture trends during bud swelling and needle flush in British Columbia. Canadian Forestry Service Bi-Monthly Research Notes 31, 24-25.
| Google Scholar |
Stocks BJ (1987a) Fire behavior in immature jack pine. Canadian Journal of Forest Research 17, 80-86.
| Crossref | Google Scholar |
Stocks BJ (1987b) Fire potential in the spruce budworm-damaged forests of Ontario. The Forestry Chronicle 63, 8-14.
| Crossref | Google Scholar |
Stocks BJ (1989) Fire behavior in mature jack pine. Canadian Journal of Forest Research 19, 783-790.
| Crossref | Google Scholar |
Stocks BJ, Lawson BD, Alexander ME, Van Wagner CE, McAlpine RS, Lynham TJ, Dubé DE (1989) The Canadian Forest Fire Danger Rating System: an overview. The Forestry Chronicle 65, 450-457.
| Crossref | Google Scholar |
Stocks BJ, Alexander ME, Wotton BM, Stefner CN, Flannigan MD, Taylor SW, Lavoie N, Mason JA, Hartley GR, Maffey ME, Dalrymple GN, Blake TW, Cruz MG, Lanoville RA (2004) Crown fire behaviour in a northern jack pine – black spruce forest. Canadian Journal of Forest Research 34, 1548-1560.
| Crossref | Google Scholar |
Sullivan AL (2009) Wildland surface fire spread modelling, 1990–2007. 2: Empirical and quasi-empirical models. International Journal of Wildland Fire 18, 369-386.
| Crossref | Google Scholar |
Tanskanen H, Venäläinen A, Puttonen P, Granström A (2005) Impact of stand structure on surface fire ignition potential in Picea abies and Pinus sylvestris forests in southern Finland. Canadian Journal of Forest Research 35, 410-420.
| Crossref | Google Scholar |
Taylor SW, Alexander ME (2006) Science, technology, and human factors in fire danger rating: the Canadian experience. International Journal of Wildland Fire 15, 121-135.
| Crossref | Google Scholar |
Thomas PH (1963) The size of flames from natural fires. Symposium (International) on Combustion 9, 844-859.
| Crossref | Google Scholar |
Thompson DK, Schroeder D, Wilkinson SL, Barber Q, Baxter G, Cameron H, Hsieh R, Marshall G, Moore B, Refai R, Rodell C, Schiks T, Verkaik GJ, Zerb J (2020) Recent crown thinning in a boreal black spruce forest does not reduce spread rate nor total fuel consumption: results from an experimental crown fire in Alberta, Canada. Fire 3, 28.
| Crossref | Google Scholar |
Van Wagner CE (1964) History of a small crown fire. The Forestry Chronicle 40, 202-205, 208-209.
| Crossref | Google Scholar |
Van Wagner CE (1965) Story of an intense crown fire at Petawawa. Pulp and Paper Magazine Canada Woodland Review Section 66, 358-361.
| Google Scholar |
Van Wagner CE (1972) Duff consumption by fire in eastern pine stands. Canadian Journal of Forest Research 2, 34-39.
| Crossref | Google Scholar |
Van Wagner CE (1977) Conditions for the start and spread of crown fire. Canadian Journal of Forest Research 7, 23-34.
| Crossref | Google Scholar |
Van Wagner CE (1990) Six decades of forest fire science in Canada. The Forestry Chronicle 66, 133-137.
| Crossref | Google Scholar |
Van Wagner CE (1993) Prediction of crown fire behavior in two stands of jack pine. Canadian Journal of Forest Research 23, 442-449.
| Crossref | Google Scholar |
Varner JM, Keyes CR (2009) Fuels treatments and fire models: errors and corrections. Fire Management Today 69(3), 47-50.
| Google Scholar |
Weber MG, Van Wagner CE, Hummel M (1987) Selected parameters of fire behavior and Pinus banksiana Lamb. regeneration in eastern Ontario. The Forestry Chronicle 63, 340-346.
| Crossref | Google Scholar |
Whitehead RJ, Russo GL, Hawkes BC, Taylor, SW, Brown BN, Armitage OB, Barclay OJ, Benton RA (2008) Effect of commercial thinning on within-stand microclimate and fine fuel moisture conditions in a mature lodgepole pine stand in southeastern British Columbia. Information Report FI-X-004. (Natural Resources Canada, Canadian Forest Service and Canadian Wood Fibre Centre: Victoria, BC, Canada)
Wotton BM, Beverly JL (2007) Stand-specific litter moisture content calibrations for the Canadian Fine Fuel Moisture code. International Journal of Wildland Fire 16, 463-472.
| Crossref | Google Scholar |
Xanthopoulos G, Wakimoto RH (1993) A time to ignition-temperature-moisture relationship for branches of three western conifers. Canadian Journal of Forest Research 23, 253-258.
| Crossref | Google Scholar |
Zhang P (1993) Model selection via multifold cross validation. The Annals of Statistics 21, 299-313.
| Crossref | Google Scholar |
Appendix 1. List of abbreviations and acronyms.
Abbreviation | Units or levels | Definition | SourceA | |
---|---|---|---|---|
BFL | – | Big Fish Lake (experiment site, Ontario) | 1 | |
BS | – | Black Spruce (Picea mariana) | 1, 17 | |
BUI | – | Buildup Index (Fire Weather Index System) | 2 | |
CF | – | Crown Fire | This study, 8 | |
CFIS | – | Crown Fire Initiation and Spread (system) | 3, 4 | |
CFO | 0 or 1 | Crown Fire Occurrence | This study, 4 | |
DBH | cm | Diameter at Breast Height | ||
DC | – | Drought Code (Fire Weather Index System) | 2 | |
DL | – | Darwin Lake (experiment site, Alberta) | 5 | |
DMC | – | Duff Moisture Code (Fire Weather Index System) | 2 | |
FBP | – | Fire Behaviour Prediction (System) | 1 | |
FFMC | – | Fine Fuel Moisture Code (Fire Weather Index System) | 2 | |
FMC | % | Foliar Moisture Content (live conifer) | 1, 8 | |
FSG | m | Fuel Strata Gap | 4 | |
FWI | – | Fire Weather Index (index or System) | 2 | |
ICFME | – | International Crown Fire Modelling Experiment (exp. site, Northwest Territories) | 6 | |
JP | – | Jack Pine (Pinus banksiana) | 7, 13 | |
KL | – | Kenshoe Lake (experiment site, Ontario) | 7 | |
LCBH | m | Live Crown (or canopy) Base Height | 8 | |
LP | – | Lodgepole Pine (Pinus contorta) | 1, 12 | |
MC | % | Moisture Content, fine dead litter | 9, 10 | |
MCC | – | Matthews Correlation Coefficient | 11 | |
MCFFMC | % | Moisture Content estimate, fine dead litter, from the FFMC; also MC.FFMC | 9 | |
MCSA | % | Stand-Adjusted Moisture Content estimate, fine dead litter | 10 | |
PG | – | Prince George (or Summit Lake; experiment site, British Columbia) | 12 | |
PL | – | Porter Lake (experiment site, Northwest Territories) | 16 | |
PM | – | Pelican Mountain (experiment site, Alberta) | 17 | |
PNFI | – | Petawawa National Forestry Institute (experiment site, Ontario) | 1 | |
ROS | m/min | Rate of Spread | 1 | |
RP | – | Red Pine (Pinus resinosa) | 1, 18 | |
SC-IM | – | Sharpsand Creek (experiment site, Ontario), Immature plots | 13 | |
SC-SM | – | Sharpsand Creek (experiment site, Ontario), Semi-Mature plots | 14 | |
SC-TH | – | Sharpsand Creek (experiment site, Ontario), Thinned plots | 13 | |
SF | – | Surface Fire | This study, 8 | |
SFC | kg/m2 | Surface Fuel Consumption | 1, 8 | |
SFC.CLS | 1, 2, or 3 | Surface Fuel Consumption Class; classes are 0–0.99, 1.0–2.0, >2.0 kg m−2 | This study, 4 | |
SFL | kg m−2 | Surface Fuel Load | 1 | |
WRP | – | White (Pinus strobus) and Red Pine (P. resinosa) species mix | 1 | |
WS10 | km h−1 | 10 m open wind speed | 15 |
ASources: 1: Forestry Canada Fire Danger Group (1992); 2: Van Wagner (1987); 3: Cruz et al. (2003); 4: Cruz et al. (2004); 5: Quintilio et al. (1977); 6: Alexander et al. (2004), Stocks et al. (2004); 7: Stocks 1989; 8: Van Wagner (1977); 9: Van Wagner (1974a); 10: Wotton and Beverly (2007); 11: Chicco and Jurman (2020); 12: Lawson (1972); 13: Stocks (1987a); 14: McRae et al. (2017); 15: Lawson and Armitage (2008); 16: Alexander et al. (1991); 17: Thompson et al. (2020); 18: Van Wagner (1968). See main text for full references.
Appendix 2. Upper stratum crowning in two-layered stands
As discussed in the main text, two sites in our dataset had clearly defined dual-layer stands with black spruce in the understorey and mid-storey beneath an upper overstorey of jack pine. These included the KL site (Fig. 1e) and most plots at the ICFME site (Fig. 1g).3 In contrast to previous analyses (e.g. Van Wagner 1993; Cruz et al. 2004), we treated the crowning process separately between these two strata. The main modelling in our study considered only the crown involvement of the mid-canopy black spruce at both sites, the lower layer proximal to the surface fuel layer.
For testing upper canopy fuel involvement, a conditional process was evaluated. Experimental plots at KL and ICFME predicted to involve crowning into the spruce layer were subsequently tested for crowning into the upper pine stratum. This was clearly a different process than that intended by the traditional notion of crown fire initiation models, with a surface fire testing the overall LCBH or FSG (Van Wagner 1977; Scott and Reinhardt 2001). However, we wished to explore whether there might be merit to using empirically based crown fire occurrence models to predict vertical flame transitioning between canopy strata.
To do this, a first assumption was made that the heat flux for upper canopy involvement originated from the crown centroid (CS) of the mid-storey spruce stratum:
where HS and LCBHS represent the mean spruce stratum height and spruce LCBH, respectively. The stratum gap between fuel layers would then be defined as
where FSGSP is the mean spruce-pine FSG and LCBHP is the pine live crown base height. The relevant plot-level measures were calculated from stand data for the ICFME (Alexander et al. 2004) and sites (see Supplementary Material). Because most of the described empirical models also require estimates of SFC, which is undefined in this context, this was only intended as an exploratory analysis at the present. We selected mid-range values of SFC as necessary and used measured or estimated values for other variables (WS10 and MCSA or MCFFMC).
Stand calculations gave mean FSGSP values of 5.7 m (range: 4.8–6.5 m) at KL and 4.3 m (range: 2.5–5.8 m) for ICFME plots. Using Model 11 with a mid-range SFC value (1.5 kg m−2; between median and mean values of 1.42 and 1.57 kg m−2, respectively) along with actual MCSA values (6.3–13.5%), crown fire occurrence was correctly predicted in 14 of 15 observations (i.e. 93% correct). Similar results (14/15 correct) were obtained using Model 9, with SFC class 2 (1.0–1.99 kg m−2) along with actual MCFFMC values (i.e. 6.5–13.5%). The two models requiring no fuel consumption estimates (Models A and B) also gave identical results, using WS10 and FSGSP alone (A) or WS, FSGSP and MCFFMC (B). In all cases, the only misclassified fire was ICFME Plot 2, a passive crown fire involving the full canopy spruce and pine layers; this burn was otherwise notable for its relatively low WS10 (7.9 km h−1) and FFMC (89.5) values for an intense crown fire involving the full canopy.
This cursory exploration of upper canopy crowning showed that such crown fire occurrence models may have the potential to help predict crown fire transitions in vertically complex stands, by adopting a conditional process of evaluating one stratum at a time. This process involves making several significant assumptions that will require further study and testing. These include vertical fuel continuity and flame propagation through the mid-canopy stratum (in our case, from the LCBHS to the CS and higher), as well as the assumption that variables such as WS10 and MC can be applied unchanged to elevated fuel strata. The greatest uncertainty is probably associated with the arbitrarily assigned SFC value (in Models 10 and 11), which, if it can be applied to upper crowning at all, should properly reflect heat flux contributions from both surface (dead, compact) and lower canopy (live, aerated) fuels. With such a small and unbalanced dataset (15 observations, with only three cases of upper CFI failure), additional observations and studies are clearly warranted to further inform this type of analysis.
Footnotes
1 Although it is not explained in detail in the report on the FBP System (Forestry Canada Fire Danger Group 1992), the C-3 fuel type models included the SC-TH plots, whereas the C-4 fuel type models included the SC-IM plots.
2 The standard FFMC model predicts litter moisture of 19.8% for an FFMC of 82. A nearly identical MCSA would be predicted to occur under the following conditions (as per Wotton and Beverly 2007): FFMC 82, DMC 38, pine stand type, moderate stand density, and SU season.
3 ICFME plots 3 and 4 had no mid-storey black spruce layer (Alexander et al. 2004) and are therefore excluded from this discussion.