A decision support tool for habitat connectivity in Australia
Patrick Norman
A
Abstract
Species connectivity mapping is a technically challenging task for conservation practitioners and nongovernment organisations to undertake as it requires experience in geographic information systems and often some computer programming.
We developed a decision support tool to provide spatial information and data on potential habitat connectivity and optimum connectivity pathways for a selection of forest-dependent vertebrate fauna in eastern and south-western Australia.
We systematically searched spatial data repositories for Australian spatial datasets for modelling connectivity. A least cost paths and patch connectivity approach was used to map potential habitat connectivity for (1) a single species – the glossy black cockatoo (Calyptorhynchus lathami) of South East Queensland, and (2) four species guilds – rainforest pigeons, gliding possums, the black cockatoos of south-western Western Australia and a landscape level forest connectivity. Optimum connectivity pathways were assessed for protection status.
In total 71 spatial datasets useful for habitat connectivity mapping were identified. Species and guild modelling found that the protection status for optimum connectivity pathways varied between 24.7% and 53.3%. A decision support mapping tool was then created to enable users to interactively explore the connectivity data and download the spatial datasets for further analysis.
The development of a decision support tool for mapping habitat connectivity in eastern and south-western Australia represents a useful platform for conservation practitioners as it provides valuable spatial information on potential connectivity pathways for forest-dependent vertebrate fauna.
The tool can aid in the prioritisation of conservation actions aimed at enhancing habitat connectivity and mitigating the impacts of habitat fragmentation on biodiversity in the two regions.
Keywords: conservation, decision support tool, environmental managers, habitat connectivity, integral index of connectivity, interactive tool, least cost paths, species habitats.
Introduction
The protection and restoration of habitat connectivity plays an important role in the conservation of species including for metapopulation dynamics and natural adaptation responses to climate change (Soulé et al. 2004; Klein et al. 2009). As climate change impacts accelerate and human activities exert growing pressures on ecosystems from agricultural expansion and intensification, urbanisation, and infrastructure development (McDonald et al. 2008; Liu et al. 2016), assessing and safeguarding habitat connectivity has become an urgent conservation priority, particularly within extensively cleared landscapes where the remaining habitat is highly fragmented (Fischer and Lindenmayer 2007). This problem poses significant challenges for conservation practitioners who aim to maintain and restore ecological connectivity within these areas (Hoekstra et al. 2005; Mackey et al. 2008; Keeley et al. 2018).
As natural landscapes give way to human-altered environments, the integrity of habitats becomes increasingly compromised. This poses a threat to the survival of many wildlife species, as the ability of individuals, including juveniles, to move and breed with other populations in neighbouring habitats or occupy locations that become vacant through local extirpation events such as bushfires, becomes limited or extinguished (Soulé et al. 2004). Maintaining habitat connectivity therefore is critical for ensuring genetic diversity and facilitating the flow of genes among populations, ultimately supporting the resilience and long-term survival of many species in the face of a changing climate (Mackey et al. 2008; Nuñez et al. 2013; Correa Ayram et al. 2016). With increasing pressures for species movement availability, information on current and potential habitat connectivity is important for monitoring and planning for species persistence within landscapes and regions (Klein et al. 2009; Edelsparre et al. 2018).
Habitat connectivity modelling in Australia is an important tool for conservation planning that aims to maintain and restore the landscape linkages essential for wildlife movement and genetic exchange (Soulé et al. 2004). Studies assessing connectivity modelling in Australia make up 6% of the global analyses assessing habitat connectivity prior to 2016 (Correa Ayram et al. 2016), highlighting the importance of connectivity assessments in the region. Previous scientific studies have demonstrated the importance of connectivity for species and species guilds survival, such as mapping connectivity for forest vertebrates through fragmented landscapes (Liu et al. 2018). In Australia habitat connectivity modelling methods have been developed and applied to help identify potential habitat corridors, most notably by Drielsma and colleagues (Drielsma et al. 2007, 2022). The need for connectivity modelling is increasing due to the impacts of climate change and land use and there is a growing demand for making the spatial outputs available to decision makers, including government agencies and community organisations. It is also important that habitat connectivity assessments are revised to take into account updated information on landscape cover change and new data sources (e.g. Norman and Mackey 2023).
Practitioners engaged in the critical task of assessing and preserving habitat connectivity face multifaceted challenges (Keeley et al. 2018, 2021). Understanding the ecological, spatial, and anthropogenic factors, and identifying current and potential critical movement pathways at scale requires spatial analyses and the use of tools and approaches that are often only accessible through coding languages and often require large compute power. Furthermore, the dynamic nature of ecosystems requires consideration of adaptive strategies that can accommodate changing conditions and emerging threats. This complexity can make it challenging for those on the frontlines of conservation to develop effective, evidence-based strategies and area-based conservation plans.
In light of these challenges, the primary objective of this study was to develop a decision support tool for conservation practitioners for enhancing habitat connectivity within forest and woodland landscapes in eastern Australia and south-western Western Australia. This tool was designed to support targeted conservation efforts by: (1) assembling and making available key spatial datasets useful for assessing and mapping habitat connectivity; (2) displaying preprocessed connectivity modelling data through a web interface for users without GIS capabilities; and (3) providing an interactive map and datasets for habitat connectivity analysis and conservation planning.
Materials and methods
The tool focused on landscapes in eastern and south-western Australia that are naturally dominated by forest and woodland ecosystems and include extensive areas that have been subject to varying degrees of deforestation and degradation. Both regions are biodiversity hotspots with large areas cleared for the conversion to agricultural lands (Mittermeier et al. 2011). These regions were selected due to their ecological significance and rich biodiversity, and because they are under immense human pressures.
We focused our connectivity analyses on: (1) a single focal species – the glossy black cockatoos (Calyptorhynchus lathami) of South East Queensland; and (2) three species guilds – rainforest pigeons, gliding possums and the black cockatoos of south-western Western Australia. Glossy black cockatoos were selected because of previous work conducted by the Glossy Black Conservancy, who mapped critical habitat resources within the study region (Glossy Black Conservancy 2022). Due to the scope of the project, the connectivity mapping for this species was restricted to the defined geographic area of South East Queensland.
For species guilds, habitat mapping was conducted at a multispecies level as guilds share fundamental habitat requirements. The rainforest pigeons included a suite of species with similar requirements, being largely frugivores, such as white-headed pigeon (Columba leucomela), topknot pigeon (Lopholaimus antarcticus) and Torresian imperial pigeon (Ducula spilorrhoa). These species are known to require fruiting tree species and require rainforests and, in some cases, wet eucalypt forests for survival (Innis 1989; Gosper and Gosper 2008). The gliding possums similarly have niche overlapping habitat requirements. Widespread Australian species within the genus of gliding possums, Petaurus (P. australis, P. breviceps and P. norfolcensis) either prefer or require intact stands of Eucalyptus forests and woodlands for feeding, breeding and roosting (Brearley et al. 2011; Lindenmayer et al. 2021; Bilney et al. 2022). Finally the three species of black cockatoos (Zanda latirostris, Z. baudinii and Calyptorhynchus banksii naso) in south-western WA are highly dependent on mature forests and woodlands for their survival (Abbott 1998; Lee et al. 2013; Rycken et al. 2022, 2023).
Additionally, to ensure that forest generalists across eastern Australia were considered for the study, a landscape level forest connectivity model was also performed to identify important areas for connectivity across the extensive area of habitat. The forest generalist model was primarily based on forest cover as the critical component for connectivity, without being constrained by the distances between patches. Furthermore, the focal species, species guilds and forest generalists currently lack connectivity models and together cover a wide range of potential movement types within the regions.
Our connectivity models comprise potential wildlife patches and important movement pathways between known or potential habitat locations. Both techniques complement each other by identifying different aspects of habitat connectivity, with the least cost path analysis providing insights into the optimal routes for movement across the landscape, whereas the integral index of connectivity emphasises the overall connectivity and cohesion of distinct habitat patches. Each species and guild’s potential movement pathway is modelled based on published information regarding their mode and scale of movement, along with other pertinent habitat and life history details. Note that given the lack of systematic wildlife surveys, most spatial habitat models for species are based on mapping the occurrence of forest areas known to have the required vegetation habitat resources needed for their reproduction, shelter and feeding, rather than being locations where the species current occupancy is verified (Guisan and Thuiller 2005; Cassini 2011).
Connectivity related datasets
A systematic approach was used to identify the spatial layers needed for modelling connectivity in the study region. Web platforms were searched for datasets relating to: vegetation, forest cover, habitat connectivity, fragmentation, streamlines, fire history, and logging history, between January and June, 2022. Platforms accessed were from a series of government sources, including states and territories intersecting the study area along with national repositories. National data sources searched included TERN (Cleverly et al. 2019), data Australia (Australian Government 2023) and Geosciences Australia (Geosciences Australia 2023). State and territory layers were sourced from each jurisdiction’s official data repositories, which included SEED (NSW Government 2023), Qspatial (Qld Government 2023), TheList (Tas Government 2023), Data Vic (Vic Government 2023) and Data WA (WA Government 2023). Additionally, global data repositories were also searched including Earth Engine Data Catalogue (Google 2023) and the Global Forest Watch open data portal (World Resources Institute 2023). All gathered spatial layers were assessed using QGIS (QGIS Development Team 2023) and checked for validity. Datasets were later compared to others across state and territory borders to check for continuity and relatedness. Globally applicable datasets were sourced during the process and later compared to those found during the search through national datasets.
Species habitat mapping
Species habitats mapping was performed through using preestablished sources or by modelling species and guilds habitats using the EcoCommons platform (EcoCommons Australia 2022) in conjunction with data from the Atlas of Living Australia (Atlas of Living Australia 2020) and eBird (Sullivan et al. 2014). Additional species related information was derived from other sources including habitats of Glossy Black Cockatoos in South East Queensland (Glossy Black Conservancy 2022) and the south-western Western Australian Black Cockatoos (Birdlife International 2023). For the gliding possums and rainforest pigeon guilds, which did not have publicly available habitat models, species habitat mapping was performed using the EcoCommons platform. The modelling platform provides access to the relevant biological data, environmental data, statistical models, and compute power (EcoCommons Australia 2022). Variable and model performance was assessed within the platform, allowing for a seamless output of species distribution models for input to the connectivity modelling. The species guilds required the use of an aggregation of distribution models for the three species using a combination of the best performing boosted regression tree and random forest species distribution models. Models were assessed using variable importance plots along with the area under the curve values (AUC).
Quantifying habitat patch connectivity
We modelled habitat patch connectivity for each species and species guild using the Integral Index of Connectivity (IIC) (Saura and Pascua-Hortal 2007) with the Makurhini R package (Godínez-Gómez and Correa Ayram 2020). The IIC metric quantifies habitat patch network interconnectivity and aids understanding of patterns and ecological dynamics within a landscape (Keeley et al. 2021). The key output of the analysis is the delta Integral Index of Connectivity (dIIC), which measures the importance of habitat patches to overall landscape connectivity by partitioning their contributions into three fractions: intra (internal size); flux (species movement); and connector (stepping stone role).
To calculate the index for each species and guild, the edge distance between patches was used, along with the maximum forest gap crossing from the literature (Table 1). The landscape level forest connectivity patches were divided by roads as these form anthropic barriers to species movements (Cork et al. 2024). Roads were defined using the OpenStreetMap selecting only for roads (OpenStreetMap Contributors 2023). Due to system memory constraints, for each iteration of the analysis a minimum patch threshold was used as shown in Table 1. All patches were then assessed for their conservation status by determining how much of their areas were located within protected areas. The resulting outputs from the analysis can be accessed using the decision support tool.
Species guild | Forest gap crossing distance (m) | Water crossing distance (m) | Exotic plantation weighting | Treeless urban environment weighting | Preferred habitat (lowest resistance score) | Minimum patch size for IIC analysis (ha) | |
---|---|---|---|---|---|---|---|
Landscape level forest connectivity | Unrestricted | Masked | 1 | 1 | All forests and woodlands | 250 | |
Rainforest pigeons | 80,000 | 80,000 | 5 | 5 | Rainforest | 10 | |
Gliding possums | 100 | 100 | Masked | Masked | Eucalypt forests and woodlands | 50 | |
Glossy black cockatoos (SEQ) | 30,000 | 20,000 | 6 | 7 | Identified ecosystems (Glossy Black Conservancy 2022) | 10 | |
Black cockatoos of south-western WA | 17,000 | Masked | 4 | 6 | Eucalypt forests and woodlands | 100 |
All were sourced or calculated from source materials (Eyre 2006; Price 2006; Drielsma and Ferrier 2009; Barrett and Love 2012; Foster et al. 2017; Rycken 2019; Drielsma et al. 2022; Glossy Black Conservancy 2022; Rycken et al. 2022). Masked values represent areas excluded from the connectivity analysis.
Optimum connectivity pathway mapping
For each species and guild, a set of criteria was applied to ensure that the connectivity models accurately represent movement requirements. This study built upon a connectivity mapping methodology developed by Drielsma et al. (2007) and Barrett and Love (2012), which uses pathways modelling based upon a resistance-based metric utilising a least cost path approach. This requires calculation of cost (movement resistance) spatial layers. Associated movement cost layers are important as they help to identify pathways by finding the path of least resistance between habitat areas (Keeley et al. 2021). The methods used for this study were also used to assess and map potential habitat connectivity for greater gliders in Queensland, Australia (Norman and Mackey 2023). Due to both study regions having forests and woodlands as the dominant growth form, the identified optimum connectivity pathways were primarily restricted to areas with tree cover greater than 10% (Hansen et al. 2013), aligning with the Food and Agricultural Organization’s definition of forests (FAO 2020). It was also assumed that all species and guilds prefer habitats in a better condition, and therefore a weighting was applied using the Habitat Condition Assessment System (HCAS) spatial layer (Harwood et al. 2016). Forest types were established using the forests of Australia dataset (Australian Government 2018).
A description of the key ecological assumptions and values for each species and guild made in the connectivity modelling is given in Table 1, including in relation to forest gap and water crossing distances, as well as habitat and disturbance weights. These values drew upon findings from two comprehensive reviews on habitat connectivity (Correa Ayram et al. 2016; Keeley et al. 2021) along with specific species values established through other source materials (Eyre 2006; Price 2006; Drielsma and Ferrier 2009; Barrett and Love 2012; Foster et al. 2017; Rycken 2019; Drielsma et al. 2022; Glossy Black Conservancy 2022; Rycken et al. 2022).
A workflow was created to streamline the analytical process and ensure a consistent and reproducible method. The pathways modelling method, based upon a resistance-based metric utilising a least cost path approach, combines both the direct paths approach with a forced randomness of start and end points within habitat areas. All programming was through a Bash script and used the following software; PostGIS (PostGIS Project Steering Committee and Others 2022), Guidos Toolbox (Vogt and Riitters 2017), OrfeoToolbox (Grizonnet et al. 2017) and Whitebox Tools (Lindsay 2016). The workflow (Fig. 1) allowed for tens of millions of paths to be assessed to reveal potential connections for target species within a given landscape, making the method workable over large geographic areas.
The methodological workflow used for the connectivity modelling based on the optimum connectivity pathway analyses and the integral index of connectivity.
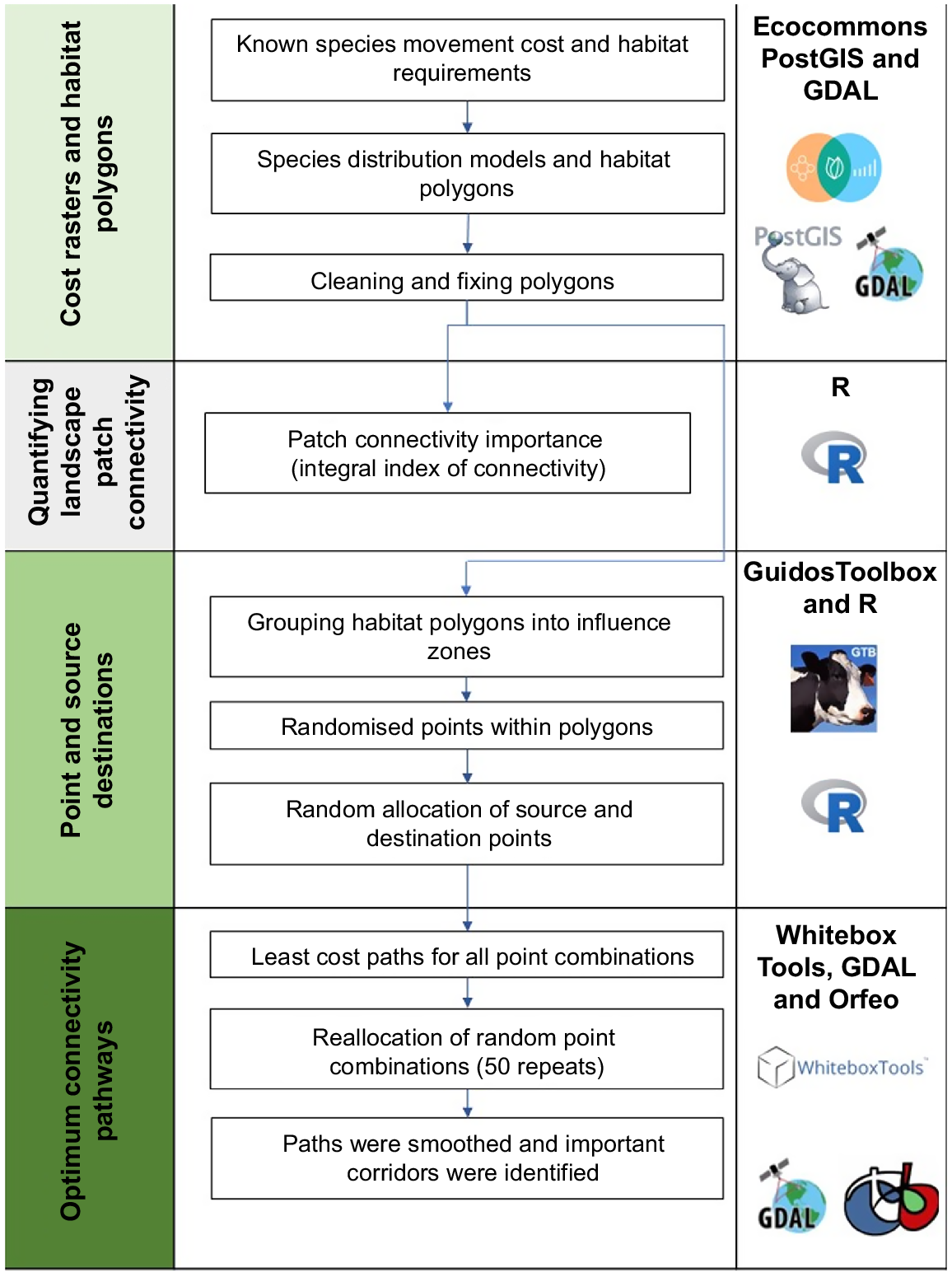
Initially, habitat patches were identified for each species and guild, and then aggregated into clusters using the Influence Zone tool in the GuidosToolbox software (Vogt and Riitters 2017). This processing step ensured that the start and finish paths were not located within the same patch, promoting the search for interpatch connectivity. Randomised points were generated throughout each cluster in proportion to patch size to serve as the start and finish points for the least cost paths using the sf R package (Pebesma 2018). By employing a randomised start and finish approach, the analysis ensured that all parts of the patches and potential connectivity pathways were equally considered, preventing any unintentional bias in pathway prioritisation. The process involved finding the nearest start and finish points between different clusters and randomly changing target clusters over 50 iterations. All paths were subsequently summed and smoothed to highlight optimal pathway importance. Finally, output rasters were normalised to values between 0 and 1.
Following the calculation of each connectivity model, we identified a set of key pathways by selecting the 99.9th percentile within each layer, using the Geospatial Data Abstraction Library (GDAL) through the bash command line and Python (GDAL/OGR 2024). This approach was chosen to focus attention on areas of highest importance for connectivity within the expansive set of potential pathways. However, we recognise that thresholding connectivity in this way did result in some discontinuous paths. Additionally, the location of each pathway in relation to protected areas was established using the Collaborative Australian Protected Areas Database 2022 (Australian Government 2022), providing a basis for integrating these findings into broader conservation planning efforts.
Results
Findings from spatial data searches
A systematic search of spatial layers revealed a range of datasets that wereeither useful for the direct assessment of connectivity conservation or that could be utilised for additional spatial prioritisation or connectivity analyses. In total, 71 spatial datasets were identified from 61 projects using the search and screening process. The identified spatial layers are listed in Supplementary Table S1. They ranged from the extensive global layers, including potential habitat pathways, such as the Global Safety Net project (Dinerstein et al. 2020) with a wide range of input species, to the fine-scale layers like the koala corridors produced by Logan City Shire, in Queensland (Logan City Council 2019).
The usefulness of these datasets depends on the approach and question assessed by platform users, species or assemblages, and the scale required. Many national datasets were found to provide seamless crossjurisdictional information applicable for broader scaled approaches to connectivity mapping. However, boundary effects are evident on the edges of jurisdictions (e.g. state boundaries within Australia), which limit many spatial datasets from being used to map connectivity at a continental scale.
Patch connectivity results
In total, 35,632 patches were assessed for connectivity using the Integral Index of Connectivity. The extent of patch areas assessed varied among the species and guild models, with the largest being the landscape level forest connectivity patches, covering an area of 69,489,682 M ha of forested habitats across 11,121 patches over 250 ha in extent. The conservation status of patches varied with 69% of rainforest pigeon habitat patches located within protected areas, whereas the other species and guilds patches had less than 35% of habitat patches within protected areas, and landscape level forests had the least at 24%.
The mean patch connectivity results (dIIC) also showed that the adequacy of the protected area network in conserving important patches of habitat differed across species and guilds (Table 2). The lower patch connectivity score (dIIC = 2.82) for the landscape level forest connectivity when compared to the average (dIIC = 3.41) suggests that much of the area located within the important patch areas is outside the current protected area network. A similar result was found for the black cockatoos of south-western WA (total dIIC = 5.13, protected area dIIC = 4.42).
Species guild | Total patch area (ha) | Total patch area in protected areas (ha) | In protected areas (%) | Mean patch connectivity score (dIIC) | Mean patch connectivity score (dIIC) in protected areas | |
---|---|---|---|---|---|---|
Landscape level forest connectivity | 68,978,384 | 16,283,723 | 23.6% | 3.41 | 2.82 | |
Rainforest pigeons | 1,521,898 | 1,042,521 | 68.5% | 5.06 | 4.97 | |
Gliding possums | 6,607,798 | 2,304,253 | 34.9% | 4.19 | 4.23 | |
Glossy black cockatoos (SEQ) | 1,545,293 | 469,126 | 30.4% | 2.94 | 3.95 | |
Black cockatoos of south-western WA | 1,473,050 | 486,995 | 33.1% | 5.13 | 4.42 |
Optimum pathway results
The priority habitat pathways for the south-western Western Australia and along the eastern seaboard of Australia are shown in Fig. 2. The pathways found were identified as the most important for each of the species and guilds as these have been restricted to the 99.9th percentile pathways. There was a marked difference between the protection status of each of the optimum connectivity pathways found (Figs 2 and 3). Optimum rainforest pigeon pathways were found to have the highest percentage of pathways protected with 53.3% located within conservation areas, whereas only 26.2% of black cockatoo of south-western WA were conserved.
The optimum connectivity pathways (99.9th percentile) for each species and guild assessed in the study shown in different colours. (a) Zoomed inset maps of south-western Western Australia and (b) eastern Australia. Areas shown as green represent forest and woodland cover with greater than 10% canopy cover (Hansen et al. 2013).
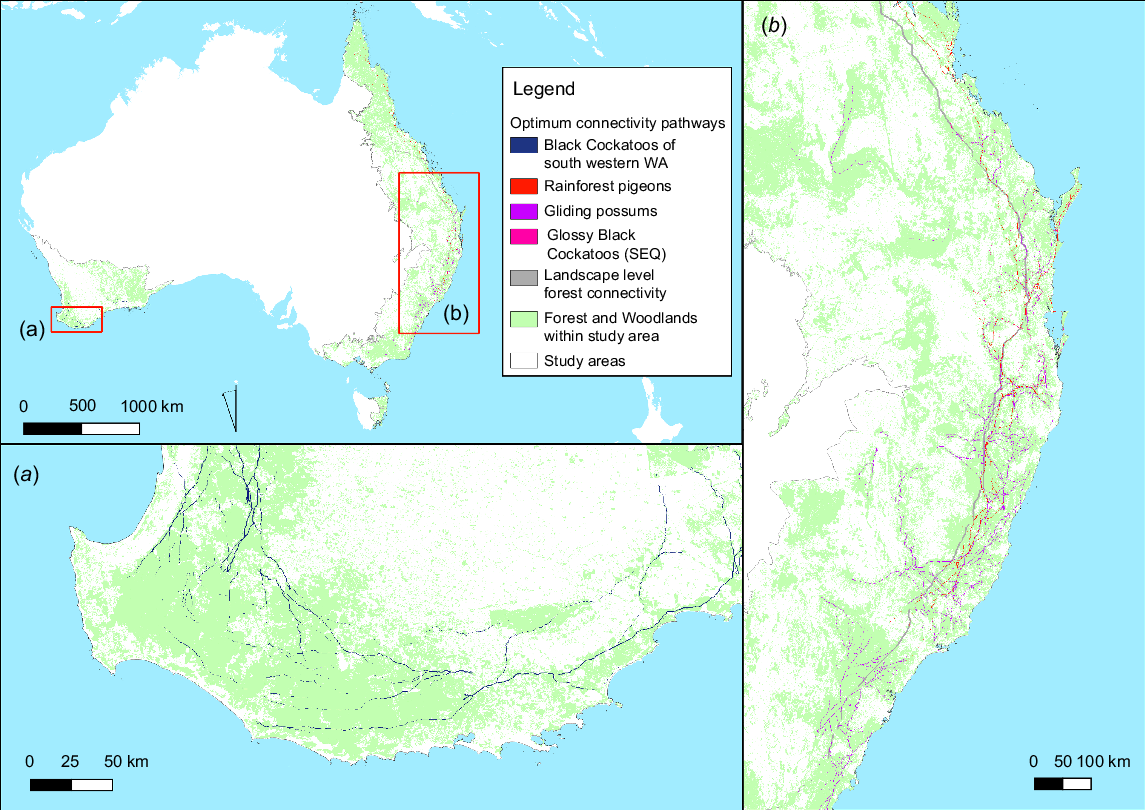
The area of important connectivity pathways (99.9th percentile) in relation to the protection status. Numbers shown above each bar are the percentage of important pathways located within protected areas (PAs).
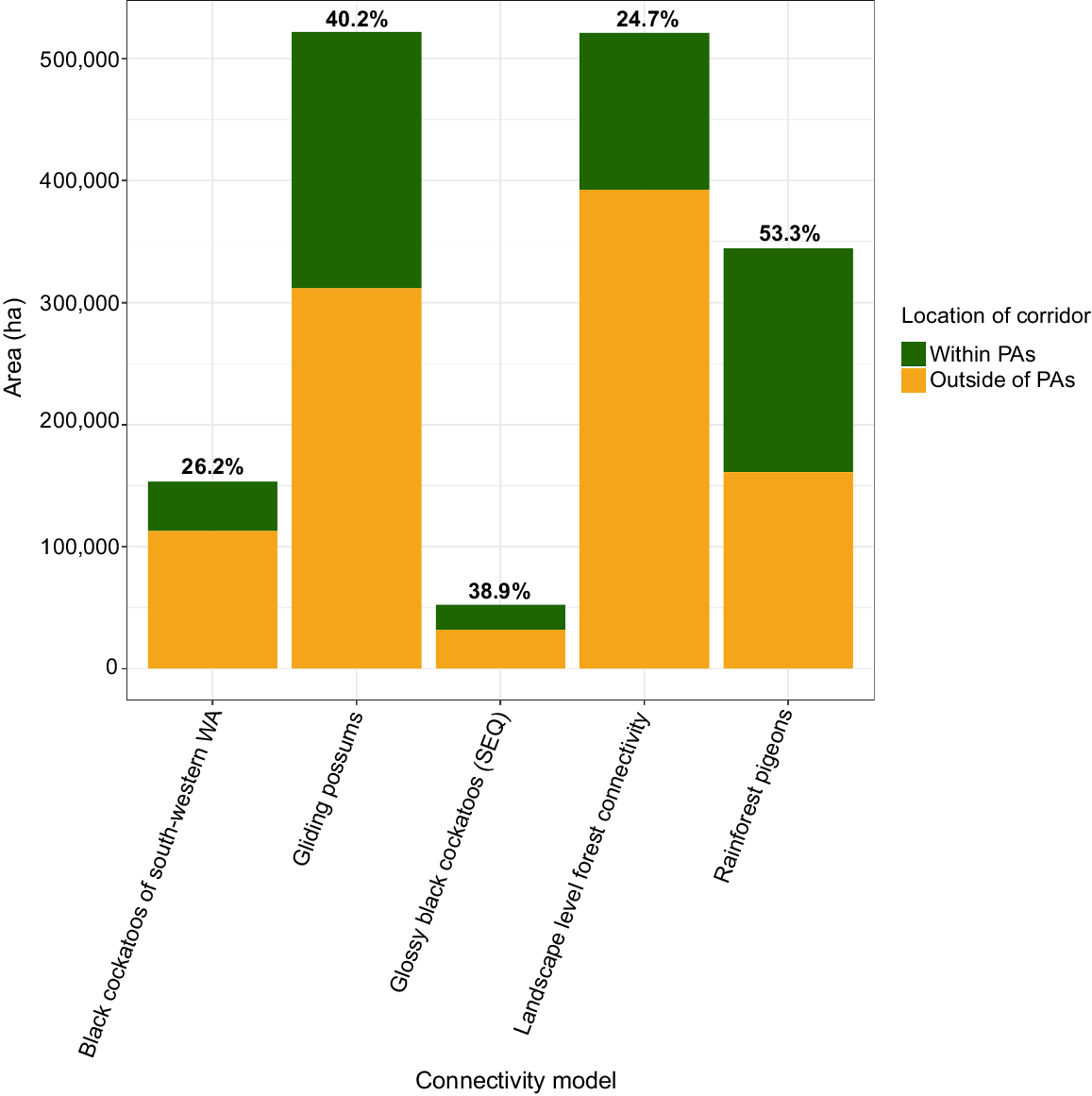
Important movement pathways were identified throughout the eastern seaboard of Australia, with clear pinch points and gaps being identified in numerous landscapes including the upper reaches of the Lockyer and Mary Valley’s in Queensland, the Hunter Valley and Southern Highlands in New South Wales and the Kilmore Gap in Victoria. Likewise in Western Australia, habitat patches north of Albany were found to be important areas for black cockatoo movements across the southern portion of their range.
Decision support tool
An online web-based support tool was created to provide conservation groups and managers with the means to access and view the connectivity pathways for planning and restoration opportunities. As advanced geographic information systems (GIS) capabilities remain limited in many community-based not-for-profit organisations, there is a need for a simple tool that is accessible on desktops and mobile devices. Datasets found during the connectivity dataset search were presented in a table along with the associated links for access. An interactive map was created to allow for rapid assessment by the user of the connectivity pathways (Fig. 4). For users wanting access to the underlying datasets, clipped raster files for each bioregion were produced and were made accessible on the interactive map via a link that retrieves these premade spatial datasets within the area of interest.
An example image of the interactive map page of the decision support website, found at www.stateofconnectivity.com. Users are able to interact with the map, along with downloading the created spatial datasets for each associated IBRA region. The map shows the patch importance over the corridor importance for gliding possums on the border between New South Wales and Queensland. The blue and teal patches and corridors represent the more important areas for connectivity found in the analyses.
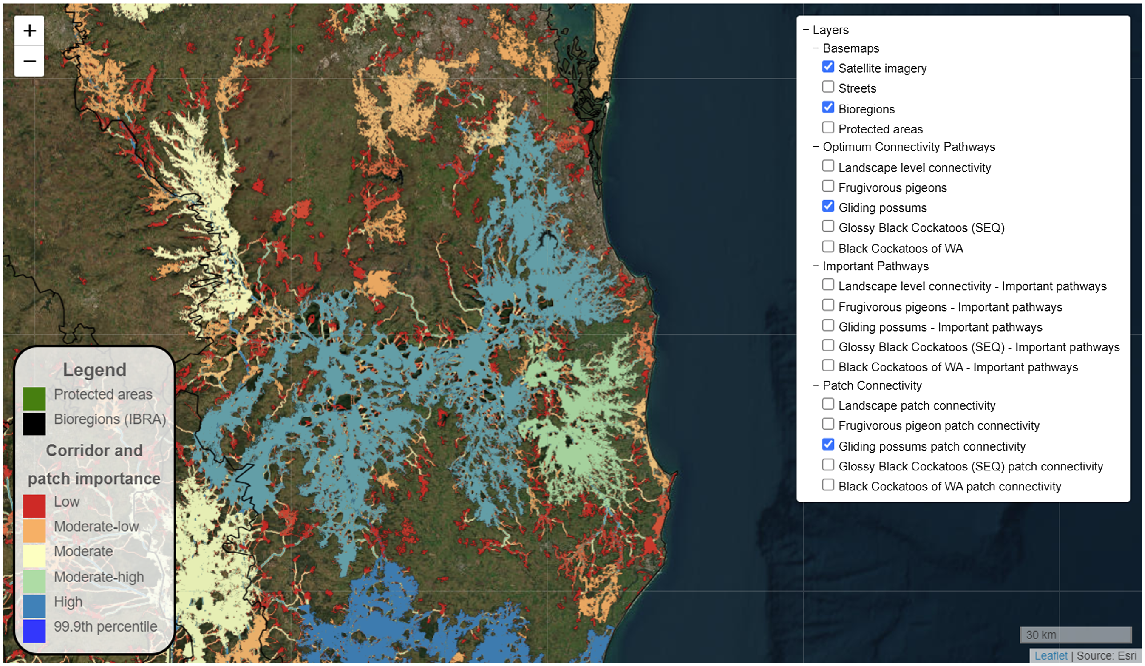
Discussion
Spatial information on habitat connectivity can support the work including conducting conservation assessment and planning, and on-ground conservation actions such as habitat restoration. Due to technical challenges however, this information is often difficult to generate and make available in ways that are relevant and useful to organisations, including community groups; who often have limited GIS capabilities. Our decision support tool provides access to spatial information that can be used for a wide range of connectivity conservation purposes including planning for wildlife movement pathways and prioritising ecological restoration efforts.
The decision support tool builds upon a foundation of research assessing habitat connectivity in south-western and eastern Australia (Barrett and Love 2012; Lechner et al. 2017; Liu et al. 2018). The work of Drielsma and colleagues (Drielsma et al. 2007, 2022) has been instrumental in shaping our methods, model parameters, and constraints. However, to avoid duplication and ensure the creation of targeted connectivity models for specific species and guilds, our study diverged from their approach by opting for randomised, forced start and end points weighted by habitat condition and patch size, rather than using a scaling window to assess habitat permeability. Our tool, similar to previous works, leverages landscape connectivity methods and incorporates updated habitat data and life history information to generate actionable insights for conservation planning, focusing on critical habitats and biodiversity corridors. Furthermore, its user-friendly interface and readily downloadable spatial data layers, present a low technical bar, allowing stakeholders with a wide range of competencies to access the model outputs, examine various conservation scenarios and evaluate their potential impacts, facilitating evidence-based decision making in wildlife habitat management.
Various studies assessing decision support tools for connectivity have utilised prebuilt software to inform decision makers (Volk et al. 2018), and connectivity mapping is available through interactive maps on a number of government websites in Australia (ACT Government 2012; Logan City Council 2019; Local Land Services 2023). Although these provide useful information for planning and mapping wildlife movement pathways, they are usually geographically constrained to a single local government area. Therefore, it is important that there is crossjurisdiction connectivity data and information that spans the broader distributional range of the target species available for conservation practitioners.
Connectivity modelling aims to produce a realistic spatial representation of a species or guild’s potential movement pathways between core habitat locations. This requires information on their habitat requirements for food, shelter and reproduction, home range size, and modes of movements, among other life history traits. Understanding the geographic locations of core source habitats and potential destinations of movements is an important first consideration that requires spatial data on native vegetation (here, the extant forest and woodland cover) and the species’ known or potential distributions. Obtaining spatial data on extant native vegetation cover is now a straightforward matter due to remotely sensed data sources that provide the ability to map and update land cover at a range of space/times scales (Gao et al. 2020; Lechner et al. 2020). Similarly, online biological databases (Atlas of Living Australia 2020; GBIF.org 2024) provide access to species distribution observations, and virtual laboratories such as EcoCommons (EcoCommons Australia 2022) provide the means to model potential and future species distributions. These data sources and tools enable connectivity modelling that is both of large extent and fine spatial resolution, such as the results presented here and made available through our web-based decision support tool. Furthermore, studies have found that potential habitat connectivity modelling can provide useful information for predicting species movements through landscapes (Braaker et al. 2014; Proctor et al. 2015; Correa Ayram et al. 2016).
Future directions
There are a number of potential future directions for our connectivity decision support tool. A user-friendly interface could be developed with streamlined user inputs including point and click menus providing seamless access to both spatial habitat data and life history information for a broad range of species. Furthermore, the functionality could be increased to enable users to upload their own data including species movement start and finishing points, and associated cost layers and ecological assumption parameters. This would make the platform more accessible and useful to a wider range of users, such as those in local governments and larger non-government organisations.
An additional future consideration is the integration of climate change dynamics into the tool’s modelling framework. As climate change continues to exert profound effects on habitats and species’ ranges, it is necessary to develop data and functions that can dynamically account for these changes in assessing wildlife habitat connectivity. Incorporating these into the tool will assist in identifying potential connectivity movement pathways for species whose distributions may shift in response to climate change as they migrate to find more suitable habitat locations (Mackey et al. 2008).
Limitations
Although modelling optimum wildlife connectivity pathways serves as a valuable initial step in recognising crucial habitat connections at a landscape level, the validation and refinement of these models require on-the-ground verification of where and how they are being used by the target species or guilds. On ground observations would help to verify, and modify where necessary, the model parameter values, taking into account factors such as microhabitat variations and local ecological nuances.
Data availability
The decision support tool, interactive maps and data is publicly available and can be found at www.stateofconnectivity.com. All associated processing scripts can be found in a Github repository (https://github.com/patrick-m-norman/HabitatConnectivityDecisionSupport/tree/main).
Declaration of funding
This research was supported by a grant from the Great Eastern Ranges Ltd and Gondwana Link Ltd.
References
Abbott I (1998) Conservation of the forest red-tailed black cockatoo, a hollow-dependent species, in the eucalypt forests of Western Australia. Forest Ecology and Management 109, 175-185.
| Crossref | Google Scholar |
ACT Government (2012) ACTmapi significant species, vegetation communities and registered trees map viewer. Available at https://app2.actmapi.act.gov.au/actmapi/index.html?viewer=ssvcrt [Accessed 7 February 2024]
Atlas of Living Australia (2020) Atlas of Living Australia. Available at http://www.ala.org.au [Accessed 5 May 2023]
Australian Government (2018) Australia’s state of the forests report 2018. Available at https://www.agriculture.gov.au/sites/default/files/abares/forestsaustralia/documents/sofr_2018/web%20accessible%20pdfs/SOFR_2018_web.pdf
Australian Government (2022) Collaborative Australian Protected Areas Database (CAPAD) 2022 – DCCEEW. Available at https://www.dcceew.gov.au/environment/land/nrs/science/capad/2022 [Accessed 30 November 2023]
Australian Government (2023) data.gov.au. Available at https://data.gov.au/data/ [Accessed 28 November 2023]
Bilney RJ, Kambouris PJ, Peterie J, Dunne C, Makeham K, Kavanagh RP, Gonsalves L, Law B (2022) Long-term monitoring of an endangered population of Yellow-bellied Glider Petaurus australis on the Bago Plateau, New South Wales, and its response to wildfires and timber harvesting in a changing climate. Australian Zoologist 42, 592-607.
| Crossref | Google Scholar |
Birdlife International (2023) Birdlife species range maps. Available at http://datazone.birdlife.org/species/requestdis
Braaker S, Moretti M, Boesch R, Ghazoul J, Obrist MK, Bontadina F (2014) Assessing habitat connectivity for ground-dwelling animals in an urban environment. Ecological Applications 24, 1583-1595.
| Crossref | Google Scholar | PubMed |
Brearley G, Bradley A, Bell S, McAlpine C (2011) Change in habitat resources and structure near urban edges and its influence on the squirrel glider (Petaurus norfolcensis) in southeast Queensland, Australia. Austral Ecology 36, 425-432.
| Crossref | Google Scholar |
Cassini MH (2011) Ecological principles of species distribution models: the habitat matching rule. Journal of Biogeography 38, 2057-2065.
| Crossref | Google Scholar |
Cleverly J, Eamus D, Edwards W, Grant M, Grundy MJ, Held A, Karan M, Lowe AJ, Prober SM, Sparrow B (2019) TERN, Australia’s land observatory: addressing the global challenge of forecasting ecosystem responses to climate variability and change. Environmental Research Letters 14, 095004.
| Crossref | Google Scholar |
Cork NA, Fisher RS, Strong N, Ferranti EJS, Quinn AD (2024) A systematic review of factors influencing habitat connectivity and biodiversity along road and rail routes in temperate zones. Frontiers in Environmental Science 12, 1369072.
| Crossref | Google Scholar |
Correa Ayram CA, Mendoza ME, Etter A, Salicrup DRP (2016) Habitat connectivity in biodiversity conservation: a review of recent studies and applications. Progress in Physical Geography: Earth and Environment 40, 7-37.
| Crossref | Google Scholar |
Dinerstein E, Joshi AR, Vynne C, Lee ATL, Pharand-Deschênes F, França M, Fernando S, Birch T, Burkart K, Asner GP, Olson D (2020) A “Global Safety Net” to reverse biodiversity loss and stabilize Earth’s climate. Science Advances 6, eabb2824.
| Crossref | Google Scholar |
Drielsma M, Ferrier S (2009) Rapid evaluation of metapopulation persistence in highly variegated landscapes. Biological Conservation 142, 529-540.
| Crossref | Google Scholar |
Drielsma M, Manion G, Ferrier S (2007) The spatial links tool: automated mapping of habitat linkages in variegated landscapes. Ecological Modelling 200, 403-411.
| Crossref | Google Scholar |
Drielsma MJ, Love J, Taylor S, Thapa R, Williams KJ (2022) General Landscape Connectivity Model (GLCM): a new way to map whole of landscape biodiversity functional connectivity for operational planning and reporting. Ecological Modelling 465, 109858.
| Crossref | Google Scholar |
EcoCommons Australia (2022) EcoCommons Australia – the platform of choice for ecological and environmental modeling. (EcoCommons, Griffith University: Nathan, Queensland) Available at https://data–explorer.app.ecocommons.org.au/ [Accessed 24 October 2023]
Edelsparre AH, Shahid A, Fitzpatrick MJ (2018) Habitat connectivity is determined by the scale of habitat loss and dispersal strategy. Ecology and Evolution 8, 5508-5514.
| Crossref | Google Scholar | PubMed |
Eyre TJ (2006) Regional habitat selection of large gliding possums at forest stand and landscape scales in southern Queensland, Australia: I. Greater glider (Petauroides volans). Forest Ecology and Management 235, 270-282.
| Crossref | Google Scholar |
FAO (2020) Global Forest Resources Assessment 2020: Terms and Definitions FRA 2020. Forest Resources Assessmnt Working Paper 188. (Food and Agricultural Organization of the United Nations) Available at https://www.fao.org/3/I8661EN/i8661en.pdf
Fischer J, Lindenmayer DB (2007) Landscape modification and habitat fragmentation: a synthesis. Global Ecology and Biogeography 16, 265-280.
| Crossref | Google Scholar |
Foster E, Love J, Rader R, Reid N, Drielsma MJ (2017) Integrating a generic focal species, metapopulation capacity, and connectivity to identify opportunities to link fragmented habitat. Landscape Ecology 32, 1837-1847.
| Crossref | Google Scholar |
Gao Y, Skutsch M, Paneque-Gálvez J, Ghilardi A (2020) Remote sensing of forest degradation: a review. Environmental Research Letters 15, 103001.
| Crossref | Google Scholar |
GBIF.org (2024) GBIF home page. Available at https://www.gbif.org/ [Accessed 7 February 2024]
GDAL/OGR (2024) GDAL/OGR geospatial data abstraction software Library. Open Source Geospatial Foundation. doi:10.5281/zenodo.5884351
Geosciences Australia (2023) Product catalogue. Available at https://ecat.ga.gov.au/geonetwork/srv/eng/catalog.search#/home [Accessed 28 November 2023]
Glossy Black Conservancy (2022) Glossy Black-Cockatoo Conservation Guidelines. Available at https://drive.google.com/file/d/1_HcJt1EPBgxVZQqZ7un_WvcghqpAepQR/view?pli=1
Google (2023) Earth engine data catalog. Google for Developers. Available at https://developers.google.com/earth-engine/datasets/catalog [Accessed 28 November 2023]
Gosper CR, Gosper DG (2008) Foods of pigeons and doves in fragmented landscapes of subtropical Eastern Australia. Australian Field Ornithology 25, 76-86.
| Google Scholar |
Grizonnet M, Michel J, Poughon V, Inglada J, Savinaud M, Cresson R (2017) Orfeo ToolBox: open source processing of remote sensing images. Open Geospatial Data, Software and Standards 2, 15.
| Crossref | Google Scholar |
Guisan A, Thuiller W (2005) Predicting species distribution: offering more than simple habitat models. Ecology Letters 8, 993-1009.
| Crossref | Google Scholar | PubMed |
Hansen MC, Potapov PV, Moore R, Hancher M, Turubanova SA, Tyukavina A, Thau D, Stehman SV, Goetz SJ, Loveland TR, Kommareddy A, Egorov A, Chini L, Justice CO, Townshend JRG (2013) High-resolution global maps of 21st-century forest cover change. Science 342, 850-853.
| Crossref | Google Scholar | PubMed |
Harwood TD, Donohue RJ, Williams KJ, Ferrier S, McVicar TR, Newell G, White M (2016) Habitat condition assessment system: a new way to assess the condition of natural habitats for terrestrial biodiversity across whole regions using remote sensing data. Methods in Ecology and Evolution 7, 1050-1059.
| Crossref | Google Scholar |
Hoekstra JM, Boucher TM, Ricketts TH, Roberts C (2005) Confronting a biome crisis: global disparities of habitat loss and protection. Ecology Letters 8, 23-29.
| Crossref | Google Scholar |
Innis GJ (1989) Feeding ecology of fruit pigeons in subtropical rainforests of South-Eastern Queensland. Wildlife Research 16, 365-394.
| Crossref | Google Scholar |
Keeley ATH, Basson G, Cameron DR, Heller NE, Huber PR, Schloss CA, Thorne JH, Merenlender AM (2018) Making habitat connectivity a reality. Conservation Biology 32, 1221-1232.
| Crossref | Google Scholar | PubMed |
Keeley ATH, Beier P, Jenness JS (2021) Connectivity metrics for conservation planning and monitoring. Biological Conservation 255, 109008.
| Crossref | Google Scholar |
Klein C, Wilson K, Watts M, Stein J, Berry S, Carwardine J, Smith MS, Mackey B, Possingham H (2009) Incorporating ecological and evolutionary processes into continental-scale conservation planning. Ecological Applications 19, 206-217.
| Crossref | Google Scholar | PubMed |
Lechner AM, Sprod D, Carter O, Lefroy EC (2017) Characterising landscape connectivity for conservation planning using a dispersal guild approach. Landscape Ecology 32, 99-113.
| Crossref | Google Scholar |
Lechner AM, Foody GM, Boyd DS (2020) Applications in remote sensing to forest ecology and management. One Earth 2, 405-412.
| Crossref | Google Scholar |
Lee JGH, Finn HC, Calver MC (2013) Ecology of black cockatoos at a mine-site in the eastern jarrah-marri forest, Western Australia. Pacific Conservation Biology 19, 76.
| Crossref | Google Scholar |
Lindenmayer DB, Blanchard W, Blair D, McBurney L, Taylor C, Scheele BC, Westgate MJ, Robinson N, Foster C (2021) The response of arboreal marsupials to long-term changes in forest disturbance. Animal Conservation 24, 246-258.
| Crossref | Google Scholar |
Lindsay JB (2016) Whitebox GAT: a case study in geomorphometric analysis. Computers & Geosciences 95, 75-84.
| Crossref | Google Scholar |
Liu Z, He C, Wu J (2016) The relationship between habitat loss and fragmentation during urbanization: an empirical evaluation from 16 World Cities. PLoS ONE 11, e0154613.
| Crossref | Google Scholar |
Liu C, Newell G, White M, Bennett AF (2018) Identifying wildlife corridors for the restoration of regional habitat connectivity: a multispecies approach and comparison of resistance surfaces. PLoS ONE 13, e0206071.
| Crossref | Google Scholar | PubMed |
Local Land Services (2023) Interactive web-mapping apps. Available at https://www.lls.nsw.gov.au/regions/greater-sydney/key-projects/interactive-web-mapping-apps [Accessed 28 November 2023]
Logan City Council (2019) Koala corridor. Available at https://data-logancity.opendata.arcgis.com/maps/koala-corridor/explore [Accessed 6 February 2023]
Mackey BG, Watson JEM, Hope G, Gilmore S (2008) Climate change, biodiversity conservation, and the role of protected areas: an Australian perspective. Biodiversity 9, 11-18.
| Crossref | Google Scholar |
McDonald RI, Kareiva P, Forman RTT (2008) The implications of current and future urbanization for global protected areas and biodiversity conservation. Biological Conservation 141, 1695-1703.
| Crossref | Google Scholar |
Norman P, Mackey B (2023) Priority areas for conserving greater gliders in Queensland, Australia. Pacific Conservation Biology 30, PC23018.
| Crossref | Google Scholar |
NSW Government (2023) SEED Portal. Available at https://www.seed.nsw.gov.au/ [Accessed 28 November 2023]
Nuñez TA, Lawler JJ, McRae BH, Pierce DJ, Krosby MB, Kavanagh DM, Singleton PH, Tewksbury JJ (2013) Connectivity planning to address climate change. Conservation Biology 27, 407-416.
| Crossref | Google Scholar | PubMed |
OpenStreetMap Contributors (2023) Planet dump. Available at https://planet.osm.org
Pebesma E (2018) Simple features for R: standardized support for spatial vector data. The R Journal 10, 439-446.
| Crossref | Google Scholar |
PostGIS Project Steering Committee and Others (2022) PostGIS, spatial and geographic objects for postgreSQL. Available at https://postgis.net
Price OF (2006) Movements of frugivorous birds among fragmented rainforests in the Northern Territory, Australia. Wildlife Research 33, 521-528.
| Crossref | Google Scholar |
Proctor MF, Nielsen SE, Kasworm WF, Servheen C, Radandt TG, Machutchon AG, Boyce MS (2015) Grizzly bear connectivity mapping in the Canada–United States trans-border region. The Journal of Wildlife Management 79, 544-558.
| Crossref | Google Scholar |
QGIS Development Team (2023) QGIS geographic information system. Available at https://www.qgis.org
Qld Government (2023) Queensland spatial catalogue. Available at https://qldspatial.information.qld.gov.au/catalogue/custom/index.page [Accessed 28 November 2023]
Rycken SJE, Warren KS, Yeap L, Donaldson R, Mawson P, Dawson R, Shephard JM (2022) Forest specialist species in the urban landscape: do different levels of urbanization affect the movements of Forest Red-tailed Black Cockatoos (Calyptorhynchus banksii naso)? Avian Conservation and Ecology 17, 11.
| Crossref | Google Scholar |
Rycken S, Warren KS, Yeap L, Jackson B, Mawson PR, Dawson R, Shephard JM (2023) Movement of Carnaby’s Cockatoo (Zanda latirostris) across different agricultural regions in Western Australia. Pacific Conservation Biology 30, PC23015.
| Crossref | Google Scholar |
Saura S, Pascua-Hortal L (2007) A new habitat availability index to integrate connectivity in landscape conservation planning: comparison with existing indices and application to a case study. Landscape and Urban Planning 83, 91-103.
| Crossref | Google Scholar |
Soulé ME, Mackey BG, Recher HF, Williams JE, Woinarski JCZ, Driscoll D, Dennison WC, Jones ME (2004) The role of connectivity in Australian conservation. Pacific Conservation Biology 10, 266-279.
| Crossref | Google Scholar |
Sullivan BL, Aycrigg JL, Barry JH, Bonney RE, Bruns N, Cooper CB, Damoulas T, Dhondt AA, Dietterich T, Farnsworth A, Fink D, Fitzpatrick JW, Fredericks T, Gerbracht J, Gomes C, Hochachka WM, Iliff MJ, Lagoze C, La Sorte FA, Merrifield M, Morris W, Phillips TB, Reynolds M, Rodewald AD, Rosenberg KV, Trautmann NM, Wiggins A, Winkler DW, Wong W-K, Wood CL, Yu J, Kelling S (2014) The eBird enterprise: an integrated approach to development and application of citizen science. Biological Conservation 169, 31-40.
| Crossref | Google Scholar |
Tas Government (2023) The list data. Available at https://www.thelist.tas.gov.au/app/content/data/index [Accessed 28 November 2023]
Vic Government (2023) Data Vic. Available at http://www.data.vic.gov.au/ [Accessed 28 November 2023]
Vogt P, Riitters K (2017) GuidosToolbox: universal digital image object analysis. European Journal of Remote Sensing 50, 352-361.
| Crossref | Google Scholar |
Volk XK, Gattringer JP, Otte A, Harvolk-Schöning S (2018) Connectivity analysis as a tool for assessing restoration success. Landscape Ecology 33, 371-387.
| Crossref | Google Scholar |
WA Government (2023) Data WA. Available at https://www.data.wa.gov.au/ [Accessed 28 November 2023]
World Resources Institute (2023) Global forest watch open data portal. Available at https://data.globalforestwatch.org/ [Accessed 28 November 2023]