Spatial resolution of shell microchemistry for tracking dispersing pelagic mussels in a large open embayment in northern New Zealand
Wenjie Wu
A
B
C
D
Abstract
The effectiveness of using shell microchemistry methods for reconstructing the pelagic dispersal of shell-forming marine organisms relies on the existence of sufficient spatial variability and temporal stability in the microchemical composition of coastal waters.
This study aimed to determine whether shell microchemistry methods can reliably infer pelagic dispersal of green-lipped mussel (Perna canaliculus) across a large and well-mixed embayment in New Zealand.
Isotopes of 12 chemical elements (7Li, 27Al, 59Co, 60Ni, 63Cu, 66Zn, 88Sr, 138Ba, 139La, 140Ce, 208Pb and 238U) were assessed from juvenile mussel shells grown in situ from 22 sites for two consecutive periods of ~5 weeks.
There was sufficient spatial variability in measured shell element concentrations to support microchemical atlases that are capable of distinguishing among regions within this large embayment, with 73% assignment accuracy. However, the temporal variability in the microchemical signatures is such that they would need to be sampled contemporaneously with animal samples to be effective for distinguishing pelagic dispersal of mussels in the embayment.
The shell microchemistry shows sufficient spatio-temporal resolution for reliably inferring pelagic dispersal of this economically and ecologically important mussel species over a large embayment. Sufficient resolution at such large scale may come from unique hydro-geological inputs.
This study confirms the feasibility of shell microchemistry method in tracking pelagic dispersal of marine organisms across a large well-mixed embayment, offering insights to improve accuracy and reduce costs for future research.
Keywords: coastal-water chemistry, estuaries, freshwater inflows, green-lipped mussel, LA-ICP-MS, larval mussel dispersal, Perna canaliculus, shell microchemistry.
Introduction
The geographic origin and pathways of dispersal for the pelagic stages of sessile marine species are vitally important for understanding their population ecology and providing critical insights to assist in managing wild populations, such as those that support fisheries and aquaculture. However, for the pelagic dispersal stage of many marine species, it is difficult to determine larval pathways because of highly changeable ocean hydrodynamics (Cowen and Sponaugle 2009), varied behaviour of planktonic stages, and the complex interactions between these two factors (Cowen and Sponaugle 2009; Pineda and Reyns 2018). The difficulty in determining the pathways of the pelagic dispersal stage is a significant issue for some aquaculture industries that rely on the harvesting of wild seed, and for fisheries managers attempting to manage metapopulations that are spread over large areas of coast (Watson et al. 2011). Understanding dispersal pathways over large areas can inform the timing and best locations for harvesting wild pelagic larvae or juveniles (i.e. seeds) and also assist in identifying important natal wild adult populations that may need to be protected to secure ongoing seed supply or to maintain genetic heterogeneity in widely dispersed metapopulation of seafood species that are extensively harvested. Wild seed or spat is the dominant source of seed supplying global mussel aquaculture, which produced over 2 million tonnes (Mg) in 2020 (Alfaro et al. 2010; Kamermans et al. 2013; Carrasco et al. 2014; Avdelas et al. 2021; South et al. 2021; Food and Agriculture Organization of the United Nations 2022). For example, New Zealand produces ~100,000 Mg of Greenshell mussels a year, with more than 90% of production derived from spat harvested from the wild from either spat-catching ropes deployed into coastal areas (10% of spat supply) or harvesting of beach-cast seaweed and other debris that is encrusted with millions of spat (80%), a phenomenon that occurs at only one location in northern New Zealand (Jeffs et al. 1999, 2018; Alfaro et al. 2004; Skelton et al. 2021). However, the effective use of wild mussel spat resources, especially for the collection of pelagic mussels that settle on spat-catching ropes, requires knowledge of the timing of mussel reproduction and patterns of dispersal in coastal waters to help ensure the effective timing and placement of spat-collecting ropes.
Besides informing wild mussel seed harvesting for aquaculture, an improved understanding of the pelagic dispersal phase in the mussel life cycle would also contribute to our understanding of population connectivity, which is fundamental to the population dynamics of wild mussel populations (Caley et al. 1996; Crowder et al. 2000). Knowledge of patterns of pelagic dispersal may also be useful for supporting restoration efforts for wild mussel populations (Norrie et al. 2020) and help in our understanding of the effect of global warming, marine heat waves and other extreme climatic phenomena on wild mussel populations (Herbert and Saunders 2018; Suchley and Alvarez-Filip 2018).
Shell microchemistry is increasingly being used as a tool for reconstructing the pathways for the pelagic dispersal stages of shell-forming marine organisms in coastal waters, especially the larvae of bivalves, such as mussels. This method relies on detecting serial changes in microquantities of a suite of metals that are sequentially incorporated during the continual formation of the calcareous shell of a pelagic stage in the lifecycle. Chemical signatures can be used to infer serial locations in the ocean on the basis of a reference atlas derived from simultaneous sampling of organisms held at sites from across the potential range of the pelagic dispersal stage (Levin 2006; Thorrold et al. 2007; Gillanders 2009). Although generally effective, the success of this method, especially its spatial resolution, relies heavily on the following two features of the ambient environmental chemical conditions of the ocean system from which samples are drawn: (1) sufficient spatial differences in environmental chemical characteristics; and (2) the temporal stability of those environmental chemical characteristics (Zacherl 2005; Gillanders 2009).
Typically, if the environmental conditions are more evenly distributed or homogeneous, any differences in the trace-element signatures of the environment can be distinguished only between widely separated sites, whereas sites in geographically close proximity will have less difference in their signature trace elements. Alternately, more complex environmental conditions owing to the variations in coastal geology, mesoscale oceanography and elemental inputs from localised watersheds will tend to provide more distinct spatial variability in trace-elemental signatures (Thorrold et al. 2007; Carson et al. 2013; Miller et al. 2013). For example, enclosed estuaries that have continual freshwater inputs carrying dissolved metal ions tend to have high spatial variation in trace-elemental signatures, whereas the spatial variation in trace elements is less pronounced for open coastlines with well mixed waters (Gillanders et al. 2001; Dunphy et al. 2011). Consequently, studies using shell microchemistry on mussel shells have confirmed much higher spatial resolution for reliably determining source locations in enclosed waters v. adjacent open coastal locations (Becker et al. 2005; Dunphy et al. 2011; Norrie et al. 2020). For example, the source location of green-lipped mussels in enclosed waters in New Zealand could be discriminated between sites only 1.2 km apart with a 90.4% re-classification success, whereas the spatial resolution in more open coastal sites decreased, to over 20 km (71.2–100%) (Norrie et al. 2020). Given the apparent differences in the extent of spatial variation in trace-elemental signatures among different coastal areas (i.e. open coast v. estuaries), it is important to assess the extent of this variability to determine the sampling design and potential resolution available for planning studies aimed at tracking the pelagic pathways of shelled organisms, such as pelagic mussel stages (Gillanders et al. 2001; Becker et al. 2005; Zacherl 2005; Niemand 2009).
Temporal variation of trace-elemental signatures at different coastal locations is also a potentially confounding factor that needs to be characterised before effectively applying microchemistry methods for tracing pelagic dispersal of shell-forming organisms (Becker et al. 2005; Zacherl 2005; Fodrie et al. 2011). Trace-elemental signatures of coastal waters can remain stable for only a few weeks (Becker et al. 2005; Cook 2011; Fodrie et al. 2011) or, alternatively, for up to several months (Gillanders 2002; Dunphy et al. 2011; Cathey et al. 2014; Bennion et al. 2019; Norrie et al. 2020), depending largely on the temporal variability of environmental conditions. Therefore, before applying microchemistry methods to tracking pelagic pathways for shelled organisms, such as pelagic mussel stages, it is useful to also identify the extent of temporal variability to assist in determining the sampling design and temporal validity of trace-element reference atlases that are used to assign locations to sampled pelagic stages.
The establishment of a large offshore green-lipped mussel farm (2500 ha when fully developed), located 8.5 km off the coast in the Bay of Plenty (BoP) in north-eastern New Zealand, has generated a need for a better understanding of the natal sources and pelagic pathways of the mussels within this coastal region that could support spat collection and seeding in the mussel farm. Consequently, this study assessed the spatial and temporal variability in the trace elements by culturing juvenile green-lipped mussels at 22 sites covering 110 km of coastline in the BoP, over two consecutive ~5-week periods. Using the trace-element data from these mussels sampled across this large embayment, a series of microchemical reference models at different spatial and temporal scales were developed and compared to determine the effective spatial and temporal resolution that is available for assessing natal sources and pathways of pelagic mussels settling onto the offshore mussel farm and for examining the capacity for genetic exchange among coastal populations that are vulnerable to overharvesting (Toone et al. 2023). Previously, we used hydrodynamic modelling of patterns of larval dispersal to validate the use of shell microchemistry of the shells of mussel settling on an offshore mussel farm in the BoP to locate their natal source (Wu et al. 2024). In this study, we more closely examine the spatial and temporal variability of the full set of microchemistry reference data set from across the BoP to better understand the potential resolution of using shell microchemistry for determining pelagic dispersal pathways.
Materials and methods
Study site
This study was undertaken in the eastern BoP, a large open-coastal embayment in north-eastern New Zealand that extends over 190 km at its entrance and covers 9.6 × 103 km of coastal waters (Ridgway and Greig 1986) (Fig. 1). Sampling occurred over ~110 km of coastline at depths to ~50 m. The bay exhibits dynamic physical characteristics and coastal circulation owing to its bathymetry, shelf width and coastline orientation. The bathymetry of the BoP consists of a shallow gradually sloping seafloor, extending to the edge of the continental shelf (i.e. 200-m depth), and further deepening to the entrance of the bay (exceeding 1000-m depth). At the northern offshore boundary of BoP, the dominant East Auckland Current (EAUC) brings warm, high-salinity waters southward along the coastline from North Cape (Ridgway and Greig 1986). At the centre of the bay, the influence of the EAUC is reduced (Longdill 2008; Montaño et al. 2023), and circulation is dominated by an Ekman upwelling-driven circulation system powered by local winds at speeds up to a maximum of 0.25 m s−1. The prevailing south-westerly winds of BoP have higher wind strengths during periods of austral autumn–winter than the summer period. The south-westerly wind flows tend to generate north-easterly surface currents in the embayment (Longdill 2008).
Map of the BoP, New Zealand, showing 17 coastal sampling sites (S1–S17, Sites 1–17) at ~6 km spacings parallel to the coastline, three sampling sites (WF, west farm; MF, mid-farm; EF, east farm) on an offshore Greenshell mussel farm and two sampling sites at the commercial mussel seed-collection area (WSA, west spat-collection area; ESA, east spat-collection area). Major rivers emptying into the BoP and geothermal regions that contain active volcanoes and potential submarine geothermal vents are identified.
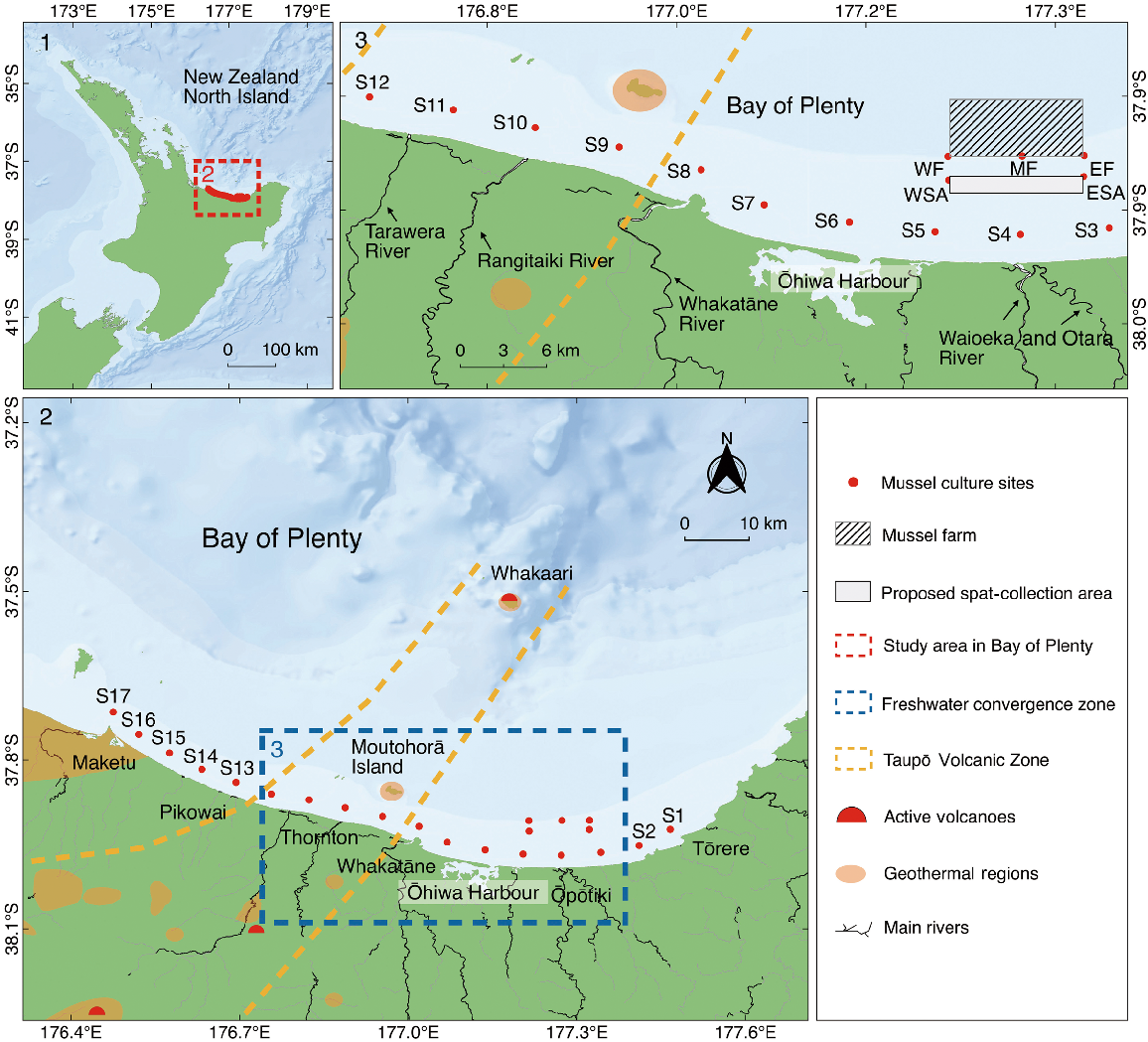
The trace-metal chemistry of the coastal waters of the BoP is influenced by a convergence of freshwater inflows into small estuaries, with three individual rivers (i.e. Tarawera River, Rangitaiki River and Whakatāne River) and one combined river system (i.e. Waioeka and Otara Rivers) that all have an average flow of more than 10 m3 s−1 (Longdill 2008) (Fig. 1). The convergence of potentially large freshwater inputs and terrestrial sediment elements from these rivers, as well as from rainfall and associated runoff (e.g. storm-water drainage), is likely to cause spatial variation in the trace-element composition of the environment in this area compared with the surrounding open coast. In addition, the western side of this freshwater convergence area is also influenced by the extremely active Taupō Volcanic Zone (TVZ) which extends north-eastward from Mount Ruapehu through the Taupō and Rotorua areas and ~50 km offshore into the BoP towards Whakaari (White Island) (Fig. 1) (Pantin and Wright 1994; Saunders 2018; Kilgour et al. 2021). The TVZ is a tectonic–volcanic structure ~350 km long and 50 km wide, and the young TVZ boundary is thought to intersect the coastline within the area ~25 km to the west of Whakatāne and east of Pikowai (Fig. 1) (Stoffers et al. 1999; Hocking et al. 2010; Wilson and Rowland 2016). Active submarine hydrothermal venting has been suggested to occur within the offshore part of the TVZ, between Moutohorā Island near the coast and Whakaari, which itself is an active volcano (Pantin and Wright 1994; Saunders 2018). Active geothermal activity, visible as large bubble plumes from the nearshore shallow-water vents (<30-m depth) located at the Moutohorā Island region (Lyons et al. 1977; Stoffers et al. 1999), is also likely to discharge trace elements directly into the ocean, resulting in further spatial variability in the elemental signatures in the seawater in this specific area (Pantin and Wright 1994; Stoffers et al. 1999; Saunders 2018). Overall, the BoP has a diverse range of coastal topographical features, including open coast, geothermal regions and freshwater inputs, which interact with the complex nearshore seawater circulation. Thus, temporal and spatial variability in the microchemical signatures of seawater across the BoP are likely to result in detectable differences in the trace-elemental chemistry of pelagic mussels that disperse in the region.
Sampling of shell material
The shell microchemistry features of green-lipped mussels that were cultured in situ across the BoP were sampled at each of 17 sites located at ~6-km intervals along the BoP coastline and ~2.5 km offshore (Fig. 1). In addition, five sites were sampled at an offshore mussel farm and the proposed mussel seed-collection site. Two consecutive 5-week sampling periods (Retrieval #1: 11 November 2020–16 December 2020 and Retrieval #2: 16 December 2020–16 January 2021) corresponded with the natural spawning season of mussels and local spatfall records (Alfaro et al. 2003, 2010; Heasman and Knight 2014). At the start of each of the two sampling periods, ~75 live green-lipped mussel plantigrades (1.5–2.0 mm in shell length) from the same larval rearing cohort from a hatchery (SPATnz Ltd) were placed inside a small nylon-mesh bag (40 × 20 cm, 1.5-mm mesh), sealed with a cable tie and deployed at each of the sampling sites. Two mesh bags containing mussels were deployed 7 m from the seafloor at each of the 17 coastal sites (Sites 1–17) and at the proposed areas for commercial mussel-seed collection (west spat-collection area, WSA; and east spat-collection area, ESA) (Fig. 1). At the remaining three sampling sites on the mussel farm (west farm, WF; mid-farm, MF; and east farm, EF), a single mesh bag of mussels was deployed on existing mooring lines at 6–10 m below the surface. Any contact of sampling equipment with metallic instruments and materials was deliberately avoided throughout the deployment and recovery of samples to minimise the effect of any metallic elements on the results of further microchemical analysis. At the end of each sampling period, each reference spat culture or spat-collection rope was recovered and placed in a separate plastic zip-lock bag and immediately stored in a freezer (−20°C) for later analysis. The nearshore sampling site was chosen 2.5 km offshore because one previous observation in the BoP suggested that the green-lipped mussel spat settled on mooring lines that extended from the surface to the seabed at 28-m depth (Heasman and Knight 2014). A distance of 2.5 km offshore of the study coastline was selected for the sampling because it corresponded to the depth range (i.e. ~0–28 m) of previous observations of mussel-spat settlement in the BoP. Additionally, because prior studies indicated higher spat abundance of this species of mussel at a depth of ~15 m than in shallower areas (5-m water depth) (Hayden and Kendrick 1992), a fixed value for the distance of ropes to the seafloor (7 m) was adopted, ensuring that the sampling rope was positioned at an average depth of ~15 m to maximise mussel-spat capture.
Shell-sample processing and microchemistry analyses
Mussels were recovered from the mesh bags at each sampling site for each sampling period, and 10 mussels were randomly selected. The recovered mussels were split open and the flesh was removed from the shells with carbon-fibre tweezers. One valve from each mussel was randomly selected and washed with Milli-Q water three times to remove any remaining soft tissues, and dried. The outer margin of each valve was separated from each shell by carbon-fibre tweezers and then attached to a gridded plastic microscope slide by double-sided Sellotape ready for mounting in laser ablation–inductively coupled plasma–mass spectrometry (LA-ICP-MS). One 100-μm spot located ~400 μm from the shell margin of each cultured mussel were laser ablated by using a one-wave deep-ultraviolet (193 nm) laser-ablation system (RESOlution-SE 193-nm laser ablation, Applied Spectra Inc.). To minimise the possible confounding impact of the presence of the remaining periostracum (Bennion et al. 2019) and other possible surface contamination or soft tissue after water rinsing, a 5-s pre-ablation procedure was implemented before the 55 s of sampling ablation to remove the periostracum layer and any contaminants on the outside of the mussel shell. This pre-ablation technique has been shown to be effective by previous studies on the same mussel species (Norrie et al. 2018, 2019, 2020). For each LA-ICP-MS sample, the peak calcium reads were identified, and the measurement period was confined to the 5 s before and after the peak calcium read within the 55 s of sampling ablation commencing. Given the varied thickness and three-dimensional structures of carbonate in the shell (Strasser et al. 2007), the data-selection method based on the peak calcium can help reduce the bias from varying shell thickness, and the potential for laser burning through the full extent of the shell. Calibration standards (NIST610 and NIST612) were measured to standardise and calibrate the results of element concentration at 20 sample intervals. Elemental composition was standardised to molar ratios relative to calcium to determine the relative concentration for each element. However, because the most common calcium isotope, 40Ca (40.078), has an atomic mass that is close to the argon carrier gas (39.948) used in ICP-MS (Peacock et al. 2007), the molar ratios of elements relative to 43Ca were thus measured as a substitute to represent the standardised relative concentration of each element, and 43Ca was used to correct for drift in elemental abundances as an internal standard in this study. Elemental measurements in LA-ICP-MS were background-corrected by subtracting mean background counts from the ablation counts. The processing of the samples was randomised to reduce the influence of machine drift. The elemental concentrations that recorded zero or negative values were considered to be below the detection limits of the instrument. To include the individual samples with low isolated elemental concentrations in the analysis to the maximum extent possible, an interpolation algorithm was applied to replace all the recorded zero or negative values with half of the minimum value of the element recorded from all shells cultured at all sampling sites (Dunphy et al. 2015). In all, 11 of 397 samples had a 27Al element reads and only one sample had a detected 66Zn reads below the detection limits of the instrument. To improve normality for further statistical analysis, a ln(x + 1) transformation was also applied on all interpolated relative concentrations of each element. All LA-ICP-MS data reduction and standardisation was performed using IOLITE 4 software (ver. 4.7.1, see https://iolite.xyz/docs/index.html; Paton et al. 2011).
Statistical analyses
To display the overall spatial and temporal variation of microchemistry within the BoP, the mean concentration for each of the 12 elements measured in the 10 mussel shells from each sampling site for both Retrieval #1 and #2 was calculated. The mean shell element concentration for each site for each of the two sampling periods was then used to fit interpolation models for predicting the element concentration for a coastal area that is ~120 km long and 15 km wide off the coastline and contains all the sampling sites in this study. The model was interpolated to a grid resolution of 550 × 440 m horizontally (6300 total grid points) within each sampling period. The function ‘gstat’ from the ‘gstat’ package (ver. 2.1-2, see https://cran.r-project.org/web/packages/gstat/index.html; Pebesma 2004; Gräler et al. 2016) in R (ver. 4.1.2, R Foundation for Statistical Computing, Vienna, Austria, see https://www.r-project.org; Ihaka and Gentleman 1996) through integrated development environment (IDE): RStudio (ver. 1.4.1717, Posit Software, PBC, Boston, MA, USA, see https://posit.co/products/open-source/rstudio/; Racine 2012) was used to fit the model using Inverse Distance Weighted (IDW) interpolation method (inverse distance power = 1) for each sampling period. The function ‘interpolate’ from the ‘raster’ package (ver. 3.6-23, see https://CRAN.R-project.org/package=raster) was then used to generate the interpolated values and the subsequent raster layer according to the fitted model.
To group the sampling sites by mussel shells with similar elemental composition, a hierarchical cluster analysis (‘Euclidean’ distance matrix, ‘Average’ clustering method) was applied to the mean concentration of elements in the 10 mussel shells at each site for each sampling period (Retrieval #1 and Retrieval #2) and for the combined samples (Retrieval #1 + #2) independently, by using ‘dist’ and ‘hclust’ function in ‘stats’ package (ver. 4.1.2, see https://stat.ethz.ch/R-manual/R-devel/library/stats/html/00Index.html; Ihaka and Gentleman 1996). For each selected length of sampling period (Retrieval #1, Retrieval #2 and Retrieval #1 + #2), a Rand index (RI) (Rand 1971) was calculated by ‘rand.index’ in ‘fossil’ package (ver. 0.4.0, see https://cran.r-project.org/web/packages/fossil/index.html; Vavrek 2011) to evaluate the similarity between the clustering of sites classified by shell microchemistry and the grouping of sites classified on the basis of the proximity of geographic location. The RI has a value between 0 and 1; values closer to 1 imply greater similarity between two clustering results. Geographic locations were clustered on the basis of similar environmental conditions (e.g. sea-surface temperature; chlorophyll-a, Chl-a) (Longdill 2008), and being separated or distinguished by segments of different main streams and rivers (i.e. Tarawera River, Rangitaiki River, Whakatāne River, and Waioeka and Otara River system with mean flow volumes of >10−3 s−1) (Longdill 2008), and submarine geothermal activity (Pantin and Wright 1994; Saunders 2018) (Fig. 1).
The BoP sampling sites were divided into five wider regions on the basis of these environmental and geographic features: (1) Ōpōtiki region (OP): the open coast region on the eastern side of BoP (Sites 1–4); (2) Ōhiwa region (OH): the freshwater convergence region with no submarine geothermal activity (Sites 5–7); (3) Thornton region (TH): the freshwater convergence region with active submarine geothermal activity (Sites 8–12), (4) Pikowai region (PI): the open coast region on the western side of BoP (Sites 13–17); and (5) farm region (FA): all of the offshore site within offshore mussel farm and seed-collection area (WF, MF, EF, WSA and ESA).
The microchemistry data for each sampling site and each sampling period were directly used or combined into each of the corresponding five wider regions (OP, OH, TH, PI and FA) and the entire study area in BoP to arrange the overall microchemistry data at three spatial scales, i.e. among sampling sites (~2–6 km), among regional areas (~12–24 km), and for the entire study area scale (~110 km). The temporal stability of the elemental composition of mussel shells in the BoP was compared at each of three spatial scales. For each of the scales, a permutational multivariate ANOVA (PERMANOVA) was applied, because assumptions for normality and homogeneity of variance were not satisfied. To examine the temporal stability at the sampling-site scale (~2–6 km), for each of the 22 sampling sites, a PERMANOVA test was implemented for the differences between Retrieval #1 and Retrieval #2 in the 12 measured concentrations of elements in the shells of mussels cultured in situ. Similarly, to test the temporal stability at the regional scale (~12–24 km), for each of the five wider regions, the concentrations of the 12 elements in mussel shells within Retrieval #1 were compared with those from Retrieval #2 with a PERMANOVA. Last, the overall temporal stability of the microchemistry of all the mussels collected within the entire BoP scale (~110 km) was tested by another PERMANOVA test.
Three additional PERMANOVAs and corresponding post hoc pairwise tests with Bonferroni adjustment were performed to study the spatial variability of microchemical signatures within the BoP for the following three temporal periods: (1) during Retrieval #1 (11 November 2020–16 December 2020); (2) during Retrieval #2 (16 December 2020–16 January 2021), and (3) during Retrieval #1 + #2 combined (11 November 2020–16 January 2021). Where a PERMANOVA was significant, post hoc pairwise tests with Bonferroni adjustment were used to identify similarities in shell microchemistry between each sampling site pair for the three different sampling-period iterations (Retrieval #1, Retrieval #2 and Retrieval #1 + #2). Three additional PERMANOVAs and post hoc tests were performed to examine regional-scale (i.e. sites grouped into OP, OH, TH, PI and FA) spatial variability in microchemical signatures within these three sampling periods (Retrieval #1, Retrieval #2 and Retrieval #1 + #2). The resemblance matrix for the PERMANOVA tests was based on Euclidean distance dissimilarities by using ‘adonis2’ function in ‘vegan’ package (ver. 2.6-4, see https://cran.r-project.org/package=vegan/; Dixon 2003), and the number of permutations was 999. The post hoc pairwise test used the function ‘pairwise.adonis2’ in ‘pairwiseAdonis’ package (ver. 0.4.1, see https://github.com/pmartinezarbizu/pairwiseAdonis) in R. A series of non-metric multidimensional scaling (NMDS) ordinations based on Euclidean distances were calculated and 3-D-plotted to show the spatial variation in mussel-shell microchemistry features for the three different sampling-period iterations (Retrieval #1, Retrieval #2 and Retrieval #1 + #2) by using ‘metaMDS’ function from package ‘vegan’ (ver. 2.6-4). The 3-D NMDS graph was plotted by ‘rgl’ package (ver. 1.2.1, see https://CRAN.R-project.org/package=rgl; Adler et al. 2003).
Because assumptions of data normality and homogeneity of variance were not satisfied, quadratic discriminant analysis (QDA) (McGarigal et al. 2000) was applied to different subsets of the reference mussel-shell microchemistry data to assess the differences in the performance of methods for establishing a shell microchemistry atlas. The first QDA model (hereafter QDA-S-All) derived a combined microchemical reference atlas by using data from the microchemical composition of all mussel shells sampled in both Retrieval #1 and Retrieval #2, with a ln(x + 1) transformation being used to improve the normality of the data (Darroch and Mosimann 1985). A stepwise variable selection process was implemented for the model to select the optimum subset of elements that contributed most effectively to the classification. The performance of the model was evaluated by a leave-one-out cross-validation (LOOCV) method (Mundry and Sommer 2007), in which each of the samples was left out in turn. A subQDA model was then established on the basis of the remaining samples, which was used to classify the left-out sample (Mundry and Sommer 2007). This process was repeated until each sample had been left out and classified. The proportion of correctly classified samples at each site and across the entire study was calculated as the site-specific LOOCV correctness rate and the overall LOOCV correctness rate respectively. To evaluate the sensitivity of the QDA model, the multiclass receiver operating characteristic (ROC) curves were generated by using the functions ‘multiclass.roc’ from the ‘pROC’ package (ver. 1.18.4, see https://cran.r-project.org/package=pROC; Robin et al. 2011) in R. The function ‘qda’ from the ‘MASS’ package (ver. 7.3-60, see https://CRAN.R-project.org/package=MASS; Venables and Ripley 2002) in statistical software R was used to accommodate differing covariances and establish the QDA model.
To evaluate the influence of lost samples in four sites during Retrieval #1, a second QDA model (QDA-S-Lost) was established using data from 18 sites (excluding Sites 4, 10, 11, 12 that had missing data from Retrieval #1). Data were analysed using the same stepwise QDA and LOOCV and multiclass ROC to test the performance and sensitivity.
To test the influence of temporal variability across retrieval periods on classification success, a third QDA model (QDA-S-Ret1) and fourth QDA model (QDA-S-Ret2) were constructed with only mussel shells sampled in Retrieval #1 or Retrieval #2 respectively. Models QDA-S-Ret1 and QDA-S-Ret2 were first analysed using the same LOOCV procedures mentioned above. A further cross-validation method was used, in which the samples in Retrieval #2 were assigned back to predicted sources by the QDA-S-Ret1 models established by Retrieval #1 reference shell microchemistry data, and vice versa. The proportion of samples that correctly assigned back to their cultured locations was calculated as the correctness rate of reclassification for each sampling site and for the entire BoP.
A final model (QDA-S-Half) tested whether increasing the sample size could improve the statistical power of discriminant analysis and the likelihood of detecting and removing true outliers (White and Ruttenberg 2007; Niemand 2009; Dechaume-Moncharmont et al. 2011; Ramos and Liow 2012; Mischler et al. 2015). This model (QDA-S-Half) was established using a random selection of half of the samples in this study, including samples from both retrieval periods. To keep the sample size equivalent to comparable studies (i.e. ~10) (e.g. Becker et al. 2005, 2007; Niemand 2009), the sites missing from one retrieval period were not included (Sites 4, 10, 11, 12). This QDA-S-Half model was also validated by LOOCV.
To perform regional-scale analyses, the shell microchemistry data of mussels were combined into five groups (OP, OH, TH, PI, FA) on the basis of their geographic location and environmental conditions (see the ‘Overall pattern of mussel-shell microchemistry in BoP’ section for a description of regional-scale environmental variability used to delineate these geographic boundaries). Following protocols for the site-specific analyses, the following five regional-scale reference shell microchemistry atlas models were performed: (1) QDA-R-All, by using regional shell microchemistry data from all sampling sites and periods; (2) QDA-R-Lost, by using regional shell microchemistry data from all sampling periods and sampling sites excluding four sites (Sites 4, 10, 11, 12); (3) QDA-R-Ret1, by using regional shell microchemistry data from Retrieval #1; (4) QDA-R-Ret2, by using regional shell microchemistry data from Retrieval #2; and (5) QDA-R-Half, by randomly selecting half of the regional shell microchemistry data from both sampling periods.
Results
Mussel recovered from referential culture
Sample collections were lost at four sampling sites (Sites 4, 10, 11 and 12) in Retrieval #1, owing to either public interference or inclement weather events. Some ICP-MS machine errors during microchemistry analyses resulted in a further loss of two mussels at sampling site WSA during Retrieval #1, and only nine mussels were retrieved at sampling site MF. By contrast, all the mussel samples from Retrieval #2 were successfully recovered and processed, with a minimum of 10 mussels per collector. Thus, in total, 397 in situ-cultured mussels (177 from Retrieval #1 and 220 from Retrieval #2) were analysed from 18 sites in Retrieval #1 and 22 sites in Retrieval #2.
Overall shell microchemistry patterns in BoP
The interpolated elemental concentrations showed different patterns among elements, and among retrieval periods for some elements (Fig. 2). Elemental concentrations were less spatially homogeneous at the area in the middle of BoP (i.e. small patches of high or low concentration of elements (e.g. 7Li, 27Al, 59Co, 66Zn) were regularly observed), corresponding to the area where five major rivers converge at their entrances to the sea (i.e. Tarawera River, Rangitaiki River, Whakatāne River, and Waioeka and Otara River system). Several elements (e.g. 7Li, 63Cu, 138Ba, 139La and 140Ce) often showed a clear boundary of low and high concentrations, often near Sampling sites 10 and 11. This area corresponds to an open coastal area to the west, with lower freshwater inputs and reduced underwater geothermal influence. Many elements had a lower relative concentration in mussel shells in the west than in the eastern BoP (e.g. 27Al, 63Cu, 88Sr 138Ba, 139La and 208Pb in Retrieval #1, and 7Li, 59Co, 60Ni, 138Ba, 139La and 140Ce in Retrieval #2). For some elements, such as 88Sr, the interpolation map showed lower relative concentrations in the coastal areas adjacent to the estuaries (i.e. around Sites 4, 8, 10 and 11) than at other nearby sites in Retrieval #2. Unfortunately, the loss of mussel samples in Retrieval #1, at Sites 4, 10 and 11, prevented confirmation of this trend in Retrieval #1.
Geographic interpolation of mean (± s.e.m.) ln(x + 1) transformed element:calcium ratios (μmol:mol) of 12 element ratios to 43Ca in juvenile green-lipped mussel shells cultured in situ at 22 sites across the BoP for two sampling periods.
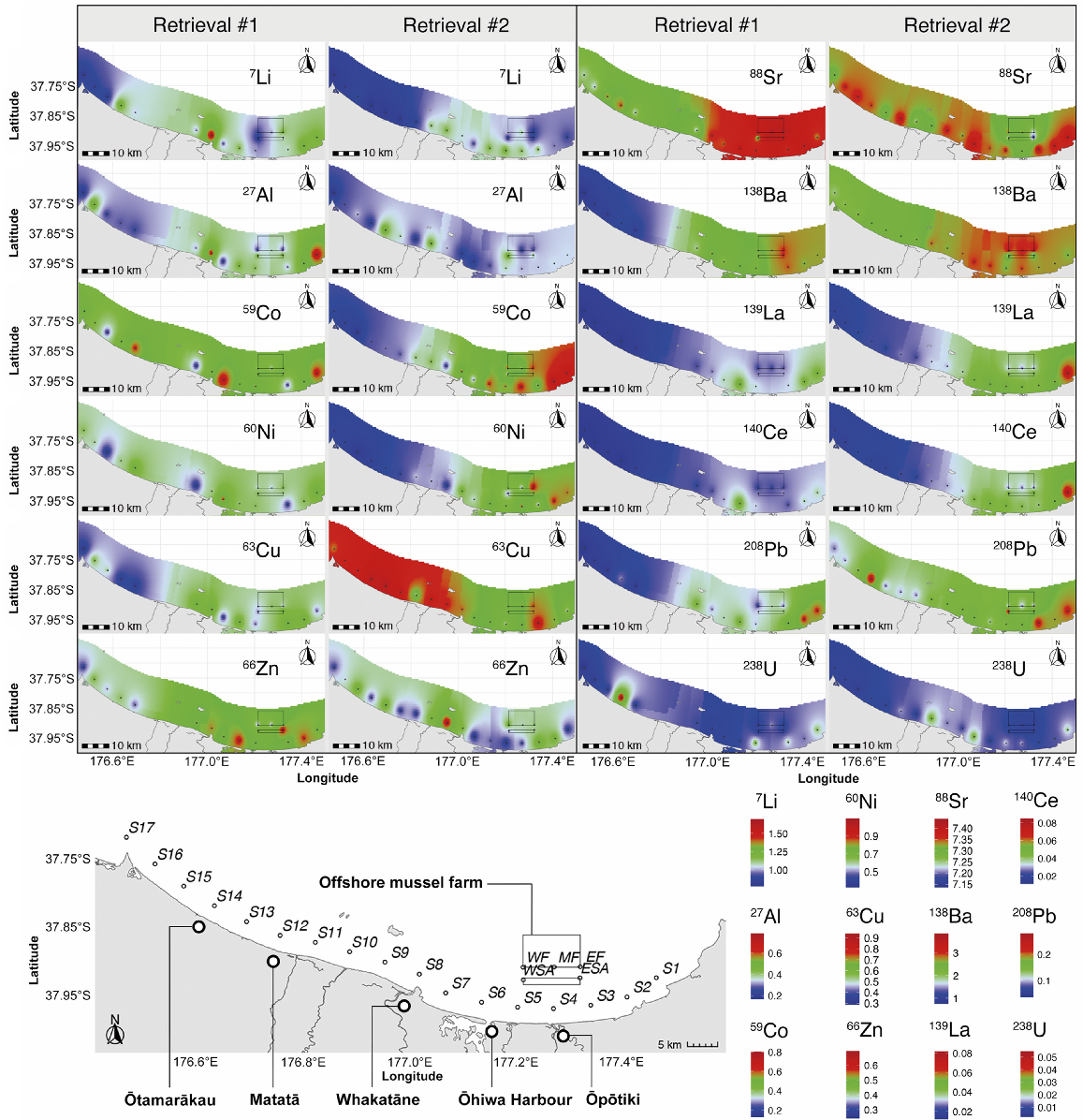
The hierarchical cluster analysis of 12 elements in the cultured mussel shells during Retrieval #1 illustrated that the entire study area can be divided into two main clusters, namely the sites on the western side of Sites 10 and 11 (i.e. Sites 13, 14, 15, 16, 17) and all remaining sites on the eastern side of BoP. The eastern sites can be further divided into four additional subclusters, with one cluster comprising five sites located mainly on or near the mussel-farm area (iSite 3, WF, MF, EF and ESA), one cluster of three sites located near the Ōhiwa Harbour (Site 5, Site 7 and WSA), and a cluster of four sites sparsely scattered in several different places (Sites 1 and 2 near Tōrere, with Sites 6 and 8 near Whakatāne), and one cluster of only one site, Site 9, noting this site is adjacent to three of the sites for which samples were lost in Retrieval #1 (Sites 10, 11, 12). The high Rand index of the geographically divided groups matrix and microchemistry clustering matrix (RI = 0.87) supported the grouping based on environmental characteristics. During the second sampling period, mean elemental concentrations for in situ-cultured mussel shells appeared to be more spatially homogeneous than during Retrieval #1 (Fig. 3).
Hierarchical cluster-analysis results of mean relative concentration of 12 elements measured in the reference mussel shell among BoP (cluster dendrogram and sites below marked by colour blocks), showing the spatially segregated-shell microchemistry features of P. canaliculus in BoP: (a–c) the clustering results for microchemistry features of 18 sampling sites in Retrieval #1, 22 sampling sites in Retrieval #2 and 22 sampling sites in Retrievals #1 and #2 combined. (d–f) The geographic grouping (OP, Ōpōtiki region; OH, Ōhiwa region; TH, Thornton region; PI, Pikowai region; FA, farm region) and clustering grouping (colour-coded) of these sampling sites. Paired panels show cluster dendrograms and colour-coded illustrations of clusters onto maps for (a, d) Retrieval #1 data only, (b, e) Retrieval #2 data only, and (c, f) combined Retrievals #1 and #2 data. (Sampling sites marked by the same colour block indicate the same shell microchemistry clustering group; sampling sites included within the same colour of line contour indicate the same geographic grouping; sampling sites with no colour-block markers in d were the four failed sampling sites, i.e. Sites 4, 10, 11 and 12.)
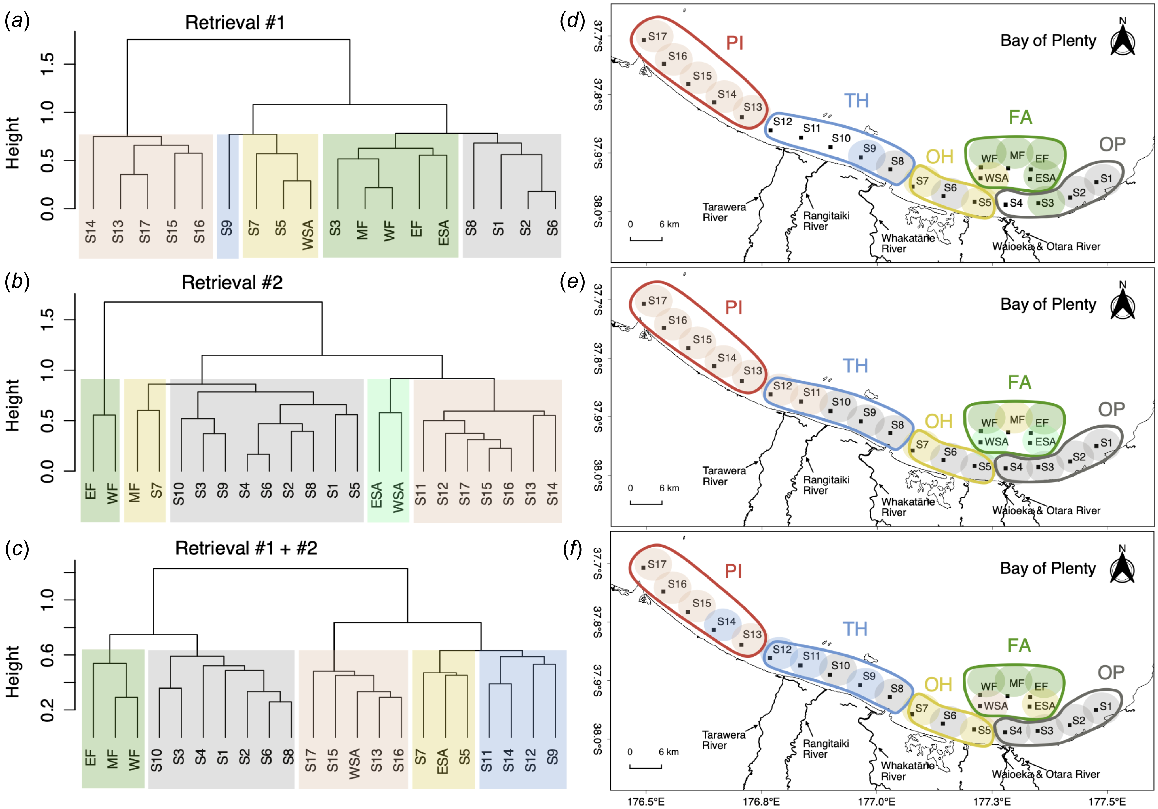
In Retrieval #2, all coastal sites were placed into two main clusters. The geographic clusters were divided by the estuarine area near Sites 10 and 11, except for Site 7, which had a closer microchemical signatures to sites on the mussel-farm region. The lower Rand index (RI = 0.77) in Retrieval #2 indicated that its microchemistry clustering pattern, although still generally consistent with the geological groupings and environmental classification, was slightly less similar to these geographic clusters than for the first sampling period.
The combined mean mussel microchemistry data from Retrieval #1 and Retrieval #2 showed similar results from the hierarchical cluster analysis. At the highest clustering level, all sampling locations were divided into two clusters. The first cluster included the three sites on the mussel farm (WF, MF, EF), four sites at the east of BoP (Sites 1, 2, 3, 4), and three sites scattered in the central BoP (Sites 6, 8, 10). The second cluster consisted of four sampling sites located at the western BoP (Sites 13, 14, 15, 16, 17), three sites found across the central region of the BoP (Sites 5, 7, 9, 11, 12), and two sites on the proposed spat-collection area (WSA and ESA). Subclusters of sites within the two clusters did not directly match the geographically divided groups (Fig. 3), rather sites located offshore of major estuaries were often clustered (e.g. Sites 6, 8, 10). Regardless, the high Rand index (RI = 0.82) suggested a high overall similarity between the microchemistry clustering results and geographically divided groups.
Temporal and spatial variability of shell microchemistry
The referential mussel spat cultured across the whole study area (~110 km) was significantly different in shell microchemical features between the two sampling periods (pseudo-F = 24.96, R2 = 0.06, P = 0.001), and microchemical signatures at smaller spatial scales within the BoP (i.e. site scale ~2–6 km: 94%; region scale ~12–24 km: 80%) were not always temporally stable. However, microchemical signatures at some sites or regions were still consistent over the two sampling periods of ~10 weeks in total. For example, Site 5 exhibited stability in microchemistry features between Retrievals #1 and #2 (pseudo-F = 1.71, R2 = 0.09, P = 0.165), and, likewise, the FA region (includes Site WF, MF, EF, WSA and ESA) (pseudo-F = 1.42, R2 = 0.015, P = 0.223).
Despite the temporal variability in microchemical characteristics at different spatial scales in BoP, significant spatial differences in shell microchemistry among sampling sites for each of the two sampling periods were shown by the PERMANOVA (Retrieval #1: pseudo-F = 17.90, R2 = 0.66, P = 0.001; Retrieval #2: pseudo-F = 9.94, R2 = 0.51, P = 0.001) (see Fig. 4 for the variation details). Within Retrieval #1, there were only five sampling-site pairs (accounting for 3.3% of a total of 153 site pairs) that showed no significant difference in shell microchemical features; all were located on the eastern side of the study area (i.e. eastern side of Ōhiwa Harbour; see Supplementary Table S1), with an average pairwise distance of 11.8 km. By contrast, for Retrieval #2, 11 sampling-site pairs had no significant difference in shell microchemical features (accounting for 4.8% of a total of 231 site pairs), and were primarily located near the Tarawera and Rangitaiki rivers, with an average pairwise distance of 13.6 km. After combining the mussels collected at each sampling site over two sampling periods, there were still significant spatial differences in shell microchemistry among sampling sites (pseudo-F = 11.55, R2 = 0.39, P = 0.001). In total, 13 sampling-site pairs showed similar shell microchemical features (see Table S1), in which most of the site pairs were geographically close to each other (e.g. MF v. WF, 5 km; Site 13 v. Site 14, 6 km), although some similar sites were more than 30 km away (e.g. Site 13 v. WSA, 43 km; WSA v. Site 16, 33 km). Most of the site pairs with no significant different in pairwise shell microchemical features were from the western BoP. Regardless of the retrieval time, open-coast sites were less likely to have significant differences in pairwise shell microchemical features (see Table S1).
NMDS plots illustrating in three dimensions the difference in ln(x + 1)-transformed element:calcium ratios (μmol:mol) of 12 element to 43Ca ratios in situ for cultured mussel shells at each sampling site in Retrieval #1, Retrieval #2 and for Retrieval #1 and #2 combined (stress = 0.05, 0.06 and 0.06 respectively). The ellipsoids show the scope of NMDS ± s.e. at each NMDS variable axis.
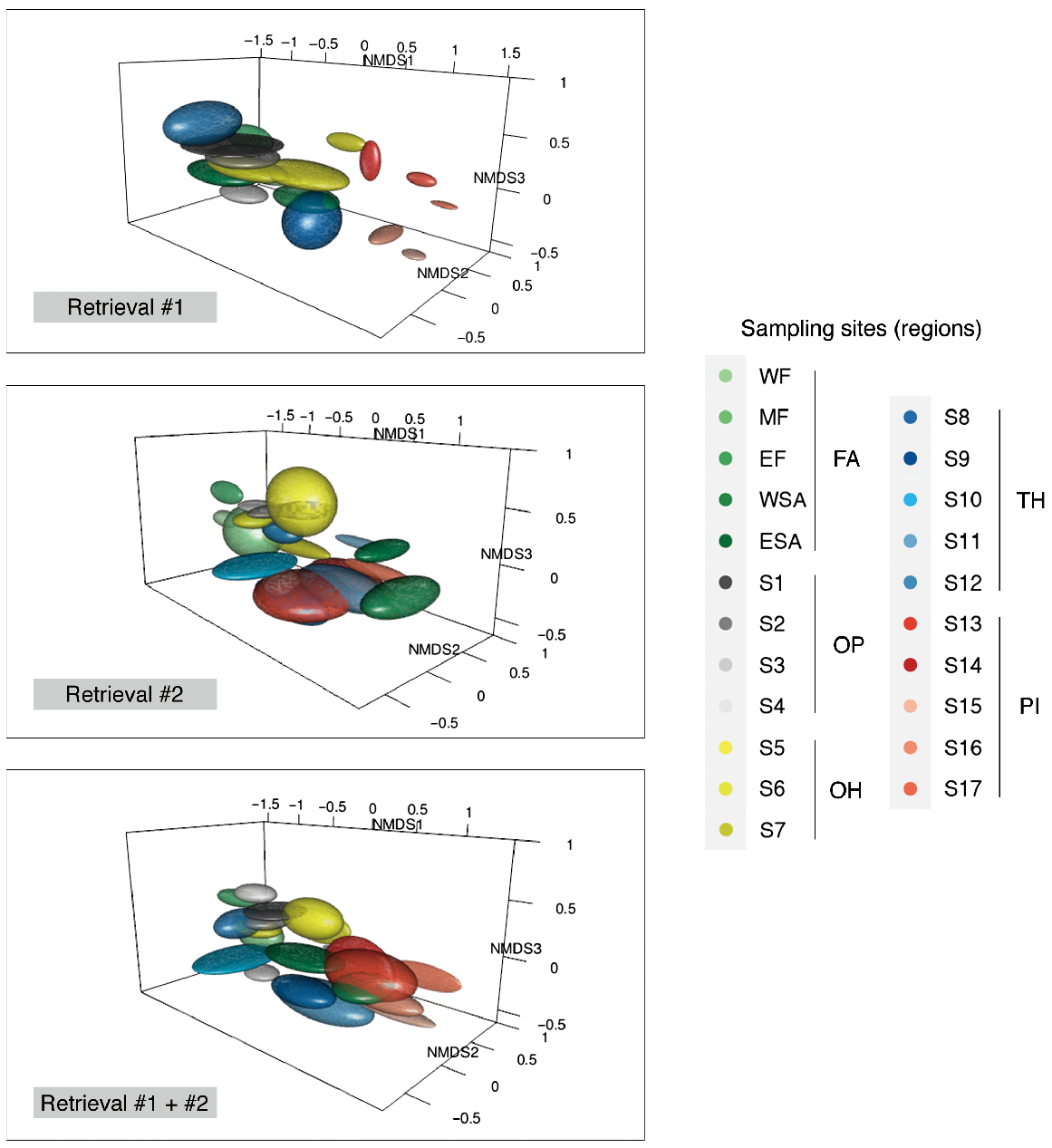
Spatial variation in shell microchemistry was observed at the regional scale (i.e. OP, OH, TH, PI, FA) across all retrieval periods, supporting assumptions about groupings based on environmental and geographic features (Retrieval #1: pseudo-F = 39.93, R2 = 0.48, P = 0.001; Retrieval #2: pseudo-F = 7.05, R2 = 0.12, P = 0.001; Retrieval #1 + #2: pseudo-F = 26.20, R2 = 0.21, P = 0.001). The shell microchemistry features between all of the post hoc region pairs were significantly different for all retrieval periods.
Establishment of a microchemistry reference atlas
The site-scale model (QDA-S-All) with all data from 22 sites used 6 of the 12 elemental ratios (7Li, 59Co, 60Ni, 138Ba, 139La, 238U) (based on the stepwise variable-selection process) to successfully classify mussel shells to their sampling sites, with an overall 57.4% LOOCV accuracy. Several elements (e.g. 7Li, 138Ba and 139La) contributed most to the classification, showing a clear boundary of low and high concentrations near Sampling sites 10 and 11 in the interpolation map of element distribution (Fig. 2). To the west of Sites 10 and 11 is an open coastal area with less freshwater input and weaker underwater geothermal influence. Furthermore, many elements in this optimum subset also had a lower relative concentration in mussel shells in the western than the eastern BoP (e.g. 138Ba and 139La in Retrieval #1, and 7Li 59Co, 60Ni, 138Ba, 139La in Retrieval #2); these spatial differences support their selection as the optimal subset of elements that contributed to the model classification.
The reclassification success rate for site-scale model QDA-S-Ret1 (i.e. 74.0%) was higher for Retrieval #1 using 18 sites than it was for all other site-scale QDA model cross-validations (see Table S2). Including sites with missing data from Retrieval #1 (i.e. Sites 4, 10, 11 and 12) resulted in an ~4.8% reduction in performance (QDA-S-Lost: 62.2% v. QDA-S-All: 57.4%). However, including 22 instead of 18 sites did increase the spatial coverage (km) of the atlas by ~24 km, which accounts for ~22% of the entire study area. The lower reclassification success rate of QDA-S-Half model (45.3%, test by LOOCV) than that of either individual retrieval model QDA-S-Ret1 (74.0%, test by LOOCV) or QDA-S-Ret2 (60.9%, test by LOOCV) suggests that a longer temporal span of the data incorporated into the model can reduce the model accuracy (when keeping sample size constant) because of the temporal variability in microchemical signal. This temporal change in microchemical signal also means that reference shell microchemistry needs to be collected contemporaneously with sampling of wild individuals. For example, when the model QDA-S-Ret2 (trained from Retrieval #2 data) was used for localising mussel-shell samples collected from Retrieval #1, the accuracy and sensitivity of the microchemical reference atlas was extremely limited (i.e. 11.8% accuracy and 0.64 sensitivity). Conversely, when the model QDA-S-Ret1 (trained from Retrieval #2 data) was tested on mussel-shell samples collected by Retrieval #2, the model had limited success in classifying mussels to their correct sampling location (i.e. 21.7% accuracy and 0.65 sensitivity). The LOOCV reclassification success rate of the QDA-S-Lost model (62.2%) was 16.9 percentage points higher than that of the QDA-S-Half model (45.3%), suggesting that doubling the sample size from n = 10 to n = 20 could improve the accuracy of the site-scale microchemistry referential atlas by 37.3%.
In general, the regional-scale models all had higher reclassification accuracy (i.e. mean ~16 percentage points higher) than did the corresponding site-scale models, regardless of the model construction and validation method used, but classification success comparisons across models showed similar patterns for the performance of regional-scale reference atlases (see Table S3). Extending the time span over which the reference shell samples were collected reduced the accuracy of the regional shell microchemistry reference atlas (i.e. LOOCV accuracy of model QDA-R-Ret1, 85.9%, and QDA-R-Half, 68.3%), although the decrease in classification success was not as large at the regional scale (17.6%) as it was at the site scale (28.7%) for the regional-scale atlas. Increasing sample size also improved the accuracy of the regional-scale microchemistry reference atlas, by 11.0% (i.e. LOOCV accuracy of model QDA-R-Half was 68.3% v. that of QDA-R-Lost was 79.3%).
Discussion
Microchemistry reference atlas for open-coast mussels
This study demonstrated that, despite the existence of temporal variability in shell microchemistry at all spatial scales across the BoP, spatial variation in the shell microchemistry was sufficient to establish an effective shell microchemistry referential atlas. The shell microchemical features of in situ-cultured mussel shells in BoP varied temporally over an ~10-week period at three different spatial scales, from the smallest site scale of ~2–6 km to the regional scale of ~12–24 km, to the entire study area of ~110 km. Meanwhile, the overall differences were sufficient for the microchemistry atlas to classify mussel shells to their site of origin with a reasonable degree of accuracy, with only 3.3–4.8% site pairs showing no significant differences in shell microchemistry at ~2–6-km spatial resolution.
Although seawater on the open coast is well mixed and therefore is expected to lack sufficient spatial variability in elemental composition for microchemical studies, several previous studies have successfully shown that it is possible to establish a microchemical reference atlas for species on the open coast (Swearer et al. 1999; Zacherl et al. 2003; Zacherl 2005). In this current study of a large open-coastal embayment of ~110 km in length with a high degree of seawater mixing, the site-scale reference atlas using data from both sampling periods was able to correctly assign 57.4% of mussel shells collected over a 2-month period to their site of origin at a resolution of ~2–6 km. If the time scale is shortened to ~1 month, the microchemical reference atlas is more accurate, reaching up to 74.0%. At a regional scale, the microchemical reference atlas has an 85.9% classification success over 1 month and 73.3% for 2 months on a spatial scale of 12–24 km.
Spatial patterns in shell microchemistry
In this study, the mean relative concentrations of 12 elements differed spatially across 22 sites within BoP for in situ-cultured mussel shells. There were some temporal differences in the patterns of trace-element concentrations between the two sampling periods. For example, there was an east–west variation in Sr concentration in Retrieval #1, with higher Sr concentrations on the open-coast region (i.e. PI region) and geothermally active areas (i.e. TH region) to the west of the Whakatāne River than on its eastern side (i.e. OH, OP, FA regions). In Retrieval #2, the pattern of Sr concentration became more dispersed, with minimal differences between the eastern and western regions described above, but with a more pronounced area of low Sr concentration on coastal areas adjacent to estuarine areas. This trend was consistent with some of the results from previous studies, in which the incorporation of Sr element into bio-generated calcite structures was suggested to be affected by seawater salinity for different marine species (Dodd and Crisp 1982; Secor et al. 1995; Kroll et al. 2016), although the correlation varied among different taxa and environmental habitats (Gillikin et al. 2005; Carré et al. 2006; Beer et al. 2011; Carson et al. 2013; Poulain et al. 2015). Further study is required to confirm whether the Sr concentration in mussel shells is positively or negatively correlated with salinity or other environmental conditions (e.g. temperature) (Kroll et al. 2016) in BoP. Unfortunately, the loss of samples at sites closest to the estuarine area of this coast (e.g. Sites 10 and 11) in Retrieval #1 affected the ability to confirm the stability of this trend.
Some elements (e.g. Li, Cu, Ba, La, Ce and Al in Retrieval #2) exhibited different distributions between the western and eastern sides of the Whakatāne River, with sampling sites in the western side of the river showing regional consistency, whereas eastern sites showed high variability. For the western side of the Whakatāne River, all the sites from Site 13 to Site 17 are located at the open-coast area with less freshwater input and reduced submarine geothermal activities. Furthermore, many of these elements also had a lower relative concentration in the western sites than in the eastern BoP (e.g. Al, Cu, Sr, Ba, La and Pb in Retrieval #1, and Li, Co, Ni, Ba, La and Ce in Retrieval #2). This may also be explained by the difference between the microchemical inflows to the open coast and other freshwater convergence zones, because previous studies have suggested that some elements, such as Cu and Pb, can be consistently lower in concentration on the open coast than in bays (Forrester and Swearer 2002). Freshwater input and nutrient runoff from land can contribute to the coastal microalgal concentration and thus increase the bioavailability of element Ba (Putten et al. 2000; Lopes 2007; Thébault et al. 2009). Other than surface run-off, nutrient-rich coastal upwelling may also be an important cause of variation in the concentration of some trace elements. For example, Ba concentration in coral aragonite has been shown to be strongly correlated with upwelling (Lea et al. 1989; Patterson et al. 1999). Given the wind-driven upwelling system dominating the BoP coast, this may also be an important factor in driving spatial variability in elemental concentrations. Additionally, gradients of some elements (e.g. Cu and Co) in BoP may also be from anthropogenic sources because there are distinct differences in the human densities between the eastern and western sides of the BoP (see https://rep.infometrics.co.nz).
The coastline of the BoP has a variety of environmental and geographic features, including two open coastal areas in the easternmost and westernmost parts of the study area, interposed by an area of freshwater convergence where several major high-flowing rivers enter the sea, and an area of intense submarine geothermal activity in the western part of this river convergence area. The sampling sites in BoP can be divided into five wider regions on the basis of these environmental and geographic features. Mean mussel microchemistry data in BoP exhibited spatially stratified features regardless of the sampling period, as shown by the results of three hierarchical cluster analysis. Although the detailed hierarchical clustering results of sites changed slightly between Retrieval #1 and Retrieval #2, the sampling locations across the east–west gradient always clustered into different groups, although the dividing line varied in location from Site 4 to Site 12, a region which contains all the estuaries of the five main rivers in the BoP (i.e. Tarawera River, Rangitaiki River, Whakatāne River, and Waioeka and Otara River system. This indistinct dividing line can also be observed in the relative concentration of elements in the interpolation map (Fig. 2), in which the elemental concentrations were less homogeneous in the area towards the middle of the BoP. These microchemical clustering features were shown by the Rand index to be similar to spatial environmental feature groupings, supporting the approach of using groupings of sites into regions to more accurately define spatial patterns in microchemical variability in the BoP.
Previous studies have generally shown that the classification success rate of microchemical reference atlases is generally higher for sites that are further apart, such as 63–100% for regions that are >110 km apart (Carson 2010; Dunphy et al. 2011). However, classification success is not always correlated with distance because of the reliance on spatial differences and temporal stability in elemental signals. For example, in one study the classification success rate of two sampling locations that were 16 km apart was 55%, whereas two sampling locations that were only 1.2 km away had a 90% classification success (Norrie et al. 2019).
Temporal variability of shell microchemistry
Sites with stable environmental characteristics, often characterised by static or stable seawater flow, typically have more stable microchemical signatures (Gillanders 2002; Becker et al. 2005; Zacherl 2005). Most previous studies examined the temporal stability of microchemical signatures within bays and harbours (Niemand 2009; Norrie 2019), and have generally determined only the temporal stability of microchemistry feature at only a few sampling sites (Becker et al. 2005; Dunphy et al. 2011; Norrie 2019) from which further conclusions about the relative importance of temporal stability and spatial variability are then drawn.
Few previous studies have explored the temporal stability of microchemical signals at a broad coastal scale. The results of this study successfully fill this gap, finding that the microchemical composition of mussel shells changed at all of three spatial scales (~2–6, ~12–24 and ~110 km) over two consecutive 5-week periods. By comparing the performance of different microchemical reference atlases established in this area with unstable microchemical characteristics and using different sampling configurations, this study found the sampling interval for the microchemical reference atlas should reflect temporal variation in microchemical signatures that are typically found at a site, and be contemporaneous with the shell formation of the organisms of interest for which the atlas will be used for determining their location. Failure to do so is likely to greatly reduce the accuracy and sensitivity of the microchemical reference atlas for tracking the source of mussel shell and may result in extremely low prediction accuracy (at only ~10–20%). Including sufficient temporal variation in microchemistry results in higher temporal resolution and helps examine in greater detail the pelagic movements of the early stages of mussels. However, too much variation can affect the establishment of stable microchemical methods for tracking larval dispersal (Carson et al. 2013).
To accurately assess the patterns of connectivity among mussel populations in this area and to estimate larval dispersal distances, we need to ensure that the length of time that microchemical reference signatures are collected cover the entire planktonic time of the larvae to be studied (Becker et al. 2005). Planktonic bivalve larvae may spend weeks in the plankton, and the pelagic larval duration (PLD) of larval green-lipped mussels is likely to be 3–6 weeks in the wild, depending on environmental conditions (Buchanan 1994; Hayden 1994; Apte and Gardner 2002; Wei et al. 2013), which is comparable to PLDs (i.e. 21–35 days) of other mussel species (Chipperfield 1953; Seed 1969; McGarigal et al. 2000; Sorte et al. 2013). Thus, our sampling period of ~5 weeks at a time should adequately cover the main larval dispersal period to create a credible microchemical reference atlas. However, some bivalve species, including green-lipped mussels, undertake post-larval or secondary dispersal, whereby they exude buoyant mucous threads which facilitate drifting in the water column. Seawater velocity is thought to have a significant impact on the ability of juveniles to resuspend or encounter substrata in the water column for both larval and post-settlement dispersal (Tortell 1976; Walters and Wethey 1996; De Montaudouin et al. 2003; Brenner and Buck 2010). Thus, a longer time period for collecting microchemistry reference signatures may be required where the intention is to utilise microchemical signatures to include a period of secondary juvenile pelagic dispersal in addition to larval dispersal.
It is noteworthy that 3 days prior to the deployment of on-site-cultured mussel samples, a significant low pressure system affected the BoP area, causing severe stormy weather, including heavy rainfall and flooding in some areas. This event resulted in a dramatic drop in temperature, a large amount of freshwater entering the inshore waters and possible salinity changes; thus, this storm event may have may have influenced seawater microchemistry and resulted in the difference in subsequent uptake of microchemistry into on-site-cultured mussel shells found in this study.
Conclusions
This study suggested that BoP has sufficient spatial variability in the mussel shell microchemical features for establishing a reasonably accurate referential atlas that can be used for inferring the past locations of settled mussels on the basis of their shell microchemistry. Significant temporal variability in microchemical signatures of in situ mussel shells at 22 sites across the BoP was found over a range of geographical scales from 2 to 110 km over two sequential 1-month periods. This was most likely to be due to the results of temporal variability in oceanographic processes in the BoP. Many elements showed a general trend of low concentrations in the west and higher concentrations in the east, and produced five specific regions of microchemical similarity consistent with the classification of environmental conditions. The microchemical structures found in this study are compatible with the geographic environmental classification patterns supporting this reference atlas for assigning newly settled mussel spat back to their place of origin.
The complexity of spatial and temporal variability in microchemical signatures raises significant difficulties in designing experimental studies, so as to balance spatial and temporal sampling design with logical and financial constraints of sample collection and analysis. To incorporate temporal variability in microchemical signatures, it is desirable that the sampling time of the microchemical reference atlas be established to cover all possible times of passage of wild larvae (and post-settlement dispersal) through the area to be studied. Increasing the number of shell samples used for establishing a referential atlas may also help improve the accuracy of microchemistry reference atlases, particularly for sites with low stability of microchemical features or areas with complex microchemical spatial variability. To improve the reference atlas, the environmental background characteristics of different sites should be investigated prior to the study, sampling sites should be selected at sites with as different environmental characteristics as possible, and the sample size should be increased (relative to logistic constraints) to incorporate variability, especially for open-coast area.
Consequently, this study assessed the spatial and temporal variability in the trace elements by culturing juvenile green-lipped mussels at 22 sites covering 110 km of coastline in the BoP, over two consecutive ~5-week periods. Using the trace-element data from these mussels, a series of microchemical reference models at different spatial and temporal scales were also developed and compared to determine the effective spatial and temporal resolution for assessing natal sources and pathways of pelagic mussels settling onto the offshore mussel farm.
Data availability
The data that support this study will be shared upon reasonable request to the corresponding author.
Declaration of funding
This work is a contribution to the Moana Project (www.moanaproject.org) and funded by the New Zealand Ministry of Business Innovation and Employment, contract number METO1801.
Acknowledgements
The authors acknowledge SPATnz Ltd for providing the hatchery-reared mussel larvae. Thanks go to Ashleigh Browne, Joe Burke and the team at MUSA Ltd for their assistance in the extensive sampling in BoP. Thanks go for the significant assistance provided from Ashleigh Browne and Stefan Spreitzenbarth in the sampling and sample processing. Thanks go to Stuart Morrow from the UoA mass spectrometry centre who provided the training and expert advice for LA-ICP-MS. The authors also thank Danny Paruru and colleagues of the Whakatōhea Māori Trust Board for continuous help and support in both initiating and progressing this research.
References
Adler D, Nenadic O, Zucchini W (2003) RGL: a R-library for 3D visualization with OpenGL. In ‘35th Symposium of the Interface: Computing Science and Statistics 2003: Security and Infrastructure Protection. Computing Science and Statistics, Vol. 35’, 12–15 March 2003, Salt Lake City, UT, USA. pp. 419–429. (Curran Associates, Inc.: Red Hook, NY, USA) Available at https://citeseerx.ist.psu.edu/document?repid=rep1&type=pdf&doi=c20764ebb8f384cfe76042c41af1ca0bd9695f7c
Alfaro AC, Jeffs AG, Hooker SH (2003) Spatial variability in reproductive behaviour of green-lipped mussel populations of northern New Zealand. Molluscan Research 23, 223-238.
| Crossref | Google Scholar |
Alfaro AC, Jeffs AG, Creese RG (2004) Bottom-drifting algal/mussel spat associations along a sandy coastal region in northern New Zealand. Aquaculture 241, 269-290.
| Crossref | Google Scholar |
Alfaro AC, McArdle B, Jeffs AG (2010) Temporal patterns of arrival of beachcast green-lipped mussel (Perna canaliculus) spat harvested for aquaculture in New Zealand and its relationship with hydrodynamic and meteorological conditions. Aquaculture 302, 208-218.
| Crossref | Google Scholar |
Apte S, Gardner JPA (2002) Population genetic subdivision in the New Zealand greenshell mussel (Perna canaliculus) inferred from single-strand conformation polymorphism analysis of mitochondrial DNA. Molecular Ecology 11, 1617-1628.
| Crossref | Google Scholar | PubMed |
Avdelas L, Avdic-Mravlje E, Borges Marques AC, Cano S, Capelle JJ, Carvalho N, Cozzolino M, Dennis J, Ellis T, Fernández Polanco JM, Guillen J, Lasner T, Le Bihan V, Llorente I, Mol A, Nicheva S, Nielsen R, van Oostenbrugge H, Villasante S, Visnic S, Zhelev K, Asche F (2021) The decline of mussel aquaculture in the European Union: causes, economic impacts and opportunities. Reviews in Aquaculture 13, 91-118.
| Crossref | Google Scholar |
Becker BJ, Fodrie FJ, McMillan PA, Levin LA (2005) Spatial and temporal variation in trace elemental fingerprints of mytilid mussel shells: a precursor to invertebrate larval tracking. Limnology and Oceanography 50, 48-61.
| Crossref | Google Scholar |
Becker BJ, Levin LA, Fodrie FJ, McMillan PA (2007) Complex larval connectivity patterns among marine invertebrate populations. Proceedings of the National Academy of Sciences 104, 3267-3272.
| Crossref | Google Scholar |
Beer NA, Wing SR, Hu Y (2011) Physical versus biological control of element incorporation into biogenic carbonate: an in situ experiment in a New Zealand fjord. Marine Ecology Progress Series 433, 289-301.
| Crossref | Google Scholar |
Bennion M, Morrison L, Brophy D, Carlsson J, Abrahantes JC, Graham CT (2019) Trace element fingerprinting of blue mussel (Mytilus edulis) shells and soft tissues successfully reveals harvesting locations. Science of The Total Environment 685, 50-58.
| Crossref | Google Scholar | PubMed |
Brenner M, Buck BH (2010) Attachment properties of blue mussel (Mytilus edulis L.) byssus threads on culture-based artificial collector substrates. Aquacultural Engineering 42, 128-139.
| Crossref | Google Scholar |
Caley MJ, Carr MH, Hixon MA, Hughes TP, Jones GP, Menge BA (1996) Recruitment and the local dynamics of open marine populations. Annual Review of Ecology and Systematics 27, 477-500.
| Crossref | Google Scholar |
Carrasco AV, Astorga M, Cisterna A, Farias A, Espinoza V, Uriarte I (2014) Pre-feasibility study for the installation of a Chilean mussel Mytilus chilensis (Hupe, 1854) seed hatchery in the lakes region, Chiles. Fisheries and Aquaculture Journal 5(3), 102.
| Crossref | Google Scholar |
Carré M, Bentaleb I, Bruguier O, Ordinola E, Barrett NT, Fontugne M (2006) Calcification rate influence on trace element concentrations in aragonitic bivalve shells: evidences and mechanisms. Geochimica et Cosmochimica Acta 70, 4906-4920.
| Crossref | Google Scholar |
Carson HS (2010) Population connectivity of the Olympia oyster in southern California. Limnology and Oceanography 55, 134-148.
| Crossref | Google Scholar |
Carson HS, López-Duarte PC, Cook GS, Fodrie FJ, Becker BJ, DiBacco C, Levin LA (2013) Temporal, spatial, and interspecific variation in geochemical signatures within fish otoliths, bivalve larval shells, and crustacean larvae. Marine Ecology Progress Series 473, 133-148.
| Crossref | Google Scholar |
Cathey AM, Miller NR, Kimmel DG (2014) Spatiotemporal stability of trace and minor elemental signatures in early larval shell of the northern quahog (hard clam) Mercenaria mercenaria. Journal of Shellfish Research 33, 247-255.
| Crossref | Google Scholar |
Chipperfield PNJ (1953) Observations on the breeding and settlement of Mytilus edulis (L.) in British waters. Journal of the Marine Biological Association of the United Kingdom 32, 449-476.
| Crossref | Google Scholar |
Cook GS (2011) Changes in otolith microchemistry over a protracted spawning season influence assignment of natal origin. Marine Ecology Progress Series 423, 197-209.
| Crossref | Google Scholar |
Cowen RK, Sponaugle S (2009) Larval dispersal and marine population connectivity. Annual Review of Marine Science 1, 443-466.
| Crossref | Google Scholar | PubMed |
Crowder LB, Lyman SJ, Figueira WF, Priddy J (2000) Source–sink population dynamics and the problem of siting marine reserves. Bulletin of Marine Science 66, 799-820 https://www.ingentaconnect.com/content/umrsmas/bullmar/2000/00000066/00000003/art00021.
| Google Scholar |
Darroch JN, Mosimann JE (1985) Canonical and principal components of shape. Biometrika 72(2), 241-252.
| Crossref | Google Scholar |
de Montaudouin X, Bachelet G, Sauriau P-G (2003) Secondary settlement of cockles Cerastoderma edule as a function of current velocity and substratum: a flume study with benthic juveniles. Hydrobiologia 503, 103-116.
| Crossref | Google Scholar |
Dechaume-Moncharmont F-X, Monceau K, Cezilly F (2011) Sexing birds using discriminant function analysis: a critical appraisal. The Auk 128, 78-86.
| Crossref | Google Scholar |
Dixon P (2003) VEGAN, a package of R functions for community ecology. Journal of Vegetation Science 14(6), 927-930.
| Crossref | Google Scholar |
Dodd JR, Crisp EL (1982) Non-linear variation with salinity of Sr/Ca and Mg/Ca ratios in water and aragonitic bivalve shells and implications for paleosalinity studies. Palaeogeography, Palaeoclimatology, Palaeoecology 38, 45-56.
| Crossref | Google Scholar |
Dunphy BJ, Millet M-A, Jeffs AG (2011) Elemental signatures in the shells of early juvenile green-lipped mussels (Perna canaliculus) and their potential use for larval tracking. Aquaculture 311, 187-192.
| Crossref | Google Scholar |
Fodrie FJ, Becker BJ, Levin LA, Gruenthal K, McMillan PA (2011) Connectivity clues from short-term variability in settlement and geochemical tags of mytilid mussels. Journal of Sea Research 65, 141-150.
| Crossref | Google Scholar |
Forrester GE, Swearer SE (2002) Trace elements in otoliths indicate the use of open-coast versus bay nursery habitats by juvenile California halibut. Marine Ecology Progress Series 241, 201-213.
| Crossref | Google Scholar |
Gillanders BM (2002) Connectivity between juvenile and adult fish populations: do adults remain near their recruitment estuaries? Marine Ecology Progress Series 240, 215-223.
| Crossref | Google Scholar |
Gillanders BM, Sanchez-Jerez P, Bayle-Sempere J, Ramos-Espla A (2001) Trace elements in otoliths of the two-banded bream from a coastal region in the south-west Mediterranean: are there differences among locations? Journal of Fish Biology 59, 350-363.
| Crossref | Google Scholar |
Gillikin DP, Lorrain A, Navez J, Taylor JW, André L, Keppens E, Baeyens W, Dehairs F (2005) Strong biological controls on Sr/Ca ratios in aragonitic marine bivalve shells. Geochemistry, Geophysics, Geosystems 6, Q05009.
| Crossref | Google Scholar |
Gräler B, Pebesma EJ, Heuvelink GB (2016) Spatio-temporal interpolation using gstat. The R Journal 8(1), 204-218 https://journal.r-project.org/archive/2016/RJ-2016-014/index.html.
| Google Scholar |
Hayden BJ (1994) What do we know about greenshell mussel spat? Seafood New Zealand. Part I 2, 45-47.
| Google Scholar |
Hocking MWA, Hannington MD, Percival JB, Stoffers P, Schwarz-Schampera U, de Ronde CEJ (2010) Clay alteration of volcaniclastic material in a submarine geothermal system, Bay of Plenty, New Zealand. Journal of Volcanology and Geothermal Research 191, 180-192.
| Crossref | Google Scholar |
Ihaka R, Gentleman R (1996) R: a language for data analysis and graphics. Journal of Computational and Graphical Statistics 5(3), 299-314.
| Crossref | Google Scholar |
Jeffs AG, Holland RC, Hooker SH, Hayden BJ (1999) Overview and bibliography of research on the greenshell mussel, Perna canaliculus, from New Zealand waters. Journal of Shellfish Research 18, 347-360.
| Google Scholar |
Jeffs AG, Delorme NJ, Stanley J, Zamora LN, Sim-Smith C (2018) Composition of beachcast material containing green-lipped mussel (Perna canaliculus) seed harvested for aquaculture in New Zealand. Aquaculture 488, 30-38.
| Crossref | Google Scholar |
Kilgour G, Kennedy B, Scott B, Christenson B, Jolly A, Asher C, Rosenberg M, Saunders K (2021) Whakaari/White Island: a review of New Zealand’s most active volcano. New Zealand Journal of Geology and Geophysics 64, 273-295.
| Crossref | Google Scholar |
Kroll IR, Poray AK, Puckett BJ, Eggleston DB, Fodrie FJ (2016) Environmental effects on elemental signatures in eastern oyster Crassostrea virginica shells: using geochemical tagging to assess population connectivity. Marine Ecology Progress Series 543, 173-186.
| Crossref | Google Scholar |
Lea DW, Shen GT, Boyle EA (1989) Coralline barium records temporal variability in equatorial Pacific upwelling. Nature 340, 373-376.
| Crossref | Google Scholar |
Levin LA (2006) Recent progress in understanding larval dispersal: new directions and digressions. Integrative and Comparative Biology 46, 282-297.
| Crossref | Google Scholar | PubMed |
Lopes RM (2007) Marine zooplankton studies in Brazil: a brief evaluation and perspectives. Anais da Academia Brasileira de Ciências 79, 369-379.
| Crossref | Google Scholar | PubMed |
Lyons GL, Giggenbach WF, Singleton RJ, Glasby GP (1977) Isotopic and chemical composition of submarine geothermal gases from the Bay of Plenty, New Zealand. New Zealand Department of Scientific and Industrial Research Bulletin 218, 65-67.
| Google Scholar |
Miller SH, Morgan SG, White JW, Green PG (2013) Interannual variability in an atlas of trace element signatures for determining population connectivity. Marine Ecology Progress Series 474, 179-190.
| Crossref | Google Scholar |
Mischler CP, Bell EA, Landers TJ, Dennis TE (2015) Sex determination of black petrels (Procellaria parkinsoni) using morphometric measurements and discriminant function analysis. Notornis 62, 57-62.
| Google Scholar |
Montaño MM, Suanda SH, Souza JMAC (2023) Modelled coastal circulation and Lagrangian statistics from a large coastal embayment: the case of Bay of Plenty, Aotearoa New Zealand. Estuarine, Coastal and Shelf Science 281, 108212.
| Crossref | Google Scholar |
Mundry R, Sommer C (2007) Discriminant function analysis with nonindependent data: consequences and an alternative. Animal Behaviour 74, 965-976.
| Crossref | Google Scholar |
Norrie CR, Dunphy BJ, Ragg NLC, Lundquist CJ (2018) Ocean acidification can interact with ontogeny to determine the trace element composition of bivalve shell. Limnology and Oceanography Letters 3, 393-400.
| Crossref | Google Scholar |
Norrie CR, Dunphy BJ, Ragg NLC, Lundquist CJ (2019) Comparative influence of genetics, ontogeny and the environment on elemental fingerprints in the shell of Perna canaliculus. Scientific Reports 9, 8533.
| Crossref | Google Scholar |
Norrie C, Dunphy B, Roughan M, Weppe S, Lundquist C (2020) Spill-over from aquaculture may provide a larval subsidy for the restoration of mussel reefs. Aquaculture Environment Interactions 12, 231-249.
| Crossref | Google Scholar |
Pantin HM, Wright IC (1994) Submarine hydrothermal activity within the offshore Taupo Volcanic Zone, Bay of Plenty continental shelf, New Zealand. Continental Shelf Research 14, 1411-1438.
| Crossref | Google Scholar |
Paton C, Hellstrom J, Paul B (2011) Iolite: freeware for the visualisation and processing of mass spectrometric data. Journal of Analytical Atomic Spectrometry 26(12), 2508-2518.
| Crossref | Google Scholar |
Patterson HM, Thorrold SR, Shenker JM (1999) Analysis of otolith chemistry in Nassau grouper (Epinephelus striatus) from the Bahamas and Belize using solution-based ICP-MS. Coral Reefs 18, 171-178.
| Crossref | Google Scholar |
Peacock E, Neff H, Rafferty J, Meaker T (2007) Using laser ablation–inductively coupled plasma–mass spectrometry (LA-ICP-MS) to source shell in shell-tempered pottery: a pilot study from North Mississippi. Southeastern Archaeology 26, 319-329.
| Google Scholar |
Pebesma EJ (2004) Multivariable geostatistics in S: the gstat package. Computers and Geoscience 30, 683-691.
| Crossref | Google Scholar |
Poulain C, Gillikin DP, Thébault J, Munaron JM, Bohn M, Robert R, Paulet Y-M, Lorrain A (2015) An evaluation of Mg/Ca, Sr/Ca, and Ba/Ca ratios as environmental proxies in aragonite bivalve shells. Chemical Geology 396, 42-50.
| Crossref | Google Scholar |
Putten EV, Dehairs F, Keppens E, Baeyens W (2000) High resolution distribution of trace elements in the calcite shell layer of modern Mytilus edulis: environmental and biological controls. Geochimica et Cosmochimica Acta 64, 997-1011.
| Crossref | Google Scholar |
Racine JS (2012) RStudio: a platform-independent IDE for R and Sweave. Journal of Applied Econometrics 27(1), 167-172.
| Crossref | Google Scholar |
Rand WM (1971) Objective criteria for the evaluation of clustering methods. Journal of the American Statistical Association 66, 846-850.
| Crossref | Google Scholar |
Ridgway NM, Greig MJN (1986) Water movements in Bay of Plenty, New Zealand. New Zealand Journal of Marine and Freshwater Research 20, 447-453.
| Crossref | Google Scholar |
Robin X, Turck N, Hainard A, Tiberti N, Lisacek F, Sanchez JC, Müller M (2011) pROC: an open-source package for R and S to analyze and compare ROC curves. BMC Bioinformatics 12, 77.
| Crossref | Google Scholar |
Secor DH, Henderson-Arzapalo A, Piccoli PM (1995) Can otolith microchemistry chart patterns of migration and habitat utilization in anadromous fishes? Journal of Experimental Marine Biology and Ecology 192, 15-33.
| Crossref | Google Scholar |
Seed R (1969) The ecology of Mytilus edulis L. (Lamellibranchiata) on exposed rocky shores – I. Breeding and settlement. Oecologia 3, 277-316.
| Crossref | Google Scholar | PubMed |
Skelton B, South PM, Jeffs AG (2021) Inefficiency of conversion of seed into marketready mussels in New Zealand’s GreenshellTM mussel (Perna canaliculus) industry. Aquaculture 560, 738584.
| Crossref | Google Scholar |
Sorte CJB, Etter RJ, Spackman R, Boyle EE, Hannigan RE (2013) Elemental fingerprinting of mussel shells to predict population sources and redistribution potential in the gulf of maine. PLoS ONE 8, e80868.
| Crossref | Google Scholar |
South PM, Delorme NJ, Skelton BM, Floerl O, Jeffs AG (2021) The loss of seed mussels in longline aquaculture. Reviews in Aquaculture 14(1), 440-455.
| Crossref | Google Scholar |
Stoffers P, Hannington M, Wright I, Herzig P, de Ronde C, Shipboard Scientific Party (1999) Elemental mercury at submarine hydrothermal vents in the Bay of Plenty, Taupo volcanic zone, New Zealand. Geology 27(10), 931-934.
| Crossref | Google Scholar |
Strasser CAA, Thorrold SRR, Starczak VRR, Mullineaux LSS, Adams D, Walther B, Elsdon T, Cohen A, Leavitt D (2007) Laser ablation ICP-MS analysis of larval shell in softshell clams (Mya arenaria) poses challenges for natural tag studies. Limnology and Oceanography: Methods 5, 241-249.
| Crossref | Google Scholar |
Suchley A, Alvarez-Filip L (2018) Local human activities limit marine protection efficacy on Caribbean coral reefs. Conservation Letters 11(5), e12571.
| Crossref | Google Scholar |
Swearer SE, Caselle JE, Lea DW, Warner RR (1999) Larval retention and recruitment in an island population of a coral-reef fish. Nature 402, 799-802.
| Crossref | Google Scholar |
Thébault J, Chauvaud L, L’Helguen S, Clavier J, Barats A, Jacquet S, Pécheyran C, Amouroux D (2009) Barium and molybdenum records in bivalve shells: geochemical proxies for phytoplankton dynamics in coastal environments? Limnology and Oceanography 54, 1002-1014.
| Crossref | Google Scholar |
Thorrold S, Zacherl D, Levin L (2007) Population connectivity and larval dispersal using geochemical signatures in calcified structures. Oceanography 20, 80-89.
| Crossref | Google Scholar |
Toone TA, Benjamin ED, Hillman JR, Handley S, Jeffs A (2023) Multidisciplinary baselines quantify a drastic decline of mussel reefs and reveal an absence of natural recovery. Ecosphere 14(3), e4390.
| Crossref | Google Scholar |
Vavrek MJ (2011) fossil: palaeoecological and palaeogeographical analysis tools. Palaeontologia Electronica 14, 1T Available at http://palaeo-electronica.org/2011_1/238/index.html.
| Google Scholar |
Venables WN, Ripley BD (2002) ‘Modern applied statistics with S’, 4th edn. (Springer: New York, NY, USA) 10.1007/978-0-387-21706-2
Walters LJ, Wethey DS (1996) Settlement and early post-settlement survival of sessile marine invertebrates on topographically complex surfaces:the importance of refuge dimensions and adult morphology. Marine Ecology Progress Series 137, 161-171.
| Crossref | Google Scholar |
Watson JR, Siegel DA, Kendall BE, Mitarai S, Rassweiller A, Gaines SD (2011) Identifying critical regions in small-world marine metapopulations. Proceedings of the National Academy of Sciences of the USA 108(43), E907-E913.
| Crossref | Google Scholar |
Wei K, Wood AR, Gardner JPA (2013) Population genetic variation in the New Zealand greenshell mussel: locus-dependent conflicting signals of weak structure and high gene flow balanced against pronounced structure and high self-recruitment. Marine Biology 160, 931-949.
| Crossref | Google Scholar |
White JW, Ruttenberg BI (2007) Discriminant function analysis in marine ecology:some oversights and their solutions. Marine Ecology Progress Series 329, 301-305.
| Crossref | Google Scholar |
Wilson CJN, Rowland JV (2016) The volcanic, magmatic and tectonic setting of the Taupo Volcanic Zone, New Zealand, reviewed from a geothermal perspective. Geothermics 59, 168-187.
| Crossref | Google Scholar |
Wu W, Chaput R, Lundquist CJ, Montaño Orozco MM, Jeffs AG (2024) Tracking the source of wild mussel spat for aquaculture using shell microchemistry and biophysical models. Aquaculture 578, 740025.
| Crossref | Google Scholar |
Zacherl DC (2005) Spatial and temporal variation in statolith and protoconch trace elements as natural tags to track larval dispersal. Marine Ecology Progress Series 290, 145-163.
| Crossref | Google Scholar |
Zacherl DC, Manríquez PH, Paradis G, Day RW, Castilla JC, Warner RR, Lea DW, Gaines SD (2003) Trace elemental fingerprinting of gastropod statoliths to study larval dispersal trajectories. Marine Ecology Progress Series 248, 297-303.
| Crossref | Google Scholar |