ACCESS-S2 seasonal forecasts of rainfall and the SAM–rainfall relationship during the grain growing season in south-west Western Australia
Rebecca Firth

A
B
C
D
Abstract
South-west Western Australia (SWWA) is home to a world class grains industry that is significantly affected by periods of drought. Previous research has shown a link between the Southern Annular Mode (SAM) and rainfall in SWWA, especially during winter months. Hence, the predictability of the SAM and its relationship to SWWA rainfall can potentially improve forecasts of SWWA drought, which would provide valuable information for farmers. In this paper, focusing on the 0-month lead time forecast, we assess the bias and skill of ACCESS-S2, the Australian Bureau of Meteorology’s current operational sub-seasonal to seasonal forecasting system, in simulating seasonal rainfall for SWWA during the growing season (May–October). We then analyse the relationship between the SAM and SWWA precipitation and how well this is captured in ACCESS-S2 as well as how well ACCESS-S2 forecasts the monthly SAM index. Finally, ACCESS-S2 rainfall forecasts and the simulation of SAM are assessed for a case study of extreme drought in 2010. Our results show that forecasts tend to have greater skill in the earlier part of the season (May–July). ACCESS-S2 captures the significant inverse SAM–rainfall relationship but underestimates its strength. The model also shows overall skill in forecasting the monthly SAM index and simulating the MSLP and 850-hPa wind anomaly patterns associated with positive and negative SAM phases. However, for the 2010 drought case study, ACCESS-S2 does not indicate strong likelihoods of the upcoming dry conditions, particularly for later in the growing season, despite predicting a positive (although weaker than observed) SAM index. Although ACCESS-S2 is shown to skillfully depict the SAM–SWWA rainfall relationship and generally forecast the SAM index well, the seasonal rainfall forecasts still show limited skill. Hence it is likely that model errors unrelated to the SAM are contributing to limited skill in seasonal rainfall forecasts for SWWA, as well as the generally low seasonal-timescale predictability for the region.
Keywords: ACCESS-S2, agriculture, Bureau of Meteorology, model evaluation, rainfall, seasonal climate forecasting, Southern Annular Mode, south-west Western Australia, wheatbelt.
1.Introduction
Australia is home to a significant grains industry that accounts for 34% of the nation’s total gross agricultural value (see the Grains Research and Development Corporation at https://grdc.com.au/about/our-industry, accessed 29 August 2024), and one of the key production regions is located in south-west Western Australia (SWWA, location shown in Fig. 1a). Grain production in SWWA is a significant driver of Western Australia’s (WA’s) economy contributing A$2 billion–A$5 billion annually (Department of Primary Industries and Regional Development 2023). The SWWA grain-growing season runs from May to October and depends heavily on rainfall for productivity, hence, episodes of drought can be particularly detrimental to crop yield and result in major losses for farmers (Stephens and Lyons 1998; Roy et al. 2021; Bourne et al. 2023). SWWA has a Mediterranean-type climate characterised by hot, dry summers and cool, wet winters with 80% of annual rainfall occurring during May–October (Wright 1974). The majority of rainfall over these months in SWWA has been attributed to frontal systems and cut off lows (Pook et al. 2012).
(a) Map showing Western Australia’s wheatbelt in brown, as defined by the ‘cropping’ category (Department of Agriculture 2019), and (b) map showing the impact of the 2010 drought on grain production in SWWA with reference to the 2006–2009 average (Department of Primary Industries and Regional Development 2018).
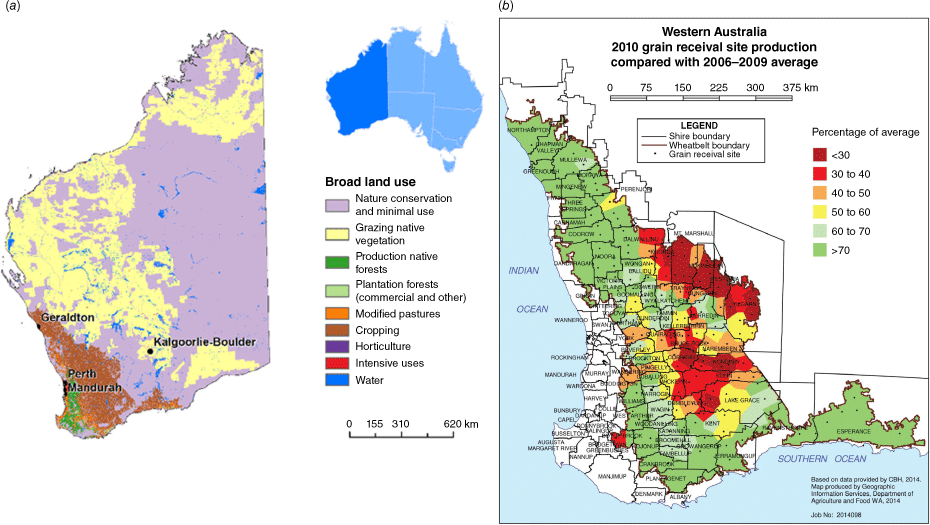
The year 2010 was one of the driest years on record for SWWA, with some weather stations recording as little as 39% of the long-term average for winter rainfall (Bureau of Meteorology 2010). Many growers suffered a significant decrease in grain production resulting in considerable financial loss. This is illustrated in Fig. 1b (Department of Primary Industries and Regional Development 2018), which shows the substantial decrease in yield for WA farmers as a result of the 2010 drought. Inland areas to the east of the wheat growing region, commonly referred to as the wheatbelt, were most severely affected with some areas experiencing less than 30% of the 2006–2009 average yield. The dry conditions in SWWA were driven by a persistent region of anomalously high pressure, fewer than average cold fronts and a lack of westerly winds during the growing season (Bureau of Meteorology 2010; Ganter 2011). As well as being one of the driest years on record, 2010 coincided with what was at that time, the highest recorded positive Southern Annular Mode (SAM) index for the June–August average, based on the record of more than 50 years (Blunden et al. 2011; Cai et al. 2011; Feng et al. 2015).
The SAM is a natural mode of climate variability that can significantly influence rainfall in the southern hemisphere (Thompson and Wallace 2000; Cai et al. 2011; Ho et al. 2012; Philip and Yu 2021). It represents the north–south movement of the westerly wind belt in the middle to higher latitudes in the southern hemisphere (Thompson and Wallace 2000). During the positive phase of the SAM, anomalously high pressure tends to dominate the mid-latitudes while anomalously low pressure dominates the high latitudes, and the westerly wind belt tends to shift southward. During the negative phase, this pattern is reversed.
Several studies have explored the link between the SAM and SWWA winter rainfall variability. Feng et al. (2010) used reanalysis and observations from 1948 to 2007 to investigate the influence of the SAM on SWWA rainfall. Their results suggested that the previously established inverse relationship between SAM and SWWA rainfall deteriorated once the year 1964, which was an extremely wet year, was removed from the dataset. However, this was refuted by Cai et al. (2011), who investigated the influence of SAM on SWWA winter rainfall (June–August) using satellite-era data for 1979–2010. Their results showed a significant relationship between SAM and SWWA rainfall. Specifically, when the SAM was positive, the number of synoptic systems that drove the rainfall was reduced in the region, having shifted poleward, and SWWA rainfall was lower (Cai et al. 2011). Similarly, Hendon et al. (2007) investigated the influence of the SAM on daily rainfall variability across Australia and demonstrated that a positive SAM was associated with a poleward shift in the rain-bearing westerlies across southern Australia, resulting in a reduction in winter rainfall in SWWA. Their results showed that the SAM explains 10–15% of the variability in SWWA weekly rainfall during winter. In a systematic review of the literature, McKay et al. (2023) evaluated observational studies from 1979 onwards to summarise and identify knowledge gaps in how changes in weather systems and large-scale climate drivers are linked to rainfall changes in the southern hemisphere. They found common threads in the reviewed literature, which showed that the impacts of the SAM on southern hemisphere rainfall are season-dependent, with the most robust relationship evident in winter, when a positive SAM is associated with reduced winter rainfall (refer to fig. 4 of McKay et al. 2023). Their literature review provides further support for the link between the positive phase of the SAM and reduced SWWA rainfall reported by Hendon et al. (2007) and Cai et al. (2011).
Accurate and reliable seasonal forecasts of SWWA rainfall can provide valuable information to growers upon which key management decisions are based, such as when to sow crops and how much fertiliser to apply and when (Born et al. 2021). Being equipped with this information can allow farmers to maximise profits in high-rainfall seasons and save costs when conditions are dry (Parton et al. 2019). The observed link between SAM and SWWA rainfall suggests that accurate forecasts of SAM could serve as a valuable indicator for predicting rainfall patterns in the SWWA region.
The Australian Bureau of Meteorology (hereafter the Bureau) provides operational forecasts of key climate drivers, including the phase of the El Niño–Southern Oscillation (ENSO), Indian Ocean Dipole (IOD) and the SAM, as well as sub-seasonal and seasonal forecasts of Australian climate (http://www.bom.gov.au/climate/ahead/). The first system in operation was the Predictive Ocean Atmosphere Model for Australia (POAMA; Alves et al. 2003; Charles et al. 2015), followed by the Australian Community Climate and Earth-System Simulator – Seasonal system (ACCESS-S1; Hudson et al. 2017), and continuing with ACCESS-S2 (Wedd et al. 2022). ACCESS-S2 became operational in October 2021 and is based on the UK Met Office Global Seasonal forecast system version 5 using the Global Coupled model configuration 2 (GloSea5-GC2; MacLachlan et al. 2015). It has been upgraded from ACCESS-S1 to include a new Bureau-developed weakly coupled data assimilation system to provide initial conditions for atmosphere, ocean, land and ice fields. Additionally, the hindcast configuration has been updated and the time period extended to 38 years as opposed to 23 years in ACCESS-S1 (Wedd et al. 2022).
Previous work evaluated the Bureau’s forecasting systems in the simulation and prediction of the SAM and its relationship to Australian sub-seasonal climate in POAMA and ACCESS-S. In POAMA, Marshall et al. (2012) found that improved winter and spring rainfall forecasts were linked to skilful prediction of the SAM index and the accurate simulation of SAM-associated climate anomalies over Australia. Additionally, impacts from ENSO on the SAM–rainfall relationship were only evident during spring and summer and only in the northern part of Australia (Marshall et al. 2012). Wedd et al. (2022) assessed the forecast performance of ACCESS-S2 in comparison to ACCESS-S1. The results showed some improvement in ACCESS-S2 for seasonal rainfall forecasts during the growing season in SWWA (refer to their fig. 24, middle panel). Increased skill was most prominent during the first part of the season (May–July). The authors indicated that this could be due to the improved soil moisture initialisation in ACCESS-S2 (Wedd et al. 2022). The prediction of the SAM at sub-seasonal and seasonal time-scales was similar for ACCESS-S1 and ACCESS-S2; however, some improvements were identified in seasonal mean SAM forecasts with ACCESS-S2 initialised in May–August (Wedd et al. 2022).
In our recent study, ACCESS-S1 seasonal rainfall forecasts were evaluated over the grain-growing season in SWWA (Firth et al. 2023). Forecasts of 3- and 6-months duration were assessed at 0- and 1-month lead-times. Limitations in the model’s ability to accurately predict rainfall for these extended forecast periods were identified. It was shown that ACCESS-S1 had a tendency to forecast close to average conditions during both extreme wet and dry years. Building on Firth et al. (2023), the aim of this study is to evaluate the skill of ACCESS-S2 in forecasting seasonal rainfall over the growing season in SWWA, and in particular in association with the SAM. As there was limited skill for seasonal forecasts of 6-months duration (Firth et al. 2023), this study focuses on forecasts of 3-months duration. The skill of these seasonal forecasts is assessed using several forecast verification measures, and a probabilistic approach is adopted by evaluating above-median forecasts (a key forecast product for Bureau services: http://www.bom.gov.au/climate/ahead/). Additionally, the relationship between the SAM and SWWA rainfall and its simulation in ACCESS-S2 is explored, including a case study of 2010, one of the driest years on record for SWWA.
2.Methods
2.1. Data
The ACCESS-S2 forecast system includes a coupled atmosphere–ocean–land surface model, which is based on the UK Met Office’s global coupled model seasonal forecast system, GloSea5-GC2 (MacLachlan et al. 2015). It comprises the Unified Model (UM; Williams et al. 2015; Walters et al. 2017) for the atmosphere, the Joint UK Land Environment Simulator (JULES; Best et al. 2011; Walters et al. 2017), the Nucleus for European Modelling of the Ocean (NEMO; Madec et al. 2008; Megann et al. 2014), and the Los Alamos sea ice model (CICE; Hunke and Lipscomb 2008). ACCESS-S2 has a horizontal resolution of ~60 km (N216) with 85 vertical levels in the atmosphere, four soil levels in the land surface model, and an ocean model resolution of 0.25° with 75 vertical levels.
The ACCESS-S2 forecasts are evaluated based on a set of retrospective forecasts known as hindcasts. The initial conditions for the hindcasts come from the reanalysis produced from the Bureau’s new data assimilation system (Wedd et al. 2022). The ACCESS-S2 hindcasts are available for the period 1981–2018 and have a seasonal hindcast integration length of 279 days. The ensemble configuration takes a time-lagged approach so that the number of ensemble members is dependent on the start date of the hindcast. The present study uses a time-lagged 21-member ensemble comprising three ensemble members, each initialised on the 1st of the month and 6 consecutive days prior (note: in the text above, the nominal start date of the 1st of the month refers to the full time-lagged ensemble). Creation of a time-lagged ensemble is common practice in seasonal prediction to increase ensemble size and better capture uncertainties. For example, the Bureau’s real-time forecast products utilise a time-lagged ensemble by combining 9 successive days of forecasts for the seasonal timescale (11 members are run every day, thus this approach builds a 99-member ensemble; Hudson et al. 2017; Wedd et al. 2022).
To assess ACCESS-S2 rainfall, the ACCESS-S2 seasonal forecasts were compared to observed seasonal rainfall calculated from monthly 5-km gridded observations from the Australian Gridded Climate Data (AGCD; Evans et al. 2020). This dataset is an interpolation of direct surface measurements recorded from a network of weather stations across Australia. The number of stations recording data varies in time and by variable, and precipitation is interpolated from 5000–7000 stations across Australia (Evans et al. 2020). The AGCD dataset is commonly used for model evaluation purposes for studies focusing on Australia (e.g. Lim et al. 2021; Zhao et al. 2021; Shao et al. 2022; Firth et al. 2023). The AGCD precipitation data for 1981–2018 were re-gridded to the 60-km ACCESS-S2 grid using the first-order conservative remapping tool, remapcon, from Climate Data Operators (https://code.mpimet.mpg.de/projects/cdo/), to enable comparison of the ACCESS-S2 forecasts with observed precipitation. A land fraction of 0.3 was used as the threshold to determine the land–sea mask.
To evaluate the relationship between the SAM and SWWA rainfall, the European Centre for Medium-Range Weather Forecasts (ECMWF) Reanalysis (ver. 5, ERA5; Hersbach et al. 2020) was used as a surrogate truth for the SAM index, mean sea level pressure (MSLP) and 850-hPa wind. The ERA5 is the fifth-generation reanalysis produced by the Copernicus Climate Change Service (C3S) at ECMWF, replacing and improving upon the previous ERA-Interim reanalysis (Dee et al. 2011). It has a horizontal resolution of ~30 km and there are 137 vertical levels from the surface up to 0.01 hPa. Reanalyses are commonly used as surrogate truth for model evaluations of the SAM (e.g. Meneghini et al. 2007; Zhang et al. 2018; Ibebuchi 2021). The MSLP and 850-hPa wind data were extracted directly from the ERA5 for 1981–2018, and monthly SAM index values were derived according to Marshall et al. (2012).
2.2. Analysis
The initial analysis involved evaluating the rainfall forecasts in ACCESS-S2 for 1981–2018 and focused on four seasonal forecasts of 3 months duration during the grain-growing season: May–July (MJJ), June–August (JJA), July–September (JAS) and August–October (ASO). All forecasts have a 0-month lead-time (e.g. for evaluation of MJJ, the nominal start-date of the lagged ensemble was 1 May). The overall rainfall bias in ACCESS-S2 was initially assessed by computing the percentage difference between the model and the AGCD observed precipitation for each seasonal forecast. The model’s performance for predicting above or below-median rainfall was then evaluated for each season by computing four categorical statistics commonly used for verifying dichotomous (yes or no) forecasts (Wilks 2011). The Bureau routinely issues forecasts of probabilities above median and this dichotomous approach is consistent with other ACCESS-S1 or ACCESS-S2 forecast model evaluations (e.g. Hudson et al. 2017). To populate the required contingency table (Table 1), the probability forecast is first converted to a category forecast. If the forecast probability of above-median rainfall is greater (less) than 50%, then it is categorised as a ‘yes’ (‘no’) event in the contingency table (Hudson et al. 2017). To determine the outcome of a forecast, the ensemble is compared against the model’s climatological median, whereas the observed median is used to determine the observed outcome (this is standard practice for seasonal prediction).
Observed | |||||
---|---|---|---|---|---|
Yes | No | Total | |||
Forecast | Yes | Hits | False alarms | Forecast yes | |
No | Misses | Correct negatives | Forecast no | ||
Total | Observed yes | Observed no | Total |
The contingency table shows the frequency of ‘yes’ and ‘no’ forecasts and occurrences and are described as:
hit – event forecast to occur and did occur;
miss – event forecast not to occur but did occur;
false alarm – event forecast to occur but did not occur; and
correct negative – event forecast not to occur and did not occur.
Accuracy (fraction correct), bias score (frequency bias), probability of detection (hit rate) and false alarm ratio were then calculated, using the following equations, for 1981–2018:
The accuracy score (Eqn 1) measures the proportion of forecasts that were correct, i.e. how often ACCESS-S2 correctly forecast an above-median rainfall event, as well as correctly not forecasting an above-median rainfall event when one did not occur.
The bias score (Eqn 2) gives an indication of whether the model has a tendency to under-forecast (bias < 1) or over-forecast (bias > 1) events by measuring the ratio of the frequency of forecast events to the frequency of observed events.
The POD (Eqn 3) measures the fraction of observed above-median rainfall events that were correctly forecast (hits) and the FAR (Eqn 4) indicates the fraction of above-median rainfall event forecasts that were false alarms. The two metrics are intended to be used in conjunction with each other.
The first step was to explore the relationship between the SAM from ERA5 and observed SWWA precipitation from AGCD. To first establish the relationship from best-available observations or reanalysis, no interpolation of ERA5 or AGCD was carried out, i.e. both datasets were kept on their native resolutions. Because the SAM has a typical decorrelation time of ~1–2 weeks (Hendon et al. 2007), the SAM analysis focused on monthly rather than seasonal timescales. The analysis involved computing the Pearson correlation coefficient for the relationship between AGCD SWWA monthly precipitation anomalies and the monthly ERA5 SAM index for the period available from the ACCESS-S2 hindcasts (1981–2018). The correlation was assessed at each grid point individually, and significance of the correlation was determined using a two-sided P-value (refer to https://docs.scipy.org/doc/scipy/reference/generated/scipy.stats.pearsonr.html for more details). This relationship was then examined in ACCESS-S2 for 0-month lead forecasts to assess how well the model simulates the SAM–rainfall relationship. For this analysis, all the ensembles for each year were included for the correlation analysis rather than the ensemble mean.
To assess ACCESS-S2’s forecast skill of the SAM, the monthly SAM index from ERA5 was compared with simulated ensemble mean, minimum and maximum monthly SAM from ACCESS-S2 for the respective months of the growing season (for 1981–2018). The assessment was carried out for 0-month lead forecasts of May–October (nominally initialised on the 1st of each month). The Pearson correlation and significance of the correlation between ERA5 and ACCESS-S2 ensemble mean SAM was computed for each month analysed. To further explore how well ACCESS-S2 captures the SAM signal, composites of MSLP and 850-hPa wind anomalies for positive and negative monthly SAM during the growing season were compared between ERA5 and the model. Throughout the study, a positive SAM event is associated with SAM index values greater than 1 and a negative SAM event is associated with a SAM index less than −1 (Marshall et al. 2012). We note that variations in ENSO have been shown to only influence the SAM–rainfall signal in northern parts of Australia and only during spring and summer (Marshall et al. 2012). In our study the effects of ENSO have not been removed since we focus on May–October rainfall in SWWA.
The year 2010 was selected as a case study due to the extreme drought during the growing season and the associated highly positive SAM. Initially, the 2010 seasonal rainfall anomalies were compared between observations (AGCD) and ACCESS-S2. Anomalies for the ACCESS-S2 hindcasts were calculated by first computing the climatology (1981–2018) from the ensemble mean hindcasts for each start date, and the climatology was then subtracted from the ensemble mean for each start date to produce the forecast anomalies. In doing so, a first-order linear correction for model bias or drift is made (Stockdale 1997). The anomalies are expressed as a percentage difference from normal rather than the absolute difference to allow for a more accurate comparison. The probability of above-median rainfall seasonal forecasts was then evaluated in ACCESS-S2. Subsequently, ACCESS-S2 skill in forecasting the monthly SAM index in 2010 at 0-month lead time was assessed, with a time series comparing observed (reanalysis) SAM from ERA5 with the forecast SAM for each month of the growing season at 0-month lead time.
To identify differences in individual ensemble members and test whether members that simulated dry conditions were associated with positive SAM, the ensembles for the 2010 rainfall forecast were ranked based on their domain sum of monthly rainfall for each month at 0-month lead time. Composites of the top and bottom tercile rainfall anomalies were then plotted with the corresponding mean SAM index and this analysis was then repeated for MSLP and 850-hPa wind anomalies. The observed (reanalysis) monthly MSLP and 850-hPa wind anomalies were also compared with ACCESS-S2 monthly ensemble mean MSLP and 850-hPa wind anomalies.
3.Results and discussion
3.1. ACCESS-S2 precipitation bias and forecast skill
The initial analysis focused on evaluating the overall precipitation bias and forecast skill for SWWA rainfall in ACCESS-S2. Fig. 2 shows the observed average precipitation and bias (ACCESS-S2 minus observations, expressed as a percentage) for the four seasonal forecasts over the growing season for 1981–2018. A pronounced gradient is evident in observed rainfall, with notably higher rainfall along the south-west coast. For all seasonal forecasts, this part of the region has a strong negative bias that is most pronounced in the south-west corner. The bias becomes slightly positive inland from the coast, and minimal in the north-east of the region. This is very similar to our previous work, Firth et al. (2023), which evaluated ACCESS-S1 SWWA seasonal rainfall forecasts for July–September and May–October. While biases inland were minimal in ACCESS-S1, the higher rainfall observed in the south-west corner was not captured by the model (Firth et al. 2023).
Observed precipitation (top panel) and precipitation bias (model minus observations expressed as a percentage, bottom panel) for respective 0-month lead ACCESS-S2 seasonal forecasts (3-month periods) over the growing season (May–October), averaged for 1981–2018.
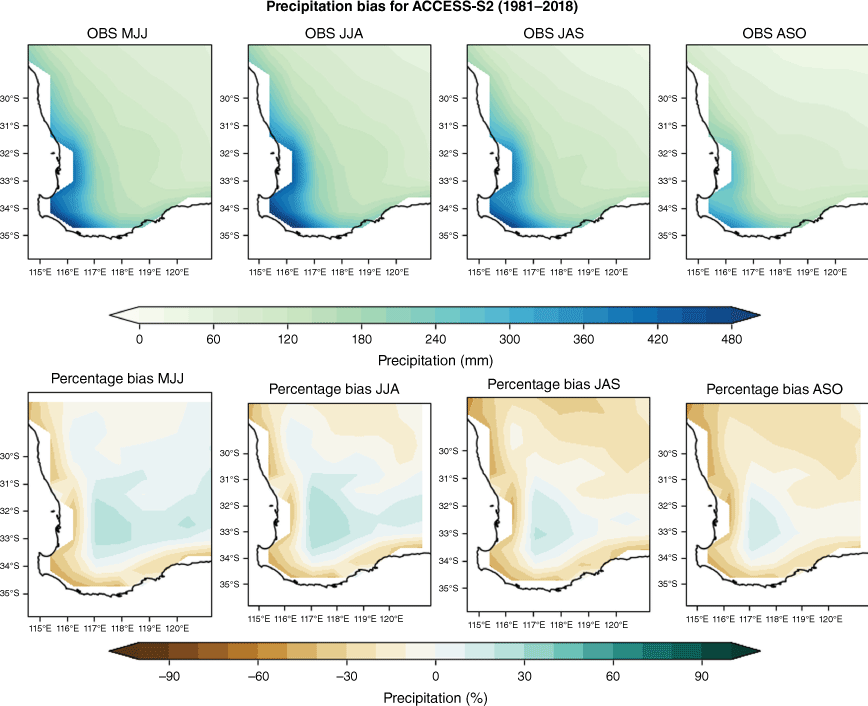
Fig. 3 shows the skill assessment for ACCESS-S2 for seasonal forecasts of above-median rainfall (refer to methods). Results show that ACCESS-S2 generally made correct forecasts for all seasons more than 50% of the time across most of the region (note that accuracies greater than 50% are better than a climatological forecast) (Fig. 3a). The MJJ forecast is the most skilful, with ACCESS-S2 achieving greater than 80% correct forecasts for a large proportion of the inland region. The ASO forecast is the weakest, with only ~50% of correct forecasts across most of the region and less than 50% across the north-east. Similarly, the bias is generally smallest earlier in the growing season (MJJ and JJA) with a slight tendency to over-forecast above-median rainfall (Fig. 3b). Later in the season (JAS and ASO), the bias is slightly increased inland and there is more of a tendency to under-forecast above-median rainfall.
Skill assessment of ACCESS-S2 for above-median seasonal rainfall forecasts for four seasons (0-month lead forecasts) within the growing season (1981–2018): (a) accuracy, (b) bias score, (c) probability of detection and (d) false alarm ratio.
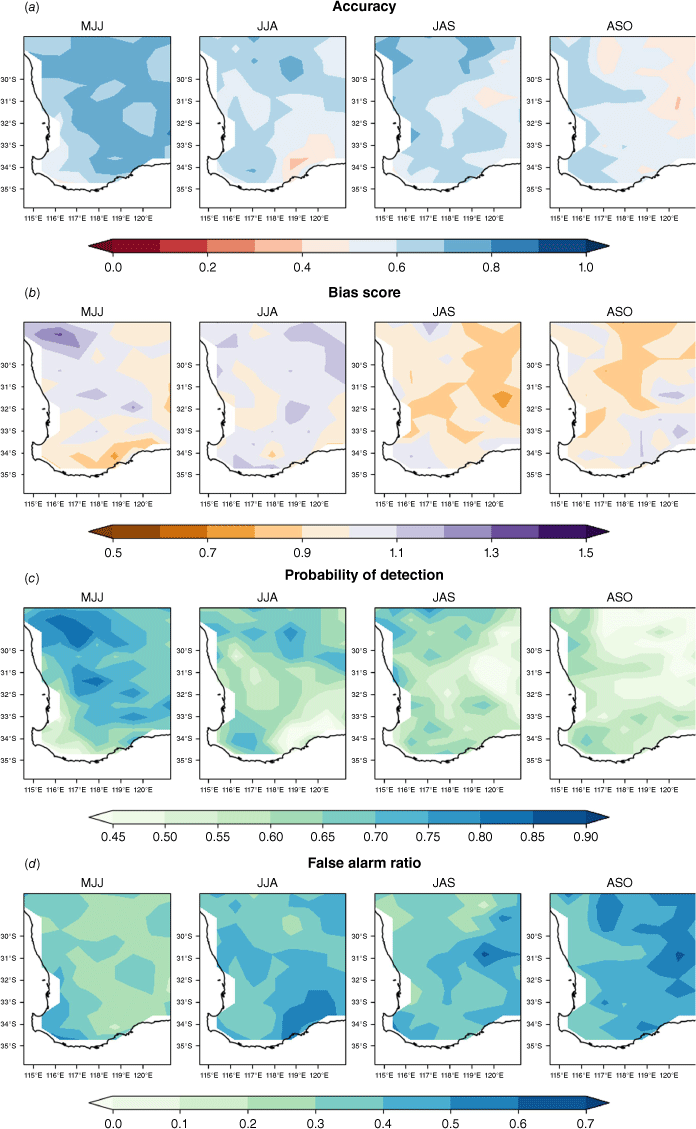
Figure 3c and 3d show the POD and FAR respectively for above-median rainfall events in ACCESS-S2. For ACCESS-S2 seasonal forecasts over the grain growing season, the signal in the two metrics is almost opposite to each other. Early in the season there is a higher hit rate (higher POD) and lower number of false alarms (lower FAR), and this is reversed later in the season. These results are also indicative of a more skilful rainfall forecast in the earlier part of the growing season.
In summary, the four metrics assessed here indicate that ACCESS-S2 generally performs better for above-median forecasts of SWWA growing-season rainfall earlier in the season, particularly for MJJ. When evaluating improvements in ACCESS-S2 compared with ACCESS-S1, Wedd et al. (2022) found that seasonal rainfall forecast performance was most improved in SWWA for the MJJ season. This was attributed to improvements in soil moisture initialisation based on a sensitivity analysis conducted by Zhao et al. (2017). Their research showed that, although the impacts of soil moisture are most pronounced for surface temperature forecasts, there is also an influence on precipitation forecast skill through complex processes that involve surface evaporation and interactions with boundary layer processes (Zhao et al. 2017).
3.2. SAM–rainfall analysis
We first focus on the SAM and SWWA rainfall relationship from best available re-analysis and observations. This is illustrated in Fig. 4 (top row), showing an inverse relationship in the south-west corner for all months, although for October this relationship is only evident across a very small area. For June and July, the inverse relationship is evident across most of the region and is significant in the south-west coast and partly inland for July. These results show a significant inverse relationship between SWWA rainfall and the SAM only across a very small part of the region and only for 2 months of the growing season. These findings corroborate previous findings of a positive SAM during winter resulting in reduced rainfall for SWWA (Hendon et al. 2007; Cai et al. 2011). Furthermore, these results also emphasise the seasonality of the relationship between the SAM and SWWA rainfall, showing that it is most pronounced during the winter months (e.g. Cai and Cowan 2006; McKay et al. 2023). Thus, the influence of a skilful simulation and prediction of the SAM on the accuracy of SWWA rainfall forecasts throughout the growing season may be limited to rainfall forecasts in winter only. The inverse relationship between the SAM and SWWA rainfall is also captured in ACCESS-S2, but the relationship is weaker in the model (Fig. 4, bottom row). This weaker than observed relationship has also been seen between the SAM and south-eastern Australian rainfall (Lim et al. 2016). It is also present in free-running present-day climate simulations using the same coupled model as used in ACCESS-S2, but there are some indications of a stronger, improved relationship in winter (JJA) in more recent model configurations (Li et al. 2023, their fig. 16). Reasons for the weaker than observed relationship are not clear.
Pearson’s correlation for precipitation and SAM in SWWA for ERA5/AGCD (top panel) and ACCESS-S2 (bottom panel). Stippling shows where correlation is significant at P < 0.05 (1981–2018).
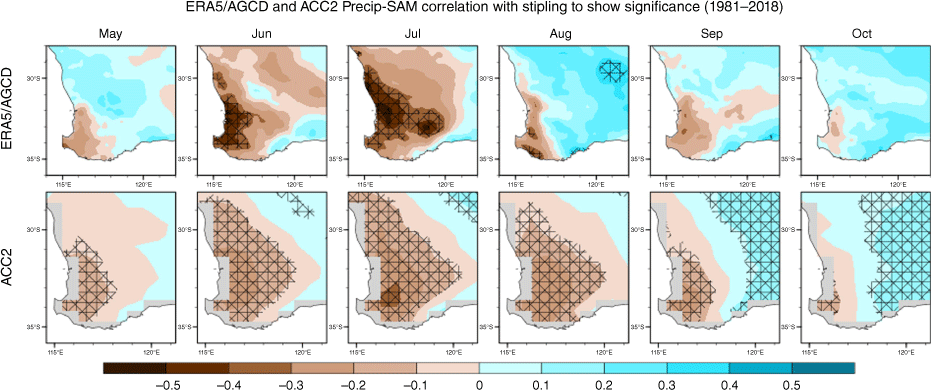
A significant inverse relationship in the south-west corner is shown for all months in ACCESS-S2 compared to only June and July in ERA5 and AGCD (Fig. 4, top row). ACCESS-S2 also shows a significant positive relationship across the east of the region in September and October that is not evident in ERA5 and AGCD. Rainfall forecasts may be improved earlier in the season due to the relationship between SAM and precipitation being stronger for these months.
Figures 5 and 6 compare the composites of MSLP and 850-hPa wind anomalies between ERA5 and ACCESS-S2 for positive and negative SAM events respectively. During positive SAM events, ERA5 shows the expected pattern of anomalously high pressure across southern Australia and the associated westerly wind belt further south is evident (Fig. 5, top panel). The positive MSLP anomaly is most pronounced during July and August. During negative SAM years, the ERA5 composites show what would be expected with anomalously low pressure across southern Australia and a shift of the westerly winds further north (Fig. 6, bottom panel). The low MSLP anomaly over Australia is most prominent in June and August. The ACCESS-S2 composites show that the model broadly captures these patterns well for both the positive and negative SAM phases and for all months during the growing season. However, there are some notable differences (Fig. 5 and 6 bottom panels). During positive SAM events, although ACCESS-S2 simulates a region of anomalously high pressure to the south-west of Australia, it is underestimated compared to what was observed for May, July and August. It is also underestimated west of Australia and over SWWA in October. Additionally, the anomalous easterly winds across large parts of Australia during August–October in ERA5 are weaker and more zonal in ACCESS-S2, particularly for September and October. The anomalously westerly winds observed across Australia for June are also notably underestimated by ACCESS-S2 (Fig. 5). For the negative SAM events, ACCESS-S2 overestimates the negative MSLP anomaly (i.e. more negative) to the south-west of Australia in May, July, September and October (Fig. 6); for June and August, it is underestimated. For July, the positive MSLP anomaly south of Australia is further south in the model. It should also be noted that the sample size for ERA5 in this analysis is mostly less than 10, therefore some discrepancies between the model and observations (ERA5) may be attributed to this limitation in the methodology and thus caution is given to overinterpreting these results.
Composites of MSLP anomalies with 850-hPa wind vector anomalies overlain for positive SAM events in ERA5 (top), ACCESS-S2 (middle) and ACCESS-S2-minus-ERA5 (bottom) for 1981–2018. ACCESS-S2 composite case numbers are for all years and ensembles, and from the 0-month lead forecasts.
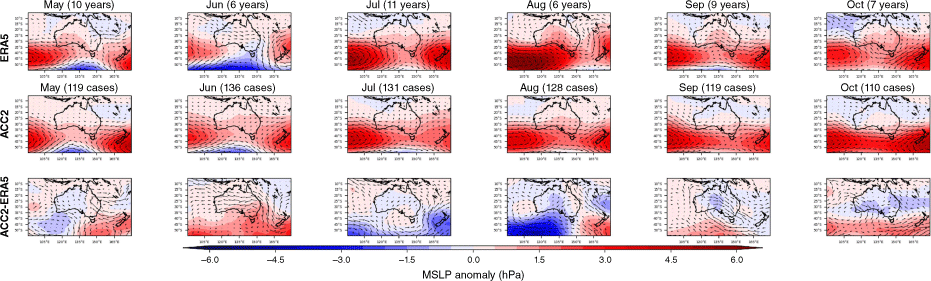
The next step focused on assessing the ability of ACCESS-S2 to predict the monthly SAM (verified against ERA5). This is illustrated in Fig. 7, which shows the 1981–2018 time series of the SAM index for months within the growing season for the ACCESS-S2 ensemble mean, minimum and maximum (0-month lead forecasts), and for ERA5. The correlation coefficient (r) and P-value are shown for each month. A significant positive correlation between the ACCESS-S2 ensemble mean SAM and ERA5 SAM was identified for all months of the growing season apart from June, suggesting that ACCESS-S2 generally performs well in forecasting the monthly SAM index (Fig. 7). The spread of the ensemble is quite large but the ERA5 SAM is consistently within the ensemble range for most months and years. However, the ERA5 SAM falls out of the ensemble range simulated by ACCESS-S2 during some of the more extreme positive or negative events (e.g. May 1989 and July 1995).
Time series showing ERA5 and the ACCESS-S2 ensemble minimum, maximum and mean SAM index for each month of the growing season (May–October, indicated in the respective rows), 1981–2018. The ACCESS-S2 forecasts are all 0-month lead forecasts verifying in the month shown in each panel. The correlation coefficient (r) and P-value are shown for each month.
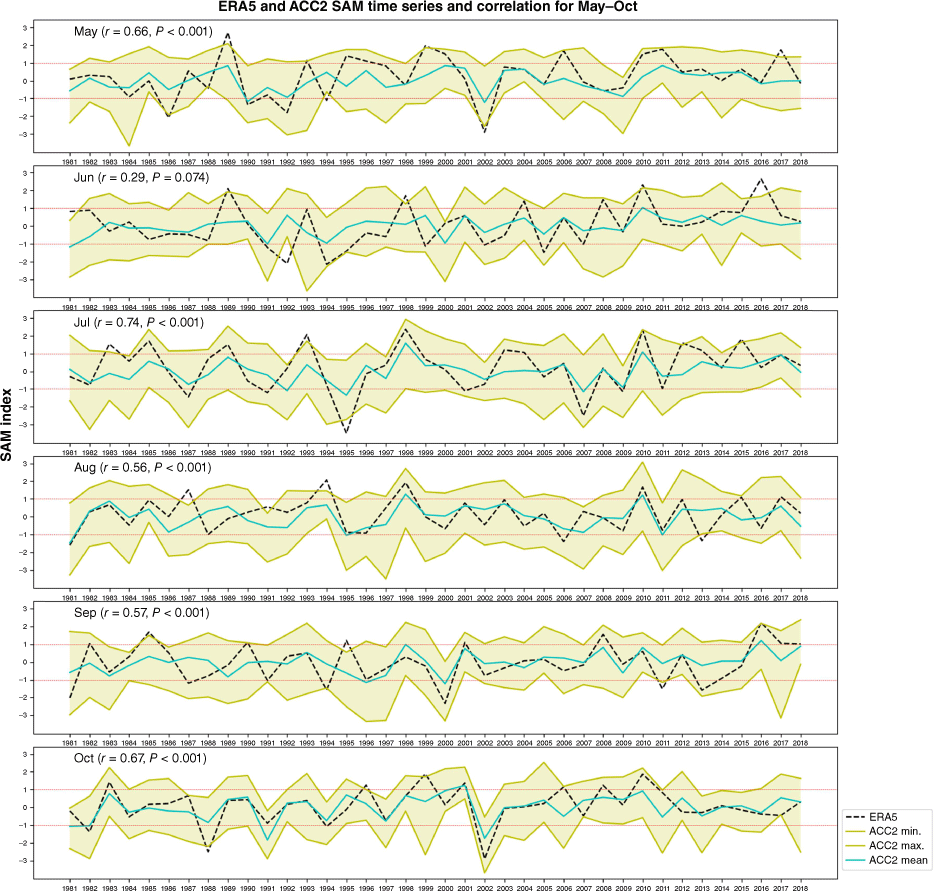
3.3. 2010 case study – a very dry year and a strong positive SAM
The observed seasonal precipitation anomaly for 2010 was well below average for all seasons across the majority of SWWA and particularly during the ASO season (Fig. 8a top panel). By comparison, the ACCESS-S2 ensemble mean anomaly (Fig. 8a, bottom panel) failed to capture the observed well-below average seasonal precipitation anomaly, with all seasons predicted to have close to average rainfall. The MJJ forecast is the closest to what was observed with a dry signal throughout the region, but it is only slightly drier than average. Fig. 8b shows ACCESS-S2’s probability of above-median rainfall for each season in 2010. An accurate forecast would suggest a very low probability of above-median rainfall; however, only for the MJJ forecast is the probability below 50% for most of the region. The JJA forecast also shows some skill for the SWWA corner. These results are consistent with our previous work focusing on ACCESS-S1, which showed that during extreme wet or dry years, ACCESS-S1 tends to forecast close to average conditions (Firth et al. 2023), showing that ACCESS-S2 is also limited in skill in predicting extreme rainfall during growing seasons in SWWA. It is noted that the forecast of above-median rainfall and negative anomalies over the north-west in MJJ (Fig. 8b) is likely due to this region receiving very low annual rainfall during the growing season so that a few rainfall events can result in the mean value being higher than the median. As a result, an above-median value (e.g. 0.1 mm) can be less than the mean and therefore present as a negative anomaly.
The 2010 seasonal precipitation anomaly (expressed as a percentage difference from normal) from (a) AGCD (top panel) and ACCESS-S2 (middle panel) and (b) ACCESS-S2 probability of above-median rainfall for 2010. The ACCESS-S2 seasonal forecasts are for a 0-month lead time.
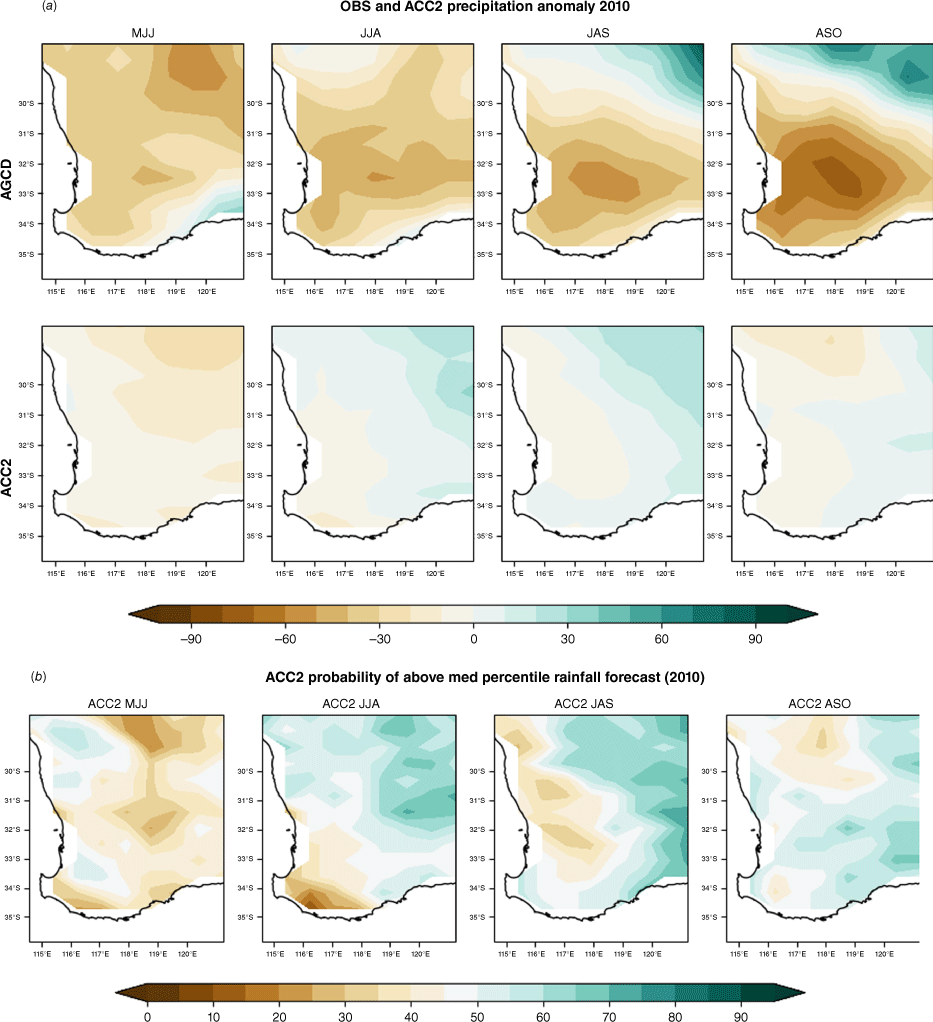
The time series of the ERA5 SAM index and ACCESS-S2 ensemble mean, minimum and maximum SAM index for the year 2010 was assessed to see how well ACCESS-S2 captured the monthly SAM index during the very dry year (Fig. 9). Note that the ACCESS-S2 forecasts are 0-lead forecasts for each month. The ensemble mean forecast of the SAM index is positive, as observed, for each respective month, and around or above the threshold of one for all months except May. However, the magnitude of the observed SAM is underestimated by the ensemble mean in most months (except August and September), and in general the forecast spread is large. This large spread is also evident across other years (Fig. 7) and indicates the need for a large ensemble to obtain the signal. Similarly large spread was seen in ACCESS-S1 forecasts of the SAM (D. Hudson, pers. comm., 12 July 2024) and is seen in NCEP forecasts of the SAM (https://www.cpc.ncep.noaa.gov/products/precip/CWlink/daily_ao_index/aao/aao_index_ensm.shtml).
Time series showing ERA5 and ACCESS-S2 minimum (min.), maximum (max.) and mean SAM for each month of the growing season in 2010. The ACCESS-S2 forecasts are 0-month lead time forecasts for each respective month.
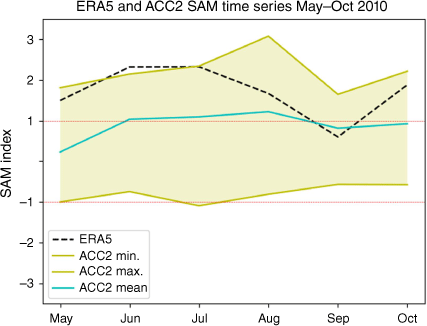
The findings from the analysis of SAM in ACCESS-S2 for 2010 somewhat contrast with what was identified in the evaluation of the precipitation forecasts: while the precipitation forecasts performed better earlier in the season (Fig. 8), forecasts of the SAM appears to be improved later in the season (Fig. 9) (although we note that the rainfall verification is for a seasonal average, whereas the SAM verification is for periods of a month).
We next stratified the respective monthly rainfall forecasts from the ensemble members into terciles for each 0-lead monthly forecast and computed the related SAM index for the stratification. The aim is to examine whether the ensemble members that forecast dryer conditions were the ones associated with stronger positive SAM values. The bottom tercile of ensemble members (for a given target month at 0-month lead), forecast dry conditions across all of the region for May–August and along the south-west for September and October, although the anomalies were notably smaller for the last 2 months of the season (Fig. 10, top panel). The top tercile was characterised by mostly wetter conditions, other than July, and the magnitude of the positive rainfall anomalies was generally smaller in comparison to the magnitude of the negative rainfall anomalies for the bottom tercile, with the exception of September and October when this was reversed (Fig. 10, bottom panel). All the mean SAM values were positive for both the top and bottom terciles. However, for some months (July and October), the mean SAM index was larger for the top tercile compared to the bottom rainfall tercile. This indicates that the SAM was not driving the rainfall response in these months. Additionally, the standard deviation of the SAM index in each sample is relatively large and often greater than the mean SAM (e.g. in May), suggesting a large spread across ensemble members in terms of whether the SAM was driving the rainfall response or not.
ACCESS-S2 composites (0-month lead monthly forecasts) of top and bottom terciles of ensemble members rainfall anomalies based on the domain sum of rainfall for 2010 in each month. The corresponding mean SAM index and standard deviation are given above each plot.
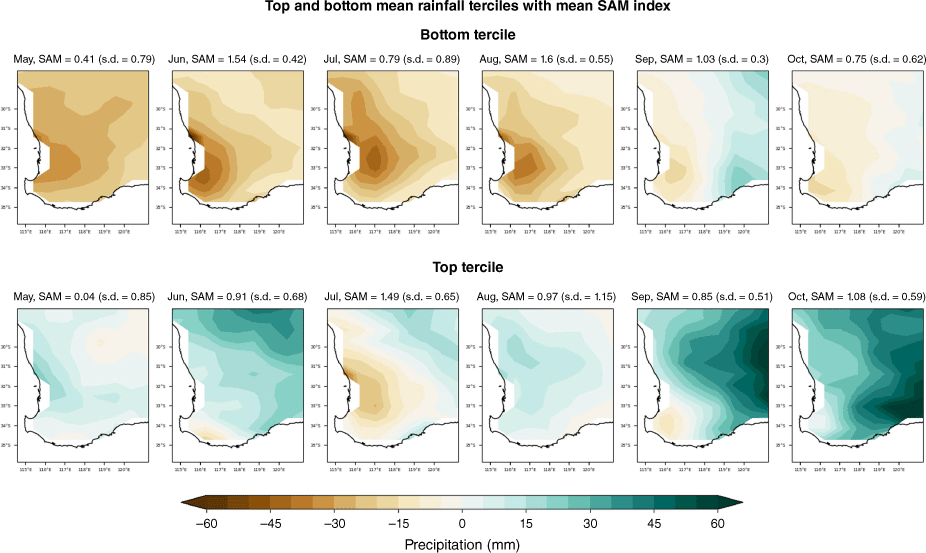
Fig. 11 (top panel) shows anomalously high MSLP for all months (except May) and anticyclonic flow across SWWA, which is characteristic of the positive SAM phase that occurred and conducive to the observed dry conditions. In June and July, the anomaly patterns were more zonally symmetric compared to the other months (Fig. 11, top panel), indicative of a higher SAM index (Fig. 9). In May and September, the observed anomaly pattern over the southern part of the domain was more meridional than in other months, which corresponds to the local minima in the SAM index (Fig. 9). The pattern of higher-than-normal sea-level pressure and anticyclonic anomalies over, and south-west of, SSWA was broadly captured by the ACCESS-S2 ensemble mean in each month. However, ACCESS-S2 also simulated anomalously high pressure across SWWA for May, where anomalously low pressure occurred in ERA5. The higher forecast skill for the 2010 MJJ season (compared to other seasons, Fig. 8) may be attributed to ACCESS-S2 incorrectly forecasting anomalously high MSLP across SWWA in May, thus producing a drier forecast that was more consistent with observations. ACCESS-S2 also forecast a weaker high-pressure anomaly than observed across SWWA for the months of June, July, September and October. During August, ACCESS-S2 underestimated the high pressure anomaly to the south-west of Australia and incorrectly forecasted a high pressure anomaly over south-eastern Australia.
ERA5 (top panel), ACCESS-S2 ensemble mean (middle panel) and ACC2-ERA5 (bottom panel) MSLP and 850-hPa wind anomalies for 2010.
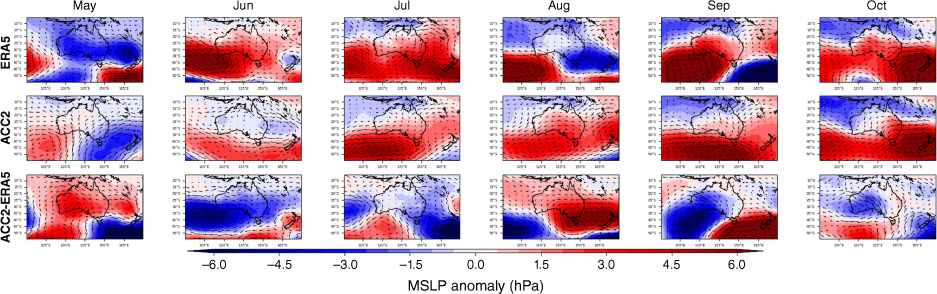
The MSLP and 850-hPa wind anomaly composite plots for the top and bottom ensemble rainfall terciles from ACCESS-S2 show mixed results (Fig. 12). For July, September and October, the top tercile plots show a stronger positive MSLP anomaly than the bottom tercile plots, and a stronger SAM index in July and October (as mentioned previously), which is contrary to what one would expect for the wetter tercile of ensemble members.
In summary, for this case study, the seasonal ACCESS-S2 rainfall forecasts are more accurate earlier in the growing season, e.g. forecasts for MJJ where the probabilistic forecasts provide some indication of the increased chance of dry conditions, although this signal is not particularly strong. In contrast, forecasts for the ASO season do not provide any indication of the upcoming dry conditions (Fig. 8). These results are somewhat aligned with the skill analysis across all years, which show a degradation in performance metrics as the growing season progresses (Fig. 3). The SAM was a key driver of the dry conditions in SWWA in 2010, being strongly positive. The 0-month lead ensemble mean forecasts of the SAM during the growing season predicted the positive SAM, albeit weaker than observed. However, while analysis of the forecast ensemble showed some indication of the SAM driving the rainfall response in some months, this was not evident in all months and there was a large spread in the results. This suggests that other possible deficiencies in the model are contributing to limitations in SWWA seasonal rainfall forecast skill in this case, rather than its simulation and prediction of the SAM and its relationship to SWWA rainfall.
4Conclusion
We evaluated the Bureau’s latest seasonal forecasting system ACCESS-S2 for rainfall forecasts in SWWA during the growing season. This included assessing the mean bias for all years and a case study of a very dry year. Given the link between the SAM and SWWA rainfall, we also evaluated the SAM–rainfall relationship in SWWA and how accurately this was captured in ACCESS-S2 as well as how well the model forecasts the SAM. Our results show an overall dry bias along the coast of SWWA for seasonal rainfall forecasts in SWWA and that seasonal forecasts earlier in the season tend to score higher for each of the verification metrics compared to later in the season, particularly for MJJ forecasts. We also demonstrated a significant inverse relationship between the SAM and SWWA rainfall for June and July that was captured in ACCESS-S2 but underestimated. In addition, the MSLP and 850-hPa wind anomalies associated with positive and negative SAM are well captured in ACCESS-S2. ACCESS-S2 forecast the observed SAM index well for 0-month lead monthly forecasts, demonstrated by a significant correlation between the forecast and ERA5 SAM for all months of the growing season apart from June.
Evaluation of a case study of 2010, which was an extremely dry growing season in SWWA and had strong positive SAM conditions, showed that ACCESS-S2 did not forecast the extent of the dry conditions, despite predicting a positive ensemble mean SAM index on a monthly basis. In addition, there was not a strong indication that SAM was driving the forecast across all months. Although ACCESS-S2 appears to capture the broad-scale patterns associated with positive and negative SAM events and provides reasonable forecasts of the SAM, it is important to remember that the SAM only accounts for ~10–15% of the variability in weekly rainfall for SWWA (Hendon et al. 2007). ACCESS-S2 is still limited in forecasting seasonal rainfall for SWWA, particularly during very dry years, which is similar to what was found in Firth et al. (2023) for ACCESS-S1. Previous work has shown that variability in sea surface temperature anomalies in the Indian Ocean affects SWWA rainfall, particularly during very wet or dry years (England et al. 2006; Samuel et al. 2006; Ummenhofer et al. 2008). While some improvements were shown in ACCESS-S2 compared to ACCESS-S1 by Wedd et al. (2022), the authors note that sea surface temperature biases in the Indian Ocean were still persistent in the ACCESS-S2 model, therefore these errors could be limiting the model’s forecasting ability for SWWA rainfall. In addition, SWWA exhibits less predictability on seasonal timescales compared to other regions due to the weaker association between SWWA rainfall and climate drivers such as ENSO (Smith et al. 2000).
Accurate and reliable seasonal forecasts are of paramount importance to a wide range of stakeholders. As such, several international initiatives provide accessible data from multi-model seasonal prediction systems, such as the C3S seasonal forecast service (https://www.ecmwf.int/en/forecasts/dataset/c3s-seasonal-forecasts). Such initiatives are a valuable resource for seasonal climate forecasting as they allow a comparison between different models and provide a multi-model ensemble prediction system, which has shown greater predictive skill than individual models (e.g. Gebrechorkos et al. 2022). Our future work will focus on how ACCESS-S2 compares to seasonal rainfall forecasts produced from climate models that contribute to C3S for the SWWA growing season.
Data availability
All datasets used as part of this study are available at the NCI (https://nci.org.au/). Scripts used in this study are available by request to the corresponding author.
Declaration of funding
Rebecca Firth is supported by a Research Training Program and top-up scholarship at Murdoch University. This research and project were undertaken with the assistance of resources and services from the National Computational Infrastructure (NCI), which is supported by the Australian Government. We thank the Australian Research Council Centre of Excellence for Climate Extremes and Prof. Andy Pitman for providing access to computer and data storage resources at the NCI. All this assistance is gratefully acknowledged.
Acknowledgements
This research and project were undertaken with the assistance of resources and services from the National Computational Infrastructure (NCI), which is supported by the Australian Government. We thank the Australian Research Council Centre of Excellence for Climate Extremes and Prof. Andy Pitman for providing access to computer and data storage resources at the NCI. All this assistance is gratefully acknowledged. Rebecca Firth is supported by an Australian Government Research Training Program Scholarship.
References
Alves O, Wang G, Zhong A, Smith N, Warren G, Marshall A, Tzeitkin A, Schiller A (2003) POAMA: Bureau of Meteorology operational coupled model seasonal forecast system. In ‘Proceedings of national drought forum’, 15 April 2003, Brisbane, Qld, Australia. pp. 49–56. (DPI Publications, Department of Primary Industries: Brisbane, Qld, Australia) Available at https://www.ecmwf.int/sites/default/files/elibrary/2003/7694-poama-bureau-meteorology-coupled-model-seasonal-forecast-system.pdf
Best M, Pryor M, Clark D, Rooney G, Essery R, Ménard C, Edwards J, Hendry M, Porson A, Gedney N (2011) The Joint UK Land Environment Simulator (JULES), model description – part 1: energy and water fluxes. Geoscientific Model Development 4(3), 677-699.
| Crossref | Google Scholar |
Blunden J, Arndt DS, Baringer MO (2011) State of the climate in 2010. Bulletin of the American Meteorological Society 92(6), S1-S236.
| Crossref | Google Scholar |
Born L, Prager S, Ramirez-Villegas J, Imbach P (2021) A global meta-analysis of climate services and decision-making in agriculture. Climate Services 22, 100231.
| Crossref | Google Scholar |
Bourne AR, Bruce J, Guthrie MM, Koh L-A, Parker K, Mastrantonis S, Veljanoski I (2023) Identifying areas of high drought risk in southwest Western Australia. Natural Hazards 118(2), 1361-1385.
| Crossref | Google Scholar |
Bureau of Meteorology (2010) Special climate statement 21. A very dry year so far in southwest Western Australia. (The Bureau of Meteorology) Available at http://www.bom.gov.au/climate/current/statements/scs21a.pdf
Cai W, Cowan T (2006) SAM and regional rainfall in IPCC AR4 models: can anthropogenic forcing account for southwest Western Australian winter rainfall reduction? Geophysical Research Letters 33(24), L24708.
| Crossref | Google Scholar |
Cai W, van Rensch P, Borlace S, Cowan T (2011) Does the Southern Annular Mode contribute to the persistence of the multidecade-long drought over southwest Western Australia? Geophysical Research Letters 38(14), L14712.
| Crossref | Google Scholar |
Charles AN, Duell RE, Wang X, Watkins AB (2015) Seasonal forecasting for Australia using a dynamical model: improvements in forecast skill over the operational statistical model. Australian Meteorological and Oceanographic Journal 65(3), 356-375.
| Crossref | Google Scholar |
Dee DP, Uppala SM, Simmons A, Berrisford P, Poli P, Kobayashi S, Andrae U, Balmaseda M, Balsamo G, Bauer P, Bechtold P, Beljaars ACM, van de Berg L, Bidlot J, Bormann N, Delsol C, Dragani R, Fuentes M, Geer AJ, Haimberger L, Healy SB, Hersbach H, Hólm EV, Isaksen L, Kållberg P, Köhler M, Matricardi M, McNally AP, Monge-Sanz BM, Morcrette J-J, Park B-K, Peubey C, de Rosnay P, Tavolato C, Thépaut J-N, Vitart F (2011) The ERA‐Interim reanalysis: configuration and performance of the data assimilation system. Quarterly Journal of the Royal Meteorological Society 137(656), 553-597.
| Crossref | Google Scholar |
Department of Agriculture (2019) About my region – Western Australia – Wheat Belt. Available at http://www.agriculture.gov.au/abares/research-topics/aboutmyregion/wa-wheat-belt#agricultural-sector [Verified 13 August 2019]
Department of Primary Industries and Regional Development (2018) The evolution of drought policy in Western Australia. Available at https://www.agric.wa.gov.au/drought-and-dry-seasons/evolution-drought-policy-western-australia?page=0%2C2
Department of Primary Industries and Regional Development (2023) Western Australian wheat industry. Available at https://www.agric.wa.gov.au/export-services/western-australian-wheat-industry [Verified 25 September 2023]
England MH, Ummenhofer CC, Santoso A (2006) Interannual rainfall extremes over southwest Western Australia linked to Indian Ocean climate variability. Journal of Climate 19(10), 1948-1969.
| Crossref | Google Scholar |
Evans A, Jones D, Smalley R, Lellyett S (2020) An enhanced gridded rainfall analysis scheme for Australia. Australian Bureau of Meteorology 66, 55-67.
| Google Scholar |
Feng J, Li J, Li Y (2010) Is there a relationship between the SAM and southwest Western Australian winter rainfall? Journal of Climate 23(22), 6082-6089.
| Crossref | Google Scholar |
Feng J, Li J, Li Y, Zhu J, Xie F (2015) Relationships among the monsoon-like southwest Australian circulation, the Southern Annular Mode, and winter rainfall over southwest Western Australia. Advances in Atmospheric Sciences 32(8), 1063-1076.
| Crossref | Google Scholar |
Firth R, Kala J, Hudson D, Evans F (2023) Evaluation of ACCESS-S1 seasonal forecasts of growing season precipitation for Western Australia’s wheatbelt region. Journal of Southern Hemisphere Earth Systems Science 73(2), 131-147.
| Crossref | Google Scholar |
Ganter C (2011) Seasonal climate summary southern hemisphere (winter 2010): a fast developing La Niña. Australian Meteorological and Oceanographic Journal 61(2), 125-135.
| Google Scholar |
Gebrechorkos SH, Pan M, Beck HE, Sheffield J (2022) Performance of state‐of‐the‐art C3S European seasonal climate forecast models for mean and extreme precipitation over Africa. Water Resources Research 58(3), e2021WR031480.
| Crossref | Google Scholar |
Hendon HH, Thompson DWJ, Wheeler MC (2007) Australian rainfall and surface temperature variations associated with the Southern Hemisphere Annular Mode. Journal of Climate 20(11), 2452-2467.
| Crossref | Google Scholar |
Hersbach H, Bell B, Berrisford P, Hirahara S, Horányi A, Muñoz‐Sabater J, Nicolas J, Peubey C, Radu R, Schepers D, Simmons A, Soci C, Abdalla S, Abellan X, Balsamo G, Bechtold P, Biavati G, Bidlot J, Bonavita M, et al. (2020) The ERA5 global reanalysis. Quarterly Journal of the Royal Meteorological Society 146(730), 1999-2049.
| Crossref | Google Scholar |
Ho M, Kiem AS, Verdon-Kidd DC (2012) The Southern Annular Mode: a comparison of indices. Hydrology and Earth System Sciences 16(3), 967-982.
| Crossref | Google Scholar |
Hudson D, Alves O, Hendon HH, Lim E-P, Liu G, Luo J-J, MacLachlan C, Marshall AG, Shi L, Wang G (2017) ACCESS-S1: the new Bureau of Meteorology multi-week to seasonal prediction system. Journal of Southern Hemisphere Earth Systems Science 67(3), 132-159.
| Crossref | Google Scholar |
Hunke E, Lipscomb W (2008) The Los Alamos sea ice model documentation and software user’s manual, Version 4.0. (Los Alamos National Laboratory) Available at https://svn-ccsm-models.cgd.ucar.edu/cesm1/alphas/branches/cesm1_5_alpha04c_timers/components/cice/src/doc/cicedoc.pdf
Ibebuchi CC (2021) On the relationship between circulation patterns, the southern annular mode, and rainfall variability in Western Cape. Atmosphere 12(6), 753.
| Crossref | Google Scholar |
Li C, Hudson D, Zhou X, Zhu H, Wheeler MC, Young G, Marzin C, Roberts L (2023) Biases and teleconnections in the Met Office Global Coupled Model version 5.0 (GC5) – insights for seasonal prediction and Australia. Journal of Southern Hemisphere Earth Systems Science 73(3), 262-279.
| Crossref | Google Scholar |
Lim E-P, Hendon HH, Hudson D, Zhao M, Shi L, Alves O, Young G (2016) Evaluation of the ACCESS-S1 hindcasts for prediction of Victorian seasonal rainfall. October 2016. Bureau Research Report 019. (The Bureau of Meteorology: Melbourne, Vic., Australia) Available at http://www.bom.gov.au/research/publications/researchreports/BRR-019.pdf
Lim E-P, Hendon HH, Shi L, de Burgh-Day C, Hudson D, King A, Trewin B, Griffiths M, Marshall A (2021) Tropical forcing of Australian extreme low minimum temperatures in September 2019. Climate Dynamics 56(11–12), 3625-3641.
| Crossref | Google Scholar |
MacLachlan C, Arribas A, Peterson K, Maidens A, Fereday D, Scaife A, Gordon M, Vellinga M, Williams A, Comer R (2015) Global seasonal forecast system version 5 (GloSea5): a high‐resolution seasonal forecast system. Quarterly Journal of the Royal Meteorological Society 141(689), 1072-1084.
| Crossref | Google Scholar |
McKay RC, Boschat G, Rudeva I, Pepler A, Purich A, Dowdy A, Hope P, Gillett ZE, Rauniyar S (2023) Can southern Australian rainfall decline be explained? A review of possible drivers. WIREs Climate Change 14(2), e820.
| Crossref | Google Scholar |
Marshall AG, Hudson D, Wheeler MC, Hendon HH, Alves O (2012) Simulation and prediction of the Southern Annular Mode and its influence on Australian intra-seasonal climate in POAMA. Climate Dynamics 38(11–12), 2483-2502.
| Crossref | Google Scholar |
Megann A, Storkey D, Aksenov Y, Alderson S, Calvert D, Graham T, Hyder P, Siddorn J, Sinha B (2014) GO 5.0: the joint NERC–Met Office NEMO global ocean model for use in coupled and forced applications. Geotechnical Model Development 7(3), 1069-1092.
| Crossref | Google Scholar |
Meneghini B, Simmonds I, Smith IN (2007) Association between Australian rainfall and the Southern Annular Mode. International Journal of Climatology 27(1), 109-121.
| Crossref | Google Scholar |
Parton KA, Crean J, Hayman P (2019) The value of seasonal climate forecasts for Australian agriculture. Agricultural Systems 174, 1-10.
| Crossref | Google Scholar |
Philip P, Yu B (2021) Temporal variations in rainfall volume in the southwest of Western Australia. Journal of Applied Meteorology and Climatology 60(1), 23-32.
| Crossref | Google Scholar |
Pook MJ, Risbey JS, McIntosh PC (2012) The synoptic climatology of cool-season rainfall in the central wheatbelt of Western Australia. Monthly Weather Review 140(1), 28-43.
| Crossref | Google Scholar |
Roy R, Kundu S, Kumar R (2021) The impacts and evidence of Australian droughts on agricultural crops and drought related policy issues – a review. International Journal of Agricultural Technology 17, 1061-1076 Available at http://www.ijat-aatsea.com/pdf/v17_n3_2021_May/21_IJAT_17(3)_2021_Roy,%20R.%20N.pdf.
| Google Scholar |
Samuel JM, Verdon DC, Sivapalan M, Franks SW (2006) Influence of Indian Ocean sea surface temperature variability on southwest Western Australian winter rainfall. Water Resources Research 42(8), W08402.
| Crossref | Google Scholar |
Shao Y, Wang QJ, Schepen A, Ryu D (2022) Introducing long‐term trends into sub‐seasonal temperature forecasts through trend‐aware post‐processing. International Journal of Climatology 42(9), 4972-4988.
| Crossref | Google Scholar |
Smith I, McIntosh P, Ansell T, Reason C, McInnes K (2000) Southwest Western Australian winter rainfall and its association with Indian Ocean climate variability. International Journal of Climatology 20(15), 1913-1930.
| Crossref | Google Scholar |
Stephens D, Lyons T (1998) Rainfall–yield relationships across the Australian wheatbelt. Australian Journal of Agricultural Research 49(2), 211-224.
| Crossref | Google Scholar |
Stockdale TN (1997) Coupled ocean–atmosphere forecasts in the presence of climate drift. Monthly Weather Review 125(5), 809-818.
| Crossref | Google Scholar |
Thompson DW, Wallace JM (2000) Annular modes in the extratropical circulation. Part I: month-to-month variability. Journal of Climate 13(5), 1000-1016.
| Crossref | Google Scholar |
Ummenhofer CC, Sen Gupta A, Pook MJ, England MH (2008) Anomalous rainfall over southwest Western Australia forced by Indian Ocean sea surface temperatures. Journal of Climate 21(19), 5113-5134.
| Crossref | Google Scholar |
Walters D, Brooks M, Boutle I, Melvin T, Stratton R, Vosper S, Wells H, Williams K, Wood N, Allen T (2017) The Met Office unified model global atmosphere 6.0/6.1 and JULES global land 6.0/6.1 configurations. Geoscientific Model Development 10(4), 1487-1520.
| Crossref | Google Scholar |
Wedd R, Alves O, de Burgh-Day C, Down C, Griffiths M, Hendon HH, Hudson D, Li S, Lim E-P, Marshall AG, Shi L, Smith P, Smith G, Spillman CM, Wang G, Wheeler MC, Yan H, Yin Y, Young G, et al. (2022) ACCESS-S2: the upgraded Bureau of Meteorology multi-week to seasonal prediction system. Journal of Southern Hemisphere Earth Systems Science 72(3), 218-242.
| Crossref | Google Scholar |
Williams K, Harris C, Bodas-Salcedo A, Camp J, Comer R, Copsey D, Fereday D, Graham T, Hill R, Hinton T (2015) The met office global coupled model 2.0 (GC2) configuration. Geoscientific Model Development 8(5), 1509-1524.
| Crossref | Google Scholar |
Wright PB (1974) Seasonal rainfall in southwestern Australia and the general circulation. Monthly Weather Review 102(3), 219-232.
| Crossref | Google Scholar |
Zhang Z, Uotila P, Stössel A, Vihma T, Liu H, Zhong Y (2018) Seasonal southern hemisphere multi-variable reflection of the southern annular mode in atmosphere and ocean reanalyses. Climate Dynamics 50, 1451-1470.
| Crossref | Google Scholar |
Zhao M, Zhang H, Dharssi I (2017) Impact of land-surface initialization on ACCESS-S1 and comparison with POAMA. Vol. 12. (The Bureau of Meteorology) Available at http://www.bom.gov.au/research/publications/researchreports/BRR-023.pdf
Zhao P, Wang QJ, Wu W, Yang Q (2021) Which precipitation forecasts to use? Deterministic versus coarser‐resolution ensemble NWP models. Quarterly Journal of the Royal Meteorological Society 147(735), 900-913.
| Crossref | Google Scholar |