Observing and forecasting the retreat of northern Australia’s rainy season
Tim Cowan



A
B
C
Abstract
According to the Australian Bureau of Meteorology, the northern Australian wet season extends through to April, which also formally marks the end of Australia’s tropical cyclone season. Mid-autumn is when the tropical dry season transition period begins, when crop farmers prepare land for annual crops or pasture–fodder harvest, or when beef cattle producers make decisions regarding stock numbers and feed rationing. Potentially knowing if the last rains of the wet season will be later or earlier than normal would be valuable information for northern sectors such as agriculture, infrastructure and tourism. The Bureau of Meteorology provides seasonal forecasts of the Northern Rainfall Onset – the date when a location has accumulated 50 mm of rain from 1 September – yet there is currently no prediction of the rainy season retreat (the Northern Rainfall Retreat, NRR). In this study, we draw on three different NRR definitions and investigate how they vary with the El Niño–Southern Oscillation and the Madden–Julian Oscillation (MJO). In general, retreats occur ~1 week later than normal across the far northern tropics following La Niña events, but little change from normal occurs for El Niño. Although most retreats occur when the MJO is weak, if the MJO is active, retreats are mostly observed in phases 6 and 7, when convection is passing through the western Pacific. Utilising the Bureau of Meteorology’s sub-seasonal to seasonal forecast system, ACCESS-S2, we show that the model has some skill in forecasting the NRR across the far northern regions at a lead time of ~2.5 months, but poor skill in the subtropics and arid locations. Verification of the 2023 NRR forecasts, highlights the challenges of predicting the timing and magnitude of daily rainfall at such a long lead time.
Keywords: Australian monsoon, beef cattle, Bureau of Meteorology, monsoon retreat, El Niño–Southern Oscillation, Madden–Julian Oscillation, prediction, wet season.
1.Introduction
The wet season or rainy season across northern Australia (north of 20°S) is defined by the Bureau of Meteorology (hereafter, The Bureau) as the period extending from October to April. The wet season encompasses the summer monsoon period, which, as usually defined, typically develops during late December, when the 850-hPa winds near Darwin (130.8°E, 12.4°S) first shift from easterly to north-westerlies, sustained over at least a few days, often accompanied by widespread rainfall (Drosdowsky 1996; Lisonbee et al. 2019). The summer monsoon normally extends through to March; however, its duration, strength and intermittency can vary due to the state of climate and weather modes like the El Niño–Southern Oscillation (ENSO) (Smith et al. 2008; Lisonbee and Ribbe 2021) and the Madden–Julian Oscillation (MJO) (Evans et al. 2014; Berry and Reeder 2016). The Australian monsoon is also influenced by convectively coupled Kelvin and Equatorial Rossby waves (Wheeler and McBride 2005), which can act to modify the intensity and tracks of rain-bearing systems like tropical lows and cyclones (Bessafi and Wheeler 2006; Tsai et al. 2021).
In response to requests from the agricultural sector, The Bureau developed the Northern Rainfall Onset (NRO) forecast product, operationally released in 2015 (Drosdowsky and Wheeler 2014; Cowan et al. 2020). Different to the summer monsoon onset, which is usually defined by winds (e.g. Zhang 2010), the NRO is defined as the accumulation of 50 mm of rain from 1 September at a given location. It was intended as an estimate of the date of stimulation of new season pasture growth after the long dry season (Lo et al. 2007). In this way the NRO is analogous to a yearly-varying version of the Green Date (also called the Green Break of Season; Balston and English 2009), defined as the historical date when a location receives 50 mm in 3 days after 1 September in 70% of years.1 Here, 70% of years is preferred to the median because it gives a grazier greater certainty, as some regions experience high variability. The forecasts of the NRO, derived from ACCESS-S2 (Australian Community Climate and Earth-System Simulator – Seasonal, ver. 2; Wedd et al. 2022), commence each year c. late May, and are generated daily by The Bureau, but only released two times per month. The NRO forecasts are stopped at the end of August, and the NRO monitoring then commences. The predictability of the NRO appears to mostly come from the strong association between NRO variability and ENSO in the preceding austral winter, particularly over north-central and north-eastern Australia (Lo et al. 2007; Cowan et al. 2020).
A similar case can also be presented for a forecast product focusing on the end of the rainy season. For example, as the wet season progresses, beef cattle producers begin planning for the upcoming dry season, the peak period for mustering, restocking and selling livestock, and this planning can be affected by the timing of the last significant rains. Similarly, farmers who grow rain-fed pasture and fodder crops in northern Australia could take advantage of knowing the likelihood of late season rain events, which can affect crop ripening and harvesting (Mollah and Cook 1996). Transport and logistics can also be affected, as well as damage to infrastructure, if late season rain events are accompanied by extreme weather systems, e.g. Severe Tropical Cyclone Ilsa2 that led to major damage to Pardoo Roadhouse, in the Pilbara region of Western Australia (WA) in mid-April 2023.
Presently, The Bureau does not forecast an end to the northern rainy season, hereafter called the Northern Rainfall Retreat (NRR); however, there is broad interest from northern Australian beef producers for such a product, if it is as useful as the NRO forecast and is determined to be as skilful (M. McDonald, pers. comm.). Even without having a skilful forecast, by knowing the long-term historical NRO and NRR dates at a particular location, for example, a grazier would be able to determine the typical duration of their rainy season and then calculate their normal pasture–forage budget for the dry season. In this study, we explore the concept of a NRR for northern Australia, focusing on when it typically occurs, its association with climate drivers like ENSO and MJO, and finally, assess the prediction of the NRR in The Bureau’s seasonal prediction model, ACCESS-S2. Our overall objective is to investigate whether a NRR forecast product is viable and of utility for northern Australia agricultural communities. In Section 2, we discuss three different NRR definitions and show how each represents the last meaningful rainfall event of the northern wet season. In Section 3, we explore the observed NRR using more than 70 years of high-quality daily rainfall observations, and then assess the hindcast skill of ACCESS-S2 in predicting the NRR. A discussion of the advantages and limitations of a possible NRR forecast product is presented in Section 4.
2.Data and methods
2.1. Observations and hindcasts
Daily gridded rainfall data are from The Bureau’s Australian Gridded Climate Data (AGCD) (ver. 1; Bureau of Meteorology 2019), provided on a 0.05° (5-km) grid. The rainfall data cover 1 January 1950 through to 31 December 2019, providing an adequate sample size to compare wet season retreats for different ENSO phases. By the late 1950s, there was an increase in the number of operational rain gauges (Jaffrés et al. 2018), although gaps remained in Arnhem Land and the Gulf Country. The spatial analysis is restricted to regions north of 30°S; however, as shown later, regions that are climatologically dry or do not have a well-defined annual cycle of rainfall can be difficult to interpret and often do not feature a retreat date (or an onset date; Smith et al. 2008). A data quality mask is applied to regions, predominantly in central WA, that have a poor daily rainfall coverage (Griffiths et al. 2023).
Hindcast and forecast data are utilised from ACCESS-S2, a model based on the UK Met Office’s GloSea5-GC2 seasonal prediction system (Wedd et al. 2022). The horizontal resolution of the atmospheric component of ACCESS-S2 is 60 km in the midlatitudes and across Australia. ACCESS-S2 also uses its own data assimilation to produce its own initial conditions instead of relying on the Met Office assimilation scheme, and has dynamic soil moisture initial conditions as opposed to climatology. Here, the 5 km calibrated hindcasts from ACCESS-S2 are used, which removes the model biases, e.g. using a quantile–quantile matching approach (Griffiths et al. 2023). This is important because ACCESS-S2 tends to have a wet climatological bias over northern Australia tropical regions in the late summer and early autumn seasons (Li Shi, pers. comm.). The hindcast period is 1981–2018, and for seasonal-scale predictions, there are three 279-day model ensemble members that have been generated on the 1st of every month and on each of the previous 8 days. This produces a 27-member time-lagged ensemble (3 members × 9 start days) with which to evaluate ACCESS-S2’s skill in predicting the NRR over the hindcast period. For the last year of the hindcast record, 2018, we only use eight start dates as there is no 1 January 2019 simulation.
2.2. NRR definitions
Based on a review of recent literature, three different ways to define the NRR are selected:
Maximum in the cumulative daily rainfall anomaly, with the anomaly relative to the multi-year annual mean (Uehling and Misra 2020; Uehling et al. 2021); hereafter termed the cumulative anomaly definition.
Backwards accumulation of daily rainfall until 50 mm is reached from the end of April, analogous to the NRO but in reverse (Lo et al. 2008); hereafter termed the backward accumulation definition.
The last 3-day burst of rainfall, based on exceeding a pre-determined rain total (Cowan et al. 2022c); hereafter termed the burst definition.
The above definitions require a fixed ‘cut-off’ date for the wet season, to prevent isolated rain events from being inadvertently considered in the post-wet season. In this study, the selected date is 30 April, in line with The Bureau’s formal definition of the end of northern Australia’s wet season and tropical cyclone season. Later in the study, we test how shifting the cut-off date to 31 May affects the NRR date. We also begin the analysis of each NRR period from 1 January. Below, the three different definitions for determining the NRR date are described in more detail, with reference to Fig. 1.
The three different methods for defining the northern rainfall retreat date, based on daily rainfall (blue bars) for Darwin during January–April 1981. The three methods are (1) maximum in the cumulative daily rainfall anomaly before 30 April (black curve and dot), (2) backwards accumulation of daily rainfall from 30 April until 50 mm is reached (red curve and dot) and (3) last ‘25 mm in 3-day’ burst before 30 April (green thick lines and dot). The left y-axis is for methods (2) and (3), and the right y-axis is for method (1). Rainfall is rounded to the nearest 0.2 mm.
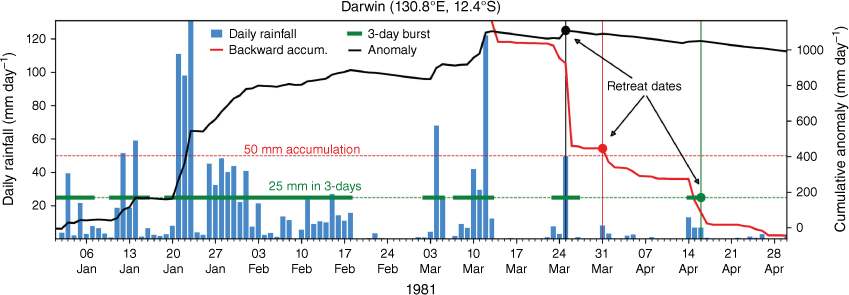
The cumulative anomaly definition stems from research into the duration of the northern Australian rainy season by Uehling et al. (2021) and Uehling and Misra (2020). This definition has been used to diagnose the onset and retreat of the Indian summer monsoon (Noska and Misra 2016) and South American rainy season (Liebmann et al. 2007). To determine the NRR using this definition, the cumulative daily anomaly of rainfall for each day i of each year k for each grid point is calculated as follows:
where the annual mean climatology of rainfall over N days and K years is as follows:
where r(n,k) is the daily rainfall over the multi-year period.
Hence, the cumulative anomaly definition describes the removal of a multi-year daily mean amount from each day’s rainfall total and then this anomaly is accumulated from a given start date (i.e. 1 January). To illustrate this, we use daily rainfall from Darwin in 1981 from 1 January to 30 April (blue bars, Fig. 1). As the wet season progresses in this year, the cumulative daily anomaly increases following each rainfall event and slowly declines during break (in rain) periods due to the negative daily anomalies (e.g. 19 February to 3 March; see black ‘anomaly’ curve in Fig. 1). The point at which reaches its maximum before 30 April is designated as the NRR (black dot, Fig. 1). In the Darwin 1981 example, the NRR occurs on 25 March. Uehling and Misra (2020) begin the summation of day n from 1 September, as they also focus on the onset; however, as our interest is solely on the retreat, we begin our anomaly summation from 1 January. If there is no discernible wet season in any given year and peak is reached at day 1, then this location is represented by a special value. This is more likely to happen in climatologically dry regions (e.g. parts of WA) or regions with highly variable rainfall, e.g. southern Queensland (Qld). This is also why the definition is better suited to the far northern regions of Australia (region highlighted in Fig. 2a) that experience monsoon rainfall, as suggested in Uehling and Misra (2020).
Median retreat day over 1950–2019 using the three definitions. Shown are (a) the peak daily cumulative anomaly, (b) the backward accumulation of daily rainfall from 30 April and (c) the last day of the last burst event (25 mm in 3 days) before 30 April. The black contour indicates 1 March (day 60). Retreat dates for nine locations are shown across northern Australia (based on a non-leap year). Red, grey and blue labels indicate the retreat definition that produces the earliest, middle and latest median retreats respectively. Any year for which the retreat day is undetermined is referred to as a non-retreat year. Regions with sparse rainfall coverage or with 30% non-retreat years across the 70-year period are masked out in grey. These mainly represent climatologically dry regions. The area encompassed by the blue dashed line represents the Australia’s monsoonal north region.
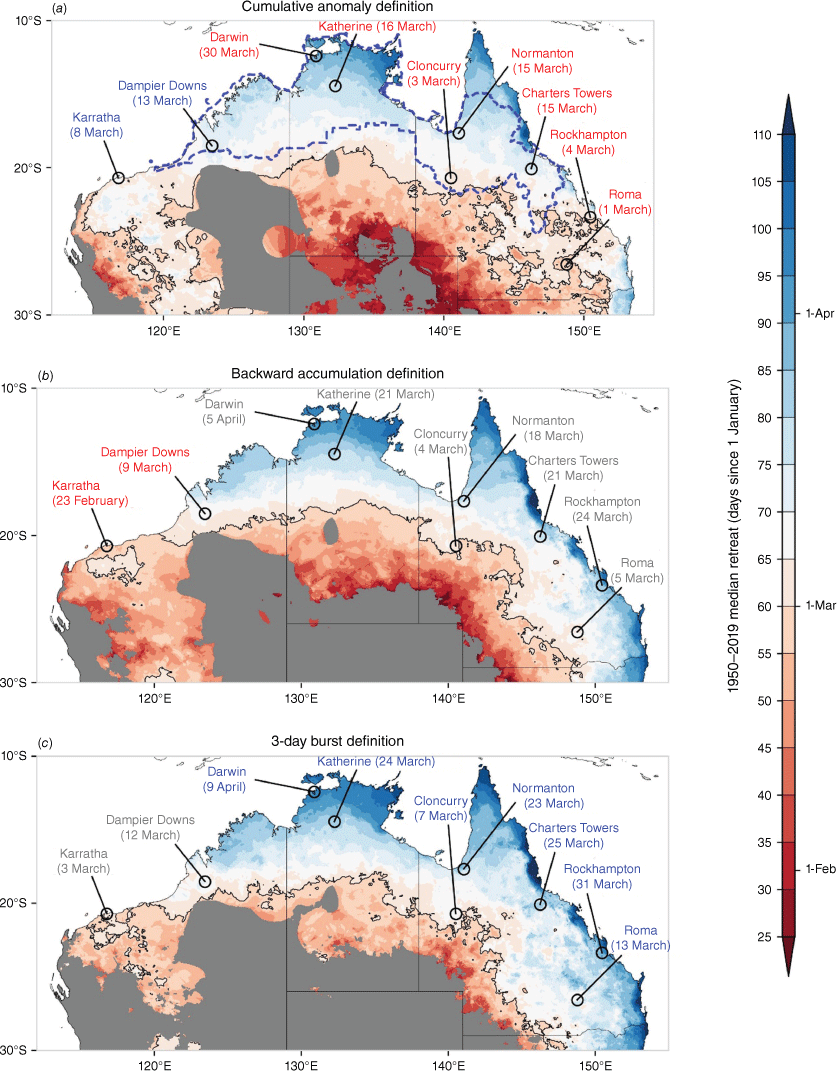
The backward (in time) accumulation definition derives from a technical report that investigated ways to improve The Bureau’s prediction of the onset and duration of wet season rainfall (Lo et al. 2008). The definition describes the accumulation of daily rainfall backward in time from 30 April until 50 mm (total) is reached. As such, it is the reverse of the NRO, and can be thought of as the start date of a period through to the end of April where a location will not exceed a total of 50 mm. In Fig. 1, this definition is shown as the red curve, displaying two small rain events during 14–16 April (26.6 mm in total) and 31 March–1 April (11.4 mm in total). The late March rain event leads to 50 mm being reached and, as such, 31 March 1981 is the backward accumulation NRR date (red dot, Fig. 1). If 50 mm of rainfall is not reached by 1 January at a given location, this is also represented by a special value. As noted in Lo et al. (2008), this occurs more frequently in climatologically dry regions meaning this definition, like definition #1, is more suited to the northern tropics.
The 3-day rainfall burst definition arose from a research study and report that accompanied The Bureau’s release of a 3-day rainfall forecast product in 2022 (Cowan et al. 2022b, 2022c). The method does not rely on accumulating rainfall (i.e. prior information is ignored), but instead focuses on the last burst of rainfall over a 3-day period before 30 April. The threshold amount for the 3-day burst is set to 25 mm, which, for the example for Darwin in 1981, occurs during 14–16 April (green line, Fig. 1). The NRR is set to the last day in the sequence of 3 days that registers at least 0.2 mm of rainfall. As such, rain should always occur on day 1 of the 3-day period; however, if days 2 or 3 do not exceed 0.2 mm day–1 and day 1 reaches 25 mm, then day 1 will be the NRR date. If either day 2 or 3 exceed 0.2 mm day–1 and the accumulation of day 1 with day 2 or day 3 reach 25 mm, then days 2 or 3 will be the NRR date. In the Fig. 1 example, this occurs on 16 April 1981 (green dot). Like the other two methods, if there is no 3-day burst of rainfall that exceeds 25 mm (from the beginning of January), then this location is given a special value for that year. This favours wet regions and disadvantages climatological dry regions.
The Fig. 1 example produces three different NRR dates and was selected for ease of graphical representation of the three definitions, but it is more common for dates to align. We return to the issue of misaligning NRR dates in Section 4. It is also more likely that the cumulative anomaly definition will be biased towards larger rainfall events, whereas the backward accumulation and burst definitions are more likely to capture isolated systems that may not be associated with intra-seasonal climate drivers or convectively coupled waves. Additionally, the backward accumulation and burst definitions are not objective, and rely on pre-determined fixed rainfall amounts, like the NRO and the 3-day rainfall forecast product (Cowan et al. 2022b). For each year of 1950–2019, we determine the NRR dates across Australian latitudes, north of 30°S. In any given year, if a grid-point does not observe a NRR, we set that value to zero. If a NRR is missing in 30% of all years over the record, that location is masked. As such, many arid locations in central Australia are masked out.
2.3. Defining ENSO and the MJO
To separate the NRR dates based on El Niño, La Niña and neutral years, we use the Oceanic NINO index (ONI) for 1950–2019. The ONI is a 3-month running mean of sea surface temperature (SST) anomalies in the NINO3.4 region (5°S–5°N, 170–120°W), calculated from the Extended Reconstructed Sea Surface Temperature dataset (ver. 5, ERSST.v5; Huang et al. 2017). Here El Niño and La Niña events are defined as when the ONI threshold is at least ±0.5°C for four consecutive 3-month seasons from September–November (year (−1)) through to December–February (year (0)). This allows us to associate the NRR dates with ENSO events that peak in austral summer, not in the development stage. This results in 25 El Niño events, of which 21 events also exceed +0.5°C in the January–March season (year (0)). The number of La Niña events selected is 22, of which 2 years (2005–06, 2008–09) do not meet the −0.5°C threshold in September–November, but well exceed the −0.5°C threshold in the January–March season and are included because their timing aligns with the timing of the NRR. As the ±0.5°C ENSO threshold is weaker than The Bureau’s operational threshold of ±0.8°C in the NINO3.4 region, our sample groups of El Niño and La Niña events contain both strong and weaker events, as well as central and eastern Pacific flavours.
The MJO is measured by the Wheeler and Hendon (2004) Real-time Multivariate MJO (RMM) index; an index calculated using daily equatorially averaged (15°S–15°N) Outgoing Longwave Radiation (OLR) (Liebmann and Smith 1996) and NCEP–NCAR zonal winds at 850 and 200 hPa (Kalnay et al. 1996). We use the RMM index from January 1975 through to April 2019 (inclusive), noting that there are missing dates from 17 March to 31 December 1978. The objective of this part of the study is to investigate if NRR dates tend to cluster within the same MJO phase (e.g. when the MJO moves into the western Pacific causing suppressed convection over northern Australia; see Evans et al. 2014). This follows the composite approach of Uehling et al. (2021), who isolated the intraseasonal oscillation using empirical orthogonal functions on the 30–60-day bandwidth and looked at both the onset and retreat of the northern rainy season in each MJO phase. We extend the composite approach for all three NRR definitions.
3.Results
3.1. Median retreat date
We first compare the 1950–2019 median NRR dates for the three definitions (Fig. 2). Additionally, the dates from nine locations across northern Australia are labelled in each map. As expected, the more northward tropical locations and those dotted along the east coast (i.e. east of the Great Dividing Range) experience a later retreat well into April, whereas inland arid regions have retreats in late January. The masked (grey) areas of northern South Australia, central WA and south-west Qld are regions that do not receive substantial wet season rainfall, with the retreat unable to be determined in at least 30% of years. In general, the cumulative anomaly definition produces earlier retreat dates for the Top End and much of Qld, and the burst definition displays later retreats. For example, Darwin shows a range of 10 days in median retreat dates, with the cumulative anomaly, backward accumulation and burst definitions showing 30 March, 5 April and 9 April respectively (Fig. 2). Further south, in the town of Katherine, the retreat range is 8 days, with the latest date of 24 March (burst definition). Across south-east Qld (e.g. Rockhampton and Roma in Fig. 2), the three retreat definitions diverge – this suggests that, like the NRO, defining a retreat date in coastal subtropical latitudes is difficult as rainfall is not overly seasonal (Drosdowsky and Wheeler 2014).
Interestingly, the pattern of the median NRR dates is not the direct opposite of the median NRO (Cowan et al. 2020) or onset based on the Uehling et al. (2021) definition. Although Cape York and far eastern Arnhem Land have quite late retreats (aside from eastern coastal regions that commonly experience dry season rain), the earliest onsets occur over Darwin and the western Top End (Drosdowsky and Wheeler 2014; Cowan et al. 2020; Uehling et al. 2021). The contrasting spatial retreat pattern could be indicative of the active phase of the MJO passing into the western Pacific – this is explored later in the study. The far west of WA (e.g. Pilbara) have the latest onsets and earliest retreats, as found using other monsoon definitions (Zhang 2010). Further north-east into the Kimberley region, late onsets c. late December–early January are typically followed by late retreats in mid-March, likely a result of a mid-autumn tropical cyclone or depression (e.g. TC Fay in March 2004).3
The strongest agreement between the three definitions occurs north of 20°S; these regions, including the Kimberley, northern NT and northern Qld, all exhibit a strong seasonality in their rainfall and reliable wet seasons (Sharmila and Hendon 2020). Regions south of 20°S either exhibit a higher coefficient of variation (west and central Australia) or do not have a well-defined wet season (e.g. south-east Qld).
For the northern red meat producers, the Green Date marks the date by which a producer would normally have received 50 mm over a 3-day period for their location in 70% of all years. Taking this approach to the NRR, we calculate the long-term 70th and 30th percentiles (for 1950–2019) for each definition, which characterises the latest (70th percentile) and earliest (30th percentile) NRR dates (Fig. 3).
Observed (left) 70th and (right) 30th percentile retreat day over 1950–2019 using three definitions. Shown are (a, b) the peak daily cumulative rainfall anomaly, (c, d) the backward daily rainfall accumulation from 30 April and (e, f) the last day of the last burst event (25 mm in 3 days) before 30 April. The black contour in (a, c, e) represents 1 April (day 91), and in (b, d, f) 1 March (day 60). Regions with sparse rainfall coverage or with 30% non-retreat years across the 70-year period are masked out in grey. An expansive list of 70th percentile values at various red meat and sugar locations across northern WA, NT and Qld are shown in Supplementary Tables S1–S3.
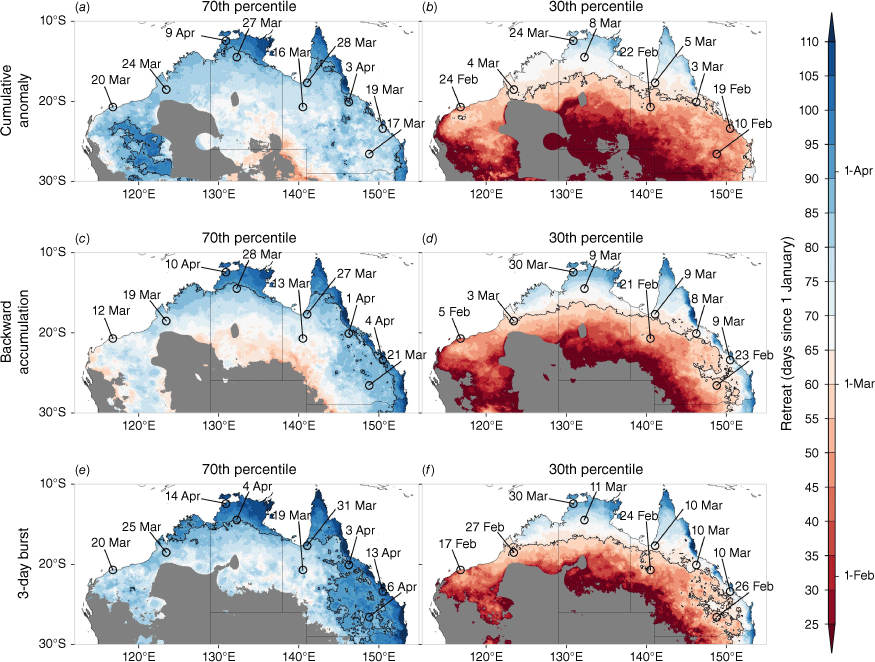
An expansive list of individual 70th percentile dates, based on the three definitions for locations across northern Australia that produce red meat or sugar, are shown in Supplementary Tables S1–S3 (for NT, WA and Qld respectively). These locations are taken from over 550 test locations introduced from the Forewarned is Forearmed project (Hudson et al. 2022). The purpose of these dates is to provide a producer with an approximate ‘decision date,’ similar to the Green Date, deriving the timing of the last meaningful rain event in the majority of years, from which dry season planning can begin (i.e. forage budgeting for livestock producers). For a location like Darwin Airport, considering the range of possible retreat dates based on the three NRR definitions, the retreat typically occurs by 9–14 April (70th percentile; Fig. 3a, c, e) but sometimes can occur as early as 24–30 March (30th percentile, Fig. 3b, d, f). These Darwin dates are much later than average estimates of early-mid March, derived from wind (Drosdowsky 1996) and seasonal rainfall total (Smith et al. 2008), but closer to other objective retreat definitions that give early April retreats (Janowiak and Xie 2003; Zeng and Lu 2004). At more southern latitudes in Qld’s Outback town of Cloncurry, the typical retreat happens by 13–19 March, but in 30% of years, can occur in late February. As with the medians, the cumulative anomaly definition generally captures the earliest retreats in the NT and Qld, irrespective of the percentile. Over much of inland eastern Qld, the burst definition gives April retreats in the 70th percentile, in contrast to the early-mid March retreats given by the other two definitions. This suggests that April rainfall events are not of sufficient magnitude to exceed the 50-mm accumulation or overcome the steady decline in the cumulative anomaly, dictated by dry spells between wet events (Fig. 1).
3.2. ENSO and retreat dates
It is well known that ENSO has a strong control on the onset of the wet season rainfall (Smith et al. 2008; Kajikawa et al. 2010; Evans et al. 2014) and the NRO (Lo et al. 2007; Drosdowsky and Wheeler 2014), and this feeds into a stronger or weaker magnitude start to the wet season (Cowan et al. 2020). These studies all utilised the Southern Oscillation Index (SOI) to describe ENSO. Using the NINO3.4 index to describe ENSO, Lisonbee and Ribbe (2021) showed that, in fact, strong La Niña events (NINO3.4 SST anomalies <−0.99°C) are associated with earlier onsets; however, little separated average onsets from weak La Niña events, neutral or El Niño events. For retreat dates based on the cumulative anomaly definition, Uehling et al. (2021) concluded that there was a patchy negative relationship to ENSO (defined as Sep–Nov NINO3.4), with peaks over the Cape York and southern Top End.
Fig. 4 displays the difference between the 1950 and 2019 median NRR and the median NRR in the El Niño and La Niña subsets. Brown shading indicates where the NRR median in ENSO years is earlier than the all-year median. For the three NRR definitions, it appears that there is very little deviation in retreats from the long-term median (±5 days) for El Niño years (Fig. 4a, c, e). Using Darwin as an example, the retreat occurrences for El Niño range between 2 days earlier to 1 day later. Further south in Katherine (south-east of Darwin), the retreat shift is 0–2 days earlier. The largest influence of El Niño is observed across southern NT and southern Qld, with the retreat dates shifting 5–10 days earlier. The El Niño-induced earlier shifts in the retreat are most striking in the cumulative anomaly definition, with the northern Qld regional centre of Charters Towers (Fig. 2) showing a retreat shift to 8 days earlier (Fig. 4a). These shifts represent ~25% of the interannual variability, as defined by the interquartile range. The typical retreat variability is ~20–25 days over northern Australia (Supplementary Fig. S1b, d, f), so the shifts due to El Niño are not overly remarkable. The earlier shifts in the southern regions in the cumulative anomaly definition stem from the fact that this definition is capturing earlier NRR dates, during which ENSO is likely to be stronger (i.e. closer to its peak).
Observed difference between the 1950 and 2019 median retreat day and the median for (a, c, e) El Niño years and (b, d, f) La Niña years for each northern rainfall retreat definition. Shown are (a, b) the peak daily cumulative rainfall anomaly, (c, d) the backward daily rainfall accumulation from 30 April and (e, f) the last day of the last burst event (25 mm in 3 days) before 30 April. El Niño and La Niña events cover the January–March period (i.e. demise of event), with events from https://origin.cpc.ncep.noaa.gov/products/analysis_monitoring/ensostuff/ONI_v5.php. The El Niño years include 1952, 1954, 1958–1959, 1964, 1966, 1969–1970, 1973, 1977–1978, 1980, 1983, 1987–1988, 1992, 1995, 1998, 2003, 2005, 2007, 2010, 2015–2016 and 2019. The La Niña years include 1950, 1955–1956, 1965, 1971–1972, 1974–1976, 1984–1985, 1989, 1996, 1999, 2000–2001, 2006, 2008–2009, 2011–2012 and 2018. Regions with sparse rainfall coverage or with 30% non-retreat years across the 70-year period are masked out in grey.
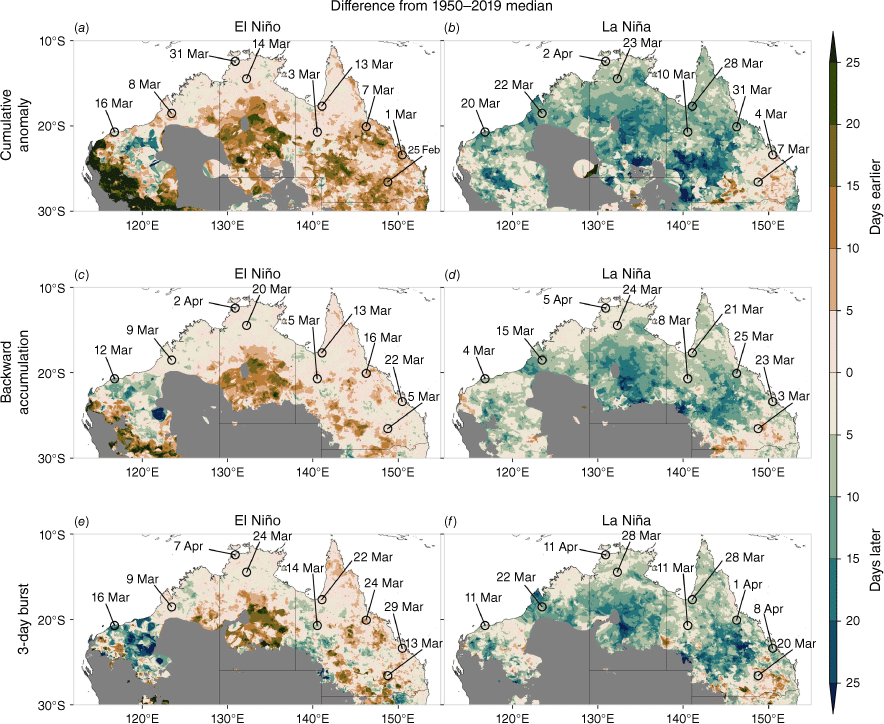
Unsurprisingly, for the La Niña subset, we see later retreats across much of northern Australia (Fig. 4b, d, e). The magnitude of the later retreats is far stronger than the El Niño-related earlier retreats across the northern tropics, suggesting an asymmetric relationship with ENSO (e.g. Chung and Power 2017; Heidemann et al. 2023). Although Uehling et al. (2021) showed that retreat dates (using the cumulative anomaly definition) across northern Australia are negatively correlated with ENSO, we suspect that this relationship is dominated by the strong association with La Niña, like the wet season onset (Lisonbee and Ribbe 2021). Of the three NRR definitions, the backward accumulation definition shows the weakest shifts over northern Australia (north of 20°S) with respect to La Niña, with an average of 7 days later, compared to 8 and 10 days for the burst and cumulative anomaly definitions respectively.
For south-east Qld, La Niña has a weaker control on the retreat dates, whereas in the north-west (e.g. Dampier Downs in the Kimberley region, and Karratha in the Pilbara) retreat dates are pushed back by 8–12 days (~30–50% of the interquartile range). For many regions, the El Niño influence (earlier retreats) is much weaker than that for La Niña (later retreats), meaning there must be a compensation during the neutral ENSO years. Indeed, we see over large swaths of northern Australia, particularly southern Top End and Cape York, that neutral years push the retreat towards earlier dates (Supplementary Fig. S2). A good example of this is in Katherine, which typically experiences a retreat date up to 12 days earlier (burst definition) in neutral ENSO years, compared to 2 days earlier for El Niño and 3–7 days later for La Niña. It is possible that the earlier retreats co-occurring with neutral ENSO conditions are related to intra-seasonal climate variations in the western Pacific in the lead-up to a developing ENSO event. Of the 23 neutral years, nine developed into El Niño events by the austral winter and five events had already surpassed the +0.5°C ONI threshold by the April–June season. Only one neutral ENSO year developed into a La Niña (2017 into 2017–18); however, this event did not surpass the −0.5°C ONI threshold until the austral spring. A lag correlation analysis between the seasonally averaged and linearly detrended ONI and NRR dates, averaged over northern Australia (north of 20°S for all Australian longitudes) suggests that there is a significant negative relationship between the NRR date and the following ONI from austral winter following the retreat (JJA+1) through to the next autumn (MAM+1) (Supplementary Table S4). A developing ENSO event (from JJA0) is also correlated with NRR, although the strength of this relationship is weaker in the burst and backward accumulation definitions. Although exploration of this relationship is beyond the scope of this paper, Evans et al. (2014) suggest that the mechanism is related to deep convection over the Maritime Continent and variations in the Walker Circulation. In summary, following the peak of La Niña events, NRR tends to occur later, whereas for El Niño events, NRR typically does not change much from normal in the far north, but occurs earlier in the subtropical east. Neutral ENSO years are associated with earlier NRR dates over the far northern tropics, although the mechanism for this is unclear and requires further exploration.
3.3. MJO and retreat dates
As shown by Uehling et al. (2021), the relationship between retreat date and the MJO phase is not homogeneous across tropical northern Australia, with phases 7 and 8 more frequently associated with retreats for the Gulf region, whereas phase 6 is linked to retreats across the Top End and along Qld’s north-east coast. In general, rainfall bursts across northern Australia that feature a non-southerly vorticity flux prior to the rain event (i.e. tropical origins) are more related to the MJO when in its active phases of 3–6 (moving from the Maritime Continent to northern Australia) (Narsey et al. 2017). To assess the state of the MJO during NRR dates, we first focus on retreats for Darwin, plotting the amplitude and phase of the MJO on the day of the NRR, for all years of 1975–2019 (Fig. 5). For the three NRR definitions when the MJO is strong (amplitude > 1), the MJO is more likely to be in phase 6 when the retreat date occurs. For the cumulative anomaly definition, the retreat dates fall in MJO phase 6 in 20% of years, whereas for the burst definition, it occurs in phase 6 in 24% of years. The cumulative anomaly and burst defined retreats occur in 20 and 26% of years respectively, when combining MJO phases 2 and 3. These phases are when convection is strong in the central Indian Ocean and suppressed conditions exist over the north-north-east of Australia in austral autumn (Cowan et al. 2023). This drops to 13% for the backward accumulation definition. One reason for this disparity might be because the backward accumulation definition does not necessarily require discrete rainfall events to surpass the 50-mm threshold, as opposed to the cumulative anomaly and burst definitions. Any rain event leading to the 50-mm accumulation could be unrelated to the MJO. Interestingly, the retreat dates based on the cumulative anomaly and burst definitions almost never or very rarely fall in MJO phases 8, 1 and 4. During autumn, phases 8 and 1 are associated with suppressed convection (i.e. positive OLR anomalies) over northern Australia (Cowan et al. 2023), so perhaps this is unsurprising. Phase 4, however, is related to strong convection stretching from the Sumatra–Java region, east across the Maritime Continent and over the northern reaches of Australia’s Top End and Cape York. Given the MJO’s strong influence on rainfall over northern Australia in phases 5–7, this infers that the last meaningful rain events of the wet season are likely to occur after phase 4. The fact that most retreats (33–40%) occur when the MJO is weak (amplitude < 1) means that the MJO may not be the dominant forcing, at least in Darwin.
Phase of the MJO on the date of the NRR for each year of 1975–2019 for (a) the peak daily cumulative anomaly definition, (b) the backwards accumulation of daily rainfall from 30 April and (c) the last day of the last burst event (25 mm in 3 days) before 30 April. Blue and red coloured dots represent March and April dates respectively.
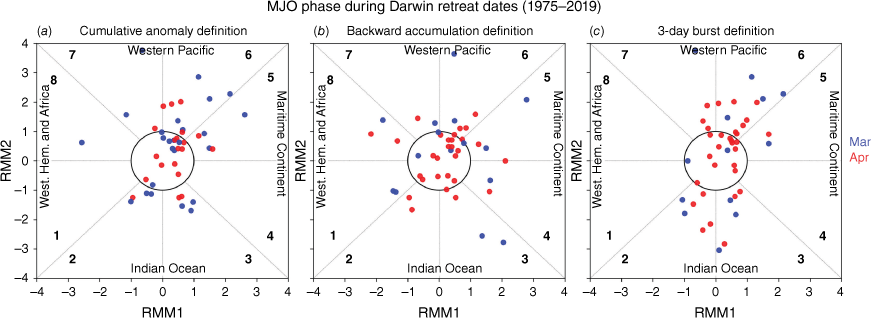
The same analysis is applied to each of the nine northern Australian locations that feature in Fig. 2, with the percentage of retreat dates (for each NRR definition) that fall into each MJO phase shown in Fig. 6. Like Darwin, the locations of Katherine, Normanton, Cloncurry and Charters Towers all show MJO phase 6 as the dominant phase, although the percentages vary between the definition dates. The percentages associated with the dominant phase are also generally weaker at those locations compared to Darwin, and there is a high degree of spread across all phases for Cloncurry and Charters Towers. In the Gulf town of Normanton, MJO phase 8 is associated with a retreat in 10% of years, approximately half of what Uehling et al. (2021) found. For Dampier Downs in WA’s Kimberley region, as well as Roma in Qld’s south-east, the retreat date is more frequently linked with MJO phase 7, occurring 15–20% of the time, although phases 6 and 7 feature in 13% of years for Dampier Downs. Further south-west, at Karratha, it is clear that MJO phase 6 is when the retreats are more likely; however, the backward accumulation definition displays more frequent dates in phase 5. This is likely because this definition captures more retreats in February (for Karratha) than for the other two definitions. When in MJO phase 5, during January–March, convection is the strongest over Karratha,4 so it is likely the backward accumulation definition is capturing the last substantial rainfall event of the wet season. By contrast, the cumulative anomaly and burst definitions show retreats in March and April for Karratha. Some locations, like Rockhampton, on Qld’s far east coast, do not show any strong association with the MJO. Indeed, what is not shown in Fig. 6 is that the majority of retreats are most associated with weak MJO activity (amplitude < 1), at close to 40% for Darwin, Charters Towers and Rockhampton. Most other locations show retreats occurring ~30% of the time when the MJO is weak, similar to other studies (Evans et al. 2014). From these results, we conclude that MJO phases 6 and 7 are associated with retreats during February–April; however, retreats can happen in nearly all phases, and the fact that these occur most often when the MJO is weak infers that other stochastic processes are more often responsible for the retreat of the rainy season.
Frequency of northern rainfall retreat that falls into each phase of the MJO, excluding the weak phase (when MJO < 1) for the nine northern Australian locations for 1975–2019. The percentages are calculated by the number of years the retreat occurs in a particular phase, divided by the total number of years.
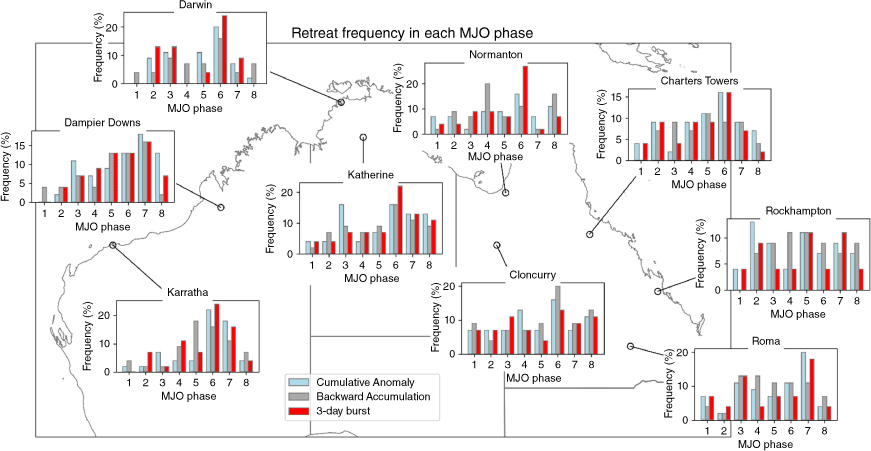
3.4. Northern rainfall retreat in ACCESS-S2
To answer the question of whether it is possible to forecast the NRR, we first need to evaluate the model biases and skill in the ACCESS-S2 hindcasts. In general, there have not been any great improvements in forecasting late summer to early autumn rainfall for northern Australia in ACCESS-S2 over its predecessor ACCESS-S1 (Wedd et al. 2022). In terms of short multi-day rainfall bursts, ACCESS-S2 shows a degeneration in prediction skill over most of the tropical north beyond a lead time of 3 weeks (Cowan et al. 2022a, 2022b). Despite this, we utilise 27 hindcasts from ACCESS-S2 for each year from 1981–82 to 2018–19 (noting that an ensemble of only 24 hindcasts is used for 2018, as explained in Section 2). The hindcast start dates cover each day from 24 December to 1 January (year + 1) for three different ensemble members. For each ensemble member and start date, we determine the NRR using each definition and then calculate the long-term model median, which we compare to the observed NRR median for 1982–2019. Fig. 7 displays the biases (i.e. difference between the model and observations) and Brier skill scores in ACCESS-S2, the latter smoothed 10× with a Gaussian (moving average low-pass) filter to remove noise. Starting with the biases, we see that ACCESS-S2 performs well at capturing the retreat dates over the far northern reaches, within ±6 days. The biases are greatest for the cumulative anomaly definition and of higher magnitude in southern Qld and the NT.
ACCESS-S2 biases and skill in median retreat date over 1982–2019 using the three northern rainfall retreat definitions. Shown are (left) the biases and (right) Brier skill scores across 38 years and 27 ensemble members for (a, b) the cumulative anomaly definition, (c, d) backward cumulative definition and (e, f) burst definition. The ACCESS-S2 biases are calculated as the difference between ACCESS-S2 and AGCD. The 27 members consist of three ensemble members that include 1 January (except 2019) and the eight prior days of the previous month to 24 December. The Brier skill score shows the percentage improvement over the climatological forecast, using the cross-validation method with the patterns smoothed 10× using a Gaussian smoothing function. Stippling indicates where the Brier skill score is above +10%, usually considered significant. Regions with sparse rainfall coverage or with 30% non-retreat years are masked out in grey.
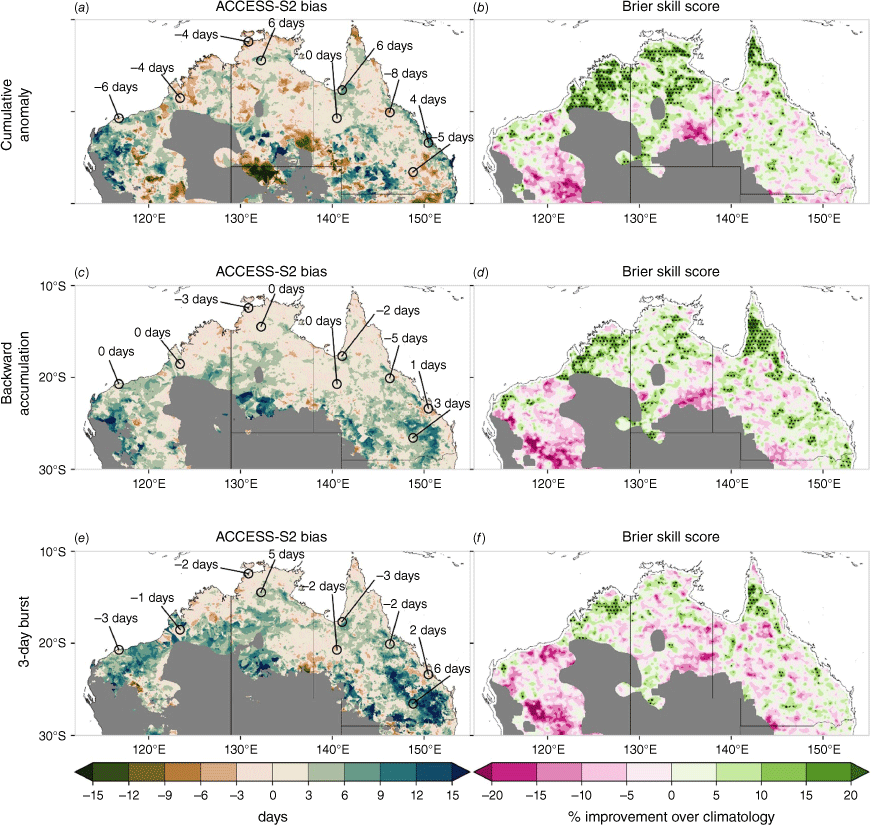
Taking a broad view, ACCESS-S2 simulates positive (i.e. later retreat) biases in both the backward accumulation and burst definitions, and given the similarities in their bias patterns, this suggests that the two definitions capture the same retreat date in both the hindcasts and observations. As the model medians are based on almost 1024 simulations the biases are small in comparison to the observed deviations seen during ENSO events. The skill of ACCESS-S2 to predict the retreat dates is good across the Kimberley, Cape York and patches of the Top End for the cumulative anomaly and backwards accumulation definitions (Fig. 7b, d), with all these regions showing a large areal extent above the 10% improvement over a climatological forecast (i.e. 50% chance of either a later or earlier retreat). Given the retreats for these regions occur between early and late March, this infers that ACCESS-S2 shows reasonable skill out to ~2.5 months from a late December forecast. The best skill is seen for the cumulative anomaly definition, whereas the burst definition has the poorest skill. This possibly reflects the fact the cumulative anomaly retreats are earlier than the burst retreats and are less susceptible to timing and magnitude errors, whereas the burst definition needs to reach at least 25 mm and it must be over consecutive days, making it more difficult to forecast. These results suggest that there could be some utility in a forecast of the NRR across the far northern tropics; however, further south, given ACCESS-S2 skill is poor (or patchy at best) and biases are relatively large, forecasting the retreat is more difficult.
4.Discussion and conclusions
This study investigated the concept of a NRR – the last meaningful rainfall event for northern Australia locations prior to the end of April – for the purpose of developing a prototype forecast product that could be utilised by graziers, crop farmers and businesses with infrastructure and tourism interests (e.g. road, building maintenance and nature parks). Any such forecast product, such as one that shows the probabilistic chance of an early or late NRR, could help in proactive decision-making in the lead-up to the northern dry season, when mustering, selling and restocking of livestock begins, and infrastructure builds re-commence and areas within National Parks reopen after the wet season.
This work identified both objective (cumulative anomaly) and subjective (back accumulation and bursts) ways of defining the NRR. Our results have shown that the cumulative anomaly definition (Uehling et al. 2021) generally leads to the earliest retreats across northern Australia, whereas the burst definition tends to produce later retreats. This may relate to the fact that the cumulative anomaly peaks after relatively high magnitude rainfall events that are directly associated with the large-scale monsoon activity. The backward accumulation and burst definitions, however, are more likely to induce a retreat through isolated synoptic activity, as shown for Darwin in 1981 (Fig. 1). Using the three NRR definitions, we have shown when locations should expect to reach their retreat based on the 70th percentile of all historical retreats, and when they could expect their earliest retreat (e.g. 30th percentile). Knowing this type of information at the location scale, as presented in Supplementary Tables S1–S3 for the 70th percentile, provides a user with a historical date with which decisions can be implemented, if, for example, a region passes its historical NRR date and there is no rain forecast in the weekly or fortnightly outlook.
We next explored the relationship between NRR and climate drivers such as ENSO and the MJO and found that La Niña pushes the retreat date back by more than 1 week in many locations. As one might expect, El Niño does lead to slightly earlier retreats over central and eastern Australia; however, there is little change across the far northern regions. This is likely due to the asymmetric relationship between ENSO and Australian rainfall (Cai and Cowan 2009; Chung and Power 2017), with the lack of a relationship between El Niño and northern Australian rainfall more obvious for central Pacific than eastern Pacific events (Heidemann et al. 2023). Research on the Australian monsoon onset has found similar non-linearities between ENSO events with only strong La Niñas having a considerable association with onsets (Lisonbee and Ribbe 2021). Other studies using the SOI to define ENSO have shown that the Australia’s monsoon retreat is not significantly correlated with ENSO (Drosdowsky 1996; Smith et al. 2008; Zhang 2010; Evans et al. 2014). Uehling et al. (2021) found a significant correlation between austral spring NINO3.4 SST anomalies and rainfall retreat over Cape York and the Top End; however, they did not separately analyse warm and cool ENSO events. For Australia’s far northern tropics, neutral ENSO conditions are associated with earlier retreats. One explanation for this might be that the retreat dates are associated with early autumn westerly wind burst activity in the western Pacific in some of those years when the MJO was tracking strongly in phases 6 and 7 in February–March in an El Niño development period (e.g. 1997, 2004 and 2014; Fedorov 2002; Hu and Fedorov 2019). Another interpretation is that the neutral ENSO relationship to the NRR might be artificial, meaning what we see is not the NRR being pushed earlier on during neutral ENSO, but that the long-term retreat date median is pushed further back by the La Niña relationship. A lead-lag analysis of ENSO with retreat dates suggests that the NRR both lags and leads ENSO (i.e. although correlations are stronger for NRR leading) – this is supported by previous work using the SOI (Evans et al. 2014). The reasons for this require further exploration. In terms of intra-seasonal drivers, the NRR is more likely to happen when the MJO is in phases 6 and 7, slightly earlier than comparative studies showing phases 7 and 8 (Evans et al. 2014; Uehling et al. 2021), although the MJO relationship is regionally and definition dependent. In short, the NRR typically occurs when convection associated with the MJO moves away from northern Australia into the western Pacific (Cowan et al. 2023), prior to the end of April.
One point of contention in our study is the selection of the beginning of the analysis on 1 January and the cut-off on 30 April. The April cut-off date is certainly subjective, designed to be consistent with the backward accumulation definition (Lo et al. 2008) and the end of The Bureau’s official tropical cyclone and wet seasons. We explored the sensitivity of the cut-off by choosing a 31 May end date; this acts to push the NRR across coastal and subtropical regions more into May (Supplementary Fig. S3), likely because of early winter rainfall events or late season ex-tropical cyclones. For example, in late May 2020, ex-tropical cyclone Mangga passed over south-west WA, with a trough to the north impacting the Kimberely coast, causing falls of 50–100 mm in 1 day (Bureau of Meteorology 2020). This event was highly out of season and resulted in logistical problems for one cattle producer trucking cattle for export from Dampier Downs, in the far north-west Kimberely region (A.-M. Huey, pers. comm.). One issue with pushing the cut-off date back to the end of May would be the degeneration of predictability in ACCESS-S2. As shown in Fig. 7, the skill of ACCESS-S2 is mostly limited to the Kimberely, Top End and Cape York regions, and the skill degrades for the backward anomaly and burst definitions. One possibility is to shift the analysis commencement date to February to allow predictions through to the end of January. Onsets earlier than February tend to occur across more arid regions (Fig. 3), and we acknowledge that the NRR is not appropriate (or even definable) across all locations, e.g. hence the restriction to the far northern tropics by Uehling et al. (2021). In fact, moving the start date to 1 February instead of 1 January only affects arid regions, and not the northern tropical regions (Supplementary Fig. S4). Issues to do with short duration wet seasons across northern South Australia and western WA were noted by Lo et al. (2008) using their backward accumulation definition, so this change to a 1 February start date should be considered as a route to improve model accuracy (i.e. shorter lead time).
A core aim of this study was to determine the potential for a NRR forecast product, and if so, whether such a product is suitable over all northern Australia and how to select the most appropriate retreat definition. Our results have clearly shown discernible differences between the three definitions in the observations and, furthermore, ACCESS-S2 is most skilful at predicting the NRR defined from the cumulative anomaly. Based on that skill result alone, the cumulative anomaly would be the natural choice for the NRR definition. However, in terms of skill across Qld as a whole, the backward accumulation definition displays good skill, particularly across the far north-east. Any forecast product would need to be restricted to north of 20°S as forecast skill is poor in the subtropics and arid parts of Australia beyond a week 2 forecast in March and April (Cowan et al. 2022b). Another option is to average the forecasts across the three definitions, or at the very least, weight the definitions based on the model hindcast skill. The issue with an averaging approach is that the forecast average should be compared to an observed average across the three definitions; however, this average observed date would not necessarily relate to an actual rainfall event. For Darwin in 1981, the average NRR across the definitions is 3 April, which does not correspond to any rainfall (Fig. 1). Another option is to specify a NRR date range for each year that spans all three dates, so for Darwin in 1981, that would be 24 March to 16 April, a range of 22 days. Such a broad range in retreat dates may be little use to some livestock producers, although it is worth noting that the interquartile range of NRR is 15–30 days in the Top End (Supplementary Fig. S1b, d, f). Another challenge is coming up with a set ‘climatological’ period with which to calculate the medians and percentiles, and base the cumulative anomaly definition off. Since 1950, there have been noticeable trends in the NRR in north-west WA and south-east Qld (Supplementary Fig. S1a, c, e). In the case of comparing model forecasts or hindcasts with observations, the best approach is to use the hindcast climatological period.
With the forecast question in mind, we next want to highlight the forecasts of the 2023 NRR for the three definitions using 15 and 28 December 2022 and 15 and 28 January 2023 start dates. These forecasts are made up of 11 members over a 9-day lag (seasonal) to create a 99-member super-ensemble, that first undergo a quantile–quantile calibration to remove biases (Griffiths et al. 2023). We want to see the distribution of ACCESS-S2 forecasts that predict an earlier (negative) or later (positive) than the long-term observed (1981–2019) median retreat. In Fig. 8, we show the results for Darwin, whereas Dampier Downs and Charters Towers results are shown in Supplementary Fig. S5. These three locations are where ACCESS-S2 displays the best hindcast skill based on the Brier skill score (Fig. 7). For Darwin, the forecasts are mixed across the definitions. Given that the 2022–23 summer was a La Niña, the statistical relationship points to a later than normal NRR, which is true for backward accumulation and burst definitions (5 days later, stars in Fig. 8); however, this is not the case for the cumulative anomaly definition (2 days early). The majority of forecasts for both December lead times predicted a later Darwin retreat, but in general, there is little change as the lead time reduces, except for fewer outliers in the 28 December forecast.
Forecasts of Darwin’s 2023 retreat date difference from the 1981–2019 observed median, from 15 and 28 December 2022 to 15 and 28 January 2023 start dates. The stars indicate the observed date for each northern rainfall retreat definition. The numbers listed above the box and whisker plots indicate the percentage of the 99 ACCESS-S2 forecasts (per start date) that predicted a later than observed median retreat. The 2023 observed retreats are 2 days early (cumulative anomaly), and 5 days later (backward accumulation and burst). The January forecasts include observations from 1 January through to the day before the start date.
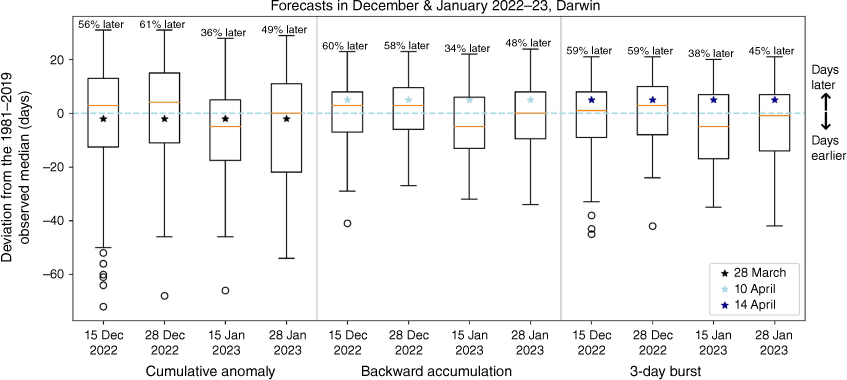
For January, we blend the observations from 1 January to the day prior to the start dates, to ensure we capture any mid-summer rain that is critical for the cumulative anomaly evolution. Surprisingly, we see a shift in the forecasts towards earlier Darwin retreats in the 15 January start date, followed by a shift back towards later dates, but not reaching the same percentages as for the December forecasts. These December and January forecasts indicate the limit of predictability for the NRR, even when the background conditions (i.e. La Niña) might suggest a higher confidence as the lead time reduces. In the late January forecasts, the model suggested a 45–49% likelihood of a later retreat at Darwin, which points to the limitation of such a forecast tool at a time of the year when monsoon depressions and rainfall events are challenging to predict at more than 2 weeks lead time (Cowan et al. 2022a). A similar evolution is seen for Dampier Downs in north-west WA (Supplementary Fig. S5), with a shift in the forecast distribution for the 15 January start date to earlier NRR likelihoods (i.e. the observed retreat dates were more than 20 days later), which produced probabilities of >60% later for the December forecasts. At Charters Towers, the burst definition produces a markedly later retreat (19 April) compared to the other two definitions (14 March), whereas the model forecasts stay within the 45–55% range of a later than normal retreat across the lead times. Therefore, in developing a forecast product, more work is needed on formalising a NRR definition that encompasses the best parts of the three used in this study, but is also meaningful to end users.
To conclude, our study has shown that forecasting the retreat tendency is possible with moderate skill at a ~2.5-month lead time; however, there are prediction challenges. These include (1) the prediction skill of an early or later than usual retreat, which is regionally patchy across large swaths of tropical savanna country in Qld’s Gulf Country and the NT, (2) climate drivers such as ENSO, which are in their demise or transition period in the austral autumn, such that forecasting retreats is more difficult than the onset and (3) the MJO not being the dominant forcing of the NRR, and only skilfully predicted at a lead time of ~30 days. Adding to this, the prediction of burst rainfall amounts that are crucial to the subjective retreat definitions is limited to 2–3 weeks lead time in ACCESS-S2 (Cowan et al. 2022b). At best, The Bureau’s current seasonal model can only provide a general tendency of whether the retreat will be earlier or later than usual but, as shown for 2023, ensemble forecasts vary from week to week. Improving the prediction of rainfall on the multi-week scale, related to processes – such as land–sea dynamics, convection and large-scale processes (Hawcroft et al. 2021) – will be critical in any future advances in forecasting the NRR in newer ACCESS versions. On a practical level, the NRR appears useful to livestock producers as an historical decision date (or range of dates) that nicely accompany their Green Date. Livestock producers can use their Green and retreat dates to make management decisions prior to the dry season, such as forage budgeting and stock numbers. For businesses that deal with infrastructure builds and maintenance or tourism operators, a retreat date may help with long-term operational planning. The next step in the development of a NRR prototype is to verify the long-term observed dates with livestock producers and trial the forecast product on users across the north, particularly those in the red meat and cropping industries.
Data availability
The daily precipitation data used in this study are available on the NCI (National Computational Infrastructure) at https://doi.org/10.25914/6009600b58196. The ACCESS-S2 daily hindcasts are also available on the NCI at https://doi.org/10.25914/60627acc3c33e. The MJO index can be downloaded from http://www.bom.gov.au/climate/mjo/. All scripts used in the analysis and to create the figures are available from the lead author on request.
Declaration of funding
The authors acknowledge the funding of this research through the Northern Australian Climate Program (NACP; grant number P.PSH.1381), in particular Meat and Livestock Australia (through the MLA Donor Company), the Queensland Government through the Drought and Climate Adaptation Program, and the University of Southern Queensland. The title of the project is Agri-Climate Outlooks for Australian Agriculture – Northern Australia Climate Program 3 (NACP3) – Innovative drought and climate variability Research, Development & Extension.
Acknowledgements
The authors thank to Dr Chen Li and Dr Li Shi from the Bureau of Meteorology for their insightful suggestions. Further thanks are extended to the external reviewers, including the Editor Dr Andréa Taschetto for helping to improve the manuscript.
References
Balston J, English B (2009) Defining and predicting the ‘break of the season’ for north-east Queensland grazing areas. The Rangeland Journal 31(1), 151.
| Crossref | Google Scholar |
Berry GJ, Reeder MJ (2016) The Dynamics of Australian Monsoon Bursts. Journal of the Atmospheric Sciences 73, 55-69.
| Crossref | Google Scholar |
Bessafi M, Wheeler MC (2006) Modulation of south Indian Ocean tropical cyclones by the Madden–Julian Oscillation and convectively coupled equatorial waves. Monthly Weather Review 134, 638-656.
| Crossref | Google Scholar |
Bureau of Meteorology (2020) Monthly Weather Review Australia May 2020. (BOM: Melbourne, Vic., Australia) Available at http://www.bom.gov.au/climate/mwr/aus/mwr-aus-202005.pdf
Cai W, Cowan T (2009) La Niña Modoki impacts Australia autumn rainfall variability. Geophysical Research Letters 36, L12805.
| Crossref | Google Scholar |
Chung C, Power S (2017) The non-linear impact of El Niño, La Niña and the Southern Oscillation on seasonal and regional Australian precipitation. Journal of Southern Hemisphere Earth Systems Science 67, 25-45.
| Crossref | Google Scholar |
Cowan T, Stone R, Wheeler MC, Griffiths M (2020) Improving the seasonal prediction of Northern Australian rainfall onset to help with grazing management decisions. Climate Services 19, 100182.
| Crossref | Google Scholar |
Cowan T, Wheeler MC, Day CDB, Nguyen H, Cobon D (2022) Multi‑week prediction of livestock chill conditions associated with the northwest Queensland floods of February 2019. Scientific Reports a 12, 1-13.
| Crossref | Google Scholar |
Cowan T, Wheeler MC, Hudson D, de Burgh-Day C, Griffiths M, Young G (2022b) Skill of ACCESS-S2 in predicting rainfall bursts over Australia. Bureau Research Report - 063.(Bureau of Meteorology) Available at http://www.bom.gov.au/research/publications/researchreports/BRR-063.pdf
Cowan T, Wheeler MC, Sharmila S, Narsey S, de Burgh-Day C (2022c) Forecasting northern Australian summer rainfall bursts using a seasonal prediction system. Weather Forecast 37, 23-44.
| Crossref | Google Scholar |
Cowan T, Wheeler MC, Marshall AG (2023) The combined influence of the Madden–Julian Oscillation and El Niño–Southern Oscillation on Australian rainfall. Journal of Climate 36, 313-334.
| Crossref | Google Scholar |
Drosdowsky W (1996) Variability of the Australian summer monsoon at Darwin: 1957–1992. Journal of Climate 9, 85-96.
| Crossref | Google Scholar |
Drosdowsky W, Wheeler MC (2014) Predicting the onset of the north Australian wet season with the POAMA dynamical prediction system. Weather and Forecasting 29(1), 150-161.
| Crossref | Google Scholar |
Evans S, Marchand R, Ackerman T (2014) Variability of the Australian monsoon and precipitation trends at Darwin. Journal of Climate 27, 8487-8500.
| Crossref | Google Scholar |
Fedorov AV (2002) The response of the coupled tropical ocean–atmosphere to westerly wind bursts. Quarterly Journal of the Royal Meteorological Society 128, 1-23.
| Crossref | Google Scholar |
Griffiths M, Smith P, Yan H, Spillman C, Young G, Hudson D (2023) ACCESS-S2: updates and improvements to postprocessing pipeline. Bureau Research Report – 082. (Bureau of Meteorology) Available at http://www.bom.gov.au/research/publications/researchreports/BRR-082.pdf
Hawcroft MK, Lavender S, Copsey D, Milton S, Rodriguez J, Tennant W, Webster S, Cowan T (2021) The benefits of ensemble prediction for forecasting an extreme event: the Queensland floods of February 2019. Monthly Weather Review 149, 2391-2408.
| Crossref | Google Scholar |
Heidemann H, Ribbe J, Freund M, Power S (2023) Variability and long-term change in Australian monsoon rainfall: a review. WIREs Climate Change 14, e823.
| Crossref | Google Scholar |
Hu S, Fedorov AV (2019) The extreme El Niño of 2015–2016: the role of westerly and easterly wind bursts, and preconditioning by the failed 2014 event. Climate Dynamics 52, 7339-7357.
| Crossref | Google Scholar |
Huang B, Thorne PW, Banzon VF, et al. (2017) Extended Reconstructed Sea Surface Temperature, Version 5 (ERSSTv5): upgrades, validations, and intercomparisons. Journal of Climate 30, 8179-8205.
| Crossref | Google Scholar |
Hudson D, Watkins AB, Ramchurn A, Marshall A (2022) Forewarned is Forearmed (FWFA): equipping farmers and agricultural value chains to proactively manage the impacts of extreme climate events. Prepared by The Bureau of Meteorology, project code B.CCH.8100. (Meat & Livestock Australia: Sydney, NSW, Australia) Available at https://www.mla.com.au/contentassets/c496bd2c72bf4a0584a6d1e39bb7db7b/b.cch.8100-final-repor.pdf
Jaffrés JBD, Cuff C, Rasmussen C, Hesson AS (2018) Teleconnection of atmospheric and oceanic climate anomalies with Australian weather patterns: a review of data availability. Earth-Science Reviews 176, 117-146.
| Crossref | Google Scholar |
Janowiak JE, Xie P (2003) A global-scale examination of monsoon-related precipitation. Journal of Climate 16, 4121-4133.
| Crossref | Google Scholar |
Kajikawa Y, Wang B, Yang J (2010) A multi-time scale Australian monsoon index. International Journal of Climatology 30, 1114-1120.
| Crossref | Google Scholar |
Kalnay E, Kanamitsu M, Kistler R, et al. (1996) The NCEP/NCAR 40-year reanalysis project. Bulletin of the American Meteorological Society 77, 437-472.
| Google Scholar |
Liebmann B, Smith CA (1996) Description of a complete (interpolated) outgoing longwave radiation dataset. Bulletin of the American Meteorological Society 77, 1275-1277.
| Google Scholar |
Liebmann B, Camargo SJ, Seth A, Marengo JA, Carvalho LMV, Allured D, Fu R, Vera CS (2007) Onset and end of the rainy season in South America in observations and the ECHAM 4.5 atmospheric general circulation model. Journal of Climate 20, 2037-2050.
| Crossref | Google Scholar |
Lisonbee J, Ribbe J (2021) Seasonal climate influences on the timing of the Australian monsoon onset. Weather and Climate Dynamics 2, 489-506.
| Crossref | Google Scholar |
Lisonbee J, Ribbe J, Wheeler M (2019) Defining the north Australian monsoon onset: a systematic review. Progress in Physical Geography: Earth and Environment 44, 398-418.
| Crossref | Google Scholar |
Lo F, Wheeler MC, Meinke H, Donald A (2007) Probabilistic forecasts of the onset of the north Australian wet season. Monthly Weather Review 135, 3506-3520.
| Crossref | Google Scholar |
Mollah WS, Cook IM (1996) Rainfall variability and agriculture in the semi-arid tropics—the Northern Territory, Australia. Agricultural and Forest Meteorology 79, 39-60.
| Crossref | Google Scholar |
Narsey S, Reeder MJ, Ackerley D, Jakob C (2017) A midlatitude influence on Australian monsoon bursts. Journal of Climate 30, 5377-5393.
| Crossref | Google Scholar |
Noska R, Misra V (2016) Characterizing the onset and demise of the Indian summer monsoon. Geophysical Research Letters 43, 4547-4554.
| Crossref | Google Scholar |
Sharmila S, Hendon HH (2020) Mechanisms of multiyear variations of Northern Australia wet-season rainfall. Scientific Reports 10, 5086.
| Crossref | Google Scholar | PubMed |
Smith IN, Wilson L, Suppiah R (2008) Characteristics of the northern Australian rainy season. Journal of Climate 21, 4298-4311.
| Crossref | Google Scholar |
Tsai WY, Lu M-M, Sui C-H, Cho Y-M (2021) Subseasonal forecasts of the northern Queensland floods of February 2019: causes and forecast evaluation. Atmosphere 12, 758.
| Crossref | Google Scholar |
Uehling J, Misra V (2020) Characterizing the seasonal cycle of the northern Australian rainy season. Journal of Climate 33, 8957-8973.
| Crossref | Google Scholar |
Uehling J, Misra V, Bhardwaj A, Karmakar N (2021) Characterizing the local variations of the northern Australian rainy season. Monthly Weather Review 149, 3995-4004.
| Crossref | Google Scholar |
Wedd R, Alves O, de Burgh-Day C, et al. (2022) ACCESS-S2: the upgraded Bureau of Meteorology multi-week to seasonal prediction system. Journal of Southern Hemisphere Earth Systems Science 72, 218-242.
| Crossref | Google Scholar |
Wheeler MC, Hendon HH (2004) An all-season real-time multivariate MJO index: development of an index for monitoring and prediction. Monthly Weather Review 132, 1917-1932.
| Crossref | Google Scholar |
Wheeler MC, McBride JL (2005) Australian–Indonesian monsoon. In ‘Intraseasonal Variability in the Atmosphere–Ocean Climate System’. (Eds WKM Lau, DE Waliser) pp. 125–173. (Springer) 10.1007/3-540-27250-X_5
Zeng X, Lu E (2004) Globally unified monsoon onset and retreat indexes. Journal of Climate 17, 2241-2248.
| Crossref | Google Scholar |
Zhang H (2010) Diagnosing Australia–Asian monsoon onset/retreat using large-scale wind and moisture indices. Climate Dynamics 35, 601-618.
| Crossref | Google Scholar |
Footnotes
1 Queensland Country Life, Thursday 29 April 2021, see https://futurebeef.com.au/wp-content/uploads/2021/04/2021-04-29-CQBeef.pdf.
2 Severe Tropical Cyclone Ilsa, 6–15 April 2023, summary, see http://www.bom.gov.au/cyclone/history/Ilsa23.shtml.
3 Severe Tropical Cyclone Fay, 16–28 March 2004, Summary, see http://www.bom.gov.au/cyclone/history/fay.shtml.
4 See OLR for January–March at http://www.bom.gov.au/climate/mjo/#tabs=Averages