Global ocean surface and subsurface temperature forecast skill over subseasonal to seasonal timescales
Grant A. Smith

A
Abstract
Subseasonal to seasonal forecasts of ocean temperatures, including extreme events such as marine heatwaves, have demonstrated utility in informing operational decision-making by marine end users and managing climate risk. Verification is critical for effective communication and uptake of forecast information, together with understanding ocean temperature predictability. The forecast skill of surface and subsurface ocean temperature forecasts from the Bureau of Meteorology’s new ACCESS-S2 seasonal prediction system are assessed here over an extended 38-year hindcast period, from 2 weeks to 6 months into the future. Forecasts of sea surface temperature (SST), heat content down to 300 m (HC300), bottom temperatures on continental shelves, and mixed layer depth are compared to both satellite observations and ocean reanalyses for the globe and the Australian region, using a variety of skill metrics. ACCESS-S2 demonstrates increased SST skill over its predecessor ACCESS-S1 at subseasonal timescales for all variables assessed. Heat content skill is particularly high in the tropics but reduced in subtropical regions especially when compared to persistence. Forecast skill for ocean temperature is higher in the austral summer months than winter at lead times up to 2 months in the Western Pacific region. Mixed layer depth is poorly predicted at all lead times, with only limited areas of skill around Australia and in the south-west Pacific region. Probability of exceedance forecasts for the 90th percentile as an indicator for marine heatwave conditions, shows adequate skill for SST, HC300 and bottom temperatures, especially near shelf regions at shorter lead times. This work will underpin the future development of an operational marine heatwave forecast service, which will provide early warning of these events and thus valuable preparation windows for marine stakeholders.
Keywords: ACCESS-S, heat content, marine heatwave, mixed layer depth, model skill, sea surface temperature, seasonal prediction, validation.
1.Introduction
The world’s oceans are warming, which combined with increasingly frequent marine heatwaves are heavily affecting marine industries and ecosystems (Masson-Delmotte et al. 2021). Marine heatwaves are estimated to have cost billions of dollars globally in the last 20 years (Smith et al. 2021). Impacts include fish kills and fishery closures (Caputi et al. 2019), changes in species migration paths (Smale et al. 2019), loss of habitat such as seagrass (Babcock et al. 2019), coral bleaching (Hughes et al. 2021) and changes in ecosystem community composition. These in turn affect coastal communities through loss of income (e.g. less tourism and reduced fishing catch), reduced success in restoration efforts and decreased coastal protection (Hartog et al. 2023).
Three of the challenges of the UN Decade for the Ocean for Sustainable Development 2021–2030 (https://oceandecade.org) are increasing community resilience to hazards (Challenge 6), sustainable fisheries (Challenge 3) and protect and restore marine ecosystems (Challenge 2). One valuable tool to help address these challenges is ocean forecasting on subseasonal to seasonal timescales. As climate pressures increase on Australia’s marine sector, there is increased demand for information around extreme events such as marine heatwaves (Hartog et al. 2023). Advance warning of anomalously warm ocean temperatures as well as marine heatwaves can provide a preparation window for marine users to implement strategies to either mitigate against impacts or capitalise on opportunities (Spillman et al. 2021). Marine managers can use this information to inform operational activities and strategic planning, brief stakeholders in advance of extreme events and better manage resources.
Accurate subseasonal (weekly and fortnightly) to seasonal forecasts have been demonstrated to support proactive management and enhanced decision making by marine stakeholders (Tommasi et al. 2017). The Australian Bureau of Meteorology has been providing an operational sea surface temperature- (SST) based seasonal outlook service for Australian waters since 2009 (Spillman 2011), upgrading to the Australian Community Climate Earth System Simulator-Seasonal (ACCESS-S) system in 2018 (Smith and Spillman 2019; Spillman and Smith 2021). Seasonal forecast products have been developed in collaboration with industry and managers for coral bleaching risk around Australia (Spillman and Smith 2021) and globally by NOAA Coral Reef Watch (Liu et al. 2018). In the fisheries sector there has been engagement for salmon aquaculture (Spillman and Hobday 2014), long-line multispecies wild fisheries (Hobday et al. 2011; Eveson et al. 2015) and recreational fisheries (Brodie et al. 2017). Preliminary marine heatwave prediction products are running in trial mode (Benthuysen et al. 2021; Spillman et al. 2021). Jacox et al. (2022) routinely produce forecasts of probability of monthly SST exceeding 90th percentile, showing predictability up to 10 months in advance depending on region, season and presence or absence of an El Niño–Southern Oscillation (ENSO) event.
These cutting-edge forecast products are based on SST, in line with the typical marine heatwave definition (Hobday et al. 2018). One of the primary reasons for this is that global high-resolution satellite-derived SST datasets are readily available, and as such climate models can be well initialised at the ocean surface for subseasonal to seasonal forecasting timescales (Smith and Spillman 2019). The adoption of SST as the basis for marine heatwaves assumes that either the particular application only requires representation of shallow depths, or that the mixed layer is relatively deep. However, subsurface ocean temperature information is also important for dynamical marine management (Amaya et al. 2023). Heat content of the upper water column is an important driver of health and productivity for fisheries and aquaculture, e.g. mussel ropes at depth and fish generally forage deeper than in the top layer of the water column (SST). The depth of the mixed layer is an important consideration for nutrient availability, primary productivity, light attenuation and food availability. The depth of this layer is also important in recharging marine heatwaves and is related to the duration of events (Alexander et al. 2018; Holbrook et al. 2020; Scannell et al. 2020). Ocean temperatures down to the continental shelf seafloor influence the health and distributions of coastal fish and benthic species such as seagrass, oysters and scallops.
Three-dimensional climate models and data reanalyses can provide insight into ocean subsurface conditions. Data reanalyses blend both surface and subsurface observations with a high degree of spatial and temporal heterogeneity to give a best estimate of the current ocean state (Oke et al. 2013; Wedd et al. 2022). These reanalyses can be utilised both to initialise climate forecasts or hindcasts and as forecast verification datasets. Subsurface observations include those provided by Argo floats, expendable bathythermographs (XBTs), drifting buoys and the TAO-TRITON array. The introduction of the Argo program in 2000 dramatically increased the coverage and density of subsurface ocean observations; however, these are still considerably fewer in both space and time than surface measurements and so estimates of the ocean state at depth have a wider range of error (Vidard et al. 2007; Gwyther et al. 2022). The impact of ocean data assimilation on forecast skill has been assessed for various models and outputs including sea surface height (e.g. Widlansky et al. 2023) and SST (e.g. Wedd et al. 2022), with improved initialisation leading to better forecast skill. Assessments of subsurface forecast skill are important not only to communicate confidence to potential end users of forecast information (e.g. Hobday et al. 2011), but also to improve the understanding of ocean predictability, particularly for extreme events. As subsurface conditions play a role in marine heatwave recharge (Holbrook et al. 2020), marine heatwave forecast skill will be influenced by both surface and subsurface temperature forecast skill.
In this study we assessed surface and subsurface ocean temperature forecast skill of ACCESS-S version 2 (ACCESS-S2), the current operational seasonal prediction system at the Australian Bureau of Meteorology (Hudson et al. 2017; Wedd et al. 2022). This assessment will support both the Bureau’s operational ocean temperature outlook (http://www.bom.gov.au/oceanography/oceantemp/sst-outlook-map.shtml) and new prototype marine heatwave forecast products in development by the Bureau and CSIRO (Spillman et al. 2021; Hartog et al. 2023). ACCESS-S2 was operationalised in October 2021 and includes a new weakly coupled ocean data assimilation scheme as well as a considerably longer hindcast (retrospective forecast) period of 1981–2018 (Wedd et al. 2022). This 38-year hindcast that runs out to 9 months into the future, together with the ocean reanalysis dataset used to initialise ACCESS-S2, was used to assess the skill of the system. We assessed SST skill globally as well as subsurface skill for the first time, specifically heat content, mixed layer depth and bottom temperatures on the Australian continental shelf. This comprehensive assessment will provide confidence in ACCESS-S ocean forecast products, both those currently in operations and new tools in development, to better inform marine stakeholder decision-making to manage climate risk.
2.Methods
2.1. Model description
ACCESS-S2 is based on the UK Met Office (UKMO) Global Coupled model 2.0 (GC2) (Williams et al. 2015), which comprises Global Atmosphere 6.0 (GA6.0), Global Land 6.0 (GL6.0), Global Ocean 5.0 (GO5.0) and Global Sea Ice 6.0 (GSI6.0). Both GA6.0 and GL6.0 are fully documented by Walters et al. (2017), whereas GO5.0 is described by Megann et al. (2014) and GSI6.0 by Rae et al. (2015). The ocean model (NEMO) grid resolution is ~25 × 25 km in the horizontal between latitudes 50°N and 50°S with 75 vertical layers down to the ocean floor. The top surface layer is 1 m thick and there are 35 levels in the top 300 m. The geographic resolution in ACCESS-S is high enough to resolve mesoscale eddies and currents in the tropics and some of the mid latitudes and is defined as ‘eddy permitting’ (Marsh et al. 2009).
The primary differences between ACCESS-S2 and its predecessor ACCESS-S1 are the implementation of a new ocean data assimilation system, developed by the Bureau of Meteorology, an ensemble generation scheme strategy with a lagged ensemble for the hindcasts to match more closely the realtime forecasts and allow a larger ensemble, and a longer hindcast dataset (1981–2018 vs 1990–2012) (Wedd et al. 2022). The ocean data assimilation system is a basic version of the Bureau of Meteorology and CSIRO coupled EnKF-C software developed by Sakov (2014) with Ensemble Optimal Interpolation (EnOI) configuration. Satellite SST, in situ temperature and salinity profiles (Argo, XBTs, drifting buoys and TAO-TRITON array) are assimilated on a weakly coupled daily cycle, with nudging of SST towards observations. The Reynolds OISSTv2 satellite SST product was used up to December 2013 in the hindcast (Reynolds et al. 2007; Banzon et al. 2016; Huang et al. 2021), and from January 2014 onwards and in realtime used the Global Australian Multi-Sensor SST Analysis (GAMSSA; Zhong and Beggs 2008) product. Altimetry is not assimilated in ACCESS-S2, unlike ACCESS-S1 that used initial conditions supplied by the UKMO (see Widlansky et al. 2023 for comparisons). The initial conditions of sea ice are from the coupled assimilation system but without the assimilation of observed sea ice, which allows sea ice to vary during the assimilation in response to the assimilation of the ocean observation and the atmospheric initial condition. For more details on the differences between ACCESS-S1 and ACCESS-S2, see Wedd et al. (2022).
A set of retrospective forecasts (hindcasts) have been generated for the first day of each month out to 9 months for the period September 1981 to December 2018. In this study, hindcast data from 1981 (partial year) were omitted and the period 1982–2018 was used. For each start date, a time lagged 27-member ensemble has been produced. For monthly forecasts, three ensemble member forecasts are produced each day starting on the first day of the month and counting back in time up to 8 days prior. For example, for a hindcast starting on 1 April, the time lagged ensemble would include three forecasts run on each of the days from 23 March to 1 April. Multi-week forecasts also have 27 ensemble members but have nine ensemble members a day counting back 3 days. For each day, ensembles are generated by perturbing atmospheric conditions within the tolerance of observations and allowing the errors to propagate through the forecasts to provide an estimate of the range of possible outcomes (Wedd et al. 2022). Ensemble mean values were calculated for each time slice by averaging the 27 ensemble members.
The primary hindcast variables assessed were SST (°C), depth integrated heat content to 300 m (HC300), mixed layer depth (MLD) and bottom temperature down to 200 m (BT200, °C). The SST is defined as the temperature of the top layer (~1-m thickness) of the ocean model. The HC300 is calculated by ACCESS-S2 as follows:
where cp is the specific heat capacity of sea water (~4000 J kg–1 K–1), ρ is the sea water density (~1024 kg m–3) and T is the temperature at depth. Note that HC300 is also calculated for locations where the depth of the water column is less than 300 m, using the total depth available. The MLD is defined in ACCESS-S2 as the depth where the density increases 0.01 kg m–3 from the reference depth of 10 m.
The BT200 was extracted by finding the deepest wet ocean cell at each location on the Australian continental shelf to a maximum depth of 200 m. Each of these variables were averaged to give monthly and fortnightly values of SST and HC300, and monthly only for MLD and BT200 for the hindcast period 1982–2018 (BT200 has a shorter period 1993–2018 to match the available reanalysis period used for verification).
Fortnightly (weeks 1 & 2 and weeks 3 & 4) and monthly model climatologies were calculated for each month and lead time in the hindcast period, where lead time is defined as the time between model start date and forecast date. For example, for a forecast issued for 1 April (which includes forecasts from 1 April and 31–30 March), weeks 1 & 2 correspond to 1–13 April and weeks 3 & 4 to 14–27 April. Monthly forecasts (which include forecasts from 1 April and 31–24 March) at lead times 0, 1 and 2 months are for April, May and June respectively. Three monthly seasonal assessments for December–January–February (DJF) and June–July–August (JJA) are produced by averaging the three monthly lead times that fall within each seasonal window. For example, for forecasts issued on 1 April, the seasonal forecast for JJA is formed by averaging lead times of 2, 3 and 4 months (i.e. June, July and August in the forecast).
Only forecasts starting on the first day of the month are considered here. These model climatologies were then removed from the fortnightly and monthly model values to give anomalies for all four variables. Anomalies were calculated for all 27 ensemble members and the ensemble mean. The 90th percentile was also calculated using all ensemble members for fortnightly and monthly SST and HC300 anomaly forecasts as this is the defined threshold for the onset of a marine heatwave (Hobday et al. 2018).
2.2. Ocean observations and reanalyses
This section provides a summary of the data products used for forecast verification. Daily SST values from Reynolds OISSTv2.1 (Reynolds et al. 2007; Banzon et al. 2016; Huang et al. 2021) for 1982–2018 were averaged to give fortnightly and monthly values. Fortnightly and monthly climatologies were calculated as the long-term average for each fortnight (weeks 1 & 2 and weeks 3 & 4) or month in the period. Observed SST anomalies were then generated by removing the appropriate fortnightly or monthly SST climatology from the fortnightly or monthly observed SST data.
Ocean reanalyses have been used in recent subsurface temperature skill assessments, chosen for their benefits in geographic and temporal coverage along with compatibility of grid resolution with NEMO-based coupled models (e.g. McAdam et al. 2022, 2023). Monthly HC300 and MLD values were extracted from the ECMWF Ocean Reanalysis System 5 (ORAS5; Zuo et al. 2019) as an independent dataset for 1982–2018. ORAS5 has the same ocean grid resolution as ACCESS-S2 and currently consists of monthly data only for the period 1958–2018 (Zuo et al. 2019). Fortnightly HC300 values were calculated from the Global Reanalysis Ensemble Product (GREP) daily data using subsurface temperatures down to a depth of 300 m (see Eqn 1) with a constant ocean density and specific heat capacity. The GREP data are only available for 1993–2018 and include the four global ocean-ice reanalyses C-GLORSv7 (Storto et al. 2015), FOAM-GloSea5 (MacLachlan et al. 2015), GLORYS2v4 (Lellouche et al. 2013) and ORAS5. Monthly bottom temperatures (BT200) for 1993–2018 were extracted from GLORYS2v4 (Garric et al. 2018).
2.3. Verification metrics
All verification metrics mentioned herein are evaluated over the hindcast periods defined alongside each variable mentioned in Section 2.1, with periods limited by their associated observational datasets. Pearson correlation values were calculated between fortnightly and monthly model ensemble means and corresponding observed–reanalysis values for SST, HC300 and MLD (BT200) anomalies. All months were used in the global skill analysis. Datasets were not detrended prior to analysis as ACCESS-S2 operational products also include trends, largely due to their presence in the initial conditions. This may lead to higher skill scores than what would occur using detrended datasets. Persistence forecasts were created by persisting recent observed–reanalysis anomalies for the duration of the forecast. Correlations between persistence and observations were then calculated to provide a minimum skill benchmark (Smith and Spillman 2019). Root mean square errors (RSME) were also calculated between the observed–reanalysis and model ensemble mean values to assess the ability of the model to capture the magnitude of observed values. Standard deviations (s.d.) of the model forecasts and observations–reanalyses were compared, and also used to normalise RMSE values. The s.d. was based on the ensemble mean for weeks 1 & 2, weeks 3 & 4 and monthly lead times across the entire hindcast period relevant for the variable being assessed.
Relative Operating Characteristic (ROC) scores indicate the model ability to forecast and discriminate between events (exceeding the 90th percentile) and non-events (below the 90th percentile), known as model resolution (Mason 1982). A series of probabilities between 0 and 1 are used to signify hit or miss thresholds, with probability calculated as a ratio between ensemble members above the 90th percentile and the total ensemble size (27 members). A hit rate, or probability of detection, is calculated at each grid point for each probability increment (0.1–1.0 at 0.1 incremental bins). The model forecast is termed as a ‘hit’ if the proportion of ensemble forecasts correctly indicating >90th percentile is greater than each probability bin. Conversely if less than the proportion of ensemble members correctly predicted >90th percentile than each probability bin then this was termed a ‘miss.’ (see Wilks 2006). These ‘hit’ and ‘miss’ counts can be combined to calculate the hit rate as follows:
where x,y is the grid point location, lt is lead time and t is model start date. Along with the hit rate, a false alarm rate is also calculated in a similar fashion; it describes the ratio between false alarms, i.e. when the model incorrectly predicts an event occurring, and compares it to the total number of false alarms and correct negatives, i.e. correct non-event predictions.
Hit rates are plotted against corresponding false alarm rate values for each probability threshold between 0 and 1, with the area under this curve known as a ROC score. A good score is within 50–100%, and scores below 50% indicate that the model is no better than random chance.
3.Results
3.1. Global
Correlations between model and observed SST anomalies for both ACCESS-S1 and ACCESS-S2 on both subseasonal and seasonal timescales are presented in Fig. 1a, b. The first two rows are for lead times weeks 1 & 2 and weeks 3 & 4, and months 0, 1, 3 and 5 are in the following three rows. The final column (Fig. 1c) shows the difference between ACCESS-S1 and ACCESS-S2 correlations. The period assessed here was limited to the ACCESS-S1 hindcast period of 1990–2012 for an equitable comparison. ACCESS-S2 shows improved skill across the tropics and north and southern Atlantic–Pacific–Indian Oceans at weeks 1 & 2, with the most notable improvement in the tropical Atlantic and the storm belt of southern Indian Ocean (Fig. 1, row 1). Conversely ACCESS-S1 shows considerably better skill in the Southern Ocean near Antarctica as well as slightly higher skill in high eddy kinetic energy regions such as Tasman Sea, Kuroshio Current, Agulhas Current retroflection and the Falklands–Malvinas Confluence for weeks 1 & 2. Skill decreases for both models in weeks 3 & 4, with ACCESS-S1 skill exceeding that of ACCESS-S2 in an increasing number of locations. From lead time of 1 month onwards, monthly skill is largely comparable between the two models, especially in the ENSO index regions of the equatorial Pacific. At longer lead times, ACCESS-S2 maintains higher skill in the tropical Atlantic Ocean, though loses skill more rapidly than ACCESS-S1 in the North Atlantic near Greenland.
Global SST anomaly ensemble mean forecast correlations with observations (OISSTv2.1) for (a) ACCESS-S1, (b) ACCESS-S2 and (c) ACCESS-S2 minus ACCESS-S1 for all start dates in 1990–2012 for lead times weeks 1 & 2, weeks 3 & 4 and 0, 1, 3 and 5 months. Only statistically significant skill is shown (P < 0.05, n = 276) with white indicating regions of no statistical significance.
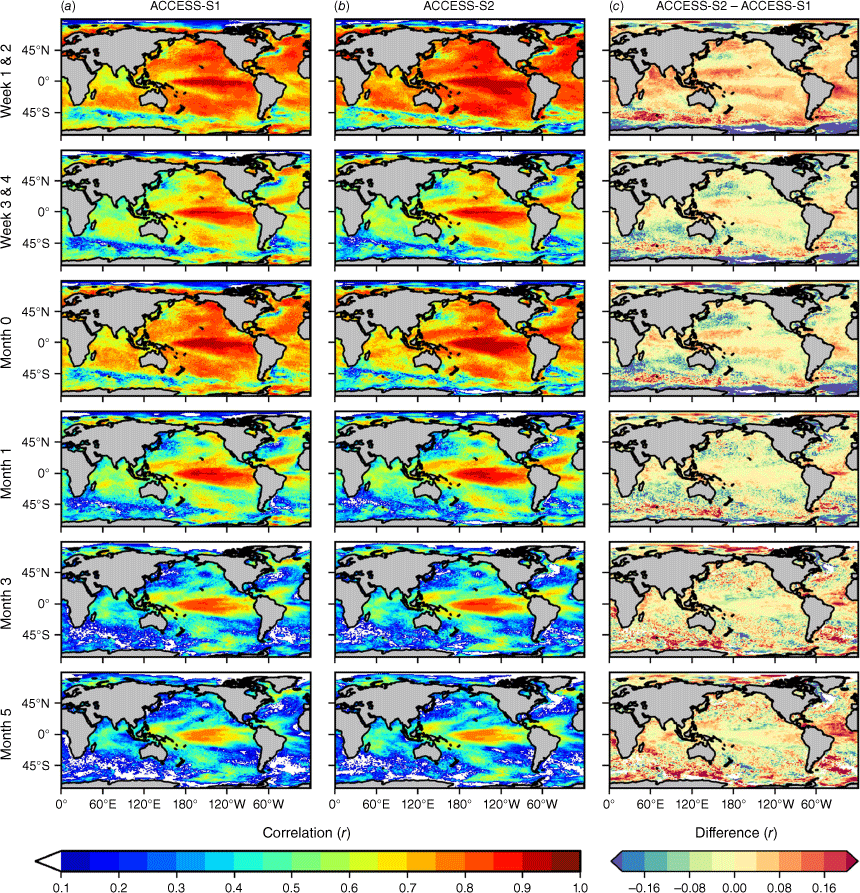
Fig. 2 shows ACCESS-S2 skill for SST anomalies for the extended hindcast period of 1982–2018 for all start dates at lead times of weeks 1 & 2, weeks 3 & 4 and 0, 2 and 4 months. Heat content was assessed for 1993–2018 for the same fortnightly periods, and 1982–2018 for monthly. Regions where persistence skill is higher than that of the model are indicated with crosshatching. Model SST anomaly correlations are highest (r > 0.8) across the tropical oceans (30°N–30°S) as well as in the far northern Pacific and North Atlantic at month 0, and generally beat persistence in these locations (Fig. 2a). Conversely, model HC300 anomaly correlations are higher than that of SST anomalies at the equator but lower in the subtropics at lead time 0 for both fortnightly and monthly prediction, and typically do not beat persistence except for a narrow equatorial band (Fig. 2b). Skill for a post Argo period (2000–2018) is likely to show an improvement compared to pre-2000 from the increased number of subsurface observations. Both the ORA5 and ACCESS-S2 reanalysis products used to ground truth the model will both suffer a similar deficiency as they also assimilate the subsurface observations available at the time, and therefore a comparison between the two time periods will not shed any light on this hypothesis. Model anomaly skill for both SST and HC300 decreases with lead time. Skill distributions are similar for SSTA and HC300 anomalies for lead times of 2 and 4 months, with skill lowest in dynamic areas driven by western boundary currents, and both show a greater reduction between months 0 and 2 than between months 2 and 4. Persistence SST anomaly skill decreases more rapidly than that of the model with lead time, indicated by progressively less hatching as lead time increases (Fig. 2a). By month 4, model SST anomaly forecasts are more skilful in most ocean basins apart from the Southern Ocean. However, while HC300 anomaly persistence skill also decreases with lead, it still beats, or is comparable to, model skill for most of the domain at lead time of 4 months (Fig. 2b).
Global ACCESS-S2 correlations for (a) SSTA with observations (Reynolds OISSTv2.1) and (b) HC300 with observations (calculated from daily GREPv2 subsurface temperatures for fortnightly, and ORAS5 for monthly) for all start dates in 1982–2018 (except for HC300 fortnightly, which is 1993–2018) for lead times weeks 1 & 2, weeks 3 & 4 and 0, 2 and 4 months. Hatching shows where model forecast skill is lower than persistence forecast skill. Only statistically significant model skill is shown (P < 0.05, n = 444) with white indicating regions of no statistical significance.
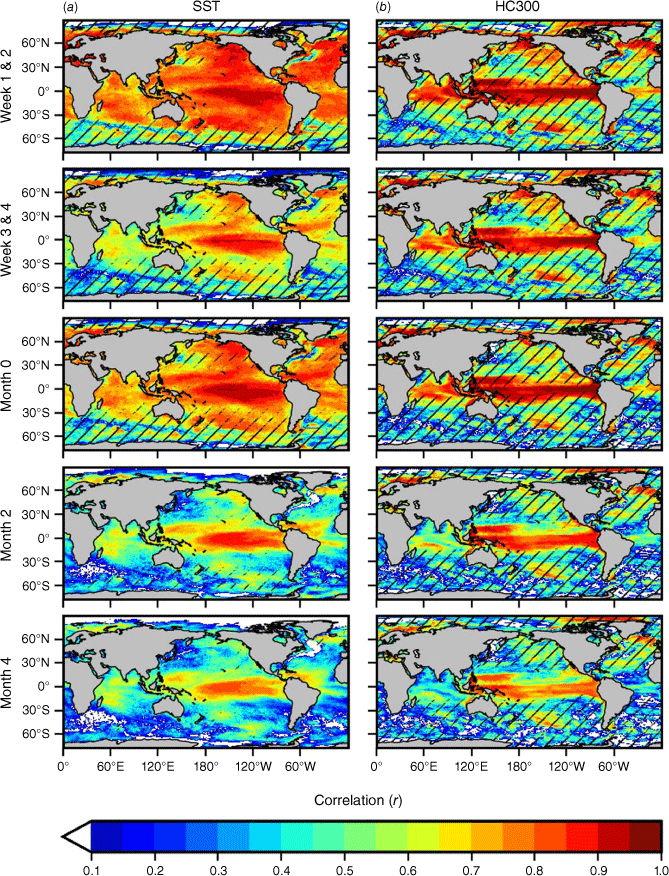
Fig. 3 shows model SST and HC300 anomaly s.d. values minus observational s.d. for all model forecasts for all months, normalised by observational s.d. Results for SST and monthly HC300 are for the period 1982–2018 and fortnightly HC300 for the period 1993–2018 (observation limited). Model SST s.d. values are similar to observations across most of the globe for shorter lead times (Fig. 3a, weeks 1 & 2, weeks 3 & 4 and month 0) apart from a region of the far southern Pacific and South Atlantic Ocean. At lead times of 2 and 4 months, the model variability is lower than observations across much of the globe, except in the tropical Pacific, Atlantic, and sections of the Southern Ocean. The tendency for model SST to slowly revert to climatology with lead time, represented here by the reduction in model ensemble mean s.d. with lead time, is an expected behaviour and summarised as part of model drift discussions in Manzanas (2020). For HC300, fortnightly forecasts show areas of model s.d. being significantly higher than observations (Fig. 3b, weeks 1 & 2 and weeks 3 & 4), especially in the Southern Ocean, South Atlantic and highly eddy kinetic regions associated with western boundary countries. From lead time of 0 month onwards, the HC300 model variability aligns more closely with the observations in these regions, but equatorial variability in the Indian and Pacific Ocean emerges as being slightly lower than observations.
Global ACCESS-S2 minus observational standard deviations for (a) SSTA with observations (OISSTv2.1) 1982–2018 and (b) HC300 with observations (calculated from daily GREPv2 subsurface temperatures for fortnightly, and ORAS5 for monthly) 1993–2018 (except for HC300 fortnightly, which is 1993–2018) for all months and lead times of weeks 1 & 2, weeks 3 & 4 and 0, 2 and 4 months.
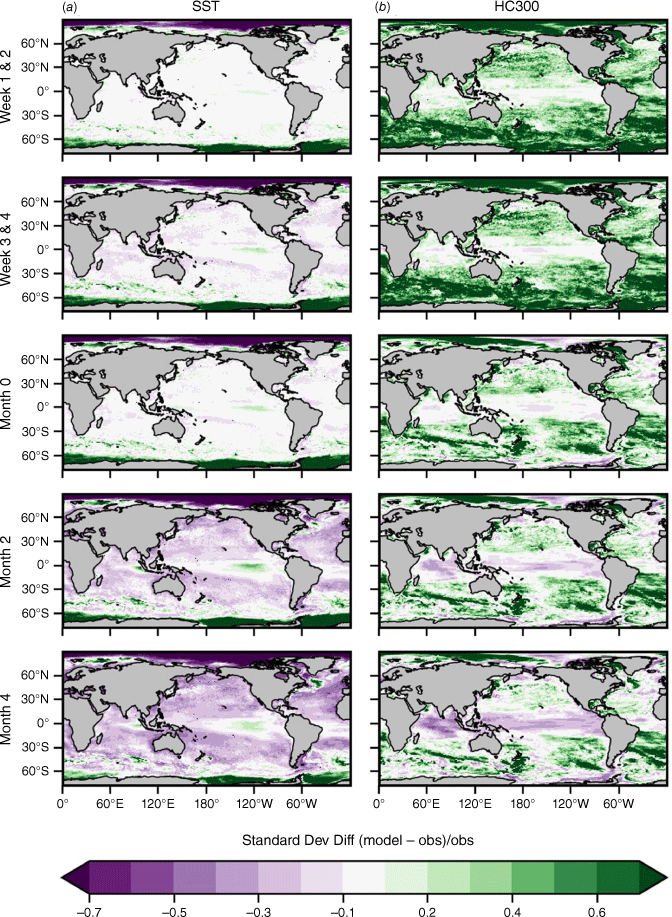
Fig. 4 presents RMS error normalised by s.d. of the observations (nRMSE) for model SST and HC300 anomalies for all start dates for 1982–2018 (HC300 fortnightly is 1993–2018). A value of 0 indicates a perfect forecast. The distributions of nRMSE <1 are similar for both SST and HC300 anomalies, though nRMSE for SST anomalies is lower over a greater proportion of the global domain at lead time of 0 months. Lowest relative nRSME values occur in the equatorial Pacific for both variables at all lead times shown (Fig. 4). Conversely, highest nRMSE values occur in the dynamic eddy fields near to western boundary currents and in the Southern Ocean and Antarctic Circumpolar Current for both variables, despite normalising with observational s.d., with higher nRMSE values for HC in the extratropics (especially in the Southern Ocean and South Atlantic). Lower nRMSE values for SST anomalies are maintained at month 4 than in HC300 anomalies.
Global ACCESS-S2 RSME normalised by standard deviation for (a) SSTA with observations (OISSTv2.1) and (b) HC300 with observations (calculated from daily GREPv2 subsurface temperatures for fortnightly, and ORAS5 for monthly) for all start dates in 1982–2018 (except for HC300 fortnightly, which is 1993–2018) for lead times of weeks 1 & 2, weeks 3 & 4 and 0, 2 and 4 months.
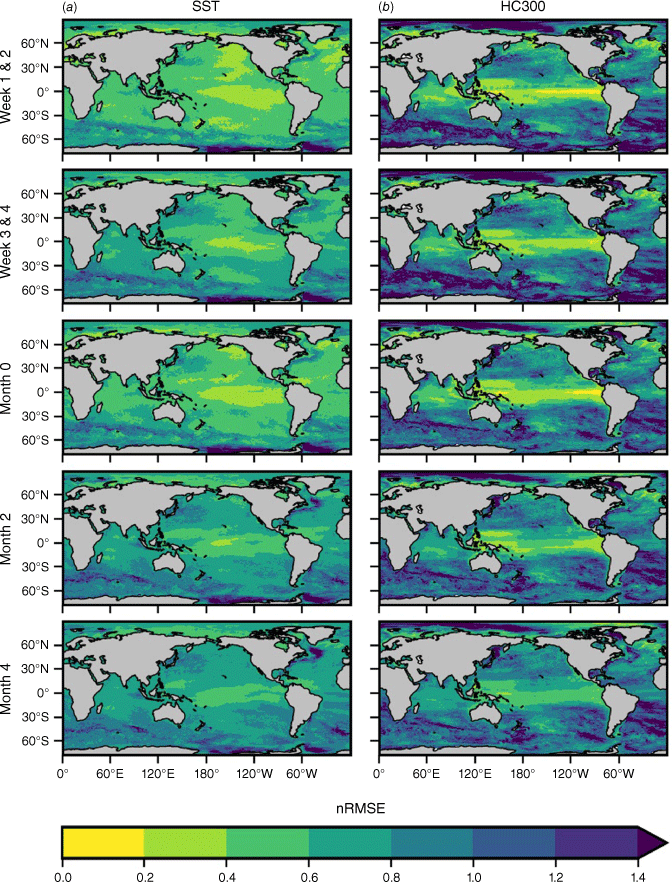
3.2. Australia and the south-west Pacific
Fig. 5 shows SST, HC300 and MLD anomaly correlation coefficients for target seasons DJF (austral summer) and JJA (austral winter) for lead times 0, 1 and 2 months. Both SST and HC300 anomalies show statistically significant skill across almost the entire Australian and south-west Pacific regions for DJF and JJA out to a lead time of 2 months. For SST, anomaly correlation coefficients are highest in the tropical Pacific for all lead times and seasons shown, though correlations are stronger in summer (DJF) indicating the predictability of ENSO that is higher towards the end of the calendar year and lowest in April–June (Wedd et al. 2022). Anomaly correlations do not degrade significantly for either season at longer lead times, although some reduction along the Australian coast and around New Zealand is evident. The SST anomaly correlations in winter (JJA; Fig. 5a) are higher across northern Australia, in the Coral Sea and around the maritime continent at longer lead times, than in summer (DJF; Fig. 5a). The HC300 anomaly correlations have similar distributions for DJF and JJA, centred closer towards the western warm pool region, with slightly higher values for summer (DJF; Fig. 5b) than winter (JJA; Fig. 5b). A thin band of lower correlations can be seen just north of the equator in winter at all lead times. The MLD anomaly correlation coefficients (Fig. 5c) are much lower than values for both SST and HC300, even at lead time of 0 month. Skill tends to be both highest off north-west Australia and either side of the equator in the Pacific for both seasons. Correlations are statistically significant over a greater proportion of the domain in winter when there is a higher signal to noise ratio than in summer. The proportion of the region shown in Fig. 5 that has statistically significant skill for MLD is ~50% at lead time of 0 for both seasons. This proportion drops to 30% at lead times of 1 and 2 months for DJF, with slightly higher values of 36 and 32% for 1 and 2 months respectively for JJA. Regions where anomaly correlations are not statistically significant at all lead times include the equatorial Pacific and south-west Pacific in summer and the western warm pool during winter (Fig. 5c).
Monthly anomaly correlations in the Australasian region for target season December–January–February (DJF) for model (a) SSTA, (b) HC300 and (c) MLD with observations (OISSTv2.1, ORAS5 and ORAS5 respectively) in 1982–2018 at lead times 0, 1 and 2 months. Similarly for target season June–July–August (JJA) for model (d) SSTA, (e) HC300 and (f) MLD with observations for the same period and lead times. Only statistically significant model skill is shown (P < 0.05, n = 37) with white indicating regions of no statistical significance.
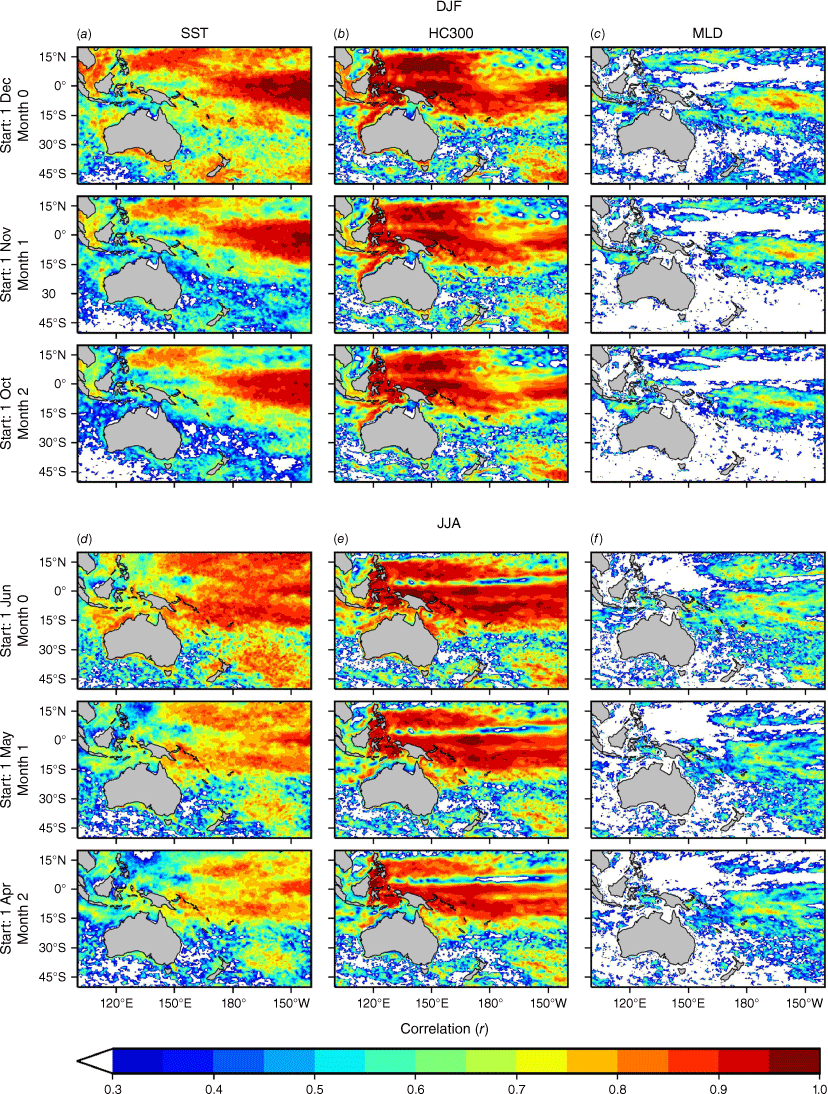
3.3. Australian region
Fig. 6 presents a closer look at MLD anomaly correlations in the Australian region (Fig. 6a), together with bottom depth temperature anomaly correlations (Fig. 6b), out to a lead time of 2 months. Model MLD anomaly correlations are higher across tropical Australia and lower in the subtropics and temperate regions at a lead time of 0 months (Fig. 6a). The model correlation remains high in the north in the Coral Sea and offshore of the North-west Shelf at lead times of 1 and 2 months, but drops below statistically significant thresholds in the Gulf of Carpentaria by 2 months. Model anomaly correlations remain acceptable in patches in the Great Australian Bight and around Tasmania from lead times of 1 month onwards. For bottom temperatures (i.e. temperature of the bottom layer of the water column down to 200-m depth) (Fig. 6b), model skill is statistically significant across the relevant domain at all lead times, with the lowest values in the Gulf of Carpentaria at lead times of 0–2 months. There is a gradient in model skill, with lowest skill inshore and increasing with depth towards the edge of the continental shelf, mostly evident at lead times of 1 and 2 months.
Monthly ACCESS-S2 anomaly correlations for (a) MLD with observations (ORAS5) 1982–2018 and (b) bottom temperature with observations (GLORYS) 1993–2018 in the Australian region for all start months at lead times 0, 1 and 2 months. Black shading denotes shallow regions where MLD consistently reaches the seabed (a), and for BT200 shows where depth >200 m (b). Only statistically significant skill is shown, with white indicating regions of no statistical significance.
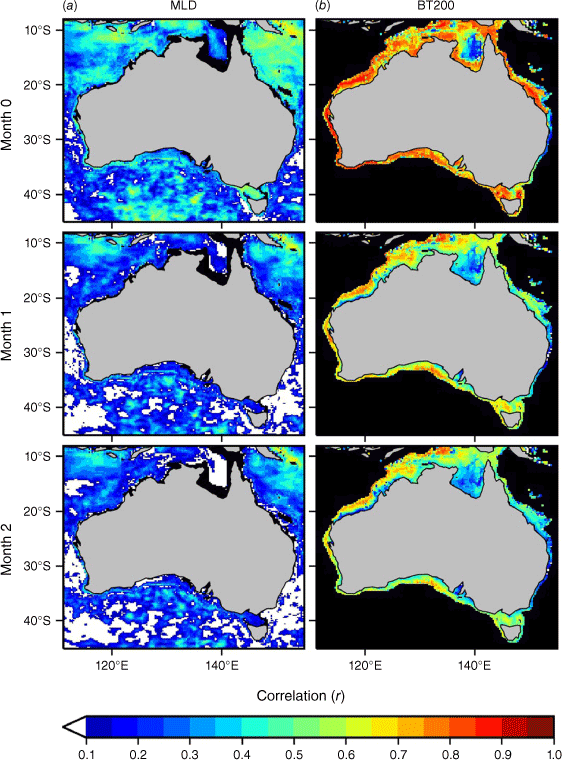
Fig. 7 shows the difference between model and observational s.d., normalised by observational s.d. (similar to the method shown in Fig. 3) at lead times 0, 1 and 2 months for MLD (Fig. 7a) and bottom temperature (Fig. 7b). The MLD s.d. ranges from comparable to observations in regions off the east and west coast and in the Southern Ocean, and considerably lower in regions around Australia including the Great Australian Bight, around Tasmania, the Gulf of Papua and near Indonesia. The MLD s.d. values are up to 70% lower than observations in parts of these regions at month 0, which increases in spatial footprint for months 1 and 2. Bottom temperature s.d. values between observations and model are similar at month 0, but also show regions where model s.d. is too low with increasing lead time for the Gulf of Carpentaria, the Great Barrier Reef, the North-west Shelf and near to Spencer Gulf and the Bonney Upwelling on the eastern side of the Great Australian Bight.
Monthly differences between ACCESS-S2 and observational standard deviation, normalised by observed standard deviation for (a) MLD with observations (ORAS5) 1982–2018 and (b) bottom temperature with observations (GLORYS) 1993–2018 in the Australian region for all start months at lead times 0, 1 and 2 months. Black shading denotes shallow regions where MLD consistently reaches the seabed (a), and for BT200 shows where depth >200 m (b).
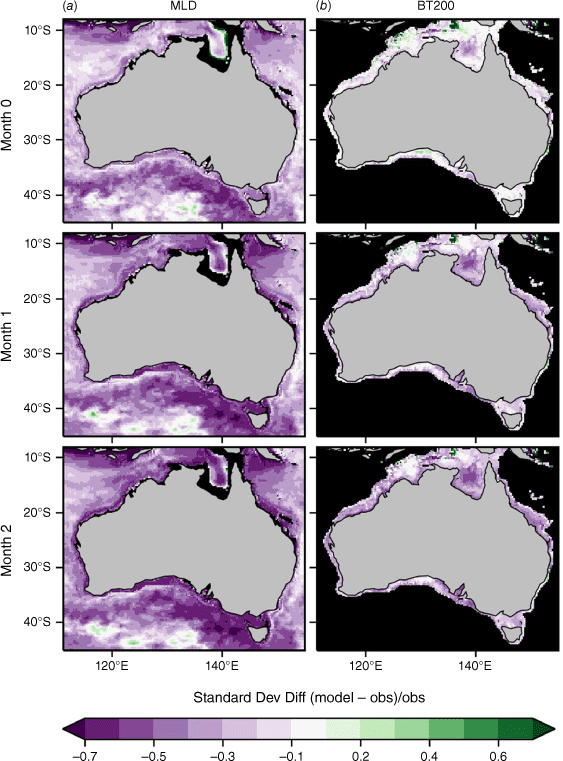
Fig. 8 shows ROC scores in exceeding the 90th percentile representing skill for fortnightly and calendar month marine heatwave predictability. Parameters shown include both surface and sub-surface, with SST, HC300 and BT200 at lead times of weeks 1 & 2, weeks 3 & 4 and 0, 2 and 4 months (fortnightly bottom temperature not available in model hindcast). Values are highest across northern Australia and on shelf regions for SST and HC300, with a fairly linear decline with lead time, with notable exceptions of HC300 off the North-west Shelf, parts of the Coral Sea and east of Tasmania at longer lead times. Lowest values for both SST and HC300 at lead times of 1 and 2 months is south of Australia, and off the coast of New South Wales. Bottom temperature ROC scores are highest at lead time of 0 months in Torres Strait, Bass Strait and many inshore regions around the Australian coastline. Only a marginal decline in ROC scores is evident at longer lead times, with most prominent declines in Torres and Bass Strait, and inshore of the Great Barrier Reef. The SST and HC300 forecasts have a reduced ability to match forecast probabilities with observed frequencies in the subtropics (Australian domain south of Tropic of Capricorn) compared to the tropics (Australian domain north of Tropic of Capricorn). This deficiency is represented by the flatter lines in panels (d) and (e) in reliability diagrams shown in Supplementary Fig. S1.
Monthly ROC scores for exceeding the 90th percentile for (a) SSTA, (b) HC300 and (c) bottom temperature (BT200) anomalies for all start dates in 1982–2018 (except for HC300 fortnightly and BT200, which are for the period 1993–2018) for lead times weeks 1 & 2, weeks 3 & 4 and 0, 2 and 4 months. Observations used were OiSSTv2.1 for SST, GREPv2 (fortnightly) and ORAS5 (monthly) for HC300, and GLORYS for BT200. Black shading denotes regions where depth >200 m for BT200 (c).
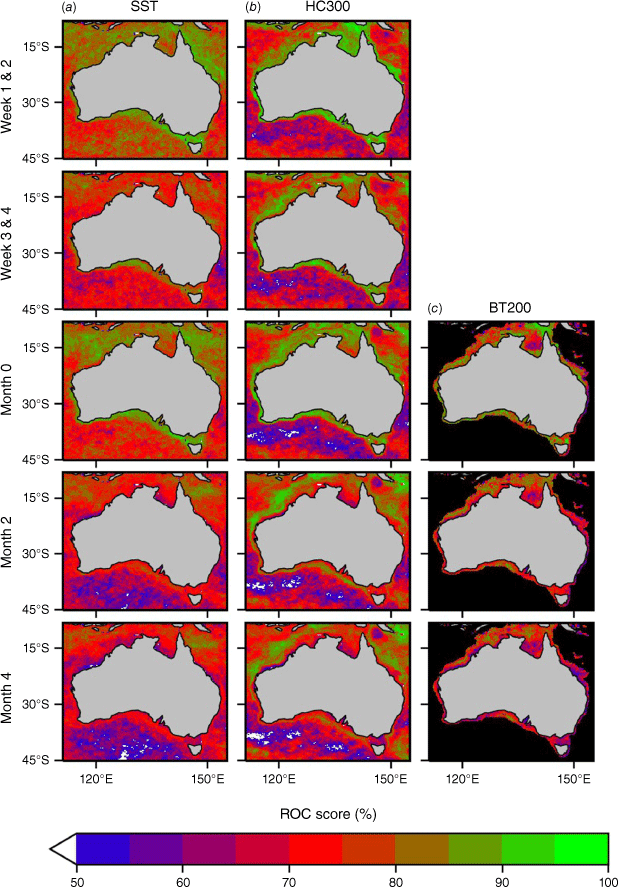
4.Discussion
The greatest improvement in SST skill in ACCESS-S2, compared to its predecessor ACCESS-S1, is on the subseasonal timescale (Fig. 1). This is due primarily to the new improved data assimilation scheme in ACCESS-S2, which reduces model shock (Wedd et al. 2022) and delivers more timely initial conditions than the ACCESS-S1 data assimilation configuration, the impacts of which are most evident at shorter lead times following model initialisation. This new data assimilation system also has benefits on monthly timescales with improved skill in the tropical Atlantic for ACCESS-S2, resolving a significant warm offset in the equatorial Atlantic Ocean in ACCESS-S1, which led to decreased skill in that region (Wedd et al. 2022). Elsewhere, however, SST skill is generally comparable between the two systems, with the exceptions of better SST skill in ACCESS-S1 for dynamic regions and in the far Southern Ocean at shorter leads (Fig. 1), likely due in part to a lack of sea ice assimilation in ACCESS-S2 (Wedd et al. 2022). Both models struggle in various higher latitude locations most likely due to model resolution not able to fully resolve mesoscale features in these regions (Hallberg 2013). Although ACCESS-S1 had altimetry assimilation in the initial conditions, Widlansky et al. (2023) showed altimetry assimilation had little effect on SST skill at shorter lead times, due to strong nudging of SST in the initial conditions to SST observations. However, several of the known deficiencies in ACCESS-S1 remain in ACCESS-S2, such as SST biases in the Indian Ocean and Maritime Continent regions and are the focus of collaboration between the Bureau of Meteorology, UKMO and other partners (Wedd et al. 2022).
For ACCESS-S2, SST anomaly skill is higher over a larger proportion of the global domain compared to HC300 skill at sub-seasonal timescales (Fig. 2). This reflects the influence of better model initialisation at the surface due to greater availability of surface observations over the hindcast period, compared to that for the subsurface. This was also noted in McAdam et al. (2022) in their analysis of heat content skill. However, when assessing ACCESS-S2 HC300 anomaly skill for the period 2000–2018, post the launch of the Argo observation program, which significantly increased the availability of subsurface observations, no significant increase in HC300 skill was seen even at short lead times (not shown). This suggests that subsurface observations are insufficient in both ACCESS-S and the reanalysis. Limitations in HC300 forecasts are possibly exacerbated by a lack of altimetry assimilation, which Widlansky et al. (2023) suggested improved HC300 skill in the subtropics out to 3 months. The advantage of better model initialisation of SST reduces with lead time however, and by lead time of 4 months, HC300 skill is higher than SST across the tropical Pacific. This is largely due to increased persistence skill for HC300 compared to SST at longer lead times (Fig. 2), due to the slower moving nature of the ocean at depth outside of the subtropics. At shorter lead times, model HC300 anomaly correlations need to exceed 0.9 to show benefits over persistence forecasts in the equatorial Pacific (Fig. 2b). The equatorial regions of the Atlantic and Pacific have strong upwelling, as Ekman transport from trade winds moves water away from the equator, indicating that ACCESS-S2 is skilful in predicting ocean temperature changes from upwelling in ocean basins. The variability of SST was shown to be too low outside the tropics at longer lead times (Fig. 3a), whereas HC300 was too high, especially in the Southern Ocean and South Atlantic (Fig. 3b).
On seasonal timescales, both SST and HC300 anomaly forecasts have highest skill in the austral summer months (DJF) compared to winter (JJA) in the western to south-west Pacific (Fig. 5) with forecasts for winter occurring during austral autumn with lowest skill for the ENSO monitoring index Niño34 (average SST anomaly for region in equatorial Pacific 170°W–120°W and 5°S–5°N) in ACCESS-S2 (although improved over ACCESS-S1) and other seasonal forecast models (Jin et al. 2008; Wedd et al. 2022). The HC300 anomaly skill exceeds that for SST for target season JJA with lead time; however, various horizontal patterns of alternating high and low skill are evident. High HC300 anomaly skill is seen along the equator in the western Pacific, contrasting with lower skill to the north of the equator along the location of the north subsurface countercurrent known as the Tsuchiya jet (Johnson and Moore 1997). Anomaly correlation coefficients are noticeably higher for both SST and HC300 across northern Australia (north of 15°S), compared to southern waters for both DJF and JJA (Fig. 5). ACCESS-S2 SST and HC300 anomaly skill around New Zealand is comparable to findings by de Burgh-Day et al. (2019) for ACCESS-S1, though improved SST skill is seen in the north and east in summer in ACCESS-S2.
The seasonal anomaly skill distribution for MLD is quite different to that of SST and HC300, with significant skill south of the equator to the east of Australia in summer (DJF), with a similar pattern in winter though over a larger spatial area (Fig. 5c). In summer typically the MLD is shallower than in winter months, with indication from correlation coefficients that shallower MLD is more challenging to forecast compared to deeper values. At monthly lead times, a north–south gradient is also evident in MLD skill with higher skill closer to the equator, compared to waters further south for all lead times (Fig. 6a). The Gulf of Carpentaria generally has low SST, HC300 and bottom temperature anomaly skill compared to adjacent regions as well. Predicted mixed layer depths were too shallow around New Zealand in ACCESS-S1 (de Burgh-Day et al. 2022), and in the Southern Ocean in the UKMO GC2 (Williams et al. 2015; Calvert et al. 2020), so low skill in these regions is not unexpected. ACCESS-S2 was also found to have low variability for both MLD and BT200 around Australia compared to observations for all lead times, which would also affect skill (Fig. 7). Mixed layer depth is highly variable on synoptic timescales (Treguier et al. 2023), which is a limiting factor in ACCESS-S2 predictions over subseasonal to seasonal timescales and contributes to lower mixed layer depth variability and skill.
ACCESS-S2, and its predecessor ACCESS-S1, have been shown to have significant skill in predicting SST around Australia at a range of lead times. The analysis presented here also indicates there is forecast skill for below the surface, specifically HC300, MLD and BT200 on the Australian continental shelf. Although skill is lower for these subsurface variables, there is still utility in using this forecast information to both inform marine user decision-making as well as further our understanding of ocean processes. The combination of these three variables, together with SST, can provide an efficient method for characterisation of the subsurface, without needing large volumes of ocean forecast data at all depth layers. For species that live at depth, SST is not always a good indicator of water temperatures that species are exposed to, particularly in deeper regions and where the mixed layer is shallow. For example, mussels are typically grown on ropes suspended in the water column, species such as scallops grow in the substrate and fish generally forage deeper than in the top layer (SST). Scales et al. (2023) found that subsurface information, particularly MLD, was a primary indicator of tuna distributions. The MLD can also provide insight into habitat and food distributions for marine species, as it is closely linked with nutrient supply to the euphotic zone and thus primary production. Bottom temperatures are important for benthic ecosystems (e.g. seagrass, kelp and coral), which provide food and habitat for many marine species and fisheries. Bottom temperature is also a significant environmental factor affecting zooplankton communities (e.g. Ershova et al. 2015), which in turn are an important part of the food chain between phytoplankton and larger invertebrates.
The model also demonstrated useful skill in predicting SST and HC300 anomaly values above the 90th percentile out to 4 months lead time, with skill higher for HC300 than SSTA (Fig. 8). This aligns with preliminary skill analysis by Spillman et al. (2021) and indicates the model has capacity to forecast marine heatwaves on fortnightly and monthly timescales up to 4 months lead time. Holbrook et al. (2020) indicated that the subsurface temperature is an important consideration in the recharge of marine heatwaves and events can occur without a surface expression. The ability to forecast extreme ocean temperatures at depth will extend our understanding of drivers of subsurface marine heatwaves, providing information to support operational decision making and agile marine management with context in terms of extreme temperatures. Forecast skill on subseasonal timescales is a step closer to the current marine heatwave definition of a five consecutive day event based on daily data (Hobday et al. 2018). Trial marine heatwave forecast products are in development to support operational decision making by Australian fisheries. These new products will complement the operational ocean outlook service for Australia (Smith and Spillman 2019; Spillman and Smith 2021), which provides climate intelligence to marine managers and businesses.
Skill analyses presented here underpin these operational ocean temperature outlooks and provide end users with confidence in forecast information. The Bureau of Meteorology will contribute ACCESS-S2 data to both the international Copernicus Climate Change Service (C3S; https://climate.copernicus.eu/) and the Subseasonal to Seasonal (S2S) Prediction Project (http://www.s2sprediction.net/), supported by the World Meteorological Organisation, in the future and a baseline skill analysis is essential for effective use of these data. New hindcasts are currently being run for January 2019 to September 2021 when ACCESS-S2 became operational to provide a continuous hindcast set up to the present day. A longer hindcast period means that further skill assessments can occur over a bigger set of climate events, offering greater confidence in reported skill. An updated hindcast period also offers flexibility in climatology period selection, with the possibility of aligning with the WMO recommended period of 1991–2020. As the ACCESS-S seasonal prediction system undergoes continuous improvement and upgrades, in collaboration with the UKMO, improvements in forecast skill are expected as model physics are better resolved and model initialisation is improved, providing more opportunities for marine applications requiring both accurate predictions of extremes and subsurface information.
5.Conclusions
These detailed skill descriptions for surface to subsurface ocean temperatures from a 38-year ACCESS-S2 hindcast on subseasonal to seasonal timescales support current and future operational outlooks for ocean temperature related applications. This includes the current Bureau of Meteorology operational ocean outlooks, the Global Seasonal Outlook produced for Department of Defence and ACCESS-S model uptake in the Pacific Island country meteorological services for Ocean Outlook Products as part of the Climate and Oceans Support Program in the Pacific (COSPPac; Powers et al. 2019). Model skill assessment in predicting extreme temperatures exceeding 90th percentile thresholds underpin plans to operationalise trial marine heatwave products currently in development (Spillman et al. 2021; Hartog et al. 2023). This also marks the first skill assessment for MLD and bottom temperature for ACCESS-S2.
Marine industries are shown to be more resilient to ocean extremes when seasonal outlooks lead to agile management response and decision-making (Hartog et al. 2023). The ability to forecast future ocean conditions is becoming increasingly important as past experience of extremes becomes less informative in a warming climate.
Data availability
The ACCESS-S2 hindcast dataset is available from the National Computational Infrastructure THREDDS server (https://dapds00.nci.org.au/thredds/catalogs/ux62/access-s2/access-s2.html). Information for access to data used for validation purposes can be sourced from their respective documentation: SST from Reynolds OISSTv2.1 (Reynolds et al. 2007; Banzon et al. 2016; Huang et al. 2021); monthly HC300 and MLD from the ECMWF Ocean Reanalysis System 5 (ORAS5; Zuo et al. 2019); and daily subsurface data from the Global Reanalysis Ensemble Product (GREP), which includes the four global ocean-ice reanalyses C-GLORSv7 (Storto et al. 2015), FOAM-GloSea5 (MacLachlan et al. 2015), GLORYS2v4 (Lellouche et al. 2013) and ORAS5.
Acknowledgements
We appreciate the assistance of Griffith Young, Morwenna Griffiths, Hailin Yan, Paul Smith and Robin Wedd (Bureau of Meteorology, Australia) for the preparation of the ACCESS-S2 hindcast set, and review of an earlier draft by Oscar Alves, Xiaobing Zhou and Ryan Holmes. We also appreciate discussions with CSIRO collaboration partners Alistair Hobday and Jason Hartog.
References
Alexander MA, Scott JD, Friedland KD, Mills KE, Nye JA, Pershing AJ, et al. (2018) Projected sea surface temperatures over the 21st century: changes in the mean, variability and extremes for large marine ecosystem regions of Northern Oceans. Elementa: Science of the Anthropocene 6, 9.
| Crossref | Google Scholar |
Amaya DJ, Jacox MG, Alexander MA, Scott JD, Deser C, Capotondi A, Phillips AS (2023) Bottom marine heatwaves along the continental shelves of North America. Nature Communications 14, 1038.
| Crossref | Google Scholar | PubMed |
Babcock RC, Bustamante RH, Fulton EA, Fulton DJ, Haywood MDE, Hobday AJ, Kenyon R, Matear RJ, Laganyi E, Richardson AJ, Vanderklift M (2019) Severe continental-scale impacts of climate change are happening now: extreme climate events impact marine habitat forming communities along 45% of Australia’s coast. Frontiers in Marine Science 6, 411.
| Crossref | Google Scholar |
Banzon V, Smith TM, Chin TM, Liu C, Hankins W (2016) A long-term record of blended satellite and in situ sea-surface temperature for climate monitoring, modeling and environmental studies. Earth System Science Data 8, 165-176.
| Crossref | Google Scholar |
Benthuysen JA, Smith GA, Spillman CM, Steinberg CR (2021) Subseasonal prediction of the 2020 Great Barrier Reef and Coral Sea marine heatwave. Environmental Research Letters 16(12), 124050.
| Crossref | Google Scholar |
Brodie S, Hobday AJ, Smith JA, Spillman CM, Hartog JR, Everett JD, Taylor MD, Gray CA, Suthers IM (2017) Seasonal forecasting of dolphinfish distribution in eastern Australia to aid recreational fishers and managers. Deep-Sea Research – II. Topical Studies in Oceanography 140, 222-229.
| Crossref | Google Scholar |
Calvert D, Nurser G, Bell MJ, Fox-Kemper B (2020) The impact of a parameterisation of submesoscale mixed layer eddies on mixed layer depths in the NEMO ocean model. Ocean Modelling 154, 101678.
| Crossref | Google Scholar |
Caputi N, Kangas M, Chandrapavan A, Hart A, Feng M, Marin M, de Lestang S (2019) Factors affecting the recovery of invertebrate stocks from the 2011 Western Australian extreme marine heatwave. Frontiers in Marine Science 6, 484.
| Crossref | Google Scholar |
de Burgh-Day CO, Spillman CM, Stevens C, Alves O, Rickard G (2019) Predicting seasonal ocean variability around New Zealand using a coupled ocean–atmosphere model. New Zealand Journal of Marine and Freshwater Research 53(2), 201-221.
| Crossref | Google Scholar |
de Burgh-Day CO, Spillman CM, Smith G, Stevens CL (2022) Forecasting extreme marine heat events in key aquaculture regions around New Zealand. Journal of Southern Hemisphere Earth Systems Science 72(1), 58-72.
| Crossref | Google Scholar |
Ershova EA, Hopcroft RR, Kosobokova KN (2015) Inter-annual variability of summer mesozooplankton communities of the western Chukchi Sea: 2004–2012. Polar Biology 38, 1461-1481.
| Crossref | Google Scholar |
Eveson JP, Hobday AJ, Hartog JR, Spillman CM, Rough KM (2015) Seasonal forecasting of tuna habitat in the Great Australian Bight. Fisheries Research 170, 39-49.
| Crossref | Google Scholar |
Garric G, Parent L, Greiner E, Drévillon M, Hamon M, Lellouche JM, et al. (2018) Performance and quality assessment of the global ocean eddy-permitting physical reanalysis GLORYS2V4 operational oceanography serving sustainable marine development. In ‘Proceedings 8th EuroGOOS International Conference’, 3–5 October 2017, Bergen, Norway. (Eds E Buch, V Fernandez, G Nolan, D Eparkhina) p. 516. (EuroGOOS: Brussels, Belgium)
Gwyther DE, Kerry C, Roughan M, Keating SR (2022) Observing system simulation experiments reveal that subsurface temperature observations improve estimates of circulation and heat content in a dynamic western boundary current. Geoscientific Model Development 15(17), 6541-6565.
| Crossref | Google Scholar |
Hallberg R (2013) Using a resolution function to regulate parameterizations of oceanic mesoscale eddy effects. Ocean Modelling 72, 92-103.
| Crossref | Google Scholar |
Hartog JR, Spillman CM, Smith G, Hobday AJ (2023) Forecasts of marine heatwaves for marine industries: reducing risk, building resilience and enhancing management responses. Deep-Sea Research – II. Topical Studies in Oceanography 209, 105276.
| Crossref | Google Scholar |
Hobday AJ, Hartog JR, Spillman CM, Alves O (2011) Seasonal forecasting of tuna habitat for dynamic spatial management. Canadian Journal of Fisheries and Aquatic Sciences 68(5), 898-911.
| Crossref | Google Scholar |
Hobday AJ, Oliver ECJ, Gupta AS, Benthuysen JA, Burrows MT, Donat MG, Holbrook NJ, Moore PJ, Thomsen MS, Wernberg T, Smale DA (2018) Categorizing and naming marine heatwaves. Oceanography 31(2), 162-173.
| Crossref | Google Scholar |
Holbrook NJ, Sen Gupta A, Oliver ECJ, et al. (2020) Keeping pace with marine heatwaves. Nature Reviews Earth & Environment 1, 482-493.
| Crossref | Google Scholar |
Huang B, Liu C, Banzon V, Freeman E, Graham G, Hankins B, Smith T, Zhang H-M (2021) Improvements of the Daily Optimum Interpolation Sea Surface Temperature (DOISST) version 2.1. Journal of Climate 34(8), 2923-2939.
| Crossref | Google Scholar |
Hudson D, Alves O, Hendon HH, Lim EP, Liu G, Luo J-J, MacLachlan C, Marshall AG, Shi L, Wang G, Wedd R, Young G, Zhao M, Zhou X (2017) ACCESS-S1: the new Bureau of Meteorology multi-week to seasonal prediction system. Journal of Southern Hemisphere Earth Systems Science 67(3), 132-159.
| Crossref | Google Scholar |
Hughes TP, Kerry JT, Connolly SR, Álvarez-Romero JG, Eakin CM, Heron SF, Gonzalez MA, Moneghetti J (2021) Emergent properties in the responses of tropical corals to recurrent climate extremes. Current Biology 31(23), 5393-5399.
| Crossref | Google Scholar | PubMed |
Masson-Delmotte V, Zhai P, Pirani A, Connors SL, Péan C, Berger S, Caud N, Chen Y, Goldfarb L, Gomis MI, Huang M, Leitzell K, Lonnoy E, Matthews JBR, Maycock TK, Waterfield T, Yelekçi O, Yu R, Zhou B (Eds) (2021) Summary for policymakers. In ‘Climate Change 2021: The Physical Science Basis. Contribution of Working Group I to the Sixth Assessment Report of the Intergovernmental Panel on Climate Change’. pp. 3–32. (Cambridge University Press: Cambridge, UK, and New York, NY, USA)
Jacox MG, Alexander MA, Amaya D, Becker E, Bograd SJ, Brodie S, Hazen EL, Pozo Buil M, Tommasi D (2022) Global seasonal forecasts of marine heatwaves. Nature 604, 486-490.
| Crossref | Google Scholar | PubMed |
Jin EK, Kinter JL, Wang B, et al. (2008) Current status of ENSO prediction skill in coupled ocean–atmosphere models. Climate Dynamics 31, 647-664.
| Crossref | Google Scholar |
Johnson GC, Moore DW (1997) The Pacific subsurface countercurrents and an inertial model. Journal of Physical Oceanography 27(11), 2448-2459.
| Crossref | Google Scholar |
Lellouche JM, Le Galloudec O, Drévillon M, Régnier C, Greiner E, Garric G, Ferry N, Desportes C, Testut CE, Bricaud C, et al. (2013) Evaluation of global monitoring and forecasting systems at Mercator Océan. Ocean Science 9, 57-81.
| Crossref | Google Scholar |
Liu G, Eakin CM, Chen M, Kumar A, De La Cour JL, Heron SF, Geiger EF, Skirving WJ, Tirak KV, Strong AE (2018) Predicting heat stress to inform reef management: NOAA Coral Reef Watch’s 4-month coral bleaching outlook. Frontiers in Marine Science 5, 57.
| Crossref | Google Scholar |
MacLachlan C, Arribas A, Peterson KA, et al. (2015) Global seasonal forecast system version 5 (GloSea5): a high-resolution seasonal forecast system. Quarterly Journal of the Royal Meteorological Society 141(698), 1072-1084.
| Crossref | Google Scholar |
Manzanas R (2020) Assessment of model drifts in seasonal forecasting: sensitivity to ensemble size and implications for bias correction. Journal of Advances in Modeling Earth Systems 12, e2019MS001751.
| Crossref | Google Scholar |
Marsh R, de Cuevas BA, Coward AC, Jacquin J, Hirschi JJM, Aksenov Y, et al. (2009) Recent changes in the North Atlantic circulation simulated with eddy-permitting and eddy-resolving ocean models. Ocean Modelling 28, 226-239.
| Crossref | Google Scholar |
Mason I (1982) A model for assessment of weather forecasts. Australian Meteorological Magazine 30, 291-303.
| Google Scholar |
McAdam R, Masina S, Balmaseda M, et al. (2022) Seasonal forecast skill of upper-ocean heat content in coupled high-resolution systems. Climate Dynamics 58, 3335-3350.
| Crossref | Google Scholar |
McAdam R, Masina S, Gualdi S (2023) Seasonal forecasting of subsurface marine heatwaves. Communications Earth & Environment 4, 225.
| Crossref | Google Scholar |
Megann A, Storkey D, Aksenov Y, Alderson S, Calvert D, Graham T, Hyder P, Siddorn J, Sinha B (2014) GO5.0: the joint NERC–Met Office NEMO global ocean model for use in coupled and forced applications. Geoscientific Model Development 7, 1069-1092.
| Crossref | Google Scholar |
Oke PR, Sakov P, Cahill ML, Dunn JR, Fiedler R, Griffin DA, Mansbridge JV, Ridgway KR, Schiller A (2013) Towards a dynamically balanced eddy-resolving ocean reanalysis: BRAN3. Ocean Modelling 67, 52-70.
| Crossref | Google Scholar |
Powers M, Begg Z, Smith G, Miles E (2019) Lessons from the Pacific Ocean portal: building pacific island capacity to interpret, apply, and communicate ocean information. Frontiers in Marine Science 6, 476.
| Crossref | Google Scholar |
Rae JGL, Hewitt HT, Keen AB, Ridley JK, West AE, Harris CM, Hunke EC, Walters DN (2015) Development of the Global Sea Ice 6.0 CICE configuration for the Met Office Global Coupled Model. Geoscientific Model Development 8, 2221-2230.
| Crossref | Google Scholar |
Reynolds RW, Smith TM, Liu C, Chelton DB, Casey KS, Schlax MG (2007) Daily high-resolution-blended analyses for sea surface temperature. Journal of Climate 20(22), 5473-5496.
| Crossref | Google Scholar |
Sakov P (2014) EnKF-C user guide. arXiv: Computer Science 2014(10), 1410.1233, v1.
| Crossref | Google Scholar |
Scales KL, Moore TS II, Sloyan B, Spillman CM, Eveson JP, Patterson TA, Williams AJ, Hobday AJ, Hartog JR (2023) Forecast-ready models to support fisheries’ adaptation to global variability and change. Fisheries Oceanography 32(4), 405-417.
| Crossref | Google Scholar |
Scannell HA, Johnson GC, Thompson L, Lyman JM, Riser SC (2020) Subsurface evolution and persistence of marine heatwaves in the northeast Pacific. Geophysical Research Letters 47(13), e2020GL090548.
| Crossref | Google Scholar |
Smale DA, Wernberg T, Oliver ECJ, Thomsen M, Harvey BP, Straub SC, Burrows MT, Alexander LV, Benthuysen JA, Donat MG, Feng M, Hobday AJ, Holbrook NJ, Perkins-Kirkpatrick SE, Scannell HA, Sen Gupta A, Payne BL, Moore PJ (2019) Marine heatwaves threaten global biodiversity and the provision of ecosystem services. Nature Climate Change 9, 306-312.
| Crossref | Google Scholar |
Smith G, Spillman C (2019) New high-resolution sea surface temperature forecasts for coral reef management on the Great Barrier Reef. Coral Reefs 38, 1039-1056.
| Crossref | Google Scholar |
Smith KE, Burrows MT, Hobday AJ, Sen Gupta A, Moore PJ, Thomsen M, Wernberg T, Smale DA (2021) Socioeconomic impacts of marine heatwaves: global issues and opportunities. Science 374(6566), eabj3593.
| Crossref | Google Scholar | PubMed |
Spillman CM (2011) Operational real-time seasonal forecasts for coral reef management. Journal of Operational Oceanography 4(1), 13-22.
| Crossref | Google Scholar |
Spillman CM, Hobday AJ (2014) Dynamical seasonal ocean forecasts to aid salmon farm management in a climate hotspot. Climate Risk Management 1, 25-38.
| Crossref | Google Scholar |
Spillman CM, Smith GA (2021) A new operational seasonal thermal stress prediction tool for coral reefs around Australia. Frontiers in Marine Science 8, 687833.
| Crossref | Google Scholar |
Spillman CM, Smith GA, Hobday AJ, Hartog JR (2021) Onset and decline rates of marine heatwaves: global trends, seasonal forecasts and marine management. Frontiers in Climate 3, 801217.
| Crossref | Google Scholar |
Storto A, Masina S, Navarra A (2015) Evaluation of the CMCC eddy-permitting global ocean physical reanalysis system (C-GLORS, 1982–2012) and its assimilation components. Quarterly Journal of the Royal Meteorological Society 142(695), 738-758.
| Crossref | Google Scholar |
Tommasi D, Stock CA, Hobday AJ, Methot R, Kaplan IC, Eveson JP, Holsman K, Miller TJ, Gaichas S, Gehlen M, et al. (2017) Managing living marine resources in a dynamic environment: the role of seasonal to decadal climate forecasts. Progress in Oceanography 152, 15-49.
| Crossref | Google Scholar |
Treguier AM, de Boyer Montégut C, Bozec A, Chassignet EP, Fox-Kemper B, Hogg A, Iovino D, Kiss AE, Le Sommer J, Li Y, Lin P, Lique C, Liu H, Serazin G, Sidorenko D, Wang Q, Xu X, Yeager S (2023) The mixed-layer depth in the Ocean Model Intercomparison Project (OMIP): impact of resolving mesoscale eddies. Geoscience Model Development 16, 3849-3872.
| Crossref | Google Scholar |
Vidard A, Anderson DLT, Balmaseda M (2007) Impact of ocean observation systems on ocean analysis and seasonal forecasts. Monthly Weather Review 135(2), 409-429.
| Crossref | Google Scholar |
Walters D, Boutle I, Brooks M, et al. (2017) The Met Office Unified Model Global Atmosphere 6.0/6.1 and JULES Global Land 6.0/6.1 configurations. Geoscientific Model Development 10, 1487-1520.
| Crossref | Google Scholar |
Wedd R, Alves O, de Burgh-Day C, Down C, Griffiths M, Hendon HH, Hudson D, Li S, Lim E-P, Marshall AG, Shi L, Smith P, Smith G, Spillman CM, Wang G, Wheeler MC, Yan H, Yin Y, Young G, Zhao M, Zhou X (2022) ACCESS-S2: the upgraded Bureau of Meteorology multi-week to seasonal prediction system. Journal of Southern Hemisphere Earth Systems Science 72(3), 218-242.
| Crossref | Google Scholar |
Widlansky MJ, Long X, Balmaseda MA, Spillman CM, Smith G, Zuo H, Yin Y, Alves O, Kumar A (2023) Quantifying the benefits of altimetry assimilation in seasonal forecasts of the upper ocean. Journal of Geophysical Research: Oceans 128(5),.
| Crossref | Google Scholar |
Williams KD, Harris CM, Bodas-Salcedo A, Camp J, Comer RE, Copsey D, Fereday D, et al. (2015) The Met Office Global Coupled model 2.0 (GC2) configuration. Geoscientific Model Development 88, 1509-1524.
| Crossref | Google Scholar |
Zuo H, Balmaseda MA, Tietsche S, Mogensen K, Mayer M (2019) The ECMWF operational ensemble reanalysis–analysis system for ocean and sea ice: a description of the system and assessment. Ocean Science 15(3), 779-808.
| Crossref | Google Scholar |