Bulk cloud microphysical properties as seen from numerical simulation and remote sensing products: case study of a hailstorm event over the La Plata Basin
Angel Liduvino Vara-Vela
A
B
C
D
E
F
Abstract
Hailstorms develop over the La Plata Basin, in south-eastern South America, more often during later winter and early austral spring, between September and October. These systems have significant socioeconomic impacts over the region. Thus, a better understanding of how atmospheric drivers modulate the formation of hailstorms is important to improve the forecast of such phenomena. In this study, we selected a hailstorm event observed over the eastern La Plata Basin during 14–15 July 2016 to evaluate the performance of the Brazilian developments on the Regional Atmospheric Modelling System (BRAMS) model. The ability of the model in simulating cloud microphysical properties was evaluated by comparing simulations driven by different global forcings against in situ and remote sensing observations. The model results showed good skill in capturing the basic characteristics of the thunderstorm, particularly in terms of the spatial distribution of hydrometeors. The simulated spatial distribution of hail covers locations where hail fall was reported. The BRAMS simulations suggest that, despite relatively low values of the convective available potential energy (CAPE) (700–1000 J kg−1), environments with strong 0–8-km bulk shear (60–70 kt, ~30.9–36.0 m s–1) can promote the formation of ice clouds and hail fall over the eastern La Plata Basin. To be more conclusive, however, further research is needed to understand how different combinations of CAPE and shear affect hail formation over the region.
Keywords: BRAMS model, cloud microphysics, hailstorms, La Plata Basin, numerical simulation, precipitation, remote sensing, SALLJ event.
1.Introduction
The La Plata Basin (LPB) is the fifth-largest river basin in the world and the second-largest in South America, just behind the Amazon basin. With an area of ~3.1 × 106 km2, it spreads across territories of Argentina, Brazil, Bolivia, Paraguay and Uruguay (blue outline in Fig. 1), and concentrates a population estimated at ~160 million inhabitants (United Nations 2016). Water and energy consumption in the LPB depend strongly on the freshwater stored in its rivers, with mesoscale convective systems (MCSs) being responsible for almost 90% of the total rainfall over the basin (Camponogara et al. 2018). The MCSs in the subtropical South American region – home to the LPB – are among the most intense thunderstorms in the world (Zipser et al. 2006), with peak occurrences between November and January (Velasco and Fritsch 1987; Durkee and Mote 2010). Long-lived MCSs over the LPB are typically triggered by a cyclonic-phase Rossby wave crossing over the Andes in conjunction with an associated cold front, which favours the intrusion of the South American Low-Level Jet (SALLJ) into the region (Mattingly and Mote 2016; Rasmussen and Houze 2016). The SALLJ has been described by numerous studies as one of the major drivers responsible for transporting heat, moisture and biomass-burning products across the Amazon to south-eastern South America (Marengo et al. 2002; Vera et al. 2006; Ulke et al. 2011; Camponogara et al. 2014; Zemp et al. 2014; Vara-Vela et al. 2021). Under local thermodynamic instability conditions, the intrusion of the SALLJ in association with the topography of the region creates a favourable environment for the development of high-impact weather systems (Salio et al. 2007; Lavin-Gullon et al. 2021). In addition, the frequency of hailstorm events over the LPB could also be influenced by the position and intensity of the SALLJ (Rasmussen and Houze 2011; Beal et al. 2020) as well as the Southern Hemisphere subtropical jet stream (Martins et al. 2017).
South-eastern South America showing the modelling domain projected on a polar stereographic plane. The blue line delineates the La Plata Basin, whereas the black cross and red circle mark the locations of radar and sounding stations respectively. The dashed ellipse indicates the region where hail fall was reported and simulated by two of the model configurations. Paraná (PR), Santa Catarina (CS), and Rio Grande do Sul (RS) states constitute the so-called southern region of Brazil.
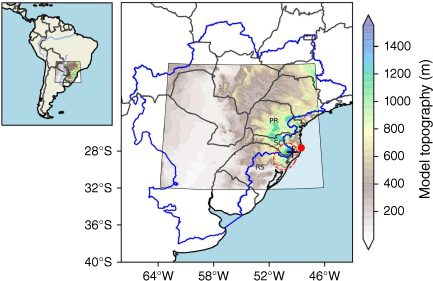
The LPB includes one of the largest hail-prone areas in the southern hemisphere, over the border region between north-eastern Argentina and southern Brazil (Cecil and Blankenship 2012). Destructive hailstorms are often observed over this mountainous region during late winter and early spring, between September and October (Martins et al. 2017; Beal et al. 2020). Hail fall events over this region are mostly reported during the late afternoon and evening hours (Martins et al. 2017). Monitoring the fall-out of hail is difficult because it is highly localised, with occurrence reports including a variety of sources which depend on population density and reporting practices (Prein and Holland 2018). Observational data based on hail pad networks can provide homogeneous time records but are commonly restricted to small areas. In recent years, the scientific community and citizen science networks, comprising volunteer farmers, have made tremendous efforts to monitor hailstorm activity over southern Brazil using hail pads (e.g. Nesbitt et al. 2021; Mantoani et al. 2023). In addition, hailstone sampling for chemical and biological characterisation has been carried out over this region (Beal et al. 2021, 2022; Mantoani et al. 2023). The chemical composition of hailstones reveals what types of aerosols can effectively act as cloud condensation nuclei (CCN) and ice nuclei (IN), thus contributing new knowledge with implications for ice formation processes within thunderstorm clouds.
Likewise, hail fall occurrences have long been one of the most challenging aspects of severe weather forecasting. Although it was widely thought in the forecasting community that storms with greater convective available potential energy (CAPE) produced larger hail, recent studies have shown that hail size does not increase monotonically with CAPE. Instead, it maximises within an intermediate range of optimal CAPE (Lin and Kumjian 2022). In addition, whereas intense convective updrafts are usually recognised as the main contributors to the growth of larger hail (Takahashi 1976; Foote 1984; Ilotoviz et al. 2016), variations in CCN concentrations can also promote hail growth (Ilotoviz et al. 2016; Marinescu et al. 2021). For example, CCN aerosol loadings in the environment can alter the temperature levels in-cloud of freezing and riming for water droplets while they are carried up to higher parts of the cloud during updrafts (Chen et al. 2017; Eirund et al. 2022). During this process, hailstone embryos are formed by the accretion of small droplets and ice crystals suspended in the cloud with increasing amounts of supercooled water droplets (Chen et al. 2019). Additionally, aerosol particles such as dust (Su and Fung 2018), soot (Mahrt et al. 2020) and primary biological aerosol particles (Jaenicke 2005; Sesartic et al. 2013; O’Sullivan et al. 2015) can act as IN and promote the formation of ice crystals. Although the current understanding of ice formation pathways in deep convective systems remains uncertain, hail growth and fall can be depicted in a very simple way, based on a simulated hailstone pseudotrajectory conducted by Dennis and Kumjian (2017), as follows: once hail embryos are formed aloft on one of the flanks of the updraft, in a region previous studies have called the ‘embryo curtain’ or ‘embryo corridor’ (e.g. Browning and Foote 1976; Ziegler et al. 1983), they fall cyclonically around the updraft and are reingested in it at the base on the front side. Thereafter, they ascend and grow rapidly up to reach their apex when they fall out of the updraft as hailstones. Finally, the colder the temperature outside the cloud, the greater the probability that they reach the ground.
Even though it is well documented that hail fall events over the LPB occur more often during late austral winter and early spring, no major efforts have been made to investigate such phenomena in detail. In fact, just a few studies using remote sensing products to evaluate the performance of atmospheric models in simulating severe thunderstorms have been conducted over the region (e.g. Camponogara et al. 2018). Thus, this study aims to evaluate the skill of the Brazilian developments on the Regional Atmospheric Modelling System (BRAMS) model in reproducing a severe thunderstorm event over the eastern LPB. To that end, remote sensing observations of precipitation and cloud optical properties available for the study region during the hailstorm event are compared with their corresponding values derived from model results. In the following section, the modelling framework will be introduced, including a description of the data sets used for the model evaluation. In Section 3, the large-scale atmospheric conditions that favoured the thunderstorm event are presented; Section 4 discusses specific thunderstorm features by comparing observations with model simulations. A summary and concluding remarks are given in Section 5.
2.Methods and observational data
2.1. Atmospheric model
The BRAMS model (Freitas et al. 2005, 2009) derives from the Regional Atmospheric Modelling System (RAMS, Pielke et al. 1992; Cotton et al. 2003), and includes new features to improve the representation of fundamental physical processes in tropical and subtropical regions (Silva et al. 2012). Recent BRAMS model versions include a set of state-of-the-art physical and chemical parameterisation schemes that extend RAMS original functionalities towards a fully integrated environmental model (Moreira et al. 2013; Freitas et al. 2017). BRAMS has been mainly applied for operational forecasts and research related to severe weather (e.g. Freire et al. 2022), urban heat islands (e.g. Souza et al. 2016), urban and remote air pollution (e.g. Bela et al. 2015), aerosol–radiation–cloud interactions, carbon and water cycles over the Amazon (e.g. Moreira et al. 2013), and volcanic ash dispersion (e.g. Pavani et al. 2016). The model source code and related preprocessors are open source and are available under the GNU General Public License at http://brams.cptec.inpe.br.
The BRAMS cloud microphysics schemes able to account for hail formation include those of RAMS Colorado State University (RAMS/CSU) (Walko et al. 1995; Meyers et al. 1997) and Thompson (Thompson and Eidhammer 2014), both two-moment bulk schemes. The cloud microphysics scheme used in this study (RAMS/CSU) predicts the mixing ratio and number concentration for seven hydrometeor categories: cloud droplets, rain, pristine ice, snow, aggregates, graupel and hail. The general gamma distribution is the basis function used for hydrometeor size in each hydrometeor category (Walko et al. 1995). Cloud droplets and rain are liquid water, but may become supercooled. Pristine ice, snow and aggregates are assumed to be pure ice, whereas graupel and hail are mixed-phase categories (ice only or a mixture of ice and liquid). Ice–rain interactions usually result in most of the collected mass and number concentrations being converted to the hail category; however, since hail is assumed to be a mixed phase hydrometeor, a look-up table for melting is applied to determine the number tendency of hail particles that completely melt into rain. This number concentration tendency is similar to the look-up table for melting of snow and aggregate hydrometeors. Although the hail category is permitted to contain liquid, excess liquid is shed to the rain category, with shedding from hail being based on the work of Rasmussen et al. (1984). Depending on atmospheric conditions, riming of graupel can also serve as a primary source of hail (Meyers et al. 1997). A detailed description of the main BRAMS physics schemes can be found in Freitas et al. (2017).
2.2. Experiment design
Three BRAMS simulations were performed for a thunderstorm event observed in the eastern LPB on 14–15 July 2016. The experimental setup consists of a single domain with 250 × 280 (latitude × longitude) horizontal grid points at a resolution of 6 km. The criteria for choosing a resolution of 6 km were to align, in terms of spatial resolution, the model outputs with satellite- and radar-based observations. For the vertical coordinate, 45 sigma-type levels were set up with grid spacing ranging from 20 to 1000 m, and the model top extending up to 23 km (~33 hPa). Initial and boundary conditions to drive the simulations were based on global data at 0.25° horizontal resolution from the National Centers for Environmental Prediction (NCEP) GFS and CFSv2 models (Saha et al. 2014), and the European Centre for Medium-Range Weather Forecasts (ECMWF) Reanalysis v5 (ERA5) model (Hersbach et al. 2020), each driving a BRAMS simulation. The GFS data are provided at 26 levels from 1000 to 10 hPa, whereas CFSv2 and ERA5 provide meteorological data at 37 levels from 1000 to 1 hPa. Meteorological fields, including temperature, relative humidity, geopotential height, zonal wind and meridional wind, are interpolated to the model grid from the original pressure-level data sets, with boundaries updated every 6 h.
The main physical parameterisations include the Rapid Radiative Transfer Model for General Circulation Model applications (RRTMG) scheme (Iacono et al. 2008) for shortwave and longwave radiation, the Mellor–Yamada scheme (Mellor and Yamada 1982) for turbulence and the RAMS/CSU scheme (Meyers et al. 1997) for cloud microphysics. Cloud droplets are assumed to nucleate from constant CCN vertical profiles fixed at 450 nuclei per kilogram of air – sensitivity tests, which involved changing the CCN concentration to evaluate its impact on ice cloud formation, were previously performed for this particular study (not shown). Overall, new ice particles are formed when CCN is enhanced, whereas hail shows a slight decrease in concentrations with increasing CCN. This result agrees well with that of Camponogara et al. (2018). However, although the BRAMS model developers recommend coupling convection schemes for horizontal resolutions usually coarser than 2 km, other studies suggest that convective schemes should be scale-dependent (Arakawa et al. 2011), with assumptions that may vary with horizontal resolution (Bryan et al. 2003; Grell and Freitas 2014). Sensitivity tests with the standard RAMS convection scheme (Tremback 1990) turned on and off were conducted for verification. However, the simulations with the convection scheme turned on yielded slightly worse outcomes; therefore, they are not presented here for the sake of simplicity. Model results presented in this study derived from the microphysics configuration that best reproduced the observed ice cloud conditions. Static geographical data (e.g. topography, land use and sea surface temperature) and masked surface fields (e.g. soil moisture) needed for the model initialisation can be found at http://brams.cptec.inpe.br/input-data/. Model simulations were integrated over time for a simulation period of 54 h, starting at 18:00 hours UTC on 13 July 2016. The main physical parameterisations and other simulation attributes are listed in Table 1.
Attribute | Model option/configuration | |
---|---|---|
Model version | 4.3 | |
Centre-point of the domain | 25.5°S, 55.0°W | |
Horizontal grid points and resolution | 250 × 280 (latitude × longitude), 6 km | |
Vertical coverage and model top | 45 sigma-type levels, 23 km | |
Time step | 15 s | |
Initial and boundary conditions | NCEP GFS, NCEP CFSv2 and ECMWF ERA5, all at 0.25° horizontal resolution | |
Simulation period | 18:00 hours UTC on 13 July 2016–00:00 hours UTC on 16 July 2016, the first 6 h were discarded as spin-up time | |
Longwave and shortwave radiation | Iacono et al. (2008) | |
Turbulence | Mellor and Yamada (1982) | |
Cloud microphysics | Walko et al. (1995) and Meyers et al. (1997) with constant CCN vertical profiles fixed at 450 nuclei per kilogram of air. Mean rain droplet diameter is diagnosed from the prognosed mixing ratio and number concentration | |
Convection initiation | No cumulus parameterisation |
2.3. Observational data
Observational data used for the model evaluation include surface accumulated precipitation from MERGE and Climate Hazards group Infrared Precipitation with Stations (CHIRPS) merging techniques, reflectivity fields from a Doppler weather radar (DWR) instrument, cloud optical properties derived from Moderate Resolution Imaging Spectroradiometer (MODIS) retrievals and vertical profiles from sounding data. MERGE combines 0.25° Tropical Rainfall Measuring Mission (TRMM) satellite precipitation estimates with in situ station data to create daily gridded rainfall time series over South America (Rozante et al. 2010). The TRMM values at locations that coincided with the observation locations were interpolated to the TRMM grid points using successive corrections of the Barnes scheme (Barnes 1973; Koch et al. 1983). CHIRPS is a 0.05° land-only precipitation database available at different time scales that incorporates satellite, model and in situ data sets (Funk et al. 2015). Both MERGE and CHIRPS provide 24-h accumulated precipitation data. Although MERGE covers the period from 12:00 to 12:00 hours UTC, CHIRPS does it from 00:00 to 00:00 hours UTC. Precipitation data from CHIRPS lack uncertainty information at the moment. Reflectivity data were collected by a DWR instrument operating in the 2.7–3.0-GHz frequency band with a coverage extending out to a range of 400 km. The DWR is installed on the top of a 1.8-km peak in southern Santa Catarina State (28.13°S, 49.47°W), the second-highest peak within the modelling domain. MODIS is an optical sensor onboard the NASA Terra and Aqua satellites that takes images for the entire Earth’s surface every 1 or 2 days, and acquires data in 36 spectral bands in a wavelength range of 0.4–14.4 μm. Statistical fields from Aqua MODIS L3 daily cloud products at 1.0° resolution (Platnick et al. 2019) are used to evaluate the model skill in reproducing the cloud water path for liquid and ice phases. Sounding data for the Florianópolis station, located off the coast in western Santa Catarina State (27.67°S, 48.55°W), are provided by the Department of Atmospheric Science at the University of Wyoming (https://weather.uwyo.edu/upperair/sounding.html). Fig. 1 shows the locations of sounding and radar stations.
2.4. Model evaluation
A number of statistical parameters can be used to evaluate the performance of atmospheric models, including the correlation coefficient (r), mean bias error (MBE) and root-mean-square error (RMSE); r is a measure of the strength and direction of the linear relationship between simulation and observation, MBE measures the mean difference between simulation and observation, and RMSE represents the square root of the mean squared error between simulation and observation. All three parameters are appropriate for evaluating model performance over multiple time and space scales and can be calculated as follows:
where, P and O represent the predictions and observations respectively; k represents the kth grid point; and N is the total number of grid points. Overbars signify means over site, domain or time.
To compare against the DWR reflectivity observations, the model’s equivalent radar reflectivity factor (Ze) was estimated following the approach of Gao and Stensrud (2012). Additionally, in order to understand the strengths and weaknesses of the model in terms of the representation of atmospheric water and ice content, the Vertically Integrated Liquid (VIL) and Vertically Integrated Ice (VII) were calculated for the model and radar reflectivity factors, following the formulations of Greene and Clark (1972) and Mosier et al. (2011) respectively. The Echo Top of 20 dBZ (ET20), indicating the highest level of a storm detected by radar, was calculated and evaluated against the corresponding value from the model. Therefore, it is possible to visualise if the vertical development of the storm observed with the DWR system is well represented by the model. The fractional skill score (FSS, Roberts and Lean 2008) was applied for evaluating reflectivity, VIL and VII for each model simulation and radar observation:
where PF(k) and PO(k) are the fractional coverages of reflectivity for the kth grid point that exceeds a given threshold, and N is the total number of grid points in the domain. Thresholds of 20 dBZ for reflectivity and 0.5 kg m−2 for VIL/VII were chosen, along with a radius of influence of 10 grid points. These thresholds were selected based on our goal to determine if the model can accurately reproduce any amount of water (reflectivity and VIL) or ice (VII) caused by convection. In terms of the radius of influence, conducting multiple tests showed that employing 10 grid points yielded results with less noise. Consequently, the overall outcome did not significantly differ when using fewer grid points, although the FSS was smaller in all cases. Furthermore, it is important to note that, due to characteristics of the radar system such as air refraction and Earth curvature, the radar’s beam moves away from the surface as it extends farther from the radar. As a result, valid data are not available at lower levels in areas distant from the radar. Therefore, as VIL and VII are calculated by integrating data across atmospheric levels, the error in these calculations increases in regions far from the radar, resulting in an underestimation of the true values.
3.Results
3.1. Case-study description
As mentioned in the introduction section, the border region between north-eastern Argentina and southern Brazil has been identified as one of the largest hail-prone areas in the Southern Hemisphere, with high hail fall frequencies during late winter and early spring. The hailstorm event selected for numerical experiments occurred in the eastern LPB on 14–15 July 2016, a month with a number of hail days significantly less than those usually reported in October, the peak hail month (Beal et al. 2020). Fig. 2 shows the evolution of the large-scale atmospheric conditions associated with the hailstorm event. Prior to the hailstorm event (12:00 hours UTC on 12 July 2016), there was strong moisture transport from the Amazon to the south-east of Brazil and the adjacent ocean due to a cyclogenesis taking place at 40°S and 55°W. Cyclogenesis over south-eastern South America is more often observed during austral wintertime (Crespo et al. 2020a, 2020b; de Jesus et al. 2021). This extratropical cyclone moved and developed over the South Atlantic and had an associated cold front that affected the weather over the continent. Circa 00:00 hours UTC on 14 July 2016, the environment over the study region still had a high moisture content (Fig. 2d), which was sustained by the cold front and a low-pressure system that were acting over the continent (20°S, 65°W). The low-pressure system deepened over the continent, giving rise to a new cyclone centred at 30°S and 50°W at 00:00 hours UTC on 15 July 2016 (Fig. 2c). From 00:00 hours UTC on 14 July 2016 onwards the jet stream accelerated while crossing over the Andes at the same time that two intense jet streaks took place near the region of interest: one over the continent (~25°S) and the other over the South Atlantic near the continent (~35°S) (Fig. 2h, i). In this way, the study region was under a jet stream bifurcation, i.e. an upper-level divergence zone corresponding to the polar exit and the equatorial entrance of these two different jet streaks. Over time, the ascent (air motion in the upward direction) intensified, reaching values below −1 Pa s−1 (Fig. 2h). The synoptic environment described here configures an SALLJ event, as previously introduced in Section 1.
(a–c) Mean sea level pressure (hPa, contour) and 500–1000-hPa thickness (decametres, dam, shaded), (d–f) horizontal wind (m s−1, vectors) and specific humidity (g kg−1, shaded) at 850 hPa, (g–i) horizontal wind (m s−1, vectors) and vertical velocity (Pa s−1, shaded) at 250 hPa at 00:00 hours (left) and 12:00 hours UTC on 14 July 2016 (centre) and at 00:00 hours UTC on 15 July 2016 (right) derived from ERA5 reanalysis. The red marker indicates the hailstorm location.
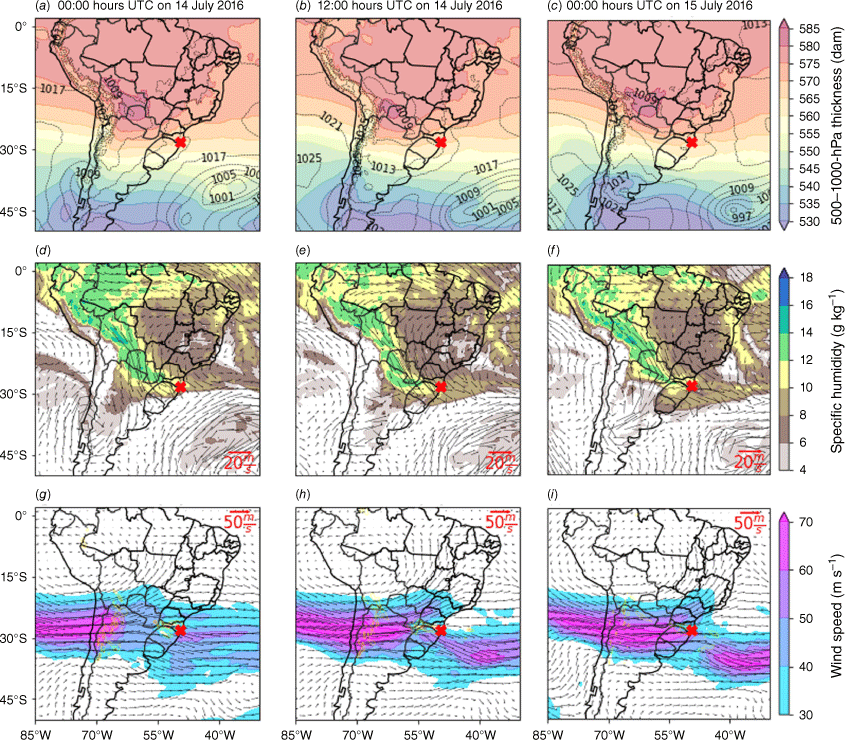
Hail fall events during the study period were reported in south-western Paraná State (e.g. Beal et al. 2020) and across various areas in southern Santa Catarina State between the end of 14 July and the beginning of 15 July 2016. South-eastern South America has a little more than half of its storms overnight, with a broad peak between 15:00 and 00:00 hours local solar time (Cecil and Blankenship 2012). Nocturnal convection over LPB is primarily triggered by orographic lifting (Romatschke and Houze 2010), highlighting the important role of the terrain in the development and timing of convection (Rasmussen and Houze 2016). According to the Civil Defence of Santa Catarina State, the hail fall event was registered in nine municipalities of the state: Lages, São Joaquim, Praia Grande, São Miguel do Oeste, Xanxerê, Peritiba, Ipira, Xaxim and Concórdia. Although the Civil Defence agency did not provide valuable information on the hailstorms, such as duration and hail size, it did report significant economic damage in São Miguel do Oeste and Praia Grande, where citizens were rendered homeless (source: https://estado.sc.gov.br/noticias/sc-registra-granizo-em-pelo-menos-nove-cidades/). Most of the affected regions are occupied by small farms and relatively poor people, for whom any hail-related damage causes several problems. The simulated spatial distribution of hail covers locations where hail fall was reported, namely São Joaquim and Praia Grande, both in southern Santa Catarina State (see Supplementary Fig. S1).
3.2. Surface accumulated precipitation
Fig. 3 shows the spatial distributions of the surface accumulated precipitation derived from MERGE, CHIRPS and BRAMS estimates. Fig. 3a, c, e and g correspond to MERGE, BRAMS/GFS, BRAMS/CFSv2 and BRAMS/ERA5, representing the 24-h surface accumulated precipitation during the period from 12:00 hours UTC on 14 July 2016 to 12:00 hours UTC on 15 July 2016. Similarly, Fig. 3b, d, f and h correspond to CHIRPS, BRAMS/GFS, BRAMS/CFSv2 and BRAMS/ERA5, representing the 48-h surface accumulated precipitation during the period from 00:00 hours UTC on 14 July 2016 to 00:00 hours UTC on 16 July 2016. CHIRPS provides accumulated precipitation data from 00:00 to 00:00 hours UTC, requiring a 48-h time period to encompass the entire hail fall event between the end of 14 July and the beginning of 15 July 2016. In contrast, MERGE covers the hail fall event with a 24-h period, from 12:00 hours UTC on 14 July 2016 to 12:00 hours UTC on 15 July 2016. In accordance with observations, rainfall is predicted in the south-eastern part of the modelling domain. However, slight to moderate differences in accumulated precipitation exist between observations and model simulations, with BRAMS/CFSv2 and BRAMS/GFS performing best against MERGE and CHIRPS, respectively. Table 2 summarises domain-wide performance statistics over land. The r between the BRAMS simulations and CHIRPS range within 0.4–0.5, whereas those between BRAMS and MERGE are 0.38–0.69. Notably, the mean bias and RMSE between BRAMS and MERGE are smaller compared to those between BRAMS and CHIRPS. Additional comparisons at the National Institute of Meteorology (INMET) site-specific locations are shown in Supplementary Fig. S2 and Supplementary Tables S1 and S2. Even though areas with maximum accumulated precipitation are well reproduced by the model in most cases, it is clear that BRAMS overestimates rainfall, with high RMSE values probably due to errors in both initial and boundary conditions as well as to model simplifications. For instance, it is initially assumed that cloud droplets nucleate from a prescribed CCN vertical profile, whereas in nature, there exists spatial variability in CCN concentrations due to prior activation in clouds (Zhao et al. 2017). The study conducted by Camponogara et al. (2018), also over the LPB, revealed that the number and intensity of downdrafts and, consequently, their total covered area increase as CCN concentration is enhanced.
Spatial distribution of surface accumulated precipitation (mm) during the periods from 12:00 hours UTC on 14 July 2016 to 12:00 hours UTC on 15 July 2016 (left panels) and from 00:00 hours UTC on 14 July 2016 to 00:00 hours UTC on 16 July 2016 (right panels). Precipitation data correspond to (a) MERGE and (b) CHIRPS merging techniques, and (c, d) BRAMS/GFS, (e, f) BRAMS/CFSv2, and (g, h) BRAMS/ERA5 simulations.
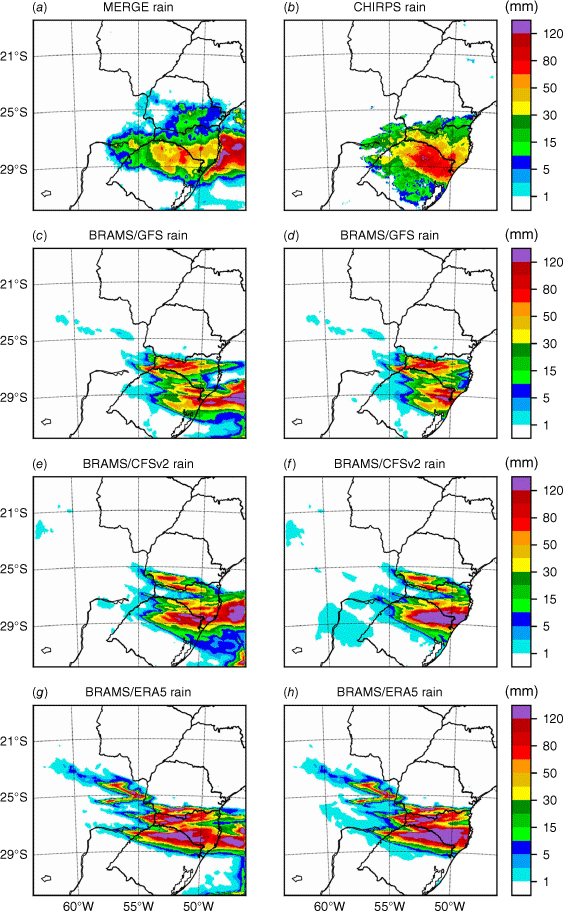
Index | BRAMS v. MERGE | BRAMS v. CHIRPS | |||||
---|---|---|---|---|---|---|---|
GFS | CFSv2 | ERA5 | GFS | CFSv2 | ERA5 | ||
Correlation | 0.38 | 0.69 | 0.52 | 0.41 | 0.56 | 0.51 | |
Mean bias (mm) | −0.85 | 0.13 | 2.93 | 0.45 | 3.70 | 5.17 | |
Root-mean-square error (mm) | 18.83 | 14.09 | 23.19 | 20.11 | 27.75 | 28.37 |
MERGE time integration of 24 h, from 12:00 hours UTC on 14 July 2016 to 12:00 hours UTC on 15 July 2016. CHIRPS time integration of 48 h, from 00:00 hours UTC on 14 July 2016 to 00:00 hours UTC on 16 July 2016. For the Correlation data, see Supplementary Fig. S3, which shows scatter plots of surface accumulated precipitation from the BRAMS/CFSv2 simulation compared to MERGE and CHIRPS merging techniques.
3.3. Vertical profiles and stability
The status of a thunderstorm development can be monitored using Skew-T Log-p diagrams produced from soundings and atmospheric models. Fig. 4 shows the vertical profiles of temperature, dew-point temperature and winds derived from the sounding launched at 00:00 hours UTC on 15 July 2016, from the Florianópolis station. Vertical profiles inferred from BRAMS simulations, at the sounding launching time and for the closest grid point to the sounding station, are also shown in Fig. 4. The simulated vertical profiles of temperature agree well with those derived from sounding data. However, all simulations have difficulties in capturing strong changes in dew-point temperature profiles. In terms of winds, the BRAMS/GFS simulation better captures the vertical profile of wind direction. As indicated in previous studies, systematic model errors in representing sharp inversions of temperature and wind shear are often related to the misrepresentation of land-use and stability (Mölders and Kramm 2010). However, the main contributing factor is that vertical profiles from atmospheric models, such as BRAMS, do not properly account for the impact of advection on thermodynamic parameters (Anand and Pal 2023). In addition, distances between model grid-points and stations elevations contribute to biases, as temperature and wind vary with height. The Florianópolis station is located in Santa Catarina Island, a small rugged island which makes the task of modelling the atmosphere over this location challenging for atmospheric scientists.
Skew-T diagrams showing the profiles of temperature (red lines, °C), dew-point temperature (green lines, °C) and wind (barbs, knots) derived from the (a) sounding (CAPE = 71.4 J kg−1; CIN = −132.6 J kg−1; 0–8-km bulk shear of 49.8 kt, ~25.6 m s–1) and the (b) BRAMS/GFS (CAPE = 439.1 J kg−1; CIN = −87.2 J kg−1; 0–8-km bulk shear of 54.4 kt, ~28.0 m s–1), (c) BRAMS/CFSv2 (CAPE = 279.5 J kg−1; CIN = −87.7 J kg−1; 0–8-km bulk shear of 58.3 kt, ~30.0 m s–1) and (d) BRAMS/ERA5 (CAPE = 160.0 J kg−1; CIN = −174.1 J kg−1; 0–8-km bulk shear of 56.1 kt, ~28.9 m s–1) simulations at 00:00 hours UTC on 15 July 2016. Vertical profiles inferred from BRAMS simulations are extracted at the sounding launching time and for the closest grid point to the Florianópolis station.
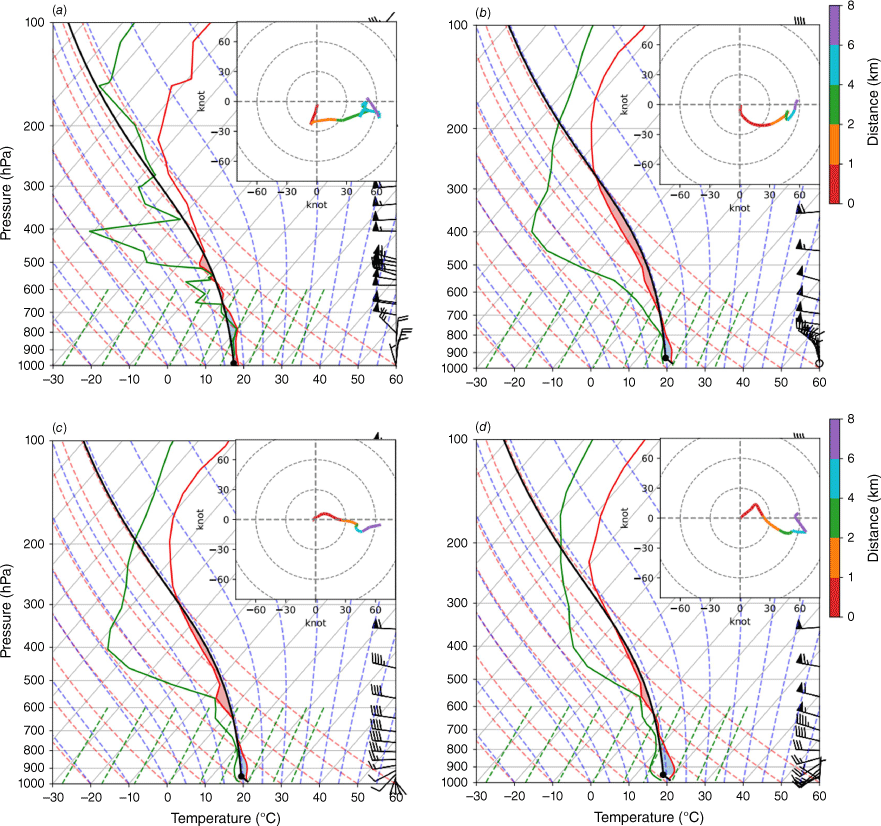
Because atmospheric instability indices are used primarily for diagnosing thermodynamic instability when they exceed certain critical values, there is the possibility of severe convection, and thus, the outbreak of a severe thunderstorm. The CAPE and the convective inhibition (CIN) are two important parameters commonly used for assessing the stability of the atmosphere in terms of moist convection. As illustrated in Fig. 4, both sounding data and model simulation depict the presence of CAPE (red areas in the diagrams) and CIN (blue areas in the diagrams), with the layer of CIN acting as a barrier to convection even when higher CAPE values are observed. Using the approach of Hobbs and Wallace (1977), the simulated CAPE and CIN are 439.1 and −87.2 J kg−1 for BRAMS/GFS respectively, and correspondingly 279.5 and −87.7 J kg−1 for BRAMS/CFSv2, and 160.0 and −174.1 J kg−1 for BRAMS/ERA5, against 71.4 and −132.6 J kg−1 obtained from sounding data. Although severe thunderstorms are usually associated with high values of CAPE (>2500 J kg−1), low values of CAPE (<800 J kg−1) can indicate that convection is possible. Based on the severe weather indices for CAPE proposed by Miller (1972) and Gordon and Albert (2000), the atmospheric condition around the sounding site falls into the weak thunderstorm group, and thus the atmospheric instability is considered low. As discussed later in Section 3.5, convection activity was dissipating over this region with clouds slowly vanishing in the process. All simulations perform similarly in terms of a 0–8-km bulk shear, i.e. the magnitude of the vector difference between the surface wind and the winds at 8 km, with respectively 54.4, 58.3 and 56.1 kt (~28.0, ~30.0 and 28.9 m s–1) for BRAMS/GFS, BRAMS/CFSv2 and BRAMS/ERA5 simulations against 49.8 kt (~25. m s–1) obtained from sounding data.
It is important to mention that, despite point-based vertical profiles from atmospheric models being often used to evaluate the development of storms, they do not usually reflect the track of radiosondes, which are continuously influenced by advection. As a result, even minor spatial or temporal deviations in the model’s representation of hailstorms can lead to the penalisation of its performance. To mitigate this issue, the adoption of a more flexible evaluation approach that takes into consideration both spatial and temporal aspects would be beneficial. For instance, establishing thresholds to delineate an acceptable range of deviations can be particularly crucial for variables like CAPE, where even minor spatial shifts may still indicate valid model performance. Other than that, point-based vertical profiles from atmospheric models can be useful in the absence of radiosondes. According to modelling results, vertical profiles from BRAMS/CFSv2 and BRAMS/ERA5 over locations where hail fall was reported and simulated by these simulations show relatively low values of CAPE of 700–1000 J kg−1, combined with a strong 0–8-km bulk shear of 60–70 kt (~30.9–36.0 m s–1; Supplementary Fig. S1 and S4). At the same time, vertical profiles from BRAMS/GFS – simulation that did not produce any surface hail concentrations – show values of CAPE even lower with 50–400 J kg−1, although a 0–8-km bulk shear of roughly the same order of 70–75 kt (36.0–38.6 m s–1; Supplementary Fig. S5). In terms of spatial distribution, regions in the north-western parts of Paraná and western parts of Santa Catarina states reach CAPE values exceeding 1600 J kg−1; however, no surface hail is produced in these areas (Supplementary Fig. S6).
3.4. Cloud optical properties
In order to analyse simulated cloud optical properties, unavoidable constraints of satellite observations such as microphysical assumptions (Yang et al. 2013), spatial and temporal resolution (Eliasson et al. 2013), and other limitations determined by instrument type (Rybka et al. 2021), must be carefully evaluated. Unfortunately, there were no high-resolution satellite data available for the study period, and model–satellite comparison is performed using MODIS cloud water path (CWP, g m−2) and cloud water effective radius (CWER, μm) data at 1.0° resolution. The CWP and CWER together help in understanding the mechanisms behind the formation of raindrops or ice particles, such as collision and coalescence (Freud and Rosenfeld 2012). Additionally, they are often used to characterise the scattering and absorption properties of clouds (Slingo 1989). The MODIS cloud optical properties data derive from multispectral reflectances for pixels identified as probably cloudy or cloudy by the cloud mask during the daytime portions of each orbit. The basic physical principle behind the calculation of MODIS cloud optical properties is the bi-spectral solar reflectance method described in Nakajima and King (1990).
Fig. 5 shows the spatial distributions of CWP for liquid and ice phases derived from MODIS products and BRAMS simulations. Owing to the limited availability of MODIS data over the thunderstorm development region, simulated CWP fields for the ice phase are calculated for 14 July 2016, whereas simulated CWP fields for the liquid phase are calculated for 15 July 2016, both based on the MODIS crossing time over the modelling domain c. 16:30 hours UTC. In general, the model simulations reproduce the observed north-west to south-east CWP fields for the liquid phase across the centre of the domain, except for some regions where simulations show significant biases. These regions include the south-central area of the modelling domain, located on the border between Brazil and Uruguay, as well as the northern regions of Paraná State (Fig. 5a, c, e and g). Regarding CWP fields for the ice phase, both observed and simulated fields show intense convection over the south-east part of the modelling domain, extending east beyond LPB up to the South Atlantic (Fig. 5b, d, f and h). In addition, CWP fields derived from BRAMS/ERA5 simulation spread over a bigger area and are slightly displaced to the north compared to the fields derived from BRAMS/CFSv2 and BRAMS/GFS simulations. Still, for the ice phase, simulated maximum CWP fields coincide with the locations of hail fall according to model results. Only simulations BRAMS/CFSv2 and BRAMS/ERA5 reproduce ground-level hail concentrations. Simulated vertical profiles from these two simulations between 16:00 and 17:00 hours UTC on 14 July 2016 over the maximum CWP areas in Fig. 5f and h (not shown) indicate that the updrafts reach the equilibrium level at ~12 km (anvil cloud), with values of up to 810.0 J kg−1 for CAPE and 61.4 kt (~31.6 m s–1) for 0–8-km bulk shear. Despite this low value of CAPE, hail fall areas derived from BRAMS/CFSv2 and BRAMS/ERA5 simulations agree well with some of the reported hail fall areas such as São Joaquim and Praia Grande in southern Santa Catarina State respectively (see Supplementary Fig. S1). Previous studies suggest that CAPE and hail size do not present a clear correlation (e.g. Johnson and Sugden 2014). Additionally, there are no conceptual models able to explain why some storms produce large hailstones whereas others produce large amounts of small hailstones (Kumjian et al. 2019). Including ice microphysics in atmospheric models in a realistic way represents a major challenge because of the wide variety of ice particle sizes, shapes and types in the atmosphere (Morrison et al. 2020).
Spatial distribution of cloud water path for liquid (left panels) and ice (right panels) phases (g m−2) as retrieved from (a, b) Aqua MODIS instrument, and as simulated with (c, d) BRAMS/GFS, (e, f) BRAMS/CFSv2, and (g, h) BRAMS/ERA5 on 14 July 2016 for ice phase and on 15 July 2016 for liquid phase. Model–satellite comparison based on the MODIS crossing time over the modelling domain (c. 16:30 hours UTC).
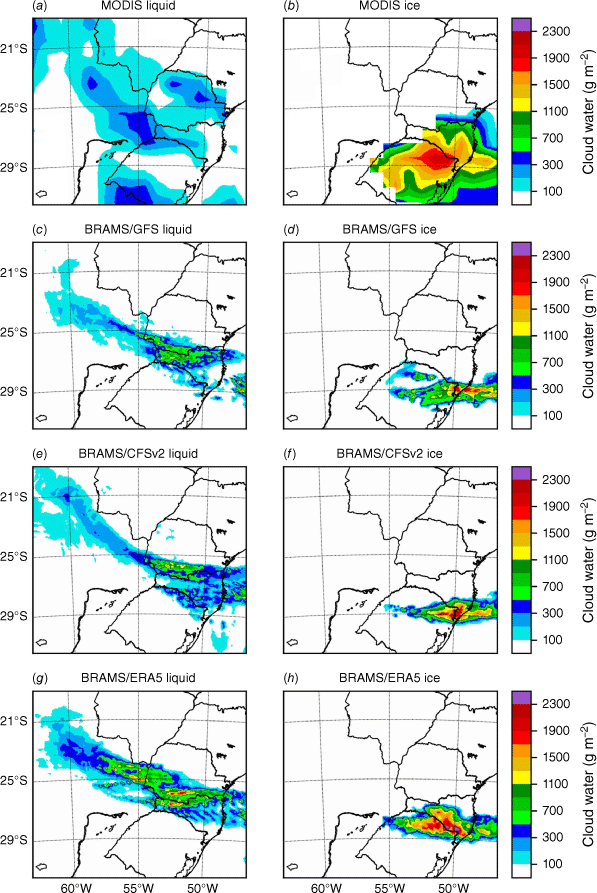
The BRAMS CWER fields are calculated following the generalised power-law expression of Liu et al. (2008):
where LWC represents the cloud liquid water content (g m−3), determined as the cloud water path divided by the cloud depth; ρw is water density (g cm−3); N is the cloud droplet number concentration (cm−3); and β is a dimensionless parameter that depends on the spectral shape of the cloud droplet distribution, set based on observation as follows:
where aβ = 0.07 and bβ = 0.14 (Liu et al. 2008; Freitas et al. 2017). Fig. 6 shows the spatial distributions of CWER derived from MODIS products and BRAMS simulations. As for MODIS, BRAMS simulations are able to reproduce qualitatively the salient features of the observed CWER pattern. Both MODIS and BRAMS show a CWER range of 6–12 μm over most cloudy regions, with the exception of some regions in the south-east part of the modelling domain where large amounts of surface accumulated precipitation are observed (Fig. 3). Over these regions (yellowish areas in Fig. 6), effective radii of up to 16 μm were both observed and simulated. Although no observations of CCN activity were available for the study period, the fact that an aerosol-sensitive quantity is predicted adequately, namely effective cloud-droplet size, is consistent with the prescribed CCN concentration set up in the model configurations. Model bias in simulation of cloud optical properties depends mainly on the aerosol loading (number concentration and chemical composition) and microphysical assumptions (Twomey 1977; Albrecht 1989). Although not shown here, our sensitivity test shows that an increase in the CCN concentration can lead to an increase in the concentration of ice hydrometeors, in accordance with previous BRAMS studies (Martins et al. 2009; Camponogara et al. 2018). Owing to the coarse resolution of the MODIS data, performance statistics were not performed in this case.
Spatial distribution of cloud water effective radius (μm) as retrieved from (a) Aqua MODIS instrument, and as simulated with (b) BRAMS/GFS, (c) BRAMS/CFSv2, and (d) BRAMS/ERA5 simulations on 15 July 2016. Model–satellite comparison based on the MODIS crossing time over the modelling domain (c. 16:30 hours UTC).
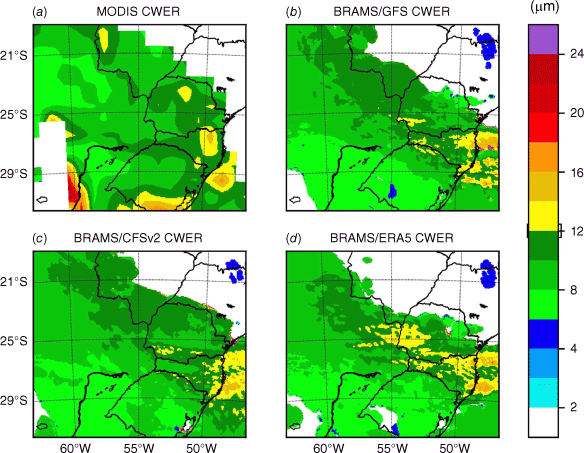
3.5. DWR-derived measurements
Doppler weather radars are remote sensing instruments widely used for monitoring intense precipitation associated with severe weather systems such as thunderstorms and hurricanes. Radar reflectivity (Z, mm6 m−3) is often used as the basis to quantify precipitation rate (R, mm h−1), with empirical Z–R relationships of the form Z = aRb being determined for any particular DWR instrument. In addition, parameters a and b are strongly dependent on the drop size distribution, and therefore on the physical processes that affect it, such as coalescence, breakup, or evaporation (Verrier et al. 2013). In this work, the predicted mixing ratios are converted to reflectivities using the approaches of Smith et al. (1975) for rainwater, and of Lin et al. (1983) and Gilmore et al. (2004) for ice, as follows:
where Z(qr) and Z(qi) represent the reflectivities for rainwater and ice respectively; ρ is the atmospheric density (kg m−3); and qr and qi are the mixing ratios of rainwater and ice (g kg−1) respectively. The model’s equivalent radar reflectivity factor (Ze) is then obtained by summing the contributions from rainwater and ice, following the approach of Gao and Stensrud (2012):
The Ze, VIL, VII and ET20 derived from the radar and model simulations at 00:00 hours UTC on 15 July 2016 are shown in Fig. 7. Convective cells over continental and maritime regions evolve differently from each other. Convection over the continent is influenced by the combined action of the SALLJ and regional topography (Fig. 1). The low-level convergence of water vapour flux and the consequent upward movement favour extreme convection and the development of a horizontally extensive (eastward-propagating), mature mesoscale convective system, lasting well into the night. Over time, convective cells over the South Atlantic region just off the coast of Brazil became weak and broke down into discrete cloud patches. This rainfall pattern is fairly well reproduced by the BRAMS simulations (not shown), but there are some differences worth mentioning. First, the storm evolution from the BRAMS/GFS and BRAMS/CFSv2 simulations is delayed compared to radar reflectivity, whereas the simulation initiated with ERA5 places the storm much closer to the observation (Fig. 7a). However, in the south-east of Santa Catarina State, where the storm was more intense according to the observation, the BRAMS/ERA5 simulation shows a much weaker reflectivity field, despite being the only simulation with reflectivity greater than 25 dBZ over the coast. Overall, ET20 values from all simulations are smoother and more than 2 km lower compared to that from radar, which shows several peaks greater than 13 km in height (Fig. 7b). This result shows that the model is not able to represent the vertical development of the system as high as that observed by the radar. In addition, the model shows an underestimation of rainwater production over the continent, with peaks ~12 kg m−2 compared to over 17 kg m−2 from observations (see Fig. 7c). By contrast, despite being placed at different locations, the ice peaks from model and radar are both comparable, of the order of 35 kg m−2. This result shows that the simulations do not accurately represent the vertical extent of the storm, although they can produce hail, as observed by the radar and reported by the media. Supplementary Fig. S7–S14 show the spatial distributions of Ze, ET20, VIL and VII derived from the DWR instrument and model simulations at 20:00, 21:00, 22:00 and 23:00 hours UTC on 14 July, and 01:00, 02:00, 03:00 and 04:00 hours UTC on 15 July 2016.
Spatial distribution of (a) reflectivity (dBZ), (b) Echo Top of 20 dBZ (km), (c) Vertically Integrated Liquid (VIL, kg m−2), and (d) Vertically Integrated Ice (VII, kg m−2) derived from the DWR instrument (first column) and from BRAMS/GFS, BRAMS/CFSv2 and BRAMS/ERA5 simulations (second, third and fourth columns respectively), at 00:00 hours UTC on 15 July 2016.
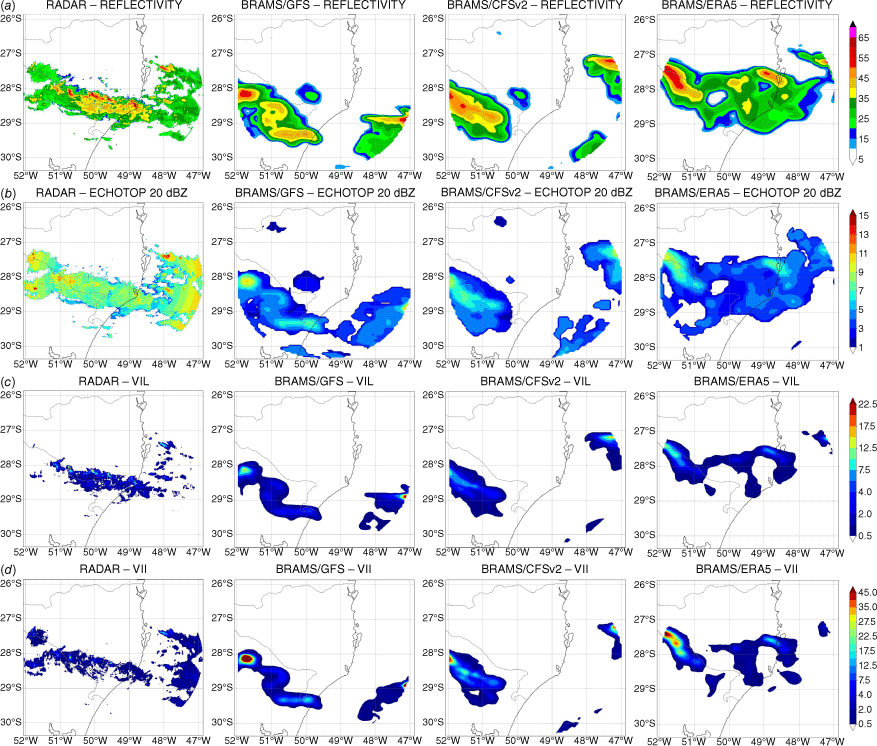
To better characterise the evolution of the quality of the simulations, the statistical measures FSS and RMSE were calculated for Ze, VIL and VII for the period from 20:00 hours UTC on 14 July to 04:00 hours UTC on 15 July 2016 (Fig. 8). In the early stage of the storm, the BRAMS/CFSv2 simulation performs better, as indicated by higher FSS values for Ze, VIL and VII (Fig. 8a, c, d respectively). Later, at 23:00 hours UTC on 14 July, the BRAMS/ERA5 simulation better locates the storm and produces more accurate predictions of rain and hail (Fig. 8c, d respectively). The BRAMS/GFS simulation has the worst performance among all simulations. The same behaviour observed in the FSS for Ze is also found in the RMSE, with a greater error for the BRAMS/GFS simulation (Fig. 8b). The RMSE values for VIL and VII (Supplementary Fig. S15 and S16) are similar for the three simulations. Possible sources of model–radar discrepancies include errors in initial and boundary conditions, mismatches between model and radar grid points, and the inherent limitations and assumptions of the radar, as discussed in Section 2.4.
4.Conclusion
Three simulations of a thunderstorm event over the LPB were performed with the Brazilian developments on the Regional Atmospheric Modelling System (BRAMS) model, each driven by a different global forcing: Global Forecast System (GFS), Climate Forecast System version 2 (CFSv2) and ECMWF Reanalysis v5 (ERA5). The selected thunderstorm event can be classified as a typical mesoscale convective system that develops in the region during austral wintertime, between June and August. An illustration of the large-scale atmospheric conditions that promoted such an event is discussed in detail in Section 3. The ability of the model in simulating cloud microphysical properties was evaluated by comparing the model output with satellite- and radar-based observations. Although comparison of the observed and simulated cloud fields revealed structural differences, the model showed a good ability to qualitatively capture the basic characteristics of the thunderstorm in terms of the spatial distribution of hydrometeors at surface and column-integrated.
Simulated vertical profiles of temperature, dew-point temperature and wind at the sounding station showed mixed results. The BRAMS/GFS simulation better represented wind, whereas temperature and dew-point temperature were better captured by the BRAMS/CFSv2 simulation. All simulations were able to represent the presence of CAPE and CIN, with positive biases for CAPE being more noticeable. Additionally, location of the maximum concentration of hydrometeors was fairly well represented by the simulations; however, they showed rather different performance in terms of intensity, with an overestimation or underestimation depending on the cloud property being analysed. For example, the surface accumulated precipitation and cloud water path for the liquid phase were overestimated, whereas the cloud water path for the ice phase was in accordance with the observed concentrations. Both observed and simulated cloud water effective radius showed similar values, meaning that BRAMS was able to capture the central tendency of this parameter. For model–radar comparison, the BRAMS/ERA5 simulation better captured the general pattern of observed rainfall, with a longitudinally elongated distribution that covered a great part of Santa Catarina State. In addition, the simulated spatial distributions of hail from the BRAMS/CFSv2 and BRAMS/ERA5 simulations covered locations where hail fall was reported (see Supplementary Fig. S1). For this case study, the BRAMS/GFS simulation showed the worst model performance, although it outperformed its counterparts in terms of surface precipitation. The BRAMS/ERA5 simulation showed the best model performance in terms of radar-derived measurements, but performed worse than the BRAMS/CFSv2 simulation in terms of surface precipitation. Possible sources of model–radar discrepancies include errors in initial and boundary conditions, mismatches between model and radar grid points, as well as the inherent limitations and assumptions of the radar.
The BRAMS simulations suggest that, despite relatively low values of CAPE (700–1000 J kg−1), environments with strong 0–8-km bulk shear (60–70 kt, ~30.9–36.0 m s−1) can promote the formation of ice clouds and hail fall over the eastern LPB. To be more conclusive, however, efforts should be made to investigate how different combinations of CAPE and shear affect hail formation over this region. The results in this study contribute to a new model evaluation of severe thunderstorms over the LPB. They also demonstrate the feasibility of comparing BRAMS simulations with remote sensing products to study the formation of severe thunderstorms over a region that is home to one of the largest hail-prone areas in the southern hemisphere.
Data availability
The BRAMS model code is freely distributed by the Centro de Previsão de Tempo e Estudos Climáticos (CPTEC) at http://brams.cptec.inpe.br/downloads/. Configuration files and preprocessing tools to replicate the modelling results in this work are available at doi.org/10.17632/cw8wrrt9jw.1.
Declaration of funding
This work was supported by a research grant (2016/06160-8) from São Paulo Research Foundation through the project ‘Sampling and modelling PBAPs at South-Southern Brazil: associated to improvements of climate models’. V. T. J. Phillips was supported by three research grants, from the Swedish Research Council for Sustainable Development (‘FORMAS’ Award 2018-01795), the Swedish Research Council (‘VR’ Award 2015-05104), and the US Department of Energy Atmospheric Sciences Research Program (grant number DE-SC0018932). N. M. Crespo acknowledges PETROBRAS (2017/00671-3) for the financial support.
Acknowledgements
We acknowledge CPTEC for providing the BRAMS model and the Rede de Meteorologia do Comando da Aeronáutica for providing the radar images.
References
Albrecht BA (1989) Aerosols, cloud microphysics and fractional cloudiness. Science 245, 1227-1230.
| Crossref | Google Scholar | PubMed |
Anand M, Pal S (2023) Exploring atmospheric boundary layer depth variability in frontal environments over an arid region. Boundary-Layer Meteorology 186, 251-285.
| Crossref | Google Scholar |
Arakawa A, Jung JH, Wu CM (2011) Toward unification of the multiscale modeling of the atmosphere. Atmospheric Chemistry and Physics 11, 3731-3742.
| Crossref | Google Scholar |
Beal A, Hallak R, Martins LD, Martins JA, Biz G, Rudke AP, Tarley CRT (2020) Climatology of hail in the triple border Paraná, Santa Catarina (Brazil) and Argentina. Atmospheric Research 234, 104747.
| Crossref | Google Scholar |
Beal A, Martins LD, Martins JA, Rudke AP, de Almeida DS, Costa LM, Tarley CRT (2021) Evaluation of the chemical composition of hailstones from triple border Paraná, Santa Catarina (Brazil) and Argentina. Atmospheric Pollution Research 12(3), 184-192.
| Crossref | Google Scholar |
Beal A, Martins JA, Rudke AP, de Almeida DS, da Silva I, Sobrinho OM, de Fátima Andrade M, Tarley CRT, Martins LD (2022) Chemical characterization of PM2.5 from region highly impacted by hailstorms in South America. Environmental Science and Pollution Research International 29, 5840-5851.
| Crossref | Google Scholar | PubMed |
Bela MM, Longo KM, Freitas SR, Moreira DS, Beck V, Wofsy SC, Gerbig C, Wiedemann K, Andreae MO, Artaxo P (2015) Ozone production and transport over the Amazon Basin during the dry-to-wet and wet-to-dry transition seasons. Atmospheric Chemistry and Physics 15, 757-782.
| Crossref | Google Scholar |
Browning KA, Foote GB (1976) Airflow and hail growth in supercell storms and some implications for hail suppression. Quarterly Journal of the Royal Meteorological Society 102, 499-533.
| Crossref | Google Scholar |
Bryan GH, Wyngaard JC, Fritsch JM (2003) Resolution requirements for the simulation of deep moist convection. Monthly Weather Review 131(10), 2394-2416.
| Crossref | Google Scholar |
Camponogara G, Silva Dias MAF, Carrió GG (2014) Relationship between Amazon biomass burning aerosols and rainfall over the La Plata Basin. Atmospheric Chemistry and Physics 14, 4397-4407.
| Crossref | Google Scholar |
Camponogara G, Faus da Silva Dias MA, Carrió GG (2018) Biomass burning CCN enhance the dynamics of a mesoscale convective system over the La Plata Basin: a numerical approach. Atmospheric Chemistry and Physics 18, 2081-2096.
| Crossref | Google Scholar |
Cecil DJ, Blankenship CB (2012) Toward a global climatology of severe hailstorms as estimated by satellite passive microwave imagers. Journal of Climate 25, 687-703.
| Crossref | Google Scholar |
Chen Q, Koren I, Altaratz O, Heiblum RH, Dagan G, Pinto L (2017) How do changes in warm-phase microphysics affect deep convective clouds? Atmospheric Chemistry and Physics 17, 9585-9598.
| Crossref | Google Scholar |
Chen Q, Yin Y, Jiang H, Chu Z, Xue L, Shi R, Zhang X, Chen J (2019) The roles of mineral dust as cloud condensation nuclei and ice nuclei during the evolution of a hail storm. Journal of Geophysical Research: Atmospheres 124(14), 14262-284.
| Crossref | Google Scholar |
Cotton WR, Pielke RA, Sr, Walko RL, Liston GE, Tremback CJ, Jiang H, McAnelly RL, Harrington JY, Nicholls ME, Carrio GG, McFadden JP (2003) RAMS 2001: current status and future directions. Meteorology and Atmospheric Physics 82, 5-29.
| Crossref | Google Scholar |
Crespo NM, da Rocha RP, de Jesus EM (2020a) Cyclone density and characteristics in different reanalysis datasets over South America. In ‘EGU2020: Sharing Geoscience Online’, 4–8 May 2020, held virtually. Session AS1.23, presentation EGU2020-11316. (EGU General Assembly) Available at https://presentations.copernicus.org/EGU2020/EGU2020-11316_presentation.pdf [Online poster presentation]
Crespo NM, da Rocha RP, Sprenger M, Wernli H (2020b) A potential vorticity perspective on cyclogenesis over centre-eastern South America. International Journal of Climatology 41, 663-678.
| Crossref | Google Scholar |
de Jesus EM, da Rocha RP, Crespo NM, Reboita MS, Gozzo LF (2021) Multi-model climate projections of the main cyclogenesis hot-spots and associated winds over the eastern coast of South America. Climate Dynamics 56, 537-557.
| Crossref | Google Scholar |
Dennis EJ, Kumjian MR (2017) The impact of vertical wind shear on hail growth in simulated supercells. Journal of the Atmospheric Sciences 74, 641-663.
| Crossref | Google Scholar |
Durkee JD, Mote TL (2010) A climatology of warm-season mesoscale convective complexes in subtropical South America. International Journal of Climatology 30, 418-431.
| Crossref | Google Scholar |
Eirund GK, Drossaart van Dusseldorp S, Brem BT, Dedekind Z, Karrer Y, Stoll M, Lohmann U (2022) Aerosol–cloud–precipitation interactions during a Saharan dust event – a summertime case-study from the Alps. Quarterly Journal of the Royal Meteorological Society 148, 943-961.
| Crossref | Google Scholar |
Eliasson S, Holl G, Buehler SA, Kuhn T, Stengel M, Iturbide‐Sanchez F, Johnston M (2013) Systematic and random errors between collocated satellite ice water path observations. Journal of Geophysical Research: Atmospheres 118, 2629-2642.
| Crossref | Google Scholar |
Foote GB (1984) A Study of hail growth utilizing observed storm conditions. Journal of Climate and Applied Meteorology 23, 84-101.
| Crossref | Google Scholar |
Freire JLM, Coelho CAS, Freitas SR, et al. (2022) Assessing the contribution of dynamical downscaling to austral autumn northeast Brazil seasonal precipitation prediction performance. Climate Services 27, 100321.
| Crossref | Google Scholar |
Freitas SR, Longo KM, Silva Dias MAF, et al. (2005) Monitoring the transport of biomass burning emissions in South America. Environmental Fluid Mechanics 5, 135-167.
| Crossref | Google Scholar |
Freitas SR, Longo KM, Silva Dias MAF, et al. (2009) The coupled aerosol and tracer transport model to the Brazilian developments on the regional atmospheric modeling system (CATT-BRAMS) – part 1: model description and evaluation. Atmospheric Chemistry and Physics 9, 2843-2861.
| Crossref | Google Scholar |
Freitas SR, Panetta J, Longo KM, et al. (2017) The Brazilian developments on the regional atmospheric modeling system (BRAMS 5.2): an integrated environmental model tuned for tropical areas. Geoscientific Model Development 10, 189-222.
| Crossref | Google Scholar | PubMed |
Freud E, Rosenfeld D (2012) Linear relation between convective cloud drop number concentration and depth for rain initiation. Journal of Geophysical Research: Atmospheres 117, D02207.
| Crossref | Google Scholar |
Funk C, Peterson P, Landsfeld M, Pedreros D, Verdin J, Shukla S, Husak G, Rowland J, Harrison L, Hoell A, Michaelsen J (2015) The climate hazards infrared precipitation with stations – a new environmental record for monitoring extremes. Scientific Data 2, 150066.
| Crossref | Google Scholar | PubMed |
Gao J, Stensrud DJ (2012) Assimilation of reflectivity data in a convective-scale, cycled 3DVAR framework with hydrometeor classification. Journal of the Atmospheric Sciences 69(3), 1054-1065.
| Crossref | Google Scholar |
Gilmore MS, Straka JM, Rasmussen EN (2004) Precipitation and Evolution sensitivity in simulated deep convective storms: comparisons between liquid-only and simple ice and liquid phase microphysics. Monthly Weather Review 132(8), 1897-1916.
| Crossref | Google Scholar |
Gordon J, Albert D (2000) A comprehensive severe weather fore-cast checklist and reference guide. TSp 10, NWS central region. Technical Service Publication. (NOAA National Weather Service: Kansas City, MO, USA) Available at https://library.metoffice.gov.uk/portal/Default/en‐GB/RecordView/Index/181168
Greene DR, Clark RA (1972) Vertically integrated liquid water – a new analysis tool. Monthly Weather Review 100(7), 548-552.
| Crossref | Google Scholar |
Grell GA, Freitas SR (2014) A scale and aerosol aware stochastic convective parameterization for weather and air quality modeling. Atmospheric Chemistry and Physics 14, 5233-5250.
| Crossref | Google Scholar |
Hersbach H, Bell B, Berrisford P, et al. (2020) The ERA5 global reanalysis. Quarterly Journal of the Royal Meteorological Society 146, 1999-2049.
| Crossref | Google Scholar |
Iacono MJ, Delamere JS, Mlawer EJ, Shephard MW, Clough SA, Collins WD (2008) Radiative forcing by long-lived greenhouse gases: calculations with the AER radiative transfer models. Journal of Geophysical Research: Atmospheres 113, D13103.
| Crossref | Google Scholar |
Ilotoviz E, Khain AP, Benmoshe N, Phillips VTJ, Ryzhkov AV (2016) Effect of aerosols on freezing drops, hail, and precipitation in a midlatitude storm. Journal of the Atmospheric Sciences 73, 109-144.
| Crossref | Google Scholar |
Jaenicke R (2005) Abundance of cellular material and proteins in the atmosphere. Science 308, 73.
| Crossref | Google Scholar | PubMed |
Johnson AW, Sugden KE (2014) Evaluation of sounding-derived thermodynamic and wind-related parameters associated with large hail events. E-Journal of Severe Storms Meteorology 9(5), 1-42.
| Crossref | Google Scholar |
Koch SE, desJardins M, Kocin PJ (1983) An interactive Barnes objective map analysis scheme for use with satellite and conventional data. Journal of Applied Meteorology and Climatology 22, 1487-1503.
| Crossref | Google Scholar |
Kumjian MR, Lebo ZJ, Ward AM (2019) Storms producing large accumulations of small hail. Journal of Applied Meteorology and Climatology 58, 341-364.
| Crossref | Google Scholar |
Lavin-Gullon A, Feijoo M, Solman S, Fernandez J, da Rocha RP, Bettolli ML (2021) Synoptic forcing associated with extreme precipitation events over southeastern South America as depicted by a CORDEX FPS set of convection-permitting RCMs. Climate Dynamics 56, 3187-3203.
| Crossref | Google Scholar |
Lin Y, Kumjian MR (2022) Influences of CAPE on hail production in simulated supercell storms. Journal of the Atmospheric Sciences 79(1), 179-204.
| Crossref | Google Scholar |
Lin YL, Farley RD, Orville HD (1983) Bulk parameterization of the snow field in a cloud model. Journal of Climate and Applied Meteorology 22(6), 1065-1092.
| Crossref | Google Scholar |
Liu Y, Daum PH, Guo H, Peng Y (2008) Dispersion bias, dispersion effect, and the aerosol-cloud conundrum. Environmental Research Letters 3, 045021.
| Crossref | Google Scholar |
Mahrt F, Kilchhofer K, Marcolli C, Gronquist P, David RO, Rosch M, Lohmann U, Kanji ZA (2020) The impact of cloud processing on the ice nucleation abilities of soot particles at cirrus temperatures. Journal of Geophysical Research: Atmospheres 125, e2019JD030922.
| Crossref | Google Scholar |
Mantoani MC, Quintino TB, Emygdio APM, Guerra LCC, Dias MAFS, Dias PLS, Rodrigues F, Silva DMC, Duo Filho VB, Rudke AP, Alves RA, Martins LD, Martins JA, Siqueira A, Boschilia SM, Carotenuto F, Šantl-Temkiv T, Phillips V, Gonçalves FLT (2023) Biological characterisation of hailstones from two storms in south Brazil. Aerobiology 1, 98-108.
| Crossref | Google Scholar |
Marengo JA, Douglas MW, Silva Dias PL (2002) The South American low-level jet east of the Andes during the 1999 LBA-TRMM and LBA-WET AMC campaign. Journal of Geophysical Research: Atmospheres 107, 8079.
| Crossref | Google Scholar |
Marinescu PJ, van den Heever SC, Heikenfeld M, et al. (2021) Impacts of varying concentrations of cloud condensation nuclei on deep convective cloud updrafts – a multimodel assessment. Journal of the Atmospheric Sciences 78, 1147-1172.
| Crossref | Google Scholar |
Martins JA, Silva Dias MAF, Gonçalves FLT (2009) Impact of biomass burning aerosols on precipitation in the Amazon: a modeling case study. Journal of Geophysical Research: Atmospheres 114(D2), D02207.
| Crossref | Google Scholar |
Martins JA, Brand VS, Capucim MN, Felix RR, Martins LD, Freitas ED, Gonçalves FLT, Hallak R, Dias MAFS, Cecil DJ (2017) Climatology of destructive hailstorms in Brazil. Atmospheric Research 184, 126-138.
| Crossref | Google Scholar |
Mattingly KS, Mote TL (2016) Variability in warm-season atmospheric circulation and precipitation patterns over subtropical South America: relationships between the South Atlantic convergence zone and large-scale organized convection over the La Plata basin. Climate Dynamics 48, 241-263.
| Crossref | Google Scholar |
Mellor GL, Yamada T (1982) Development of a turbulence closure model for geophysical fluid problems. Reviews of Geophysics 20, 851-875.
| Crossref | Google Scholar |
Meyers MP, Walko RL, Harrington JY, Cotton WR (1997) New RAMS cloud microphysics parameterization. Part II: the two-moment scheme. Atmospheric Research 45, 3-39.
| Crossref | Google Scholar |
Miller RC (1972) Notes on analysis and severe storm forecasting procedures of the Air Force Global Weather Central. Technical Report 200 (Rev.). (Air Weather Service, US Air Force) Available at https://apps.dtic.mil/sti/pdfs/AD0744042.pdf
Mölders N, Kramm G (2010) A case study on wintertime inversions in Interior Alaska with WRF. Atmospheric Research 95, 314-332.
| Crossref | Google Scholar |
Moreira DS, Freitas SR, Bonatti JP, Mercado LM, Rosário NMÉ, Longo KM, Miller JB, Gloor M, Gatti LV (2013) Coupling between the JULES land-surface scheme and the CCATT-BRAMS atmospheric chemistry model (JULES-CCATT-BRAMS1.0): applications to numerical weather forecasting and the CO2 budget in South America. Geoscientific Model Development 6, 1243-1259.
| Crossref | Google Scholar |
Morrison H, van Lier-Walqui M, Fridlind AM, Grabowski WW, Harrington JY, Hoose C, Korolev A, Kumjian MR, Milbrandt JA, Pawlowska H, Posselt DJ, Prat OP, Reimel KJ, Shima SI, van Diedenhoven B, Xue L (2020) Confronting the challenge of modeling cloud and precipitation microphysics. Journal of Advances in Modeling Earth Systems 12(8), e2019MS001689.
| Crossref | Google Scholar | PubMed |
Mosier RM, Schumacher C, Orville RE, Carey LD (2011) Radar nowcasting of cloud-to-ground lightning over Houston, Texas. Weather and Forecasting 26(2), 199-212.
| Crossref | Google Scholar |
Nakajima T, King MD (1990) Determination of the optical thickness and effective particle radius of clouds from reflected solar radiation measurements. Part I: theory. Journal of the Atmospheric Sciences 47, 1878-1893.
| Crossref | Google Scholar |
Nesbitt SW, Salio PV, Ávila E, et al. (2021) A storm safari in subtropical South America: Proyecto RELAMPAGO. Bulletin of the American Meteorological Society 102(8), E1621-E1644.
| Crossref | Google Scholar |
O’Sullivan D, Murray BJ, Ross JF, Whale TF, Price HC, Atkinson JD, Umo NS, Webb ME (2015) The relevance of nanoscale biological fragments for ice nucleation in clouds. Scientific Reports 5, 8082.
| Crossref | Google Scholar | PubMed |
Pavani CAB, Freitas SR, Lima WFA, Coelho SMSdC, Rosário NMÉd, Moreira DS, Yoshida MC (2016) Incluindo funcionalidades no modelo BRAMS para simular o transporte de cinzas vulcânicas: descrição e análise de sensibilidade aplicada ao evento eruptivo do Puyehue em 2011. Revista Brasileira de Meteorologia 31(4), 377-393 [In Portuguese].
| Crossref | Google Scholar |
Pielke RA, Cotton WR, Walko RL, Tremback CJ, Lyons WA, Grasso LD, Nicholls ME, Moran MD, Wesley DA, Lee TJ, Copeland JH (1992) A comprehensive meteorological modeling system – RAMS. Meteorology and Atmospheric Physics 49, 69-91.
| Crossref | Google Scholar |
Prein AF, Holland GJ (2018) Global estimates of damaging hail hazard. Weather and Climate Extremes 22, 10-23.
| Crossref | Google Scholar |
Rasmussen KL, Houze RA (2011) Orogenic convection in subtropical South America as seen by the TRMM satellite. Monthly Weather Review 139, 2399-2420.
| Crossref | Google Scholar |
Rasmussen KL, Houze RA (2016) Convective Initiation near the Andes in subtropical South America. Monthly Weather Review 144, 2351-2374.
| Crossref | Google Scholar |
Rasmussen RM, Levizzani V, Pruppacher HR (1984) A Wind tunnel and theoretical study on the melting behavior of atmospheric ice particles: III. experiment and theory for spherical ice particles of radius > 500 μm. Journal of the Atmospheric Sciences 41, 381-388.
| Crossref | Google Scholar |
Roberts NM, Lean HW (2008) Scale-selective verification of rainfall accumulations from high-resolution forecasts of convective events. Monthly Weather Review 136(1), 78-97.
| Crossref | Google Scholar |
Romatschke U, Houze RA (2010) Extreme summer convection in South America. Journal of Climate 23, 3761-3791.
| Crossref | Google Scholar |
Rozante JR, Moreira DS, de Goncalves LGG, Vila DA (2010) Combining TRMM and surface observations of precipitation: technique and validation over South America. Weather Forecasting 25(3), 885-894.
| Crossref | Google Scholar |
Rybka H, Burkhardt U, Köhler M, Arka I, Bugliaro L, Görsdorf U, Horváth Á, Meyer CI, Reichardt J, Seifert A, Strandgren J (2021) The behavior of high-CAPE (convective available potential energy) summer convection in large-domain large-eddy simulations with ICON. Atmospheric Chemistry and Physics 21, 4285-4318.
| Crossref | Google Scholar |
Saha S, Moorthi S, Wu X, et al. (2014) The NCEP climate forecast system version 2. Journal of Climate 27, 2185-2208.
| Crossref | Google Scholar |
Salio P, Nicolini M, Zipser EJ (2007) Mesoscale convective systems over southeastern South America and their relationship with the South American low-level jet. Monthly Weather Review 135, 1290-1309.
| Crossref | Google Scholar |
Sesartic A, Lohmann U, Storelvmo T (2013) Modelling the impact of fungal spore ice nuclei on clouds and precipitation. Environmental Research Letters 8, 014029.
| Crossref | Google Scholar |
Silva CMS, de Freitas SR, Gielow R (2012) Numerical simulation of the diurnal cycle of rainfall in SW Amazon basin during the 1999 rainy season: the role of convective trigger function. Theoretical and Applied Climatology 109, 473-483.
| Crossref | Google Scholar |
Slingo A (1989) A GCM parameterization for the shortwave radiative properties of water clouds. Journal of the Atmospheric Sciences 46, 1419-1427.
| Crossref | Google Scholar |
Smith PL, Myers CG, Orville HD (1975) Radar reflectivity factor calculations in numerical cloud models using bulk parameterization of precipitation. Journal of Applied Meteorology and Climatology 14(6), 1156-1165.
| Crossref | Google Scholar |
Souza DO, Alvalá RCS, Nascimento MG (2016) Urbanization effects on the microclimate of Manaus: a modeling study. Atmospheric Research 167, 237-248.
| Crossref | Google Scholar |
Su L, Fung JCH (2018) Investigating the role of dust in ice nucleation within clouds and further effects on the regional weather system over East Asia – part 1: model development and validation. Atmospheric Chemistry and Physics 18, 8707-8725.
| Crossref | Google Scholar |
Takahashi T (1976) Hail in an axisymmetric cloud model. Journal of the Atmospheric Sciences 33, 1579-1601.
| Crossref | Google Scholar |
Thompson G, Eidhammer T (2014) A study of aerosol impacts on clouds and precipitation development in a large winter cyclone. Journal of the Atmospheric Sciences 71, 3636-3658.
| Crossref | Google Scholar |
Twomey S (1977) The influence of pollution on the shortwave albedo of clouds. Journal of the Atmospheric Sciences 34, 1149-1152.
| Crossref | Google Scholar |
Vara-Vela AL, Herdies DL, Alvim DS, Vendrasco ÉP, Figueroa SN, Pendharkar J, Reyes Fernandez JP (2021) A new predictive framework for Amazon forest fire smoke dispersion over South America. Bulletin of the American Meteorological Society 102, E1700-E1713.
| Crossref | Google Scholar |
Velasco I, Fritsch JM (1987) Mesoscale convective complexes in the Americas. Journal of Geophysical Research: Atmospheres 92, 9591-9613.
| Crossref | Google Scholar |
Vera C, Baez J, Douglas M, Emmanuel CB, Marengo J, Meitin J, Nicolini M, Nogues-Paegle J, Paegle J, Penalba O, Salio P, Saulo C, Silva Dias MA, Silva Dias P, Zipser E (2006) The South American Low-Level Jet Experiment. Bulletin of the American Meteorological Society 87, 63-78.
| Crossref | Google Scholar |
Verrier S, Barthès L, Mallet C (2013) Theoretical and empirical scale dependency of Z-R relationships: evidence, impacts, and correction. Journal of Geophysical Research: Atmospheres 118, 7435-7449.
| Crossref | Google Scholar |
Walko RL, Cotton WR, Meyers MP, et al. (1995) New RAMS cloud microphysics parameterization: part I: the single-moment scheme. Atmospheric Research 38, 29-62.
| Crossref | Google Scholar |
Yang P, Bi L, Baum BA, Liou K-N, Kattawar GW, Mishchenko MI, Cole B (2013) Spectrally consistent scattering, absorption, and polarization properties of atmospheric ice crystals at wavelengths from 0.2 to 100 µm. Journal of the Atmospheric Sciences 70, 330-347.
| Crossref | Google Scholar |
Zemp DC, Schleussner C-F, Barbosa HMJ, van der Ent RJ, Donges JF, Heinke J, Sampaio G, Rammig A (2014) On the importance of cascading moisture recycling in South America. Atmospheric Chemistry and Physics 14, 13337-13359.
| Crossref | Google Scholar |
Zhao DF, Buchholz A, Tillmann R, Kleist E, Wu C, Rubach F, Kiendler-Scharr A, Rudich Y, Wildt J, Mentel TF (2017) Environmental conditions regulate the impact of plants on cloud formation. Nature Communications 8, 14067.
| Crossref | Google Scholar |
Ziegler CL, Ray PS, Knight NC (1983) Hail growth in an Oklahoma multicell storm. Journal of the Atmospheric Sciences 40, 1768-1791.
| Crossref | Google Scholar |
Zipser EJ, Cecil DJ, Liu C, Nesbitt SW, Yorty DP (2006) Where are the most intense thunderstorms on Earth? Bulletin of the American Meteorological Society 87, 1057-1072.
| Crossref | Google Scholar |