Davide Cammarano and Simon Cook
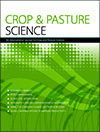
Volume 74 Number 6 2023
Special IssueDigital Agriculture
Davide Cammarano and Simon Cook
The Graincast™ suite of tools was developed to monitor crop productivity in near real-time across the Australian landscape. Four separate tools were created: the C-Crop model, which monitors crop yields with satellite; CropID, which monitors the crop species and areas of production; the Graincast™ app, which provides field-scale estimates of crop production and soil-water use; and Field Boundaries (ePaddocks), which identifies every cropped field across the landscape. The paper describes the design process, including data acquisition, machine learning, testing, validation, human-centred design and the delivery of outputs to end-users.
CP21386 Abstract | CP21386 Full Text | CP21386PDF (2.8 MB) Open Access Article
CP21594Current status of and future opportunities for digital agriculture in Australia

Digital agriculture in Australia is considered immature and its adoption ad hoc, despite a relatively advanced technology innovation sector. Increasing digital maturity and adoption in Australian agriculture will require working with end users to better design and integrate technology, improvements to supporting legislation and education, better knowledge brokering and exchange, better conversion of data to decisions, and further improvement to enabling technologies. Agronomists and early adopter farmers will play a significant role in closing the technology-end user gap.
CP21594 Abstract | CP21594 Full Text | CP21594PDF (1.6 MB) Open Access Article
CP21593The complex adoption pathways of digital technology in Australian livestock supply chains systems
The complex adoption pathways of digital technology in Australian livestock supply chains systems are discussed in this paper. We argue that – since quality appears to be the major basis on which Australian red meat producers will compete in global markets – the broad adoption of digital technology will prove increasingly essential to future growth of this supply chain. We discuss how value is created and how digital transformation involves organisations, people, learning and processes.
CP21593 Abstract | CP21593 Full Text | CP21593PDF (1.7 MB) Open Access Article
CP21759How digital is agriculture in a subset of countries from South America? Adoption and limitations









The status of digital agriculture (DA) in South America (SA) is unknown. This article quantified adoption of existing DA technologies, identified limitations for DA adoption, implementing a literature review and case studies. Sustainable metrics were summarised to benchmark DA impact. GPS guidance systems, mapping tools, mobile apps and remote sensing were the most adopted DA technologies in SA. The most reported limitations to adoption were technology cost, lack of training, limited number of companies providing services, and unclear benefits from DA. The proposed metrics to benchmark DA benefits will allow stakeholders to design targeted initiatives to promote DA towards sustainability of food production in the region.
CP21759 Abstract | CP21759 Full Text | CP21759PDF (2.6 MB) | CP21759Supplementary Material (773 KB) Open Access Article
CP22065Digital agriculture in Europe and in France: which organisations can boost adoption levels?
Worldwide, agriculture is facing the challenges of digital and green transformations of its practices. This article describes how, in Europe and in France, an organisational ecosystem is developing to tackle these transformations in digital agriculture and facilitate their coevolution. Through European and national strategies, the structuring of research and innovation initiatives and capacity building, we describe how organisations are investing in the creation and deployment of digital technologies and solutions for safer and more sustainable food systems.
CP22065 Abstract | CP22065 Full Text | CP22065PDF (1.5 MB) Open Access Article
CP21624Whither digital agriculture in India?


We try to determine whether digital agriculture technologies can correct widespread operational inefficiencies in Indian agriculture.. We observe digital agriculture’s transition from individual farms to the whole value chain despite infrastructural and policy obstacles. India’s public policy shows signs of a longstanding investment and collaboration in the sector.
Innovations in agriculture have contributed to the emergence of a new branch of biological sciences known as phenomics. Phenomics study the broad range of traits in plants and/or environment parameters relying on cutting-edge technologies combining different types of sensors and imaging systems to be employed in both greenhouses and open fields for large-scale trials. These automated technologies generate an extremely large and heterogeneous number of complex variables that require artificial-intelligence processing systems for the management of data.
CP21710Insect detection from imagery using YOLOv3-based adaptive feature fusion convolution network
This paper introduces an insect detection framework based on an improved YOLOv3 network to detect tiny insects on an agricultural pest dataset (Pest24). We applied data augmentation techniques to regularise the dataset, the adaptive feature fusion module is then integrated to reuse features at different level. The resulting features are then fed into the YOLOv3 object detector network for detection (localisation and classification of insects). The dataset was evaluated on different state-of-the-art detectors for comparison.
CP21710 Abstract | CP21710 Full Text | CP21710PDF (4.7 MB) Open Access Article
Deep machine learning-based weed detection techniques can offer automatic and practical solutions for large cropping areas. In this work, we construct a benchmark dataset by combining several small datasets and thoroughly evaluate five state-of-the-art deep learning models to classify crop and weed datasets and thoroughly evaluate five state-of-the-art deep learning models to classify crop and weed.
CP21626 Abstract | CP21626 Full Text | CP21626PDF (4.7 MB) Open Access Article