Linkage mapping and whole-genome predictions in canola (Brassica napus) subjected to differing temperature treatments
Chadwick B. Koscielny

A Plant Breeding Research & Development, Corteva agriscience, Carman, MB R0G 0J0, Canada.
B Biostatistics, Corteva agriscience, 8305 NW 62nd Avenue, Johnston, IA 50131-7060, USA.
C Breeding Technologies, Corteva agriscience, 596779 Country Road 59N, Woodstock, ON N4S 7W1, Canada.
D Department of Plant Science, University of Manitoba, 222 Agriculture Building, Winnipeg, MB R3T 2N2, Canada.
E Corresponding author. Email: rob.duncan@umanitoba.ca
Crop and Pasture Science 71(3) 229-238 https://doi.org/10.1071/CP19387
Submitted: 4 October 2019 Accepted: 25 December 2019 Published: 1 April 2020
Journal compilation © CSIRO 2020 Open Access CC BY-NC-ND
Abstract
Canola (Brassica napus L.) is grown on >8 Mha in Canada and is sensitive to high temperatures; therefore, research on breeding methodologies to improve heat-stress tolerance is warranted. This study utilised a doubled-haploid population created from two parents (PB36 and PB56) that differed in their ability to set seed following growth at high temperatures. The experiment was designed to identify potential quantitative trait loci (QTLs) responsible for conferring tolerance to increased temperatures, and to utilise this population as a test case for evaluating the prospects of whole-genome prediction. The population was phenotyped in a split-plot, randomised complete block experimental design at three locations with two planting-date treatments. The first planting date was during the normal planting period (control), and the second planting was timed to experience increased average temperatures (1.7°C, 2.0°C and 1.2°C) and increased number of days with maximum temperatures above the critical temperature of 29.5°C (4, 12 and 3 days). The stress treatment reduced yield on average by 16.7%. There were 66 QTLs discovered across the nine traits collected. Given the quantitative nature of the traits collected, the ability to use whole-genome prediction was investigated. The prediction accuracies ranged from 0.14 (yield) to 0.66 (1000-seed weight). Prediction had higher accuracy within the stress treatment than within the control treatment for seven of the nine traits, demonstrating that phenotyping within a stress environment can provide valuable data for whole-genome predictions.
Additional keywords: breeding methods, climate change, predictive breeding.
Introduction
Breeding for heat-stress tolerance in cool-season crops such as canola (Brassica napus L.) is necessary to ensure continued genetic gain in a warming environment. The impact of increased temperatures on canola was demonstrated through an analysis of growers’ canola yields over 34 years in Saskatchewan, Canada, which found that for every 1°C increase in temperature, yield declined by 75.5 kg ha–1(Kutcher et al. 2010). The threshold maximum temperature at which canola yields have been found to decline was reported to be 29.5°C (Morrison and Stewart 2002). In 2016, canola was planted on 8.23 Mha in Canada (Statistics Canada 2016). Using this information, a 1°C increase in mean temperature could translate to an annual loss in canola production of >0.5 Mt in Canada. Progress has been made with respect to heat tolerance of crops such as broccoli (Brassica oleracea L.), cowpea (Vigna unguiculata (L.) Walp.) and potato (Solanum tuberosum L.) through conventional breeding (Driedonks et al. 2016). Considering this progress and the increased availability of genomic data, breeders may exploit this information to decrease the time it takes to discover and integrate a new trait such as tolerance to increased temperatures into their germplasm and cultivars.
Genetic linkage mapping when combined with phenotypic data enables the discovery of quantitative trait loci (QTLs) (Xu 2010). These QTLs enable the implementation of marker-assisted selection (MAS) or marker-assisted backcrossing breeding strategies to retain the trait of interest through generations without costly phenotypic screening (Collard et al. 2005). For QTLs to be highly effective within breeding programs, they must explain a significant proportion of the variation and be stable across environments and populations (Collard et al. 2005). QTLs discovered for abiotic stress traits such as waterlogging and drought have been mapped in B. napus (Li et al. 2014), although the maximum amount of phenotypic variation explained by any one QTL was relatively low, with a maximum of 12.56%. A review of abiotic stress QTLs by Collins et al. (2008) lists a multitude of QTLs; however, attempting to utilise many QTLs with minor effects for genetic gain of a single trait is inefficient and difficult to manage within a breeding program (Bernardo 2016).
A review by Jha et al. (2014), lists numerous QTLs responsible for conferring heat tolerance within many species including Arabidopsis thaliana L., barley (Hordeum vulgare L.), maize (Zea mays L.), potato, rice (Oryza sativa L.), sorghum (Sorghum bicolor (L.) Moench), tomato (Lycopersicon esculentum Mill.) and wheat (Triticum aestivum L.), thereby demonstrating the quantitative nature of heat-stress response. With increased access to whole-genome marker data, breeders are able to employ whole-genome prediction (WGP) for highly quantitative traits (Jannink et al. 2010). Unlike QTL discovery using linkage mapping, WGP models do not have stringent statistical significance limits and are therefore able to utilise all genetic signals detected through the analysis (Meuwissen et al. 2001). With the use of WGP, breeders are able to predict performance of untested genotypes and therefore decrease the time necessary to identify favourable allelic combinations for highly quantitative traits within the germplasm (Heffner et al. 2010). In maize, WGP has been more effective for improving grain yield under drought stress than phenotypic selection alone (Vivek et al. 2017). WGP has been applied to B. napus doubled-haploid (DH) populations for yield and quality traits with varying degrees of success, depending on the population structure, number of individuals within the population, marker density and trait heritability (Würschum et al. 2014; Zou et al. 2016). With respect to heat stress, Bita and Gerats (2013) discuss the difficulty in using low-effect QTLs for improving quantitative traits such as tolerance to increased temperatures and suggest WGP as a promising alternative.
This research utilised a DH mapping population of 148 genotypes derived from two canola inbred lines that differed in their ability to set seed under heat stress (Koscielny et al. 2018b). The objectives of this research were to: (i) identify QTLs responsible for conferring tolerance to increased temperatures, and (ii) utilise this population to assess the prospects of WGP for improving stress tolerance. Both MAS and WGP are useful breeding strategies, but selecting the optimal strategy is imperative in a competitive research area such as plant breeding. This research applies both approaches across control and high-temperature environments and discusses strengths and challenges of each strategy.
Materials and methods
Plant materials
Seed from the F1 of a cross between two B. napus DH genotypes, PB36 (heat tolerant) and PB56 (heat susceptible), as determined during a prior growth-chamber experiment (Koscielny et al. 2018b) were used as donors to create a DH population. These 148 microspore-derived DH genotypes were seed-increased in a greenhouse in Carman, Manitoba, Canada, to provide sufficient seed for field trials.
Field experiments and environmental data
Field experiments were set up in a split-plot randomised complete block design with two replications. The main effect was planting date with an early and a late planting date, whereby the reproductive period of the second planting was shifted to a later time in order to align with increased summer temperatures. The subplot was genotype. The field experiments were set up in Carman, Manitoba (49.4903, –98.0027) in 2015 and 2017, and in Viluco, Chile (–33.7965, –70.8075) in 2015. Carman soil was of sandy loam texture, and both treatments received the same fertiliser blend of nitrogen, phosphorus, potassium and sulfur (N-P-K-S, 89-34-0-22 kg ha–1) applied before planting. Viluco soil was of a loam texture, and both treatments had the same fertiliser blend of N-P-K-S (125-61-69-0 kg ha–1) applied through the irrigation at 20 and 40 days after planting. The genotypes were seeded in a 3-m row with a 0.5-m spacing between rows. The 2015 and 2017 Carman sites were rainfed, and volumetric water content to a depth of 0.18 m was collected weekly using a TDR 100 Soil Moisture Meter (Spectrum Technologies, Aurora, IL, USA). The 2015 Viluco site was irrigated with drip-tape irrigation supplying 288 mm water; in addition, rainfall of 83.5 mm and 6.2 mm occurred for the first and second planting dates, respectively. Irrigation applications were made every 5 days to ensure that no differences in treatment were due to soil moisture.
Temperatures were collected every 30 min with a 2000 Series Watchdog weather station (Spectrum Technologies). For the second planting date, the average daily mean, maximum and minimum temperatures were higher at all environments, and therefore this planting date will be referred to as the stress treatment (ST), with the first planting date being referred to as the control treatment (CT) (Table 1). Number of days to start of flowering (from planting date to date of first flower), duration of flowering (from date of first flower to date of last flower), days to physiological maturity (from planting date until seed in the bottom third of the main raceme had turned brown), seed yield from the middle 1 m of the row (g), and 1000-seed weight (g) were collected on all plots. Seed-quality parameters were estimated with an NIRSystem 6500 (Foss, Hillerød, Denmark); oil and protein content are presented as a percentage of dry weight, glucosinolate content as μmol g–1, and saturate content as the sum of C12:0, C14:0, C16:0, C18:0, C20:0, C22:0 and C24:0 expressed as a percentage of total fatty acids (Daun et al. 1994).
![]() |
Phenotypic analyses
Statistical analysis of the phenotypic data was completed by using ASReml 4 (VSN International, Hemel Hempstead, UK) to estimate the best linear unbiased predictions (BLUPs) for all entries (Gilmour et al. 2009). The model used was y = Xb + Zu + e, where y was the observed phenotype, b the fixed terms for matrix X, u the random terms for matrix Z, and e the error matrix N(0,Σ) where Σ was an AR1 × AR1 structure (Gilmour et al. 1997). For the single-site analysis, an overall mean and treatment (CT, ST) were considered as fixed effects. Random effects were row and column nested within treatment, entry, and treatment × entry. The multi-location model had overall mean, treatment, location and treatment × location as fixed effects. Random effects were row and column nested within location and treatment, entry, treatment × entry, location × entry, location × treatment × rep, and location × treatment × entry. The analysis to generate the BLUPs for the WGP was a multi-location analysis with the control and heat treatments being analysed independently. Here, overall mean and location was treated as a fixed effect. Random effects were row and column nested within location, entry, and location × entry. The fitted WGP models are thus ‘treatment-specific’. Heritability (H2) was calculated within treatment by using the following model (Cullis et al. 2006):
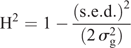
where s.e.d. is the average standard error of the differences between entries, and is the sum of the genotypic and genotype × environment variance assuming a compound symmetry structure (Smith et al. 2005). R 3.3.1 (The R Foundation, Vienna) was used to calculate the Pearson correlation coefficients (r) and the t-test for the trait relationship matrices.
Genotypic analysis
The two parental genotypes had previously been fingerprinted with 1788 and 1663 single-nucleotide polymorphisms (SNPs) for PB36 and PB56, respectively. This information was used to select 526 polymorphic TaqMan SNP markers for the DH population to be genotyped by using an array tape (Douglas Scientific, Alexandria, MN, USA). Once SNPs were assessed for missing data and segregation distortion, 368 SNPs were used to create the final linkage map in MapDisto 1.7 (Lorieux 2012) with an average distance of 4.7 cM between SNPs and a total genetic distance of 1725 cM. The genetic map, and the phenotypic BLUPs, were used to identify QTLs through composite interval mapping using 1000 permutations at a P-value of 0.05 in Windows QTL Cartographer V2.5_011 (WinQTL) (Wang et al. 2012), and only QTLs having a significant LOD score based on the permutation test are reported. QTLs present in all three locations were reported through a combined analysis and defined as ‘stable’ QTLs, whereas those present in only one or two locations are reported on a location-specific basis as ‘putative’ QTLs. Genomic BLUP (GBLUP) was used as a WGP model (Meuwissen et al. 2001), fitted with the rrBLUP R package (Endelman 2011) in R 3.3.1. Specifically, the model was y = μ + g + e where y is the vector of phenotypic values (BLUPs), obtained from the phenotypic analysis described before. The intercept is denoted by μ. The genotypic values in vector g (i.e. the genomic estimated breeding values, or GEBV) were assumed to be distributed as g and the residuals as e
, with I being the identity matrix. The matrix G is the genomic relationship matrix of the entries and was calculated as described by Endelman (2011) with the rrBLUP package, from the same 368 SNPs used for the QTL mapping. The rrBLUP package uses restricted maximum likelihood (REML) for estimating the variance components
and
. Prediction accuracy was evaluated in a random cross-validation. The training set used for fitting the model comprised a random sample of 104 genotypes (70%), with the remaining 44 genotypes (30%) used as the validation set (Jan et al. 2016). Two prediction scenarios were considered: prediction within a treatment (‘within’, e.g. using a ST training set to predict the performance of the validation lines under ST), and prediction across treatments (‘across’, e.g. using a ST training set to predict the performance of the validation lines under CT). The prediction accuracy (i.e. correlation between predicted and true genetic values) was calculated by dividing the Pearson correlation coefficient between predicted and observed trait values of the validation genotypes by the square root of the heritability estimated for the corresponding treatment (Legarra et al. 2008). The cross-validation was replicated 500 times for each treatment and the results summarised as the mean and standard deviation of the prediction accuracies.
Results
Planting dates
The mean, maximum and minimum temperatures for the second planting date were on average 1.6°C, 1.9°C and 1.2°C warmer than those of the first planting date (Table 1). The number of days >29.5°C was greater for the second planting date, with Carman 2015 having four more days >29.5°C, Viluco 2015 having 14 more and Carman 2017 having three more. These two factors of increased mean temperatures and increased number of days above the critical threshold temperature provide evidence that genotypes sown on the second planting date would have experienced increased temperature stress. Soil moisture was monitored at both Carman locations, with the CT having slightly higher water content in 2015 and slightly lower water content in 2017 than the ST, and Viluco was irrigated to ensure moisture availability was equal across planting dates.
Phenotypic analysis
There was a significant effect of treatment, with a decline in seed yield (YIELD) for the ST at all locations ranging from 14.6% to 18.2%, with the combined analysis having a 16.7% decline (Supplementary Materials table S1, available at the journal’s website). All traits for both treatments at all locations had significant differences within population (table S1). The DH population exhibited transgressive segregation for all traits and treatments, and at all locations. The number of days to flower initiation (DYSFLB), duration of flowering (FLWDUR), and days to maturity (MAT) all declined within the ST, whereas glucosinolate content (GLU) increased (Fig. 1).
The combined analysis for FLWDUR, 1000-seed weight (TSW), protein percentage (PRO), and GLU had a significant negative correlation with YIELD in both treatments, whereas oil percentage (OIL) had significant positive correlation with YIELD (Fig. 2). MAT was negatively correlated with YIELD in both ST and CT, although the negative correlation was only statistically significant in ST.
QTL mapping
Linkage mapping analysis identified 66 QTLs across the nine traits and two treatments (table S2). Eight of these QTLs were stable throughout the three environments and the combined analysis. The remaining 58 putative QTLs were found in one or two of the locations. The maximum amount of variation explained with a single QTL was 45.2% in the CT and 54.1% in the ST for DYSFLB (Table 2). This QTL was at the same location on linkage group A02 in both treatments, but only at a single location (Viluco 2015). However, no stable QTLs were found for DYSFLB. The greatest amount of variation explained by a single stable QTL was for FLWDUR (17.7%) in the ST. A QTL associated with YIELD was found in the ST at Viluco 2015 on linkage group A02 and explained 11.4% of the variation. This QTL overlapped with QTLs for FLWDUR and TSW found within the Carman 2015 environment and a QTL for saturated fatty acid content (SAT) within the same environment. Seed-quality traits accounted for 28 putative and four stable QTLs across both treatments. OIL had a stable QTL in the ST compared with the CT, whereas PRO had a single stable QTL present in both treatments. Although OIL and PRO were highly correlated, the QTLs did not overlap; however, they were adjacent on linkage group A09.
![]() |
Whole genome prediction
The broad-sense heritability ranged from a low of 0.64 for YIELD in the CT to a high of 0.97 for GLU in the ST (Table 3). For the ‘within’ prediction scenario, the average prediction accuracy ranged from 0.14 for YIELD in the ST to a high of 0.66 for TSW. In general, the ‘within’ prediction accuracy was higher for the ST than for the CT for all traits except YIELD and SAT (Table 3). Using the ST training set to predict performance in CT decreased the prediction accuracy for DYSFLB and YIELD compared with use of a CT training set (i.e. ‘within’ CT) (Table 4). However, when the CT training set was used for predicting performance in the ST, the accuracies declined for all traits relative to the ‘within’ ST prediction, with the exception of YIELD. Traits related to plant development (DYSFLB, FLWDUR and MAT) showed the greatest decrease in accuracy, with a drop of 0.20, 0.17 and 0.15, respectively. Only a minor decrease in accuracy from ‘within’ to ‘across’ was observed for TSW and the seed-quality traits (OIL, PRO, GLU, SAT).
![]() |
![]() |
Discussion
Yield losses of 75.5–133 kg ha–1 have been reported with a 1°C rise in mean temperatures (Nuttall et al. 1992; Kutcher et al. 2010). Morrison and Stewart (2002), indicated that 29.5°C was the threshold temperature at which canola yields decline. Considering that the ST had an increase >1°C in mean temperature and an increased number of days >29.5°C, these increased temperatures played a role in the decreased yield within the ST. Breeding is the genetic gain through selection, with breeders utilising tools to increase the response to selection by increasing selection intensity and heritability while minimising the amount of time required to describe a phenotype accurately (Cooper et al. 2014). For success, breeders therefore need to allocate finite resources strategically to maximise genetic gain. MAS processes using QTLs and WGP are tools that have shown promise in enabling increased genetic gain (Varshney et al. 2016). This research attempted to identify specific QTLs responsible for conferring tolerance to increased temperatures, and although there was a putative QTL discovered for YIELD within the ST that was absent from the CT, the instability across environments and low amount of variation explained limit the practical utility. Further investigation across populations and environments would be required before the implementation of a MAS strategy. The relatively high prediction accuracies found, and the high number of putative QTLs discovered, suggest that a WGP breeding strategy would be more promising than developing a MAS or marker-assisted backcrossing program for the traits collected within this experiment. We evaluated the utility of a popular WGP strategy of using phenotypic data from a subset of a DH population to train WGP models for predicting untested genotypes. This strategy has the potential to increase the response to selection by utilising larger populations without the need for increased resources because the increased resource requirement for genotyping could be offset by a reduction in phenotyping (Riedelsheimer and Melchinger 2013).
The parameter DYSFLB did not have any relationship with YIELD, and although there were several QTLs explaining a larger portion of the variation within specific environments, these QTLs did not respond differently across treatments. The QTL that explained the most variation (45.3% CT, 54.0% ST) at Viluco 2015 was located on linkage group A02, which aligns with the existing literature for this trait within B. napus (Chen et al. 2010; Nelson et al. 2014; Javed et al. 2016). Given that this QTL was found within the environment that had shorter days, it may be a candidate QTL if germplasm is being transferred from long-day to short-day environments. The time to initiation of flowering in B. napus has been shown to be influenced by photoperiod and thermal time (Nelson et al. 2014). Although the difference in photoperiod was minor between these two treatments, this difference, combined with the increased temperatures in the ST, could potentially strengthen the genetic signal and thereby increase the amount of genetic variation explained by the QTL in the ST. In the absence of photoperiod differences or vernalisation requirements, days to initiation of flowering has proven to be a quantitative trait (Javed et al. 2016). The results in this study show that DYSFLB can be predicted accurately with WGP. This suggests that utilising WGP to select for earlier flowering has the potential to minimise interaction of higher summer temperatures with the reproductive stage of B. napus and improve the avoidance of heat stress (Jung and Müller 2009; Franks 2011). Previous research has shown that traits with few QTLs that explain more variation can be used with higher prediction accuracy (Riedelsheimer et al. 2012). The higher DYSFLB accuracy in the ST compared to the CT may be due to the increased amount of variance explained by the QTLs within the ST.
A stable QTL for FLWDUR in the ST but not the CT requires further investigation because this QTL was stable across environments and explained 17.7% of the phenotypic variation. The negative correlation between FLWDUR and YIELD (shorter flowering duration leading to higher yield) within this population suggests that the ability to develop a large sink strength earlier in the flowering period was linked to higher yield. The ability to predict FLWDUR by using WGP with greater accuracy in the ST than the CT might again be due to the presence of a stable QTL with relatively large effect within the ST. Numerous studies have reported on days to the initiation of flowering, but none could be found reporting on the flowering duration within B. napus. The association of FLWDUR with YIELD, the stable QTL and WGP results warrant further investigation of the importance of this parameter.
The QTL mapping of MAT resulted in the discovery of a stable QTL within the CT. Similar to reports in previous literature, there were several putative QTLs demonstrating the quantitative nature of this trait (Shi et al. 2009). Although the stable QTL was discovered only within the CT, the highest WGP accuracy was found within the ST. This might be because of the considerable increase in heritability, which is expected to increase accuracy (Daetwyler et al. 2010). The reduction in prediction accuracies of DYSFLB, FLWDUR and MAT for the ST with a CT training set demonstrates the unique impact of the ST on these traits. These reductions were not unexpected given the influence of temperature on B. napus plant development (Koscielny et al. 2018a).
The stable QTL on C03 within both treatments for TSW has potential for breeding applications if it can be confirmed across additional populations. Previous QTL mapping of seed weight within B. napus has discovered several QTLs, but there are no reports of the C03 QTL discovered in this DH population (Fan et al. 2010; Ding et al. 2012; Fu et al. 2015). Given the number of QTLs found within this population, the previous literature and high prediction accuracy, future research could focus on using WGP when attempting to select for large seed size. Selection for large seed size will require careful assessment of yield because large seed can be negatively related to seed yield, and this covariance must be considered during selection. The ST had very little impact on QTL mapping and WGP enabling flexibility in phenotyping TSW.
A single QTL for YIELD was found at Carman 2015 across both treatments. There was a single QTL for YIELD found in the ST, but it was present only within a single environment and, thus, may not be useful within a breeding program. It is already accepted that yield is a highly quantitative trait; however, research has also shown the quantitative impact of abiotic stresses such as heat and drought on yield (Pinto et al. 2010; Jha et al. 2014; Thudi et al. 2014). No stable QTLs were discovered in this population for YIELD in the ST, which indicates that WGP is the only viable marker-based approach for selecting for YIELD under heat stress. The discovery that prediction accuracy in the ST was considerably lower than in CT may be due to factors such as heritability. Thus, the lower heritability in the ST was likely partially responsible for the lower accuracy. The lower heritability for YIELD in the ST was not unexpected and has been reported in previous studies (Witcombe et al. 2008). In addition, the quantitative nature of the heat-stress response and the absence of any major QTLs suggest that yield under increased temperatures has an even more complex genetic architecture than under the CT, leading to a negative impact on prediction accuracy (Daetwyler et al. 2010). The decrease in prediction accuracy for YIELD in the CT when the ST was used as the training set and the increase in prediction accuracy in the ST when the CT was used as training set is possibly a consequence of these effects on heritability.
The results demonstrating lower OIL and increased PRO on average within the ST agree with the findings of Daun (2007) in which significant variation was found across eight varieties in the relationship of annual temperatures and oil or protein content. Utilising MAS for improving seed-quality traits within well-adapted inbreds would be challenging given that there are several reports of QTLs for seed-quality traits within B. napus (Yan et al. 2009; Chen et al. 2010; Javed et al. 2016) and the large number of minor-effect QTLs discovered within this population. The seed-quality traits had high heritability and accuracies ranging from 0.38 to 0.56. These values were lower than reported previously (Jan et al. 2016; Zou et al. 2016); however, this can be explained by the larger training population sizes utilised in those studies. Würschum et al. (2014) reported on prediction accuracies within DH families consisting of few individuals and reported accuracies similar to, or slightly lower than, the results reported here. Previous studies on seed-quality traits have reported both a significant genotype × environment interaction (Shafii et al. 1992; Delourme et al. 2006) and minimal impact of genotype × environment interaction (Gunasekera et al. 2006; Aslam et al. 2009). The similarity of accuracy for ‘within’ and ‘across’ predictions reported in this study suggests minimal genotype × treatment interaction for the seed-quality traits (OIL, PRO, GLU and SAT). Therefore, because of the high heritability and the seemingly low impact of environmental stresses such as high temperature, data on seed-quality traits could be collected from a limited set of environments, providing flexibility in the collection of these data and potentially offering the ability to reduce required resources.
These results provide insight and direction for use of linkage mapping and WGP for tolerance to increased temperatures in canola. It is prudent to point out the limitations and directions of further study. Several studies have published QTLs that are population-specific (Bernardo 2016). Further research on the stable QTLs need to be performed in additional populations. The population size in this study limited the size of the training and prediction sets; thus, an increase in population sizes would improve the power of the experiment. Previous research has shown that increased phenotypic heritability has a greater impact on WGP accuracies than training set population size and marker density (Zhang et al. 2017). Given this information future research would benefit from ensuring sufficient resources are deployed to ensure accurate phenotyping once a minimum marker density is available on the germplasm of interest. This information could provide future cost savings by enabling breeding programs to phenotype a subset of germplasm continually for creation of robust training sets while predicting large numbers of genotypes with low-density genotypic information. Several studies in bi-parental maize populations have indicated that increasing marker density beyond 300 with an average distance of 5 cM did not noticeably increase WGP accuracies (Combs and Bernardo 2013; Technow and Totir 2015; Zhang et al. 2015).
Conclusion
Linkage mapping has provided valuable insight into qualitative traits, but its practical use in breeding for quantitative traits such as yield or abiotic stress tolerance, in which many minor QTL effects are involved, has proven impractical (Bita and Gerats 2013). This research has shown that although it is possible to discover stable QTLs that differ across treatments, this same set of data can be deployed in a WGP strategy. With the exception of yield, all traits had prediction accuracies in the ST similar to or higher than in the CT, suggesting that phenotyping within higher temperature environments enables the generation of phenotypic data that can be used for WGP while providing the opportunity to screen for future warming environments. Further investigation into the ability to utilise WGP for making genetic gain under high-temperature stress should be conducted to enable gain within this complex abiotic stress trait ensuring genetic gain can be maintained or increased in a warming climate.
Conflicts of interest
The authors declare there is no conflicts of interest.
Author contributions
Concept and design of this experiment: CBK, SW, and RW; acquisition of the data: CBK; analysis and interpretation of the data: CBK, SWG and FT. Manuscript drafted by CBK and revised by SWG, FT and RWD. All authors approved this version of the manuscript for publication.
Acknowledgements
This research was supported by Corteva agriscience. Sincere appreciation to Sarika Saini, Victoria Mungall, Tyler Huck, Rodrigo Bravo, Xiuqiang Huang, Raju Jetty and Erin Lemky for their technical support in this research. This research did not receive any specific funding.
References
Aslam MN, Nelson MN, Kailis SG, Bayliss KL, Speijers J, Cowling WA (2009) Canola oil increases in polyunsaturated fatty acids and decreases in oleic acid in drought-stressed Mediterranean-type environments. Plant Breeding 128, 348–355.| Canola oil increases in polyunsaturated fatty acids and decreases in oleic acid in drought-stressed Mediterranean-type environments.Crossref | GoogleScholarGoogle Scholar |
Bernardo R (2016) Bandwagons I, too, have known. Theoretical and Applied Genetics 129, 2323–2332.
| Bandwagons I, too, have known.Crossref | GoogleScholarGoogle Scholar | 27681088PubMed |
Bita CE, Gerats T (2013) Plant tolerance to high temperature in a changing environment: scientific fundamentals and production of heat stress-tolerant crops. Frontiers in Plant Science 4, 273
| Plant tolerance to high temperature in a changing environment: scientific fundamentals and production of heat stress-tolerant crops.Crossref | GoogleScholarGoogle Scholar | 23914193PubMed |
Chen G, Geng J, Rahman M, Liu X, Tu J, Fu T, Li G, McVetty PBE, Tahir M (2010) Identification of QTL for oil content, seed yield, and flowering time in oilseed rape (Brassica napus). Euphytica 175, 161–174.
| Identification of QTL for oil content, seed yield, and flowering time in oilseed rape (Brassica napus).Crossref | GoogleScholarGoogle Scholar |
Collard BCY, Jahufer MZZ, Brouwer JB, Pang ECK (2005) An introduction to markers, quantitative trait loci (QTL) mapping and marker-assisted selection for crop improvement: The basic concepts. Euphytica 142, 169–196.
| An introduction to markers, quantitative trait loci (QTL) mapping and marker-assisted selection for crop improvement: The basic concepts.Crossref | GoogleScholarGoogle Scholar |
Collins NC, Tardieu F, Tuberosa R (2008) Quantitative trait loci and crop performance under abiotic stress: where do we stand? Plant Physiology 147, 469–486.
| Quantitative trait loci and crop performance under abiotic stress: where do we stand?Crossref | GoogleScholarGoogle Scholar | 18524878PubMed |
Combs E, Bernardo R (2013) Accuracy of genome-wide selection for different traits with constant population size, heritability, and number of markers. The Plant Genome 6, 1–7.
| Accuracy of genome-wide selection for different traits with constant population size, heritability, and number of markers.Crossref | GoogleScholarGoogle Scholar |
Cooper M, Messina CD, Podlich D, Totir LR, Baumgarten A, Hausmann NJ, Wright D, Graham G (2014) Predicting the future of plant breeding: complementing empirical evaluation with genetic prediction. Crop & Pasture Science 65, 311–336.
| Predicting the future of plant breeding: complementing empirical evaluation with genetic prediction.Crossref | GoogleScholarGoogle Scholar |
Cullis BR, Smith AB, Coombes NE (2006) On the design of early generation variety trials with correlated data. Journal of Agricultural Biological & Environmental Statistics 11, 381–393.
| On the design of early generation variety trials with correlated data.Crossref | GoogleScholarGoogle Scholar |
Daetwyler HD, Pong-Wong R, Villanueva B, Woolliams JA (2010) The impact of genetic architecture on genome-wide evaluation methods. Genetics 185, 1021–1031.
| The impact of genetic architecture on genome-wide evaluation methods.Crossref | GoogleScholarGoogle Scholar | 20407128PubMed |
Daun J (2007) Quality of canola (Brassica napus L.) varieties in Western Canada: evaluation of variability due to genetic, year and environmental conditions using data from Canadian Grain Commission Harvest Surveys and from Environment Canada meteorological stations. In ‘Proceedings 12th International Rapeseed Congress’. 26–30 March 2007, Wuhan, China. (Eds T Fu, C Guan) pp. 10–14. (Science Press: Beijing)
Daun JK, Clear KM, Williams P (1994) Comparison of three whole seed near-infrared analyzers for measuring quality components of canola seed. Journal of the American Oil Chemists’ Society 71, 1063–1068.
| Comparison of three whole seed near-infrared analyzers for measuring quality components of canola seed.Crossref | GoogleScholarGoogle Scholar |
Delourme R, Falentin C, Huteau V, Clouet V, Horvais R, Gandon B, Specel S, Hanneton L, Dheu JE, Deschamps M, Margale E, Vincourt P, Renard M (2006) Genetic control of oil content in oilseed rape (Brassica napus L.). Theoretical and Applied Genetics 113, 1331–1345.
| Genetic control of oil content in oilseed rape (Brassica napus L.).Crossref | GoogleScholarGoogle Scholar | 16960716PubMed |
Ding G, Zhao Z, Liao Y, Hu Y, Shi L, Long Y, Xu F (2012) Quantitative trait loci for seed yield and yield-related traits, and their responses to reduced phosphorus supply in Brassica napus. Annals of Botany 109, 747–759.
| Quantitative trait loci for seed yield and yield-related traits, and their responses to reduced phosphorus supply in Brassica napus.Crossref | GoogleScholarGoogle Scholar | 22234558PubMed |
Driedonks N, Rieu I, Vriezen WH (2016) Breeding for plant heat tolerance at vegetative and reproductive stages. Plant Reproduction 29, 67–79.
| Breeding for plant heat tolerance at vegetative and reproductive stages.Crossref | GoogleScholarGoogle Scholar | 26874710PubMed |
Endelman JB (2011) Ridge regression and other kernels for genomic selection with R package rrBLUP. The Plant Genome 4, 250–255.
| Ridge regression and other kernels for genomic selection with R package rrBLUP.Crossref | GoogleScholarGoogle Scholar |
Fan C, Cai G, Qin J, Li Q, Yang M, Wu J, Fu T, Liu K, Zhou Y (2010) Mapping of quantitative trait loci and development of allele-specific markers for seed weight in Brassica napus. Theoretical and Applied Genetics 121, 1289–1301.
| Mapping of quantitative trait loci and development of allele-specific markers for seed weight in Brassica napus.Crossref | GoogleScholarGoogle Scholar | 20574694PubMed |
Franks SJ (2011) Plasticity and evolution in drought avoidance and escape in the annual plant Brassica rapa. New Phytologist 190, 249–257.
| Plasticity and evolution in drought avoidance and escape in the annual plant Brassica rapa.Crossref | GoogleScholarGoogle Scholar | 21210818PubMed |
Fu Y, Wei D, Dong H, He Y, Cui Y, Mei J, Wan H, Li J, Snowdon R, Friedt W, Li X, Qian W (2015) Comparative quantitative trait loci for silique length and seed weight in Brassica napus. Scientific Reports 5, 14407
| Comparative quantitative trait loci for silique length and seed weight in Brassica napus.Crossref | GoogleScholarGoogle Scholar | 26394547PubMed |
Gilmour AR, Cullis BR, Verbyla AP (1997) Accounting for natural and extraneous variation in the analysis of field experiments. Journal of Agricultural, Biological, & Environmental Statistics 2, 269–293.
| Accounting for natural and extraneous variation in the analysis of field experiments.Crossref | GoogleScholarGoogle Scholar |
Gilmour AR, Gogel BJ, Cullis BR, Thompson R (2009) ‘ASReml user guide. Release 3.0.’ (VSN International: Hemel Hempstead, UK)
Gunasekera CP, Martin LD, Siddique KHM, Walton GH (2006) Genotype by environment interactions of Indian mustard (Brassica juncea L.) and canola (B. napus L.) in Mediterranean-type environments: 1. Crop growth and seed yield. European Journal of Agronomy 25, 1–12.
| Genotype by environment interactions of Indian mustard (Brassica juncea L.) and canola (B. napus L.) in Mediterranean-type environments: 1. Crop growth and seed yield.Crossref | GoogleScholarGoogle Scholar |
Heffner EL, Lorenz AJ, Jannink J-L, Sorrells ME (2010) Plant breeding with genomic selection: gain per unit time and cost. Crop Science 50, 1681–1690.
| Plant breeding with genomic selection: gain per unit time and cost.Crossref | GoogleScholarGoogle Scholar |
Jan HU, Abbadi A, Lücke S, Nichols RA, Snowdon RJ (2016) Genomic prediction of testcross performance in canola (Brassica napus). PLoS One 11, e0147769
| Genomic prediction of testcross performance in canola (Brassica napus).Crossref | GoogleScholarGoogle Scholar | 26824924PubMed |
Jannink J-L, Lorenz AJ, Iwata H (2010) Genomic selection in plant breeding: from theory to practice. Briefings in Functional Genomics 9, 166–177.
| Genomic selection in plant breeding: from theory to practice.Crossref | GoogleScholarGoogle Scholar | 20156985PubMed |
Javed N, Geng J, Tahir M, McVetty PBE, Li G, Duncan RW (2016) Identification of QTL influencing seed oil content, fatty acid profile and days to flowering in Brassica napus L. Euphytica 207, 191–211.
| Identification of QTL influencing seed oil content, fatty acid profile and days to flowering in Brassica napus L.Crossref | GoogleScholarGoogle Scholar |
Jha UC, Bohra A, Singh NP (2014) Heat stress in crop plants: its nature, impacts and integrated breeding strategies to improve heat tolerance. Plant Breeding 133, 679–701.
| Heat stress in crop plants: its nature, impacts and integrated breeding strategies to improve heat tolerance.Crossref | GoogleScholarGoogle Scholar |
Jung C, Müller AE (2009) Flowering time control and applications in plant breeding. Trends in Plant Science 14, 563–573.
| Flowering time control and applications in plant breeding.Crossref | GoogleScholarGoogle Scholar | 19716745PubMed |
Koscielny CB, Gardner SW, Duncan RW (2018a) Impact of high temperature on heterosis and general combining ability in spring canola (Brassica napus L.). Field Crops Research 221, 61–70.
| Impact of high temperature on heterosis and general combining ability in spring canola (Brassica napus L.).Crossref | GoogleScholarGoogle Scholar |
Koscielny CB, Hazebroek J, Duncan RW (2018b) Phenotypic and metabolic variation among spring Brassica napus L. genotypes during heat stress. Crop & Pasture Science 69, 284–295.
| Phenotypic and metabolic variation among spring Brassica napus L. genotypes during heat stress.Crossref | GoogleScholarGoogle Scholar |
Kutcher HR, Warland JS, Brandt SA (2010) Temperature and precipitation effects on canola yields in Saskatchewan, Canada. Agricultural and Forest Meteorology 150, 161–165.
| Temperature and precipitation effects on canola yields in Saskatchewan, Canada.Crossref | GoogleScholarGoogle Scholar |
Legarra A, Robert-Granié C, Manfredi E, Elsen J-M (2008) Performance of genomic selection in mice. Genetics 180, 611–618.
| Performance of genomic selection in mice.Crossref | GoogleScholarGoogle Scholar | 18757934PubMed |
Li Z, Mei S, Mei Z, Liu X, Fu T, Zhou G, Tu J (2014) Mapping of QTL associated with waterlogging tolerance and drought resistance during the seedling stage in oilseed rape (Brassica napus). Euphytica 197, 341–353.
| Mapping of QTL associated with waterlogging tolerance and drought resistance during the seedling stage in oilseed rape (Brassica napus).Crossref | GoogleScholarGoogle Scholar |
Lorieux M (2012) MapDisto: Fast and efficient computation of genetic linkage maps. Molecular Breeding 30, 1231–1235.
| MapDisto: Fast and efficient computation of genetic linkage maps.Crossref | GoogleScholarGoogle Scholar |
Meuwissen THE, Hayes BJ, Goddard ME (2001) Prediction of total genetic value using genome-wide dense marker maps. Genetics 157, 1819–1829.
Morrison MJ, Stewart DW (2002) Heat stress during flowering in summer Brassica. Crop Science 42, 797–803.
| Heat stress during flowering in summer Brassica.Crossref | GoogleScholarGoogle Scholar |
Nelson MN, Rajasekaran R, Smith A, Chen S, Beeck CP, Siddique KHM, Cowling WA (2014) Quantitative trait loci for thermal time to flowering and photoperiod responsiveness discovered in summer annual-type Brassica napus L. PLoS ONE 9, e102611
| Quantitative trait loci for thermal time to flowering and photoperiod responsiveness discovered in summer annual-type Brassica napus L.Crossref | GoogleScholarGoogle Scholar | 25061822PubMed |
Nuttall WF, Moulin AP, Townly-Smith LJ (1992) Yield response of canola to nitrogen, phosphorus, precipitation, and temperature. Agronomy Journal 84, 765–768.
| Yield response of canola to nitrogen, phosphorus, precipitation, and temperature.Crossref | GoogleScholarGoogle Scholar |
Pinto RS, Reynolds M, Mathews K, McIntyre CL, Olivares-Villegas J-J, Chapman S (2010) Heat and drought adaptive QTL in a wheat population designed to minimize confounding agronomic effects. Theoretical and Applied Genetics 121, 1001–1021.
| Heat and drought adaptive QTL in a wheat population designed to minimize confounding agronomic effects.Crossref | GoogleScholarGoogle Scholar | 20523964PubMed |
Riedelsheimer C, Melchinger AE (2013) Optimizing the allocation of resources for genomic selection in one breeding cycle. Theoretical and Applied Genetics 126, 2835–2848.
| Optimizing the allocation of resources for genomic selection in one breeding cycle.Crossref | GoogleScholarGoogle Scholar | 23982591PubMed |
Riedelsheimer C, Czedik-Eysenberg A, Grieder C, Lisec J, Technow F, Sulpice R, Altmann T, Stitt M, Willmitzer L, Melchinger AE (2012) Genomic and metabolic prediction of complex heterotic traits in hybrid maize. Nature Genetics 44, 217–220.
| Genomic and metabolic prediction of complex heterotic traits in hybrid maize.Crossref | GoogleScholarGoogle Scholar | 22246502PubMed |
Shafii B, Mahler KA, Price WJ, Auld DL (1992) Genotype × environment interaction effects on winter rapeseed yield and oil content. Crop Science 32, 922–927.
| Genotype × environment interaction effects on winter rapeseed yield and oil content.Crossref | GoogleScholarGoogle Scholar |
Shi J, Li R, Qiu D, Jiang C, Long Y, Morgan C, Bancroft I, Zhao J, Meng J (2009) Unraveling the complex trait of crop yield with quantitative trait loci mapping in Brassica napus. Genetics 182, 851
| Unraveling the complex trait of crop yield with quantitative trait loci mapping in Brassica napus.Crossref | GoogleScholarGoogle Scholar | 19414564PubMed |
Smith AB, Cullis BR, Thompson R (2005) The analysis of crop cultivar breeding and evaluation trials: an overview of current mixed model approaches. The Journal of Agricultural Science 143, 449–462.
| The analysis of crop cultivar breeding and evaluation trials: an overview of current mixed model approaches.Crossref | GoogleScholarGoogle Scholar |
Statistics Canada (2016) CANSIM tables: Field and special crops. Statistics Canada, Ottawa. Available at: http://www.statcan.gc.ca/tables-tableaux/sum-som/l01/cst01/prim11a-eng.htm (accessed 5 December 2017).
Technow F, Totir RL (2015) Using Bayesian multilevel whole genome regression models for partial pooling of training sets in genomic prediction. Genes, Genomes, Genetics 5, 1603–1612.
| Using Bayesian multilevel whole genome regression models for partial pooling of training sets in genomic prediction.Crossref | GoogleScholarGoogle Scholar |
Thudi M, Upadhyaya HD, Rathore A, Gaur PM, Krishnamurthy L, Roorkiwal M, Nayak SN, Chaturvedi SK, Basu PS, Gangarao NVPR, Fikre A, Kimurto P, Sharma PC, Sheshashayee MS, Tobita S, Kashiwagi J, Ito O, Killian A, Varshney RK (2014) Genetic dissection of drought and heat tolerance in chickpea through genome-wide and candidate gene-based association mapping approaches. PLoS One 9, e96758
| Genetic dissection of drought and heat tolerance in chickpea through genome-wide and candidate gene-based association mapping approaches.Crossref | GoogleScholarGoogle Scholar | 24801366PubMed |
Varshney RK, Singh VK, Hickey JM, Xun X, Marshall DF, Wang J, Edwards D, Ribaut J-M (2016) Analytical and decision support tools for genomics-assisted breeding. Trends in Plant Science 21, 354–363.
| Analytical and decision support tools for genomics-assisted breeding.Crossref | GoogleScholarGoogle Scholar | 26651919PubMed |
Vivek BS, Krishna GK, Vengadessan V, Babu R, Zaidi PH, Kha LQ, Mandal SS, Grudloyma P, Takalkar S, Krothapalli K, Singh IS, Ocampo ETM, Xingming F, Burgueño J, Azrai M, Singh RP, Crossa J (2017) Use of genomic estimated breeding values results in rapid genetic gains for drought tolerance in maize. The Plant Genome 10, 1–8.
| Use of genomic estimated breeding values results in rapid genetic gains for drought tolerance in maize.Crossref | GoogleScholarGoogle Scholar |
Wang S, Basten CJ, Zeng ZB (2012) ‘Windows QTL Cartographer 2.5.’ (Department of Statistics, North Carolina State: Raleigh, NC, USA)
Witcombe JR, Hollington PA, Howarth CJ, Reader S, Steele KA (2008) Breeding for abiotic stresses for sustainable agriculture. Philosophical Transactions of the Royal Society of London. Series B, Biological Sciences 363, 703–716.
| Breeding for abiotic stresses for sustainable agriculture.Crossref | GoogleScholarGoogle Scholar | 17761467PubMed |
Würschum T, Abel S, Zhao Y (2014) Potential of genomic selection in rapeseed (Brassica napus L.) breeding. Plant Breeding 133, 45–51.
| Potential of genomic selection in rapeseed (Brassica napus L.) breeding.Crossref | GoogleScholarGoogle Scholar |
Xu Y (2010) ‘Molecular plant breeding.’ (CABI: Wallingford, UK)
Yan XY, Li JN, Fu FY, Jin MY, Chen L, Liu LZ (2009) Co-location of seed oil content, seed hull content and seed coat color QTL in three different environments in Brassica napus L. Euphytica 170, 355–364.
| Co-location of seed oil content, seed hull content and seed coat color QTL in three different environments in Brassica napus L.Crossref | GoogleScholarGoogle Scholar |
Zhang X, Perez-Rodriguez P, Semagn K, Beyene Y, Babu R, Lopez-Cruz MA, San Vincent F, Olsen M, Buckler E, Jannick JL, Prasanna BM, Crossa J (2015) Genomic prediction in biparental tropical maize populations in water-stressed and well-watered environments using low-density and GBS SNPs. Heredity 114, 291–299.
| Genomic prediction in biparental tropical maize populations in water-stressed and well-watered environments using low-density and GBS SNPs.Crossref | GoogleScholarGoogle Scholar | 25407079PubMed |
Zhang A, Wang H, Beyene Y, Samagn K, Liu Y, Cao S, Ciu Z, Ruan Y, Burgueno J, San Vincent F, Olsen M, Prasanna BM, Crossa J, Yu H, Zhang X (2017) Effect of trait heritability, training population size and marker density on genomic prediction accuracy estimation in 22 bi-parental tropical maize populations. Frontiers in Plant Science 8, 1916
| Effect of trait heritability, training population size and marker density on genomic prediction accuracy estimation in 22 bi-parental tropical maize populations.Crossref | GoogleScholarGoogle Scholar | 29167677PubMed |
Zou J, Zhao Y, Liu P, Shi L, Wang X, Wang M, Meng J, Reif JC (2016) Seed quality traits can be predicted with high accuracy in Brassica napus using genomic data. PLoS One 11, e0166624
| Seed quality traits can be predicted with high accuracy in Brassica napus using genomic data.Crossref | GoogleScholarGoogle Scholar | 28030633PubMed |