A comprehensive functional trait database of seagrasses in tropical Queensland
Chieh Lin
A
B
Abstract
Seagrasses form an important habitat that provides diverse ecosystem services essential for both the environment and people. In tropical Queensland, Australia, these meadows hold significant economic and cultural value, serving as nurseries for marine species and sustaining dugongs and green turtles. The biomass and size of tropical seagrass meadows in Queensland varies considerably and are influenced by various factors, both biotic and abiotic.
Functional trait-based approaches can improve the estimation of seagrass-meadow resilience and services provision by describing the relationship between environment and individual performance. To support these approaches, we provide a seagrass functional-trait database focusing on resilience and function provision for tropical Queensland.
We employed a combination of literature reviews, database searches, botanical information, and structured expert elicitation to target 17 functional traits across 13 seagrass species in tropical Queensland.
We developed a traits database to inform functional trait-based approaches to assessing seagrass-meadow resilience and dynamics. The outputs included trait information for approximately 78% of the targeted traits (of 221 unique trait–seagrass combinations).
With current information on functional traits, we can improve the estimation of resilience and ecosystem services for tropical Queensland seagrass species. We have also highlighted trait data gaps and areas for further research.
We have provided examples of applying this database within the tropical Queensland context, with the potential to facilitate regional comparative studies. Our database complements existing plant-trait databases and serves as a valuable resource for future trait-based seagrass research in tropical Queensland.
Keywords: database, ecosystem services, functional trait, plant morphology, resilience, seagrasses, trait-based approach, tropical Queensland.
Introduction
Functional traits are specific characteristics of individual organisms that directly affect how well they perform or survive in their environment (Violle et al. 2007; Nock et al. 2016). These traits are crucial ecological indicators that can predict ecological dynamics with a well-established cause and effect relationships between the traits and the targeted ecological process (Streit and Bellwood 2023). For instance, in Eucalyptus trees, bark traits relate to flammability and shifting of bark traits of a forest can indicate fire-frequency changes (Grootemaat et al. 2017). Establishing clear cause-and-effect relationships can help us understand ecosystem services and monitor processes such as increasing fire frequency as a result of climate change. Monitoring traits rather than species alone advances our understanding of ecosystem function and health as different species might share similar traits, known as trait redundancy (Laliberté and Legendre 2010; Nash et al. 2016). Trait-based methods are widely applied in disciplines such as ecology and biological conservation, including the assessment of the vulnerability of ecosystem services to a changing environment (LeRoy Poff et al. 2006; Butt and Gallagher 2018; Wood and Dupras 2021).
The application of trait-based approaches to seagrass ecology has been very limited despite its widespread application in other communities (Moreira-Saporiti et al. 2023). Seagrass meadows are globally distributed and play a crucial role in marine ecosystems (Larkum et al. 2006; Dunic et al. 2021). They serve as nursery grounds for fish and prawn species (Jinks et al. 2019; Jones et al. 2021), and provide feeding habitats for herbivorous marine mammals and sea turtles (Kelkar et al. 2013; Tol et al. 2016). Additionally, seagrass meadows offer valuable ecosystem services such as coastal protection through wave attenuation and significant carbon storage in sediment (Fonseca and Cahalan 1992; Lavery et al. 2013; Johnson et al. 2017; Twomey et al. 2020).
In the tropical Queensland region of Australia (Fig. 1), a diverse range of 12–15 seagrass species have been documented, dependent on inclusion of rare species and definition of species complex (Waycott et al. 2004; Carter et al. 2021a). The total area of seagrass meadows in the Great Barrier Reef (GBR) region of tropical Queensland (Fig. 1) estimated by habitat modelling is from 35,000 (Coles et al. 2015) to 88,331 km2 (Carter et al. 2021b). These meadows are mixed-species meadows and exhibit dynamic changes over time and often with evidence of herbivory (Fig. 2). Several large-scale seagrass-loss events have been recorded in Queensland over the past 30 years (Poiner et al. 1993; Preen et al. 1995; Rasheed et al. 2014; McKenna et al. 2015; James Cook University 2022). These losses have sometimes been caused by a single hazard event, but more commonly are a result of multiple impacts, such as consecutive weather events that inhibit growth and/or cause direct physical damage to meadows (McKenna et al. 2015). Notably, not all seagrass meadows respond to individual or multiple events in the same way; for instance, meadows in the southern GBR displayed a much faster recovery rate than did meadows in the central GBR during a series of hazard events in 2011 (McKenzie et al. 2016). Trait-based methods offer a promising solution to understand the high variability in seagrass meadows and the consequence of meadow dynamics on service delivery. Rather than characterising a seagrass meadow solely on the basis of their species composition, these methods focus on the combination of traits exhibited by the seagrass present in the meadow. This approach allows for a direct link between the characteristics of the seagrass meadow and its performance in response to environmental conditions.
Seagrass presence records in tropical Queensland from 1985 to 2018. Seagrass records are sourced from the TropWATER, James Cook University seagrass monitoring programs (Carter et al. 2021a, 2024).
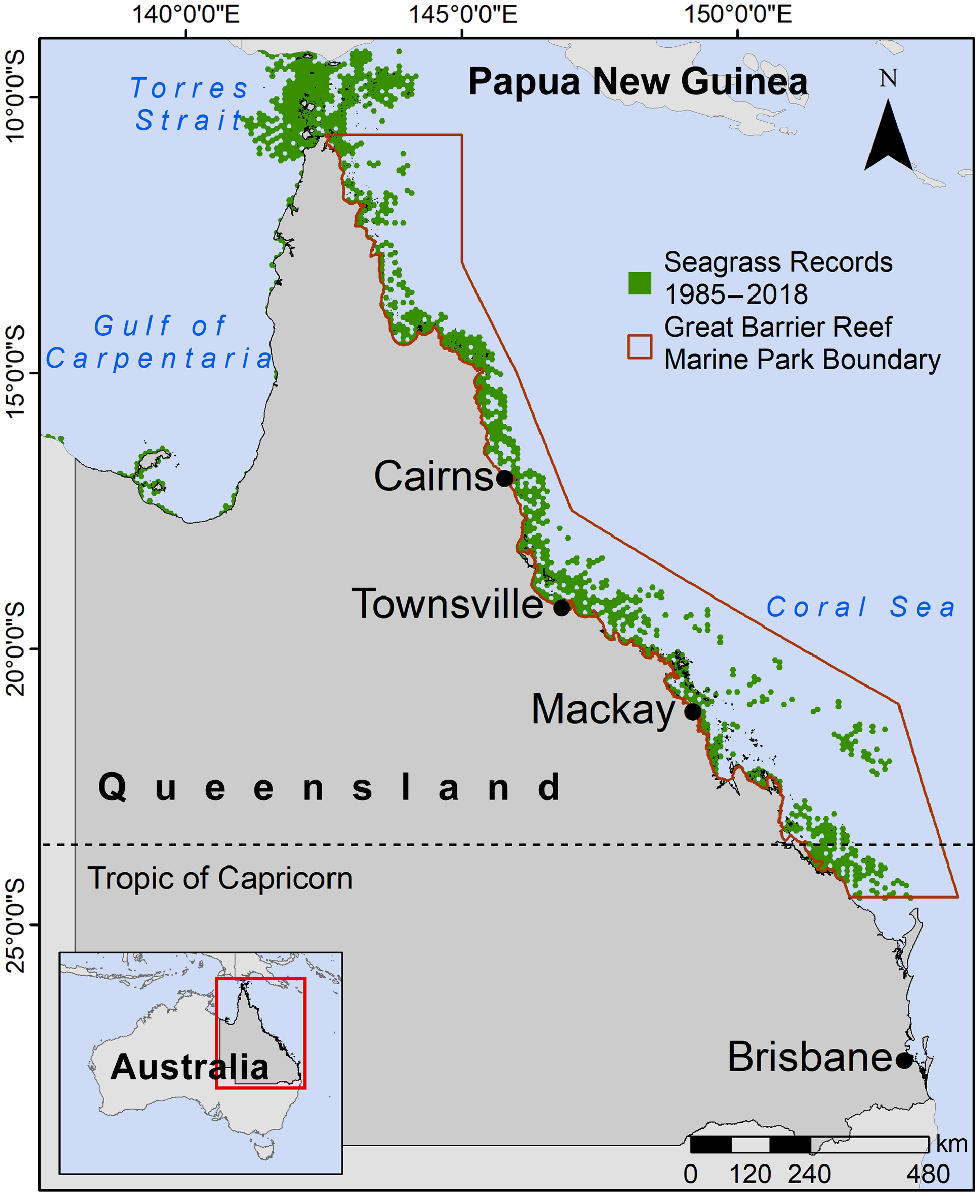
Mixed-species seagrass meadows at Cape Pallarenda, Townsville (19°11′01.3″S, 146°45′53.7″E), showcasing trait diversity and the ecosystem services they provide. (a) General view of these intertidal seagrass meadows. (b) Clear and dense dugong feeding trails indicated with the orange bars. (c) A mixed meadow composed mainly of Halophila ovalis (oval-shaped leaves) and Halodule uninervis (thin needle-like leaves). (d) Halodule uninervis (thin, green needle-like leaves, indicated with a yellow circle) co-exists with Zostera muelleri subsp. Capricorni (brown strap-like leaves, indicated with red star) in this mixed meadow.
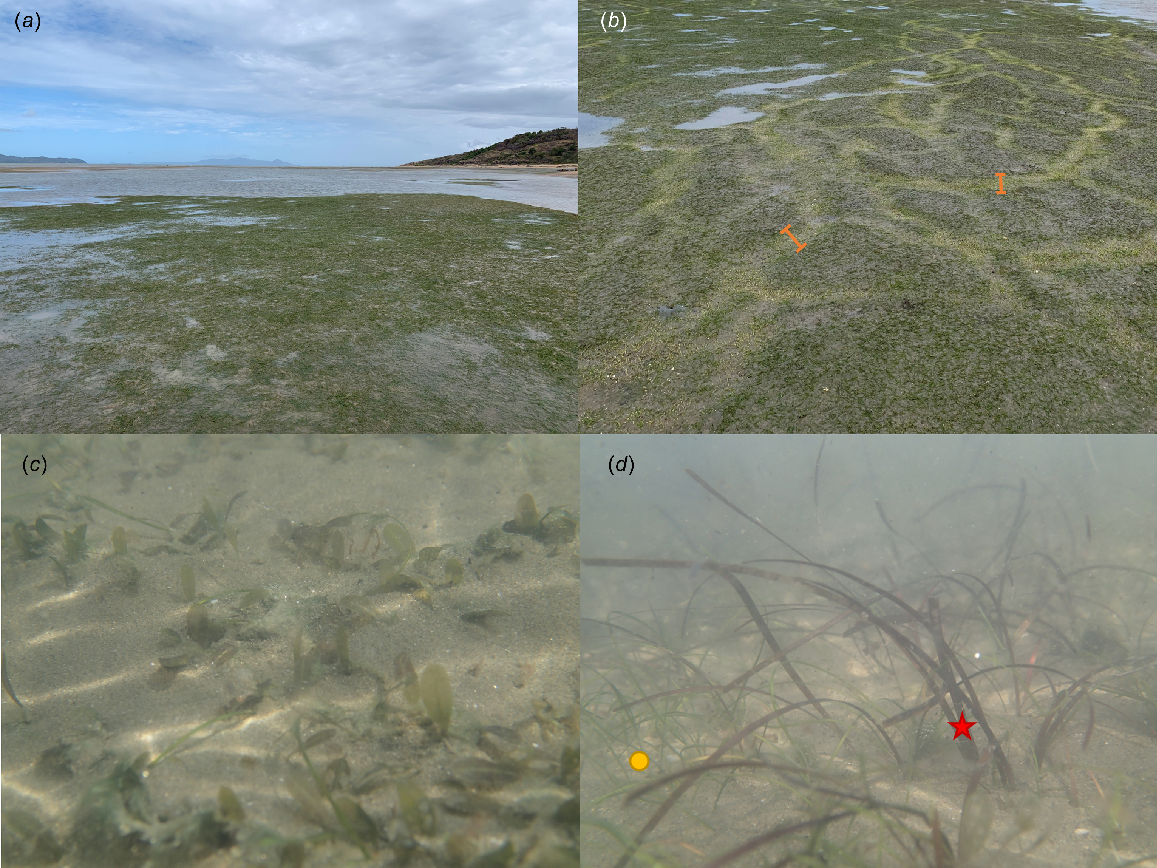
There are two significant knowledge gaps that need to be addressed to effectively apply trait-based approaches in tropical Queensland. First, establishing solid causal relationships between traits and their functional outcomes is crucial (Funk et al. 2017; Streit and Bellwood 2023). Second, there is a lack of comprehensive trait data for seagrass species, which has limited previous research, such as a large-scale coastal protection estimation (De Battisti 2021). Existing curated plant-trait databases, such as TRY Plant Trait Database (Kattge et al. 2020) and Austraits (Falster et al. 2021), have limited information related to aquatic plants, especially submerged seagrass species. Although the current trend of using trait-based approaches is increasing (Moreira-Saporiti et al. 2023), global or local seagrass trait data are hard to gather. Recently, two publicly accessible databases, namely Seagrass TraitDB (De los Santos et al. 2022) and a peer-reviewed paper focused on seagrass meadow structural traits (Strydom et al. 2023), have emerged. Seagrass TraitDB aims to integrate global empirical data for seagrass traits and is in a data-collection stage. Strydom et al. (2023) used a literature-based approach to gather information from global seagrass research to provide 11 traits at the genus level. Collecting traits at a genus level is useful for understanding general patterns or trends within a group of related species, but does not support finer-grained analysis of characteristics specific to individual organisms, including mechanisms that are crucial to resilience, such as reproductive success.
The objective of our study was to establish a robust knowledge base for the application of trait-based methods to tropical seagrass species in Queensland through the development of a functional-traits database. To accomplish this, our approach involved several key steps. First, we identified a comprehensive set of seagrass traits that have the potential to influence seagrass recovery, resistance, and ecosystem functioning. Subsequently, we used a combination of literature, database and botanical information searches and structured expert elicitation to collect data on the values of the identified traits. Expert elicitation allowed us to overcome gaps in published information by collecting knowledge systematically and transparently from experts (Martin et al. 2012; Hemming et al. 2018a). Through appropriate question design, the results obtained from expert elicitation can provide quantitative estimations with associated uncertainty (Adams-Hosking et al. 2016). It is common practice to supplement information when empirical published data are unavailable, as has been seen in fields such as conservation (Gallagher et al. 2021) and ecological modelling (James et al. 2010; Kuhnert et al. 2010). In our study, this approach allowed us to complement the information gathered from the literature search and further enhance our understanding of seagrass traits in a comprehensive and robust manner. Finally, we collaborated with existing platforms to make this set of seagrass traits readily available for further scientific research and management. By establishing this solid foundation of seagrass traits, we have facilitated future advancements in understanding and managing tropical seagrass ecosystems using trait-based methods.
Methods
The study extent covered the tropical Queensland coastline (Fig. 1), starting in the south at Gladstone, Queensland, Australia (23°50′32″S, 151°17′19″E), and extending to the western end at Burketown, Queensland, Australia (16°32′11″S, 138°00′12″E). We focused on seagrass species in shallow meadows because of limited information for deep-water meadows (deeper than 10–15 m). We included the following 13 well-defined seagrass species occurring in tropical Queensland: Cymodocea rotundata (Ascherson & Schweinfurth, 1870), Oceana serrulata (Byng & Christenh, 2018), Enhalus acoroides (Royle, 1839), Halophila capricorni (Larkum, 1995), Halophila decipiens (Ostenfeld, 1902), Halophila ovalis (Hooker f., 1858), Halophila spinulosa (Ascherson, 1875), Halophila tricostata (M.Greenway, 1979), Halodule uninervis (Ascherson 1882), Syringodium isoetifolium (Dandy, 1939), Thalassodendron ciliatum (Hartog 1970), Thalassia hemprichii (Ascherson, 1871), and Zostera muelleri subsp. Capricorni (S.W.L.Jacobs, 2006). Regarding species complexes, Halophila ovata, Halophila minor, and Halophila colesi/australis are grouped into Halophila ovalis because of their morphological similarities, often making them indistinguishable in the field surveys. Similarly, Halodule pinifolia is included in Halodule uninervis for the same reason (Carter et al. 2021a).
To maximise the usability of our tropical Queensland seagrass database, we follow the guidelines of creating a generic plant-trait database (Kattge et al. 2011). We developed a comprehensive list of targeted traits on the basis of their relevance to seagrass recovery, resistance, and ecosystem services, drawing insights from previous studies (Larkum et al. 2018; O’Brien et al. 2018; Johnson et al. 2021). This selection was guided by our research focus on understanding the dynamics of seagrass meadows in this region, their conservation purpose, and the value of the seagrass meadows through their ecosystem services. Seventeen traits were chosen and their definitions along with their relevance to recovery, resistance, or ecosystem services are detailed in Table 1. Additionally, these traits are cross-referenced with full trait names and TraitID in the TRY Plant Trait Database whenever applicable. In this format, our database facilitates accessibility for other researchers and mitigates the issue of unstandardised plant traits. A few traits were considered important in the seagrass ecology discipline but not included in the TRY Plant Trait Database; so, they were included in this project without providing the TraitID. Recognising that traits can exhibit variations within a single species owing to factors such as habitat condition, seasonality, or genetics (Albert et al. 2011; Bolnick et al. 2011), supplementary information associated with the data collection (e.g. date of collection, measurement method, and coordinates of the location site) is required to allow further examination of the traits. We reported the biotic and abiotic content of the collected trait when applicable.
Trait name | TraitID | Full trait name | Unit | Definition | Rationale for relevance to resilience and ecosystem services | |
---|---|---|---|---|---|---|
Leaf width | 145 | Leaf width | mm | Measurement of the widest portion of the leaf | Wider leaves are subject to higher drag force created by waves, but provide stronger wave-attenuation ability than do thinner leaves (van Rooijen et al. 2018; Twomey et al. 2020) | |
Leaf length | 144 | Leaf length | mm | Total length of the leaf | Leaf length affects the leaf length to width ratio, which can further affect the wave-attenuation ability and structure complexity of the meadow (Twomey et al. 2020; Jones et al. 2021) | |
Leaf lifespan | 12 | Leaf lifespan (longevity) | Day | The number of days that a leaf stays on a plant | Leaf lifespan is a leaf economic property and affects the local asexual recovery ability. Shorter leaf lifespan generally indicates faster growing (O’Brien et al. 2018) | |
Leaf nitrogen content per leaf dry mass | 660 | Leaf nitrogen (N) content organic per leaf dry mass | mg per dry mass | The nitrogen content in the leaf and it is expressed as percentage of leaf dry weight | Dugong and sea turtles might target seagrass with high nutrients to improve the efficiency of feeding (Tol et al. 2016) | |
Rhizome diameter | – | – | mm | On a cross section of a rhizome, the length of a segment from one end of the rhizome to another end through the centre | Rhizomes act as anchor for seagrass. Wide rhizomes provide stronger resistant force to drag force created by waves than do thin rhizomes. A meadow that contains large size seagrass tends to attract herbivores (Tol et al. 2016) | |
Rhizome density | – | – | Count per m2 | The number of rhizomes per square metre | Dense rhizomes can reduce resuspension and increase the blue carbon storage of a meadow (Gacia and Duarte 2001) | |
Rhizome elongation rate | – | – | mm per week | The horizontal elongation length of rhizomes per week | Indicates local asexual recovery ability. After disturbance, surviving ramets could form large genets again with clonal growth. (O’Brien et al. 2018) | |
Shoot weight | 403 | Plant biomass and allometry: Shoot dry mass (plant aboveground dry mass) per plant | mg | The dry weight of a group of leaves that grow from one single vertical stem plus the dry weight of that vertical stem | Physical size. Wide rhizomes provide stronger resistance to drag forces created by waves than do thin rhizomes | |
Shoot density | – | – | Count per m2 | The number of shoots (leaves based on one single vertical stem) per square metre | A dense meadow has the ability to create strong wave attenuation. Density can also affect meadow structure and habitat availability (Koch et al. 2006; Twomey et al. 2020) | |
Low light tolerance | 788 | Species light requirement | Day | The time required to no shoots surviving under completed shaded condition | The days that seagrass can survive under 100% light shading condition. Excessive suspension of particles owing to weather events, coastal development, and dredging programs can cause deficient light for seagrass meadow in natural environment (Collier et al. 2016) | |
Canopy height | 773 | Crown (canopy) height | mm | The vertical length of the aboveground plant part | A high canopy is subject to high drag forces created by waves but provides stronger wave attenuation ability than do thinner leaves (van Rooijen et al. 2018; Twomey et al. 2020) | |
Seedbank | 33/2809 | Seed (seedbank) longevity/seedbank duration | Unitless | Presence or absence of the seedbank in the wild | Seedbanks can affect the potential of local recovery of a seagrass meadow after disturbance (Larkum et al. 2018; Jarvis et al. 2021) | |
Seed dormancy | 1109 | Seed viability | Unitless | The ability of seed staying viable after 3 months | Supporting local sexual recovery. After a local population is reduced or has died out, seeds can germinate and perform a fast recovery depending on environmental conditions. (Larkum et al. 2018; Jarvis et al. 2021) | |
Dispersal potential of sexual diaspores | 1263 | Dispersal unit floating capacity | Unitless | The grade of dispersal potential of dispersal unity; it is a combination of floating ability and viability | Buoyancy of most important sexual diaspores indicate the sexual and external recovery ability of a species. Buoyancy contributes only partly to dispersal because environmental factors, such as current and wind, should also be considered (Larkum et al. 2006) | |
Total biomass | – | – | mg per m2 | The dry weight of the whole plant, which includes both above and belowground parts | High biomass of a meadow is an indication of high resistance because heavier species will be more resistant to drag force. High-biomass meadows are positively related to ecosystem services because they may attract more dugong and turtle than do low biomass meadows (Lavery et al. 2013; Tol et al. 2016; Jinks et al. 2019; Jones et al. 2021) | |
Aboveground biomass | 3446 | Plant biomass and allometry: whole plant aboveground dry mass | mg per m2 | The dry weight of the aboveground parts of the plant | Beside the effects of biomass discussed above, the aboveground biomass is especially important to estimate available habitat and wave attenuation | |
Belowground biomass | 2555 | Plant biomass and allometry: belowground plant organ dry mass per plant | mg per m2 | The dry weight of the belowground parts of the plant | Beside the effects of biomass discussed above, the belowground biomass is especially important to estimate nutrient storage thus recovery rate after disturbance |
TraitID and Full trait name are sourced from ‘TRY Plant Trait Database’ (https://www.try-db.org/TryWeb/Home.php; Kattge et al. 2020), if applicable. Units and definitions are supplied.
We reviewed three types of existing seagrass-trait resources (published literature, trait database, and botanical information). First, a systematic literature search was performed using Scopus (https://www.scopus.com) and Web of Science (https://www.webofscience.com) to retrieve relevant articles published between 1980 and 2021. The search used a combination of keywords, namely ‘each seagrass species name’, ‘resistance’, ‘recovery’, ‘resilience’, ‘functioning’, and ‘each trait name’ (as listed in Table 1). The articles from the two databases were merged and duplicates removed. An initial screening was performed by assessing the title and abstract to remove studies associated with species not included in our study. Further screening was then conducted to categorise studies on the basis of their geographic region and measurement method. Priority was given to studies or measurements conducted in tropical Queensland, emphasising their inclusion in the database. Additionally, preference was given to data that recoded trait variation, such as seasonal fluctuations or variations in water depth, because these are valuable for capturing the breadth of seagrass traits. Second, the following trait databases were searched for relevant information: TRY Plant Trait Database (Kattge et al. 2020), Austraits (Falster et al. 2021), and Seagrass TraitDB (De los Santos et al. 2022). Trait data were extracted following the protocol of each database. Finally, we sourced botanical information from Flora of Australia (2024). There could be a potential bias associated with missing seagrass studies that are not reported in English. However, the area of interest for this study was tropical Queensland and all the published studies in Australia are reported in English.
An expert elicitation process was employed to gather information on functional traits and meadow properties that were not documented in existing literature. We used the IDEA protocol method developed by Hemming et al. (2018a), which comprises the following four stages: ‘investigate,’ ‘discussion,’ ‘estimate,’ and ‘aggregate’. The expert elicitation was conducted under the approval from the JCU Human Research Ethics Committee (H8879). The experts were identified as any person that had sufficient field-work experience of seagrasses in tropical Queensland. All experts were provided with an information sheet, and participation was completely voluntary (Supplementary File S1). The participants responded to the survey anonymously, but a unique ID was recorded to trace multiple responses from one person. Two general background questions were included in the survey, including, what sector the experts work, and their experience on seagrass.
To address the challenges of low response rate for particular species and the lengthy survey duration, we made slight modifications to the IDEA protocol in our study. The ‘investigate’ stage was conducted online by using an online survey platform SurveyMonkey (Momentive Inc., https://www.surveymonkey.com/). To make sure the experts share the same understanding of the purpose of the survey, we presented a detailed introduction with survey location maps, brief seagrass species information with figures, and a trait definition for each question (Fig. S1). In total, 28 trait/meadow properties from 13 species were included in the survey. Every question followed the four-step question format (Speirs-Bridge et al. 2010), which asked the participant to answer the highest plausible, lowest plausible, best guess, and confidence level. This question setup is intended to mitigate overconfidence in estimation, and confidence levels were applied to standardise material in the discussion section (Hemming et al. 2018b). The survey period spanned from 17 October 2022 to 10 March 2023. The experts were asked to base their answer on meadows they encountered along the tropical Queensland coast, from Gladstone to Burketown.
All expert responses were standardised using the formula outlined in the IDEA protocol (Hemming et al. 2018a). The standardised and anonymous results were presented in figures and shared with the participants in the ‘discussion’ phase to collect their feedback regarding the group average (Supplementary Table S1, File S3). Because of a high consensus among experts at the ‘discussion’ phase, the ‘estimate’ stage, which involved a second-round survey, was omitted. The ‘aggregate’ stage adhered to the instructions of the IDEA protocol and generated the final results.
Results
Table 2 presents the trait values obtained from a comprehensive review of the literature, trait databases, and botanical information. In total, 629 articles were initially retrieved from literature databases, and subsequent filters were applied to exclude studies conducted outside tropical regions, as well as those focused on seagrass-related organisms such as adhesive algae and bacterial communities. This initial filtering resulted in a final set of 59 articles. From these articles, 16 were selected for inclusion on the basis of the availability of trait measurements. In addition to peer-reviewed articles, trait data from trait databases along with botanical information were also included. Overall, 151 trait values were collected, with 39 sourced from botanical information and 117 from literature. Five trait values were derived from dual data sources to provide a value range. The Austraits database contained the leaf width and length values that are sourced from the Flora of Australia, as cited in Table 2. The ideal database comprises species trait values accompanied by measures of variation such as standard error and quantile. Nevertheless, owing to variable data quality, less preferred data such as genus-level data were included with additional annotation (Table 2).
Trait | CR | OS | EA | HC | HD | HO | HS | HT | HU | SI | TC | TH | ZC | |
---|---|---|---|---|---|---|---|---|---|---|---|---|---|---|
Leaf width (mm) | 2.0–4.0B | 4.0–9.0B | 12.5–15.0B | 5.0–9.0B | 2.5–6.5B | 5.0–15.0B | 1.5–3.5B | 2.0–4.0B | 0.5–4.0B | 0.5–1.5B | 6.0–12.0B | 4.0–10.0B | 1.5–4.5B | |
Leaf length (mm) | 70.0–150.0B | 60.0–200.0B | 300.0–1500.0B | 15.0–30.0B | 10.0–25.0B | 10.0–45.0B | 5.0–20.0B | 12.0–20.0B | 50.0–150.0B | 300.0 (max)B | 100.0–150.0B | 100.0–400.0B | 50.0–400.0B | |
Leaf lifespan (days) | – | – | – | – | 8.6C | 12.4C | 74.0C | – | – | 52.0C | – | – | 56.0C | |
Leaf nitrogen content per leaf dry mass (mg per dry mass) | – | – | – | – | – | – | – | – | – | – | – | – | – | |
Rhizome diameter (mm) | 2.0B | 2.0–3.0B | 15.0 (max)B,C | 0.9–1.5B | 0.8–1.0B,C | 1.0–2.0B | 1.0–1.5B | 1.0B,C | 1.0–2.0B | 1–2.5B | 5.0–6.0B,C | 4.0–5.0B,C | 0.5–2.5B | |
Rhizome density (count per m2) | – | – | – | – | – | – | – | – | – | – | – | – | – | |
Rhizome elongation rate (mm per week) | 411.0C | 216.0C | 0.9C | – | 215.0C | 574.0C | – | – | 136.5C | 75.0C | – | 87.6C | – | |
Shoot weight (mg) | 23.4C | 38.8C | 436C | – | 2.3C | 0.9C | – | – | 9.4C | 14.5C | 605C | 73C | 91.3C | |
Shoot density (count per m2) | 306.25–529.0D | 250.0E | – | – | – | 58.4–780.0D,F | 215.0–316.0E | – | 564.0–3922.0D,E | 333.0–1561.0D | – | 12.0–2182.4F | 565.0–752.0D | |
Low-light tolerance (days) | – | – | – | – | – | 30.0–38.0G,H | – | – | 100.0–119.0G,I | – | – | 133.0I | 76.0I | |
Canopy height (mm) | – | 86.0E | – | – | – | 20.0E | 32.0–40.0E | – | 81.0–85.0E | – | – | – | 66.0–250.0E | |
Seedbank (unitless)A | TransientJ | TransientJ | NoJ | PersistentJ | PersistentJ | PersistentJ | PersistentJ | PersistentJ | PersistentJ | PersistentJ | NoJ | NoJ | TransientJ | |
Seed dormancy (unitless)A | DistinctJ | DistinctJ | NoJ | DistinctJ | DistinctJ | DistinctJ | DistinctJ | DistinctJ | DistinctJ | DistinctJ | Not distinctJ | NoJ | DistinctJ | |
Dispersal potential of sexual diaspores (unitless)A | PoorK | PoorK | GoodK | Moderate/poorK | Moderate/poorK | Moderate/poorK | Moderate/poorK | Moderate/poorK | PoorK | PoorK | GoodK | GoodK | Good/moderateK | |
Total biomass (mg per m2) | 192.0L | 101.0L | 1019.0L | – | 6.0L | 19.0L | 63.0L | 6.0L | 70.0L | 62.0L | – | 349.0L | 133.0L | |
Aboveground biomass (mg per m2) | 49.92L | 26.26L | 132.47L | – | 3.24L | 6.84L | 27.09L | 3.96L | 11.2L | 16.74L | – | 41.88L | 33.25L | |
Belowground biomass (mg per m2) | 142.08L | 74.74L | 866.53L | – | 2.76L | 12.16L | 35.91L | 2.04L | 58.8L | 45.26L | – | 307.12L | 99.75L |
The detailed geographic range of each reference, sampling location and species information are included in Supplementary File S3 and Table S2.
CR, Cymodocea rotundata; OS, Oceana serrulata; EA, Enhalus acoroides; HC, Halophila capricorni; HD, Halophila decipiens; HO, Halophila ovalis; HS, Halophila spinulosa; HT, Halophila tricostata; HU, Halodule uninervis; SI, Syringodium isoetifolium; TC, Thalassodendron ciliatum; TH, Thalassia hemprichii; ZC, Zostera muelleri subsp. Capricorni; –, value is not available in any of the sources.
Reference sources: BFlora of Australia (2024), CDuarte (1991), DRasheed (2000), EJinks et al. (2019), FSaunders et al. (2015), GLongstaff and Dennison (1999), HLongstaff and Loneragan (1999), ICollier et al. (2011), JLarkum et al. (2018), KLarkum et al. (2006), LCollier et al. (2021).
In total, 16 experts were invited to participate in the survey, and we gathered five responses, all from researchers in academic institutions, with experience ranging from 5 to >20 years. Of the 28 questions asked, 23 questions received multiple responses, varying from two to five individual responses. The group-aggregated averages, derived from these responses, are presented in Table 3. On reviewing the group-aggregated average values (File S2) at the ‘discussion’ phase, the experts generally agreed that most of the elicited values were reasonable.
Species | CR | OS | EA | HC | HD | HO | HS | HT | HU | SI | TC | TH | ZC | |
---|---|---|---|---|---|---|---|---|---|---|---|---|---|---|
Canopy height (mm) | 210 | – | 550 | NE | 19.33 | 26.67 | 156.67 | 122.5 | 200 | 190 | 825 | 176.67 | 250 | |
Low-light tolerance (days) | 20.6 | 65 | 38.5 | NE | 13.33 | – | 16.33 | 12.5 | – | 25 | 35 | 28.33 | 22.67 | |
Rhizome elongation rate (mm per week) | – | – | – | NE | – | – | 66.67 | NE | – | – | NE | – | 16.67 |
This table includes only the 23 questions that received more than two responses (Table S1).
CR, Cymodocea rotundata; OS, Oceana serrulata; EA, Enhalus acoroides; HC, Halophila capricorni; HD, Halophila decipiens; HO, Halophila ovalis; HS, Halophila spinulosa; HT, Halophila tricostata; HU, Halodule uninervis; SI, Syringodium isoetifolium; TC, Thalassodendron ciliatum; TH, Thalassia hemprichii; ZC, Zostera muelleri subsp. Capricorni; –, not included in the expert survey; NE, not more than two responses from expert.
Discussion
Our project aims to establish a robust foundation for a trait-based approach to seagrass resilience and services research in tropical Queensland. In pursuit of this goal, we focused on 17 functional traits associated with recovery, resistance, and ecosystem services for 13 seagrass species in tropical Queensland. Of 221 traits, data were collected for 78% (174 traits values). Among the compiled traits, 23 trait values (13%) were obtained from structured expert elicitation, demonstrating the importance of expert knowledge as a source for ecological or conservation studies (Martin et al. 2012; Mallen-Cooper et al. 2020). By publishing, our results can assist future seagrass trait-based research in tropical Queensland and, potentially, regional comparison projects, given that the suite of species is common to much of the tropical Indo Pacific region. The missing 22% of traits also indicate important knowledge gaps and areas for further research and data collection on seagrass traits.
The collated trait values obtained from our study reflect the diversity of morphological and life-history strategies of seagrass species in tropical Queensland. For example, morphologically, the canopy height of E. acorodies is 28 times greater than that of H. decipiens. The life-history trait of low-light tolerance ranged from 13 days for H. decipiens to 133 days for T. hemprichii. This diversity of traits observed across species is an indication of the wide array of ecosystem function and services provided by seagrass meadows in tropical Queensland (Mason et al. 2005; Moreira-Saporiti et al. 2023). For instance, structural diversity, such as aboveground structure, plays important roles for supporting diverse fish assemblages, (Jinks et al. 2019; Jones et al. 2021). Additionally, biomass influences the foraging behaviour of dugongs (Tol et al. 2016) and determines the blue carbon storage capabilities of meadows (Lavery et al. 2013; Johnson et al. 2017). Furthermore, some species exhibit similar values in certain traits; for instance, H. uninervis, S. isoetifolium, and H. spinulosa all have biomass within the range of 60–70 mg/m2. Trait redundancy indicates the resilience of this ecosystem because, even though species composition might alter because of interference, the overall functional diversity might stay the same if multiple species play a similar functional role in the community (Laliberte et al. 2010). In the case of tropical seagrass, the similar biomass of H. uninervis, S. isoetifolium, and H. spinulosa could stabilise the function provided by the meadow, even if one species decreases in number, because other species might take over the space and bring the meadow to a new state with similar functioning.
Despite our integration of the literature, trait databases, botanical information and expert elicitation, some species or traits still have limited information. Of the 13 species in this study, H. capricorni, H. decipiens, and H. tricostata had the least information available in the literature. Furthermore, in the expert elicitation, H. capricorni did not receive more than two expert responses for any of the questions. This species-specific lack of data is likely a result of the low abundance of these species in tropical Queensland (Waycott et al. 2004). Traits that can be measured by a single individual morphological property, such as leaf dimension or rhizome features, are more commonly documented in existing data and are typically easier for experts to respond to. In contrast, traits that involve biotic or abiotic factors, such as herbivory pressure, often have less available information in the literature or the responses from experts are more inconsistent (Table S1). For instance, the nutrient content of leaves presents challenges in data collection, with only one published study reporting this value, and the raw data being inaccessible, leaving only average values available for reference (Duarte 1990). Experts reported a lack of confidence in providing information on nutrient content. The lack of information reflects the further need for research into seagrass traits associated with nutrient content, low-light tolerance, and seed dormancy. Additionally, traits that influence interactions between herbivores and seagrasses are under-represented in the literature. These traits often required long-term measurements, such as, shoot formation rate and leaf elongation rate, or specialised tools, (e.g. leaf toughness; De los Santos et al. 2016), which limits available data. However, some of difficult-to-measure traits (hard traits) can be inferred from easier-to-measure traits (soft traits) (Hodgson et al. 1999). This approach, employed in previous research, showed that certain hard traits exhibit strong correlation with the other soft traits and this link offers a pathway to reduce the effort required for collecting hard traits, particularly physiological traits such as nutrient content and air-exposure tolerance (Belluau and Shipley 2018). We encourage future research to include a broader range of trait measurements and explore the relationship between soft and hard traits.
Our database has the potential to inform seagrass trait-based studies in other tropical regions that share the same species. For example, our database provides essential structural trait information for assessing the value of seagrass meadows in supporting fisheries production (Jones et al. 2021) or estimating coastal protection capacity (Christianen et al. 2013). When integrated with other databases, our local database provides useful information for broader-scale regional comparisons (Vandewalle et al. 2010). For example, assessing the impact of land-use changes on functional diversity across eco-regions or exploring functional diversity in habitats with size and connectivity variation worldwide (Jacquet et al. 2017). Future research endeavours should aim to explore causal relationships between functional traits and ecosystem dynamics. For instance, by quantifying recovery and resistance abilities of seagrass meadows on the basis of functional-trait values and meadow composition, we can elucidate meadow dynamics in a trait-based context. However, it is also important to recognise the limitation of a trait-based approach, particularly when interaction between organisms and the environment are driven by location-specific factors, such as epiphyte abundance (Balata et al. 2007). In cases where epiphytes contribute significantly to meadow recovery, traits alone may not fully explain resilience, and other methods may be required.
Various studies have demonstrated the importance of incorporating intraspecific trait variation in plant-trait studies (Bolnick et al. 2011; Westerband et al. 2021). Plants have the potential to vary in their traits according to environmental conditions or genetic composition, which can change the performance of the plant or affect the habitat it forms (Hughes 2014). In some cases, our database reported traits from literature that publish only a single value for each trait, such as rhizome elongation rate and shoot weight. This limitation has the potential to restrict the application of our database. To improve trait-data quality and its broader application in research, we encourage researchers to publish raw data or at least provide more comprehensive details when publishing trait information, such as minimum and maximum values, sample date, and sample size. One highly comprehensive approach for raw data is using the free and open-source platform, Open Science Framework (OSF), which encompasses data management plans, version control, and data sharing (Sullivan et al. 2019). It is also beneficial to condense raw data in a more compact format and provide it to other types of repositories that are available under an open Creative Commons licence, such as eAtlas in Queensland (https://eatlas.org.au/home) and PANGAEA (Felden et al. 2023).
Conclusions
Our project has provided a fundamental resource for implementing a trait-based approach for seagrass studies within tropical Queensland and other tropical areas that share similar species assemblages. Our primary focus was to understand seagrass resilience and the provision of ecosystem services, with the careful selection of 17 traits on the basis of their relevance to these processes. The innovative mixed-method approach we employed involved creating a plant-trait database by amalgamating literature, databases, botanical information and expert elicitation. This method allowed us to collect 78% of our targeted traits, affirming the cost-effectiveness of gathering trait information from experts when it is unavailable for specific species or traits in a published form. The uneven distribution of collected traits among species highlights knowledge gaps, underscoring the significance of further empirical data collection targeted to traits. Moreover, although our database is designed for tropical Queensland, it also serves as a valuable source for broader geographic-scale studies.
Data availability
The data collected in this study are available in the article and online supplementary material.
Declaration of funding
This study is supported by the Australian Research Council Centre of Excellence for Coral Reef Studies, Australian Research Council Linkage Projects (LP 210300851) and the Australian Government Research Training Program Scholarship.
Acknowledgements
We extend our sincere gratitude to all the participants in the expert elicitation survey for generously sharing their invaluable expertise on tropical seagrasses.
References
Adams-Hosking C, McBride MF, Baxter G, Burgman M, de Villiers D, Kavanagh R, Lawler I, Lunney D, Melzer A, Menkhorst P, Molsher R, Moore BD, Phalen D, Rhodes JR, Todd C, Whisson D, McAlpine CA (2016) Use of expert knowledge to elicit population trends for the koala (Phascolarctos cinereus). Diversity and Distributions 22, 249-262.
| Crossref | Google Scholar |
Albert CH, Grassein F, Schurr FM, Vieilledent G, Violle C (2011) When and how should intraspecific variability be considered in trait-based plant ecology? Perspectives in Plant Ecology, Evolution and Systematics 13, 217-225.
| Crossref | Google Scholar |
Balata D, Nesti U, Piazzi L, Cinelli F (2007) Patterns of spatial variability of seagrass epiphytes in the north-west mediterranean sea. Marine Biology 151, 2025-2035.
| Crossref | Google Scholar |
Belluau M, Shipley B (2018) Linking hard and soft traits: physiology, morphology and anatomy interact to determine habitat affinities to soil water availability in herbaceous dicots. PLoS ONE 13, e0193130.
| Crossref | Google Scholar | PubMed |
Bolnick DI, Amarasekare P, Araújo MS, Bürger R, Levine JM, Novak M, Rudolf VHW, Schreiber SJ, Urban MC, Vasseur DA (2011) Why intraspecific trait variation matters in community ecology. Trends in Ecology and Evolution 26, 183-192.
| Crossref | Google Scholar | PubMed |
Butt N, Gallagher R (2018) Using species traits to guide conservation actions under climate change. Climatic Change 151, 317-332.
| Crossref | Google Scholar |
Carter AB, Mckenna SA, Rasheed MA, Collier C, McKenzie L, Pitcher R, Coles R (2021a) Synthesizing 35 years of seagrass spatial data from the Great Barrier Reef World Heritage Area, Queensland, Australia. Limnology and Oceanography Letters 6, 216-226.
| Crossref | Google Scholar |
Carter AB, Collier C, Lawrence E, Rasheed MA, Robson BJ, Coles R (2021b) A spatial analysis of seagrass habitat and community diversity in the Great Barrier Reef World Heritage Area. Scientific Reports 11, 22344.
| Crossref | Google Scholar |
Carter A, McKenna S, Rasheed MA, Taylor H, van de Wetering C, Chartrand K, et al. (2024) Seagrass spatial data synthesis from north-east Australia, Torres Strait and Gulf of Carpentaria, 1983 to 2022. Limnology and Oceanography Letters 9(1), 7-22.
| Crossref | Google Scholar |
Christianen MJA, van Belzen J, Herman PMJ, van Katwijk MM, Lamers LPM, van Leent PJM, Bouma TJ (2013) Low-canopy seagrass beds still provide important coastal protection services. PLoS One 8, e62413.
| Crossref | Google Scholar | PubMed |
Coles RG, Rasheed MA, McKenzie LJ, Grech A, York PH, Sheaves M, McKenna S, Bryant C (2015) The Great Barrier Reef World Heritage area seagrasses: managing this iconic Australian ecosystem resource for the future. Estuarine, Coastal and Shelf Science 153, A1-A12.
| Crossref | Google Scholar |
Collier CJ, Uthicke S, Waycott M (2011) Thermal tolerance of two seagrass species at contrasting light levels: implications for future distribution in the Great Barrier Reef. Limnology and Oceanography 56(6), 2200-2210.
| Crossref | Google Scholar |
Collier CJ, Adams MP, Langlois L, Waycott M, O’Brien KR, Maxwell PS, McKenzie L (2016) Thresholds for morphological response to light reduction for four tropical seagrass species. Ecological Indicators 67, 358-366.
| Crossref | Google Scholar |
Collier CJ, Langlois LM, McMahon KM, Udy J, Rasheed M, Lawrence E, et al. (2021) What lies beneath: predicting seagrass below-ground biomass from above-ground biomass, environmental conditions and seagrass community composition. Ecological Indicators 121, 107156.
| Crossref | Google Scholar |
De Battisti D (2021) The resilience of coastal ecosystems: a functional trait-based perspective. Journal of Ecology 109, 3133-3146.
| Crossref | Google Scholar |
De los Santos C, Onoda Y, Vergara J, Pérez-Lloréns J, Bouma T, La Nafie Y, Cambridge M, Brun F (2016) A comprehensive analysis of mechanical and morphological traits in temperate and tropical seagrass species. Marine Ecology Progress Series 551, 81-94.
| Crossref | Google Scholar |
De los Santos CB, Machado J, De Moro G, Cox C, Santos R (2022) Seagrass TraitDB: global data base of seagrass traits. Available at http://seagrasses.ccmar.ualg.pt
Duarte CM (1990) Seagrass nutrient content. Marine Ecology Progress Series 67, 201-207.
| Crossref | Google Scholar |
Duarte CM (1991) Allometric scaling of seagrass form and productivity. Marine Ecology Progress Series 77, 289-300.
| Crossref | Google Scholar |
Dunic JC, Brown CJ, Connolly RM, Turschwell MP, Côté IM (2021) Long-term declines and recovery of meadow area across the world’s seagrass bioregions. Global Change Biology 27, 4096-4109.
| Crossref | Google Scholar | PubMed |
Falster D, Gallagher R, Wenk E, Wright IJ, Indiarto D, Andrew SC, Baxter C, Lawson J, Allen S, Fuchs A, Monro A, Kar F, Adams MA, Ahrens CW, Alfonzetti M, Angevin T, Apgaua DMG, Arndt S, Atkin OK, Atkinson J, Auld T, Baker A, von Balthazar M, Bean A, Blackman CJ, et al. (2021) AusTraits, a curated plant trait database for the Australian flora. Scientific Data 8, 254.
| Crossref | Google Scholar |
Felden J, Möller L, Schindler U, Huber R, Schumacher S, Koppe R, Diepenbroek M, Glöckner FO (2023) PANGAEA: Data Publisher for Earth & Environmental Science. Scientific Data 10, 347.
| Crossref | Google Scholar | PubMed |
Flora of Australia (2024) Australian biological resources study. Canberra. Available at http://www.ausflora.org.au [Viewed 10 February 2024].
Fonseca MS, Cahalan JA (1992) A preliminary evaluation of wave attenuation by four species of seagrass. Estuarine, Coastal and Shelf Science 35, 565-576.
| Crossref | Google Scholar |
Funk JL, Larson JE, Ames GM, Butterfield BJ, Cavender-Bares J, Firn J, Laughlin DC, Sutton-Grier AE, Williams L, Wright J (2017) Revisiting the Holy Grail: using plant functional traits to understand ecological processes. Biological Reviews 92, 1156-1173.
| Crossref | Google Scholar | PubMed |
Gacia E, Duarte CM (2001) Sediment retention by a mediterranean Posidonia oceanica meadow: the balance between deposition and resuspension. Estuarine, Coastal and Shelf Science 52, 505-514.
| Crossref | Google Scholar |
Gallagher RV, Butt N, Carthey AJR, Tulloch A, Bland L, Clulow S, Newsome T, Dudaniec RY, Adams VM (2021) A guide to using species trait data in conservation. One Earth 4, 927-936.
| Crossref | Google Scholar |
Grootemaat S, Wright IJ, Van Bodegom PM, Cornelissen JHC, Shaw V (2017) Bark traits, decomposition and flammability of Australian forest trees. Australian Journal of Botany 65, 327-338.
| Crossref | Google Scholar |
Hemming V, Burgman MA, Hanea AM, McBride MF, Wintle BC (2018a) A practical guide to structured expert elicitation using the IDEA protocol. Methods in Ecology and Evolution 9, 169-180.
| Crossref | Google Scholar |
Hemming V, Walshe TV, Hanea AM, Fidler F, Burgman MA (2018b) Eliciting improved quantitative judgements using the IDEA protocol: a case study in natural resource management. PLoS ONE 13, e0198468.
| Crossref | Google Scholar | PubMed |
Hodgson JG, Wilson PJ, Hunt R, Grime JP, Thompson K (1999) Allocating C-S-R plant functional types: a soft approach to a hard problem. Oikos 85, 282.
| Crossref | Google Scholar |
Hughes AR (2014) Genotypic diversity and trait variance interact to affect marsh plant performance. Journal of Ecology 102, 651-658.
| Crossref | Google Scholar |
Jacquet C, Mouillot D, Kulbicki M, Gravel D (2017) Extensions of Island Biogeography Theory predict the scaling of functional trait composition with habitat area and isolation. Ecology Letters 20, 135-146.
| Crossref | Google Scholar | PubMed |
James Cook University (2022) Turtles, dugongs in danger from seagrass loss. James Cook University media release. Available at https://www.jcu.edu.au/news/releases/2022/may/turtles-dugongs-in-danger-from-seagrass-loss
James A, Choy SL, Mengersen K (2010) Elicitator: an expert elicitation tool for regression in ecology. Environmental Modelling & Software 25, 129-145.
| Crossref | Google Scholar |
Jarvis JC, McKenna SA, Rahseed MA (2021) Seagrass seed bank spatial structure and function following a large-scale decline. Marine Ecology Progress Series 665, 75-87.
| Crossref | Google Scholar |
Jinks KI, Brown CJ, Rasheed MA, Scott AL, Sheaves M, York PH, Connolly RM (2019) Habitat complexity influences the structure of food webs in Great Barrier Reef seagrass meadows. Ecosphere 10, e02928.
| Crossref | Google Scholar |
Johnson RA, Gulick AG, Bolten AB, Bjorndal KA (2017) Blue carbon stores in tropical seagrass meadows maintained under green turtle grazing. Scientific Reports 7, 13545.
| Crossref | Google Scholar |
Johnson AJ, Shields EC, Kendrick GA, Orth RJ (2021) Recovery dynamics of the seagrass Zostera marina following mass mortalities from two extreme climatic events. Estuaries and Coasts 44, 535-544.
| Crossref | Google Scholar |
Jones BL, Nordlund LM, Unsworth RKF, Jiddawi NS, Eklöf JS (2021) Seagrass structural traits drive fish assemblages in small-scale fisheries. Frontiers in Marine Science 8, 640528.
| Crossref | Google Scholar |
Kattge J, Ogle K, Bönisch G, Díaz S, Lavorel S, Madin J, Nadrowski K, Nöllert S, Sartor K, Wirth C (2011) A generic structure for plant trait databases. Methods in Ecology and Evolution 2, 202-213.
| Crossref | Google Scholar |
Kattge J, Bönisch G, Díaz S, Lavorel S, Prentice IC, Leadley P, Tautenhahn S, Werner GDA, Aakala T, Abedi M, Acosta ATR, Adamidis GC, Adamson K, Aiba M, Albert CH, Alcántara JM, Alcázar CC, Aleixo I, Ali H, et al. (2020) TRY plant trait database – enhanced coverage and open access. Global Change Biology 26, 119-188.
| Crossref | Google Scholar | PubMed |
Kelkar N, Arthur R, Marba N, Alcoverro T (2013) Green turtle herbivory dominates the fate of seagrass primary production in the Lakshadweep islands (Indian Ocean). Marine Ecology Progress Series 485, 235-243.
| Crossref | Google Scholar |
Koch EW, Sanford LP, Chen S-N, Shafer DJ, Smith JM (2006) ‘Waves in Seagrass Systems: Review and Technical Recommendations.’ (Defense Technical Information Center: Washington, DC, USA) doi:10.21236/ADA458760
Kuhnert PM, Martin TG, Griffiths SP (2010) A guide to eliciting and using expert knowledge in Bayesian ecological models. Ecology Letters 13, 900-914.
| Crossref | Google Scholar | PubMed |
Laliberté E, Legendre P (2010) A distance-based framework for measuring functional diversity from multiple traits. Ecology 91, 299-305.
| Crossref | Google Scholar | PubMed |
Laliberte E, Wells JA, DeClerck F, Metcalfe DJ, Catterall CP, Queiroz C, Aubin I, Bonser SP, Ding Y, Fraterrigo JM, McNamara S, Morgan JW, Merlos DS, Vesk PA, Mayfield MM (2010) Land-use intensification reduces functional redundancy and response diversity in plant communities. Ecology Letters 13, 76-86.
| Crossref | Google Scholar | PubMed |
Larkum AWD, Orth RJ, Duarte CM (2006) ‘Seagrasses: biology, ecology, and conservation.’ (Springer: Dordrecht, Netherlands) 10.1007/978-1-4020-2983-7
Larkum AWD, Kendrick GA, Ralph PJ (2018) ‘Seagrasses of Australia: structure, ecology and conservation.’ (Springer International Publishing) doi:10.1007/978-3-319-71354-0
Lavery PS, Mateo M-Á, Serrano O, Rozaimi M (2013) Variability in the Carbon Storage of Seagrass Habitats and Its Implications for Global Estimates of Blue Carbon Ecosystem Service. PLoS ONE 8, e73748.
| Crossref | Google Scholar | PubMed |
LeRoy Poff NL, Olden JD, Vieira NKM, Finn DS, Simmons MP, Kondratieff BC (2006) Functional trait niches of North American lotic insects: traits-based ecological applications in light of phylogenetic relationships. Journal of the North American Benthological Society 25, 730-755.
| Crossref | Google Scholar |
Longstaff BJ, Dennison WC (1999) Seagrass survival during pulsed turbidity events: the effects of light deprivation on the seagrasses Halodule pinifolia and Halophila ovalis. Aquatic Botany 65, 105-121.
| Crossref | Google Scholar |
Longstaff BJ, Loneragan NR, O’donohue MJ, Dennison WC (1999) Effects of light deprivation on the survival and recovery of the seagrass Halophila ovalis (R. Br.) Hook. Journal of Experimental Marine Biology and Ecology 234(1), 1–-27.
| Crossref | Google Scholar |
Mallen-Cooper M, Bowker MA, Antoninka AJ, Eldridge DJ (2020) A practical guide to measuring functional indicators and traits in biocrusts. Restoration Ecology 28, S56-S66.
| Crossref | Google Scholar |
Martin TG, Burgman MA, Fidler F, Kuhnert PM, Low-Choy S, Mcbride M, Mengersen K (2012) Eliciting expert knowledge in conservation science. Conservation Biology 26, 29-38.
| Crossref | Google Scholar | PubMed |
Mason NWH, Mouillot D, Lee WG, Wilson JB (2005) Functional richness, functional evenness and functional divergence: the primary components of functional diversity. Oikos 111, 112-118.
| Crossref | Google Scholar |
McKenna S, Jarvis J, Sankey T, Reason C, Coles R, Rasheed M (2015) Declines of seagrasses in a tropical harbour, North Queensland, Australia, are not the result of a single event. Journal of Biosciences 40, 389-398.
| Crossref | Google Scholar | PubMed |
Moreira-Saporiti A, Teichberg M, Garnier E, Cornelissen JHC, Alcoverro T, Björk M, Boström C, Dattolo E, Eklöf JS, Hasler-Sheetal H, Marbà N, Marín-Guirao L, Meysick L, Olivé I, Reusch TBH, Ruocco M, Silva J, Sousa AI, Procaccini G, Santos R (2023) A trait-based framework for seagrass ecology: trends and prospects. Frontiers in Plant Science 14, 1088643.
| Crossref | Google Scholar | PubMed |
Nash KL, Graham NAJ, Jennings S, Wilson SK, Bellwood DR (2016) Herbivore cross-scale redundancy supports response diversity and promotes coral reef resilience. Journal of Applied Ecology 53, 646-655.
| Crossref | Google Scholar |
Nock CA, Vogt RJ, Beisner BE (2016) Functional traits. In ‘eLS’. pp. 1–8. (John Wiley & Sons: Chichester, UK) doi:10.1002/9780470015902.a0026282
O’Brien KR, Waycott M, Maxwell P, Kendrick GA, Udy JW, Ferguson AJP, Kilminster K, Scanes P, McKenzie LJ, McMahon K, Adams MP, Samper-Villarreal J, Collier C, Lyons M, Mumby PJ, Radke L, Christianen MJA, Dennison WC (2018) Seagrass ecosystem trajectory depends on the relative timescales of resistance, recovery and disturbance. Marine Pollution Bulletin 134, 166-176.
| Crossref | Google Scholar | PubMed |
Preen AR, Lee Long WJ, Coles RG (1995) Flood and cyclone related loss, and partial recovery, of more than 1000 km2 of seagrass in Hervey Bay, Queensland, Australia. Aquatic Botany 52, 3-17.
| Crossref | Google Scholar |
Rasheed MA, McKenna SA, Carter AB, Coles RG (2014) Contrasting recovery of shallow and deep water seagrass communities following climate associated losses in tropical north Queensland, Australia. Marine Pollution Bulletin 83, 491-499.
| Crossref | Google Scholar | PubMed |
Saunders MI, Bayraktarov E, Roelfsema CM, Leon JX, Samper-Villarreal J, Phinn SR, et al. (2015) Spatial and temporal variability of seagrass at Lizard Island, Great Barrier Reef. Botanica Marina 58(1), 35-49.
| Crossref | Google Scholar |
Speirs-Bridge A, Fidler F, McBride M, Flander L, Cumming G, Burgman M (2010) Reducing overconfidence in the interval judgments of experts. Risk Analysis 30, 512-523.
| Crossref | Google Scholar | PubMed |
Streit RP, Bellwood DR (2023) To harness traits for ecology, let’s abandon ‘functionality’. Trends in Ecology & Evolution 38, 402-411.
| Crossref | Google Scholar | PubMed |
Strydom S, McCallum R, Lafratta A, Webster CL, O’Dea CM, Said NE, Dunham N, Inostroza K, Salinas C, Billinghurst S, Phelps CM, Campbell C, Gorham C, Bernasconi R, Frouws AM, Werner A, Vitelli F, Puigcorbé V, D’Cruz A, McMahon KM, Robinson J, Huggett MJ, McNamara S, Hyndes GA, Serrano O (2023) Global dataset on seagrass meadow structure, biomass and production. Earth System Science Data 15, 511-519.
| Crossref | Google Scholar |
Sullivan I, DeHaven A, Mellor D (2019) Open and reproducible research on open science framework. Current Protocols Essential Laboratory Techniques 18, e32.
| Crossref | Google Scholar |
Tol SJ, Coles RG, Congdon BC (2016) Dugong dugon feeding in tropical Australian seagrass meadows: implications for conservation planning. PeerJ 4, e2194.
| Crossref | Google Scholar | PubMed |
Twomey AJ, O’Brien KR, Callaghan DP, Saunders MI (2020) Synthesising wave attenuation for seagrass: drag coefficient as a unifying indicator. Marine Pollution Bulletin 160, 111661.
| Crossref | Google Scholar | PubMed |
van Rooijen A, Lowe R, Ghisalberti M, Conde-Frias M, Tan L (2018) Predicting current-induced drag in emergent and submerged aquatic vegetation canopies. Frontiers in Marine Science 5, 449.
| Crossref | Google Scholar |
Vandewalle M, de Bello F, Berg MP, Bolger T, Dolédec S, Dubs F, Feld CK, Harrington R, Harrison PA, Lavorel S, da Silva PM, Moretti M, Niemelä J, Santos P, Sattler T, Sousa JP, Sykes MT, Vanbergen AJ, Woodcock BA (2010) Functional traits as indicators of biodiversity response to land use changes across ecosystems and organisms. Biodiversity and Conservation 19, 2921-2947.
| Crossref | Google Scholar |
Violle C, Navas M-L, Vile D, Kazakou E, Fortunel C, Hummel I, Garnier E (2007) Let the concept of trait be functional!. Oikos 116, 882-892.
| Crossref | Google Scholar |
Westerband AC, Funk JL, Barton KE (2021) Intraspecific trait variation in plants: a renewed focus on its role in ecological processes. Annals of Botany 127, 397-410.
| Crossref | Google Scholar | PubMed |
Wood SLR, Dupras J (2021) Increasing functional diversity of the urban canopy for climate resilience: Potential tradeoffs with ecosystem services? Urban Forestry and Urban Greening 58, 126972.
| Crossref | Google Scholar |