Review of approaches and challenges for the validation of satellite-based active fire products in savannah ecosystems
Simon Ramsey
A
Abstract
Satellite remote sensing is a critical tool for continental and synoptic monitoring and mapping of savannah wildfires. Satellite active fire products, which report on the time and location of a fire and may further characterise fire by estimating fire radiative power (FRP), provide valuable utility for savannah fire management and carbon accounting. These applications require that satellite measurements are of high accuracy, which can only be determined through validation. However, acquiring reference data for validation that is a representative of the fire conditions at the time of satellite image capture is challenging, due to rapid changes in fire behaviour and the inherent safety considerations of collecting field data during fire events. This review explores traditional and contemporary methods used to assess the accuracy and consistency of fire detections and FRP derived from satellite data in savannah ecosystems, with a focus on the approaches and challenges in collecting suitable reference data for a phenomenon as dynamic, ephemeral, and hazardous as wildfire. From this synthesis, we present generalised frameworks for the validation and intercomparison of satellite active fire products within savannah ecosystems.
Keywords: Active fire, Earth observation, fire detection, fire radiative power, intercomparison, savannah, satellite, validation.
Introduction
Savannahs are the most fire prone terrestrial biome, accounting globally for around 75% of annual ignitions (Andela et al. 2019) and over 70% of burned area (Giglio et al. 2018). Defined broadly as tropical and subtropical grasslands characterised by an open tree canopy and continuous grass understorey, savannahs experience seasonal fires that occur predominantly in the monsoonal dry season. Vast areas of savannah burn annually, whether ignited intentionally for agricultural, pastural or customary management, by arson, by accidental ignitions, or ignited naturally by lightning strikes (Archibald et al. 2009; Cochrane 2009; Beringer et al. 2015). Savannah fires account for around 62% of global biomass burning emissions (Van Der Werf et al. 2017), which has encouraged extensive research into savannah fire management to mitigate greenhouse gas emissions (Russell-Smith et al. 2017).
Demand for accurate, timely and frequent landscape-scale information on savannah fire activity necessitated the adoption of satellite sensors as an objective means to characterise the location, spread and extent of fire events. This is achieved through either the thermal remote sensing of active fires, primarily by targeting the middle infra-red (~4.0 µm; hereafter MIR) and thermal infra-red (~8–12 µm; hereafter TIR) radiation released through the combustion of vegetation and organic matter (Wooster et al. 2021), or mapping burned area by detecting fire induced change between successive satellite images using the decrease in reflectance in near infra-red (~0.8–1.2 µm) and short-wave infra-red (~1.6–2.2 µm) wavelengths characteristic of burned vegetation (Chuvieco et al. 2019). Operational active fire and burned area products have predominantly used information from sensors onboard low earth orbit satellites, such as the Moderate Resolution Imaging Spectroradiometer (MODIS) (Giglio et al. 2016; Giglio et al. 2018), which provide near-global surveillance with intermittent observations (for example, twice per day) depending on the sensor field of view, altitude and inclination. Geostationary satellites are increasingly used to detect and monitor active fires, which while constrained to a particular hemisphere, provide improved temporal sampling allowing more opportunities to observe fire activity and the possibility of earlier first detections (Prins et al. 1998; Roberts and Wooster 2008; Hally et al. 2016). By nature, detecting fires by the thermal radiation emitted can only be achieved while combustion is occurring, allowing information on fire activity to be provided with very low latency times, but with the limitation that more ephemeral fires may go undetected (Wooster et al. 2013). Savannah fires are predominantly surface fires, which spread rapidly relative to the temporal sampling of low earth orbiting satellites (Roy et al. 2010). Low earth orbit satellites may not overpass with the frequency needed to adequately sample fire progression leading to an underestimation of the total area burned. This stands in contrast to the optical sensing approaches used to detect the charcoal signal of burn scars, which require a period of post-fire spectral change, but can provide an improved estimate of the cumulative burned area (Wooster et al. 2013). For applications where accurate estimates of the area affected by fire activity are required, such as the estimation of pyrogenic emissions (Van Der Werf et al. 2017) and fire regimes (Archibald et al. 2010; Chuvieco et al. 2021), burned area products are often more suitable (Pereira 2003). However, the coarse resolution satellites in operational use often fail to detect smaller fires leading to significant omission errors (Randerson et al. 2012; Roteta et al. 2019; Lizundia-Loiola et al. 2020; Ramo et al. 2021). In recent years, considerable progress has been made in estimating pyrogenic emissions from fire radiative power (FRP, units: mW) (Wooster et al. 2005), a remotely sensed measure of the instantaneous energy released from active fires (Ichoku and Kaufman 2005; Kumar et al. 2011; Nguyen and Wooster 2020; Nguyen et al. 2023). Fire radiative energy (FRE), the temporal integral of FRP, is considered linearly proportional to the amount of fuel consumed over the integrated period, allowing biomass burning emissions estimates to be provided in near real time (Kaiser et al. 2012; Mota and Wooster 2018).
The utility of fire products for research and land management is greatly enhanced if accompanied by accuracy estimates to guide appropriate usage, by reporting the likelihood of false alarms, the minimum detection capabilities of a given sensor or algorithm, and the conditions where performance may be degraded. Assessments of active fire product performance are undertaken through two primary means: (1) validation through comparison with independent reference data, which is an acceptable proxy for reality (Justice et al. 2000); or (2) by intercomparing active fire products from different algorithms/sensors (Li et al. 2001). Validation of active fire detections is typically reported through detection probabilities and commission/omission error rates to assess the absolute fire detection capabilities of a given satellite and algorithm. Intercomparing system outputs from different active fire products provides an indication of performance by assessing the consistency and shortcomings between products, while validation is required to determine product accuracy (Justice et al. 2013). The accuracy information obtained through validation is used to identify any potential shortcomings in a product, and guide improvements to the products and underlying algorithms (Justice et al. 2013).
Protocols for the validation of satellite burned area products are well established (Boschetti et al. 2009; Padilla et al. 2017; Franquesa et al. 2022) guiding appropriate reference data acquisition and validation procedures. Assessing the accuracy of active fire detections and FRP retrievals using reference data that is representative of the fire conditions imaged by the satellite surveillance systems inherently more challenging to acquire, due to safety considerations and the highly dynamic nature of wildfire where rapid spread rates and fluctuations in fire intensity severely restricts the interval within which reference data will remain representative (Wooster et al. 2021).
The aim of this paper is to review the methods used to evaluate satellite active fire products as published in the literature, and to identify the requirements for best practice in savannah ecosystems. To achieve this aim, we first review the unique conditions of savannah ecosystems and how this affects active fire product performance and validation procedures. Next, we review and synthesise the approaches used to validate and intercompare satellite-derived fire detections and FRP at a global scale to identify the challenges that are encountered. From this assessment we provide a generalised framework for the validation and intercomparison of satellite active fire products in savannah ecosystems.
Savannah biome characteristics and validation challenges
Savannahs are the most fire prone terrestrial biome and the predominant source of pyrogenic emissions (Van Der Werf et al. 2017), with extensive areas burning annually or biennially (Russell-Smith et al. 2007; Yates et al. 2008; Pricope and Binford 2012; Oliveira et al. 2013; Pereira Júnior et al. 2014). Savannah fire regimes have been significantly altered by human activity, with most fires purpose lit to achieve land management and carbon abatement aims (van Wilgen et al. 2007; Archibald et al. 2010; Murphy and Russell-Smith 2010; Meyer et al. 2012; Archer-Nicholls et al. 2015; Russell-Smith et al. 2017, 2021; Edwards et al. 2021). The broad applications of satellite active fire products for savannah fire management and carbon accounting necessitates that any fire maps or measurements of savannah fire intensity produced from satellite data are of high accuracy with error rates known and reported.
Variations in fire dynamics and patterning are evident across the major savannah regions of Africa, Australia, and South America. These distinctions stem from continental differences in land use and management, population density, and differing degrees of regulation by environmental drivers, including moisture, temperature and soil fertility, which govern fire frequency and tree basal area (Yates et al. 2008; Lehmann et al. 2014). The traits of the vegetation in savannahs strongly influences fire frequency whereby fire is enabled by a high density of grasses that cure rapidly in the absence of overstorey vegetation, which maintains an open canopy, initiating positive feedback between savannah and fire (Van Langevelde et al. 2003; Lehmann et al. 2011). Dry season fire frequency is linked to the drastic shift between the wet and dry seasons, with fire broadly increasing with higher rainfall in the monsoonal wet season that facilitates higher fine fuel production (Russell-Smith et al. 2007; Lehmann et al. 2014; Karp et al. 2023). Savannah fires can spread rapidly under the hot, dry and low humidity conditions typical of the dry season, with rates of spread up to around 2 m s−1 under favourable conditions (Govender et al. 2006). Savannah fire spread is strongly influenced by moisture content, where fires will typically not reach their full spread potential until the late dry season, associated with the progressive curing of grasses and litter accumulation (Yates et al. 2008; Oliveira et al. 2015). Shorter periods of burning and spread have been found to be associated with tree cover (Eva and Lambin 1998; Giglio 2007), where trees maintain a higher level of ambient humidity and restrict fuel combustion. The heterogeneous nature of savannah environments results in patches that dry at different rates (Laris 2005). In an Australian savannah context, this results in a mosaic where a regime of small, fragmented fires in the early dry season reduces the size and intensity of late dry season fires (Russell‑Smith et al. 1997). Conversely, in southern African savannahs, no significant increase in fire size or intensity has been reported between early and late dry season likely driven by higher rural population densities and fire management (Archibald et al. 2010).
Fire ignition in the savannah dry season is physically possible at any time of day with fire activity increasing significantly in the early afternoon (Eva and Lambin 1998; Giglio 2007; Roberts et al. 2009; Andela et al. 2015). A comparison of total fire counts between Terra and Aqua MODIS active fire detections indicated that within the west African savannahs, fire activity increased significantly in the early afternoon during the daily maximum of the FRP diurnal cycle (Csiszar et al. 2005). A significant undercounting of active fire pixels in savannahs has been reported for MODIS-type 1 km sensors, with omissions increasing away from the early afternoon peak of the diurnal fire cycle (Sperling et al. 2020). The patch regime of savannah biomes, where a higher number of low intensity fires predominate, can lead to an underestimation of fire pixels and FRP from coarse resolution sensors as discriminating lower intensity fires from the ambient thermal background becomes increasingly challenging as the pixel area increases (Roberts et al. 2009; Sperling et al. 2020).
Lessons from the validation and intercomparison of global active fire products
Satellite active fire products are recognised as having reached stage 3 on the Committee on Earth Observation Satellites (CEOS) Land Product Validation (LPV) hierarchy (National Aeronautics and Space Administration: Goddard Space Flight Center 2023), which required evaluations of product accuracy against in situ or equally suitable reference data and intercomparisons with similar products to determine consistency across a significant set of locations and time periods over globally representative locations. This has been largely achievable through the ability to globally validate active fire detections from MODIS with fire masks derived from the Advanced Spaceborne Thermal Emission and Reflection Radiometer (ASTER), operating concurrently onboard the Terra satellite, and near coincident Landsat observations. MODIS has provided a suitable benchmark to assess the consistency of active fire detections through intercomparisons with products for other low earth orbit (hereafter LEO) satellite sensors, such as the Visible Infra-red Imaging Radiometer Suite (VIIRS) and the Sea and Land Surface Temperature Radiometer (SLSTR), and active fire products for geostationary (hereafter GEO) satellites. The availability of comparable moderate-resolution short-wave infra-red (1.1–3 µm; hereafter SWIR) imagery from Landsat-class sensors and Sentinel-2 is limited to a window of around 30 min, between 10:00 hours and 10:30 hours at the equator, which limits their utility for validating active fire products for LEO sensors with orbits outside of this window. Due to a lack of coincident moderate-resolution satellite observations, VIIRS active fire products are currently at stage 1 on the CEOS LPV hierarchy, with any progress towards higher stages dependent on the availability of suitable quality reference datasets (National Aeronautics and Space Administration: Goddard Space Flight Center 2019).
While validation of active fire detections can be achieved with contemporaneous satellite sensors, the validation of fire characteristics poses a greater challenge. Satellite FRP retrievals are a designated Global Climate Observing System (GCOS) Essential Climate Variable (ECV) and are at the lowest validation stage according to the CEOS LPV hierarchy (National Aeronautics and Space Administration: Goddard Space Flight Center 2023). The GCOS ECV program (GCOS 2016) and the CEOS LPV working groups (Boschetti et al. 2009) have proposed target specifications for FRP retrieval uncertainty, with a goal of limiting it to 10% integrated over a pixel (Wooster et al. 2021). Achieving this goal requires accurate and unsaturated radiance data acquired across a significant number of globally representative locations. The currently operational sensors with a suitable spatial resolution for validating active fire products, such as Landsat OLI and Sentinel-2 MSI, are unsuitable for validating FRP as they are not designed to accommodate the range of temperatures from the ambient background (around 300 K) to high intensity fire events (exceeding 1200 K) (Wooster et al. 2021).
Validation
The validation of active fire products is achieved by two primary means. The first involves determining the detection limits of a given satellite and algorithm by assessing the likelihood that fires of different sizes (Morisette et al. 2005a, 2005b; Csiszar et al. 2006; Hall et al. 2019, 2023) or temperatures (Maier et al. 2013) are successfully detected. The second involves determining error rates using a reference dataset which is a suitable proxy for reality (Csiszar et al. 2006; Schroeder et al. 2008a). Both methods require independent and representative reference data that is inherently more accurate than the product under evaluation and is suitable for the unambiguous identification of the fire area or temperature conditions within the satellite pixel footprint at time of satellite image capture.
Validation approaches using synthetic data
Detection limits under optimised conditions have been estimated using synthetic data produced to represent fires of different sizes and temperatures against representative background conditions. Giglio et al. (1999) used simulated Advanced Very High Resolution Radiometer (AVHRR) MIR and TIR data containing fires of different temperatures and sizes to compare the performance of three algorithms under a variety of surface and atmospheric conditions, using an atmospheric model to calculate atmospheric transmission and thermal conditions for appropriate latitudes. Surfaces were produced representing the typical conditions for different biomes, with each biome characterised by assigning MIR and TIR values to each cell by drawing randomly from normal distributions of seasonal average temperatures and emissivities. The results indicated that the detection limits for algorithms from the same sensor can show significant variation (Giglio et al. 1999). A modification of this approach was repeated to evaluate an improved version of the contextual algorithm used in the MODIS Fire and Thermal Anomalies product (Giglio et al. 2003a) and a multi-year active fire datasets for the Visible and Infra-red Scanner (VIRS) (Giglio et al. 2003b). Schroeder et al. (2014b) assessed the theoretical detection limits of the Suomi VIIRS 375 m product by simulating fire radiances against observed background conditions obtained from a random selection of day-time and night-time imagery. The simulated fires and real background radiances were area-weighted to produce realistic brightness values representative of actual fire conditions. While the use of synthetic data provides a means to statistically characterise the performance of algorithms and assess the theoretical capabilities, validation using real world data is necessary to fully understand the detection limits of active fire products (Hawbaker et al. 2008).
Validation approaches using reference data
Wildfires pose a threat to human safety and expose ground instrumentation to severe conditions, making the collection of synchronous reference data especially challenging (Schroeder et al. 2014a). The highly dynamic nature of wildfire makes it necessary for reference data to be acquired near coincident to satellite image capture, within an acceptable time window of typically less than ±15 min, which varies with biogeographical conditions (Csiszar and Schroeder 2008). Properly timed ground measurements may be obtained for prescribed burns, but it is logistically difficult to obtain measurements which describe the conditions at the scale of an image pixel (Csiszar and Schroeder 2008) for large areas typical of savannah burns. Ground-based radiation measurements can provide an important link for the validation of airborne measurements, which in turn provide invaluable reference data for assessing the detection capabilities of satellite sensors and validating FRP retrievals (Schroeder et al. 2014a; Dickinson et al. 2016). However, studies of this nature are limited by cost, safety, and logistical considerations (Giglio et al. 2016). MODIS airborne simulator (MAS) data, captured during the SCAR-B experiment to aid in the development of the MODIS active fire products (Kaufman et al. 1998), was used by Sperling et al. (2020) to determine the impact on pixel size on fire detection performance and FRP underestimation in the Brazilian cerrado, by degrading the original 50 m pixels to various spatial resolutions comparable to coarse resolution sensors (Sperling et al. 2020). High resolution multispectral data has been collected for fires in the United States of America using the airborne Autonomous Modular Sensor (AMS), onboard NASA’s Ikhana unmanned aircraft (Ambrosia et al. 2011), providing an invaluable data source for assessing the flaming, smouldering and background regions observed within a fire detection (Peterson et al. 2013; Schroeder et al. 2014a). It has been demonstrated that strong agreement (<1% error) is achievable between near-coincident ground and airborne measurements, after atmospheric correction, providing an important bridge between ground and spaceborne data (Schroeder et al. 2014a).
The inherent challenge in obtaining reference data for a statistically meaningful analysis promotes the use of satellite imagery as a substitute for ground truth. The broad spatial coverage of moderate-resolution satellite systems, such as Landsat, enables the entire active fire line to be represented in conjunction with the fire free background used by many contextual active fire algorithms to select flaming pixels for classification (Schroeder et al. 2011). The ASTER instrument operating in tandem with MODIS on board the Terra satellite, acquired spatially and temporally coincident observations which have been used as a surrogate for the true distribution of active fires at near-nadir (within ±8.55°) view angles within MODIS scenes (Giglio et al. 2016). Further validation of off-nadir MODIS viewing geometries was achieved by supplementing ASTER observations with Landsat TM scenes, which have complimentary sensor characteristics and overpass times (Schroeder et al. 2011). ASTER and Landsat-derived fire masks have been widely used to validate satellite active fire detections for MODIS (Morisette et al. 2005a, 2005b; Csiszar et al. 2006) and geostationary active fire products (Schroeder et al. 2008a, 2008b; Hall et al. 2019, 2023). The high spatial resolution of ASTER allowed the detection probability of MODIS active fire detections to be assessed, using the absolute number of ASTER pixels within a MODIS detection as a proxy for fire size. However, as the reference data is typically collected by spatially higher resolution satellite with longer orbit cycles, validation opportunities are limited to a small number of instances at specific local times (Csiszar et al. 2006). While effective for validating active fire detections, the SWIR bands of Landsat and ASTER used to map fire extents frequently saturate (Giglio et al. 2008), making the sensors unsuitable for fire characterisation and validating FRP. To compensate, some studies have used ground measurements of fire temperature to supplement fire masks derived from ASTER and Landsat (Schroeder et al. 2008a, 2010).
Acquiring coincident reference data for evaluating active fire products for GEO sensors is simplified by the high temporal frequency of observations (Csiszar et al. 2006; Wooster et al. 2021). However, GEO satellites typically have a larger instantaneous field of view (IFOV) than LEO sensors, due to their far higher orbits, which effectively reduces the fraction of a pixel occupied by fire. This has the advantage of reducing the likelihood of pixel saturation for higher intensity fires, with the trade-off of a decreased likelihood of detecting low intensity fires. As a result, algorithms for GEO sensors typically have a higher rate of omission when compared to LEO products. A limitation of validating products for GEO satellites against a LEO product is that evaluations are limited to within a short window of the reference satellite system at a consistent local time (Csiszar et al. 2006). This significantly reduces the size of the sample and restricts the evaluation to a snapshot of a diurnal fire cycle (Giglio 2007). Due to the high temporal frequency of geostationary satellites, many fires that are undetected by a GEO product at the time of overpass by the reference system are detected at other points during the fires full lifecycle (Wooster et al. 2015), thus confounding the reported rate of omission for GEO satellite products.
Due to the challenge in obtaining a large sample of synchronous reference data, multiple studies have compared active fire products to burned area maps. Hantson et al. (2013) compared the area of global fire maps produced from MODIS active fire detections to Landsat burned area reference maps (Hantson et al. 2013). Hawbaker et al. (2008) compared MODIS fire clusters to fire perimeter polygons produced from manually interpreted pre- and post-fire Landsat imagery to assess the influence of fire size, geographic region and cloud cover on detection rates (Hawbaker et al. 2008). Validation of coarse resolution active fire observations using burn scars can be problematic, however, due to the sub-pixel nature of the fires and as errors of omission cannot be well addressed (Justice et al. 2013).
Samplings issues
Correlating radiation measurements obtained in situ to fire characteristics derived from satellite imagery is complex due to several limitations that affect the precision and accuracy of measurements. Airborne and spaceborne measurements are impacted by the attenuation of radiation along the atmospheric column between the sensor and fire, which affects the accuracy of retrievals if not corrected for given the deployment altitude of the sensor and the obliquity of the viewing angle (Peterson and Wang 2013; Schroeder et al. 2014a). Space and airborne radiation measurements and derived FRP are impacted by vegetation canopy structure and leaf area index (LAI), as radiation transmittance is significantly reduced in closed canopy biomes (Roberts et al. 2018a). Furthermore, clouds significantly impact retrievals of fire brightness temperature from satellite by blocking outgoing radiation, reflecting sunlight, and confounding the background statistics required by contextual algorithms to define thresholds (Freeborn et al. 2014a). Clouds must either be masked or accommodated for the prevent signal contamination and errors of commission. This becomes increasingly challenging as pixel size increases, as gross checks for cloud become decreasingly effective (Engel et al. 2021a).
Calculations of FRP are heavily influenced by the spatial configuration of fires within the pixel footprint and the radiance of adjacent features caused by the point spread function (PSF) of the satellite instrument, a phenomenon arising from the change in detector sensitivity towards the edge of a pixel (Schroeder et al. 2010). This occurs as the ground sampling distance elongates with increasing scan angle, due to the increased line-of-sight distances and the earth’s curvature (Freeborn et al. 2011). Co-registration errors occur if the specific PSF for each spectral band deviate near the pixel edge, affecting the accuracy of temperature measurements by distributing fire radiance into neighbouring pixels (Peterson et al. 2013). Multiple studies have demonstrated that a fire occupying the centre of a pixel could produce a significantly higher estimate of FRP compared to a fire on the edge of the pixel footprint (Calle et al. 2009; Schroeder et al. 2010; Freeborn et al. 2014b). Freeborn et al. (2011) demonstrated that MODIS active fire products can detect fires down to a 5 MW minimum at nadir, which increased to up to 44 MW at the scan edge. This limitation is offset by an increase in scan-to-scan overlap, where at the swath edges, the same fire may be sensed in multiple consecutive scans (Wolfe et al. 2002), an artefact referred to as the ‘bow-tie’ effect. Freeborn et al. (2014b) quantified the precision of MODIS FRP measurements by exploiting the bow-tie effect to collect near-simultaneous overlapping samples of the same fire from consecutive scans, allowing the uncertainty between samples to be determined. The findings concluded that per-pixel flux estimates can show significant variation but decrease to less than 5% at ±1σ when aggregated over large areas (Freeborn et al. 2014b). To counteract the increase in sensor area across swath, VIIRS and SLSTR include a pixel aggregation scheme, which limits the pixel area increase with scan angle and effectively removes the bow-tie effect (Zhang et al. 2017).
Intercomparisons
Intercomparing products obtained from different satellite sensing systems (Wickramasinghe et al. 2018; Xu et al. 2020), or comparing multiple algorithms for the same sensor (Li et al. 2001; Filizzola et al. 2017), can provide an indication of the gross differences between products and potential for insight into the cause for those differences (Justice et al. 2013). Consistency between fire maps produced by independent products can be characterised through a confusion matrix and the accuracy indices derived from it, such as commission and omission error rates (Foody 2002). However, a major caveat of intercomparing satellite active fire products is that they are often produced by near identical means and are based on the same radiometric signal (Csiszar et al. 2006). As a result, both products may be subject to the same deficiencies and bias errors. As such, when intercomparing active fire products both datasets should be treated as related, where a consistent level of error is established, unlike independent validation where errors in the reference data are negligible or non-existent (Oliva and Schroeder 2015; Wickramasinghe et al. 2018). This encourages the prior validation of the reference product using in situ or equally suitable observations acquired from field campaigns or from aircraft to strengthen the comparison (Cihlar et al. 1997; Csiszar et al. 2006).
The MODIS Fire and Thermal Anomalies product has been widely used as the reference dataset to assess the performance of new sensors and algorithms for both GEO and LEO products, primarily due to the extensive validation of near-nadir observations that was achievable using concurrent ASTER observations. Initial assessments of VIIRS products have been achieved through intercomparison with the near-synchronous MODIS Aqua product (Csiszar et al. 2014; Schroeder et al. 2014b; Zhang et al. 2017) to compare the absolute number of fire pixels detected, and through a direct comparison of the 375 m and 750 m VIIRS products (Schroeder et al. 2014b). Active fire products for SLSTR (Xu et al. 2020; Xu and Wooster 2023), and the global night-time product for AATR (Arino et al. 2012) have been evaluated through an intercomparison to MODIS in a similar manner. The MODIS active fire products have also provided a baseline for the evaluation of GEO active fire products (Roberts et al. 2015; Xu et al. 2017, 2021; Chatzopoulos‑Vouzoglanis et al. 2022, 2023), allowing the intercomparison of different algorithms to be performed more consistently (Freeborn et al. 2014a).
Fire maps produced from Landsat-class sensors have been used as a baseline to directly compare the error rates of GEO active fire products for GOES ABI over the Americas and the Spinning Enhanced Visible and Infra-Red Imager (SEVIRI) over Africa and Europe (Hall et al. 2019, 2023). To ensure synchronicity between observations and consistency in fire conditions between datasets, Landsat scenes were restricted to within a 5-min window of the mid-scan time of GOES ABI and within 6 min of MSG SEVIRI. The high spatial resolution of Landsat, 30 m at nadir, allowed the detection probabilities of both products to be assessed by estimating the effective area covered by fire as mapped by Landsat within the pixel footprints. While it is theoretically possible that Landsat OLI can detect fires down to 1 m2 (Schroeder et al. 2016), each reference fire pixel was treated as corresponding to a 900-m2 fire to allow the fire fractional area within the pixel footprint to be estimated so that the intercomparison could be normalised.
Samplings differences
The vast differences in sampling of active fires by different satellite systems severely impairs any attempt at a direct comparison. This is especially true when comparing products for sensors with different orbits, due to the vast differences in spatial and temporal resolutions between GEO and LEO systems, or when comparing algorithms that derive background statistics by different means (Hall et al. 2019; Chatzopoulos‑Vouzoglanis et al. 2022). Some studies have mitigated this using temporal composites to facilitate the intercomparison between GEO and LEO satellite products over a fixed period (Freeborn et al. 2014a; Wickramasinghe et al. 2018). Engel et al. (2021a, 2021b) reconstructed MODIS and VIIRS swaths to facilitate a comparison with Himawari active fire detections. To avoid errors caused by data misregistration when intercomparing GEO (SEVIRI) and LEO (MODIS) fire products, Calle et al. (2008) divided the study region into squared blocks of varying lengths and fitted linear models to active fire counts obtained within each block by each sensor. This approach was replicated with the addition of a temporal dimension (Amraoui et al. 2010).
Due to differences in the PSF for each sensor, intercomparisons between products from different satellites often exclude observations obtained at high scan angles. An intercomparison between a GEO active fire product and MODIS, without excluding high scan angle observations, may incorrectly record a successful detection by the GEO sensor as an error of commission should these scan angle dependent variations have caused a fire to go undetected (Freeborn et al. 2014a; Roberts et al. 2015). MODIS observations were limited to a scan angle within ±30° when validating SEVIRI observations (Roberts et al. 2015), at which point the MODIS pixel area increases up to a maximum of 1.7 km2, compared to the at nadir 1.0 km2 pixel area (Freeborn et al. 2011, 2014a). These restrictions were later replicated when using MODIS observations to evaluate Himawari-8 AHI, MTSAT and FY2 observations (Xu et al. 2017), GOES ABI (Xu et al. 2021) and Sentinel-3 SLSTR (Xu et al. 2020).
Comparisons of FRP are typically performed using fire clusters or aggregations (Xu et al. 2017, 2021; Chatzopoulos‑Vouzoglanis et al. 2022) due to the high variability in FRP measurements for individual pixels related to the location of a fire within the pixel (Peterson et al. 2013; Freeborn et al. 2014b; Li et al. 2018; Roberts et al. 2018b; Fu et al. 2020), and the along-scan pixel overlap, which leads to an overestimation of FRP on a per pixel basis (Schroeder et al. 2010). Freeborn et al. (2014a) used 0.5° grid aggregations when evaluating the SEVIRI Fire Thermal Anomaly (FTA) algorithm, comparing the seasonal sum of FRP within each grid cell to MODIS retrievals, while Roberts et al. (2005) intercompared total FRP retrievals from SEVIRI and MODIS, both in terms of fire clusters and regional-scale retrievals. Errors in FRP estimates derived from individual fire pixel clusters can remain significant, due to the PSF of the satellite instrument, improper characterisation of the fire background and omission of small fire lines (Schroeder et al. 2010).
Good practice for active fire product validation and intercomparison in savannah ecosystems
The generally short duration and highly variable rate of spread of fires of savannah grassland fires (Stocks et al. 1996; Govender et al. 2006) constrains reference data acquisition for validating active fire detections. Landscape and continental scale assessments of active fire product performance have relied upon Landsat-class or equivalent sensors (e.g. Sentinel-2, ASTER) to validate detections, however, no contemporary Landsat-equivalent sensor operates outside of 10:00–10:30 hours equatorial crossing window, severely limiting the utility of Landsat-class sensors and Sentinel-2 for validating detections from LEO sensors with afternoon equator crossings, such as Suomi and NOAA-20 VIIRS, and limits the validation of GEO active fire detections to a period of lower diurnal fire activity. To circumvent the difficulties inherent in obtaining suitable validation data, Maier et al. (2013) directly used FRP to assess the minimum detection capabilities of the MODIS sensor in the northern Australian savannas. The results estimated a smallest detectable size of 70 m2 for a savannah fire with a 1100-K active flaming front if observed at nadir, increasing to 300 m2 at the edge of the swath, whereas fires smouldering at 600 K would need to be 600 m2 to be detected by MODIS at nadir (Maier et al. 2013). VIIRS detection rates have been found to exceed 80% for fires larger than 10 ha in savannahs validated against Landsat-derived burned area maps, while the short duration and high spread rates of savannah fires resulted in a higher commission and omission rate than forest biomes (Oliva and Schroeder 2015). Low intensity fires are unlikely to be detected by GEO satellite products due to the far larger pixel footprint, resulting in significant omission errors (Roberts et al. 2018b).
The absence of high-resolution reference data (i.e. Landsat-class or equivalent) from contemporaneous satellites for validating active fire detections and FRP effectively necessitates the acquisition of airborne and ground-based measurements to derive burned area and infra-red radiance. Sensors able to render pixels with less than 30 m ground resolution and with a high dynamic range able to accommodate temperatures ranging from flaming fire fronts (>1200 K) and background temperatures (~300 K) used by most contextual active fire algorithms to derive thresholds can provide highly suitable data for assessing the detection capabilities of active fire products and the accuracy of FRP retrievals (Schroeder et al. 2014b). The high dynamic range required to resolve fire temperature fields poses a challenge for presently available single band and broad band MIR thermal cameras, but they are becoming increasingly available and suitable for deriving FRP (Wooster et al. 2005; Paugam et al. 2013; Allison et al. 2016). For example, Paugam et al. (2021) demonstrated a methodology for orthorectifying thermal imagery collected from helicopter-borne MIR and TIR handheld cameras from savannah experimental burns acquired coincident to the overpass by Suomi VIIRS and the Technologie Erprobungs Träger-1 (TET-1) experimental satellite (Paugam et al. 2021).
While the timing of controlled burns can be planned to coincide with a set of satellites being in an ideal position to observe the fire, whether suitable in situ measurements can be obtained is limited by the weather conditions, particularly cloud cover, and for reasons of safety, wind. There are further logistic and cost considerations involved in having an aircraft on hand and ready to fly with the appropriate sensor to acquire measurements synchronous to satellite image capture (Dickinson et al. 2016). While significant progress has been made in recent years, larger datasets of airborne measurements captured across a range of satellite view angles and times of day are needed to comprehensively validate FRP retrievals (Paugam et al. 2021).
Validation
Detection rates
A processing workflow for determining the detection rates of an active fire product and the preparation of airborne and satellite imagery for use as reference data is in Fig. 1. The workflow assumes that any reference satellite imagery is available in an analysis ready format and georeferenced. Assessing the probability that fires of a range of sizes can be detected by a satellite active fire product, by describing the positive detection rate (%) as a function of the fire fractional area within a satellite image pixel, requires reference data suitable for the unambiguous identification of fire presence. Reference fire datasets can be produced using a range of algorithms designed for delineating fire perimeters from airborne MIR imagery (e.g. Valero et al. 2018), multispectral satellite imagery (e.g. Schroeder et al. 2016), or by manual interpretation. The agreement rules describe the spatial and temporal ranges where a match between an active fire detection and a reference dataset can be treated as valid, such as defining a maximum tolerance between non-synchronous observations to reduce the displacement of the fire front between datasets.
Example processing workflow for the validation of satellite active fire detections by determining the pixel-level fire fractional area compared to reference data obtained synchronous to satellite image capture. (a) Unsaturated MIR brightness temperatures permit the further validation of FRP and minimum detectable fire temperature.
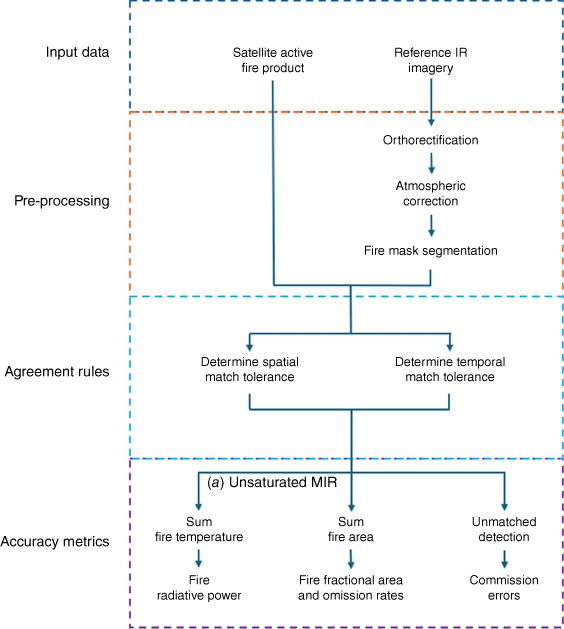
Suitable reference data can be derived from operational multispectral sensors such as Landsat and Sentinel-2, which can resolve fire perimeters with a spatial resolution of 30 m or less. Assessments of this nature treat the entire pixel of the reference dataset to be completely filled with fire (e.g. Morisette et al. 2005a; Schroeder et al. 2008a; Giglio et al. 2016; Hall et al. 2023), leading to an established overestimation of fire area. The utility of satellite observations for evaluating the detection limits of a given product is largely restricted to validating GEO active fire products and LEO sensors with synchronous overpass times.
Thermal cameras can be used to obtain airborne measurements with spatial resolutions of under 1 m in both mounted and handheld configurations, but it is logistically challenging to obtain a large sample of observations for a statistically significant analysis. The typically high spread rate of savannah fires severely restricts the interval between satellite image acquisition and when reference data will remain representative of the imaging conditions, making high frequency sampling highly desirable. Return times from fixed-wing aircraft within 1 min are achievable. Rotary-wing aircraft can provide higher frequency sampling of fire behaviour with the compromise of restricting area coverage. Both aircraft types can provide highly valuable measurements of fire activity for validating satellite observations if planned to coincide with satellite image capture.
Fire radiative power
Validating the accuracy of FRP retrievals imposes the additional restriction that fire radiation measurements are unsaturated (Fig. 1). In addition, to be able to directly compare satellite-derived FRP from ground and airborne measurements, the degree of atmospheric attenuation between each sensor needs to be accounted for (e.g. Schroeder et al. 2014a; Dickinson et al. 2016; Paugam et al. 2021). The frequent saturation of the SWIR wavebands of multispectral sensors and lack of a 4-µm fire sensitive band restricts their utility to assessing the detection limits of a given product as a function of fire fractional area.
Optimal reference data would consist of unsaturated MIR measurements around 4 µm of known high accuracy (within 2σ) with coverage that spans an entire fire line, smouldering areas and the surrounding ambient background used by contextual active fire algorithms to derive thresholds. Accommodating the range of temperatures from the background (~300 K) to flaming fire fronts (>1200 K) remains a challenge for thermal imagers, due to the trade-off between saturation temperature and sensitivity and a smaller IFOV increases the fraction of the image pixel occupied by high-temperature components of fire.
Reporting error and agreement
The accuracy of an active fire product at local scales can be determined through a one-way error matrix analysis to determine the rate of commission errors (i.e. the probability of a false alarm) and omission errors (i.e. the probability that fire presence is undetected) for each pixel of the active fire product. This requires an independent reference dataset of unambiguous fire presence and absence over equivalent spatial and temporal extents to the product under evaluation. An example workflow for validating active fire detections against a reference fire mask is in Fig. 2. Error rates can be further stratified to assess performance under a range of environmental conditions, such as canopy cover, viewing angles or by detection confidence.
Processing workflow for the one-way error matrix validation of satellite active fire detections by comparison to a higher accuracy reference dataset of unambiguous fire presence and absence. The same general workflow is used to intercompare active fire products by substituting the reference fire map for a second active fire product.
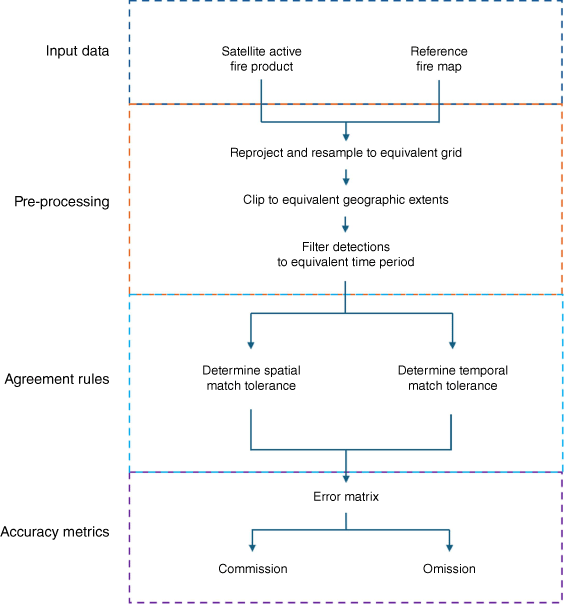
Error rates can be determined over large geographic areas using independent active fire or burned area maps of known higher accuracy that can be temporally and spatially aggregated to equivalent periods. This may require that areas are removed from the evaluation where fire conditions in the reference dataset cannot unequivocally be determined, such as due to cloud obscuration.
Matrix analysis and the associated statistics are also used to determine the agreement between satellite active fire products using the same general workflow (Fig. 2). To determine the consistency between active fire products in terms of information content it is necessary to compare products using identical methods, using rules, which determine how matches between detections are defined in both space and time. Implementation of a direct comparison often requires that both active fire products under comparison are resampled to the same spatial scale, geometric grids, and spatial extents. Intercomparing instantaneous FRP estimates for different satellite products is only achievable if observations from each satellite are approximately synchronous. It is more feasible to assess the consistency between FRP products by comparing statistics derived from integrated FRP estimates that have been aggregated spatially (e.g. fire detection clusters or grid cells) and temporally (e.g. months or seasons).
Conclusions
Consistent methodologies allow active fire products to be evaluated in a representative and unbiased way. This increases confidence in the products and helps to inform new fire detection algorithms and systems by identifying limits of current products. This subsequently contributes to understanding the uncertainty that may be present in applications where such products are applied in savannah ecosystems, including mapping fire frequency and distribution, biomass burning emissions estimates and understanding land cover dynamics.
Procedures for validating satellite active fire detections are well established. However, acquiring reference data coincident with satellite image capture, which is a suitable proxy for reality significantly hinders validation attempts. Comprehensive global validation has been achievable for the MODIS Fire and Thermal Anomalies product due to the presence of the higher order spatial resolution ASTER instrument operating concurrently on board the Terra satellite. As a result of these efforts, MODIS became a benchmark for intercomparing active fire products developed for both LEO and GEO satellite systems, allowing the consistency of detections between products to be evaluated.
This review presents a set of generalised processing workflows for the validation and intercomparison of satellite active fire detections and FRP estimates. While these workflows are tailored to savannah ecosystems, they are broadly applicable to all terrestrial biomes. Assessing the accuracy of FRP retrievals poses a considerable challenge, as unsaturated measurements of temperatures ranging from the ambient background to high intensity fire at a suitably high spatial sampling are not routinely available and are difficult to acquire. Collaborative efforts are desirable to acquire the properly timed field and airborne measurements needed to evaluate the accuracy of satellite-derived FRP retrievals across a statistically significant number of locations and time periods. A shared archive of unsaturated measurements sampled from fires of a range of sizes and temperatures would provide invaluable information for validating and improving satellite active fire product accuracy and consistency.
Data availability
Data sharing is not applicable as no new data were generated or analysed during this study.
References
Allison R, Johnston J, Craig G, Jennings S (2016) Airborne optical and thermal remote sensing for wildfire detection and monitoring. Sensors 16(8), 1310.
| Crossref | Google Scholar |
Ambrosia VG, Wegener S, Zajkowski T, Sullivan DV, Buechel S, Enomoto F, Lobitz B, Johan S, Brass J, Hinkley E (2011) The Ikhana unmanned airborne system (UAS) western states fire imaging missions: from concept to reality (2006–2010). Geocarto International 26, 85-101.
| Crossref | Google Scholar |
Amraoui M, DaCamara CC, Pereira JMC (2010) Detection and monitoring of African vegetation fires using MSG-SEVIRI imagery. Remote Sensing of Environment 114, 1038-1052.
| Crossref | Google Scholar |
Andela N, Kaiser JW, van der Werf GR, Wooster MJ (2015) New fire diurnal cycle characterizations to improve fire radiative energy assessments made from MODIS observations. Atmospheric Chemistry and Physics 15(15), 8831-8846.
| Crossref | Google Scholar |
Andela N, Morton DC, Giglio L, Paugam R, Chen Y, Hantson S, van der Werf GR, Randerson JT (2019) The Global Fire Atlas of individual fire size, duration, speed and direction. Earth System Science Data 11, 529-552.
| Crossref | Google Scholar |
Archer-Nicholls S, Lowe D, Darbyshire E, Morgan WT, Bela MM, Pereira G, Trembath J, Kaiser JW, Longo KM, Freitas SR, Coe H, McFiggans G (2015) Characterising Brazilian biomass burning emissions using WRF-Chem with MOSAIC sectional aerosol. Geoscientific Model Development 8, 549-577.
| Crossref | Google Scholar |
Archibald S, Roy DP, van WILGEN BW, Scholes RJ (2009) What limits fire? An examination of drivers of burnt area in Southern Africa. Global Change Biology 15, 613-630.
| Crossref | Google Scholar |
Archibald S, Scholes RJ, Roy DP, Roberts G, Boschetti L (2010) Southern African fire regimes as revealed by remote sensing. International Journal of Wildland Fire 19, 861-878.
| Crossref | Google Scholar |
Arino O, Casadio S, Serpe D (2012) Global night-time fire season timing and fire count trends using the ATSR instrument series. Remote Sensing of Environment 116, 226-238.
| Crossref | Google Scholar |
Beringer J, Hutley LB, Abramson D, Arndt SK, Briggs P, Bristow M, Canadell JG, Cernusak LA, Eamus D, Edwards AC, Evans BJ, Fest B, Goergen K, Grover SP, Hacker J, Haverd V, Kanniah K, Livesley SJ, Lynch A, Maier S, Moore C, Raupach M, Russell-Smith J, Scheiter S, Tapper NJ, Uotila P (2015) Fire in Australian savannas: from leaf to landscape. Global Change Biology 21, 62-81.
| Crossref | Google Scholar | PubMed |
Boschetti L, Roy D, Justice C (2009) ‘International global burned area satellite product validation protocol. Part I-Production and Standardization of Validation Reference Data’. pp. 1–11. Available at https://lpvs.gsfc.nasa.gov/PDF/BurnedAreaValidationProtocol.pdf
Calle A, González‐Alonso F, Merino de Miguel S (2008) Validation of active forest fires detected by MSG‐SEVIRI by means of MODIS hot spots and AWiFS images. International Journal of Remote Sensing 29, 3407-3415.
| Crossref | Google Scholar |
Calle A, Casanova JL, González-Alonso F (2009) Impact of point spread function of MSG-SEVIRI on active fire detection. International Journal of Remote Sensing 30, 4567-4579.
| Crossref | Google Scholar |
Chatzopoulos-Vouzoglanis K, Reinke KJ, Soto-Berelov M, Engel C, Jones SD (2022) Comparing geostationary and polar-orbiting satellite sensor estimates of Fire Radiative Power (FRP) during the Black Summer Fires (2019–2020) in south-eastern Australia. International Journal of Wildland Fire 31, 572-585.
| Crossref | Google Scholar |
Chatzopoulos-Vouzoglanis K, Reinke KJ, Soto-Berelov M, Jones SD (2023) One year of near-continuous fire monitoring on a continental scale: Comparing fire radiative power from polar-orbiting and geostationary observations. International Journal of Applied Earth Observation and Geoinformation 117, 103214.
| Crossref | Google Scholar |
Chuvieco E, Mouillot F, van der Werf GR, San Miguel J, Tanasse M, Koutsias N, García M, Yebra M, Padilla M, Gitas I, Heil A, Hawbaker TJ, Giglio L (2019) Historical background and current developments for mapping burned area from satellite Earth observation. Remote Sensing of Environment 225, 45-64.
| Crossref | Google Scholar |
Chuvieco E, Pettinari ML, Koutsias N, Forkel M, Hantson S, Turco M (2021) Human and climate drivers of global biomass burning variability. Science of The Total Environment 779, 146361.
| Crossref | Google Scholar | PubMed |
Cihlar J, Chen J, Li Z (1997) On the validation of satellite-derived products for land applications. Canadian Journal of Remote Sensing 23, 381-389.
| Crossref | Google Scholar |
Cochrane MA (2009) ‘Tropical fire ecology: climate change, land use, and ecosystem dynamics.’ (Springer: Berlin, Heidelberg) 10.1007/978-3-540-77381-8
Csiszar I, Denis L, Giglio L, Justice CO, Hewson J (2005) Global fire activity from two years of MODIS data. International Journal of Wildland Fire 14(2), 117.
| Crossref | Google Scholar |
Csiszar IA, Schroeder W (2008) Short-Term observations of the temporal development of active fires from consecutive same-day ETM+ and ASTER imagery in the Amazon: implications for active fire product validation. IEEE Journal of Selected Topics in Applied Earth Observations and Remote Sensing 1, 248-253.
| Crossref | Google Scholar |
Csiszar IA, Morisette JT, Giglio L (2006) Validation of Active Fire Detection From Moderate-Resolution Satellite Sensors: The MODIS Example in Northern Eurasia. IEEE Transactions on Geoscience and Remote Sensing 44, 1757-1764.
| Crossref | Google Scholar |
Csiszar I, Schroeder W, Giglio L, Ellicott E, Vadrevu KP, Justice CO, Wind B (2014) Active fires from the Suomi NPP visible infrared imaging radiometer suite: product status and first evaluation results. Journal of Geophysical Research: Atmospheres 119, 803-816.
| Crossref | Google Scholar |
Dickinson MB, Hudak AT, Zajkowski T, Loudermilk EL, Schroeder W, Ellison L, Kremens RL, Holley W, Martinez O, Paxton A, Bright BC, O’Brien JJ, Hornsby B, Ichoku C, Faulring J, Gerace A, Peterson D, Mauceri J (2016) Measuring radiant emissions from entire prescribed fires with ground, airborne and satellite sensors – RxCADRE 2012. International Journal of Wildland Fire 25, 48-61.
| Crossref | Google Scholar |
Edwards A, Archer R, De Bruyn P, Evans J, Lewis B, Vigilante T, Whyte S, Russell-Smith J (2021) Transforming fire management in northern Australia through successful implementation of savanna burning emissions reductions projects. Journal of Environmental Management 290, 112568.
| Crossref | Google Scholar | PubMed |
Engel CB, Jones SD, Reinke KJ (2021a) Real-time detection of daytime and night-time fire hotspots from geostationary satellites. Remote Sensing 13, 1627.
| Crossref | Google Scholar |
Engel CB, Jones SD, Reinke K (2021b) A seasonal-window ensemble-based thresholding technique used to detect active fires in geostationary remotely sensed data. IEEE Transactions on Geoscience and Remote Sensing 59, 4947-4956.
| Crossref | Google Scholar |
Eva H, Lambin EF (1998) Remote sensing of biomass burning in tropical regions. Remote Sensing of Environment 64(3), 292-315.
| Crossref | Google Scholar |
Filizzola C, Corrado R, Marchese F, Mazzeo G, Paciello R, Pergola N, Tramutoli V (2017) RST-FIRES, an exportable algorithm for early-fire detection and monitoring: description, implementation, and field validation in the case of the MSG-SEVIRI sensor. Remote Sensing of Environment 192, e2-e25.
| Crossref | Google Scholar |
Foody GM (2002) Status of land cover classification accuracy assessment. Remote Sensing of Environment 80, 185-201.
| Crossref | Google Scholar |
Franquesa M, Stehman SV, Chuvieco E (2022) Assessment and characterization of sources of error impacting the accuracy of global burned area products. Remote Sensing of Environment 280, 113214.
| Crossref | Google Scholar |
Freeborn PH, Wooster MJ, Roberts G (2011) Addressing the spatiotemporal sampling design of MODIS to provide estimates of the fire radiative energy emitted from Africa. Remote Sensing of Environment 115, 475-489.
| Crossref | Google Scholar |
Freeborn P, Wooster M, Roberts G, Xu W (2014a) Evaluating the SEVIRI Fire Thermal Anomaly Detection Algorithm across the Central African Republic using the MODIS active fire product. Remote Sensing 6, 1890-1917.
| Crossref | Google Scholar |
Freeborn PH, Wooster MJ, Roy DP, Cochrane MA (2014b) Quantification of MODIS fire radiative power (FRP) measurement uncertainty for use in satellite-based active fire characterization and biomass burning estimation. Geophysical Research Letters 41, 1988-1994.
| Crossref | Google Scholar |
Fu Y, Li R, Wang X, Bergeron Y, Valeria O, Chavardès RD, Wang Y, Hu J (2020) Fire detection and fire radiative power in forests and low-biomass lands in Northeast Asia: MODIS versus VIIRS fire products. Remote Sensing 12, 2870.
| Crossref | Google Scholar |
GCOS (2016) The Global Observing System for Climate: Implementation Needs, GCOS200. Available at https://library.wmo.int/doc_num.php?explnum_id=3417
Giglio L (2007) Characterization of the tropical diurnal fire cycle using VIRS and MODIS observations. Remote Sensing of Environment 108, 407-421.
| Crossref | Google Scholar |
Giglio L, Kendall JD, Justice CO (1999) Evaluation of global fire detection algorithms using simulated AVHRR infrared data. International Journal of Remote Sensing 20, 1947-1985.
| Crossref | Google Scholar |
Giglio L, Descloitres J, Justice CO, Kaufman YJ (2003a) An enhanced contextual fire detection algorithm for MODIS. Remote Sensing of Environment 87, 273-282.
| Crossref | Google Scholar |
Giglio L, Kendall JD, Mack R (2003b) A multi-year active fire dataset for the tropics derived from the TRMM VIRS. International Journal of Remote Sensing 24, 4505-4525.
| Crossref | Google Scholar |
Giglio L, Csiszar I, Restás Á, Morisette JT, Schroeder W, Morton D, Justice CO (2008) Active fire detection and characterization with the advanced spaceborne thermal emission and reflection radiometer (ASTER). Remote Sensing of Environment 112, 3055-3063.
| Crossref | Google Scholar |
Giglio L, Boschetti L, Roy DP, Humber ML, Justice CO (2018) The Collection 6 MODIS burned area mapping algorithm and product. Remote Sensing of Environment 217, 72-85.
| Crossref | Google Scholar | PubMed |
Giglio L, Schroeder W, Justice CO (2016) The collection 6 MODIS active fire detection algorithm and fire products. Remote Sensing of Environment 178, 31-41.
| Crossref | Google Scholar | PubMed |
Govender N, Trollope WSW, Van Wilgen BW (2006) The effect of fire season, fire frequency, rainfall and management on fire intensity in savanna vegetation in South Africa. Journal of Applied Ecology 43, 748-758.
| Crossref | Google Scholar |
Hall JV, Zhang R, Schroeder W, Huang C, Giglio L (2019) Validation of GOES-16 ABI and MSG SEVIRI active fire products. International Journal of Applied Earth Observation and Geoinformation 83, 101928.
| Crossref | Google Scholar |
Hall JV, Schroeder W, Rishmawi K, Wooster M, Schmidt CC, Huang C, Csiszar I, Giglio L (2023) Geostationary active fire products validation: GOES-17 ABI, GOES-16 ABI, and Himawari AHI. International Journal of Remote Sensing 44, 3174-3193.
| Crossref | Google Scholar |
Hally B, Wallace L, Reinke K, Jones S (2016) Assessment of the utility of the advanced himawari imager to detect active fire over Australia. ISPRS - International Archives of the Photogrammetry, Remote Sensing and Spatial Information Sciences XLI-B8, 65-71.
| Crossref | Google Scholar |
Hantson S, Padilla M, Corti D, Chuvieco E (2013) Strengths and weaknesses of MODIS hotspots to characterize global fire occurrence. Remote Sensing of Environment 131, 152-159.
| Crossref | Google Scholar |
Hawbaker TJ, Radeloff VC, Syphard AD, Zhu Z, Stewart SI (2008) Detection rates of the MODIS active fire product in the United States. Remote Sensing of Environment 112, 2656-2664.
| Crossref | Google Scholar |
Ichoku C, Kaufman YJ (2005) A method to derive smoke emission rates from MODIS fire radiative energy measurements. IEEE Transactions on Geoscience and Remote Sensing 43, 2636-2649.
| Crossref | Google Scholar |
Justice C, Belward A, Morisette J, Lewis P, Privette J, Baret F (2000) Developments in the ‘validation’ of satellite sensor products for the study of the land surface. International Journal of Remote Sensing 21(17), 3383-3390.
| Crossref | Google Scholar |
Justice C, Csiszar I, Boschetti L, Korontzi S, Schroeder W, Giglio L, Vadrevu KP, Roy D (2013) Satellite monitoring and inventory of global vegetation fire. In ‘Vegetation fires and global change: Challenges for concerted international action’. (Ed. JG Goldammer) (Global Fire Monitoring Centre (GFMC)) Available at https://gfmc.online/latestnews/Vegetation-Fires-Global-Change-UN-White-Paper-GFMC-2013.pdf
Kaiser JW, Heil A, Andreae MO, Benedetti A, Chubarova N, Jones L, Morcrette JJ, Razinger M, Schultz MG, Suttie M, van der Werf GR (2012) Biomass burning emissions estimated with a global fire assimilation system based on observed fire radiative power. Biogeosciences 9, 527-554.
| Crossref | Google Scholar |
Karp AT, Uno KT, Berke MA, Russell JM, Scholz CA, Marlon JR, Faith JT, Staver AC (2023) Nonlinear rainfall effects on savanna fire activity across the African Humid Period. Quaternary Science Reviews 304, 107994.
| Crossref | Google Scholar |
Kaufman YJ, Kleidman RG, King MD (1998) SCAR-B fires in the tropics: properties and remote sensing from EOS-MODIS. Journal of Geophysical Research 103, 31955-31968.
| Crossref | Google Scholar |
Kumar SS, Roy DP, Boschetti L, Kremens R (2011) Exploiting the power law distribution properties of satellite fire radiative power retrievals: a method to estimate fire radiative energy and biomass burned from sparse satellite observations. Journal of Geophysical Research 116, D19303.
| Crossref | Google Scholar |
Laris PS (2005) Spatiotemporal problems with detecting and mapping mosaic fire regimes with coarse-resolution satellite data in savanna environments. Remote Sensing of Environment 99(4), 412-424.
| Crossref | Google Scholar |
Lehmann CER, Archibald SA, Hoffmann WA, Bond WJ (2011) Deciphering the distribution of the savanna biome. New Phytologist 191(1), 197-209.
| Crossref | Google Scholar |
Lehmann CER, Anderson TM, Sankaran M, Higgins SI, Archibald S, Hoffmann WA, Hanan NP, Williams RJ, Fensham RJ, Felfili J, Hutley LB, Ratnam J, San Jose J, Montes R, Franklin D, Russell-Smith J, Ryan CM, Durigan G, Hiernaux P, Haidar R, Bowman DMJS, Bond WJ (2014) Savanna vegetation-fire-climate relationships differ among continents. Science 343(6170), 548-552.
| Crossref | Google Scholar |
Li Z, Kaufman YJ, Ichoku C, Fraser R, Trishchenko A, Giglio L, Jin JZ, Yu X (2001) A review of AVHRR-based active fire detection algorithms: Principles, limitations, and recommendations. In ‘Global and Regional Vegetation Fire Monitoring from Space, Planning and Coordinated International Effort’. (Eds FJ Ahern, JG Goldammer, CO Justice) pp. 199–225. (Kugler Publications: The Hague, The Netherlands) Available at https://www.google.com.au/books/edition/Global_and_Regional_Vegetation_Fire_Moni/AHazSEi62pMC?hl=en&gbpv=0
Li F, Zhang X, Kondragunta S, Csiszar I (2018) Comparison of fire radiative power estimates from VIIRS and MODIS observations. Journal of Geophysical Research: Atmospheres 123, 4545-4563.
| Crossref | Google Scholar |
Lizundia-Loiola J, Otón G, Ramo R, Chuvieco E (2020) A spatio-temporal active-fire clustering approach for global burned area mapping at 250 m from MODIS data. Remote Sensing of Environment 236, 111493.
| Crossref | Google Scholar |
Maier SW, Russell-Smith J, Edwards AC, Yates C (2013) Sensitivity of the MODIS fire detection algorithm (MOD14) in the savanna region of the Northern Territory, Australia. ISPRS Journal of Photogrammetry and Remote Sensing 76, 11-16.
| Crossref | Google Scholar |
Meyer CP, Cook GD, Reisen F, Smith TEL, Tattaris M, Russell-Smith J, Maier SW, Yates CP, Wooster MJ (2012) Direct measurements of the seasonality of emission factors from savanna fires in northern Australia. Journal of Geophysical Research 117, D20305.
| Crossref | Google Scholar |
Morisette JT, Giglio L, Csiszar I, Justice CO (2005a) Validation of the MODIS active fire product over Southern Africa with ASTER data. International Journal of Remote Sensing 26, 4239-4264.
| Crossref | Google Scholar |
Morisette JT, Giglio L, Csiszar I, Setzer A, Schroeder W, Morton D, Justice CO (2005b) Validation of MODIS active fire detection products derived from two algorithms. Earth Interactions 9, 1-25.
| Crossref | Google Scholar |
Mota B, Wooster MJ (2018) A new top-down approach for directly estimating biomass burning emissions and fuel consumption rates and totals from geostationary satellite fire radiative power (FRP). Remote Sensing of Environment 206, 45-62.
| Crossref | Google Scholar |
Murphy BP, Russell-Smith J (2010) Fire severity in a northern Australian savanna landscape: the importance of time since previous fire. International Journal of Wildland Fire 19, 46-51.
| Crossref | Google Scholar |
National Aeronautics and Space Administration: Goddard Space Flight Center (2019) VIIRS NASA fire product validation. Available at https://viirsland.gsfc.nasa.gov/Val/Fire Val.html
National Aeronautics and Space Administration: Goddard Space Flight Center (2023) Validation framework. CEOS working group on calibration and validation: Land product validation subgroup. Available at https://lpvs.gsfc.nasa.gov/index.html
Nguyen HM, Wooster MJ (2020) Advances in the estimation of high Spatio-temporal resolution pan-African top-down biomass burning emissions made using geostationary fire radiative power (FRP) and MAIAC aerosol optical depth (AOD) data. Remote Sensing of Environment 248, 111971.
| Crossref | Google Scholar |
Nguyen HM, He J, Wooster MJ (2023) Biomass burning CO, PM and fuel consumption per unit burned area estimates derived across Africa using geostationary SEVIRI fire radiative power and Sentinel-5P CO data. Atmospheric Chemistry and Physics 23, 2089-2118.
| Crossref | Google Scholar |
Oliva P, Schroeder W (2015) Assessment of VIIRS 375m active fire detection product for direct burned area mapping. Remote Sensing of Environment 160, 144-155.
| Crossref | Google Scholar |
Oliveira SLJ, Turkman MAA, Pereira JMC (2013) An analysis of fire frequency in tropical savannas of northern Australia, using a satellite-based fire atlas. International Journal of Wildland Fire 22(4), 479.
| Crossref | Google Scholar |
Oliveira SLJ, Campagnolo ML, Price OF, Edwards AC, Russell-Smith J, Pereira JMC (2015) Ecological implications of fine-scale fire patchiness and severity in tropical savannas of northern Australia. Fire Ecology 11(1), 10-31.
| Crossref | Google Scholar |
Padilla M, Olofsson P, Stehman SV, Tansey K, Chuvieco E (2017) Stratification and sample allocation for reference burned area data. Remote Sensing of Environment 203, 240-255.
| Crossref | Google Scholar |
Paugam R, Wooster MJ, Roberts G (2013) Use of handheld thermal imager data for airborne mapping of fire radiative power and energy and flame front rate of spread. IEEE Transactions on Geoscience and Remote Sensing 51(6), 3385-3399.
| Crossref | Google Scholar |
Paugam R, Wooster MJ, Mell WE, Rochoux MC, Filippi J-B, Rücker G, Frauenberger O, Lorenz E, Schroeder W, Main B, Govender N (2021) Orthorectification of helicopter-borne high resolution experimental burn observation from infra red handheld imagers. Remote Sensing 13, 4913.
| Crossref | Google Scholar |
Pereira JMC (2003) Remote sensing of burned areas in tropical savannas. International Journal of Wildland Fire 12, 259-270.
| Crossref | Google Scholar |
Pereira Júnior AC, Oliveira SLJ, Pereira JMC, Turkman MAA (2014) Modelling fire frequency in a cerrado savanna protected area. PLoS ONE 9(7), e102380.
| Crossref | Google Scholar |
Peterson D, Wang J (2013) A sub-pixel-based calculation of fire radiative power from MODIS observations: 2. Sensitivity analysis and potential fire weather application. Remote Sensing of Environment 129, 231-249.
| Crossref | Google Scholar |
Peterson D, Wang J, Ichoku C, Hyer E, Ambrosia V (2013) A sub-pixel-based calculation of fire radiative power from MODIS observations: 1: Algorithm development and initial assessment. Remote Sensing of Environment 129, 262-279.
| Crossref | Google Scholar |
Pricope NG, Binford MW (2012) A spatio-temporal analysis of fire recurrence and extent for semi-arid savanna ecosystems in southern Africa using moderate-resolution satellite imagery. Journal of Environmental Management 100, 72-85.
| Crossref | Google Scholar |
Prins EM, Feltz JM, Menzel WP, Ward DE (1998) An overview of GOES-8 diurnal fire and smoke results for SCAR-B and 1995 fire season in South America. Journal of Geophysical Research 103, 31821-31835.
| Crossref | Google Scholar |
Ramo R, Roteta E, Bistinas I, van Wees D, Bastarrika A, Chuvieco E, van der Werf GR (2021) African burned area and fire carbon emissions are strongly impacted by small fires undetected by coarse resolution satellite data. Proceedings of the National Academy of Sciences of the United States of America 118, e2011160118.
| Crossref | Google Scholar | PubMed |
Randerson JT, Chen Y, van der Werf GR, Rogers BM, Morton DC (2012) Global burned area and biomass burning emissions from small fires. Journal of Geophysical Research 117, G04012.
| Crossref | Google Scholar |
Roberts GJ, Wooster MJ (2008) Fire detection and fire characterization over Africa using Meteosat SEVIRI. IEEE Transactions on Geoscience and Remote Sensing 46, 1200-1218.
| Crossref | Google Scholar |
Roberts G, Wooster MJ, Perry GLW, Drake N, Rebelo LM, Dipotso F (2005) Retrieval of biomass combustion rates and totals from fire radiative power observations: application to southern Africa using geostationary SEVIRI imagery. Journal of Geophysical Research 110, D21111.
| Crossref | Google Scholar |
Roberts G, Wooster MJ, Lagoudakis E (2009) Annual and diurnal African biomass burning temporal dynamics. Biogeosciences 6, 849-866.
| Crossref | Google Scholar |
Roberts G, Wooster MJ, Xu W, Freeborn PH, Morcrette JJ, Jones L, Benedetti A, Jiangping H, Fisher D, Kaiser JW (2015) LSA SAF Meteosat FRP products – Part 2: evaluation and demonstration for use in the Copernicus Atmosphere Monitoring Service (CAMS). Atmospheric Chemistry and Physics 15, 13241-13267.
| Crossref | Google Scholar |
Roberts G, Wooster M, Lauret N, Gastellu-Etchegorry J, Lynham T, McRae D (2018a) Investigating the impact of overlying vegetation canopy structures on fire radiative power (FRP) retrieval through simulation and measurement. Remote Sensing of Environment 217, 158-171.
| Crossref | Google Scholar |
Roberts G, Wooster M, Xu W, He J (2018b) Fire activity and fuel consumption dynamics in sub-Saharan Africa. Remote Sensing 10, 1591.
| Crossref | Google Scholar |
Roteta E, Bastarrika A, Padilla M, Storm T, Chuvieco E (2019) Development of a Sentinel-2 burned area algorithm: generation of a small fire database for sub-Saharan Africa. Remote Sensing of Environment 222, 1-17.
| Crossref | Google Scholar |
Roy DP, Boschetti L, Giglio L (2010) Remote sensing of global savanna fire occurrence, extent, and properties. In ‘Ecosystem function in savannas: measurement and modeling at landscape to global scales’. (Eds MJ Hill, NP Hanan) pp. 239–254. (CRC Press) 10.1201/b10275-22
Russell-Smith J, Ryan PG, Durieu R (1997) A LANDSAT MSS-derived fire history of Kakadu National Park, Monsoonal Northern Australial, 1980-94: seasonal extent, frequency and patchiness. The Journal of Applied Ecology 34(3), 748.
| Crossref | Google Scholar |
Russell-Smith J, Yates CP, Whitehead PJ, Smith R, Craig R, Allan GE, Thackway R, Frakes I, Cridland S, Meyer MCP, Gill AM (2007) Bushfires ‘down under’: patterns and implications of contemporary Australian landscape burning. International Journal of Wildland Fire 16(4), 361.
| Crossref | Google Scholar |
Russell-Smith J, Monagle C, Jacobsohn M, Beatty RL, Bilbao B, Millán A, Vessuri H, Sánchez-Rose I (2017) Can savanna burning projects deliver measurable greenhouse emissions reductions and sustainable livelihood opportunities in fire-prone settings? Climatic Change 140, 47-61.
| Crossref | Google Scholar |
Russell-Smith J, Yates C, Vernooij R, Eames T, van der Werf G, Ribeiro N, Edwards A, Beatty R, Lekoko O, Mafoko J, Monagle C, Johnston S (2021) Opportunities and challenges for savanna burning emissions abatement in southern Africa. Journal of Environmental Management 288, 112414.
| Crossref | Google Scholar | PubMed |
Schroeder W, Prins E, Giglio L, Csiszar I, Schmidt C, Morisette J, Morton D (2008a) Validation of GOES and MODIS active fire detection products using ASTER and ETM+ data. Remote Sensing of Environment 112, 2711-2726.
| Crossref | Google Scholar |
Schroeder W, Ruminski M, Csiszar I, Giglio L, Prins E, Schmidt C, Morisette J (2008b) Validation analyses of an operational fire monitoring product: the hazard mapping system. International Journal of Remote Sensing 29, 6059-6066.
| Crossref | Google Scholar |
Schroeder W, Csiszar I, Giglio L, Schmidt CC (2010) On the use of fire radiative power, area, and temperature estimates to characterize biomass burning via moderate to coarse spatial resolution remote sensing data in the Brazilian Amazon. Journal of Geophysical Research 115, D21121.
| Crossref | Google Scholar |
Schroeder W, Csiszar I, Giglio L, Ellicott E, Justice C (2011) Satellite Active Fire Product Validation Using High Spatial Resolution Reference Data. In ‘34th International Symposium on Remote Sensing of Environment - The GEOSS Era: Towards Operational Environmental Monitoring’. 4 p. https://www.isprs.org/proceedings/2011/ISRSE-34/211104015Final00178.pdfhttps://www.isprs.org/proceedings/2011/ISRSE-34/211104015Final00178.pdf
Schroeder W, Ellicott E, Ichoku C, Ellison L, Dickinson MB, Ottmar RD, Clements C, Hall D, Ambrosia V, Kremens R (2014a) Integrated active fire retrievals and biomass burning emissions using complementary near-coincident ground, airborne and spaceborne sensor data. Remote Sensing of Environment 140, 719-730.
| Crossref | Google Scholar |
Schroeder W, Oliva P, Giglio L, Csiszar IA (2014b) The New VIIRS 375m active fire detection data product: algorithm description and initial assessment. Remote Sensing of Environment 143, 85-96.
| Crossref | Google Scholar |
Schroeder W, Oliva P, Giglio L, Quayle B, Lorenz E, Morelli F (2016) Active fire detection using Landsat-8/OLI data. Remote Sensing of Environment 185, 210-220.
| Crossref | Google Scholar |
Sperling S, Wooster MJ, Malamud BD (2020) Influence of satellite sensor pixel size and overpass time on undercounting of cerrado/savannah landscape-scale Fire Radiative Power (FRP): an assessment using the MODIS airborne simulator. Fire 3, 11.
| Crossref | Google Scholar |
Stocks BJ, van Wilgen BW, Trollope WSW, McRae DJ, Mason JA, Weirich F, Potgieter ALF (1996) Fuels and fire behavior dynamics on large‐scale savanna fires in Kruger National Park, South Africa. Journal of Geophysical Research: Atmospheres 101(D19), 23541-23550.
| Crossref | Google Scholar |
Valero MM, Rios O, Pastor E, Planas E (2018) Automated location of active fire perimeters in aerial infrared imaging using unsupervised edge detectors. International Journal of Wildland Fire 27, 241-256.
| Crossref | Google Scholar |
Van Der Werf GR, Randerson JT, Giglio L, van Leeuwen TT, Chen Y, Rogers BM, Mu M, van Marle MJE, Morton DC, Collatz GJ, Yokelson RJ, Kasibhatla PS (2017) Global fire emissions estimates during 1997–2016. Earth System Science Data 9, 697-720.
| Crossref | Google Scholar |
Van Langevelde F, Van De Vijver CADM, Kumar L, Van De Koppel J, De Ridder N, Van Andel J, Skidmore AK, Hearne JW, Stroosnijder L, Bond WJ, Prins HHT, Rietkerk M (2003) Effects of fire and herbivory on the stability of savanna ecosystems. Ecology 84(2), 337-350.
| Crossref | Google Scholar |
van Wilgen BW, Govender N, Biggs HC (2007) The contribution of fire research to fire management: a critical review of a long-term experiment in the Kruger National Park, South Africa. International Journal of Wildland Fire 16, 519-530.
| Crossref | Google Scholar |
Wickramasinghe C, Wallace L, Reinke K, Jones S (2018) Intercomparison of Himawari-8 AHI-FSA with MODIS and VIIRS active fire products. International Journal of Digital Earth 13, 454-473.
| Crossref | Google Scholar |
Wolfe RE, Nishihama M, Fleig AJ, Kuyper JA, Roy DP, Storey JC, Patt FS (2002) Achieving sub-pixel geolocation accuracy in support of MODIS land science. Remote Sensing of Environment 83, 31-49.
| Crossref | Google Scholar |
Wooster MJ, Roberts G, Perry GLW, Kaufman YJ (2005) Retrieval of biomass combustion rates and totals from fire radiative power observations: FRP derivation and calibration relationships between biomass consumption and fire radiative energy release. Journal of Geophysical Research 110, D24311.
| Crossref | Google Scholar |
Wooster MJ, Roberts G, Smith AMS, Johnston J, Freeborn P, Amici S, Hudak AT (2013) Thermal remote sensing of active vegetation fires and biomass burning events. In ‘Thermal infrared remote sensing’. Remote sensing and digital image processing. (Eds C Kuenzer, S Dech) pp. 347–390. (Springer Netherlands: Dordrecht) 10.1007/978-94-007-6639-6_18
Wooster MJ, Roberts G, Freeborn PH, Xu W, Govaerts Y, Beeby R, He J, Lattanzio A, Fisher D, Mullen R (2015) LSA SAF Meteosat FRP products – Part 1: algorithms, product contents, and analysis. Atmospheric Chemistry and Physics 15, 13217-13239.
| Crossref | Google Scholar |
Wooster MJ, Roberts GJ, Giglio L, Roy DP, Freeborn PH, Boschetti L, Justice C, Ichoku C, Schroeder W, Davies D, Smith AMS, Setzer A, Csiszar I, Strydom T, Frost P, Zhang T, Xu W, de Jong MC, Johnston JM, Ellison L, Vadrevu K, Sparks AM, Nguyen H, McCarty J, Tanpipat V, Schmidt C, San-Miguel-Ayanz J (2021) Satellite remote sensing of active fires: history and current status, applications and future requirements. Remote Sensing of Environment 267, 112694.
| Crossref | Google Scholar |
Xu W, Wooster MJ (2023) Sentinel-3 SLSTR active fire (AF) detection and FRP daytime product - Algorithm description and global intercomparison to MODIS, VIIRS and landsat AF data. Science of Remote Sensing 7, 100087.
| Crossref | Google Scholar |
Xu W, Wooster MJ, Kaneko T, He J, Zhang T, Fisher D (2017) Major advances in geostationary fire radiative power (FRP) retrieval over Asia and Australia stemming from use of Himarawi-8 AHI. Remote Sensing of Environment 193, 138-149.
| Crossref | Google Scholar |
Xu W, Wooster MJ, He J, Zhang T (2020) First study of Sentinel-3 SLSTR active fire detection and FRP retrieval: night-time algorithm enhancements and global intercomparison to MODIS and VIIRS AF products. Remote Sensing of Environment 248, 111947.
| Crossref | Google Scholar |
Xu W, Wooster MJ, He J, Zhang T (2021) Improvements in high-temporal resolution active fire detection and FRP retrieval over the Americas using GOES-16 ABI with the geostationary Fire Thermal Anomaly (FTA) algorithm. Science of Remote Sensing 3, 100016.
| Crossref | Google Scholar |
Yates CP, Edwards AC, Russell-Smith J (2008) Big fires and their ecological impacts in Australian savannas: size and frequency matters. International Journal of Wildland Fire 17(6), 768.
| Crossref | Google Scholar |
Zhang T, Wooster MJ, Xu W (2017) Approaches for synergistically exploiting VIIRS I- and M-Band data in regional active fire detection and FRP assessment: a demonstration with respect to agricultural residue burning in Eastern China. Remote Sensing of Environment 198, 407-424.
| Crossref | Google Scholar |