Climate and weather drivers in southern California Santa Ana Wind and non-Santa Wind fires
Jon E. Keeley A B * , Michael Flannigan C , Tim J. Brown D , Tom Rolinski E , Daniel Cayan F , Alexandra D. Syphard G , Janin Guzman-Morales H and Alexander Gershunov FA
B
C
D
E
F
G
H
Abstract
Autumn and winter Santa Ana Winds (SAW) are responsible for the largest and most destructive wildfires in southern California.
(1) To contrast fires ignited on SAW days vs non-SAW days, (2) evaluate the predictive ability of the Canadian Fire Weather Index (CFWI) for these two fire types, and (3) determine climate and weather factors responsible for the largest wildfires.
CAL FIRE (California Department of Forestry and Fire Protection) FRAP (Fire and Resource Assessment Program) fire data were coupled with hourly climate data from four stations, and with regional indices of SAW wind speed, and with seasonal drought data from the Palmer Drought Severity Index.
Fires on non-SAW days were more numerous and burned more area, and were substantial from May to October. CFWI indices were tied to fire occurrence and size for both non-SAW and SAW days, and in the days following ignition. Multiple regression models for months with the greatest area burned explained up to a quarter of variation in area burned.
The drivers of fire size differ between non-SAW and SAW fires. The best predictor of fire size for non-SAW fires was drought during the prior 5 years, followed by a current year vapour pressure deficit. For SAW fires, wind speed followed by drought were most important.
Keywords: aiutumn fires, Canadian Fire Weather Index, drought, summer fires, vapour pressure deficit, VPD, wind speed.
Introduction
Wildfires have greatly increased in size and frequency in recent decades throughout the western USA due to a combination of global changes including population growth, fire management practices and climate change (Abatzoglou and Williams 2016; Syphard et al. 2017; Liang and Hurteau 2023; MacDonald et al. 2023). Within regions such as California, the factors driving increased fire activity vary geographically (Keeley and Syphard 2017) and are often tied to patterns of ignitions (Peterson et al. 2011; Faivre et al. 2014; Syphard and Keeley 2015). Climate largely influences fire activity through its influence on biomass production and fuel aridity (Bradstock 2010; Jolly et al. 2015). There is evidence that global warming is exacerbating fire regimes leading to larger wildfires (Williams et al. 2019). However, anticipating future fire activity is complicated by the complexity and interactions between weather, vegetation, and people (Flannigan et al. 2009).
Understanding the future trajectory of fires is of extreme importance, but modelling studies yield varying indications regarding the extent and location of changes in future fire patterns. Findings indicate that the number and size of wildfires throughout the western USA are linked to the climate with variations in moisture availability being the key (Abatzoglou and Kolden 2013), and often reflected in patterns of vapour pressure deficit (VPD) (Williams et al. 2015). However, the predictors of large fires vary regionally (Stavros et al. 2014), and Brey et al. (2020) found that for the western USA summer fires, other climate and weather parameters were more useful than VPD.
Complicating our understanding of the role of how climate and weather impact fire behaviour is the observation that antecedent weather a week or more before the date of ignition, as well as weather conditions for a week or more afterward, are linked to many large fire events (Abatzoglou and Kolden 2011; Barbero et al. 2014; Stavros et al. 2014). One study for large fires across the western USA found that conditions on the day of ignition were correlated with peak fire daily growth but not final size or duration (Potter 2018b). Climate and weather before (Cayan et al. 2022) and after ignition in particular are often critical in determining the rate of growth and ultimately fire size (Potter 2023).
Both climate changes and localised weather conditions play a role in determining fire occurrence and behaviour, and indices for predicting fires have been an important means of preparing for fire events since the early part of the 20th century (Hardy and Hardy 2007). Numerous fire danger indices have been developed with varying success. This is likely due to the considerable regional variation exhibited by drivers of fire activity (Mees and Chase 1991; Stavros et al. 2014). For example, in southern California it was found that simple weather parameters such as temperature, relative humidity and wind alone were better predictors of fire size than more complex burn indices (Schoenberg et al. 2007; Madadgar et al. 2020). Furthermore, linear models demonstrate that autumn precipitation is significantly tied to the number of autumn fires, but the prior year spring precipitation is a better predictor of area burned (Keeley 2004). Taking an alternative lumped approach, it has been shown that the timing and amount of autumn precipitation plays a critical role in area burned by large (>100 ha) autumn and early winter fires, with 89% of the area burned occurring from fires that started before the onset of significant precipitation (Cayan et al. 2022).
Another fire risk metric is the Haines Index that has been widely utilised, but has a number of limitations including the large spatial variation in this index across regions (Winkler et al. 2007) and some consider it to be of limited value (Lu et al. 2011; Potter 2018a). The Canadian Fire Weather Index (CFWI) has proven useful for predicting North American boreal forest fires (Stocks et al. 1989; Waddington et al. 2012) as well as fires on other northern hemisphere continents (e.g. Viegas et al. 1999; Dimitrakopoulos et al. 2011; Tian et al. 2011). CFWI uses hourly measures of temperature, precipitation, relative humidity and wind speed to produce several metrics of daily conditions assumed to be associated with extreme fire behaviour. One of these metrics is the Fire Weather Index (FWI), which, when coupled with fuel type, is a quantitative measure of expected fire intensity and is widely used as a general index of fire danger. Another commonly used metric is the Fine Fuel Moisture Code (FFMC) that is a rating of the moisture content of litter and other fine fuels, indicating the relative ease of ignition and flammability of fine fuels, with increasing fire danger as FFMC increases.
In southern California the most damaging fires are those that occur during autumn and winter Santa Ana Wind (SAW) events, although most non-SAW fires occur at other times of the year (Jin et al. 2015; Syphard et al. 2021). SAWs are foehn-type winds that are annual events in southern California, and similar winds are associated with extreme fires in north coastal California (Nauslar et al. 2018) and in Oregon (Abatzoglou et al. 2021). Recent climate model projections suggest that SAW activity may diminish somewhat in future decades (Pierce et al. 2018; Guzman‐Morales and Gershunov 2019), but these event-specific effects may be counteracted by warming effects and generally drier autumn climate that could increase SAW fires, based on the CFWI (Goss et al. 2020). Further complicating the understanding of how SAW winds impact fires is the observation that different synoptic weather conditions can result in hot SAWs or cold SAWs (Gershunov et al. 2021), and evidence of increasing frequency of conditions conducive to hazardous fires during winter months (Guirguis et al. 2023), both of which have the potential for affecting wildfire activity. However, these climatic influences are best evaluated alongside other over-riding factors such as ignition sources (Keeley et al. 2021), and thus there is need for a more thorough understanding of the reliability of this index for predicting fire events in southern California for both SAW and non-SAW fires. It seems likely they differ given that these two types of fires occur at different times of the year.
This study examines the value of these indices in predicting fire outcomes for both Santa Ana Wind (SAW) driven fires (defined as a fire that ignited on the day of a SAW event) and fires that occur on other days (non-SAW). In addition, we examine the independent effect of relative humidity, mean daily wind speed, and the vapour pressure deficit (VPD), plus antecedent seasonal and annual drought events with data from the Palmer Drought Severity Index (PDSI) (Dai 2011). For Santa Ana Wind days we investigated the fire activity relative to the SAW Regional Index (SAWRI), a metric for the regional mean wind speed during periods of consistent northeasterly winds (Guzman‐Morales et al. 2016).
Methods
Our focus was on the southern California region defined by Guzman‐Morales et al. (2016) in generating the SAW Regional index. We utilised weather station data for four stations in four southern California counties (Fig. 1) that had a long history of hourly climate data (Desert Research Institute; https://wrcc.dri.edu/Projects/data.php). The two stations with the longest record (1950–2020), were San Diego International Airport in San Diego County and Los Angeles International Airport in Los Angeles County. Two other stations with a more limited records (1998–2020) were selected specifically because they are more interior sites; they were the Riverside Municipal Airport in Riverside County and the Chino Airport in San Bernardino County. All of these stations are potentially affected by Santa Ana Wind (SAW) corridors - from north to south: Newhall Pass, Cajon Pass, Banning Pass, and the Hwy 8 Corridor (Moritz et al. 2010; Rolinski et al. 2019).
South coast region in this study and the four climate stations distributed across four counties, and perimeters for fires over 10,000 ha; yellow for non-SAW fires and dots for SAW fires. National Oceanographic and Atmospheric Administration California Division 6.
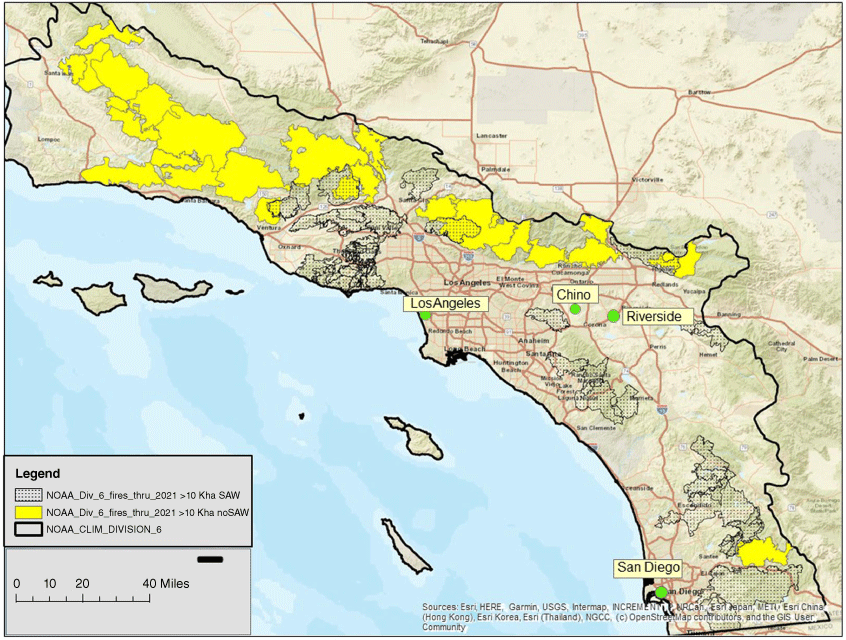
Hourly data for temperature, precipitation, relative humidity, and wind speed were used in calculating the CFWI fire weather indices as described by Van Wagner (1987) using an R package outlined in Wang et al. (2017). The FWI is a numeric rating of fire intensity that is commonly used as a general index of fire danger; the FFMC is a numeric rating of the moisture content of fine fuels and is an indicator of ignition and flammability; and the Drought Code (DC), fuel moisture measure.
The Palmer Drought Severity Index (PDSI) for the NOAA Region 6, which is approximately comparable with the region Guzman‐Morales et al. (2016) used to generate the SAW Regional Index (SAWRI) for, was obtained from the National Center for Atmospheric Research (NCAR) (https://climatedataguide.ucar.edu/climate-data/palmer-drought-severity-index-pdsi#, accessed 1 January 2023) for the years 1950–2020.
SAW days for 1950–2018 were from Guzman‐Morales and Gershunov (2019), based on the daily downscaling of the National Center for Environmental Prediction/National Center for Atmospheric Research (NCEP/NCAR) Global Reanalysis 1. The SAW Regional Index (SAWRI) was calculated as the regional mean wind speed during periods of consistent northeasterly winds over the southern California region. These data were available through 2018. For the years 2019–2020, SAWRI was computed as the maximum windspeed for periods with consistent northeasterly winds, as described by Rolinski et al. (2019).
Fire history included ignitions from Santa Barbara to San Diego counties for the years 1950–2020 from the CAL FIRE FRAP fire history database (http://frap.fire.ca.gov/data/frapgisdata). This region is comparable to the NOAA South Coast Division 6, although not directly overlapping the SAWRI area as defined by Guzman‐Morales and Gershunov (2019). Atmospheric circulation patterns that characterise SAW days have imposed a much larger footprint that includes much of Division 6 (Cayan et al. 2022). FRAP is a spatially explicit database that provides day of ignition, final fire size and cause, and is relatively complete for this time period for fires >40 ha, though a number of additions and corrections were made as described in Keeley et al. (2021). Here we define SAW fires as those that ignited on a day with a SAWRI >1. This differs from the criterion used by Cayan et al. (2022) where a SAW day was defined as one that registered a SAWRI >0.5, and the day before and the day after registered SAWRI >0. Comparing these approaches showed that this definition included 2% more SAW fires and 5% more area burned by SAW fires. Although SAW fires and their area burned are defined by a SAW event on the date of ignition, many SAW fires continue burning for days after ignition and sometimes after the SAW event is over. Sorting out the proportion of area burned on SAW vs non-SAW days is not possible with the FRAP database. Even if one could, it would be complicated by the observation that SAW related characteristics such as extremely low relative humidity and high temperatures, due to the dry adiabatic warming, continue for days after the northeastern winds have subsided (Keeley et al. 2009).
Results
SAW vs non_SAW fires
Over the period from 1950 to 2020, there were 3219 Santa Ana Wind (SAW) days, and fires ignited on 12% of those days. Over this same period, there were 22,704 non-SAW days, and 12% of those days had ignitions. Total area burned from ignitions on SAW days was 1.461 million ha and on non-SAW days 2.103 million ha. Distribution of fires by size classes showed that the majority (62%) of fires smaller than 100 ha were during non-SAW days (Supplementary Table S1). Although there was an order of magnitude more fires ignited on non-SAW days, the majority (56%) of fires over 50,000 ha started on SAW days, and these included the three largest fires. The largest single ignition was the Thomas Fire ignited on 4 December 2017 that burned 114,082 ha; however, four fires ignited on the day of the 25 October 2003 SAW event burned a substantially greater area of 194,311 ha due to the Cedar (109,546 ha), Simi (43,533 ha), Old (37,000 ha), and Padua (4232 ha) fires.
These large fires occurred during the last couple of decades, although during the whole period of record from 1950 to 2020, there was a highly significant (P < 0.001) decline in mean and median fire size due to increasing numbers of smaller fires on both SAW and non-Saw days (Supplementary Fig. S1). Although there was a highly significant drop over time in fire size, variability within a year was huge and so variance in fire size was large, accounting for the very low r2 (r2 = 0.08 for both SAW and non-SAW). Comparing the first half of the record (1950–1984) with the second half (1985–2020) showed that the total area burned for both types of fires increased in the second half of the record and the mean fire size for non-SAW fires was significantly greater in the second half of the record (Table 1). Also, the ignition sources changed over the early vs late periods. During the last 35 years, there was a marked increase in the known cause of fires. In the past 36 years, a major cause of area burned by fires ignited on non-SAW days was lightning, however, this was not a cause of fires on SAW days. During the recent period for non-SAW and SAW fires, equipment use and arson were important ignition sources. Powerline failures accounted for the bulk of area burned on SAW days but was a minor cause on non-SAW days.
Non-SAW | SAW | ||||||
---|---|---|---|---|---|---|---|
1950–1984 | 1985–2020 | 1950–1984 | 1985–2020 | ||||
N | 11,239 | 11,509 | 1540 | 1635 | |||
Total area burned (ha) | 803,470 | 1,299,600 | 593,800 | 867,900 | |||
Mean fire size (ha) | 71 | 112 | P = 0.020 | 385 | 531 | P = 0.451 | |
Unknown ignitions (%) | 48 | 12 | 45 | 4 | |||
Lightning (%) | 4 | 22 | 0 | 0 | |||
Equipment use (%) | 3 | 22 | <1 | 24 | |||
Debris burning (%) | 6 | 46 | 25 | 1 | |||
Arson (%) | 6 | 18 | 14 | 18 | |||
Powerline (%) | 4 | 7 | 22 | 45 |
Non-SAW fires occurred in all months of the year, peaking in June through September, whereas SAW fires were rare in March through June, absent in July and August, but peaked in October through December (Fig. 2a, c). The bulk of the area burned by fires ignited on non-SAW days was during the months of June to September (Fig. 2b), whereas on SAW days it was September to December (Fig. 2d), with substantially greater area burned in October than other months. September was a month of substantial burning that occurred more or less equally on SAW and non-SAW days. In addition to being temporally separated, non-SAW and SAW fires tended to be somewhat different in their spatial distribution, with more area burned from large non-SAW fires in more interior areas than large SAW fires (Fig. 1).
Fire weather indices
Considering only those days when an ignition occurred, there was a highly significant relationship between the FWI and fire size for non-SAW days at all four climate stations (Fig. 3a–d); however, due to the extreme range in fire sizes in all years, the variance (r2) explained by these models was low, i.e. the FWI had limited predictive value for fire size. For SAW days, there was also a significant relationship between FWI and fire size, and the r2 value was slightly higher for the two interior stations (Fig. 3f, g).
Individual fires plotted by size for levels of FWI at the four climate stations; adjusted r2 for bivariate regression of log ha and FWI. (a–d) Non-SAW fires in blue for the four climate stations, and (e–h) SAW in red for the four stations.
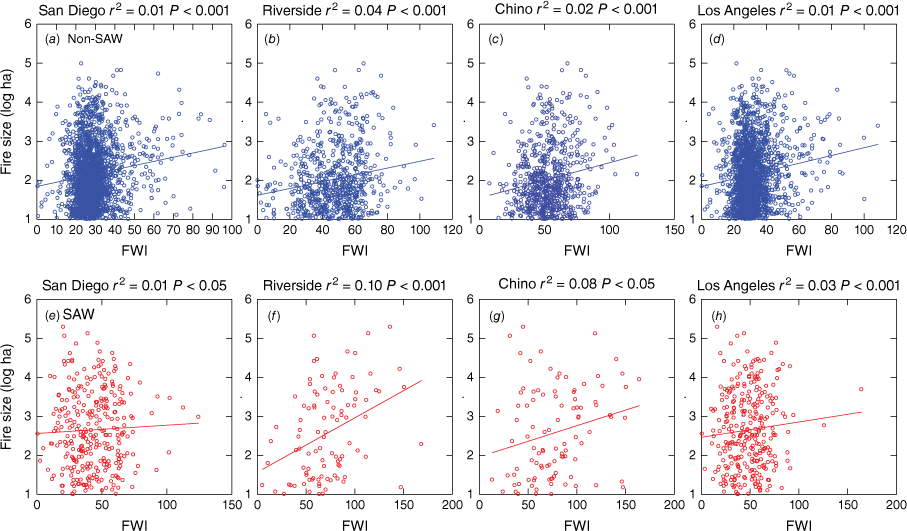
The Fine Fuel Moisture Content (FFMC) for ignitions on non-SAW days was significantly related to fire size at all stations (Fig. 4a–d), but for SAW days, it was significant at only two stations (Fig. 4e–h). The Drought Code (DC) was significantly related to fire size ignited on non-SAW days at three stations, and slightly significant on SAW days at three stations (Fig. 5).
Individual fires plotted by size for levels of FFMC at the four climate stations; adjusted r2 for bivariate regression of log ha and FFMC. (a–d) Non-SAW in blue for the four stations, and (e–h) SAW in red for the four stations.
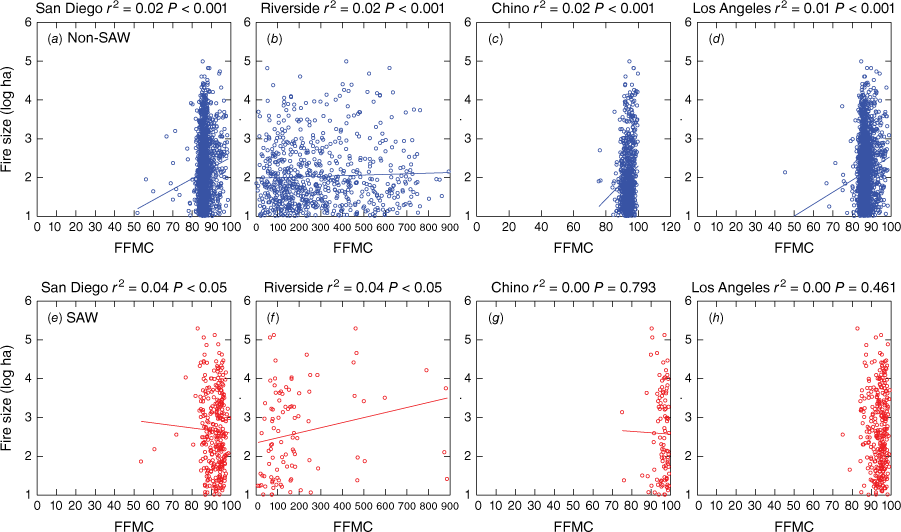
Individual fires plotted by size for levels of DC at the four climate stations; adjusted r2 for bivariate regression of log ha and DC. (a–d) Non-SAW in blue for the four stations, and (e–h) SAW in red for the four stations.
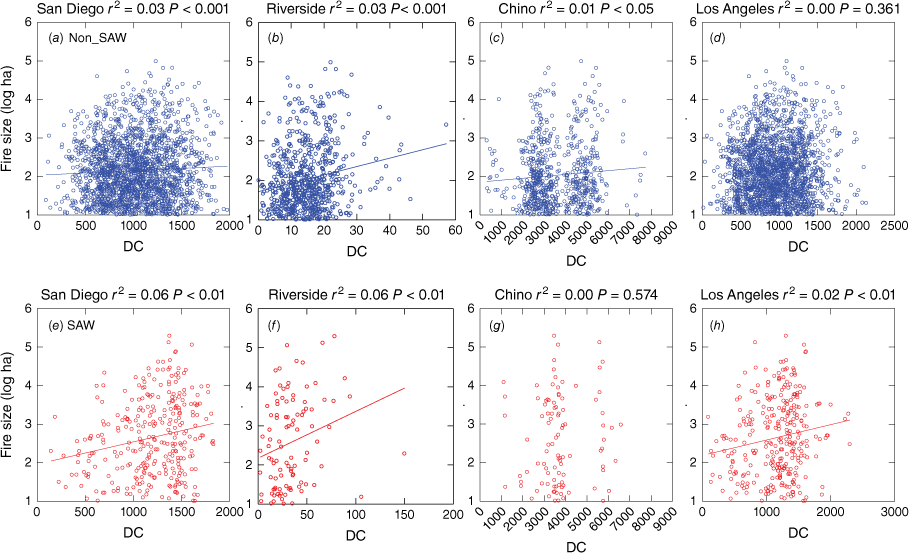
To examine these CFWI parameters at a finer temporal scale we investigated FWI, FFMC and DC by month for both non-SAW and SAW days at all four climate stations. First, using a two-sample t-test, we asked is there a significant difference in these two indices for days when no fire was initiated and those days when a fire was ignited (Supplementary Table S2). The pattern was similar across all four climate stations. FWI was significantly different on days with a fire in all months for non-SAW fires, and for the months October–February for SAW fires. The FFMC was also significantly different on days with fire relative to non-fire days, however, during the months of greatest area burned for non-SAW (June–September) and SAW (October–December) fires, the difference was only around 1–2%. The DC showed some significant differences between fire and non-fire days but not in most of the months when the greatest area was burned.
We then asked the question does the FWI, FFMC or DC provide a clue as to whether fires will be large (>10,000 ha) or small (<1000 ha), and using a two-sample t-test, the answer was no. For all four climate stations for the months with the highest area burned there was no significant difference in the FWI, FFMC and DC between large and small fires as defined here (data not shown).
When we focused on megafires (>25,000 ha) relative to much smaller fires (100–999 ha) for the two long term data sets at Los Angeles and San Diego (Table 2), these indices did illustrate some useful associations. As part of our analysis, we investigated these indices on the day of ignition, and the average for the week prior to ignition, and the week after ignition. For non-SAW days these indices did not provide much of an indicator of megafires. However, as shown in Table 2, for SAW days there were many indicators using the index on the day of ignition, the week before and the week after.
FWI | FFMC | DC | |||||
---|---|---|---|---|---|---|---|
−Week | +Week | −Week | +Week | −Week | +Week | ||
San Diego | |||||||
Month | Non-SAW | Non-SAW | Non-SAW | ||||
7 | NS | NS | NS | NS | NS | NS | |
8 | NS | 5* | NS | 5* | NS | NS | |
9 | NS | NS | NS | NS | NS | NS | |
SAW | SAW | SAW | |||||
9 | NS | NS | NS | NS | NS | NS | |
10 | NS | NS | NS | NS | 1*2*3*5*wk* | 2*3*4*wk* | |
11 | 0*** | 0***5* | 0***1***5*** | 0***1***5*** | NS | NS | |
12 | 1**5* | NS | 1** | 1** | NS | NS | |
Los Angeles | |||||||
Month | Non-SAW | Non-SAW | Non-SAW | ||||
7 | NS | NS | NS | NS | NS | NS | |
8 | NS | NS | NS | NS | NS | NS | |
9 | NS | NS | NS | NS | NS | NS | |
SAW | SAW | SAW | |||||
9 | NS | 1*2*3*wk* | NS | 1*2*wk* | 0*1*2*3*4*5*wk* | 0*1*2*3*4*5*wk* | |
10 | 1***2*wk** | NS | NS | NS | 0*1*2*3*4*5*wk* | 0*1*2*3*4*5*wk* | |
11 | 1** | 2***4**5 wk** | 1*** | 2*3*4**5***wk* | NS | NS | |
12 | 1* | 1**2**3***wk** | NS | 1*2*3***wk* | NS | NS |
Other climate and weather factors
Climate and weather parameters beyond the CFWI metrics were compared using a two sample t-test between days without and with fire for both non-SAW and SAW days (Table 3). For non-SAW days there was generally a highly significant difference in temperature. This was not always the case with SAW days. Daily average windspeed was generally not significantly different on days when fires ignited on both non-SAW and SAW days.
Temperature | Wind speed | VPD | |||||||||||||||||
---|---|---|---|---|---|---|---|---|---|---|---|---|---|---|---|---|---|---|---|
Non-SAW | SAW | Non-SAW | SAW | Non-SAW | SAW | ||||||||||||||
Month | No-fire | Fire | No-fire | Fire | No-fire | Fire | No-fire | Fire | No-fire | Fire | No-fire | Fire | |||||||
San Diego | |||||||||||||||||||
1 | 16.5 | 18.7 | * | 18.7 | 20.7 | ** | NS | NS | 0.9 | 1.3 | * | 1.4 | 1.8 | ** | |||||
2 | 16.9 | 18.9 | * | 19.6 | 21.6 | * | NS | 14.0 | 15.8 | * | 0.8 | 1.2 | * | 2.0 | 1.5 | * | |||
3 | 17.5 | 20.5 | *** | 20.4 | 24.2 | ** | NS | NS | 0.9 | 1.4 | *** | 1.5 | 2.4 | * | |||||
4 | 18.5 | 21.5 | *** | NS | NS | NS | 0.9 | 1.5 | *** | NS | |||||||||
5 | 19.2 | 20.8 | *** | NS | NS | NS | 0.9 | 1.1 | *** | NS | |||||||||
6 | 20.4 | 21.8 | *** | . | . | . | NS | . | . | . | 0.8 | 1.0 | *** | . | . | . | |||
7 | 22.7 | 23.5 | *** | . | . | . | 16.5 | 17 | ** | . | . | . | 0.9 | 1.0 | *** | . | . | . | |
8 | 23.7 | 24.4 | *** | . | . | . | NS | . | . | . | 1.0 | 1.1 | *** | . | . | . | |||
9 | 23.3 | 25.0 | *** | NS | NS | NS | 1.1 | 1.3 | *** | NS | |||||||||
10 | 21.8 | 23.8 | *** | 23.7 | 25.4 | *** | NS | NS | 1.1 | 1.5 | *** | 1.8 | 2.3 | *** | |||||
11 | 19.4 | 21.6 | *** | 21.2 | 23.8 | *** | NS | NS | 1.0 | 1.5 | *** | 1.7 | 2.3 | *** | |||||
12 | 17.0 | 19.1 | *** | 18.4 | 21.2 | *** | NS | NS | 0.9 | 1.3 | ** | 1.3 | 2.0 | *** | |||||
Riverside | |||||||||||||||||||
1 | NS | NS | NS | 13.7 | 22.3 | ** | NS | 1.9 | 2.3 | ** | |||||||||
2 | 16.6 | 20.8 | ** | NS | NS | NS | 1.1 | 1.9 | ** | NS | |||||||||
3 | 19.3 | 22.9 | *** | 22.6 | 26.9 | * | NS | NS | 1.4 | 2.0 | ** | 2.3 | 3.2 | * | |||||
4 | 21.5 | 25.9 | *** | NS | NS | NS | 1.7 | 2.7 | *** | NS | |||||||||
5 | 23.5 | 27.2 | *** | NS | 11.8 | 13.1 | * | NS | 1.8 | 2.7 | *** | NS | |||||||
6 | 27.1 | 29.6 | *** | . | . | . | 11.9 | 12.8 | * | . | . | . | 2.3 | 2.8 | *** | . | . | . | |
7 | 31.1 | 32.8 | *** | . | . | . | NS | . | . | . | 3 | 3.5 | *** | . | . | . | |||
8 | 31.9 | 33.4 | *** | . | . | . | NS | . | . | . | 3.3 | 3.7 | *** | . | . | . | |||
9 | 29.9 | 32.2 | *** | NS | NS | NS | 3.1 | 3.6 | *** | NS | |||||||||
10 | 25.3 | 28.1 | *** | NS | 9.0 | 11.4 | ** | 12.4 | 18.4 | ** | 2.3 | 3.0 | *** | 3.1 | 3.6 | * | |||
11 | 20.8 | 24.7 | *** | 22.8 | 25.9 | ** | NS | NS | 1.7 | 2.5 | *** | 2.3 | 3 | ** | |||||
12 | 16.5 | 19.5 | * | 18.8 | 20.3 | * | NS | 14.5 | 19.9 | * | 1.1 | 1.7 | * | 1.8 | 2 | * | |||
Chino | |||||||||||||||||||
1 | NS | 20.2 | 22.5 | * | NS | NS | NS | 1.8 | 2.3 | ** | |||||||||
2 | 17.0 | 21.1 | ** | 21.4 | 24.3 | * | 10.6 | 14.3 | * | NS | 1.1 | 1.8 | ** | 2.1 | 2.7 | * | |||
3 | 19.6 | 23.3 | ** | 23.1 | 27.3 | * | NS | NS | 1.4 | 2.0 | ** | NS | |||||||
4 | 22.0 | 26.9 | *** | NS | NS | NS | 1.7 | 2.9 | *** | NS | |||||||||
5 | 24.3 | 27.9 | *** | NS | NS | NS | 1.9 | 2.7 | *** | NS | |||||||||
6 | 27.6 | 30.1 | *** | . | . | . | NS | . | . | . | 2.3 | 3.0 | *** | . | . | . | |||
7 | 31.6 | 33.6 | *** | . | . | . | 14.2 | 15 | * | . | . | . | 3.0 | 3.6 | *** | . | . | . | |
8 | 32.7 | 34.1 | *** | . | . | . | NS | . | . | . | 3.4 | 3.8 | *** | . | . | . | |||
9 | 30.7 | 33.3 | *** | NS | NS | NS | 3.1 | 3.9 | *** | NS | |||||||||
10 | 26.1 | 29.1 | *** | NS | NS | 13.9 | 19.3 | ** | 2.3 | 3.2 | *** | NS | |||||||
11 | 21.3 | 25.2 | *** | 23.3 | 27.2 | ** | NS | NS | 1.7 | 2.5 | *** | 2.3 | 3.2 | *** | |||||
12 | 16.9 | 20.5 | *** | 19 | 21.6 | *** | NS | 13.6 | 19.3 | * | 1.1 | 1.8 | ** | 1.7 | 2.2 | *** | |||
Los Angeles | |||||||||||||||||||
1 | 16.0 | 19.4 | * | 19.3 | 21.8 | *** | 11.9 | 12.6 | NS | 10.2 | 11.6 | NS | 0.8 | 1.6 | ** | 1.6 | 2.1 | *** | |
2 | 16.4 | 19.1 | ** | 20.2 | 22.9 | ** | 14.7 | 13.7 | NS | 12.4 | 10.8 | NS | 0.8 | 1.3 | ** | 1.6 | 2.2 | *** | |
3 | 17.1 | 19.8 | *** | 20.9 | 23.8 | * | 17.8 | 16.1 | * | 15.3 | 20.9 | NS | 0.8 | 1.3 | ** | 1.7 | 2.3 | NS | |
4 | 18.3 | 21.2 | *** | 23.4 | 27.6 | NS | 19.7 | 20.3 | NS | 19.7 | 17.7 | NS | 0.9 | 1.4 | *** | 2.1 | 3.0 | NS | |
5 | 19.4 | 21.1 | *** | 27.1 | 25.8 | NS | 19.4 | 19.5 | NS | . | . | 0.9 | 1.2 | *** | 2.0 | 2.4 | NS | ||
6 | 20.7 | 21.9 | *** | . | . | . | 18.4 | 18.8 | NS | . | . | . | 0.9 | 1.1 | *** | . | . | . | |
7 | 22.9 | 23.4 | *** | . | . | . | 18.1 | 18.6 | ** | . | . | . | 1.1 | 1.2 | *** | . | . | . | |
8 | 23.5 | 24.0 | *** | . | . | . | 18.3 | 18.3 | NS | . | . | . | 1.1 | 1.3 | *** | . | . | . | |
9 | 23.0 | 24.5 | *** | 26.9 | 29.5 | NS | 17.6 | 17.7 | NS | 18.7 | 17.3 | NS | 1.1 | 1.5 | *** | 2.7 | 3.3 | NS | |
10 | 21.6 | 23.7 | *** | 24.9 | 27.1 | *** | 16.4 | 16.7 | NS | 15.4 | 13.9 | * | 1.1 | 1.7 | *** | 2.2 | 3.0 | *** | |
11 | 19.3 | 21.8 | *** | 22.2 | 25.1 | *** | 13.9 | 13.9 | NS | 11.2 | 11.6 | NS | 1.1 | 1.7 | *** | 2.0 | 2.7 | *** | |
12 | 16.7 | 19.6 | *** | 18.9 | 21.7 | *** | 11.9 | 11.9 | NS | 10.7 | 11.6 | NS | 0.9 | 1.5 | ** | 1.5 | 2.1 | *** |
It is generally considered that one of the strongest drivers of fire activity in the western USA is the vapour pressure deficit (VPD), and this was significantly related to fire size on non-SAW days but not on SAW days (Fig. 6). However, on a monthly basis, VPD was significantly higher on days when fires ignited vs days with no fire for non-SAW in nearly all months; for SAW days, this was significant for both winter and autumn months (Table 3).
Individual fires plotted by size for levels of VPD at the four climate stations; adjusted r2 for bivariate regression of log ha and VPD. (a–d) Non-SAW in blue for the four stations, and (e–h) SAW in red for the four stations.
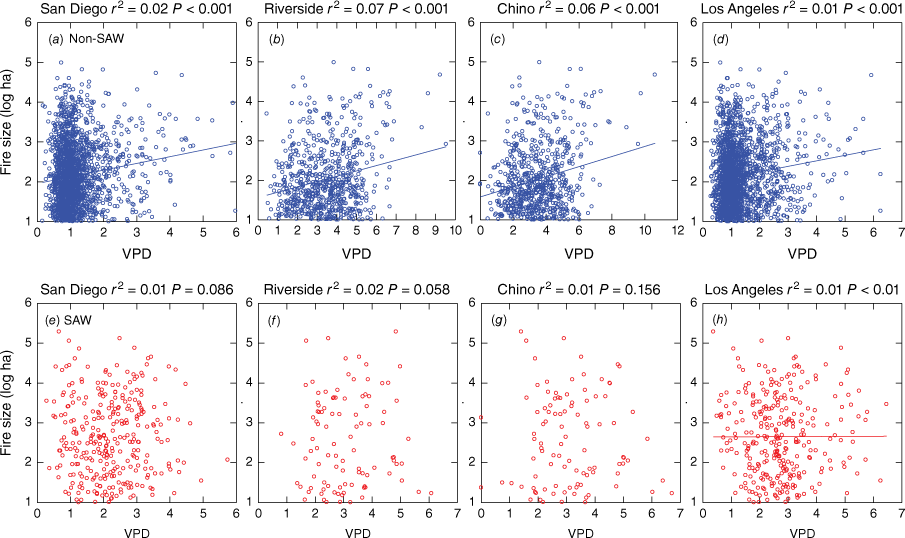
We addressed the question of whether average temperature, VPD and average wind speed differed on days when fire ignitions resulted in megafires (>25,000 ha) vs much smaller fires (100–999 ha) for the two long term data sets at San Diego and Los Angeles (Table 4). We investigated these indices for the day of ignition, the average for the week prior to ignition, and the week after ignition. The more southerly station, San Diego, showed no significant differences in temperature for the day of ignition or the week before or after for both non-SAW and SAW days. However, for Los Angeles for non-SAW days there was a highly significant difference for temperature the week prior to fires in July and the week after in September. On SAW days the only significant effect of temperature was in the week following ignition.
Temperature | Wind speed | VPD | |||||
---|---|---|---|---|---|---|---|
−Week | +Week | −Week | +Week | −Week | +Week | ||
San Diego | |||||||
Month | Non-SAW | Non-SAW | Non-SAW | ||||
7 | NS | NS | NS | 5** | NS | 5* | |
8 | NS | NS | 3*wk* | 1* | NS | NS | |
9 | NS | NS | NS | NS | wk* | NS | |
SAW | SAW | SAW | |||||
9 | NS | NS | NS | wk* | NS | NS | |
10 | NS | NS | NS | NS | 1* | NS | |
11 | NS | NS | NS | NS | 0***2* | NS | |
12 | NS | NS | NS | 5** | 1***5** | wk* | |
Los Angeles | |||||||
Month | Non-SAW | Non-SAW | Non-SAW | ||||
7 | 5*** | NS | NS | 3* | NS | NS | |
8 | NS | NS | NS | NS | NS | NS | |
9 | NS | wk** | 3**wk* | NS | NS | 4*5* | |
SAW | SAW | SAW | |||||
9 | NS | NS | NS | NS | NS | wk* | |
10 | NS | NS | NS | 2* | 1* | NS | |
11 | NS | 0***4*5** | NS | NS | 0***2* | 4**5*** | |
12 | NS | 3** wk* | NS | 2*4*** | NS | 3* |
In the week prior to ignition, average wind speed did show a significant effect on fires ignited on non-SAW days. but no such effect was found for fires ignited on SAW days. However, during the week following ignitions occurring on SAW days, average wind speed was significantly higher than the control group. Unlike this average wind speed from the four stations data, the SAWRI (the regional mean wind speed during periods of consistent northeasterly winds), was strongly tied to the fire size in some months, as determined by using t-tests comparing megafires (>25,000 ha) vs small fires (<1000 ha) (data not shown, but summarised here). For October (the month with the greatest area burned) (Fig. 2), there was a significant difference (P < 0.05) of the average SAWRI between small vs megafires for the week prior to ignition, a highly significant difference (P < 0.001) of the SAWRI on the day of ignition, and significant differences of SAWRI on the day after ignition (P < 0.05), and average over the week after (P < 0.01). For November and December the average of this index for the week after ignition was also significantly higher (P < 0.05) for megafires than small fires.
Annually, there are many SAW events without a fire ignition. Throughout the period 1950–2020, the probability of a fire during a SAW event (i.e. the number of SAW fires/SAW events) increased during this period with a P < 0.050 (r2 = 0.051). Thus, there was a significant trend in increasing likelihood of a fire during a SAW event, though the annual variability was huge, resulting in a rather low r2.
Vapour pressure deficit (VPD), which was significantly related to fire size (Fig. 6) on non-SAW days, showed only a slightly significant effect on megafires for the month of September (Table 4). Ironically, for SAW days when there was little relationship between VPD and fire size (Fig. 6), there was a significantly higher VPD before and after days of ignition for megafires (Table 4).
The effect of the Palmer Drought Severity Index (PDSI) on fire size, considering PDSI vs monthly area burned for non-SAW and SAW days is illustrated in Fig. 7. For fires ignited on non-SAW days, bivariate regression analysis showed PDSI explained a substantial amount of variation in area burned, particularly in the months of January and February. For fires ignited on SAW days, there were significant effects of PDSI on fire size for the months from October to January.
Monthly distribution from January to December of the adjusted r2 for bivariate regression of log ha and PDSI for each season, and average of annual PDSI plus PDSI for the prior year (2 years drought index), and prior 2 years (3 years drought), prior 3 years (4 years drought), and prior 4 years (5 years drought). *indicates P < 0.05, **P < 0.01, ***P < 0.001.
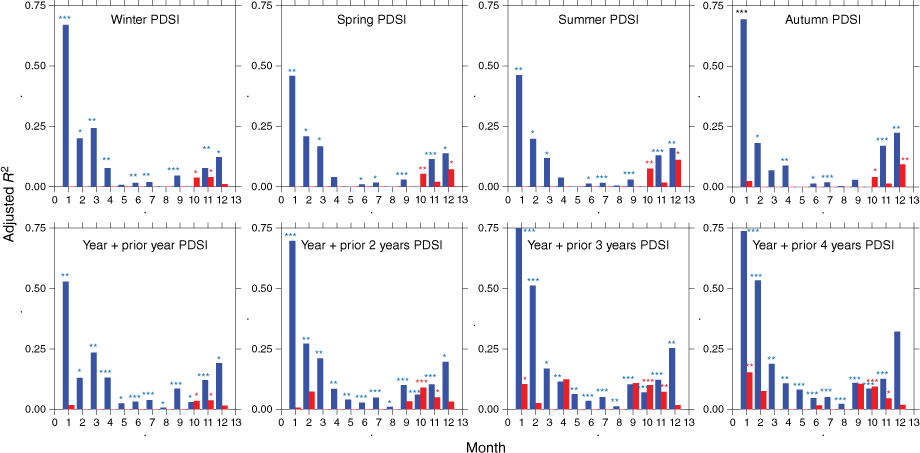
Regression models of log area burned (Table 5) were developed on a monthly basis using all of the variables examined in this study. For fires ignited on non-SAW days in months with substantial area burned, variance accounted for between 0.05 and 0.16. Across all months the most important driver of fire size from non-SAW day ignitions was the extent of drought (PDSI) in the prior 5 years. For SAW days during October, the month with the largest area burned, the model was highly significant with an r2 of 0.23 and the major driver was the SAWRI measure of wind speed. For the other 3 months with substantial area burned, the model for September did not yield significant results, but the models for November and December models were significant with the SAWRI also being the strongest driver.
Non-SAW | ||
---|---|---|
Month | ||
5 | Log ha burned = Drought prior 5 years + temperature (day 0); r2 = 0.12, P < 0.001 | |
6 | Log ha burned = Drought prior 5 years; r2 = 0.05, P < 0.001 | |
7 | Log ha burned = Drought prior 5 years + VPD (day 0); r2 = 0.07, P < 0.001 | |
8 | Log ha burned = Drought prior 5 years + VPD (day 0) + Sum PDSI, Spring PDSI; r2 = 0.06, P < 0.001 | |
9 | Log ha burned = Drought prior 5 years + VPD (day 0); r 2 = 0.16, P < 0.001 | |
10 | Log ha burned = Drought prior 5 years + VPD (day 0); r2 = 0.11, P < 0.001 |
SAW | ||
---|---|---|
Month | ||
10 | Log ha burned = R1Dindex (day 0) + Spring PDSI + Summer PDSI; r2 = 0.23, P < 0.001 | |
11 | Log ha burned = R1Dindex (day 0) + Drought prior 4years; r2 = 0.29, P < 0.001 | |
12 | Log ha burned = R1Dindex (day 0) + Spring PDSI; r2 = 0.11, P < 0.01 |
Discussion
In southern California SAW fires are responsible for the largest and most destructive wildfires (Syphard et al. 2021; Abatzoglou et al. 2023), however, as shown here, fires ignited on non-SAW days account for many more fires than SAW fires (seven times as many) and more (30%) area burned. The proportion of non-SAW to SAW fires has varied somewhat over time, with some studies reporting more equal levels of burning for these two types of fire (Jin et al. 2014, 2015; Kolden and Abatzoglou 2018). Of particular interest is that extreme SAW events are not a good predictor of extreme fire events since they are entirely dependent on the coincidence of an extreme event with a human ignition, most commonly a powerline failure (Keeley et al. 2021). As noted here, the probability of a SAW event leading to a fire has increased significantly over the last 71 years. Since these fires are dependent on human ignitions, it is possible this increase is due to increased population growth and associated increase in the electrical grid. Global warming could be an explanation for this pattern except that climate attributes are not strongly tied to SAW fires (Keeley et al. 2021).
Non-SAW fires are often referred to as summer fires as the bulk of area burned occurs during the summer months June–August; however, much more area burned in September is due to non-SAW fires than SAW fires. SAW fires dominate in October through December. Although the frequency of non-SAW fires tends to be greatest in more interior landscapes (Kolden and Abatzoglou 2018), the distribution of large fires over 10,000 ha shows the main differences to be that SAW fires dominate in the southern part of the southern California region and non-SAW fires in the northern portion (Fig. 1).
Predicting the likelihood of both fire types is critical to providing sufficient fire protection and it appears the best predictors differ between these two types of fires. The CFWI has been used to predict fire conditions for SAW fires (Goss et al. 2020), and here we evaluated several indices derived from the CFWI to assess their relative utility for predicting both non-SAW and SAW fires. The Fire Weather Index (FWI) is commonly used as a general index of fire danger, and although it is significantly related to fire size for both non-SAW and SAW days (Fig. 2), its utility for predicting fire size is limited. This is because both non-SAW and SAW fires vary by 4–5 orders of magnitude annually, and models derived from the FWI explain very little of this year-to-year variance. In other words, despite the statistical association, indices such as FWI provide limited predictive value in southern California (Schoenberg et al. 2007). On the other hand, for all months with substantial area burned (June–September for non-SAW fires, October–December for SAW fires), days with an ignition have a FWI significantly greater than non-fire days in that month. Thus, at a monthly temporal scale, the FWI is a reliable indicator of fire potential for both types of fire.
Of particular value to fire managers is the capacity to predict conditions likely to lead to large fires, which are defined and labelled differently by researchers; very large wildfires (VLWF), extreme wildfire events (EWE), fires of unusual size (FOUS), and megafires are examples (Stavros et al. 2014; Tedim et al. 2018; Potter 2023; Linley et al. 2022, respectively). We prefer the latter term, in part because it doesn’t carry an initialism. The threshold over which fires are categorised as large varies markedly with different regions, partially because the presence of contiguous fuels varies geographically and is an important contributor to potential fire size. Here we consider megafires as those >25,000 ha (61,775 ac), which in southern California is generally associated with the most destructive fires (Syphard et al. 2021). Conditions on the day of an ignition are important, but our results show that conditions in the days before and after ignition also need to be considered. For non-SAW fires, the FWI is of little value in discerning when fires would become megafires; however, FWI may be a good predictor of megafires during the SAW season (Table 2). In general, the FWI on the day of ignition is less useful than this index in the days before and after ignition.
The Fine Fuel Moisture Code (FFMC), is a numeric rating of the moisture content of dead fine fuels and is inversely tied to fire danger. This metric is significantly greater on days when a fire occurs, than on non-fire days, year round for non-SAW days and autumn and winter months for SAW days. In short, FFMC was greater on days when an ignition occurred; however, for most months the difference was rather subtle; typically just a few percent. As a metric for predicting megafires, it is of limited value for non-SAW days; however, late fall and early winter SAW fires showed this had a significant effect on megafires, and this is consistent with the role of precipitation on these fires (Cayan et al. 2022). It would seem that this effect is primarily on dead fine fuels as fuel moisture in live fuels in autumn is frequently at its lowest point for living chaparral biomass in most years (Keeley et al. 2009). FFMC in the days following SAW fire ignition has the most consistently significant effect.
The Drought Code (DC) as a predictor of fires varies with location and length of the record. The present study showed that on non-SAW days it was generally greater on days when an ignition occurred, but for SAW days that was only the case for autumn months in the long-term data sets from San Diego and Los Angeles, and not for the more recent years for Riverside and Chino. As a predictor of megafires it is not significant for fires that ignite on non-SAW days, but it is significant for fires that ignite on SAW days in early autumn months. Models using the Palmer Drought Severity Index (PDSI), and temperature, average wind speed and VPD showed that long term drought was the most important determinant of fire size on non-SAW days. For SAW days, drought was an important factor, but the greatest impact was the SAW Regional Index. In short, drought appears to be the most important factor for non-SAW days and Santa Ana wind speed for SAW days (Table 5).
Vapour pressure deficit (VPD), has been shown to be highly correlated with area burned in the southwest USA (Mueller et al. 2020; Balch et al. 2022). In southern California VPD appears to be linked to megafires in July and September for non-SAW days and October–December on SAW days, but is not the overriding factor (Table 5).
In summary, when using the CFWI to predict fire occurrence potential in southern California, the FWI may be the most useful for both SAW and non-SAW fires, although using it in combination with the FFMC and DC may provide a more robust prediction. None of the three indices were valuable for predicting fire size overall, nor were they helpful in predicting megafires on non-SAW days. However, all three showed some potential for predicting SAW megafires in some months, although the effect was greater for the days before and after a fire. Of course, if used to predict the potential for a megafire, the weeks before the event would be the most useful to monitor for fire danger. In addition, long term drought needs to be considered in evaluating fire danger and the potential for human ignitions (Keeley et al. 2021).
Conflicts of interest
Alexandra Syphard and Tim Brown are Associate Editors of International Journal of Wildland Fire. To mitigate this potential conflict of interest they didn’t have any editor-level access during the peer review process. The authors declare no other conflicts of interest.
Declaration of funding
This research was supported by the U.S. Geological Survey, Thompson Rivers University, Natural Resource Science, BC, Canada, Western Regional Climate Center, Reno, NV, Southern California Edison, Los Angeles, CA, Scripps Institution of Oceanography, University of California, San Diego, CA, and the Conservation Biology Institute, 136 SW Washington Ave, Corvalis, OR.
Acknowledgements
Any use of trade, firm or product names is for descriptive purposes only and does not imply endorsement by the U.S. Government. Thanks to Anne Pfaff for assistance with data acquisition and figures.
References
Abatzoglou JT, Kolden CA (2011) Relative importance of weather and climate on wildfire growth in interior Alaska. International Journal of Wildland Fire 20, 479-486.
| Crossref | Google Scholar |
Abatzoglou JT, Kolden CA (2013) Relationships between climate and macroscale area burned in the western United States. International Journal of Wildland Fire 22, 1003-1020.
| Crossref | Google Scholar |
Abatzoglou JT, Williams AP (2016) Impact of anthropogenic climate change on wildfire across western US forests. Proceedings of the National Academy of Sciences 113, 11770-11775.
| Crossref | Google Scholar | PubMed |
Abatzoglou JT, Juang CS, Williams AP, Kolden CA, Westerling AL (2021) Increasing synchronous fire danger in forests of the western United States. Geophysical Research Letters 48, e2020GL091377.
| Crossref | Google Scholar |
Abatzoglou JT, Kolden CA, Williams AP, Sadegh M, Balch JK, Hall A (2023) Downslope wind-driven fires in the western United States. Earth’s Future 5, e2022EF003471.
| Crossref | Google Scholar |
Balch JK, Abatzoglou JT, Joseph MB, Koontz MJ, Mahood AL, McGlinchy J, Cattau ME, Williams AP (2022) Warming weakens the night-time barrier to global fire. Nature 602, 442-448.
| Crossref | Google Scholar | PubMed |
Barbero R, Abatzoglou Jt, Steel EA, Larkin NK (2014) Modeling very large-fire occurrences over the continental United States from weather and climate forcing. Environmental Research Letters 9, 124009.
| Crossref | Google Scholar |
Bradstock RA (2010) A biogeographic model of fire regimes in Australia: current and future implications. Global Ecology and Biogeography 19, 145-158.
| Crossref | Google Scholar |
Brey SJ, Barnes EA, Pierce JR, Swann AL, Fischer EV (2020) Past variance and future projections of the environmental conditions driving western US summertime wildfire burn area. Earth’s Future 9, e2020EF001645.
| Crossref | Google Scholar | PubMed |
Cayan DR, DeHaan LL, Gershuvov A, Guzman-Morales J, Keeley JE, Mumford J, Syphard AD (2022) Autumn precipitation: the competition with Santa Winds in determining fire outcomes in southern California. International Journal of Wildland Fire 31, 1056-1067.
| Crossref | Google Scholar |
Dai A (2011) Characteristics and trends in various forms of the Palmer Derought Severity index during 1900-2008. Journal of Geophysical Research 116, D12115.
| Crossref | Google Scholar |
Dimitrakopoulos AP, Bemmerzouk AM, Mitsopoulos ID (2011) Evaluation of the Canadian fire weather index system in an eastern Mediterranean environment. Meteorological Applications 18, 83-93.
| Crossref | Google Scholar |
Faivre N, Jin Y, Goulden ML, Randerson JT (2014) Controls on the spatial pattern of wildfire ignitions in Southern California. International Journal of Wildland Fire 23, 799-811.
| Crossref | Google Scholar |
Flannigan MD, Krawchuk MA, de Groot WJ, Wotton BM, Gowman LM (2009) Implications of changing climate for global wildland fire. International Journal of Wildland Fire 18(5), 483-507.
| Crossref | Google Scholar |
Gershunov A, Guzman Morales J, Hatchett B, Guirguis K, Aguilera R, Shulgina T, Abatzoglou JT, Cayan D, Pierce D, Williams P, Small I, Clemesha R, Schwarz L, Benmarhnia T, Tardy A (2021) Hot and cold flavors of southern California’s Santa Ana winds: their causes, trends, and links with wildfire. Climate Dynamics 57, 2233-2248.
| Crossref | Google Scholar | PubMed |
Goss M, Swain DL, Abatzoglou JT, Sarhadi A, Kolden CA, Williams AP, Diffenbaugh NS (2020) Climate change is increasing the likelihood of extreme autumn wildfire conditions across California. Environmental Research Letters 15, 094016.
| Crossref | Google Scholar |
Guirguis KA, Gershunov A, Hatchett B, Shulgina T, DeFlorio MJ, Subramanian AC, Guzman Morales J, Aguilera R, Clemesha R, Corringham TW, Delle Monache L, Reynolds D, Tardy A, Small I, Ralph RM (2023) Winter wet–dry weather patterns driving atmospheric rivers and Santa Ana winds provide evidence for increasing wildfire hazard in California. Climate Dynamics 60, 1729-1749.
| Crossref | Google Scholar |
Guzman‐Morales J, Gershunov A, Theiss J, Li H, Cayan D (2016) Santa Ana Winds of Southern California: Their climatology, extremes, and behavior spanning six and a half decades. Geophysical Research Letters 43, 2827-2834.
| Crossref | Google Scholar |
Guzman‐Morales J, Gershunov A (2019) Climate change suppresses Santa Ana winds of Southern California and sharpens their seasonality. Geophysical Research Letters 46, 2772-2780.
| Crossref | Google Scholar |
Hardy CC, Hardy CE (2007) Fire danger rating in the United States of America: an evolution since 1916. International Journal of Wildland Fire 16, 217-231.
| Crossref | Google Scholar |
Jin Y, Randerson JT, Faivre N, Capps S, Hall A, Goulden ML (2014) Contrasting controls on wildland fires in Southern California during periods with and without Santa Ana winds. Journal of Geophysical Research: Biogeosciences 119, 432-450.
| Crossref | Google Scholar |
Jin Y, Goulden ML, Faivre N, Veraverbeke S, Sun F, Hall A, Hand MS, Hook S, Randerson JT (2015) Identification of two distinct fire regimes in Southern California: implications for economic impact and future change. Environmental Research Letters 10, 094005.
| Crossref | Google Scholar |
Jolly WM, Cochrane MA, Freeborn PH, Holden ZA, Brown TJ, Williamson GJ, Bowman DM (2015) Climate-induced variations in global wildfire danger from 1979 to 2013. Nature communications 6, 7537.
| Crossref | Google Scholar | PubMed |
Keeley JE (2004) Impact of antecedent climate on fire regimes in coastal California. International Journal of Wildland Fire 13, 173-182.
| Crossref | Google Scholar |
Keeley JE, Syphard AD (2017) Different historical fire-climate relationships in California. International Journal of Wildland Fire 26, 253-268.
| Crossref | Google Scholar |
Keeley JE, Safford H, Fotheringham CJ, Franklin J, Moritz M (2009) The 2007 southern California wildfires: lessons in complexity. Journal of Forestry 107, 287-296.
| Crossref | Google Scholar |
Keeley JE, Guzman-Morales J, Gershunov A, Syphard AD, Cayan D, Pierce DW, Flannigan M, Brown TJ (2021) Ignitions explain more than temperature or precipitation in driving Santa Ana wind fires. Science Advances 7, eabh2262.
| Crossref | Google Scholar | PubMed |
Kolden CA, Abatzoglou JT (2018) Spatial Distribution of Wildfires Ignited under Katabatic versus Non-Katabatic Winds in Mediterranean Southern California USA. Fire 1, 19.
| Crossref | Google Scholar |
Liang S, Hurteau MD (2023) Novel climate–fire–vegetation interactions and their influence on forest ecosystems in the western USA. Functional Ecology 37, 2126-2142.
| Crossref | Google Scholar |
Linley GD, Jolly CJ, Doherty TS, Geary WL, Armenteras D, Belcher CM, et al. (2022) What do you mean,‘megafire’? Global Ecology and Biogeography 31, 1906-1922.
| Crossref | Google Scholar |
Lu W, Charney JJ, Zhong S, Bian X, Liu S (2011) A North American regional reanalysis climatology of the Haines Index. International Journal of Wildland Fire 20, 91-103.
| Crossref | Google Scholar |
MacDonald GT, Wall T, Enquist CAF, LeRoy SR, et al. (2023) Drivers of California’s changing wildfires: a state-of-the-knowledge synthesis. International Journal of Wildland Fire 32, 1039-1058.
| Crossref | Google Scholar |
Madadgar S, Sadegh M, Chiang F, Ragno E, AghaKouchak A (2020) Quantifying increased fire risk in California in response to different levels of warming and drying. Stochastic Environmental Research and Risk Assessment 34, 2023-2031.
| Crossref | Google Scholar |
Mees R, Chase R (1991) Relating burning index to wildfire workload over broad geographic areas. International Journal of Wildland Fire 1, 235-238.
| Crossref | Google Scholar |
Moritz MA, Moody TJ, Krawchuk MA, Hughes M, Hall A (2010) Spatial variation in extreme winds predicts large wildfire locations in chaparral ecosystems. Geophysical Research Letters 37(4), L04801.
| Crossref | Google Scholar |
Mueller SE, Thode AE, Margolis EQ, Yocom LL, Young JD, Iniguez JM (2020) Climate relationships with increasing wildfire in the southwestern US from 1984 to 2015. Forest Ecology and Management 460, 17861.
| Crossref | Google Scholar |
Nauslar NJ, Abatzoglou JT, Marsh PT (2018) The 2017 North Bay and Southern California fires: a case study. Fire 1, 18.
| Crossref | Google Scholar |
Peterson SH, Moritz MA, Morais ME, Dennison PE, Carlson JM (2011) Modelling long-term fire regimes of southern California shrublands. International Journal of Wildland Fire 20, 1-16.
| Crossref | Google Scholar |
Pierce DW, Kalansky JF, Cayan D (2018) ‘Climate, drought, and sea level rise scenarios for California’s fourth climate change assessment.’ (California’s Fourth Climate Change Assessment, California Energy Commission and California Natural Resources Agency) Available at http://www.climateassessment.ca.gov/techreports/docs/20180827-Projections_CCCA4-CEC- 2018-006.pdf
Potter B (2018a) The Haines Index–it’s time to revise it or replace it. International Journal of Wildland Fire 27, 437-440.
| Crossref | Google Scholar |
Potter BE (2018b) Quantitative evaluation of the Haines Index’s ability to predict fire growth events. Atmosphere 9, 177.
| Crossref | Google Scholar |
Potter BE (2023) Examining the influence of mid-tropospheric conditions and surface wind changes on extremely large fires and fire growth days. International Journal of Wildland Fire 32, 777-795.
| Crossref | Google Scholar |
Rolinski T, Capps SB, Zhuang W (2019) Santa Ana winds: a descriptive climatology. Weather and Forecasting 34, 257-275.
| Crossref | Google Scholar |
Schoenberg FP, Chang CH, Keeley JE, Pompa J, Woods J, Xu H (2007) A critical assessment of the burning index in Los Angeles County, California. International Journal of Wildland Fire 16, 473-483.
| Crossref | Google Scholar |
Stavros EN, Abatzoglou J, Larkin NK, McKenzie D, Steel EA (2014) Climate and very large wildland fires in the contiguous western USA. International Journal of Wildland Fire 23, 899-914.
| Crossref | Google Scholar |
Stocks BJ, Lawson BD, Alexander ME, Wagner CV, McAlpine RS, Lynham TJ, Dube DE (1989) D.E., 1989. The Canadian forest fire danger rating system: an overview. Forestry Chronicle 65, 450-457.
| Crossref | Google Scholar |
Syphard AD, Keeley JE (2015) Location, timing and extent of wildfire vary by cause of ignition. International Journal of Wildland Fire 24, 37-47.
| Crossref | Google Scholar |
Syphard AD, Keeley JE, Pfaff AH, Ferschweiler K (2017) Human presence diminishes the importance of climate in driving fire activity across the United States. Proceedings of the National Academy of Sciences 114, 13750-13755.
| Crossref | Google Scholar | PubMed |
Syphard AD, Rustigian-Romsos H, Keeley JE (2021) Multiple-scale relationships between vegetation, the wildland-urban interface, and structure loss to wildfire in California. Fire 4, 12.
| Crossref | Google Scholar |
Tedim F, Leone V, Amraoui M, Bouillon C, Coughlan MR, Delogu GM, Fernandes PM, Ferreira C, McCaffrey S, McGee TK, Parente J (2018) Defining extreme wildfire events: difficulties, challenges, and impacts. Fire 1, 9.
| Crossref | Google Scholar |
Tian X, McRae DJ, Jin J, Shu L, Zhao F, Wang M (2011) Wildfires and the Canadian Forest Fire Weather Index system for the Daxing’anling region of China. International Journal of Wildland Fire 20, 963-973.
| Crossref | Google Scholar |
Viegas DX, Bovio G, Gerreira A, Nosenzo A, Sol B (1999) Comparative study of various methods of fire danger evaluation in southern Europe. International Journal of Wildland Fire 9, 235-246.
| Crossref | Google Scholar |
Waddington JM, Thompson DK, Wotton M, Quinton WL, Flannigan MD (2012) Examining the utility of the Canadian Forest Fire Weather Index System in boreal peatlands. Canadian Journal of Forest Research 42, 47-58.
| Crossref | Google Scholar |
Wang X, Wotton M, Cantin A, Parisien M-A, Anderson K, Moore B, Flannigan MD (2017) cffdrs: An R package for the Canadian Forest Fire Danger Rating System. Ecological Processes 6, 5.
| Crossref | Google Scholar |
Williams AP, Seager R, Abatzoglou JT, Cook BI, Smerdon JE, Cook ER (2015) Contribution of anthropogenic warming to California drought during 2012–2014. Geophysical Research Letters 42, 6819-6828.
| Crossref | Google Scholar |
Williams AP, Abatzoglou JT, Gershunov A, Guzman‐Morales J, Bishop DA, Balch JK, Lettenmaier DP (2019) Observed impacts of anthropogenic climate change on wildfire in California. Earth’s Future 7, 892-910.
| Crossref | Google Scholar |
Winkler JA, Potter BE, Wilhelm DF, Shadbolt RP, Piromsopa K, Bian X (2007) Climatological and statistical characteristics of the Haines Index for North America. International Journal of Wildland Fire 16, 139-152.
| Crossref | Google Scholar |