Performance of operational fire spread models in California
Adrián Cardil

A Technosylva Inc, La Jolla, CA, USA. Email: smonedero@tecnosylva.com, spurdy@technosylva.com, manavarrete@tecnosylva.com, rquilez@tecnosylva.com
B Department of Crop and Forest Sciences, University of Lleida, Lleida, Spain. Email: sergio.demiguel@udl.cat
C Joint Research Unit CTFC - AGROTECNIO - CERCA, Solsona, Spain.
D CAL FIRE Sacramento Headquarters Intel, Sacrimento, CA, USA. Email: phillip.selegue@fire.ca.gov, geoff.Marshall@fire.ca.gov, tim.chavez@fire.ca.gov
E USDA Forest Service, Pacific Southwest Region, Operations Southern California. Email: kristen.allison@usda.gov
F Forest Fire Laboratory, Department of Forest Engineering, University of Córdoba, Campus de Rabanales, 14071, Córdoba, Spain.
G Forest Biometrics and Remote Sensing Laboratory (Silva Lab), School of Forest, Fisheries and Geomatics Sciences, University of Florida, PO Box 110410, Gainesville, FL 32611, USA. Email: c.silva@ufl.edu
Abstract
Wildfire simulators allow estimating fire spread and behaviour in complex environments, supporting planning and analysis of incidents in real time. However, uncertainty derived from input data quality and model inherent inaccuracies may undermine the utility of such predictions.
We assessed the performance of fire spread models for initial attack incidents used in California through the analysis of the rate of spread (ROS) of 1853 wildfires.
We retrieved observed fire growth from the FireGuard (FG) database, ran an automatic simulation with Wildfire Analyst Enterprise and assessed the accuracy of the simulations by comparing observed and predicted ROS with well-known error and bias metrics, analysing the main factors influencing accuracy.
The model errors and biases were reasonable for simulations performed automatically. We identified environmental variables that may bias ROS predictions, especially in timber areas where some fuel models underestimated ROS.
The fire spread models’ performance for California is in line with studies developed in other regions and the models are accurate enough to be used in real time to assess initial attack fires.
This work allows users to better understand the performance of fire spread models in operational environments and opens new research lines to further improve the performance of current operational models.
Keywords: fire behaviour, fire simulation modelling, Rothermel, Wildfire Analyst.
Introduction
Wildfires are a natural disturbance that shape the ecosystems and landscapes (Pausas and Keeley 2009) although they are a growing threat to the environment, economical assets and population in many regions worldwide (Molina-Terrén et al. 2019). In fact, substantial amounts of financial resources have been invested in fire management aiming to reduce the damage associated with unintended consequences of wildfires and improve safety for the population (Liang et al. 2008; Cardil and Molina 2015; Stocks and Martell 2016). Fire agencies rely on suites of wildfire analysis products that are designed to meet the needs of operational response and mitigation planning. Given the challenges that first responders and decision-makers were faced with to adequately predict fire spread and potential impacts, and dispatch the most appropriate resources every time a wildfire is detected in California, California Department of Forestry and Fire Protection (CAL FIRE) (2019), through a request for innovative ideas in March 2019, selected Wildfire Analyst Enterprise (WFA-e) to evaluate initial attack fires and calculate wildfire risk based on automatic simulations. In this project, automatic wildfire spread simulations are conducted aiming to (1) forecast risk days in advance to support operational decision making (readiness levels, resource allocation and release of timely and effective public warnings for disaster management (Cruz et al. 2018; Monedero et al. 2019; Ramirez et al. 2019)); (2) quantify asset and territory risk to inform on the most effective mitigation and asset hardening options. However, fire analysts can also generate on-demand spread predictions in response to active incidents for safe fire suppression strategies and tactics. These simulations are usually performed manually, adjusting the input data and calibrating the models in real time to optimise the model’s performance (Cardil et al. 2019).
The wildfire rate of spread (ROS) is determined by fuel availability, topography and weather (Barrows 1951). Several mathematical approaches have been developed in the last decades aiming at predicting fire behaviour. However, semi-empirical approaches such as Rothermel’s model (Rothermel 1972) have been the most widely used and evaluated fire spread models on Earth owing to simplicity in understanding, use and computational efficiency (Cruz and Alexander 2013; Cruz et al. 2018; Ascoli et al. 2022; Minsavage-Davis and Davies 2022). The capability of accurately predicting the fire spread is directly linked to the model’s inherent inaccuracy derived from its limitations and assumptions (Albini 1976; Ascoli et al. 2015; Vacchiano and Ascoli 2015) and input data quality, which are all factors that may undermine the utility of such predictions for decision-making (Cruz and Alexander 2013; Benali et al. 2017; Ramirez et al. 2019). Therefore, it seems essential to quantify the model’s performance and identify factors leading to unsatisfactory biases and errors. Cruz and Alexander (2013) conducted a comprehensive survey of studies, gathering a database of 1278 paired predicted vs observed ROS values and analysed the accuracy of ROS models. Other studies in several fire-prone areas assessed how new improvements in current fire spread models may enhance the performance relative to the older counterparts (Anderson et al. 2015; Cruz et al. 2018). More recently, Ascoli et al. (2022) used the Rothermel package in R (Vacchiano and Ascoli 2015) to test standard and custom fuel models against a global fire behaviour dataset (BONFIRE) and analysed model performance in different fuel types and ecosystems.
Recent advances in technology have allowed monitoring the fire progression of most wildfires every 15 min in the United States of America (USA) through the National Fireguard Detections product (FG). These data, when available for use on a fire, provide unprecedented capabilities to analyse factors influencing fire behaviour and compare the observed and predicted ROS in fires distributed across different and complex landscapes. Despite California being a major fire hotspot in the Americas, there is no extensive scientific analysis of operational fire spread models allowing analysing of their performance and drivers leading to model inaccuracies. This work assesses the predictive accuracy of the fire spread models currently used in California automatically in operational settings under different environmental conditions using 1853 fires from 2019 to 2021. The analysis identifies in what conditions the models may over- or underestimate the ROS and, subsequently, the burned area and associated fire impacts on buildings and other assets. We highlight that the simulations were performed automatically with no manual refinement of input data or model adjustments (Cardil et al. 2019). The analysis is based on well-known error metrics and statistical approaches (Cruz et al. 2018) aiming to compare the predicted with observed ROS values.
Methods
Study area
This study was developed in California (Fig. 1), a region dominated by mediterranean climatic conditions, known to foster recurrent large wildfires (Pyne et al. 1998). Fire-prone weather situations such as long and dry summers with thunderstorm episodes, low relative humidity and strong dry winds are typical of this region (Sugihara and Barbour 2006). Specifically, from September to May, the dry Diablo and Santa Ana winds arriving from the Great Basin and the Mojave Desert, with high desiccating potential and high wind speed (Li and Banerjee 2021) boost high ROS. The main vegetation types are shrubs, coniferous forest and herbaceous fine fuels according to the United States National Land Cover Database (39.03, 18.59 and 13.47%, respectively) (Jin et al. 2019). Fuel load, specifically dead materials, has increased in forests since 2010 in large areas of the State owing to successful fire suppression, timber harvesting practices and recent insect disturbances, potentially leading to fast and intense wildfires (van Wagtendonk et al. 2018).
Wildfire ignitions retrieved from FireGuard in California from October 2019 to November 2021. Each fire has an associated rate of spread (ROS) for the first burning period (up to 8 h) obtained by averaging the ROS of all FG polygons by incident. The zoomed-scale box shows three fires with the associated FG polygons for the burning period (the largest fire is the Mountain View fire).
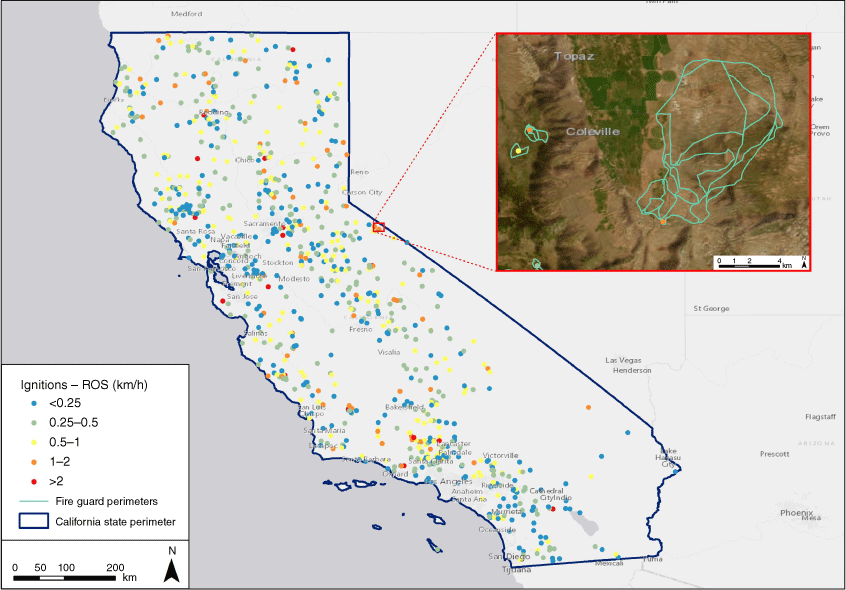
Fire progression dataset (FireGuard)
The National Fireguard Detections product (FG) provides wildfire detection and monitoring across the USA (US Department of Defense 2021). The program is operated in conjunction with the National Geospatial Intelligence Agency’s Firefly algorithm, which collects data from multiple sources and sensors, including imagery from satellites and unmanned aerial vehicles (National Guard Association 2021). It allows the detection of new wildfires as well as areas of significant fire growth, which is especially useful in remote regions and during the first 24–48 h when this information is usually scarce. Updates on fire progression are often provided every 15 min. The product provides a set of independent polygons representing sections of the fire front. To obtain a quantitative fire behaviour dataset from the raw data, polygons are classified into incidents and perimeters are analysed to obtain relevant metrics like burned area, maximum ROS, weather, fuels and terrain conditions.
To classify polygons into individual incidents (fires), we use a grid-growing clustering algorithm (Fig. 2; Zhao et al. 2015). All polygon centroids are projected into a 3D grid covering the spatio-temporal dimensions of the dataset using a 3 km in space and 24 h in time cell size resolution. This process leads to a density 3D matrix representing the number of elements at each cell. Clusters are defined as those groups of interconnected cells with at least a given number of centroids (islands of connected valid cells within the grid). To identify these clusters, we loop through the 3D matrix searching for a seed cell not belonging to any previously found cluster and assign it a new cluster ID (identity). That initial cell is then expanded (grown) among all connecting valid cells around it using a fast-marching method with eight degrees of freedom, and assigning the same cluster ID to all visited cells. This process is done iteratively until the loop is completed and all valid cells have an ID. The method assures that any FG polygon in the cluster has at least one neighbouring polygon within 2√2 cell size distance, which is ~67 h in time and 8.4 km in space.
Extraction of ROS vectors from the FireGuard (FG) dataset: (a) retrieval and storing of the FG data in a database; (b) projection of FG polygons into a 3D matrix representing space and time. Clustering identification of hotspot islands inside the grid. (c) Example of a wildfire that shows the evolution in time of a set of FG polygons with their associated maximum ROS vector (bold line), the secondary spread vectors, and the overall perimeter (dashed yellow line). Vector colours are based on their ROS.
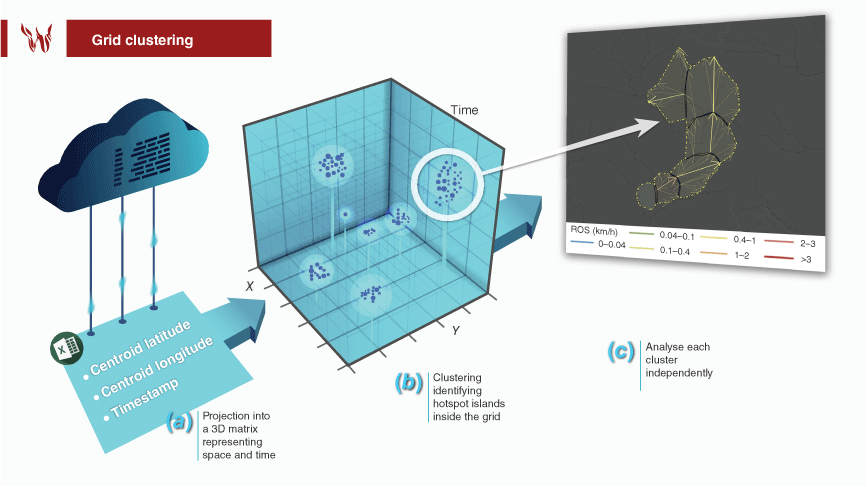
Once all clusters are identified, polygons in each incident are ordered in time, increased in resolution (number of vertices), and trimmed down to remove overlapping sections with previous polygons in time. Then a parent–child hierarchy of polygons is constructed based on the Hausdorf distance of each polygon with respect to the surrounding ones (Fig. 2). More explicitly, we compute and identify the vertices leading to the maximum distance from a point in a polygon to the closest point in the surrounding ones. If the child polygon is in contact with other polygons, the Hausdorf distance is computed only with respect to those shared vertices. If the child polygon is not in contact with any other polygon, it is assumed that it was created by spotting, and the Hausdorf distance is computed with respect to all polygons within the last 8 h. Polygons that have no valid distance vector are considered to be independent ignition sources not caused by any other polygon. The maximum ROS vector of a polygon is given by the Hausdorf distance vector divided by the arrival time difference between the parent and child polygon. Once the parent–child hierarchy is established, we can also compute all secondary spread vectors by computing the minimum distance from each vertex of the source polygon to the target one divided by the elapsed time between them. The number of these vectors will be equal to the number of vertices in the child polygon. Finally, to compute the overall perimeter length and burn area during the incident, a multi-polygon geometry representing the overall fire perimeter of the incident at each time step is created by computing the dissolved union of all previous polygons in time (the union of polygons without internal vertices, Fig. 2).
Through the Integrated Reporting of Wildland Fire Information (IRWIN) framework, which integrates different dispatch systems across the US, we automatically evaluated more than 30 000 fires in the study period (October 2019 to November 2021) from the reported locations. Of those incidents, a total of 1853 wildfires occurred in California and had FG data associated with their unique IRWIN IDs. We removed prescribed fires and wildfires with a duration less than 1 h and selected the first FG polygon later used as ignition source to simulate the fire growth. We used the first burning period (up to 8 h from the fire start) to compare the simulated ROS and observed ROS obtained with FG. In Fig. 3, we show four wildfires with associated ROS in each FG polygon and the fire simulation performed with WFA-e. To complete the fire behaviour dataset, fuel, terrain characteristics and weather conditions (see section 2.3 for further details) were assigned to each FG spread polygon through a polygon-based approach with zonal statistics.
Fire progression (FireGuard data) and simulation of four wildfires through Wildfire Analyst Enterprise (WFA-e) in California: (a) Mountain View fire (lat. 38.515; lon. −119.465; 2020/11/17); (b) Chaparral fire (lat. 33.485; lon. −117.399; 2021/08/28); (c) Bridge fire (lat. 38.921; lon. −121.037; 2021/09/05); (d) French fire (lat. 35.687; lon. −118.55; 2021/08/18); fote that the FG polygons and WFA-e simulated fire progression have the same time duration.
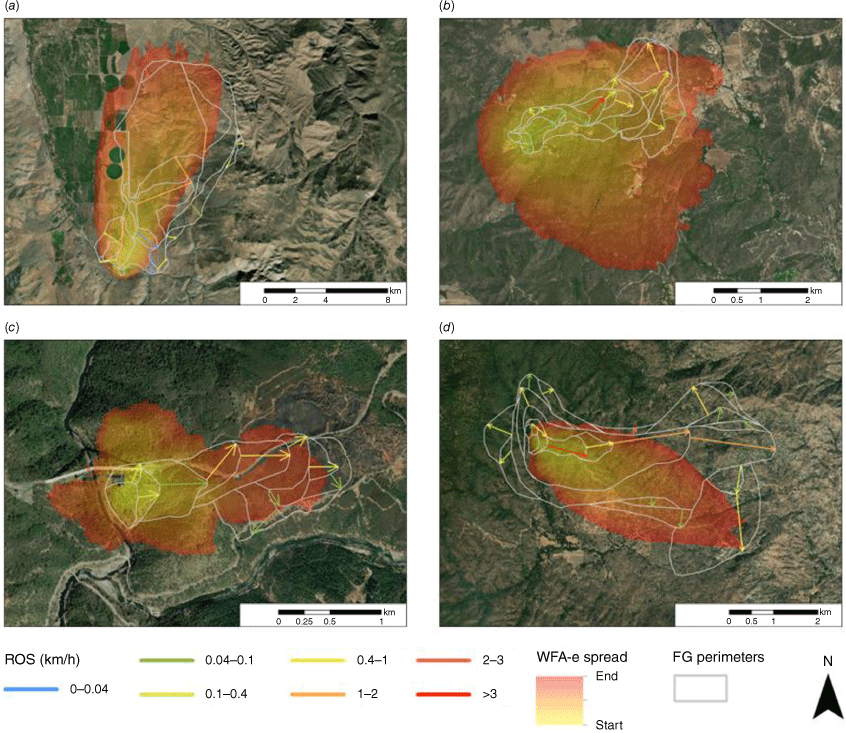
Fire modelling with Wildfire Analyst Enterprise (WFA-e)
Fire modelling was carried out using WFA-e, which provides real-time analysis of wildfire behaviour and simulates the spread of wildfires to directly support multi-agency wildfire incident management. Currently, many public and private entities, such as natural resource agencies, electrical investor-owned utilities, insurance and forestry companies strongly rely on WFA-e, which facilitates planning and operational decision-making. We automatically simulated 1853 fires using the first FG polygon of each incident as an ignition source based on well-known semi-empirical fire spread models currently used in California including Rothermel’s (1972) surface fire spread model, Van Wagner’s (1977) crown fire initiation model, Rothermel’s (1991) crown fire spread model, Albini’s (1979) spotting model, Andrews’ (2012) conversion factor and wind profile and the Minimum Travel Time evolution algorithm (Finney 2002). Note that the simulations performed in the present work were executed automatically with forecast data and no adjustments in input data or models aiming to better understand the feasibility of fire behaviour predictions in operational settings. We calculated the average ROS from FG and the average simulated ROS with WFA-e during real fire duration up to 8 h after the fire ignition (first burning period; Fig. 3).
Pre-fire improved high-resolution surface fuel types (Scott and Burgan 2005) and canopy characteristics maps (canopy cover, canopy height, canopy base height and canopy bulk density) at 30-m pixel resolution were generated to perform the fire simulations. The fire behaviour outputs had the same spatial resolution (i.e. 30 m). The surface fuel maps modelled by Technosylva Inc. are updated bi-monthly and based on an object-based image analysis. This approach grouped homogeneous vegetation areas into vector objects later classified with machine learning algorithms (random forest) trained with ground-truth data (the overall classification accuracy was 90% using 30% of data for validation and 70% for training; see more details in the Supplementary Material S1). The canopy characteristics were derived from LiDAR data (US Geological Survey, USGS) when available and models trained with Sentinel 2-A in the rest of the State (r = 0.92). We leveraged hourly high-resolution weather data at 2 km using an optimally configured version of the Weather Research and Forecasting model. This mesoscale numerical weather prediction system was designed for operational forecasting applications of everyday fire conditions, precipitation events and California’s notorious offshore wind events (Brewer and Clements 2020). Average wind fields at 10 m height above the ground were used to run the simulations. Nelson’s (2000) model was used to estimate dead fuel moisture content based on weather inputs. Live fuel moisture content was retrieved from Technosylva’s machine learning model trained with the US National Fuel Moisture Database (WFAS 2022).
Statistical analysis
We compared the ROS predicted by WFA-e and observed through FG based on four different well-known metrics (Cruz et al. 2018): (1) ROS residual representing the difference between the predicted and observed ROS. Therefore, a positive residual indicates an overestimation; (2) mean absolute error (MAE), representing the average of the absolute error (Eqn 1); (3) mean bias error (MBE), representing the average bias between the predicted and observed values (Eqn 2); (4) mean absolute percentage error (MAPE), a measure of prediction accuracy of a forecasting method in statistics that expresses the accuracy in relative terms (Eqn 3).
where FGi represents the ROS measured through FG, WFAi is the estimated ROS from WFA-e and n the number of wildfires.
Statistical graphics were used to represent model errors and biases associated with the fire spread models (Cruz et al. 2018). The outliers and the goodness of fit associated with the fire models were assessed through visualising residual plots and the aforementioned error and bias metrics. A histogram of raw residuals was plotted to examine whether the observations followed a normal distribution or were biased. Scatterplots were applied to identify outliers, skewness and kurtosis.
In order to statistically analyse the performance of the fire simulations considering major fuel type groups, we aggregated the Scott and Burgan (2005) fuel types in four categories as follows: Grass Fuel Types (GR, 101–109), Grass–Shrub Fuel Types (GS, 121–124), Shrub Fuel Types (SH, 141–149), and Timber–Litter–Understorey Fuel Types (TL, 161–189).
Results
The average ROS measured from FG data for all analysed fires was 0.55 km/h, with extreme fires with a sustained ROS of 1.45 and 2.91 km/h (quantile 95 and 99% respectively, Fig. 1). The fires spreading on timber areas were the fastest on average (0.66 km/h) compared with Grass (0.55 km/h), Grass–Shrub (0.48 km/h) and Shrub fires (0.42 km/h). The comparison between the observed and predicted ROS and the influence of the different environmental variables on ROS are evaluated in this section and Figs 4 and 5. Binning the mean MBE and MAE by fuel type and environmental factors exposed which factors a given simulation was most sensitive to. Fuel type was the key contributor to ROS bias and wind speed impacts were secondary. Both live and dead fuel moisture had noticeably smaller contributions to MBE, which implies improvements to fuel mapping and wind forecasts are the most critical pieces to a successful implementation of the Rothermel spread model.
Fire spread model’s performance analysis for 1853 wildfires that occurred in California in the 2019–2021 period: (a) histogram of ROS residuals by fuel type based on Scott and Burgan (2005); (b) ROS mean absolute error (MAE); (c) ROS mean bias error (MBE); (d) ROS mean absolute percentage error (MAPE). GR: Grass Fuel Types 101–109; GS: Grass–Shrub Fuel Types 121–124; SH: Shrub Fuel Types 141–149; TL: Timber–Litter–Understorey Fuel Types 161–189.
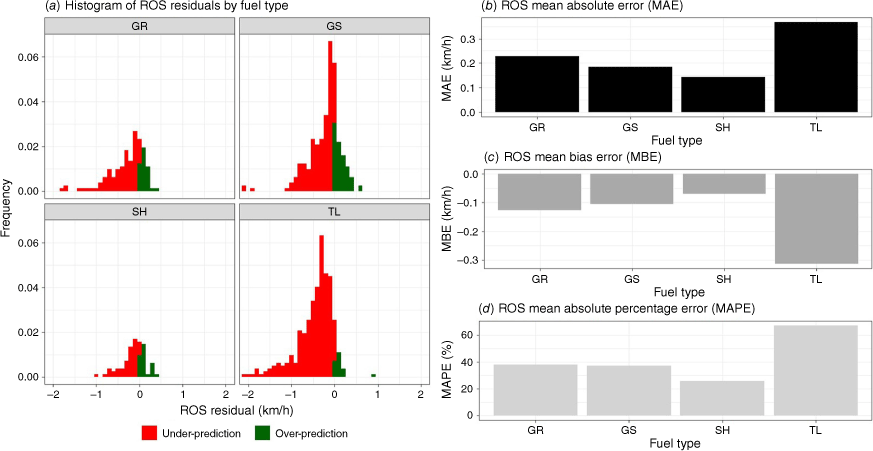
Scatterplot of the observed and predicted ROS and mean bias error (MBE) by fuel type (Scott and Burgan 2005), wind speed, dead fuel moisture content and herbaceous live fuel moisture content for 1853 wildfires that occurred in California in the 2019–2021 period. GR: Grass Fuel Types 101–109; GS: Grass–Shrub Fuel Types 121–124; SH: Shrub Fuel Types 141–149; TL: Timber–Litter–Understorey Fuel Types 161–189.
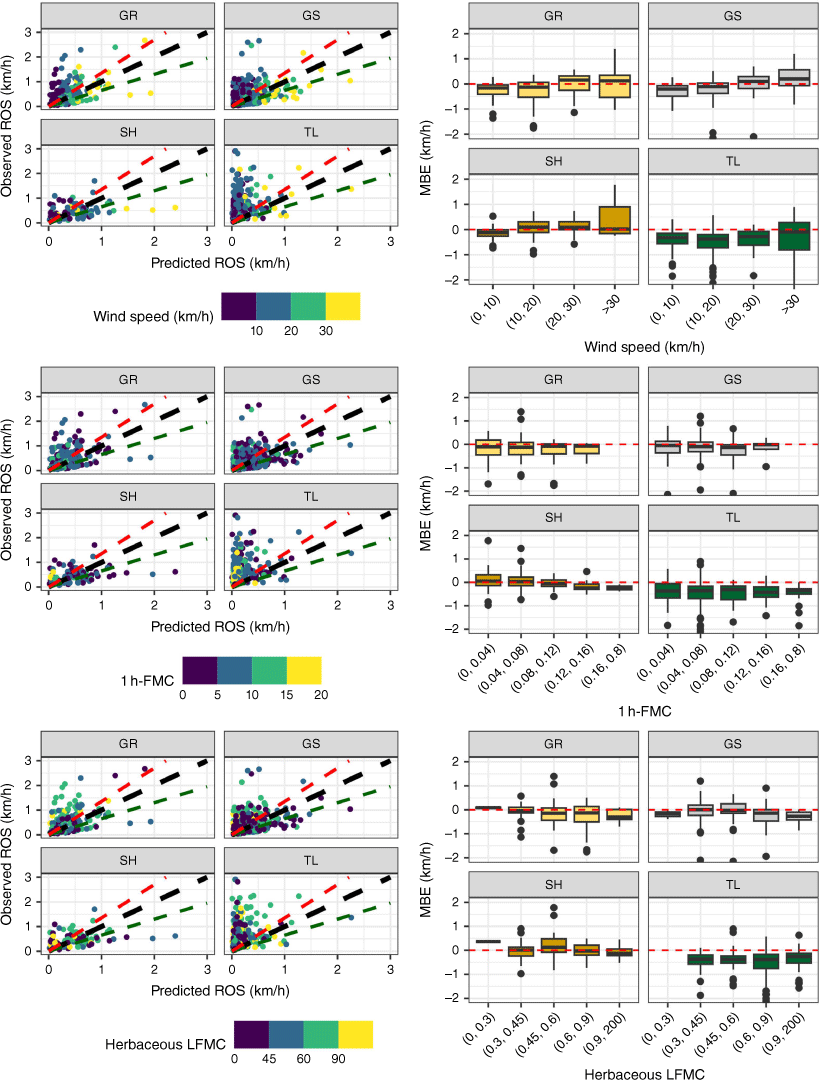
Overall accuracy of fire spread models
The fire spread models had an average MAPE of 47% for automatic fire simulations although this error metric meaningfully varied among fuel types. Overall, the model produced the best predictions for Shrub (SH, MAPE 26%), Grass (GR, MAPE 38%) and Grass–Shrub (GS, MAPE 39%) Scott and Burgan (2005) fuel types (Fig. 4). The lowest MAE (0.14 km/h), MBE (−0.07 km/h) and MAPE (26%) values were found for the SH fuel types, followed by GS and GR fuel types respectively (Fig. 4). Conversely, we found the highest MAE, MBE and MAPE in timber (TL) fuel types (Fig. 4) with a MAPE value of ~67%. Although fire spread models tended to underpredict ROS based on MBE values, the values were relatively low for GR, GS and SH fuel types, as shown in the histogram of ROS residuals (Fig. 4a, c). However, the models had a preponderant ROS underprediction bias in most timber fuels as shown by the histogram of ROS residuals, MAE and MBE metrics leading to the high MAPE (Fig. 4).
Environmental factors influencing model accuracy
The fire spread model’s accuracy was influenced by different environmental variables (input data) beyond fuel types including wind speed and fuel moisture (Fig. 5). Overall, high wind speeds led to ROS overestimation whereas the model’s ROS prediction was underestimated in fires with low wind speed, especially in the timber fires. Wind speed did not bias the ROS in SH fuel types but did bias the ROS in both GR and GS fuel types, especially when wind speed was higher than 20 km/h, as shown in Fig. 5. Although fire spread models systematically underestimated the ROS in timber fuel types, the median MBE of fires spreading under high wind speed conditions (>30 km/h) was low, probably because the crown fire spread models were activated and predicted active crown fire activity, subsequently increasing the simulated ROS. However, note that the boxplot shown in Fig. 5 for high wind speed conditions (>30 km/h) and timber fuel types is very wide, representing that single fire simulations may have high errors depending on environmental conditions and the activation of crown fire spread models. When active crowing fire behaviour was not predicted by the models owing to high canopy base high or low canopy bulk density, the ROS was generally lower than observed.
Both live and dead fuel moisture had noticeably smaller contributions to MBE. High fine dead fuel moisture content(FMC; 1 h time lag) led to underestimations of ROS predictions in all fuel types and live fuel moisture content (LFMC; herbaceous and woody) impacted ROS depending on fuel types. Whereas the ROS on fuel types with grass fuel load (GR and GS) was biased by the herbaceous LFMC, fires spreading on SH fuel types were not biased as expected (Fig. 5). The opposite was found for woody LFMC, resulting in biased ROS only on GS and SH fuel types.
Discussion
Fire agencies rely on semi-empirical models to daily predict fire behaviour aiming at assessing fire risk and improving the safety and effectiveness of fire suppression operations (Cardil et al. 2019). Thus, it is a priority to better understand the model’s performance under different environmental conditions to prove the feasibility and accuracy of outputs and further improve the current fire models. In fact, Rothermel’s (1972) model is the most widely evaluated fire spread model on Earth (Cruz and Alexander 2013; Cruz et al. 2018). In the present paper, the observed (FG) and predicted (WFA-e) ROS were compared analytically with a dataset of 1853 fires based on well-known error and bias metrics (Cruz et al. 2018). The amount, nature and quality of fire behaviour observations that FG data provide with extremely high temporal resolution have allowed a better understanding of the model’s performance and quantification of the effect of model input variables (i.e. fuel types, wind speed, fuel moisture content, etc.) on the model’s accuracy. The major caveats of previous research studies were associated with the availability of fire behaviour observations as well as inadequate input data (Cruz and Alexander 2013). Having overcome those obstacles, this analysis provides strong insights on the ROS predictions in operational environments.
The errors of fire behaviour predictions found in this work may be derived from intrinsic limitations and assumptions of fire spread models as well as external factors such as the quality of input data or local processes not captured by models (i.e. pyroconvection, local wind fields influenced by relief, etc.) (Ramirez et al. 2019) and the FG data. Although FG data provide unprecedented capabilities to validate the fire spread models, we recognise that small inaccuracies in the fire progression maps may occur, subsequently affecting the comparison between the observed and predicted ROS and associated errors. We used improved input data (fuels and weather) to run the simulations aiming to minimise the effect of input data quality in the model’s performance. In fact, improvements with simulating fire behaviour have arisen mostly from these external factors such as better mapping of fuel characteristics and moisture (Sullivan and Matthews 2013; Perrakis et al. 2014; Pimont et al. 2016), significant enhancements in weather forecasting with the operational implementation of mesoscale weather models and physically based downscaling techniques that can predict wind speed and direction over complex topography (Forthofer et al. 2009; Wagenbrenner et al. 2016), and the integration of fire behaviour models into software packages (Finney 1998, 2006; Ramírez et al. 2011; Monedero et al. 2019). Unfortunately, our study was not able to disaggregate errors resulting from all of these factors. Also, it is worth noting that all the fire simulations were performed automatically, exposing a new source of error given that the fire analyst can often adjust input data and calibrate the simulations in real time to adjust the simulated fire growth to the real fire progression (Cardil et al. 2019). For instance, it is well known how wind fields may change spatially owing to topography and how this effect may dramatically impact the fire simulations. This is a forecast error that may be easily addressed using observational data such as from weather stations near a given fire, and such input corrections did not occur with the simulations analysed herein. In this sense, Cruz and Alexander (2013) found that the lowest errors were associated with prescribed fires where fuel and weather inputs would have been measured on site. Therefore, the errors shown in this manuscript would strongly decrease with the knowledge of advanced users and integration of other data sources such as weather station data (Rothermel and Rinehart 1983; Stratton 2006; Alexander and Cruz 2013; Andrews 2014).
Overall, the fire spread model had moderate MAE and MAPE and low bias for Shrub (SH), Grass (GR) and Grass–Shrub (GS) Scott and Burgan (2005) fuel types, similarly to other studies (Cruz et al. 2018). Conversely, we found the highest MAE, MBE and MAPE in timber fuel types (Fig. 4) with a MAPE value of 67% with a preponderant ROS underprediction bias, an error type leading to potentially negative consequences in operational decision making (Cheney and Gould 1995). Previous studies found the lowest MAE values in timber and logging slash fuel types but also the largest MAPE (76%) values. These metrics were derived from the range of observed ROS values in these fuel types, which were substantially lower than in grasslands and shrublands fuel types (Cruz et al. 2018). However, in our study, we found the highest MAE and MBE values in timber fuel types because the database contained fires with high ROS that were not accurately predicted. This may be related to convective fire behaviour leading to local winds in the fire front not considered by the weather models or the difficulty of predicting active crown fire behaviour with the current fire spread models. Nevertheless, the effect of forest understorey fuel types on the ROS underprediction could probably be associated with the oversensitivity of the model to fuel bed depth and compactness (Catchpole et al. 1993). Thus, new custom fuel families adapted to California are needed to improve the accuracy of fire simulations. In this sense, we optimised custom timber fuel types for California based on genetic algorithms (Ascoli et al. 2015) using the data presented in this work, minimising the model bias and error substantially (MAPE 37%). The model’s accuracy was also influenced by other input variables beyond fuel types. Low wind speeds and high fuel moisture (dead and live) led to ROS underpredictions. This would probably be related to limitations of current wind field models to capture local patterns such as diurnal winds, abrupt landscapes driving turbulence, changes in wind direction or even pyroconvection that could increase the wind speed on the surface and prediction of crown fire behaviour in timber fuel types. Although attempts to reformulate and improve the Rothermel model have occurred in the past (Catchpole et al. 1993; Sandberg et al. 2007), the reality is that the model has yet to be replaced despite past recommendations for enhancing predictive fire models (Andrews 2014).
Our work supports the need for improvements proposed by Andrews (2014) and suggests that new enhancements (custom fuel families, improved input data including high-resolution wind fields, operational coupled fire–atmosphere models to capture pyroconvection, etc.) are still needed to decrease the error and bias of fire spread models, especially in timber areas. However, this research work provides promising results on the use of wildfire modelling for assessing fire spread potential during the initial attack in operational environments. In this sense, fire agencies can improve their preparedness and response in real time by systematically simulating all fire alerts and calculating fire risk metrics across the landscape. This procedure supports readiness and dispatching levels and drives the prioritisation of prevention activities. Also, electrical utilities can evaluate the fire risk and consequences, mitigate and prevent catastrophic wildfires. Finally, the fire spread models are also used to evaluate the extended attack incidents. Assessment of the model’s performance for this use case would require further work given that fire analysts can further calibrate the models in real time and the complexity of the fire environment is exacerbated owing to the aforementioned phenomena, such as pyroconvection, that may play a key role in explaining fire behaviour.
Conclusions
This work concludes that the fire spread model’s performance for California is in line with previous studies developed in other regions and the models are accurate enough to be used in real-time operations, especially with the use of adjustment modes that allow the calibration of predictions using field data (Artès et al. 2015; Cardil et al. 2019). Note that Cruz and Alexander (2013) highlighted that a ±35% error would be a reasonable and conservative standard for model performance of fire spread rate for research studies and could, understandably, result in wider error intervals in operational environments when simulations are performed automatically.
The accuracy of fire behaviour outputs is modulated by environmental conditions, especially fuel types and wind speed, which may bias ROS predictions. However, we also recognise that there are challenges regarding the effect of pyroconvection on local wind fields and the estimation of ROS in timber areas. The results of this evaluation suggest that the accuracy of fire simulations may be improved with newer models aiming to address the spread of fires in timber areas, crown fire behaviour modelling and the effect of pyroconvection in weather conditions and fire behaviour. The FG data and the algorithms developed in this research to calculate ROS vectors represent a crucial enhancement to better analyse the fire spread model’s performance for calibration efforts, developing new fire spread models and creating new fuel families. Our approach addresses issues arising from the use of long fire runs, encompassing at times variations in fuel types, the estimation of fuel characteristics across the landscape, and the averaging of wind speed over broad spatial and temporal scales.
References
Alexander ME, Cruz MG (2013) Are the applications of wildland fire behaviour models getting ahead of their evaluation again? Environmental Modelling & Software 41, 65-71.
| Crossref | Google Scholar |
Anderson WR, Cruz MG, Fernandes PM, McCaw L, Vega JA, Bradstock RA, Fogarty L, Gould J, McCarthy G, Marsden-Smedley JB, Matthews S, Mattingley G, Pearce HG, van Wilgen BW (2015) A generic, empirical-based model for predicting rate of fire spread in shrublands. International Journal of Wildland Fire 24, 443-460.
| Crossref | Google Scholar |
Andrews PL (2014) Current status and future needs of the BehavePlus Fire Modeling System. International Journal of Wildland Fire 23, 21-33.
| Crossref | Google Scholar |
Artès T, Cardil A, Cortés A, Margalef T, Molina D, Pelegrín L, Ramírez J (2015) Forest Fire Propagation Prediction Based on Overlapping DDDAS Forecasts. Procedia Computer Science 51, 1623-1632.
| Crossref | Google Scholar |
Ascoli D, Vacchiano G, Motta R, Bovio G (2015) Building Rothermel fire behaviour fuel models by genetic algorithm optimisation. International Journal of Wildland Fire 24, 317-328.
| Crossref | Google Scholar |
Ascoli D, Moris J, Sil Â, Fernandes P (2022) Using the Rothermel package in R to test standard and custom fuel models against global fire behavior data. Environmental Sciences Proceedings 17, 86.
| Crossref | Google Scholar |
Benali A, Sá ACL, Ervilha AR, Trigo RM, Fernandes PM, Pereira JMC (2017) Fire spread predictions: Sweeping uncertainty under the rug. Science of The Total Environment 592, 187-196.
| Crossref | Google Scholar |
Brewer MJ, Clements CB (2020) The 2018 Camp Fire: Meteorological Analysis Using In Situ Observations and Numerical Simulations. Atmosphere 11, 47.
| Crossref | Google Scholar |
California Department of Forestry and Fire Protection (CAL FIRE) (2019) Request for Innovative Ideas (RFI2) Wildfire Management. Available at: https://caleprocure.ca.gov/event/3540/0000012234 [last accessed 3 October 2023]
Cardil A, Molina DM (2015) Factors Causing Victims of Wildland Fires in Spain (1980–2010). Human and Ecological Risk Assessment: An International Journal 21, 67-80.
| Crossref | Google Scholar |
Cardil A, Monedero S, Silva CA, Ramirez J (2019) Adjusting the rate of spread of fire simulations in real time. Ecological Modelling 395, 39-44.
| Crossref | Google Scholar |
Catchpole EA, Catchpole WR, Rothermel RC (1993) Fire Behavior Experiments in Mixed Fuel Complexes. International Journal of Wildland Fire 3, 45-57.
| Crossref | Google Scholar |
Cheney NP, Gould JS (1995) Fire Growth in Grassland Fuels. International Journal of Wildland Fire 5, 237-247.
| Crossref | Google Scholar |
Cruz MG, Alexander ME (2013) Uncertainty associated with model predictions of surface and crown fire rates of spread. Environmental Modelling & Software 47, 16-28.
| Crossref | Google Scholar |
Cruz MG, Alexander ME, Sullivan AL, Gould JS, Kilinc M (2018) Assessing improvements in models used to operationally predict wildland fire rate of spread. Environmental Modelling & Software 105, 54-63.
| Crossref | Google Scholar |
Finney MA (2002) Fire growth using minimum travel time methods. Canadian Journal of Forest Research 32, 1420-1424.
| Crossref | Google Scholar |
Finney MA (2006) An overview of FlamMap fire modeling capabilities. In ‘Fuels Management – How to Measure Success: Conference Proceedings’, 28–30 March 2006, Portland, OR (Eds PL Andrews, BW Butler) pp. 213–220. (USDA Forest Service, Rocky Mountain Research Station, Proceedings RMRS‐P 41: Fort Collins, CO)
Jin S, Homer C, Yang L, Danielson P, Dewitz J, Li C, Zhu Z, Xian G, Howard D (2019) Overall Methodology Design for the United States National Land Cover Database 2016 Products. Remote Sensing 11, 2971.
| Crossref | Google Scholar |
Li S, Banerjee T (2021) Spatial and temporal pattern of wildfires in California from 2000 to 2019. Scientific Reports 11, 8779.
| Crossref | Google Scholar |
Liang J, Calkin DE, Gebert KM, Venn TJ, Silverstein RP (2008) Factors influencing large wildland fire suppression expenditures. International Journal of Wildland Fire 17, 650-659.
| Crossref | Google Scholar |
Minsavage-Davis CD, Davies GM (2022) Evaluating the Performance of Fire Rate of Spread Models in Northern European Calluna vulgaris Heathlands. Fire 5, 46.
| Crossref | Google Scholar |
Molina-Terrén DM, Xanthopoulos G, Diakakis M, Ribeiro L, Caballero D, Delogu GM, Viegas DX, Silva CA, Cardil A (2019) Analysis of forest fire fatalities in southern Europe: Spain, Portugal, Greece and Sardinia (Italy). International Journal of Wildland Fire 28, 85-98.
| Crossref | Google Scholar |
Monedero S, Ramirez J, Cardil A (2019) Predicting fire spread and behaviour on the fireline. Wildfire analyst pocket: A mobile app for wildland fire prediction. Ecological Modelling 392, 103-107.
| Crossref | Google Scholar |
National Guard Association (2021) Senators Push to Extend FireGuard Program. Available at https://www.ngaus.org/about-ngaus/newsroom/senators-push-extend-fireguard-program [verified 4 October 2022]
Nelson Jr RM (2000) Prediction of diurnal change in 10-h fuel stick moisture content. Canadian Journal of Forest Research 30, 1071-1087.
| Crossref | Google Scholar |
Pausas JG, Keeley JE (2009) A burning story: the role of fire in the history of life. BioScience 59, 593-601.
| Crossref | Google Scholar |
Perrakis DDB, Lanoville RA, Taylor SW, Hicks D (2014) Modeling Wildfire Spread in Mountain Pine Beetle-Affected Forest Stands, British Columbia, Canada. Fire Ecology 10, 10-35.
| Crossref | Google Scholar |
Pimont F, Parsons R, Rigolot E, de Coligny F, Dupuy J-L, Dreyfus P, Linn RR (2016) Modeling fuels and fire effects in 3D: Model description and applications. Environmental Modelling & Software 80, 225-244.
| Crossref | Google Scholar |
Ramirez J, Monedero S, Silva CA, Cardil A (2019) Stochastic decision trigger modelling to assess the probability of wildland fire impact. Science of The Total Environment 694, 133505.
| Crossref | Google Scholar |
Sandberg DV, Riccardi CL, Schaaf MD (2007) Reformulation of Rothermel’s wildland fire behaviour model for heterogeneous fuelbeds. Canadian Journal of Forest Research 37, 2438-2455.
| Crossref | Google Scholar |
Stocks BJ, Martell DL (2016) Forest fire management expenditures in Canada 1970–2013. The Forestry Chronicle 92, 298-306.
| Crossref | Google Scholar |
Sullivan AL, Matthews S (2013) Determining landscape fine fuel moisture content of the Kilmore East ‘Black Saturday’ wildfire using spatially extended point-based models. Environmental Modelling & Software 40, 98-108.
| Crossref | Google Scholar |
US Department of Defense (2021) Defense Department Imagery Information Aids Wildland Firefighters. Available at https://www.defense.gov/News/Releases/Release/Article/2764368/defense-department-imagery-information-aids-wildland-firefighters/ [verified 4 October 2022]
Vacchiano G, Ascoli D (2015) An Implementation of the Rothermel Fire Spread Model in the R Programming Language. Fire Technology 51, 523-535.
| Crossref | Google Scholar |
Van Wagner CE (1977) Conditions for the start and spread of crown fire. Canadian Journal of Forest Research 7, 23-34.
| Crossref | Google Scholar |
Wagenbrenner NS, Forthofer JM, Lamb BK, Shannon KS, Butler BW (2016) Downscaling surface wind predictions from numerical weather prediction models in complex terrain with WindNinja. Atmospheric Chemistry and Physics 16, 5229-5241.
| Crossref | Google Scholar |
WFAS (2022) Wildland Fire Assessment System (WFAS) database. Available at https://www.wfas.net/index.php/national-fuel-moisture-database-moisture-drought-103 [accessed on January 2022]
Zhao Q, Shi Y, Liu Q, Fränti P (2015) A grid-growing clustering algorithm for geo-spatial data. Pattern Recognition Letters 53, 77-84.
| Crossref | Google Scholar |