Future regional increases in simultaneous large Western USA wildfires
Seth McGinnis



A RAL/CISL, NCAR, Boulder, CO 80307, USA.
B Evans School of Public Policy & Governance, University of Washington, Seattle, WA 98195, USA.
C Oak Ridge Institute for Science and Education, Oak Ridge, TN 37831, USA.
International Journal of Wildland Fire 32(9) 1304-1314 https://doi.org/10.1071/WF22107
Submitted: 27 June 2022 Accepted: 13 July 2023 Published: 1 August 2023
© 2023 The Author(s) (or their employer(s)). Published by CSIRO Publishing on behalf of IAWF. This is an open access article distributed under the Creative Commons Attribution-NonCommercial-NoDerivatives 4.0 International License (CC BY-NC-ND)
Abstract
Background: Wildfire simultaneity affects the availability and distribution of resources for fire management: multiple small fires require more resources to fight than one large fire does.
Aims: The aim of this study was to project the effects of climate change on simultaneous large wildfires in the Western USA, regionalised by administrative divisions used for wildfire management.
Methods: We modelled historical wildfire simultaneity as a function of selected fire indexes using generalised linear models trained on observed climate and fire data from 1984 to 2016. We then applied these models to regional climate model simulations of the 21st century from the NA-CORDEX data archive.
Key results: The results project increases in the number of simultaneous 1000+ acre (4+ km2) fires in every part of the Western USA at multiple return periods. These increases are more pronounced at higher levels of simultaneity, especially in the Northern Rockies region, which shows dramatic increases in the recurrence of high return levels.
Conclusions: In all regions, the models project a longer season of high simultaneity, with a slightly earlier start and notably later end. These changes would negatively impact the effectiveness of fire response.
Implications: Because firefighting decisions about resource distribution, pre-positioning, and suppression strategies consider simultaneity as a factor, these results underscore the importance of potential changes in simultaneity for fire management decision-making.
Keywords: climate change, climate change impact assessment, fire management, NA-CORDEX, regional climate modelling, simultaneous fire, statistical modelling, western USA, wildfire, wildland fire.
Introduction
Numbers of large wildland fires and area burned have risen in the last few decades in Western USA (USGCRP 2017; Iglesias et al. 2022). In addition, attribution studies have demonstrated that climate change is at least partially responsible for these observed increases (Abatzoglou and Williams 2016; Williams et al. 2019; Goss et al. 2020). Warming, altered large-scale circulations, and the associated increased aridity have also led to an increase in fire weather conditions (Zhuang et al. 2021), longer fire seasons (Westerling et al. 2016), drier fuels (Williams et al. 2019), and larger burned areas (Zhang et al. 2019). However, as observed in some locations, increased precipitation may act to counter these effects, producing wetter fuel, at least in some years in some locations, given the high spatial and temporal heterogeneity of precipitation in the Western USA (Zhang et al. 2021). These competing factors do not contribute to a straightforward projection of how climate change will influence wildfire.
An additional factor that has received less attention in the fire-climate literature is potential changes in wildfire simultaneity. Simultaneous large wildfires or ‘megafires’ (fires with a final burned area of 1000 acres/4.05 km2 or more) pose a problem for wildfire management (Podschwit and Cullen 2020). All else being equal, it generally takes more resources to fight multiple fires than a single fire with the same total area (Podschwit and Cullen 2020; Abatzoglou et al. 2021). Per-hectare suppression costs typically decrease with fire size, making fire perimeter a better estimator of cost than area (Smith and González-Cabán 1987), and multiple fires will have more perimeter than a single fire with the same area. In addition, the need to travel between incidents reduces the efficiency of resources (Bednar et al. 1990), and fighting multiple fires simultaneously in one region requires the additional resources and coordination of Area Command (U.S. National Park Service 2017). Although crews and equipment can be deployed to serve multiple fires that do not co-occur, there are more variables to consider and more decisions to make in fighting multiple co-occurring fires (Podschwit and Cullen 2020; Cullen et al. 2021).
It is likely that wildfire simultaneity is influenced by climate (Bowman et al. 2011), and there is evidence that synchronicity of wildfires in North America has increased over the observed record (Iglesias et al. 2022). For example, Podschwit and Cullen (2020) demonstrated that synchronous wildfires in the United States have increased during the observed period 1984–2015, related this to climatic indicators, and showed that simultaneous wildfires are correlated with preparedness levels. Abatzoglou et al. (2021) demonstrated a correlation between days with high demand for national fire management resources and days with high synchronous fire danger, defined as days when the Canadian Fire Weather Index exceeds a high locally defined threshold over a large areal fraction of forested lands in the Western USA. They found significant trends in synchronous fire danger in both the observational record and in future projections, based on 18 CMIP5 (Coupled Model Intercomparison Project Phase 5) climate simulations and the RCP (Representative Concentration Pathway) 4.5 and 8.5 scenarios. These results suggest that wildfire simultaneity is likely to increase as well, but because simultaneity was inferred based on the spatial extent of thresholded values across the region, they do not address questions of when and where it will do so in terms of the spatial and temporal scales relevant to stakeholder practitioners. We add to this work by evaluating multiple fire indexes as predictors of simultaneity and modelling simultaneity directly.
Questions of significant interest remain, including how climate change will affect wildfire simultaneity over time and how those changes compare with the uncertainties associated with different representations of the future climate system.
To begin to answer these questions, we project the effects of climate change on simultaneous large wildfire occurrence at the level of Geographic Area Coordination Centers (GACCs). GACCs are the geographic units used in many fire management decisions, making this spatial scale highly operationally relevant to stakeholders (Cullen et al. 2022). Similarly, we used a bi-weekly timestep in the analysis to accommodate both the timescale of decision-making and the uncertainty associated with reporting of fires. We also analyse changes in simultaneity in terms of return levels (threshold values expected to be reached or exceeded on average once per period) in order to use a framing that is operationally relevant.
Materials and methods
We modelled historical large wildfire simultaneity at the GACC level as a function of various fire indexes that can be calculated from climate variables using generalised linear models (GLMs). We tested a variety of different fire indexes and methods of aggregating them from daily grid cell values to bi-weekly GACC values; after selecting the best predictor for each GACC, we then applied the corresponding GLM to fire indexes calculated from RCM (Regional Climate Model) outputs. We trained the GLMs on observed climate and fire data from 1984 to 2016 using 11-fold cross-validation.
Data
We used the Monitoring Trends in Burn Severity (MTBS; MTBS Project 2021) dataset for counts and locations of large wildfires, which we define as incidents coded as type ‘Wildfire’ and having a final area of 1000 acres (4.05 km2) or more. The MTBS dataset shows a sharp drop in fires below that size, which suggests it is the effective threshold of reliable reporting. This is also roughly the lower bound on size for fires that result in noteworthy fire management activity as indicated by the generation of an Incident Management Situation Report (E. Belval 2022, pers. comm., 31 October). We investigated the effects of using a higher size threshold and found that although this would change return levels and the choice of best predictor, it does not affect the overall pattern of change.
To calculate simultaneity, we counted the number of such fires in each GACC using non-overlapping 2-week windows. This both aligns with the timescale of decision-making in wildfire management and accounts for uncertainty associated with the temporal extent of large wildfires, which often last for more than a day but for which only the date of ignition (which may itself be uncertain) is reported in the MTBS database (Podschwit and Cullen 2020).
For observed climate data, we averaged gridMET data (Abatzoglou 2013) to 0.25° resolution, matching the resolution of the climate model outputs. gridMET is a gridded observational data product that provides daily high-resolution meteorological data for the contiguous United States of multiple impacts-relevant variables, including daily temperature extremes, precipitation, humidity, and surface winds. From these data, we calculated seven fire indexes: (1) Canadian Fire Weather Index (CFWI; Van Wagner and Pickett 1985); (2) Keetch–Byram Drought Index (KBDI; Keetch and Byram 1968; Alexander 1990); (3) Modified Fosberg Fire Weather Index (mFFWI; Goodrick 2002); (4) 100-h Fuel Moisture; (5) 1000-h Fuel Moisture; (6) Energy Release Component; and (7) Burning Index (FM100, FM1000, ERC, and BI, respectively; Cohen and Deeming 1985). For ERC and BI, we calculated two versions using different fuel models: one (ERC-G) using fuel model G, which is commonly used as the default; and one (ERC-L) using the fuel type covering the largest fraction of the grid cell using data from the NFDRS Fuel Model Map (Burgan et al. 1998). We developed a library of NCAR Command Language (Brown et al. 2012) functions to calculate the fire indexes (Kessenich and McGinnis 2022). The input variables used to calculate each fire index are presented in Supplementary Table S1.
Regional climate models (RCMs) are dynamical (physics-based) models run at high resolution over a limited area using boundary conditions from coarser global climate model (GCM) simulations as drivers. The regional climate RCM data we used comes from the NA-CORDEX archive (Mearns et al. 2017). We used all simulations driven by CMIP5 GCMs under the RCP8.5 emissions trajectory that had all the variables needed to calculate the fire indexes in Supplementary Table S1. Although RCP8.5 is a high-end scenario in the absence of additional targeted climate policy (i.e. with no reductions in emissions), it is a useful scenario for assessing impacts of climate change, especially in the near- and mid-term (Schwalm et al. 2020a, 2020b; Steel et al. 2022). Moreover, from the point of view of risk assessment, this is a high-risk scenario and thus important to consider in any risk assessment (Riahi et al. 2022). It is therefore also a useful scenario from the point of view of considering future adaptation possibilities, since if one can adapt to a high-end scenario, it is likely that one can adapt to lower scenarios. (Hallegatte 2009). There were 13 simulations total, 10 at 25-km resolution and three at 50-km resolution, comprising various combinations of six RCMs and five GCMs, as detailed in Supplementary Table S2. The GCMs span 85% of the range of equilibrium climate sensitivities (ECS) seen in the full CMIP5 ensemble (Bukovsky and Mearns 2020), covering a range of 2.4–4.6°C versus the full range of 2.1–4.7°C, with a mean and 90% confidence interval of 3.5 ± 1.1°C versus 3.2 ± 1.3°C for the full ensemble (Flato et al. 2013). The results of these simulations are representative of the mid- to upper range of plausible futures and have overlap with RCP4.5 results at mid-century (IPCC 2013a, 2013b; Rendfrey et al. 2018). Although NA-CORDEX simulations exist for other RCPs, we do not consider them here because the ensembles are considerably smaller, comprising seven simulations from four RCMs and four GCMs that span only 15% of the full CMIP5 ECS range (3.3–3.7°C, with a mean and 90% confidence interval of 3.5 ± 0.3°C) and therefore do not encompass the same level of structural (i.e. combined model) uncertainty. For each simulation, we used data that had been regridded to a common 0.25° (or 0.50°, as appropriate) latitude–longitude grid, and bias-corrected using Cannon’s multivariate MBCn algorithm (Cannon 2018) against gridMET observations, to compute the fire indexes in Supplementary Table S1.
Statistical modelling
We fit hurdle GLMs to predict the number of simultaneous large wildfires per GACC as a function of fire index. The hurdle model has two components: one GLM that predicts the probability of zero counts; and another that predicts non-zero count values. Using separate components for occurrence and level of simultaneity handles the highly-zero-inflated nature of the data. We used a GLM with binomial response and a logit-link function for the occurrence model, and a GLM with negative binomial response and log-link function for the count model of simultaneity level. A negative binomial models the heavy tail of distribution better than a Poisson distribution. We used the hurdle model from the R (R Core Team 2021) package ‘pcsl’ (Zeileis et al. 2008; Jackman et al. 2020) and fit parameters using maximum likelihood estimation.
Because simultaneity data is bi-weekly at the GACC level, we needed to aggregate the daily 25-km fire index data to match. We first averaged the fire index data spatially over each GACC, then aggregated it in time over 2-week windows using a range of five different possible statistics: the minimum, maximum, average, and 20th and 80th percentiles. In early stages of this research, we tested other methods of spatial aggregation based on exceedance of a threshold associated with observed fires in the historical record, but later abandoned them because they did not perform well compared with the spatial average.
For each GACC, we fit 90 GLMs in total, one for every combination of the nine fire indexes (including the two variants of ERC and BI), five temporal aggregation methods, and two grid scales. We then selected the predictor for each GACC with the best AIC (Akaike Information Criterion) weight. In every case, the best predictor at 0.25° resolution matched the best predictor at 0.5° resolution. We fit the models on 33 years of data from 1984 to 2016 using 11-fold cross-validation, for 30 years of training data and 3 years of holdout data in each fold. We also evaluated the GLMs’ ability to predict non-zero levels of simultaneity using the area under the Precision-Recall curve (PRC), which is a better measure of performance than the Receiver–Operator Curve when the classes are imbalanced (Saito and Rehmsmeier 2015).
For every GACC except Northern California (NC), the fit for the selected GLM was good, with p-values less than 0.01, high PRC values, and cross-validation RMSEs comparable to the values expected for comparing the distributional mean to a single sample. GLM validation plots can be found in Supplementary Figs S1–S7. In Northern California, the GLM had good performance predicting non-zero simultaneity, but had difficulty predicting higher levels of simultaneity. This may be because the NC GACC has the smallest area, which, all else being equal, reduces both the total number of fires and the level of simultaneity, and therefore reduces the effective sample size for fitting a statistical model.
With two exceptions, the best predictor of simultaneity in every GACC was 2-week average CFWI. In the Northwest (NW) GACC, it was narrowly beaten by 2-week 80th-percentile CFWI, and in the Southwest (SW) GACC, the best predictor was instead 2-week average FM100. The Canadian Fire Weather Index rates the potential frontal fire intensity modelled for a mature pine stand, where higher CFWI values indicate higher fire intensity. It combines elements from the Canadian Forest Fire Danger Rating System, including fuel moisture codes, initial spread component, and buildup index (De Groot 1987). Fire intensity is related to flame length and difficulty of fire suppression (Alexander and De Groot 1988).
We tested the effect of using different-size thresholds for the definition of large wildfire, considering 1000-, 2000-, 5000-, and 10 000-acre (4.05, 8.1, 20.25, and 40.5 km2) cutoffs, and found that our conclusions were qualitatively unchanged by this choice. Although simultaneity levels decrease because fewer fires are included, the patterns of change remain the same. The choice of best predictor is generally insensitive to the size threshold below a fairly high level: at the 5000-acre (20.25 km2) threshold, the best predictor for the SW GACC changes from FM100 to ERC-G, and at 10 000 acres (40.5 km2), the NW GACC switches from CFWI to FM100 and the RM and SC GACCs change to FM1000. However, in all cases, although the new best predictors have a higher AIC value, the performance of the best predictors for lower thresholds remains near-optimal and is very close to that of the new best options. The performance metrics (RMSE and PRC) we used for evaluation are negatively affected by using a lower threshold, but that is to be expected because the number of fires that are counted is reduced, so both the magnitude and frequency of non-zero simultaneity are also reduced. This effect is shown in Fig. 1. Because the results are qualitatively unchanged by the choice of threshold, we have chosen to focus on the 1000-acre (4.05 km2) threshold for this analysis because it aligns well with the scale of decision-making.
Performance of GLMs (circles) predicting simultaneity compared with statistics of observed simultaneity (crosses) for different fire-size thresholds. The GLMs have good accuracy in predicting the presence/absence of fire as measured by the area under the precision-recall curve (y-axis). They also have low cross-validation RMSE (x-axis) relative to the variance of observed non-zero simultaneity. Using a smaller fire-size threshold results in more frequent observed non-zero simultaneity (y-axis) and higher variance (x-axis), because the number of fires considered increases, which in turn reduces performance metrics.
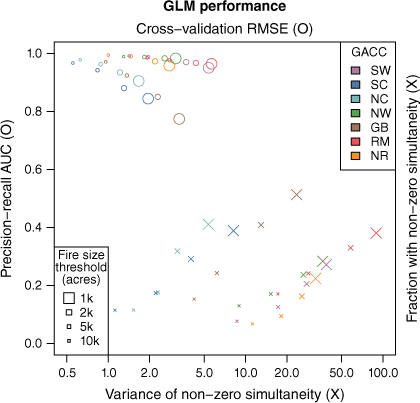
Analysis
To project future large wildfire simultaneity under climate change, we applied the best-performing GLM in each GACC to the corresponding fire index predictors calculated from the 13 NA-CORDEX simulations. This produced a 150-year bi-weekly sequence of probabilities for different simultaneity levels, from which we calculated the average probability of reaching or exceeding a given level of simultaneity in a period.
We used 1-, 2-, 5-, and 10-year return levels for these thresholds because those values are operationally relevant for fire management. Marsha (2012) describes different Preparedness Levels (PLs) used for fire management in the Northwest (NW) GACC as corresponding to conditions of large fire simultaneity, such that ‘a PL 5 could be expected to occur on the average of about once every 5 years while a PL 4 might be expected to occur every other year. PL 3 would essentially occur every year,’ (p. 2). Current procedures for determining PL are based less on absolute number of fires and more on anticipated degree of resource utilisation (NWCC 2020), but viewing changes in wildfire simultaneity through the lens of these return levels brings this research into alignment with the stakeholder frame of reference.
We used a 10 000-sample bootstrap to estimate the order statistics corresponding to 1-, 2-, 5-, and 10-year return levels of observed GACC-level simultaneity in the MTBS dataset. Note that because simultaneity values are integers, these thresholds are as well, whereas the expected average number of threshold exceedances per period projected by the GLMs is non-integer, and can differ from the return level even when applied to historical data.
Results
To evaluate changes in large wildfire simultaneity due to climate change, we calculated the expectation value of the number of bi-weekly periods where the number of simultaneous large wildfires in a GACC exceeded various return levels, as projected by GLMs (generalised linear models), which were fit to observational data and applied to output from an ensemble of 13 regional climate models (RCMs) using the RCP8.5 future emissions scenario (Mearns et al. 2017; Bukovsky and Mearns 2020). We then averaged these values over 30-year moving windows, distilling the projections down to a single value per RCM, window, and return level, and normalised them to the total number per return period (i.e. the number of events above the 2-year return level per 2 years, events above the 5-year threshold per 5 years, etc.). Fig. 2 summarises these results.
(a) Map of the Western USA GACCs. (b) Associated 1-, 2-, 5-, and 10-year return levels of bi-weekly simultaneity estimated from the historical record (1984–2016). (c–f) Boxplots of the projected number of returns per return period for different 30-year windows (mid-point given on X-axis), colour-coded by GACC. Each box represents model-average results from an ensemble of 13 RCMs. Boxes span the interquartile range, with a bar at the median; whiskers extend to the minimum and maximum values without outliers (represented by dots), which are points more than 1.5 × the IQR beyond the 75th percentile. Note the different y-axis scales on panels (c, d) vs (e, f).
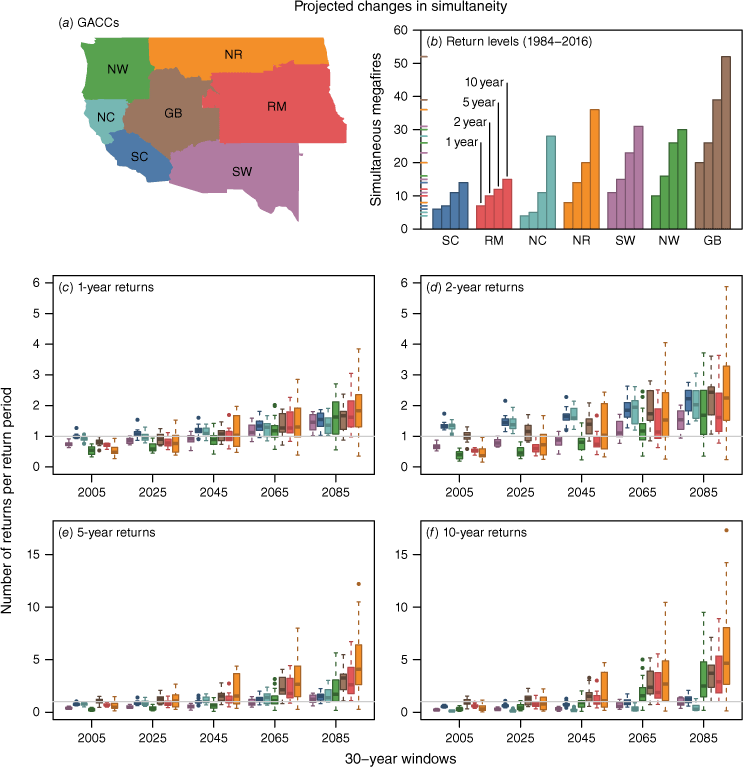
Fig. 2 shows a general pattern of increase in simultaneity in all GACCS and at all return levels. The spread across the ensemble of RCMs also increases with time. Some individual models show decreases (as indicated by the lower whiskers), and increases are not always monotonic, but the median of the projections trends upwards throughout the 21st century in every GACC and at every return level. Note that the models tend to underestimate historical simultaneity levels; this could reflect weaknesses in the statistical models, the RCMs, and/or the bias-correction of the RCMs, but the trend over time is much larger than this error. Although the return levels have been normalised to the same baseline, the recurrences of higher return levels show comparatively larger increases; there are very few projections that show a doubling of the number of 1-year return level exceedances, and more than half the models in more than half the GACCs project at least a doubling in the frequency of the 10-year return level.
The Northern Rockies GACC shows the most dramatic increases, especially at high return levels, where by end of century the historical 10-year return level of more than 35 simultaneous large wildfires is occurring almost five times per decade for a majority of projections. The Great Basin, Rocky Mountain, and Northwest GACCs also show substantial increases in recurrence across all return levels. The high-level increases in the Southwest, Southern California, and Northern California GACCs are more modest, and to some extent are obscured by the comparatively larger increases in the other GACCs. In the Northern California GACC in particular, the GLM had difficulty predicting high levels of simultaneity, so in this case the results may be more indicative of a lack of statistical power in the observations than of the effect of climate change. The upward trends in predicted simultaneity reflect corresponding trends in the GLM predictors for each GACC (see Methods section for details).
Fig. 3 shows changes in the seasonal timing of simultaneity; in general, the future patterns resemble amplified versions of the historical patterns. In all GACCs, most or all of the models project a shift toward a longer season of potential high simultaneity, with a slightly earlier start and a notably later end. This is clear in the Northwest (e) and Northern Rockies (h) GACCs, and especially noticeable in the Rocky Mountain (g) GACC, where most models show a period of high simultaneity above the historical peak extending from late June through early September. The median probability of exceeding the 1-year return level is roughly doubled in this period for these regions, and some individual models project increases up to four times higher. In the Southwest GACC (b), a few models show the emergence of a secondary season of high simultaneity in the fall with probabilities around a third of the historical peak. In the Rocky Mountain (g) and Northern Rockies (h) GACCs, the median of the ensemble reaches its maximum 2–4 weeks later in the future period, indicating that most models show a shift in the peak of simultaneity in these regions. Individual model results are visualised in Supplementary Figs S8 and S9.
(a) Map of Western USA GACCs. (b–h) Boxplots of the seasonal variation in projected simultaneity on a bi-weekly basis for each GACC. Projections for historical (1985–2015) and future (2030–2060) 30-year windows are shown in light and dark colours, respectively. Each box represents model-average results from an ensemble of 13 RCMs. Results shown are for the 1-year return level; longer-period return levels show a similar pattern, but with lower overall probabilities and correspondingly more noise.
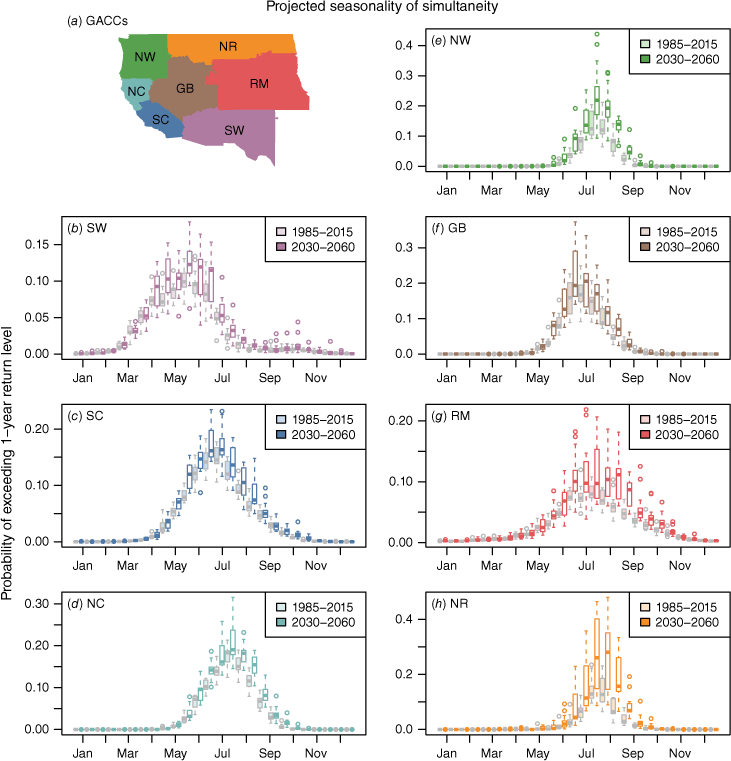
Discussion
Based on our statistical modelling, the NA-CORDEX ensemble of RCM simulations projects increases in the number of simultaneous 1000+ acre (4+ km2) wildfires in every part of the Western USA. These increases are more pronounced at higher levels of simultaneity, which means that not only do ‘bad years’ happen more frequently, the more extreme they are, the worse they get. The length of the season of high simultaneity also increases everywhere and extends later into the year, most notably in the northern and eastern parts of the region, and especially in the Rocky Mountain GACC. Late-fall simultaneity may increase in the Southwest GACC. These results align with the projections made by Abatzoglou, et al. (2021), while adding regional detail and information about seasonality and interannual variability.
Anticipated increases in the level of simultaneous fire can serve as a proxy for increased demand for fire response (Podschwit and Cullen 2020). National preparedness levels in the USA, which dictate and influence both requests for fire-response resource sharing and also the likelihood these requests will be filled (National Interagency Fire Center 2020), are correlated with the number of simultaneous fires actively burning, and positive trends in the number of simultaneous fires have been detected in recent years in some regions of the western United States (Podschwit and Cullen 2020). In 2021, a record number of days were spent at national PL 5 and a record number of days were spent at national PL 4 and 5 combined (National Interagency Coordination Center 2022). Additionally in 2021, the national preparedness level was elevated to PL 5 at the earliest point in the year in the past 10 years, on July 14, due in part to significant shared resources being committed to multiple geographic areas experiencing large simultaneous fire incidents (USDA Forest Service 2021). With greater numbers of simultaneous fires projected in the future, the decision context can expect to become more overwhelmed. Responses to this evolution could range from substantially elevating the pace and scale of fuel treatment to intentional changes in policies around hiring and budgeting for fire response. Because simultaneity serves as a proxy variable for resource strain, the ability to share resources will likely decrease with increased simultaneity if the amount of available resources and staff are not increased to meet management needs.
Wildfires are a necessary piece of the landscape in the western USA, and historically, forest ecosystems were adapted to low-intensity burning. A combination of natural ignitions and Indigenous burning practices created a patchwork variety of fuel types and fire conditions, which helped slow the growth and lessen the intensity of burning. However, decades of fire suppression have led to a buildup of fuels in large uninterrupted areas, shifting the fire landscape towards larger, more severe fires (Prichard et al. 2021). This analysis implicitly assumes that current fuel conditions will persist and continue to provide conditions conducive to large fires. However, mitigation and management of fuel availability would reduce the propensity for large fires, which would in turn reduce simultaneity of those fires.
This analysis also assumes no climate mitigation actions are taken to reduce total atmospheric CO2 concentrations in its use of RCP8.5. Although RCP8.5 is useful for assessing risk through at least mid-century, climate mitigation policy (Schwalm et al. 2020a, 2020b; Steel et al. 2022) – or use of a different scenario in our analysis – would likely reduce the severity of these end-of-century projections. However, this reduction is likely to be comparatively small. Abatzoglou et al. (2021) explored both RCP8.5 and RCP4.5, using a different approach to simultaneity and a statistical rather than dynamical approach to downscaling the CMIP5 data, and found that although RCP4.5 did show a lesser increase in synchronicity, the results were not greatly different from those of the RCP8.5 scenario, especially for the mid-century period. Their results show that using RCP4.5 reduces the amount of change by around 15% in mid-century and around 25% later in the century, whereas the spread due to choice of GCM is typically around 130% of the amount of change. This is in line with Collins et al. (2013), who showed that the difference in overall warming associated with these scenarios is still comparatively small in 2050, and is considerably less than the uncertainty associated with choice of GCM. The uncertainty across GCM–RCM combinations is thus more important for practitioners to consider than the uncertainty across concentration scenarios, particularly for the mid-century.
Evaluation of changes in simultaneity among regions is beyond the scope of this paper, but would be of considerable interest to the stakeholder practitioner community. Resource-sharing between GACCs is an important element of fire management that depends critically on differences in the level of need among regions; resources aren’t available to be shared between two GACCs if both are facing a peak in fire activity at the same time (Podschwit and Cullen 2020).
The Northern California GACC presents a challenge for statistical modelling, which may be due to its size. It would likely improve the statistical power of the observational record to subsume this GACC into other, larger GACCs for the purposes of analysis, but how to do so in a manner that balances matching the region of analysis to the physical system with performing analysis using a framework that is useful to stakeholders is a prime candidate for the collaborative coproduction of knowledge by scientists and practitioners. This issue could also reflect a weakness of the GLM; this GACC has the heaviest distributional tail, as reflected by the steep increase in return levels at longer return periods, and the statistical model may need further refinements to better handle poorly-sampled extremes.
The NA-CORDEX ensemble exhibits considerable spread in the projections, and some models project surprisingly extreme increases. For example, in the Northern Rockies GACC, the most extreme simulation projects that simultaneity at the historical 10-year return level will occur more than once per year by the last third of the century. Further analysis would be warranted to determine the credibility of such an extreme. Because fire indexes combine many different simulation outputs, often in a cumulative fashion, they may prove to be sensitive detectors of salient differences in RCM behaviour in these regions. Evaluation of the variability of individual RCM projections across different GACCs could provide insight into differences in the patterns of climate change projected by different models.
Although this analysis does not include all possible combinations of model and scenario, the results are representative of the middle to upper range of plausible responses, and are appropriate for use in risk-averse planning. Note that there are lower-bound scenarios associated with lower concentration pathways and models with lower ECS that are likely to result in futures that are closer to historical conditions. Because the lowest responses resulting from the simulations included in this analysis are at or below historical levels, it is entirely plausible and even likely that lower end of these scenarios overlaps with the upper end of the lower-bound scenarios. This viewpoint is agnostic with respect to the relative probabilities of different models and scenarios, and in line with the concept of ‘model democracy’ used in CMIP5. In addition, GCMs selected for downscaling in the CORDEX program were typically those that exhibited good performance in reproducing historical observations of surface temperature and precipitation. Thus, these simulations tend to be weighted heavily in approaches that use ensemble weighting schemes to reduce uncertainty. We are therefore confident that these simulations represent a range of outcomes that are not just possible but plausible. Finally, note that for many of the variables used as inputs to fire indexes, model skill is harder to verify than it is for temperature, making it important to consider a wide variety of models. In the context of long-term decision-making and finite computational resources, we posit that the deeper structural (combined model) uncertainty considered in this analysis is of greater concern than the uncertainty associated with a lower change scenario that generally resembles a reduced version of a higher-end scenario.
All that being said, we can make some determinations regarding the credibility of the NA-CORDEX simulations. In general, it has been well documented that simulations with regional climate models add value to the reproduction of current climate and projections of future climate (Giorgi et al. 2016; Doblas-Reyes et al. 2021) compared with their driving GCMs. Regarding this dataset specifically, various evaluations have been conducted that reflect improvements in the simulation of current climate and likely greater credibility of future climates (Gibson et al. 2019; Prein et al. 2019; Xu et al. 2019; Gutiérrez et al. 2021). It should also be noted that much of the work about the effect of future climate on fire weather has been performed using statistical downscaling (Abatzoglou and Brown 2012; Stavros et al. 2014; Parks et al. 2016). However, some serious limitations of statistical downscaling have been documented (Dixon et al. 2016; Kotamarthi et al. 2021), such that depending on the specific approach used, statistical downscaling results under conditions of climatic change may be suspect, particularly in areas of complex terrain such as the mountainous west. Although spatial averaging to the GACC scale eliminates the greater detail provided by dynamical downscaling, RCMs are better at reproducing local and regional scale weather and circulation that is important in determining characteristics of the fire weather indexes, which are calculated before the averaging takes place; although the influence of higher resolution is reduced by averaging, it is not eliminated. Therefore, there are some general advantages and added value in the NA-CORDEX dataset. A more thorough analysis of the dataset from the point of view of added value would be desirable.
Data availability
Data and code used for this paper are available on figshare. DOI: 10.6084/m9.figshare.19750891.
Declaration of funding
This material is based upon work supported by the National Center for Atmospheric Research, which is a major facility sponsored by the National Science Foundation under Cooperative Agreement No. 1852977. This work was supported by the National Science Foundation’s Growing Convergence Research Award No. 2019762 and by the US Department of Energy Regional and Global Climate Modelling program award DOE DE-SC0016605.
Author contributions
SM, LM, AC, HP, MB: Conceptualisation. SM, LK: Data Curation. SM: Formal Analysis. LM, AC, MB: Funding Acquisition. SM, HP: Methodology. LM, AC, MB: Project Administration. SM: Resources. SM, LK: Software. SM: Validation. SM, LK: Visualisation. SM, LK, LM, AC, MB: Writing – original draft. SM, LK, LM, AC, HP, MB: Writing – review and editing.
Acknowledgements
We gratefully acknowledge the National Science Foundation’s Growing Convergence Research Program (Award Number 2019762 ‘Managing Future Risk of Increasing Simultaneous Megafire’) for support of this research. We acknowledge the World Climate Research Programme’s Working Group on Regional Climate, and the Working Group on Coupled Modelling, former coordinating body of CORDEX and responsible panel for CMIP5. We also thank the climate modelling groups (listed in Supplementary Table S2) for producing and making available their model output. We also acknowledge the US Department of Defense Environmental Security Technology Certification Program for its support of the NA-CORDEX data archive. Finally, we thank the anonymous reviewers and the editors for their very helpful comments, which strengthened this manuscript substantially.
References
Abatzoglou JT (2013) Development of gridded surface meteorological data for ecological applications and modelling. International Journal of Climatology 33, 121-131.
| Crossref | Google Scholar |
Abatzoglou JT, Brown TJ (2012) A comparison of statistical downscaling methods suited for wildfire applications: STATISTICAL DOWNSCALING FOR WILDFIRE APPLICATIONS. International Journal of Climatology 32, 772-780.
| Crossref | Google Scholar |
Abatzoglou JT, Williams AP (2016) Impact of anthropogenic climate change on wildfire across western US forests. Proceedings of the National Academy of Sciences 113, 11770-11775.
| Crossref | Google Scholar |
Abatzoglou JT, Juang CS, Williams AP, Kolden CA, Westerling AL (2021) Increasing Synchronous Fire Danger in Forests of the Western United States. Geophysical Research Letters 48, e2020GL091377.
| Crossref | Google Scholar |
Alexander ME (1990) Computer Calculation of the Keetch Byram Drought Index—Programmers Beware! Fire Management Notes 51, 23-25 Available at http://www.climateanalyzer.science/python/dashboards/keetch_byram_corrected.pdf.
| Google Scholar |
Bednar LF, Mees R, Strauss D (1990) Fire Suppression Effectiveness for Simultaneous Fires: An Examination of Fire Histories. Western Journal of Applied Forestry 5, 16-19.
| Crossref | Google Scholar |
Bowman DMJS, Balch J, Artaxo P, Bond WJ, Cochrane MA, D’Antonio CM, DeFries R, Johnston FH, Keeley JE, Krawchuk MA, Kull CA, Mack M, Moritz MA, Pyne S, Roos CI, Scott AC, Sodhi NS, Swetnam TW (2011) The human dimension of fire regimes on Earth: The human dimension of fire regimes on Earth. Journal of Biogeography 38, 2223-2236.
| Crossref | Google Scholar |
Brown D, Brownrigg R, Haley M, Huang W (2012) ‘NCAR Command Language (NCL).’ (UCAR/NCAR - Computational and Information Systems Laboratory (CISL)) 10.5065/D6WD3XH5
Bukovsky MS, Mearns LO (2020) Regional climate change projections from NA-CORDEX and their relation to climate sensitivity. Climatic Change 162, 645-665.
| Crossref | Google Scholar |
Burgan RE, Klaver RW, Klaver JM (1998) Fuel Models and Fire Potential From Satellite and Surface Observations. International Journal of Wildland Fire 8(3), 159-170.
| Crossref | Google Scholar |
Cannon AJ (2018) Multivariate quantile mapping bias correction: an N-dimensional probability density function transform for climate model simulations of multiple variables. Climate Dynamics 50, 31-49.
| Crossref | Google Scholar |
Cohen JD, Deeming JE (1985) The National Fire Danger Rating System: basic equations. General Technical Report PSW-82. (USDA Forest Service, Pacific Southwest Forest and Range Experiment Station) Available at https://www.fs.usda.gov/psw/publications/documents/psw_gtr082/psw_gtr082.pdf
Collins M, Knutti R, Arblaster J, Dufresne J-L, Fichefet T, Friedlingstein P, Gao X, Gutowski WJ, Johns T, Krinner G, Shongwe M, Tebaldi C, Weaver AJ, Wehner M (2013) Chapter 12 - Long-term climate change: Projections, commitments and irreversibility. ‘Climate Change 2013: The Physical Science Basis. IPCC Working Group I Contribution to AR5’. (Ed IPCC) (Cambridge University Press: Cambridge) Available at http://www.climatechange2013.org/images/report/WG1AR5_Chapter12_FINAL.pdf
Cullen AC, Axe T, Podschwit H (2021) High-severity wildfire potential – associating meteorology, climate, resource demand and wildfire activity with preparedness levels. International Journal of Wildland Fire 30, 30-41.
| Crossref | Google Scholar |
Cullen AC, Prichard SJ, Abatzoglou JT, Dolk A, Kessenich L, Bloem S, Bukovsky MS, Humphrey R, McGinnis S, Skinner H, Mearns LO (2023) Growing convergence research: Coproducing climate projections to inform proactive decisions for managing simultaneous wildfire risk. Risk Analysis, risa.14113. doi:10.1111/risa.14113.
De Groot WJ (1987) Interpreting the Canadian forest fire weather index (FWI) system. In ‘Proceedings of the Fourth Central Region Fire Weather Committee Scientific and Technical Seminar’, Winnipeg, Manitoba, CA. (Canadian Forestry Service: Northern Forestry Centre, Edmonton, Alberta, CA) Available at https://cfs.nrcan.gc.ca/publications?id=23688
Dixon KW, Lanzante JR, Nath MJ, Hayhoe K, Stoner A, Radhakrishnan A, Balaji V, Gaitán CF (2016) Evaluating the stationarity assumption in statistically downscaled climate projections: is past performance an indicator of future results? Climatic Change 135, 395-408.
| Crossref | Google Scholar |
Doblas-Reyes FJ, Sörensson AA, Almazroui M, Dosio A, Gutowski WJ, Haarsma R, Hamdi R, Hewitson B, Kwon W-T, Lamptey BL, Maraun D, Stephenson TS, Takayabu I, Terray L, Turner A, Zuo Z (2021) Linking Global to Regional Climate Change. In ‘Climate Change 2021: The Physical Science Basis. Contribution of Working Group I to the Sixth Assessment Report of the Intergovernmental Panel on Climate Change’. (Eds V Masson-Delmotte, P Zhai, A Pirani, SL Connors, C Péan, S Berger, N Caud, Y Chen, L Goldfarb, MI Gomis, M Huang, K Leitzell, E Lonnoy, JBR Matthews, TK Maycock, T Waterfield, O Yelekçi, R Yu, B Zhou) pp. 1363–1512. (Cambridge University Press: Cambridge, UK and New York, NY, USA) doi:10.1017/9781009157896.012
Flato G, Marotzke J, Abiodun B, Braconnot P, Chou SC, Collins W, Cox P, Driouech F, Emori S, Eyring V, Forest C, Gleckler P, Guilyardi E, Jakob C, Kattsov V, Reason C, Rummukainen M (2013) Evaluation of Climate Models. In ‘Climate Change 2013: The Physical Science Basis. Contribution of Working Group I to the Fifth Assessment Report of the Intergovernmental Panel on Climate Change’. (Cambridge University Press: Cambridge, UK and New York, NY, USA)
Gibson PB, Waliser DE, Lee H, Tian B, Massoud E (2019) Climate Model Evaluation in the Presence of Observational Uncertainty: Precipitation Indices over the Contiguous United States. Journal of Hydrometeorology 20, 1339-1357.
| Crossref | Google Scholar |
Giorgi F, Torma C, Coppola E, Ban N, Schär C, Somot S (2016) Enhanced summer convective rainfall at Alpine high elevations in response to climate warming. Nature Geoscience 9, 584-589.
| Crossref | Google Scholar |
Goodrick SL (2002) Modification of the Fosberg fire weather index to include drought. International Journal of Wildland Fire 11, 205-211.
| Crossref | Google Scholar |
Goss M, Swain DL, Abatzoglou JT, Sarhadi A, Kolden CA, Williams AP, Diffenbaugh NS (2020) Climate change is increasing the likelihood of extreme autumn wildfire conditions across California. Environmental Research Letters 15, 094016.
| Crossref | Google Scholar |
Gutiérrez JM, Jones RG, Narisma GT, Alves LM, Amjad M, Gorodetskaya IV, Grose M, Klutse NAB, Krakovska S, Li J, Martínez-Castro D, Mearns LO, Mernild SH, Ngo-Duc T, van den Hurk B, Yoon J-H (2021) Atlas. In ‘Climate Change 2021: The Physical Science Basis. Contribution of Working Group I to the Sixth Assessment Report of the Intergovernmental Panel on Climate Change’. (Eds V Masson-Delmotte, P Zhai, A Pirani, SL Connors, C Péan, Y Chen, L Goldfarb, MI Gomis, M Huang, K Leitzell, E Lonnoy, JBR Matthews, TK Maycock, T Waterfield, O Yelekçi, R Yu, B Zhou) pp. 1927–2058. (Cambridge University Press: Cambridge, UK and New York, NY, USA) doi:10.1017/9781009157896.021
Hallegatte S (2009) Strategies to adapt to an uncertain climate change. Global Environmental Change 19, 240-247.
| Crossref | Google Scholar |
Iglesias V, Balch JK, Travis WR (2022) U.S. fires became larger, more frequent, and more widespread in the 2000s. Science Advances 8, eabc0020.
| Crossref | Google Scholar |
IPCC (2013a) Annex I: Atlas of Global and Regional Climate Projections. In ‘Climate Change 2013: The Physical Science Basis. Contribution of Working Group I to the Fifth Assessment Report of the Intergovernmental Panel on Climate Change’. (Cambridge University Press: Cambridge, UK and New York, NY, USA)
IPCC (2013b) Annex I: Atlas of Global and Regional Climate Projections Supplementary Material RCP8.5. In ‘Climate Change 2013: The Physical Science Basis. Contribution of Working Group I to the Fifth Assessment Report of the Intergovernmental Panel on Climate Change’. (Cambridge University Press: Cambridge, UK and New York, NY, USA)
Jackman S, Tahk A, Zeileis A, Maimone C, Fearon J, Meers Z (2020) pscl: Political Science Computational Laboratory. Available at https://CRAN.R-project.org/package=pscl
Keetch JJ, Byram GM (1968) A Drought Index for Forest Fire Control. SE-38. (USDA Forest Service: Southeastern Forest Experiment Station, Asheville, NC) Available at https://www.srs.fs.usda.gov/pubs/rp/rp_se038.pdf
Kessenich L, McGinnis S (2022) ‘NCAR/fire-indices: Fire Weather Index Collection in NCL.’ (Zenodo) 10.5281/ZENODO.6534001
Kotamarthi R, Hayhoe K, Mearns L, Wuebbles D, Jacobs J, Jurado J (2021) ‘Downscaling Techniques for High-Resolution Climate Projections: From Global Change to Local Impacts.’ (Cambridge University Press) 10.1017/9781108601269
Mearns L, McGinnis S, Korytina D, Arritt R, Biner S, Bukovsky M, Chang H-I, Christensen O, Herzmann D, Jiao Y, Kharin S, Lazare M, Nikulin G, Qian M, Scinocca J, Winger K, Castro C, Frigon A, Gutowski W (2017) The NA-CORDEX dataset. 10.5065/D6SJ1JCH
MTBS Project (2021) MTBS Data Access: Fire Level Geospatial Data. (USDA Forest Service/US Geological Survey) Available at http://mtbs.gov/direct-download
National Interagency Coordination Center (2022) NICC Wildland Fire Summary and Statistics Annual Report 2021. (National Interagency Coordination Center, National Interagency Fire Center: Boise, ID) Available at https://www.nifc.gov/sites/default/files/NICC/2-Predictive%20Services/Intelligence/Annual%20Reports/2021/annual_report_0.pdf
National Interagency Fire Center (2020) National Wildland Fire Preparedness Levels. Available at https://www.nifc.gov/sites/default/files/2020-09/National_Preparedness_Levels.pdf
NWCC (2020) ‘Pacific Northwest Interagency Preparedness Plan, 2020 Update.’ (Northwest Interagency Coordination Center: Portland, OR) Available at https://gacc.nifc.gov/nwcc/content/pdfs/2020 Update_NW_PreparednessPlan.pdf
Parks SA, Miller C, Abatzoglou JT, Holsinger LM, Parisien M-A, Dobrowski SZ (2016) How will climate change affect wildland fire severity in the western US? Environmental Research Letters 11, 035002.
| Crossref | Google Scholar |
Podschwit H, Cullen A (2020) Patterns and trends in simultaneous wildfire activity in the United States from 1984 to 2015. International Journal of Wildland Fire 29, 1057-1071.
| Crossref | Google Scholar |
Prein AF, Bukovsky MS, Mearns LO, Bruyère CL, Done JM (2019) Simulating North American Weather Types With Regional Climate Models. Frontiers in Environmental Science 7, 36.
| Crossref | Google Scholar |
Prichard SJ, Hessburg PF, Hagmann RK, Povak NA, Dobrowski SZ, Hurteau MD, Kane VR, Keane RE, Kobziar LN, Kolden CA, North M, Parks SA, Safford HD, Stevens JT, Yocom LL, Churchill DJ, Gray RW, Huffman DW, Lake FK, Khatri‐Chhetri P (2021) Adapting western North American forests to climate change and wildfires: 10 common questions. Ecological Applications 31, e02433.
| Crossref | Google Scholar |
R Core Team (2021) ‘R: A Language and Environment for Statistical Computing.’ (R Foundation for Statistical Computing: Vienna, Austria) Available at https://www.R-project.org/
Rendfrey TS, Bukovsky MS, McGinnis SA (2018) NA-CORDEX Visualization Collection. 10.5065/90ZF-H771
Riahi K, Schaeffer R, Arango J, Calvin K, Guivarch C, Hasegawa T, Jiang K, Kriegler E, Matthews R, Peters GP, Rao A, Robertson S, Sebbit AM, Steinberger J, Tavoni M, van Vuuren DP (2022) Mitigation pathways compatible with long-term goals. In ‘Climate change 2022: Mitigation of climate change. Contribution of Working Group III to the Sixth Assessment Report of the Intergovernmental Panel on Climate Change’. pp. 295–408. (Cambridge University Press: Cambridge, UK and New York, NY, USA) 10.1017/9781009157926.005
Saito T, Rehmsmeier M (2015) The Precision-Recall Plot Is More Informative than the ROC Plot When Evaluating Binary Classifiers on Imbalanced Datasets. PLoS One 10, e0118432.
| Crossref | Google Scholar |
Schwalm CR, Glendon S, Duffy PB (2020a) RCP8.5 tracks cumulative CO2 emissions. Proceedings of the National Academy of Sciences 117, 19656-19657.
| Crossref | Google Scholar |
Schwalm CR, Glendon S, Duffy PB (2020b) Reply to Hausfather and Peters: RCP8.5 is neither problematic nor misleading. Proceedings of the National Academy of Sciences 117, 27793-27794.
| Crossref | Google Scholar |
Smith EL, González-Cabán A (1987) Estimating cost of large-fire suppression for three Forest Service Regions. Research Note PSW-RN-391. (USDA Forest Service: Pacific Southwest Forest and Range Experiment Station, Berkeley, CA) Available at https://www.fs.usda.gov/psw/publications/documents/psw_rn391/
Stavros EN, Abatzoglou JT, McKenzie D, Larkin NK (2014) Regional projections of the likelihood of very large wildland fires under a changing climate in the contiguous Western United States. Climatic Change 126, 455-468.
| Crossref | Google Scholar |
Steel D, DesRoches CT, Mintz-Woo K (2022) Climate change and the threat to civilization. Proceedings of the National Academy of Sciences 119, e2210525119.
| Crossref | Google Scholar |
USDA Forest Service (2021) National Wildland Fire Preparedness Level 5. News Release (USDA Forest Service: Malheur National Forest, Oregon) Available at https://www.fs.usda.gov/detail/malheur/news-events/?cid=FSEPRD935029
USGCRP (2017) Climate Science Special Report: Fourth National Climate Assessment, Vol. I. (Eds DJ Wuebbles, DW Fahey, KA Hibbard, DJ Dokken, BC Stewart, TK Maycock) (U.S. Global Change Research Program). 10.7930/J0J964J6
U.S. National Park Service (2017) Wildland Fire: Incident Command System Levels. Available at https://www.nps.gov/articles/wildland-fire-incident-command-system-levels.htm
Van Wagner CE, Pickett TL (1985) Equations and FORTRAN program for the Canadian Forest Fire Weather Index System. (Canadian Forestry Service, Petawana National Forestry Institute) Available at https://cfs.nrcan.gc.ca/pubwarehouse/pdfs/19973.pdf
Westerling AL, Brown TJ, Schoennagel T, Swetnam TW, Turner MG, Veblen TT (2016) Climate and Wildfire in Western US Forests. In ‘Forest Conservation in the Anthropocene: Science, Policy, and Practice’. (Eds VA Sample, RP Bixler, C Miller) pp. 43–55. (University Press of Colorado) 10.5876/9781607324591.c003
Williams AP, Abatzoglou JT, Gershunov A, Guzman‐Morales J, Bishop DA, Balch JK, Lettenmaier DP (2019) Observed Impacts of Anthropogenic Climate Change on Wildfire in California. Earth’s Future 7, 892-910.
| Crossref | Google Scholar |
Xu Z, Han Y, Yang Z (2019) Dynamical downscaling of regional climate: A review of methods and limitations. Science China Earth Sciences 62, 365-375.
| Crossref | Google Scholar |
Zeileis A, Kleiber C, Jackman S (2008) Regression Models for Count Data in R. Journal of Statistical Software 27, 1-25.
| Crossref | Google Scholar |
Zhang Y, Xiang Q, Yu C, Bao J, Ho HC, Sun S, Ding Z, Hu K, Zhang L (2019) Mortality risk and burden associated with temperature variability in China, United Kingdom and United States: Comparative analysis of daily and hourly exposure metrics. Environmental Research 179, 108771.
| Crossref | Google Scholar |
Zhang F, Biederman JA, Dannenberg MP, Yan D, Reed SC, Smith WK (2021) Five Decades of Observed Daily Precipitation Reveal Longer and More Variable Drought Events Across Much of the Western United States. Geophysical Research Letters 48, e2020GL092293.
| Crossref | Google Scholar |
Zhuang Y, Fu R, Santer BD, Dickinson RE, Hall A (2021) Quantifying contributions of natural variability and anthropogenic forcings on increased fire weather risk over the western United States. Proceedings of the National Academy of Sciences 118, e2111875118.
| Crossref | Google Scholar |