Forest fuel bed ignitability under marginal fire weather conditions in Eucalyptus forests
Jane G. Cawson A B and Thomas J. Duff AA School of Ecosystem and Forest Sciences, University of Melbourne, Burnley Campus, 500 Yarra Boulevard, Richmond, Vic. 3121, Australia.
B Corresponding author. Email: jane.cawson@unimelb.edu.au
International Journal of Wildland Fire 28(3) 198-204 https://doi.org/10.1071/WF18070
Submitted: 15 May 2018 Accepted: 17 December 2018 Published: 8 February 2019
Abstract
Fires burning under marginal fire-weather conditions tend to be patchy in terms of their spatial coverage. This patchiness is partially driven by variability in the ignitability of the fuel bed. An understanding of fuel-bed ignitability through space and time would help fire managers to more effectively carry out prescribed burns to achieve desired levels of burn coverage in Eucalyptus forests. We sought to identify the key fuel-bed attributes influencing ignitability under marginal weather conditions. We recorded ignition successes and failures at 45 points within 5 operational prescribed burns and used the data to build logistic regression models to predict the probability of ignition as a function of fuel-bed attributes. Models were ranked using an information theoretic approach. The four highest ranked models explained 48–54% of the variance in ignitability. Surface fine-fuel moisture content (FFMC) and overall fuel hazard (i.e. fuel arrangement) were the strongest predictors of ignitability, occurring in all four highest ranking models. Both surface FFMC and overall fuel hazard were negatively related to ignition likelihood, contradicting a commonly assumed positive relationship between fuel hazard and flammability. Our field method to measure ignition success could be applied across more prescribed burns to develop operationally useful models of ignitability.
Additional keywords: fire behaviour, fire management, flammability, forest management, fuel hazard, fuel moisture, mosaic, patchiness, prescribed burning, wildfire.
Introduction
Fires burning under marginal fire-weather conditions (e.g. during prescribed burns) are characteristically patchy in terms of their spatial coverage and severity (Knapp and Keeley 2006; Penman et al. 2007). Burning conditions are considered marginal when fire can be controlled with relative ease and self-extinguishment may occur overnight (Tolhurst and Cheney 1999). This generally occurs when there are low wind speeds, high relative humidities and low temperatures. Under such conditions, gullies and polar-facing slopes are typically less flammable and therefore more likely to remain unburnt or experience low intensity fire while ridges and equatorial-facing slopes are likely to burn at moderate intensities (Penman et al. 2007). This patchiness is often a desired outcome of prescribed burns, particularly when a burn occurs in an ecologically sensitive area where unburnt patches act as wildfire refuges (Robinson et al. 2013) or intercept erosive runoff (Cawson et al. 2013). Furthermore, areas of low flammability may be intentionally used as passive barriers to fire spread, during a prescribed burn – in essence, acting as boundaries (Tolhurst and Cheney 1999). To successfully implement prescribed burns and achieve a desired level of patchiness, fire managers need to be able to predict ignitability under marginal fire-weather conditions. Next to ignitability, other determinants of burn success are the fire intensity and proportion of hazardous fuels consumed.
Spatial and temporal variation in fuel-bed ignitability is likely a key determinant of patterns in fire coverage within prescribed burns. Ignitability, how easily the fuel ignites given an ignition source, is one important component of flammability (Anderson 1970). It has been studied extensively in the laboratory, primarily in relation to leaves and plant organs (reviewed by Varner et al. 2015; Pausas et al. 2017). In these studies, several factors have been identified as important to ignitability, including moisture content, chemical composition, leaf size and particle density. However, it is unclear how these laboratory findings apply in wildland environments because of differences in fuel-bed structure and heat fluxes between the laboratory and field (Fernandes and Cruz 2012; Varner et al. 2015). Few studies specifically measure ignitability in the field (one exception is Dimitrakopoulos et al. 2010) but the factors broadly recognised as important to ignition at this scale are fuel moisture, arrangement and composition. Fuel moisture influences the amount of heat required to raise the fuel to ignition temperature and is a core component of fire-behaviour models (Keane 2015; Sullivan 2017). Fuel arrangement (e.g. bulk density and continuity) has been shown to influence fire-spread rates (Cheney et al. 1992; McCaw et al. 2012) and hence is likely to influence the likelihood of an ignition to be sustained. Species composition may influence ignitability where there are differences in volatile oil content or structural differences between species (e.g. leaf size, leaf arrangement) (Zylstra et al. 2016; Wyse et al. 2017). Understanding the drivers of ignitability at field scales is critical for predicting fire coverage.
Fire managers routinely collect information about fuel moisture in the field before deciding where and when to conduct a prescribed burn. Prescribed-burning guidelines provide moisture thresholds for successful burn implementation for surface fine fuel and sometimes profile fine fuel (e.g. Sneeuwjagt and Peet 1985; Forest Fire Management Victoria 2017); guidance for hazard sticks may also be provided (e.g. Marsden-Smedley 2011). However, there is a lack of information about moisture thresholds for other fuel elements, e.g. dead elevated litter, bark or live vegetation. Fire managers also typically evaluate fuel quantity and structure within a proposed burn unit. In Australia, this is done using visual hazard guides (Hines et al. 2010; Gould et al. 2011) where hazard scores are assigned to fuel strata on the basis of fuel amount, continuity and composition. Hazard scores (or the fuel loads derived from them) are input into fire-behaviour models to predict likely fire spread and intensity (e.g. Cheney et al. 2012), with an assumption of a positive association between hazard and intensity.
The aim of our study was to identify the key fuel-bed attributes influencing ignitability in eucalypt forest under marginal fire weather conditions. We hypothesised that surface fine-fuel moisture content would be the most important predictor of ignition success, but that ignition models could be significantly improved by considering the moisture content of other fuel components and fuel arrangement. To investigate this, we developed a methodology that can be applied during operational prescribed burns to link ignition outcomes to key attributes of the fuel being ignited.
Methods
We observed fuel bed ignitability within five prescribed burns undertaken for fuel management purposes in the Central Highlands region of Victoria, Australia (Table 1). Each burn encompassed several forest types (Department of Environment Land Water and Planning 2016) enabling us to measure ignition across a wide range of fuel conditions to find general patterns (Fig. S1 of the Supplementary material). Overstoreys were dominated by one or more Eucalyptus species. Understoreys in Wet and Damp forest typically included small tree species (e.g. Bedfordia arborescens, Pomaderris aspera) and ferns (e.g. Cyathea australis, Polystichum proliferum). Understoreys in Dry forest contained scleromorphic species (e.g. Monotoca scoparia, Hakea decurrens). Litter beds were near continuous, with depths from 1 to 70 mm.
![]() |
Burns were ignited with hand-held drip torches (3 : 1 ratio of diesel to unleaded petrol) from external edges and aerial incendiaries for internal ridgelines. We worked alongside hand-ignition crews, assessing fuel-bed attributes (fuel moisture, fuel hazard, vegetation type) at points, immediately before those points were ignited and then recorded ignition success or failure at each point. All ignition attempts were lit as backing fires (i.e. burning downhill or into the wind) (Tolhurst and Cheney 1999, p. 81). We assessed 45 ignition attempts over 8 burning days. Ignition attempts were considered independent if they were more than 500 m apart, more than 1 h apart or in different forest types.
Ignition attempts were deemed successful if burning was sustained and spread beyond the initial point(s) of ignition, and unsuccessful if the fire self-extinguished once flaming from the initial point(s) of ignition subsided. Field crews constantly modified their lighting techniques to accommodate changing fuel and weather conditions, so the degree of effort required to ignite the sampling points varied. The least intensive ignition method involved lighting individual spot fires with a spacing of 5 m. The most intensive ignition method involved strip lighting five strips of fires with 2-m gaps between each strip. If the fire did not sustain after maximum effort was applied, then ignition was deemed unsuccessful for that ignition attempt.
Fuel moisture was determined gravimetrically, with samples oven-dried for 24 h at 105°C (Matthews 2010). Fuel-bed components sampled were: (1) surface fine fuel – top 10 mm of leaf litter; (2) subsurface fine fuel – bottom 10 mm of leaf litter in contact with soil; (3) dead elevated fine fuel – dead leaves suspended in vegetation; and (4) live fine fuel – leaves of dominant grass or shrub species.
Fuel arrangement was assessed using the Overall Fuel Hazard Guide (as per Hines et al. 2010), which is a standard method for measuring fuel arrangement in Australia. Hazard scores for each fuel strata (surface, near surface, elevated and bark) were visually determined based on fuel amount, continuity and live-to-dead ratio. Those scores were then combined to determine an Overall Hazard Score (1, low hazard; 2, moderate hazard; 3, high hazard; 4, very high hazard; and 5, extreme hazard). In addition, we used Ecological Vegetation Groups (Department of Environment Land Water and Planning 2016) as a broad measure of fuel arrangement and species composition. Table S1 of the Supplementary material depicts fuel-bed attributes as a function of forest type.
Logistic-regression models of ignition success were built using all combinations of predictor variables but with the number of predictors in any model limited to three to avoid overfitting (56 different models were derived). The predictor variables were:
-
surface fine-fuel moisture content (FFMC),
-
subsurface FFMC,
-
dead elevated FFMC,
-
live FFMC,
-
surface fuel hazard score,
-
overall fuel hazard score, and
-
vegetation type.
The logistic regression model had the following form:
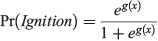
where the logit g(x) is given as:

Pr(Ignition) is the probability of ignition, xi are the independent variables and βi are the coefficients.
All statistical analysis was done in the R statistical programming language (ver. 3.4.3, R Foundation for Statistical Computing, Vienna, Austria). Akaike’s information criterion (AIC) corrected for small sample size (AICc) was used to compare levels of support for models (Burnham and Anderson 1998). Each model was assigned an Akaike weight, which is the probability that the model is the most parsimonious. The highest ranked models (within two AIC units of the best model) are presented in the results. Model selection was undertaken using the R package glmulti (Calcagno 2013).
Goodness of fit was determined using −2 log(Likelihood), McFadden R2 (likelihood ratio index) and Hosmer and Lemeshow’s goodness of fit test (Hosmer et al. 2013). Partial-residual plots (otherwise known as term plots) were derived for the highest-ranked model. In these plots, the predictor is plotted against the partial residual, which is a sum of the residual and the term for each predictor (or in other words, the part of the response not explained by the other predictors). These plots enable us to visualise the contribution of each predictor to ignition probability.
Results
The burns were conducted in autumn (March to May). Weather conditions were mild, with temperatures ranging from 16.5 to 28.2°C, relative humidity ranging from 22 to 79%, Forest Fire Danger Index (FFDI) (McArthur 1967) ranging from 1 to 24 and Keetch–Byram Drought Index (KBDI) (Keetch and Byram 1968) ranging from 101 to 140 (Table 1). Ignitions occurred in the surface or near-surface fuels, but where the ignition sustained, flaming often incorporated the shrub strata.
The model selection process identified four models that were within two AICc units of the best model (Table 2). All shortlisted models produced an adequate fit to the data: Fadden R2 ranged from 0.48 to 0.54; −2 log(Likelihood) ranged from 26.82 to 30.51 and the Hosmer and Lemeshow goodness of fit tests were not significant (P > 0.05), which means there was no significant difference between the observed and expected frequency distributions of ignition. The highest-ranked model included three predictor variables: surface FFMC, dead elevated FFMC and overall fuel hazard. As shown in the partial-residual plots (Fig. 1), all three predictor variables negatively influenced the probability of ignition, which is expected for FFMC but surprising for overall fuel hazard. Surface FFMC and overall fuel hazard were stronger predictors of ignition than dead elevated FFMC, as indicated by their steeper slopes in the partial-residual plots and larger coefficients in Table 2.
Surface FFMC was included in all four of the highest ranked models. For the single parameter model (ranked 16th in Table 2; Fig. S2), the ignition probability was 0.75 when surface FFMC was 18%. Overall fuel hazard was included in all four of the highest ranked models. Overall fuel hazard only occurred over a limited range (from High to Extreme) and there were few ignition points where the hazard was in High or Very High categories, which likely limits its strength as a predictor. Although dead elevated FFMC, subsurface FFMC and vegetation type were all included in one of the four highest ranked models, their influence in these models was not statistically significant (i.e. P > 0.05).
Discussion
Fuel moisture was a strong predictor of ignition success. This is not unexpected considering numerous field and laboratory flammability studies have identified fuel moisture as an important determinant of ignitability (e.g. Blackmarr 1972; Dimitrakopoulos and Papaioannou 2001; Plucinski and Anderson 2008; Dimitrakopoulos et al. 2010; Ellis 2015) and fire-behaviour models invariably include landscape dryness metrics (e.g. McArthur 1962; Sneeuwjagt and Peet 1985; Forestry Canada Fire Danger Group 1992).
Surface FFMC was in all four of the highest-ranked models. This result supports our hypothesis that surface FFMC would be the most important factor. Surface fuels are a key component of the fuel bed supporting the spread of fire (Gould et al. 2011; Keane 2015). Accordingly, if the surface fuels are too wet to burn then the fire will not be sustained. Additionally, surface fuels are the fuel component likely to have the greatest direct exposure to drip-torch ignitions (Tolhurst and Cheney 1999). The 18% surface-moisture threshold for a 75% probability of ignition corresponds with the upper end of moisture thresholds recommended for prescribed burning in eucalypt forests (Sneeuwjagt and Peet 1985; Tolhurst and Cheney 1999; Slijepcevic et al. 2015). Surface FFMC is a function of both atmospheric conditions (varying at sub-daily timescales) and soil moisture (varying at weekly to monthly timescales) (Viney 1991; Matthews 2014). The strong relationship between surface FFMC and ignition success suggests that both sub-daily and seasonal processes are important to ignition success.
Dead elevated and subsurface FFMC were selected once each in the four highest-ranked models but their contribution in these models was not statistically significant. This result suggests dead elevated FFMC and subsurface FFMC are weak predictors of ignition. Given they represent the dryness of the air (dead elevated) and soil (subsurface), they are a function of the same drivers of surface FFMC, so are likely to have limited independent variation.
Overall fuel hazard was in all four of the top ranked models, with increasing hazard associated with reduced ignition probability. This negative association between hazard and the probability of ignition seems at odds with the commonly assumed positive relationship between fuel hazard and flammability. Higher fuel hazards are associated with more intense fire behaviour (Hines et al. 2010; McCaw et al. 2012). Our results suggest that, in areas of higher fuel hazard, the probability of successful ignition may be reduced. This could be due to high hazards representing areas of dense live vegetation. Under marginal burning conditions, there may be insufficient heat to ignite dense live vegetation. Therefore, rather than adding to the intensity of the fire, dense near-surface and elevated fuels may act as a heat sink that retards fire spread. Furthermore, in our study, the locations with higher fuel hazards often occurred in wetter, more productive parts of the landscape where mesic species were more abundant than scleromorphic species; mesic species may be intrinsically more difficult to ignite (Dickinson and Kirkpatrick 1985; Gill and Moore 1996). A limitation of using overall fuel hazard in this study was that it was measured as ‘Extreme’ at most ignition points, potentially limiting its predictive ability. Future studies should consider using more specific measures of fuel structure and composition rather than hazard scores to provide a potentially more precise indication of the factors driving ignition.
Other indicators of fuel arrangement – surface hazard and vegetation type – were also considered in the model selection process. Surface hazard did not appear in the highest ranked models, potentially because it was accounted for already within overall fuel hazard. Vegetation type appeared in one of the highest ranked models, suggesting that there may be some other characteristics of the vegetation not captured in the overall fuel-hazard score that influence ignition (e.g. species composition or the amount of solar radiation). However, because vegetation type only appeared in one high-ranking model, support for it as a predictor of ignition success was weak relative to overall fuel hazard.
Although our highest ranked models for ignition explain a reasonable amount of variation in the data, there is a large portion that remains unexplained. That unexplained variation could be due to other factors not measured in the study such as localised weather conditions, litter-bed composition, solar radiation, dominant plant species and fuel-structure attributes not captured by fuel hazard. Further research is required to explore other variables that might explain ignition and strengthen our ability to predict fuel attributes at spatial scales (100–500 m) relevant to prescribed burning.
Conclusion
Models to accurately predict fuel bed ignitability under marginal burning conditions have the potential to improve the efficiency of prescribed burning operations. We developed a method for measuring ignitability in operational prescribed burns and found that surface FFMC and overall fuel hazard were important determinants of ignition. Further data collection is needed to build operationally useful ignition models.
Conflicts of interest
The authors declare that they have no conflicts of interest.
Declaration of funding
This research was funded by a supplementary project titled ‘Managing Bushfire in Tall Mist Forests – Fuel Hazard and Moisture Relationships’ within the integrated Forest Ecosystem Research program, a forest research program funded by the Victorian Government’s Department of Environment, Water, Land and Planning (DEWLP).
Acknowledgements
Thanks to Craig Baillie, Simon Murphy, April Gloury, Brett Cirulis, Paul Bentley and Tara Penman for assistance with data collection, and thanks to the fire managers from the Department of Environment, Land, Water and Planning for enabling us to work in their prescribed burns. The two anonymous reviewers are thanked for their thoughtful suggestions, which improved the quality of the manuscript.
References
Anderson HE (1970) Forest fuel ignitibility. Fire Technology 6, 312–319.| Forest fuel ignitibility.Crossref | GoogleScholarGoogle Scholar |
Blackmarr WH (1972) Moisture content influences ignitability of slash pine litter. USDA Forest Service, Southeastern Forest and Range Experiment Station, Research Note SE-173. (Asheville, NC, USA)
Burnham KP, Anderson DR (1998) ‘Model selection and inference. A practical information-theoretic approach.’ (Springer-Verlag: New York, NY, USA)
Calcagno V (2013) ‘Package ‘glmulti.’ Model selection and multimodel inference made easy. Available at https://cran.r-project.org/web/packages/glmulti/glmulti.pdf [Verified 22 January 2019]
Cawson JG, Sheridan GJ, Smith HG, Lane PNJ (2013) Effects of fire severity and burn patchiness on hillslope-scale surface runoff, erosion and hydrological connectiveity in a prescribed burn. Forest Ecology and Management 310, 219–233.
| Effects of fire severity and burn patchiness on hillslope-scale surface runoff, erosion and hydrological connectiveity in a prescribed burn.Crossref | GoogleScholarGoogle Scholar |
Cheney NP, Gould JS, Knight I (1992) A prescribed burning guide for young regrowth forests of silvertop ash. Forestry Commission of New South Wales. (Sydney, NSW, Australia)
Cheney NP, Gould JS, McCaw WL, Anderson WR (2012) Predicting fire behaviour in dry eucalypt forest in southern Australia. Forest Ecology and Management 280, 120–131.
| Predicting fire behaviour in dry eucalypt forest in southern Australia.Crossref | GoogleScholarGoogle Scholar |
Department of Environment Land Water and Planning (2016) Native vegetation – modelled 2005 ecological vegetation classes (with bioregional conservation status). (Victorian State Government: Melbourne, Vic., Australia) Available at https://www.data.vic.gov.au/data/dataset/native-vegetation-modelled-2005-ecological-vegetation-classes-with-bioregional-conservation-status [Verified 1 February 2018]
Dickinson KJM, Kirkpatrick JB (1985) The flammability and energy content of some important plant species and fuel components in the forests of southeastern Tasmania. Journal of Biogeography 12, 121–134.
| The flammability and energy content of some important plant species and fuel components in the forests of southeastern Tasmania.Crossref | GoogleScholarGoogle Scholar |
Dimitrakopoulos AP, Papaioannou KK (2001) Flammability assessment of mediterranean forest fuels. Fire Technology 37, 143–152.
| Flammability assessment of mediterranean forest fuels.Crossref | GoogleScholarGoogle Scholar |
Dimitrakopoulos AP, Mitsopoulos ID, Gatoulas K (2010) Assessing ignition probability and moisture of extinction in a Mediterranean grass fuel. International Journal of Wildland Fire 19, 29–34.
| Assessing ignition probability and moisture of extinction in a Mediterranean grass fuel.Crossref | GoogleScholarGoogle Scholar |
Ellis PFM (2015) The likelihood of ignition of dry-eucalypt forest litter by firebrands. International Journal of Wildland Fire 24, 225–235.
| The likelihood of ignition of dry-eucalypt forest litter by firebrands.Crossref | GoogleScholarGoogle Scholar |
Fernandes PM, Cruz MG (2012) Plant flammability experiments offer limited insight into vegetation-fire dynamics interactions. New Phytologist 194, 606–609.
| Plant flammability experiments offer limited insight into vegetation-fire dynamics interactions.Crossref | GoogleScholarGoogle Scholar | 22288940PubMed |
Forest Fire Management Victoria (2017) Bushfire management manual 3. Fuel management. (Melbourne, Vic., Australia)
Forestry Canada Fire Danger Group (1992) Development and structure of the Canadian forest fire behaviour prediction system. Forestry Canada, Science and Sustainable Development Directorate. (Ottawa, ON, Canada)
Gill AM, Moore PHR (1996) Ignitability of leaves of Australian plants. Centre for Plant Biodiversity Research, CSIRO Plant Industry. (Canberra, ACT, Australia)
Gould JS, McCaw WL, Cheney NP (2011) Quantifying fine fuel dynamics and structure in dry eucalypt forest (Eucalyptus marginata) in Western Australia for fire management. Forest Ecology and Management 262, 531–546.
| Quantifying fine fuel dynamics and structure in dry eucalypt forest (Eucalyptus marginata) in Western Australia for fire management.Crossref | GoogleScholarGoogle Scholar |
Hines F, Tolhurst KG, Wilson AAG, McCarthy GJ (2010) Overall fuel hazard assessment guide, 4th edn. Fire Management Branch, Department of Sustainability and Environment, report number 82. (Melbourne, Vic., Australia)
Hosmer DW, Lemeshow S, Sturdivant RX (2013) ‘Applied Logistic Regression.’ (Wiley: Hoboken, NJ, USA)
Keane RE (2015) ‘Wildland Fuel Fundamentals and Application.’ (Springer International Publishing: Cham, Switzerland)
Keetch JJ, Byram GM (1968) A drought index for forest fire control. USDA Forest Service, Research Paper SE-38. (Asheville, NC, USA)
Knapp EE, Keeley JE (2006) Heterogeneity in fire severity within early season and late season prescribed burns in a mixed-conifer forest. International Journal of Wildland Fire 15, 37–45.
| Heterogeneity in fire severity within early season and late season prescribed burns in a mixed-conifer forest.Crossref | GoogleScholarGoogle Scholar |
Marsden-Smedley JB (2011) Planned burning in Tasmania. III. Revised guidelines for conductuing planned burning. Tasforests 19, 122–134.
Matthews S (2010) Effect of drying temperature on fuel moisture content measurements. International Journal of Wildland Fire 19, 800–802.
| Effect of drying temperature on fuel moisture content measurements.Crossref | GoogleScholarGoogle Scholar |
Matthews S (2014) Dead fuel moisture research: 1991–2012. International Journal of Wildland Fire 23, 78–92.
| Dead fuel moisture research: 1991–2012.Crossref | GoogleScholarGoogle Scholar |
McArthur AG (1962) Control burning in Eucalypt forests. Commonwealth of Australia. Forestry and Timber Bureau Leaflet 80. (Canberra, ACT, Australia)
McArthur AG (1967) Fire behaviour in eucalypt forests. Commonwealth of Australia, Forestry and Timber Bureau Leaflet 107. (Canberra, ACT, Australia)
McCaw WL, Gould JS, Cheney NP, Ellis PFM, Anderson WR (2012) Changes in behaviour of fire in dry eucalypt forest as fuel increases with age. Forest Ecology and Management 271, 170–181.
| Changes in behaviour of fire in dry eucalypt forest as fuel increases with age.Crossref | GoogleScholarGoogle Scholar |
Pausas JG, Keeley JE, Schwilk DW (2017) Flammability as an ecological and evolutionary driver. Journal of Ecology 105, 289–297.
| Flammability as an ecological and evolutionary driver.Crossref | GoogleScholarGoogle Scholar |
Penman TD, Kavanagh RP, Binns DL, Melick DR (2007) Patchiness of prescribed burns in dry sclerophyll eucalypt forests in south-eastern Australia. Forest Ecology and Management 252, 24–32.
| Patchiness of prescribed burns in dry sclerophyll eucalypt forests in south-eastern Australia.Crossref | GoogleScholarGoogle Scholar |
Plucinski MP, Anderson WR (2008) Laboratory determination of factors influencing successful point ignition in the litter layer of shurbland vegetation. International Journal of Wildland Fire 17, 628–637.
| Laboratory determination of factors influencing successful point ignition in the litter layer of shurbland vegetation.Crossref | GoogleScholarGoogle Scholar |
Robinson NM, Leonard SWJ, Ritchie EG, Bassett M, Chia EK, Buckingham S, Gibb H, Bennett AF, Clarke MF (2013) REVIEW: Refuges for fauna in fire-prone landscapes: their ecological function and importance. Journal of Applied Ecology 50, 1321–1329.
| REVIEW: Refuges for fauna in fire-prone landscapes: their ecological function and importance.Crossref | GoogleScholarGoogle Scholar |
Slijepcevic A, Anderson WR, Matthews S, Anderson DH (2015) Evaluating models to predict daily fine fuel moisture content in eucalypt forest. Forest Ecology and Management 335, 261–269.
| Evaluating models to predict daily fine fuel moisture content in eucalypt forest.Crossref | GoogleScholarGoogle Scholar |
Sneeuwjagt R, Peet GB (1985) ‘Forest Fire Behaviour Tables for Western Australia’, 3rd edn. (Western Australian Department of Conservation and Land Management, Perth, WA, Australia)
Sullivan AL (2017) Inside the inferno: fundamental processes of wildland fire behaviour. Part 2: Heat transfer and interactions. Current Forestry Reports 3, 150–171.
| Inside the inferno: fundamental processes of wildland fire behaviour. Part 2: Heat transfer and interactions.Crossref | GoogleScholarGoogle Scholar |
Tolhurst, KG, Cheney, NP (1999) ‘Synopsis of the Knowledge used in Prescribed Burning in Victoria.’ (Department of Natural Resources and Environment, Fire Management: Melbourne, Vic., Australia)
Varner JM, Kane JM, Kreye JK, Engber E (2015) The Flammability of Forest and Woodland Litter: a Synthesis. Current Forestry Reports 1, 91–99.
| The Flammability of Forest and Woodland Litter: a Synthesis.Crossref | GoogleScholarGoogle Scholar |
Viney NR (1991) A review of fine fuel moisture modelling. International Journal of Wildland Fire 1, 215–234.
| A review of fine fuel moisture modelling.Crossref | GoogleScholarGoogle Scholar |
Wyse SV, Perry GLW, Curran TJ (2017) Shoot-level flammability of species mixtures is driven by the most flammable species: implications for vegetation-fire feedbacks favouring invasive species. Ecosystems 21, 886–900.
| Shoot-level flammability of species mixtures is driven by the most flammable species: implications for vegetation-fire feedbacks favouring invasive species.Crossref | GoogleScholarGoogle Scholar |
Zylstra P, Bradstock RA, Bedward M, Penman TD, Doherty MD, Weber RO, Gill AM, Cary GJ (2016) Biophysical mechanistic modelling quantifies the effects of plant traits on fire severity: species, not surface fuel loads, determine flame dimensions in eucalypt forests. PLoS One 11, e0160715
| Biophysical mechanistic modelling quantifies the effects of plant traits on fire severity: species, not surface fuel loads, determine flame dimensions in eucalypt forests.Crossref | GoogleScholarGoogle Scholar | 27529789PubMed |