Quantifying merging fire behaviour phenomena using unmanned aerial vehicle technology
Alexander Filkov

A School of Ecosystem and Forest Sciences, University of Melbourne, Creswick, Vic. 3363, Australia.
B Bushfire and Natural Hazards Cooperative Research Centre, Melbourne, Vic. 3002, Australia.
C Corresponding author. Email: alexander.filkov@unimelb.edu.au
International Journal of Wildland Fire 30(3) 197-214 https://doi.org/10.1071/WF20088
Submitted: 10 June 2020 Accepted: 20 November 2020 Published: 10 December 2020
Journal Compilation © IAWF 2021 Open Access CC BY
Abstract
Catastrophic wildfires are often a result of dynamic fire behaviours. They can cause rapid escalation of fire behaviour, increasing the danger to ground-based emergency personnel. To date, few studies have characterised merging fire behaviours outside the laboratory. The aim of this study was to develop a simple, fast and accurate method to track fire front propagation using emerging technologies to quantify merging fire behaviour at the field scale. Medium-scale field experiments were conducted during April 2019 on harvested wheat fields in western Victoria, Australia. An unmanned aerial vehicle was used to capture high-definition video imagery of fire propagation. Twenty-one junction and five inward parallel fire fronts were identified during the experiments. The rate of spread (ROS) of junction fire fronts was found to be at least 60% higher than head fire fronts. Thirty-eight per cent of junction fire fronts had increased ROS at the final stage of the merging process. Furthermore, the angle between two junction fire fronts did not change significantly in time for initial angles of 4–14°. All these results contrast with previous published work. Further investigation is required to explain the results as the relationship between fuel load, wind speed and scale is not known.
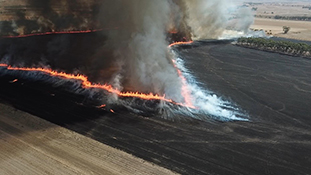
Keywords: automatic georeferencing, fast post-processing, field experiments, fire front propagation and tracking, merging fire fronts, operational and management application, remote measurements, UAV.
References
Cheney N, Gould J (1997) Fire growth and acceleration. International Journal of Wildland Fire 7, 1–5.| Fire growth and acceleration.Crossref | GoogleScholarGoogle Scholar |
Cheney NP, Gould J, Anderson W (1993) The influence of fuel, weather and fire shape variables on fire-spread in grasslands. International Journal of Wildland Fire 3, 31–44.
| The influence of fuel, weather and fire shape variables on fire-spread in grasslands.Crossref | GoogleScholarGoogle Scholar |
Coen JL, Cameron M, Michalakes J, Patton EG, Riggan PJ, Yedinak KM (2013) WRF-Fire: coupled weather–wildland fire modeling with the weather research and forecasting model. Journal of Applied Meteorology and Climatology 52, 16–38.
| WRF-Fire: coupled weather–wildland fire modeling with the weather research and forecasting model.Crossref | GoogleScholarGoogle Scholar |
Doogan M (2006) ‘The Canberra firestorm: inquests and inquiry into four deaths and four fires between 8 and 18 January 2003: Vol. I.’ (ACT Magistrates Court: Canberra, ACT, Australia)
Fernández-Guisuraga JM, Sanz-Ablanedo E, Suárez-Seoane S, Calvo L (2018) Using unmanned aerial vehicles in post-fire vegetation survey campaigns through large and heterogeneous areas: opportunities and challenges. Sensors 18, 586
| Using unmanned aerial vehicles in post-fire vegetation survey campaigns through large and heterogeneous areas: opportunities and challenges.Crossref | GoogleScholarGoogle Scholar |
Filkov AI, Duff TJ, Penman TD (2018) Improving fire behaviour data obtained from wildfires. Forests 9, 81
| Improving fire behaviour data obtained from wildfires.Crossref | GoogleScholarGoogle Scholar |
Filkov AI, Duff TJ, Penman TD (2020a) Frequency of dynamic fire behaviours in Australian forest environments. Fire 3, 1–19.
| Frequency of dynamic fire behaviours in Australian forest environments.Crossref | GoogleScholarGoogle Scholar |
Filkov AI, Ngo T, Matthews S, Telfer S, Penman TD (2020b) Impact of Australia’s catastrophic 2019/20 bushfire season on communities and environment. Retrospective analysis and current trends. Journal of Safety Science and Resilience 1, 44–56.
| Impact of Australia’s catastrophic 2019/20 bushfire season on communities and environment. Retrospective analysis and current trends.Crossref | GoogleScholarGoogle Scholar |
Frangieh N, Morvan D, Meradji S, Accary G, Bessonov O (2018) Numerical simulation of grassland fires behavior using an implicit physical multiphase model. Fire Safety Journal 102, 37–47.
| Numerical simulation of grassland fires behavior using an implicit physical multiphase model.Crossref | GoogleScholarGoogle Scholar |
Hilton J, Sharples J, Sullivan A, Swedosh W (2017) Simulation of spot fire coalescence with dynamic feedback. In ‘MODSIM2017: 22nd International congress on modelling and simulation’, 3–8 December 2017, Hobart, Tas., Australia (Eds G Syme, D Hatton MacDonald, B Fulton, J Piantadosi) pp. 1111–1117. (Modelling and Simulation Society of Australia and New Zealand)
Hilton JE, Sullivan AL, Swedosh W, Sharples J, Thomas C (2018) Incorporating convective feedback in wildfire simulations using pyrogenic potential. Environmental Modelling & Software 107, 12–24.
| Incorporating convective feedback in wildfire simulations using pyrogenic potential.Crossref | GoogleScholarGoogle Scholar |
Hua L, Shao G (2017) The progress of operational forest fire monitoring with infrared remote sensing. Journal of Forestry Research 28, 215–229.
| The progress of operational forest fire monitoring with infrared remote sensing.Crossref | GoogleScholarGoogle Scholar |
Macdonald O (2017) Getting to know ArcGIS Pro. The Cartographic Journal 54, 284–285.
| Getting to know ArcGIS Pro.Crossref | GoogleScholarGoogle Scholar |
Merino L, Caballero F, Martínez-de-Dios JR, Maza I, Ollero A (2012) An unmanned aircraft system for automatic forest fire monitoring and measurement. Journal of Intelligent and Robotic Systems: Theory and Applications 65, 533–548.
| An unmanned aircraft system for automatic forest fire monitoring and measurement.Crossref | GoogleScholarGoogle Scholar |
Moran CJ, Seielstad CA, Cunningham MR, Hoff V, Parsons RA, Queen L, Sauerbrey K, Wallace T (2019) Deriving fire behavior metrics from UAS imagery. Fire 2, 36
| Deriving fire behavior metrics from UAS imagery.Crossref | GoogleScholarGoogle Scholar |
Morvan D, Frangieh N (2018) Wildland fires behaviour: wind effect versus Byram’s convective number and consequences upon the regime of propagation. International Journal of Wildland Fire 27, 636–641.
| Wildland fires behaviour: wind effect versus Byram’s convective number and consequences upon the regime of propagation.Crossref | GoogleScholarGoogle Scholar |
Plucinski MP, Anderson WR (2008) Laboratory determination of factors influencing successful point ignition in the litter layer of shrubland vegetation. International Journal of Wildland Fire 17, 628–637.
| Laboratory determination of factors influencing successful point ignition in the litter layer of shrubland vegetation.Crossref | GoogleScholarGoogle Scholar |
Ranganathan A (2004) The Levenberg–Marquardt algorithm. Available at https://www.academia.edu/660131/The_levenberg_marquardt_algorithm [Verified 27 November 2020]
Raposo JR, Viegas DX, Xie X, Almeida M, Figueiredo AR, Porto L, Sharples J (2018) Analysis of the physical processes associated with junction fires at laboratory and field scales. International Journal of Wildland Fire 27, 52–68.
| Analysis of the physical processes associated with junction fires at laboratory and field scales.Crossref | GoogleScholarGoogle Scholar |
Ross T (1993) Bělehrádek-type models. Journal of Industrial Microbiology 12, 180–189.
| Bělehrádek-type models.Crossref | GoogleScholarGoogle Scholar |
Rossa CG (2017) The effect of fuel moisture content on the spread rate of forest fires in the absence of wind or slope. International Journal of Wildland Fire 26, 24–31.
| The effect of fuel moisture content on the spread rate of forest fires in the absence of wind or slope.Crossref | GoogleScholarGoogle Scholar |
Sullivan AL, Swedosh W, Hurley RJ, Sharples JJ, Hilton JE (2019) Investigation of the effects of interactions of intersecting oblique fire lines with and without wind in a combustion wind tunnel. International Journal of Wildland Fire 28, 704–719.
| Investigation of the effects of interactions of intersecting oblique fire lines with and without wind in a combustion wind tunnel.Crossref | GoogleScholarGoogle Scholar |
Tedim F, Leone V, Amraoui M, Bouillon C, Coughlan RM, Delogu MG, Fernandes MP, Ferreira C, McCaffrey S, McGee KT, Parente J, Paton D, Pereira GM, Ribeiro ML, Viegas DX, Xanthopoulos G (2018) Defining extreme wildfire events: difficulties, challenges, and impacts. Fire 1, 9
| Defining extreme wildfire events: difficulties, challenges, and impacts.Crossref | GoogleScholarGoogle Scholar |
Thomas CM, Sharples JJ, Evans JP (2017) Modelling the dynamic behaviour of junction fires with a coupled atmosphere–fire model. International Journal of Wildland Fire 26, 331–344.
| Modelling the dynamic behaviour of junction fires with a coupled atmosphere–fire model.Crossref | GoogleScholarGoogle Scholar |
Viegas DX (2012) Extreme fire behaviour. In ‘Forest management: technology, practices and impact.’ (Eds ACB Cruz, REG Correia) pp. 1–56 (Nova Science Publishers, Inc.: New York, NY, USA)
Viegas DX, Raposo JR, Davim DA, Rossa CG (2012) Study of the jump fire produced by the interaction of two oblique fire fronts. Part 1. Analytical model and validation with no-slope laboratory experiments. International Journal of Wildland Fire 21, 843–856.
| Study of the jump fire produced by the interaction of two oblique fire fronts. Part 1. Analytical model and validation with no-slope laboratory experiments.Crossref | GoogleScholarGoogle Scholar |
Viegas DX, Raposo J, Figueiredo A (2013) Preliminary analysis of slope and fuel bed effect on jump behavior in forest fires. Procedia Engineering 62, 1032–1039.
| Preliminary analysis of slope and fuel bed effect on jump behavior in forest fires.Crossref | GoogleScholarGoogle Scholar |
Williams J, Hamilton L (2005) The mega-fire phenomenon: toward a more effective management model. A concept paper. Brookings Institution. (Washington, DC, USA) Available at http://www.bushfirecrc.com/sites/default/files/managed/resource/mega-fire_concept_paper_september_20_ 2005.pdf [Verified 9 September 2019]