SNP-bin linkage analysis and genome-wide association study of plant height in soybean
Jiajing Wang A , Bo Hu A , Shanshan Huang B , Xiping Hu B , Mahfishan Siyal A , Chang Yang A , Hengxing Zhao A , Tao Yang A , Haoran Li A , Yongqin Hou A , Cuiqiao Liu A , Xu Sun A , Raja Rameez Veesar A , Wen-Xia Li A and Hailong Ning
A Key Laboratory of Soybean Biology, Ministry of Education, Key Laboratory of Soybean Biology and Breeding/Genetics, Ministry of Agriculture, Northeast Agricultural University, Harbin 150030, China.
B Key Laboratory of Crop Biotechnology Breeding of the Ministry of Agriculture, Beidahuang Kenfeng Seed Co. Ltd, Harbin, China.
Crop & Pasture Science 73(3) 222-237 https://doi.org/10.1071/CP21128
Submitted: 25 February 2021 Accepted: 1 October 2021 Published: 25 January 2022
© 2022 The Author(s) (or their employer(s)). Published by CSIRO Publishing. This is an open access article distributed under the Creative Commons Attribution-NonCommercial-NoDerivatives 4.0 International License (CC BY-NC-ND)
Abstract
As the major source of edible protein and oil, the global demand for soybean (Glycine max (L.) Merr.) is increasing. Plant height is closely related to yield; therefore, understanding the genetic basis of plant height will help to improve soybean plant type and increase seed yield. In this study, quantitative trait loci (QTLs) and nucleotides (QTNs) for soybean plant height were detected by linkage analysis and association analysis. A high-density map containing 2225 bin markers was constructed by using 108 342 SNPs of a recombinant inbred line population (named RIL3613) of 120 lines for linkage analysis. In total, 39 QTLs were detected, including 16 QTLs that were repeatedly detected in multiple environments. Association analysis was performed by using 63 306 SNPs from a germplasm population of 455 natural soybean accessions. In total, 62 QTNs were detected, and 26 QTNs were repeatedly detected by multiple methods. Fourteen QTNs were distributed in the intervals of six multiple-environment QTLs by comparing the results of association analysis and linkage analysis. With pathway analysis, six candidate genes were identified as being associated with plant height. These results contribute to analysis of the genetic basis of plant height and will promote marker-assisted selection for breeding ideal plant shape.
Keywords: Genes, GWAS, linkage analysis, plant height, QTL, QTN, SNP-bin marker, soybean.
Introduction
Soybean (Glycine max (L.) Merr.) is a major source of edible protein and oil, widely used in feed, food and industry (Lee et al. 2019). With the continuous development of food processing technology, the demand for soybean globally has been increasing. Therefore, increasing yield is an important breeding goal for soybean. Plant height is closely related to yield; a plant that is too short will have reduced yield, whereas a plant that is too tall will easily lodge, leading to a reduction in yield. Plant height is positively correlated with pod number per plant and node number of the main stem (Akhter and Sneller 1996; Chang et al. 2018). Plant height is a typical quantitative trait controlled by multiple genes and influenced by environment (Lee et al. 1996). It is important to identify and study quantitative trait loci (QTLs) related to plant height in order to guide marker-assisted selection (MAS) and research the molecular basis of the trait.
On a basis of a genetic linkage map, linkage analysis of QTL mapping is the primary means of studying the inheritance of complex traits. In total, 230 QTLs associated with plant height have been listed in SoyBase (http://soybase.org/, 10 November 2020). However, soybean genetic maps in most previous studies were constructed based on low-throughput molecular markers such as restriction fragment length polymorphism (RFLP), amplified fragment length polymorphism (AFLP) and simple sequence repeat (SSR) markers (Keim et al. 1997; Xia et al. 2007; Priolli et al. 2015). The lack of high-throughput molecular markers meant insufficient precision of mapped QTLs in the larger intervals, for which it was difficult to apply QTLs to predict candidate genes, further limiting MAS for plant height. Single nucleotide polymorphism (SNP) markers have become important in the construction of genetic maps and have being widely used to map QTLs for flowering period (Adewusi et al. 2017), branch number (Yang et al. 2017), protein and oil content (Patil et al. 2018; Seo et al. 2019), and 100-seed weight (Karikari et al. 2019). For plant height, Lee et al. (2015) mapped six QTLs in a genetic map containing 516 SNP markers, and Cao et al. (2019) mapped eight and 12 QTLs with a high-density genetic map of two recombinant inbred line (RIL) populations containing 3958 and 2600 SNP-bin markers, respectively.
Genome-wide association study (GWAS), also known as linkage disequilibrium (LD) mapping, is another common method for mapping QTLs of complex quantitative traits (Lü et al. 2016). With the continuous development of molecular markers, GWAS based on high-density SNPs has been applied to the analysis of various complex quantitative traits in various soybean populations. Qi et al. (2020) identified 118 quantitative trait nucleotides (QTNs) controlling 100-seed weight of soybean, with the use of 109 676 SNP markers from 144 four RILs. Li et al. (2018) identified six SNPs controlling soybean oil content and six SNPs controlling soybean protein content with the use of 1536 SNPs from 421 natural populations. Jing et al.(2019) identified 14 SNPs related to soybean plant height with the use of 33 149 SNPs from 185 natural populations. Compared with linkage analysis using an artificial mapping population, association analysis using a natural population could be capable of detecting more alleles (Yu and Buckler 2006; Tian et al. 2011; Li et al. 2018). Natural populations will accumulate a large number of recombinations in the long-term evolutionary process, and the results of GWAS with natural populations have higher resolution, which is beneficial for predicting candidate genes (Sonah et al. 2015). However, association analysis is prone to false positive results (Cao et al. 2017). By the combination of linkage analysis and GWAS, results could be verified mutually firstly, and then the interval size of linkage analysis could be shortened for the prediction of candidate genes and the false positive rate of association analysis could be decreased. At present, there are few reports on the combination of the two methods to study plant height of soybean (Cao et al. 2017; Li et al. 2020).
On basis of the QTL mapping, more genes involved in formation of plant height have been mined. Plant height is affected by stem growth habit and growth period genes (Tang 2020; Wang 2020), and is controlled by a large number of QTLs. However, only a few related genes have been cloned and the molecular genetic mechanism underlying plant height is unclear. More genes need to be screened and identified.
Here, QTL mapping of soybean plant height has been conducting using the combination of linkage analysis of a RIL population derived from the cross of Dongnong L13 × Heihe 36, and GWAS of a germplasm population of 455 natural varieties. The candidate genes associated with soybean plant height were then predicted and verified by RT-qPCR analysis. The objective was to lay a foundation from which to probe the genetic basis and accelerate MAS for soybean plant shape breeding.
Materials and methods
Plant materials
The plant material included a linkage panel and a germplasm panel. The linkage panel consisted of 120 RILs derived from a cross between two soybean cultivars, Dongnong L13 and Heihe 36, which differed in plant height. Dongnong L13 was bred by crossing Heinong 40 and Jiujiao 5640, and Heihe 36 was bred by crossing Bei 87-9 and Jiusan 90-66. The cross was performed in Harbin and F1 seeds were harvested in 2008. After the pseudo hybrid crosses were eliminated in 2009, seeds were planted in Harbin (126.63°E, 45.75°N) in summer and Yacheng (109.00°E, 17.50°N) in winter from 2010 to 2014. The single-seed descent method was applied to treat the offspring in each generation. Then a RIL population containing 120 lines was obtained, named as RIL3613, with the detailed procedure stated in Ning et al. (2018). The germplasm panel comprised 455 soybean accessions including four landraces, 387 domestic and 44 foreign cultivars.
Field experiments and phenotypic data collection
The RIL3613 population was planted in 21 environments, and the germplasm population was planted in Harbin and Shuangyashan (E131.16°, N46.64°) in 2018 and 2019, respectively (Supplementary Table S1). A replicated randomised block design was adopted in the experiment. Each block contained 15 lines, and there were three replicates. Ridges were 3 m long and 0.67 m wide, on which the seeds were sown in single row with the plant spacing set as shown in Table S1. Field management was similar to the local field management.
Plant height was defined as the length from cotyledon mark to the top of main stem, in cm, and was investigated in the field after maturity. Ten plants in the middle of each ridge were randomly selected and for plant height measurement. The mean of 10 plants was used as the observed value of the plot, and the mean of the observed values of the three replications was used for QTL and QTN mapping.
Analyses of phenotypic variation
A frequency distribution histogram was drawn and descriptive statistics analyses was performed according to the average value of three repeated phenotypes of plant height in each environment. Analysis of variance (ANOVA) and estimation of generalised heritability were performed according to the three repeated phenotypic values of plant height in multiple environments. The statistical model of ANOVA for multiple environments was as follows:

where xeijk is the observed value of ith genotype of kth block in jth replication of eth environment; μ is the grand average; Gi is the ith genotype effect; Ee is the eth environment effect; Rj is the jth replication effect; Bk is the kth block in jth replication; RBjk is the interaction effect between jth replication and kth block; Bk(Gi) is ith genotype in kth block; ERej is interaction between eth environment and jth replication; EBek is interaction between eth environment and kth block; ERBejk is interaction effect among eth environment and jth replication and kth block; EBek(Gi) is ith genotype under interaction between eth environment and kth block; and εeijk is the error effect following N(0, σ2).
Generalised heritability for multiple environments was estimated as:
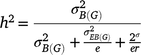
where h2 is the generalised heritability of mean over multiple environments, is the variance of genotype under block,
is the variance of genotype under environment × block interaction, σ2 is the error variance, e is the number of environments, and r is the number of repetitions in each environment. Significance of each factor was tested by the general linear model method and variance estimated by the mixed method implemented in SAS 9.2 (SAS Institute, Cary, NC, USA).
SNP genotyping
All lines in RIL3613 and the germplasm population were genotyped, with SoySNP660K BeadChip (Beijing Boao Biotechology, Beijing, China) and SoySNP180K BeadChip (Beidahuang Kenfeng Seed, Harbin, China), respectively. In total, 108 342 SNPs and 63 306 SNPs were obtained on 20 chromosomes after quality filtering of the two populations based on the criteria of allele frequency (MAF) >0.05 and maximum missing sites per SNP <10% (Belamkar et al. 2016).
Bin marker map and QTL analysis
The possible crosspoints for the SNPs of RIL3613 were determined by the Python 2.7 package SNPbinner (https://github.com/solgenomics/SNPbinner), with the minimum distance between crosspoints in base pairs of 15 SNPs. The representative bins were then created by the aggregate breakpoints resulting from crosspoints for the whole population (the minimum size of each bin is 30 kb) and were used to determine the genotypes for each RIL. The bin markers obtained were used to construct the bin linkage map with the use of QTL IciMapping version 4.1 (https://www.isbreeding.net).
Detection of additive QTLs was performed by two mapping methods, interval mapping (IM-ADD) (Lander and Botstein 1994) and inclusive composite interval mapping (ICIM-ADD) (Wang 2009), via QTL IciMapping version 4.1. The scan step was set to 1.00 cM and logarithm of odds (LOD) threshold was set to 2.50. For ICIM-ADD, the P-value for entering variables (PIN value) was set to 0.001. The QTLs were named by the method of McCouch et al. (1997).
Genome-wide association studies
Li et al. (2020) describe the population structure and LD of the germplasm population, and the results showed that there were two subgroups (K = 2), which contained 132 (29.01%) and 323 (70.99%) accessions, respectively. The LD decay distance was estimated to be 86 kb.
According to the population structure and LD results, the mrMLM.GUI software package (Zhang et al. 2020) was used for GWAS. Six methods were used to identify significant QTNs: mrMLM (Wang et al. 2016), FASTmrMLM (Tamba and Zhang 2018), FASTmrEMMA (Wen et al. 2019), pLARmEB (Zhang et al. 2017), ISIS EM-BLASSO (Tamba et al. 2017) and pKWmEB (Ren et al. 2018). In the first stage, the critical P-value of FASTmrEMMA was set to 0.005, and the critical P-value of the other methods was set to 0.01. In the final stage, the critical LOD value of significant QTNs was set to 3. All matrices used in the analysis process were also calculated by the software itself.
Identification of potential candidate genes
The candidate genes were identified from the Phytozome website (https://phytozome.jgi.doe.gov/) in the 43 kb flanking attenuation region of QTN loci that were located in the intervals of QTLs and the QTLs with shorter intervals (<500 kb) identified in the multiple environments. The genes expressed in the stem were then identified. Finally, the candidate genes related to plant height were identified through the gene annotation information and protein function in the NCBI database (https://www.ncbi.nlm.nih.gov/) and pathway analysis in the Kyoto Encyclopedia of Genes and Genomes (KEGG) (https://www.kegg.jp/).
Validation of candidate genes
The relative expression level of candidate genes in the two parents, Heihe 36 and Dongnong L13, was verified with the use of RT-qPCR. Starting from the R1 period and then every 10 days, the main stem of the third top node was sampled with three replications. Total RNA was extracted by using an OminiPlant RNA Kit (Dnase I) (CWBIO, Jiangsu, China). First-strand cDNA was synthesised with EasyScript One-Step gDNA Removal and cDNA Synthesis SuperMix kit (TransGen Biotech, Beijing, China) with the use of 2 μg total RNA. RT-qPCR was conducted by Roche LightCycler 96 (Roche, Basel, Switzerland) with SYBR Green (TOYOBO, Osaka, Japan), and the reaction system contained the following components: 10 μL SYBR Green Realtime PCR Master Mix, 0.8 μL each primer (10 μM), 6.4 μL distilled water, and 2 μL diluted cDNA. RT-qPCR was run under the following conditions: pre-denaturation 95°C for 30 s; PCR 40 cycles of 95°C for 5 s, 60°C for 20 s and 72°C for 15 s; melting curve analysis 95°C for 10 s, 65°C for 60 s and 97°C for 1 s. All PCR reactions were repeated three times. Data were processed by the 2−ΔΔCt method, with FBOX (Bansal et al. 2015) as the reference gene. The primers are shown in Table S2.
Results
Phenotypic variation of plant height
The absolute values of kurtosis and skewness were <1 in the vast majority of environments (except E12, E17 and E23) for the RIL3613 population and germplasm population, which indicated that plant height was controlled by multiple genes in these two populations (Table S3). The coefficient of variation (CV) of plant height in all environments ranged from 8.19% to 22.40% for the RIL3613 population and from 17.71% to 20.38% for the germplasm population, which indicated that plant height was greatly influenced by environment (Fig. S1, Table S3). The ANOVA showed that there were significant differences for genotype and genotype × environment interaction effects (Table 1), which indicated that different QTLs and QTNs might be detected in different environments. The multi-environmental heritability values of RIL3613 and germplasm populations were 83% and 72%, respectively, indicating that plant height was affected by the genetic effect.
![]() |
Genetic map construction and QTL analysis of plant height
The high-density map of the RIL3613 population was constructed by using 2225 bin markers covering all 20 linkage groups (LGs). The total length of the map was 2969.84 cM with an average distance of 1.33 cM between adjacent markers. The average length of each LG was 148.50 cM for the RIL3613 linkage map, and the average number of markers in each LG was 111.25 (Table 2, Fig. S2).
![]() |
Linkage analysis was performed with the use of the high-density bin map of the RIL3613 population by IM-ADD and ICIM-ADD methods. In total, 39 plant height QTLs were identified in the RIL3613 population. QTLs were detected in 12 environments as follows: E1 (1), E4 (3), E5 (19), E6 (15), E8 (3), E9 (2), E12 (2), E13 (2), E14 (1), E16 (5), E18 (1) and E19 (1). The QTLs were located on 14 linkage groups (Table S4, Fig. 1). Higher environmental variation increased the statistical error and reduced the significance of QTL detection, which resulted in the inability to detect QTLs in the other nine environments. The number of QTLs detected in each linkage group ranged from 1 to 6, and the phenotypic variation explanation (PVE) ranged from 1.11% to 18.99% (Fig. 2). There were eight QTLs (qPH-15-3, qPH-16-3, qPH-3-4, qPH-16-1, qPH-16-5, qPH-5-2, qPH-6-2, qPH-18-3) with PVE >10%, and these could be considered the main QTLs controlling plant height.
Among 16 QTLs detected in multiple environments, 14 QTLs were detected in E5 and E6, one in E8 and E9, and one in E12 and E18 with PVE of 1.16–18.99% (Table 3). The additive effects of the 16 QTLs were all negative, indicating that the synergistic genes were derived from male parent (Heihe 36). Furthermore, the physical intervals of qPH-5-1, qPH-6-2, qPH-6-4, qPH-6-5, qPH-16-3 and qPH-18-2 were <500 kb, which could be applied to the prediction of candidate genes involving plant height formation.
![]() |
QTNs detected by multilocus GWAS methods
Among 62 QTNs for plant height on 18 chromosomes (except chromosomes 11 and 20) detected by GWAS (Table S5), 20 were identified by mrMLM, 10 by FASTmrMLM, three by FASTmrEMMA, 18 by pLARmEB, 25 by ISIS EM-BLASSO and 29 by pKWmEB (Fig. 3). Of all QTNs, 15 were detected in E22, 23 in E23, 13 in E24 and 11 in E25 (Fig. 4). Single QTNs could explain phenotypic variation of 8.79 × 10−12–13.12% (Fig. 5).
![]() |
Detected by multiple methods (Table 4), 26 QTNs and the QTN effect (positive or negative) were consistent among different methods for all QTLs. Of these QTNs, four were detected by four methods, eight by three methods, and 14 by two methods (Fig. 3).
![]() |
Co-detected results by linkage analysis and association analysis
The 62 QTNs identified by association analysis in the germplasm panel were compared with the 39 QTLs identified by linkage analysis in the RIL3613 population. Fourteen QTNs detected in the germplasm panel were located in the intervals of nine QTLs identified in RIL3613: AX-90488156 in the interval of qPH-3-4; AX-90381430, AX-90340071, AX-90340537, AX-90345308 and AX-90394421 in the interval of qPH-4-1; AX-90381345 in the interval of qPH-5-2; AX-90475130, AX-90417410 and AX-90512501 in the interval of qPH-6-3; AX-90408071 and AX-90422072 in the interval of qPH-12-1; AX-90424485 and AX-90437138 in the interval of qPH-16-1 (Fig. 6).
Potential candidate gene determination
Potential candidate genes were searched within shorter intervals (<500 kb) of six QTLs (qPH-5-1, qPH-6-2, qPH-6-4, qPH-6-5, qPH-16-3 and qPH-18-2), which were repeatedly identified in multiple environments by linkage analysis. In total, 162 genes were identified, of which 140 were expressed in stems. The 140 genes were used for pathway analysis and the results showed that 46 (32.9%) of 140 genes were annotated in 70 pathways in the KEGG database (http://www.keg.jp/) (Table S6, Fig. S3).
Potential candidate genes were also searched within the 43 kb flanking attenuation region of 14 QTNs (AX-90488156, AX-90381430, AX-90340071, AX-90340537, AX-90345308, AX-90394421, AX-90381345, AX-90475130, AX-90417410, AX-90512501, AX-90408071, AX-90422072, AX-90424485 and AX-90437138), which were identified in the QTL intervals. In total, 91 genes were identified, of which 80 genes were expressed in stems. These 80 genes were used for pathway analysis, which showed that 37 (46.2%) of 80 genes were annotated in 39 pathways in the KEGG database (http://www.keg.jp/) (Table S7, Fig. S4).
According to the annotation information and metabolic function information, eight genes (Glyma.05G066200, Glyma.06G306300, Glyma.16G143600, Glyma.18G044400, Glyma.18G045100, Glyma.06G185500, Glyma.06G186400, Glyma.06G187000) were selected as potential candidate genes that might be directly or indirectly related to plant height (Table 5).
![]() |
Validation of candidate genes
The RT-qPCR technique was used to identify the relative expression levels of the eight candidate genes in the two parents. The results showed that the plant height of Dongnong L13 and Heihe 36 continued to increase from R1 to Day 40 after flowering, with significant differences in plant height at all periods. The expression levels of Glyma.05G066200, Glyma.06G306300, Glyma.06G187000 and Glyma.06G186400 in the parents increased continuously from R1 to Day 20 and then decreased from Day 20 to Day 30 (Fig. 7b, c, h, i). The expression levels of Glyma.16G143600, Glyma.18G044400, Glyma.18G045100 and Glyma.06G185500 in the parents increased from R1 to Day 10 and then decreased from Day 10 to 30 (Fig. 7d–g). Seven of the eight candidate genes (except Glyma.18G044400) showed significant differential expression at one or more periods between the two parents, and the expression levels of these seven genes of Dongnong L13 were higher than those of Heihe 36.
Discussion
As a typical quantitative trait, plant height has been studied mainly by linkage analysis or association analysis. In previous studies, the low-density genetic map of a small number of RFLP, AFLP and SSR molecular markers might result in lower detection and large intervals of QTLs. Furthermore, phenotypic data from single-environment field experiments were prone to error. With objective of lower false positive QTLs, higher thresholds of LOD were usually set (determined by permutation) to declare the existence of a QTL (Fang et al. 2020). With the continuous development of molecular marker technology, linkage maps were constructed by high density markers (such as SNP). In addition, multiple background populations and the multi-environment field experiments were utilised to collect phenotype data. In order to detect QTLs/QTNs with higher detection power and a lower false positive ratio, multiple statistical models with lower significance standards were simultaneously used to analyse the data in the multiple environments. Then the repeatedly identified QTLs/QTNs were considered authentic (Fulton et al. 1997; Li et al. 2020). We used two genetic panels with high-density SNP markers and 25 planting environments in a complex experiment to collect plant height phenotypes. Multiple statistical methods were applied for linkage analysis and GWAS. For linkage analysis, IM (Lander and Botstein 1994) and ICIM (Wang 2009) are different in theory. With IM, it is reasonable to infer the specific location of QTLs from intervals, the estimated QTL effects and position are unbias if there is only one QTL on one chromosome. ICIM has low sampling error and high precision and efficiency of detection. Furthermore, the LOD value 2.5 could guarantee high detection power.
For the association analysis, compared with single-locus MLM (Yu et al 2006), six methods for multiple-locus GWAS were different in theory, including mrMLM (Wang et al. 2016), FASTmrMLM (Tamba and Zhang 2018), FASTmrEMMA (Wen et al. 2019), pLARmEB (Zhang et al. 2017), ISIS EM-BLASSO (Tamba et al. 2017) and pKWmEB (Ren et al. 2018). None of these six multilocus methods required Bonferroni correction. Therefore, many more QTN loci could be detected. In addition to different algorithms for the selection of correlated markers, all of these methods have been applied to the selected markers in one model. Then all effects were estimated in the model by empirical Bayes method, and further, all non-zero effects were identified by the likelihood ratio test. These methods had high confidence and accuracy with low false-positive rates (Zhang et al. 2020).
Recently, combinations of association and linkage analysis have been used to verify QTLs/QTNs in soybean. Li et al. (2020) compared 77 QTNs for oil identified from a germplasm population with 103 oil QTLs identified from FW-RIL and found that 17 QTNs for oil were located in the intervals of oil QTLs. Fang et al. (2020) compared 10 QTNs for plant height identified in RIL6013 with nine plant height QTLs identified in RIL6013 in multiple environments and found that four of the QTNs for plant height were located in the intervals of plant height QTLs. In the present study, fourteen QTNs detected in the germplasm panel were located in the intervals of nine QTLs identified in the RIL3613 population, which confirmed the accuracy of linkage analysis and association analysis. The combination of linkage analysis and association analysis effectively reduced the interval size of QTLs and the false positives of QTNs. It was beneficial to the search for potential candidate genes.
There are about 230 QTLs and 95 QTNs associated with plant height covering each chromosome in SoyBase (http://soybase.org/20201110). In this study, 16 QTLs for plant height were detected in multiple environments, and 14 of these QTLs showed the inclusion in or overlap with intervals of QTLs detected in previous studies (Table S8): qPH-5-1 (Sun et al. 2006), qPH-5-2 (Kabelka et al. 2004; Sun et al. 2006), qPH-6-2 (Guzman et al. 2007), qPH-6-5 (Josie et al. 2007), qPH-6-4 (Gai et al. 2007), qPH-7-1 (Mansur et al. 1993; Orf et al. 1999; Specht et al. 2001), qPH-9-1 (Kabelka et al. 2004; Wang et al. 2004; Pathan et al. 2013), qPH-9-2 (Palomeque et al. 2009; Rossi et al. 2013), qPH-10-1 (Mian et al. 1998; Wang et al. 2004), qPH-12-1 (Lee et al. 1996; Specht et al. 2001; Eskandari et al. 2013), qPH-12-2 (Lee et al. 1996), qPH-15-2 (Sun et al. 2006; Liu et al. 2011), qPH-18-2 (Pathan et al. 2013) and qPH-20-1 (Sebolt et al. 2000; Chapman et al. 2003; Guzman et al. 2007). qPH-16-3 and qPH-16-5 were new QTLs. The 62 QTNs identified in this study were not overlapping or close to reported QTNs, indicating that these were new QTNs. These results confirmed the validity of QTLs/QTNs, which proves the accuracy of this study.
Based on the pathway analysis of 140 genes of QTLs identified in multiple environments within shorter intervals, a total of five genes (Table 5) may regulate the growth and development of soybean, further affecting plant height and yield of soybean. Glyma.05G066200 is involved in the synthesis of glutamate dehydrogenase (NAD(P)+), which plays a key role in nodule formation of soybean, affecting nitrogen metabolism and further affecting the growth and development of soybean (Grzechowiak et al. 2020). Glyma.06G306300 is involved in the synthesis of ATG8, one of the core components in the formation of autophagosomes, which directly mediates the expansion of autophagosomes, and whose transcripts are highly inducible in starvation. GmATG8c can promote plant growth and increase yield (Xia et al. 2012). Glyma.16G143600 is involved in the synthesis of PsbO, which can stabilise the photochemical efficiency of PSII and increase chlorophyll content to promote plant growth and increase yield under different abiotic stress factors such as cold, heat, strong light and salinity) (Sasi et al. 2018). Glyma.18G044400 is involved in the synthesis of UTP—glucose-1-phosphate uridylyltransferase, which is involved in sucrose or polysaccharide metabolism and cell wall biosynthesis, and may promote the development of plant fibre cells and affect plant height and plant growth and development (Li et al. 2014). Glyma.18G045100 is involved in the synthesis of polyamine oxidases, which play an important role in plant growth and development under biotic and abiotic stresses (especially temperature) (Gholizadeh and Mirzaghaderi 2020).
Based on the pathway analysis of 80 genes from 14 QTNs identified in the multiple environment QTL intervals, we conclude that a total of three genes (Table 5) may regulate soybean growth and development, further affecting plant height and soybean yield. Glyma.06G185500 is involved in the synthesis of protein farnesyltransferase subunit beta, which regulates the meristem of stems and flowers, and which is widely used in plant growth and development (Kang et al. 2013). Glyma.06G187000 is involved in the synthesis of two-component ARR-A family regulators, which are partially redundant negative regulators of cytokinin signaling. Cytokinin regulates many aspects of plant growth and development (Wan et al. 2011). Glyma.06G186400 is involved in the synthesis of mannose-1-phosphate guanylyltransferase, which is essential to the integrity of cell wall, morphogenesis and vitality, and may be related to plant stem growth (Lukowitz et al. 2001).
According to RT-qPCR results, the expression levels of six candidate genes (Glyma.05G066200, Glyma.06G306300, Glyma.16G143600, Glyma.18G045100, Glyma.06G185500 and Glyma.06G186400) were higher in Dongnong L13 than in Heihe 36, and the positive regulation of plant height was consistent with the functional information of the genes. Glyma.06G187000 could regulate cytokinin through negative feedback. The differences in expression levels between parents were not statistically significant from R1 to Day 20, whereas expression levels were significantly higher in Dongnong L13 than Heihe 36 at Day 30, which indicated that Glyma.06G187000 may not have a direct effect on plant height. Glyma.18G044400 may not have a key effect on plant height because no significant difference was observed in expression level between the two parents. These results could provide preliminary evidence for the potential role of these genes in plant height.
Conclusions
By linkage analysis and association analysis, 16 plant height QTLs were repeatedly detected in multiple environments in the RIL3613 population and 62 plant height QTNs were detected in the germplasm panel. Six candidate genes were identified that might affect plant height. These results will benefit future studies on the genetic basis of soybean plant height and promote MAS for breeding ideal plant shape.
Supplementary material
Supplementary material is available online.
Data availability
The data that support this study cannot be publicly shared due to privacy reasons and may be shared upon reasonable request to the corresponding author if appropriate.
Conflicts of interest
The authors declare no conflicts of interest.
Declaration of funding
This research was funded by Hundred-Thousand and Million Project of Heilongjiang Province for Engineering and Technology Science Soybean Breeding Technology Innovation and New Cultivar Breeding, grant number 2019ZX16B01; and National Key Research and Development Program of China, grant number 2017YFD0101303-6.
References
Adewusi OF, Odiyi AC, Akinyele BO (2017) Identification of genomic region governing yield related characters in soybean, Glycine max (L.) Merrill using SNP markers. Journal of Advances in Biology & Biotechnology 15, 1–9.| Identification of genomic region governing yield related characters in soybean, Glycine max (L.) Merrill using SNP markers.Crossref | GoogleScholarGoogle Scholar |
Akhter M, Sneller CH (1996) Yield and yield components of early maturing soybean genotypes in the mid-south. Crop Science 36, 877–882.
| Yield and yield components of early maturing soybean genotypes in the mid-south.Crossref | GoogleScholarGoogle Scholar |
Bansal R, Mittapelly P, Cassone BJ, Mamidala P, Redinbaugh MG, Michel A (2015) Recommended reference genes for quantitative pcr analysis in soybean have variable stabilities during diverse biotic stresses. PLoS ONE 10, e0134890
| Recommended reference genes for quantitative pcr analysis in soybean have variable stabilities during diverse biotic stresses.Crossref | GoogleScholarGoogle Scholar | 26244340PubMed |
Belamkar V, Farmer AD, Weeks NT, Kalberer SR, Blackmon WJ, Cannon SB (2016) Genomics-assisted characterization of a breeding collection of Apios americana, an edible tuberous legume. Scientific Reports 6, 34908
| Genomics-assisted characterization of a breeding collection of Apios americana, an edible tuberous legume.Crossref | GoogleScholarGoogle Scholar | 27721469PubMed |
Cao Y, Li S, Wang Z, Chang F, Kong J, Gai J, Zhao T (2017) Identification of major quantitative trait loci for seed oil content in soybeans by combining linkage and genome-wide association mapping. Frontiers in Plant Science 8, 1222
| Identification of major quantitative trait loci for seed oil content in soybeans by combining linkage and genome-wide association mapping.Crossref | GoogleScholarGoogle Scholar | 28747922PubMed |
Cao Y, Li S, Chen G, Wang Y, Bhat JA, Karikari B, Kong J, Gai J, Zhao T (2019) Deciphering the genetic architecture of plant height in soybean using two RIL populations sharing a common M8206 parent. Plants 8, 373
| Deciphering the genetic architecture of plant height in soybean using two RIL populations sharing a common M8206 parent.Crossref | GoogleScholarGoogle Scholar |
Chang F, Guo C, Sun F, Zhang J, Wang Z, Kong J, He Q, Sharmin RA, Zhao T (2018) Genome-wide association studies for dynamic plant height and number of nodes on the main stem in summer sowing soybeans. Frontiers in Plant Science 9, 1184
| Genome-wide association studies for dynamic plant height and number of nodes on the main stem in summer sowing soybeans.Crossref | GoogleScholarGoogle Scholar | 30177936PubMed |
Chapman A, Pantalone VR, Ustun A, Allen FL, Landau-Ellis D, Trigiano RN, Gresshoff PM (2003) Quantitative trait loci for agronomic and seed quality traits in an F2 and F4:6 soybean population. Euphytica 129, 387–393.
| Quantitative trait loci for agronomic and seed quality traits in an F2 and F4:6 soybean population.Crossref | GoogleScholarGoogle Scholar |
Eskandari M, Cober ER, Rajcan I (2013) Genetic control of soybean seed oil. II. QTL and genes that increase oil concentration without decreasing protein or with increased seed yield. Theoretical and Applied Genetics 126, 1677–1687.
| Genetic control of soybean seed oil. II. QTL and genes that increase oil concentration without decreasing protein or with increased seed yield.Crossref | GoogleScholarGoogle Scholar | 23536049PubMed |
Fang Y, Liu S, Dong Q, Zhang K, Tian Z, Li X, Li W, Qi Z, Wang Y, Tian X, Song J, Wang J, Yang C, Jiang S, Li W-X, Ning H (2020) Linkage analysis and multi-locus genome-wide association studies identify QTNs controlling soybean plant height. Frontiers in Plant Science 11, 9
| Linkage analysis and multi-locus genome-wide association studies identify QTNs controlling soybean plant height.Crossref | GoogleScholarGoogle Scholar | 32117360PubMed |
Fulton TM, Beck-Bunn T, Emmatty D, Eshed Y, Lopez J, Petiard V, Uhlig J, Zamir D, Tanksley SD (1997) QTL analysis of an advanced backcross of Lycopersicon peruvianum to the cultivated tomato and comparisons with QTLs found in other wild species. Theoretical and Applied Genetics 95, 881–894.
| QTL analysis of an advanced backcross of Lycopersicon peruvianum to the cultivated tomato and comparisons with QTLs found in other wild species.Crossref | GoogleScholarGoogle Scholar |
Gai J, Wang Y, Wu X, Chen S (2007) A comparative study on segregation analysis and QTL mapping of quantitative traits in plants: with a case in soybean. Frontiers of Agriculture in China 1, 1–7.
| A comparative study on segregation analysis and QTL mapping of quantitative traits in plants: with a case in soybean.Crossref | GoogleScholarGoogle Scholar |
Gholizadeh F, Mirzaghaderi G (2020) Genome-wide analysis of the polyamine oxidase gene family in wheat (Triticum aestivum L.) reveals involvement in temperature stress response. PLoS ONE 15, e0236226
| Genome-wide analysis of the polyamine oxidase gene family in wheat (Triticum aestivum L.) reveals involvement in temperature stress response.Crossref | GoogleScholarGoogle Scholar | 32866160PubMed |
Grzechowiak M, Sliwiak J, Jaskolski M, Ruszkowski M (2020) Structural studies of glutamate dehydrogenase (isoform 1) from Arabidopsis thaliana, an important enzyme at the branch-point between carbon and nitrogen metabolism. Frontiers in Plant Science 11, 754
| Structural studies of glutamate dehydrogenase (isoform 1) from Arabidopsis thaliana, an important enzyme at the branch-point between carbon and nitrogen metabolism.Crossref | GoogleScholarGoogle Scholar | 32655590PubMed |
Guzman PS, Diers BW, Neece DJ, St. Martin SK, LeRoy AR, Grau CR, Hughes TJ, Nelson RL (2007) QTL associated with yield in three backcross-derived populations of soybean. Crop Science 47, 111–122.
| QTL associated with yield in three backcross-derived populations of soybean.Crossref | GoogleScholarGoogle Scholar |
Jing Y, Zhao X, Wang J, Lian M, Teng W, Qiu L, Han Y, Li W (2019) Identification of loci and candidate genes for plant height in soybean (Glycine max) via genome-wide association study. Plant Breeding 138, 721–732.
| Identification of loci and candidate genes for plant height in soybean (Glycine max) via genome-wide association study.Crossref | GoogleScholarGoogle Scholar |
Josie J, Alcivar A, Rainho J, Kassem MA (2007) Genomic regions containing QTL for plant height, internodes length, and flower color in soybean [Glycine max (L.) Merr]. BIOS 78, 119–126.
| Genomic regions containing QTL for plant height, internodes length, and flower color in soybean [Glycine max (L.) Merr].Crossref | GoogleScholarGoogle Scholar |
Kabelka EA, Diers BW, Fehr WR, LeRoy AR, Baianu IC, You T, Neece DJ, Nelson RL (2004) Putative alleles for increased yield from soybean plant introductions. Crop Science 44, 784–791.
| Putative alleles for increased yield from soybean plant introductions.Crossref | GoogleScholarGoogle Scholar |
Kang NY, Cho C, Kim J (2013) Inducible expression of Arabidopsis response regulator 22 (ARR22), a type-C ARR, in transgenic Arabidopsis enhances drought and freezing tolerance. PLoS ONE 8, e79248
| Inducible expression of Arabidopsis response regulator 22 (ARR22), a type-C ARR, in transgenic Arabidopsis enhances drought and freezing tolerance.Crossref | GoogleScholarGoogle Scholar | 24244460PubMed |
Karikari B, Chen S, Xiao Y, Chang F, Zhou Y, Kong J, Bhat JA, Zhao T (2019) Utilization of interspecific high-density genetic map of RIL population for the QTL detection and candidate gene mining for 100-seed weight in soybean. Frontiers in Plant Science 10, 1001
| Utilization of interspecific high-density genetic map of RIL population for the QTL detection and candidate gene mining for 100-seed weight in soybean.Crossref | GoogleScholarGoogle Scholar | 31552060PubMed |
Keim P, Schupp JM, Travis SE, Clayton K, Zhu T, Shi L, Ferreira A, Webb DM (1997) A high-density soybean genetic map based on AFLP markers. Crop Science 37, 537–543.
| A high-density soybean genetic map based on AFLP markers.Crossref | GoogleScholarGoogle Scholar |
Lander ES, Botstein D. (1994) Mapping mendelian factors underlying quantitative traits using RFLP linkage map. Genetics 136, 185–199.
| Mapping mendelian factors underlying quantitative traits using RFLP linkage map.Crossref | GoogleScholarGoogle Scholar |
Lee SH, Bailey MA, Mian MA, Shipe ER, Ashley DA, Parrott WA, Hussey RS, Boerma HR (1996) Identification of quantitative trait loci for plant height, lodging, and maturity in a soybean population segregating for growth habit. Theoretical and Applied Genetics 92, 516–523.
| Identification of quantitative trait loci for plant height, lodging, and maturity in a soybean population segregating for growth habit.Crossref | GoogleScholarGoogle Scholar | 24166318PubMed |
Lee S, Jun TH, Michel AP, Rouf Mian MR (2015) SNP markers linked to QTL conditioning plant height, lodging, and maturity in soybean. Euphytica 203, 521–532.
| SNP markers linked to QTL conditioning plant height, lodging, and maturity in soybean.Crossref | GoogleScholarGoogle Scholar |
Lee S, Van K, Sung M, Nelson R, LaMantia J, McHale LK, Mian MAR (2019) Genome-wide association study of seed protein, oil and amino acid contents in soybean from maturity groups I to IV. Theoretical and Applied Genetics 132, 1639–1659.
| Crossref |
Li N, Wang L, Zhang W, Takechi K, Takano H, Lin X (2014) Overexpression of UDP-glucose pyrophosphorylase from Larix gmelinii enhances vegetative growth in transgenic Arabidopsis thaliana. Plant Cell Reports 33, 779–791.
| Overexpression of UDP-glucose pyrophosphorylase from Larix gmelinii enhances vegetative growth in transgenic Arabidopsis thaliana.Crossref | GoogleScholarGoogle Scholar | 24408396PubMed |
Li Y-H, Reif JC, Hong H-L, Li H-H, Liu Z-X, Ma Y-S, Li J, Tian Y, Li Y-F, Li W-B (2018) Genome-wide association mapping of QTL underlying seed oil and protein contents of a diverse panel of soybean accessions. Plant Science 266, 95–101.
| Genome-wide association mapping of QTL underlying seed oil and protein contents of a diverse panel of soybean accessions.Crossref | GoogleScholarGoogle Scholar | 29241572PubMed |
Li X, Zhang K, Sun X, Huang S, Wang J, Yang C, Siyal M, Wang C, Guo C, Hu X, Li W-X, Ning H (2020) Detection of QTL and QTN and candidate genes for oil content in soybean using a combination of four-way-RIL and germplasm populations. The Crop Journal 8, 802–811.
| Detection of QTL and QTN and candidate genes for oil content in soybean using a combination of four-way-RIL and germplasm populations.Crossref | GoogleScholarGoogle Scholar |
Liu W, Kim MY, Van K, Lee Y-H, Li H, Liu X, Lee S-H (2011) QTL identification of yield-related traits and their association with flowering and maturity in soybean. Journal of Crop Science and Biotechnology 14, 65–70.
| QTL identification of yield-related traits and their association with flowering and maturity in soybean.Crossref | GoogleScholarGoogle Scholar |
Lü H-Y, Li H-W, Fan R, Li H-Y, Yin J-Y, Zhang J-J, Zhang D (2016) Genome-wide association study of dynamic developmental plant height in soybean. Canadian Journal of Plant Science 97, 308–315.
| Genome-wide association study of dynamic developmental plant height in soybean.Crossref | GoogleScholarGoogle Scholar |
Lukowitz W, Nickle TC, Meinke DW, Last RL, Conklin PL, Somerville CR (2001) Arabidopsis cyt1 mutants are deficient in a mannose-1-phosphate guanylyltransferase and point to a requirement of N-linked glycosylation for cellulose biosynthesis. Proceedings of the National Academy of Sciences of the United States of America 98, 2262–2267.
| Arabidopsis cyt1 mutants are deficient in a mannose-1-phosphate guanylyltransferase and point to a requirement of N-linked glycosylation for cellulose biosynthesis.Crossref | GoogleScholarGoogle Scholar | 11226227PubMed |
Mansur LM, Orf JH, Lark KG (1993) Determining the linkage of quantitative trait loci to RFLP markers using extreme phenotypes of recombinant inbreds of soybean (Glycine max L. Merr.). Theoretical and Applied Genetics 86, 914–918.
| Determining the linkage of quantitative trait loci to RFLP markers using extreme phenotypes of recombinant inbreds of soybean (Glycine max L. Merr.).Crossref | GoogleScholarGoogle Scholar | 24193997PubMed |
McCouch SR, Cho YG, Yano M, Paul E, Blinstrub M, Morishima H, Kinoshita T (1997) Report on QTL nomenclature. Rice Genetics Newsletter 14, 11–13.
Mian MAR, Ashley DA, Vencill WK, Boerma HR (1998) QTLs conditioning early growth in a soybean population segregating for growth habit. Theoretical and Applied Genetics 97, 1210–1216.
| QTLs conditioning early growth in a soybean population segregating for growth habit.Crossref | GoogleScholarGoogle Scholar |
Ning H, Yuan J, Dong Q, Li W, Xue H, Wang Y, Tian Y, Li W-X (2018) Identification of QTLs related to the vertical distribution and seed-set of pod number in soybean [Glycine max (L.) Merri]. PLoS ONE 13, e0195830
| Identification of QTLs related to the vertical distribution and seed-set of pod number in soybean [Glycine max (L.) Merri].Crossref | GoogleScholarGoogle Scholar | 29664958PubMed |
Orf JH, Chase K, Jarvik T, Mansur LM, Cregan PB, Adler FR, Lark KG (1999) Genetics of soybean agronomic traits. I. Comparison of three related recombinant inbred populations. Crop Science 39, 1642–1651.
| Genetics of soybean agronomic traits. I. Comparison of three related recombinant inbred populations.Crossref | GoogleScholarGoogle Scholar |
Palomeque L, Li-Jun L, Li W, Hedges B, Cober ER, Rajcan I (2009) QTL in mega-environments. II. Agronomic trait QTL co-localized with seed yield QTL detected in a population derived from a cross of high-yielding adapted × high-yielding exotic soybean lines. Theoretical and Applied Genetics 119, 429–436.
| QTL in mega-environments. II. Agronomic trait QTL co-localized with seed yield QTL detected in a population derived from a cross of high-yielding adapted × high-yielding exotic soybean lines.Crossref | GoogleScholarGoogle Scholar | 19462149PubMed |
Pathan SM, Vuong T, Clark K, Lee JD, Shannon JG, Roberts CA, Ellersieck MR, Burton JW, Cregan PB, Hyten DL, Nguyen HT, Sleper DA (2013) Genetic mapping and confirmation of quantitative trait loci for seed protein and oil contents and seed weight in soybean. Crop Science 53, 765–774.
| Genetic mapping and confirmation of quantitative trait loci for seed protein and oil contents and seed weight in soybean.Crossref | GoogleScholarGoogle Scholar |
Patil G, Vuong TD, Kale S, Valliyodan B, Deshmukh R, Zhu C, Wu X, Bai Y, Yungbluth D, Lu F, Kumpatla S, Shannon JG, Varshney RK, Nguyen HT (2018) Dissecting genomic hotspots underlying seed protein, oil, and sucrose content in an interspecific mapping population of soybean using high-density linkage mapping. Plant Biotechnology Journal 16, 1939–1953.
| Dissecting genomic hotspots underlying seed protein, oil, and sucrose content in an interspecific mapping population of soybean using high-density linkage mapping.Crossref | GoogleScholarGoogle Scholar | 29618164PubMed |
Priolli RHG, Campos JB, Stabellini NS, Pinheiro JB, Vello NA (2015) Association mapping of oil content and fatty acid components in soybean. Euphytica 203, 83–96.
| Association mapping of oil content and fatty acid components in soybean.Crossref | GoogleScholarGoogle Scholar |
Qi Z, Song J, Zhang K, Liu S, Tian X, Wang Y, Fang Y, Li X, Wang J, Yang C, Jiang S, Sun X, Tian Z, Li W, Ning H (2020) Identification of QTNs controlling 100-seed weight in soybean using multilocus genome-wide association studies. Frontiers in Genetics 11, 689
| Identification of QTNs controlling 100-seed weight in soybean using multilocus genome-wide association studies.Crossref | GoogleScholarGoogle Scholar | 32765581PubMed |
Ren W-L, Wen Y-J, Dunwell JM, Zhang Y-M (2018) pKWmEB: integration of Kruskal–Wallis test with empirical Bayes under polygenic background control for multi-locus genome-wide association study. Heredity 120, 208–218.
| pKWmEB: integration of Kruskal–Wallis test with empirical Bayes under polygenic background control for multi-locus genome-wide association study.Crossref | GoogleScholarGoogle Scholar | 29234158PubMed |
Rossi ME, Orf JH, Liu L-J, Dong Z, Rajcan I (2013) Genetic basis of soybean adaptation to North American vs. Asian mega-environments in two independent populations from Canadian × Chinese crosses. Theoretical and Applied Genetics 126, 1809–1823.
| Genetic basis of soybean adaptation to North American vs. Asian mega-environments in two independent populations from Canadian × Chinese crosses.Crossref | GoogleScholarGoogle Scholar | 23595202PubMed |
Sasi S, Venkatesh J, Daneshi RF, Gururani MA (2018) Photosystem II extrinsic proteins and their putative role in abiotic stress tolerance in higher plants. Plants 7, 100
| Photosystem II extrinsic proteins and their putative role in abiotic stress tolerance in higher plants.Crossref | GoogleScholarGoogle Scholar |
Sebolt AM, Shoemaker RC, Diers BW (2000) Analysis of a quantitative trait locus allele from wild soybean that increases seed protein concentration in soybean. Crop Science 40, 1438–1444.
| Analysis of a quantitative trait locus allele from wild soybean that increases seed protein concentration in soybean.Crossref | GoogleScholarGoogle Scholar |
Seo JH, Kim KS, Ko JM, Choi MS, Kang BK, Kwon SW, Jun TH (2019) Quantitative trait locus analysis for soybean (Glycine max) seed protein and oil concentrations using selected breeding populations. Plant Breeding 138, 95–104.
| Quantitative trait locus analysis for soybean (Glycine max) seed protein and oil concentrations using selected breeding populations.Crossref | GoogleScholarGoogle Scholar |
Sonah H, O’Donoughue L, Cober E, Rajcan I, Belzile F (2015) Identification of loci governing eight agronomic traits using a GBS-GWAS approach and validation by QTL mapping in soya bean. Plant Biotechnology Journal 13, 211–221.
| Identification of loci governing eight agronomic traits using a GBS-GWAS approach and validation by QTL mapping in soya bean.Crossref | GoogleScholarGoogle Scholar | 25213593PubMed |
Specht JE, Chase K, Macrander M, Graef GL, Chung J, Markwell JP, Germann M, Orf JH, Lark KG (2001) Soybean response to water. a QTL analysis of drought tolerance. Crop Science 41, 493–509.
| Soybean response to water. a QTL analysis of drought tolerance.Crossref | GoogleScholarGoogle Scholar |
Sun D, Li W, Zhang Z, Chen Q, Ning H, Qiu L, Sun G (2006) Quantitative trait loci analysis for the developmental behavior of soybean (Glycine max L. Merr.). Theoretical and Applied Genetics 112, 665–673.
| Quantitative trait loci analysis for the developmental behavior of soybean (Glycine max L. Merr.).Crossref | GoogleScholarGoogle Scholar | 16365761PubMed |
Tamba CL, Zhang Y-M (2018) A fast mrMLM algorithm for multi-locus genome-wide association studies. biorxiv
| A fast mrMLM algorithm for multi-locus genome-wide association studies.Crossref | GoogleScholarGoogle Scholar |
Tamba CL, Ni Y-L, Zhang Y-M (2017) Iterative sure independence screening EM-Bayesian LASSO algorithm for multi-locus genome-wide association studies. PLoS Computational Biology 13, e1005357
| Iterative sure independence screening EM-Bayesian LASSO algorithm for multi-locus genome-wide association studies.Crossref | GoogleScholarGoogle Scholar | 28141824PubMed |
Tang KQ (2020) Molecular mechanism of GmLIM1 gene regulation of soybean plant height. PhD dissertation, University of Chinese Academy of Sciences, China.
Tian F, Bradbury PJ, Brown PJ, Hung H, Sun Q, Flint-Garcia S, Rocheford TR, McMullen MD, Holland JB, Buckler ES (2011) Genome-wide association study of leaf architecture in the maize nested association mapping population. Nature Genetics 43, 159–162.
| Genome-wide association study of leaf architecture in the maize nested association mapping population.Crossref | GoogleScholarGoogle Scholar | 21217756PubMed |
Wan X, Mo A, Liu S, Yang L, Li L (2011) Constitutive expression of a peanut ubiquitin-conjugating enzyme gene in Arabidopsis confers improved water-stress tolerance through regulation of stress-responsive gene expression. Journal of Bioscience and Bioengineering 111, 478–484.
| Constitutive expression of a peanut ubiquitin-conjugating enzyme gene in Arabidopsis confers improved water-stress tolerance through regulation of stress-responsive gene expression.Crossref | GoogleScholarGoogle Scholar | 21193345PubMed |
Wang J-K (2009) Inclusive composite interval mapping of quantitative trait genes. Acta Agronomica Sinica 35, 239–245.
| Inclusive composite interval mapping of quantitative trait genes.Crossref | GoogleScholarGoogle Scholar |
Wang CS (2020) Function study on soybean GmGAI gene regulating flowering time via gibberellin pathway. Masters dissertation, Northeast Agricultural University, China.
Wang D, Graef GL, Procopiuk AM, Diers BW (2004) Identification of putative QTL that underlie yield in interspecific soybean backcross populations. Theoretical and Applied Genetics 108, 458–467.
| Identification of putative QTL that underlie yield in interspecific soybean backcross populations.Crossref | GoogleScholarGoogle Scholar | 14504749PubMed |
Wang S-B, Feng J-Y, Ren W-L, Huang B, Zhou L, Wen Y-J, Zhang J, Dunwell JM, Xu S, Zhang Y-M (2016) Improving power and accuracy of genome-wide association studies via a multi-locus mixed linear model methodology. Scientific Reports 6, 19444
| Improving power and accuracy of genome-wide association studies via a multi-locus mixed linear model methodology.Crossref | GoogleScholarGoogle Scholar | 26787347PubMed |
Wen Y-J, Zhang Y-W, Zhang J, Feng J-Y, Dunwell JM, Zhang Y-M (2019) An efficient multi-locus mixed model framework for the detection of small and linked QTLs in F2. Briefings in Bioinformatics 20, 1913–1924.
| An efficient multi-locus mixed model framework for the detection of small and linked QTLs in F2.Crossref | GoogleScholarGoogle Scholar | 30032279PubMed |
Xia Z, Tsubokura Y, Hoshi M, Hanawa M, Yano C, Okamura K, Ahmed TA, Anai T, Watanabe S, Hayashi M, Kawai T, Hossain KG, Masaki H, Asai K, Yamanaka N, Kubo N, Kadowaki K-I, Nagamura Y, Yano M, Sasaki T, Harada K (2007) An integrated high-density linkage map of soybean with RFLP, SSR, STS, and AFLP markers using a single F2 population. DNA Research 14, 257–269.
| An integrated high-density linkage map of soybean with RFLP, SSR, STS, and AFLP markers using a single F2 population.Crossref | GoogleScholarGoogle Scholar | 18192280PubMed |
Xia T, Xiao D, Liu D, Chai W, Gong Q, Wang NN (2012) Heterologous expression of ATG8c from soybean confers tolerance to nitrogen deficiency and increases yield in Arabidopsis. PLoS ONE 7, e37217
| Heterologous expression of ATG8c from soybean confers tolerance to nitrogen deficiency and increases yield in Arabidopsis.Crossref | GoogleScholarGoogle Scholar | 22629371PubMed |
Yang G, Zhai H, Wu H-Y, Zhang X-Z, Lü S-X, Wang Y-Y, Li Y-Q, Hu B, Wang L, Wen Z-X, Wang D-C, Wang S-D, Kyuya H, Xia Z-J, Xie F-T (2017) QTL effects and epistatic interaction for flowering time and branch number in a soybean mapping population of Japanese × Chinese cultivars. Journal of Integrative Agriculture 16, 1900–1912.
| QTL effects and epistatic interaction for flowering time and branch number in a soybean mapping population of Japanese × Chinese cultivars.Crossref | GoogleScholarGoogle Scholar |
Yu J, Buckler ES (2006) Genetic association mapping and genome organization of maize. Current Opinion in Biotechnology 17, 155–160.
| Genetic association mapping and genome organization of maize.Crossref | GoogleScholarGoogle Scholar | 16504497PubMed |
Yu J, Pressoir G, Briggs WH, Vroh Bi I, Yamasaki M, Doebley JF, McMullen MD, Gaut BS, Nielsen DM, Holland JB, Kresovich S, Buckler ES (2006) A unified mixed-model method for association mapping that accounts for multiple levels of relatedness. Nature Genetics 38, 203–208.
| A unified mixed-model method for association mapping that accounts for multiple levels of relatedness.Crossref | GoogleScholarGoogle Scholar | 16380716PubMed |
Zhang J, Feng J-Y, Ni Y-L, Wen Y-J, Niu Y, Tamba CL, Yue C, Song Q, Zhang Y-M (2017) pLARmEB: integration of least angle regression with empirical Bayes for multilocus genome-wide association studies. Heredity 118, 517–524.
| pLARmEB: integration of least angle regression with empirical Bayes for multilocus genome-wide association studies.Crossref | GoogleScholarGoogle Scholar | 28295030PubMed |
Zhang YW, Tamba CL, Wen YJ, Li P, Ren WL, Ni YL, Gao J, Zhang YM (2020) mrMLM v4.0. An R platform for multi-locus genome-wide association studies. Genomics, Proteomics & Bioinformatics 18, 481–487.
| mrMLM v4.0. An R platform for multi-locus genome-wide association studies.Crossref | GoogleScholarGoogle Scholar |