Wildfire in rainforest margins is associated with variation in mammal diversity and habitat use
Rhiannon R. Bird

A
B
C
Abstract
Rainforests typically evolved without the influence of fire or with only low-intensity fires, making them vulnerable to contemporary increases in fire frequency and intensity. Rainforest-associated species are predicted to be less adaptable than generalist species, but little is known about how fire in rainforest margins affects their habitat use and behaviour.
We investigated how mammal community composition, diversity, probability of habitat use and probability of movement were affected by wildfires that occurred in rainforest margins in South East Queensland, Australia, in 2019–20.
We deployed camera traps at 23 sites spanning the following three fire-habitat categories: unburnt rainforest (UR), burnt rainforest (BR) and surrounding burnt sclerophyll forest (BS), and used passive detection to analyse habitat use and behaviour.
Fire-habitat category had little influence on community composition. Species diversity was highest in unburnt rainforest compared with burnt rainforest and surrounding burnt sclerophyll forest. The probability of habitat use was highest in unburnt rainforest for both the long-nosed bandicoot (Perameles nasuta, estimated mean probability (95% CI): UR = 57.1% (22.98, 85.6), BR = 0%, BS = 0%) and the fawn-footed melomys (Melomys cervinipes: UR = 100%, BR = 87.5% (46.3, 98.3), BS = 50% (20.0, 80.0)). Probability of movement for the bush rat (Rattus fuscipes) increased with increasing elevation in burnt sclerophyll and was unaffected by elevation in unburnt and burnt rainforest.
Rainforest margins that experienced wildfire had reduced species diversity, most likely owing to a reduction in habitat use by rainforest-associated species. Movement patterns of mammal species were complex and not consistently related to recent fire history.
Rainforest margins and associated species are likely to be negatively affected by increasing wildfire intensity and frequency. In addition to urgent climate-change mitigation, land management that prevents wildfire incursion is likely to benefit rainforest communities in future.
Keywords: behavioural ecology, bushfire recovery, community ecology, ecological modelling, fire ecology, habitat use, mammal diversity, mammal ecology, wildfire.
Introduction
Many ecosystems around the world evolved in landscapes regularly shaped by fire (Keeley and Pausas 2022). In fire-prone regions, periodic fire events foster ecosystem resilience by reducing fuel loads and promoting landscape complexity, biodiversity and ecosystem services (Spies et al. 2014). However, climate change and other interacting stressors are altering fire regimes globally (Flannigan et al. 2013; Kelly et al. 2020). The occurrence of high-severity wildfires at larger spatial scales is set to continue in the future (Flannigan et al. 2013; Stephens et al. 2014; Bowman et al. 2020). Understanding how wildfire events affect ecosystems, and how they subsequently recover, is essential to develop management strategies to mitigate negative consequences of wildfire.
Gullies and pole-facing slopes are exposed to less solar radiation and, thus, have higher moisture content, which naturally supports rainforest vegetation (Wood et al. 2011; Robinson et al. 2013). Rainforests receive a high annual rainfall and have high humidity (Leigh 1975; Yates et al. 1988), which buffers rainforest vegetation from fire (Wood et al. 2011; Robinson et al. 2013). This means that rainforests have historically avoided being affected by repeated fire events (Russell-Smith and Stanton 2002; Cochrane 2003), and that resident plant communities have low proportions of species with fire-adaptive traits (e.g. especially post-fire seeding; Clarke et al. 2015; Lawes et al. 2022), compared with fire-prone ecosystems. When fires do occur in rainforests, they are typically infrequent, of low intensity, and most often occur in drier rainforest or only in rainforest margins (Sanford et al. 1985; Cochrane and Laurance 2002; Steffensen 2020). With drought severity and length increasing in many regions as a result of climate change (Vicente-Serrano et al. 2020; Laidlaw et al. 2022), rainforest margins are becoming more frequently affected by wildfires (Vicente-Serrano et al. 2020; Laidlaw et al. 2022). Rainforest fire can cause tree mortality, which creates gaps and subsequent changes in microclimate (Brando et al. 2014; De Frenne et al. 2021). Fire in rainforests can cause a positive feedback, whereby more flammable species invade rainforest margins, which may drive further increases in fire frequency (Cochrane and Laurance 2008; Fletcher et al. 2020; Hines et al. 2020). While there is evidence of resprouting in rainforest tree species after fire (Baker et al. 2022), little is known about the trajectory of ecosystem recovery in rainforest margins. Will they recover to their pre-disturbance state? Or will fire cause a shift into an alternative ecosystem state?
Animal population recovery after fire is shaped by a complex suite of factors including species’ biology, niche width, geographic context, resource availability, vegetation recovery, landscape fire regime, weather and local climate (Kelly et al. 2012; Nimmo et al. 2014; van Mantgem et al. 2015; Banks et al. 2017; Smith 2018). Generalist species might recolonise burnt areas more quickly than specialists do, especially in habitats with a long recovery time (Zwolak and Foresman 2007; Smith et al. 2013; Ondei et al. 2021). By contrast, specialist animal species associated with one or a few habitat plant species or functional types are likely to be more sensitive to changes in fire regimes (Charles et al. 2024). Increased dominance of generalist species can reduce species diversity in recently burnt areas (Ondei et al. 2021). Understanding the mechanisms behind fire-related changes in animal communities is critical to design appropriate management strategies. For example, post-fire recolonisation might be impossible if dispersal is limited, which has led to translocation of species that have become locally extinct following fire (Mitchell et al. 2022). However, translocation will fail if animals are moved to areas without habitat or food resources. Research that quantifies dispersal, behaviour and movement post-fire could therefore contribute to understanding whether active management is warranted (Smith et al. 2016; Nimmo et al. 2019).
Fire can affect animal movement and behaviour in the short term (during and immediately after fires) and in the long term through indirect influences on movement during post-fire habitat succession (Hulton VanTassel and Anderson 2018; Nimmo et al. 2019). Immediately after fire, resources are depleted, which can increase competition and predation risk and thus alter behaviour (Sutherland and Dickman 1999; Nimmo et al. 2019; Doherty et al. 2022). Animals in safe environments tend to display behaviours such as foraging, eating, grooming and reproductive behaviours (Middleton et al. 2013; Hegab et al. 2015). In unsafe environments, animals are more likely to have faster and more directional movement to avoid and escape predation (Middleton et al. 2013; Hutchen and Hodges 2019). Thus, if the post-fire environment represents an unsafe environment, this should be reflected in high-intensity directional movement. Studies of animal movement could therefore indicate the extent of post-fire recovery in animal populations and assist post-fire conservation management.
In this study, we quantified the recovery of a mammal community in rainforest margins ~15 months after an Australian wildfire. Specifically, we aimed to investigate the effect of fire and habitat type on mammal community composition and diversity. We also investigated the probability of habitat use and movement for individual species. On the basis of theoretical and empirical research on fire and habitat specialisation (Smith et al. 2013; Charles et al. 2024), we hypothesised that wildfire would more negatively affect rainforest-associated species than habitat generalists. This knowledge will enhance our understanding of how animals recover from wildfires in rainforests, improving the capacity for conservation managers to appropriately manage ecosystems post-fire in rainforest margins.
Materials and methods
Study location and fire characteristics
This study was conducted in Main Range National Park (27°48′57″S, 152°15′56″E) within the Gondwana World Heritage Area (WHA), South East Queensland, Australia. The Gondwana WHA covers 366,507 ha across New South Wales (308,942 ha) and Queensland (59,121 ha) and contains vast tracts of subtropical rainforest across 40 protected areas (Department of Climate Change, Energy, the Environment and Water 2020). The climate is subtropical, and the annual average rainfall of the area is 1043 mm (Fick and Hijmans 2017). The annual mean temperature is 15.9°C, with mean maximum and minimum temperatures of 27.2 and 3.2°C (Fick and Hijmans 2017). In Queensland, ~51% of the Gondwana WHA is rainforest vegetation (Broad Vegetation Groups, BVG, 1–7) whereas 47% is sclerophyllous eucalypt vegetation (BVG 8–17) (Neldner et al. 2019).
Our study sites were at Cunningham’s Gap (Fig. 1), ~120 km south-west of Queensland’s capital city Brisbane, ranging in elevation from 543 to 917 m above sea level. This area experienced unprecedented wildfires between September 2019 and January 2020 (Nolan et al. 2020), following severe drought and record-high fire weather (Hines et al. 2020). Approximately 46% of the Queensland section of the Gondwana WHA was affected by fire during this event. In Main Range National Park, these wildfires affected 60% of sclerophyll and 17% of rainforest vegetation (Hines et al. 2021), with much of the fire-affected area at rainforest margins (Fig. 1).
(a) Location of 23 study sites within the Gondwana World Heritage Area, South East Queensland, Australia. The area, encompassing rainforest within surrounding eucalypt (sclerophyll) vegetation, was heavily affected by fire in 2019, with fire burning into the rainforest margins. Our study sites represented the following three fire-habitat categories: (b) unburnt rainforest, (c) burnt rainforest and (d) burnt sclerophyll. Rainforest vegetation was classified as Broad Vegetation Groups 1–7 (Neldner et al. 2019).
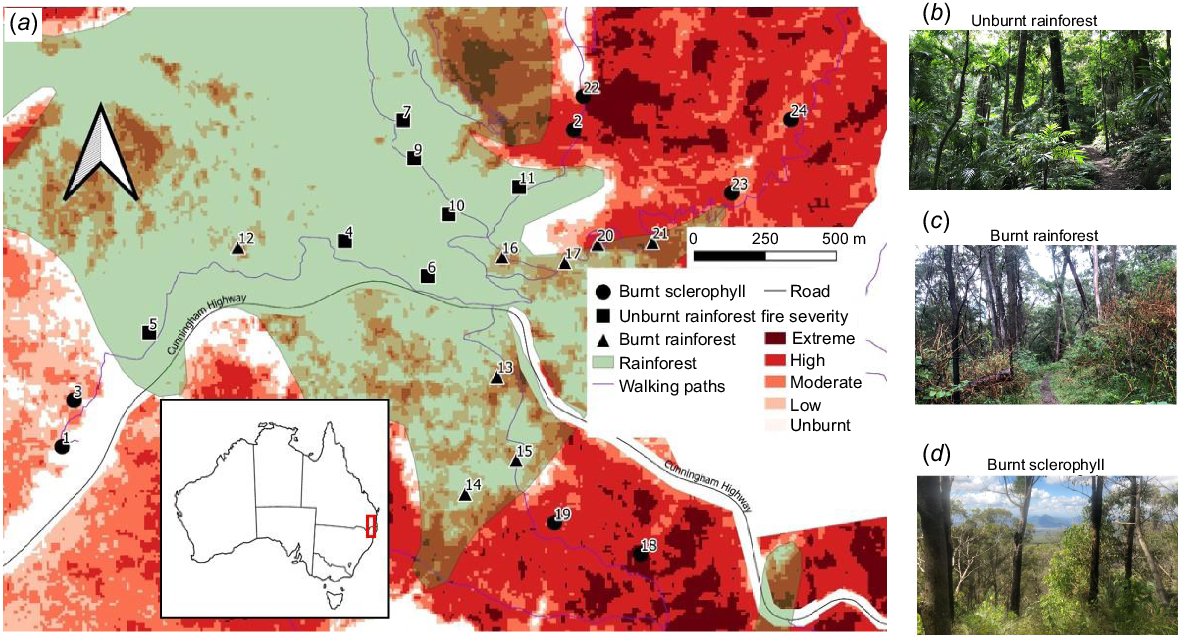
Study design and data collection
Fire-severity data were obtained for our study sites from the Queensland Government Open Data Portal accessed in February of 2021 (Queensland Government 2021). Sites were chosen on the basis of satellite-mapped fire severity (Hines et al. 2021), which was verified visually during placing of camera traps by using evidence of burned trees in burned areas. Most burnt rainforest sites were burned at low to moderate severity, whereas most of the burned sclerophyll sites were burned at high to extreme severity (Fig. 1). The sclerophyll eucalypt forests in the region are managed by government agencies, often with low to moderate planned burns, which attempt to mitigate impact of severe unplanned fires (Queensland Herbarium 2024).
Given our interest in rainforest margins, we selected study sites spanning the rainforest margins and compared them with sites in the rainforest interior and in the surrounding eucalypt (open sclerophyll) vegetation. Margins, by their nature, only span a few hundred metres, a spatial scale exceeding the daily movement distances of many small to medium-sized mammals (Miles et al. 1981). Mammals using these margins are therefore also likely to use the surrounding habitat in addition to margins. Our study thus quantifies mammal activity and habitat use in burnt rainforest margins, rather than residency. This helped us understand the extent to which burnt margins represent either ‘intact’ rainforest for mammals to use or disturbed surrounding vegetation. Fire penetration varied from 0 to 300 m into the rainforest and burn severity was not uniform across this area. We did not sample unburnt eucalypt vegetation because almost all the eucalypt vegetation in the study area was burnt at moderate to high severity (Fig. 1).
We classified sites into the following three ‘fire-habitat’ categories, a factorial variable incorporating both habitat and burn status: unburnt rainforest (Fig. 1b), burnt rainforest (Fig. 1c) and burnt sclerophyll (Fig. 1d). Although burn status and habitat type were confounded by the nature of fire in this system (typically burning large areas of sclerophyll forest and only rainforest margins), our analysis accounted for variation in distance to the rainforest boundary at each site (described below). We thus focused on the combined influence of burn status and habitat in our investigation. After selecting sites broadly on the basis of fire-habitat category, most sites were selected in the proximity to walking tracks because regenerating rainforest vegetation is functionally impenetrable owing to regrowth of native and non-native early successional plant species. Sites were at least 100 m from each other, with an average distance between sites being 265 m (range = 124–439 m). A total of 23 sites was included in the final study design, including 8 in burnt sclerophyll, 8 in burnt rainforest and 7 in unburnt rainforest (Fig. 1). Although this study was limited in the number of sites, we interpreted our results in the context of this design and also conducted rarefaction and extrapolation to assess the effects of incomplete sampling.
At each site, we installed two camera traps between 0.5 and 3 m of one another, one vertically facing down, ~55 cm from the ground, and one horizontally, pointing parallel to the ground, ~100 cm from the ground. The only exception to this was Site 15, which had only a horizontal camera because of a field logistical error (which we accounted for in our analysis, described below). Camera-trap brands differ in their performance in detecting animals (Driessen et al. 2017). However, because of equipment constraints, we used a combination of Swift Enduro (n = 29) and Reconyx HC600 HyperFire (n = 16) (Churchill et al. 2023; Frost et al. 2023; Watchorn et al. 2024). To reduce the risk of influencing results from different camera brands, we assigned all horizontal cameras as Swift Enduros, whereas vertical cameras (which we predicted to have a lower capture rate) were a mix of Swift and Reconyx cameras (randomly assigned). The settings were identical on both camera brands. The balanced setting was used for night mode to minimise blur and maximise range. Camera sensitivity was set at moderate, and five photos were taken per trigger to maximise the chance of species identification and allow behaviour to be recorded. After the fifth photo was taken, there was a 30-s delay before cameras could be triggered again. All cameras were activated simultaneously for a total of 30 days (26 April−25 May 2021), ~15 months post-fire. Vegetation directly in front of the field of view that was likely to create false triggers was carefully moved aside, but we did not clear vegetation to the extent that it was likely to affect animal behaviour.
Data on vegetation structure were recorded at each site for the understorey (0–1 m), mid-storey (1–5 m) and canopy (>5 m). Each stratum was assessed on a four-point scale, as open (1), sparse (2), moderate (3) or dense (4). This protocol was based on appendix B in a standardised Vegetation Assessment Guide (open = 0–25%, sparse = 25–50%, moderate = 50–75%, dense = 75–100%) (Department of Environment 2013). Estimates were made visually by the same person (RRB) in the area above and surrounding the cameras. This coarse method of habitat assessment was designed to supplement the remotely sensed fire-severity data. Data on environmental co-variates were included in our analysis to assess the influence of other key environmental variables and survey bias, in addition to fire and habitat type. These data were obtained using standard functions in QGIS, namely, distance to highway (range = 72.58–1183.36 m; average = 382 m), distance to nearest walking path (range = 7.8–186.51 m; average = 39.67 m) and distance to rainforest boundary (range = 4.09–459.72 m; average = 178.24 m). Elevation (range = 543–906.89 m; average = 751.01 m) data were obtained from an online tool using study site positions and sea level as an altimetric reference, with a margin of error of ~10 m (dCode, see https://www.dcode.fr/earth-elevation, accessed 23 June 2021).
Prior to modelling, correlation analysis was conducted on all environmental co-variates and fire-habitat category (Supplementary Fig. S1), with Pearson correlation coefficient of ≥|0.7| being considered highly correlated (Dormann et al. 2007). Canopy cover was highly correlated with fire-habitat category (Pearson correlation coefficient = −0.75, Fig. S1) and was removed from the final set of environmental co-variates.
Image processing
Images were sorted manually to remove ‘false triggers’ (i.e. images with no animals present) and non-mammal species. Mammals (native and introduced) were identified to the lowest taxonomic level possible following Van Dyck et al. (2013). Animals were considered independent using a combination of time elapsed since last trigger (10 min) and the spatial position of individuals within each image.
Each image containing an animal was initially assigned to one of seven behavioural categories (using the sequence of images to assist categorising behaviour). There were too few data within these categories for analysis, so they were further classified into movement behaviour (walking or running, hopping or jumping, and climbing) and stationary behaviour (grooming, foraging or eating, alert and sitting still). Following initial image processing, data were sent to two ecologists with local mammal experience to check identifications and data were amended following their advice.
Response variable definition
We analysed mammal community composition (dissimilarity), diversity and, for individual species, the probability of habitat use and probability of movement. All variables were analysed at the site level (23 observations), except for movement, which was analysed at the level of independent capture events.
Community composition was quantified using a principal component analysis (PCA), which reduces correlated variables (represented by a site × species matrix of abundances) into a series of uncorrelated components (Abdi and Williams 2010). PCA was performed using the ‘prcomp’ function, in base R (ver. 4.2.0, R Foundation for Statistical Computing, Vienna, Austria, see https://www.r-project.org/).
Probability of habitat use was analysed using presence or absence data from independent capture events from camera traps. To ensure adequate data for probability of habitat-use analysis, any species that had absences at more than 85% of sites was excluded from analysis (Phascolarctos cinereus and Sus scrofa excluded).
We analysed probability of movement for species with more behavioural observations than double the number of study sites (>46 observations), because species with less data were found to lead to model-fitting problems. This excluded the following five of the nine recorded species with sparse data: Phascolarctos cinereus, Sus scrofa, Antechinus stuartii, Perameles nasuta and Trichosurus caninus. For each independent capture event, we classified behaviour (defined in the Image Processing section) as movement (1) or stationary (0), such that we could model the probability of movement for each species separately. For each independent event, we used the most common behaviour from all images in sequence, as being representative of their behaviour state. Of the 925 animal observations, 15 (1.6%) displayed an equal amount of both behaviours and were excluded from the analysis.
Analysis
We conducted preliminary analysis to determine whether including Site 15, which had only a horizontal and no vertical camera, would bias our results. Across all sites, horizontal cameras captured a greater number of individuals (mean = 32.7, range = 0–200) than did vertical cameras (mean = 7.4, range = 0–74). Fitting models with and without Site 15 showed that model rankings were not affected by including this site (data not shown); thus, we retained Site 15 in our final data set.
We modelled community composition by using the first two components of the PCA, which explained the majority of the variation in the mammal community composition (95.78%). We plotted these two principal components to visualise community composition and the species contributing most to community-level variation. The first two principal components were then modelled using linear regression in base R (R Foundation for Statistical Computing).
To determine whether estimates of species diversity would be affected by incomplete sampling and variability across fire-habitat categories, we used rarefaction and extrapolation of Hill numbers (effective number of species) (Chao et al. 2014). Hill numbers are directly comparable to each other because they are mathematically unified, with the parameter q representing variability in the effect of evenness on species diversity (Chao et al. 2014). The orders of q are as follows: species richness (q = 0), Shannon diversity (q = 1) and Simpson diversity (q = 2) (Chao et al. 2014). Rarefaction and extrapolation of Hill numbers allowed us to compare species diversity assemblages in the three fire-habitat categories and make statistical inferences despite incomplete sampling (Chao et al. 2014). We used the R package iNEXT (ver. 3.0.0, T. C. Hsieh, K. H. Ma and A. Chao, see https://cran.r-project.org/package=iNEXT) to produce rarefaction and extrapolation curves for all fire-habitat categories by using both sample size-based and coverage-based estimates for the three orders of q following Chao et al. (2014). We inferred differences among fire-habitat categories when confidence intervals (from 200 bootstrapped replications) did not overlap.
Probability of habitat use of individual species was analysed at the site level by using binomial generalised linear models with a logit-link function in base R (R Foundation for Statistical Computing). Probability of movement was analysed at the level of an individual capture event by using generalised linear mixed models in the R package lme4 (ver. 1.1-36, see https://github.com/lme4/lme4/; Bates et al. 2015). For the movement models, we included a random effect for site to account for spatial clustering of movement observations within sites.
For community composition and probability of movement, we used a two-stage model-selection procedure (Burnham and Anderson 2002). Because of data limitations (23 observations), we did not fit more than one co-variate with the fire-habitat category design variable in any analysis. First, we fitted fire-habitat category with one environmental co-variate in an additive and interactive formulation and selected the best-fitting formulation to use in the second stage. We then fitted each co-variate with fire-habitat category, using its best-fitting formulation (additive or interactive) to determine which set of variables best explained the data. Each set of models was fitted with a fire-habitat category only model (no co-variates) and a null model (no predictor variation), as a baseline against which to compare the more parameterised models. Models were ranked by second-order Akaike information criterion (AICc) using AICcmodavg (ver. 2.3-1, M. J. Mazerolle, see https://cran.r-project.org/package=AICcmodavg). The model with the lowest AICc was assumed to have the greatest fit and models within ΔAICc < 2 were assumed to be equivalent. For probability of habitat use, the above model structures were too complex to obtain meaningful results. We thus analysed the probability of habitat use for each species with only two univariate models, namely, the fire-habitat category model and the null model. The ΔAICc between these two models was used to determine the strength of the fire-category effect, by using the same process as for the other response variables.
To estimate the effect of each variable in the top model for community composition, we used the ‘predict’ function in R (R Foundation for Statistical Computing) and calculated confidence intervals as estimate ±1.96 × standard error. For probability of habitat use and probability of movement (i.e. models with a logit-link function), parameter estimates and confidence intervals were derived on the link scale then back-transformed using the ‘invlogit’ function in the arm package (ver. 1.13-1, A. Gelman and Y. Su, see https://CRAN.R-project.org/package=arm) to ensure they were bound between 0 and 1.
Results
A total of 38,390 images was collected during this study, 15,361 (40%) of which contained at least one animal (thus 23,029 images (60%) were false triggers). The following nine mammal species were identified to species level (Supplementary Table S1): Melomys cervinipes (fawn-footed melomys), Rattus fuscipes (bush rat), Thylogale stigmatica (red-legged pademelon), Thylogale thetis (red-necked pademelon), T. richosurus caninus (short-eared brushtail possum), Perameles nasuta (long-nosed bandicoot), Antechinus stuartii (brown antechinus), Phascolarctos cinereus (koala) and Sus scrofa (pig).
The first two principal components explained the majority (95.78%) of variation in mammal species composition (PC1 = 58.17% and PC2 = 37.60% variance explained, Fig. S2a). Visually, principal component analysis showed some overlap between burnt rainforest and burnt sclerophyll sites, whereas unburnt rainforest sites tended to cluster separately (Fig. S2b). Clusters were driven mainly by T. stigmatica on PC1 and R. fuscipes and M. cervinipes on PC2 (Fig. S3). However, statistical models indicated no effect of fire-habitat category or any environmental co-variates on either of the first two principal components (the null model was ranked highest, Table S2). The second-ranked model for PC2 was within ΔAIC < 2 of the null model and included an interaction between fire-habitat category and mid-storey (Table S2, Fig. S4).
For sample size-based estimates of species diversity, rarefaction and extrapolation indicated that the effective number of species was highest in unburnt rainforest for species richness (q = 0) and Shannon’s diversity (q = 1) (Fig. 2a, b). However, for q = 0, this difference was evident only for small sample sizes (<200 individuals) and not large sample sizes (<200 individuals, Fig. 2a). For q = 2, the sample size-based estimates were lowest in the burnt sclerophyll forest and similarly high in unburnt and burnt rainforests (Fig. 2c). Coverage-based estimates of diversity showed a similar pattern, being highest in unburnt rainforest for q = 0 and q = 1, whereas burnt sclerophyll and burnt rainforest were more similar in species diversity (Fig. 2d, e). For q = 2, burnt sclerophyll had the lowest estimated species diversity (Fig. 2f), whereas the burnt and unburnt rainforest sites did not differ. Taken together, the results showed an increase in species diversity from burnt sclerophyll to burnt rainforest and unburnt rainforest. They also suggest that dominance and rarity (i.e. species evenness) influence the ability to detect fire and habitat effects on species diversity. Differences among fire-habitat categories were more evident for estimates incorporating evenness (q > 0), than for q = 0, which does not incorporate evenness.
(a–c) Sample size-based and (d–f) coverage-based estimates of species diversity (±95% confidence intervals) by using rarefaction (solid lines) and extrapolation (dashed lines) on the basis of Hill numbers (q = 0, 1, 2) for mammal communities in three fire-habitat categories. Solid symbols and line represent the reference sample (total number of observed individuals). UBR, unburnt rainforest; BR, burnt rainforest; BS, burnt sclerophyll.
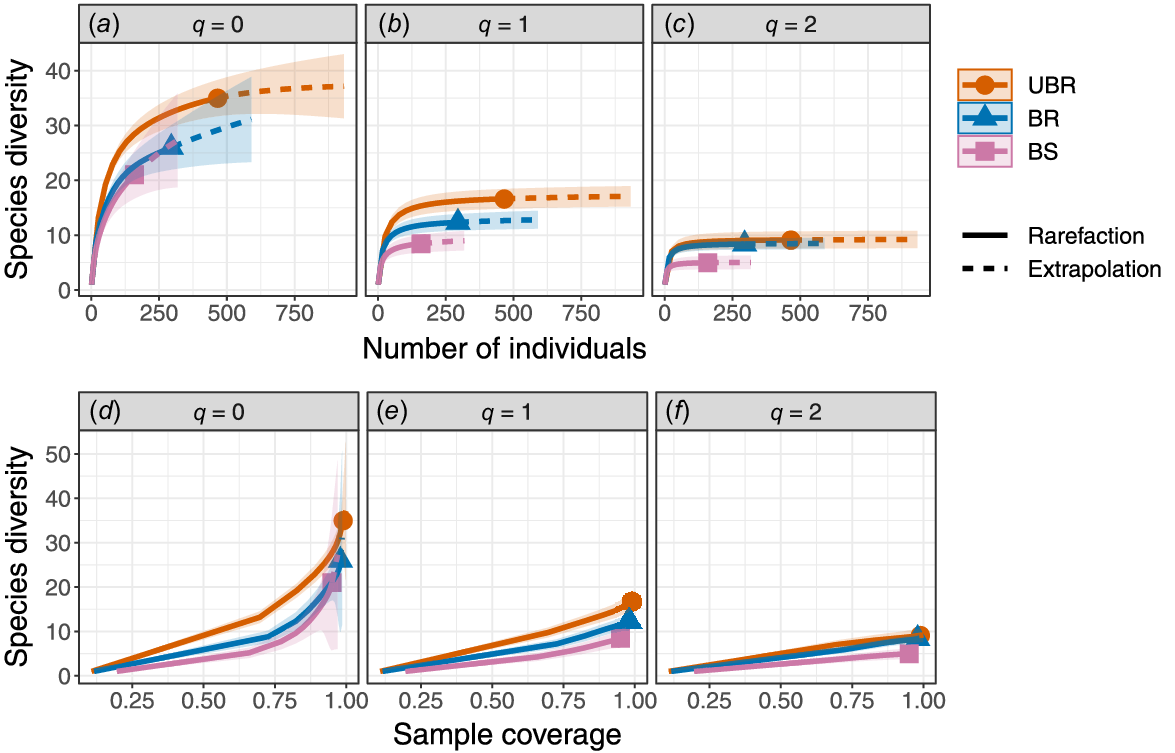
Probability of habitat use was associated with fire-habitat category weakly in M. cervinipes (ΔAICc = −1.89) and more strongly in P. nasuta (ΔAICc = −6.62) (Fig. 3, Table 1). Melomys cervinipes was found at 100% of unburnt rainforest sites, 87.5% of burnt rainforest sites and 50% of burnt sclerophyll sites (Fig. 3a). Perameles nasuta was found at 57.1% of unburnt rainforest sites and was completely absent from other fire-habitat categories (Fig. 3b). There was no effect of fire-habitat category on the probability of habitat use for other mammal species (Table 1, Fig. S5).
Estimated probability of habitat use (±95% confidence interval) for (a) Melmomys cervinipes and (b) Perameles nasuta in fire-affected rainforest margins of South East Queensland, Australia. Asterisks (*) indicate the inability to calculate confidence intervals as presence or absence was absolute for that species in these fire-habitat categories. UBR, unburnt rainforest; BR, burnt rainforest; BS, burnt sclerophyll.
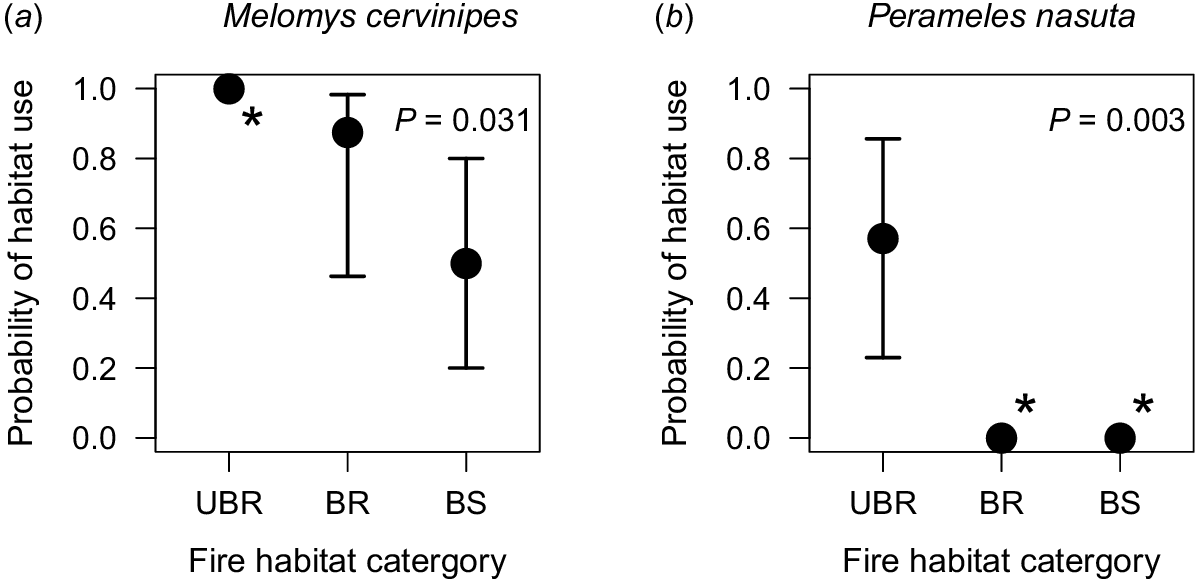
Species | Term | Estimate | Standard error | P-value | ΔAICc | |
---|---|---|---|---|---|---|
Antechinus stuartii | Intercept (unburnt rainforest) | 0.288 | 0.764 | 0.159 | 1.39 | |
Burnt rainforest | −1.386 | 1.118 | ||||
Burnt sclerophyll | −2.234 | 1.313 | ||||
Melomys cervinipes | Intercept (unburnt rainforest) | 19.566 | Intercept at boundary | 0.031 | −1.89 | |
Burnt rainforest | −17.62 | – | ||||
Burnt sclerophyll | −19.566 | – | ||||
Perameles nasuta | Intercept (unburnt rainforest) | 0.288 | 0.764 | 0.003 | −6.62 | |
Burnt rainforest | −20.854 | Boundary | ||||
Burnt sclerophyll | −20.854 | Boundary | ||||
Rattus fuscipes | Intercept (unburnt rainforest) | 19.566 | Intercept at boundary | 0.465 | 3.54 | |
Burnt rainforest | −17.62 | – | ||||
Burnt sclerophyll | −17.62 | – | ||||
Thylogale stigmatica | Intercept (unburnt rainforest) | 0.916 | 0.837 | 0.378 | 3.13 | |
Burnt rainforest | −0.405 | 1.111 | ||||
Burnt sclerophyll | −1.427 | 1.111 | ||||
Thylogale thetis | Intercept (unburnt rainforest) | −0.288 | 0.764 | 0.565 | 3.93 | |
Burnt rainforest | −0.811 | 1.118 | ||||
Burnt sclerophyll | 0.288 | 1.041 | ||||
Trichosurus caninus | Intercept (unburnt rainforest) | 0.288 | 0.764 | 0.159 | 1.39 | |
Burnt rainforest | −1.386 | 1.118 | ||||
Burnt sclerophyll | −2.234 | 1.314 |
P-values were calculated for the fire-habitat category term rather than individual levels within that term. For each species, the change in AICc (ΔAICc) between the null model and the fire-habitat category model is shown.
Probability of movement was affected by fire-habitat category and environmental co-variates for R. fuscipes (Table 2). The top-ranked model for probability of movement in R. fuscipes included an interaction between fire-habitat category and elevation (Fig. 4). Elevation had little effect on probability of movement for R. fuscipes in burnt and unburnt rainforest (Fig. 4a, b) and a positive effect on probability of movement in burnt sclerophyll forest (Fig. 4c). For M. cervinipes, the second-ranked model (fire-habitat category × elevation) was within ΔAICc < 2 relative to the first-ranked null model (Table S3, Fig. S6). There were no notable effects on probability of movement for any other species (Table S3).
Models | K | AICc | ΔAICc | AICc Wt | Cum. Wt | log L | |
---|---|---|---|---|---|---|---|
FHC × Elevation | 7 | 470.19 | 0.00 | 0.93 | 0.93 | −227.93 | |
FHC + Distance to highway | 5 | 476.48 | 6.29 | 0.04 | 0.97 | −233.15 | |
Null | 2 | 479.19 | 9.00 | 0.01 | 0.98 | −237.58 | |
FHC + Distance to path | 7 | 479.70 | 9.51 | 0.01 | 0.99 | −232.68 | |
FHC | 4 | 480.50 | 10.30 | 0.01 | 0.99 | −236.19 | |
FHC + Mid-storey | 7 | 481.26 | 12.07 | 0.00 | 1.00 | −233.96 | |
FHC + Distance to rainforest boundary | 5 | 482.31 | 12.11 | 0.00 | 1.00 | −236.06 | |
FHC + Understorey | 6 | 484.44 | 14.25 | 0.00 | 1.00 | −236.10 |
Movement was modelled as a function of fire-habitat category (FHC) and environmental co-variates. For all other species, the null model was ranked highest (Table S3). K, number of parameters; AICc, second order Akaike Information Criterion; ΔAICc, change in AICc; AICc Wt, AICc weight; Cum. Wt, cumulative AICc weight; log L, logarithmic likelihood of model given the data.
Estimated probability of movement (±95% confidence interval) of Rattus fuscipes in fire-affected rainforest margins of South East Queensland, Australia. The top-ranked model included an interactive effect of elevation and fire-habitat category: (a) unburnt rainforest (b) burnt rainforest (c) burnt sclerophyll.
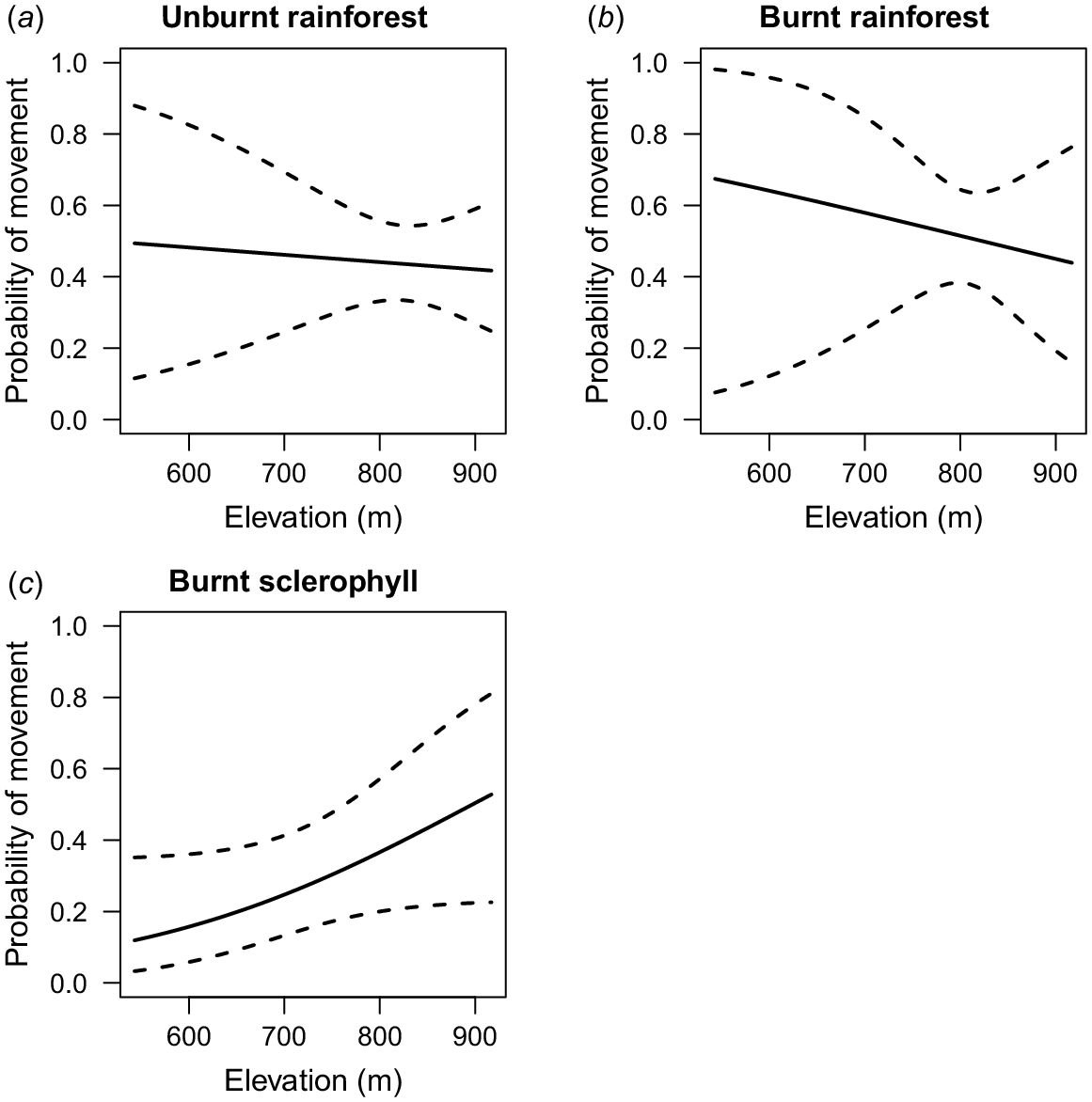
Discussion
We examined the impact of an Australian wildfire on a mammal community in rainforest margins and found that community composition was not strongly affected by a variable describing the fire status and habitat type (‘fire-habitat category’). Rarefaction and extrapolation showed a trend for unburnt rainforests to have higher species diversity than in burnt rainforest and burnt sclerophyll forest. The ability to detect differences in mammal species diversity among fire-habitat categories was therefore influenced by community evenness (rarity and dominance), with differences becoming more evident when evenness was incorporated in the diversity estimates. Fire-habitat category affected the probability of habitat use of two rainforest-associated mammal species; P. nasuta used only unburnt rainforest habitat, and M. cervinipes used both burnt and unburnt rainforest habitats, with lower habitat use in surrounding burnt sclerophyll forest. Movement of R. fuscipes increased with an increasing elevation in sclerophyll forest, but was unaffected by elevation in burnt and unburnt rainforest. This study has highlighted that fire in rainforest affects species diversity and the probability of habitat use in rainforest-associated species. Fire also created variation in movement for one generalist species, indicating that animals alter their behaviour when fire interacts with environmental features.
Community composition was not strongly affected by fire-habitat category in our study, but there was a visual trend for community dissimilarity between unburnt areas and burnt areas. By contrast, other studies have found strong effects of fire (including burnt area vs unburnt area, time since fire and fire mosaic) on community composition (Briani et al. 2004; Francl and Small 2013; Cazetta and Vieira 2021; Zylinski et al. 2022). One particular study, in a neotropical savanna, found that the lack of arboreal mammals in burnt areas led to significant difference in assemblages (Cazetta and Vieira 2021). Although not strong, the slight variation in community composition between the unburnt rainforest and burnt areas was likely to be driven by the low probability of habitat use of P. nasuta and M. cervinipes in burnt areas. Bandicoots, including P. nasuta, are ecosystem engineers (Valentine et al. 2017, 2018; Halstead et al. 2020) because they dig to obtain food (invertebrates, fungi and tubers), leading to higher soil moisture content, reduced fuel load, and changes in soil properties, including increased organic carbon, microbial activity and fungi (Valentine et al. 2017, 2018; Halstead et al. 2020; Ryan et al. 2020; Hopkins et al. 2021). Thus, changes in even one species following wildfires could potentially have a disproportionate influence on ecosystem function. Loss of these kinds of species could affect ecosystem recovery (Byers et al. 2006). More research on the time it takes for fire-sensitive ecosystem engineers to recover in burnt areas is necessary to understand their contribution to ecosystem recovery.
Rarefaction and extrapolation allowed us to examine species diversity while accounting for incomplete sampling of the mammal community and potentially low detectability. Species diversity tended to be highest in unburnt rainforest, lower in burnt rainforest and lowest overall in burnt sclerophyll forest, depending on the extent to which community evenness was incorporated (i.e. variation in q). Coupled with our results of habitat use, this is likely because there are more rainforest-associated mammals using unburnt rainforest. Mammal diversity in other studies has shown variable responses to fire events, including increases, decreases and no change (Kelly et al. 2012; Sharp Bowman et al. 2017; He et al. 2019; Santosa and Kwatrina 2020; Ondei et al. 2021). Our results are important because there is limited research on mammal diversity in burnt rainforest, given that rainforests have not typically experienced frequent or intense wildfire. The results suggest that unburnt rainforests are important for mammal biodiversity. However, larger sample sizes than we were able to use here are needed to more confidently generalise patterns in rainforest species diversity.
In our study, habitat use was affected by fire-habitat category for two rainforest-associated species (M. cervinipes and P. nasuta had the greatest habitat use in unburnt rainforest) but not for habitat generalists. Despite being a rainforest-associated species, M. cervinipes was able to persist in burnt rainforest, a response found in other melomys species (Liedloff et al. 2018). By contrast, P. nasuta was found only in unburnt rainforest, suggesting that this species is fire sensitive and requires unburnt habitat for nesting and foraging (Pardon et al. 2003; MacGregor et al. 2015; Fisher et al. 2021). Conversely, P. nasuta in other areas shows increased habitat use following fire, suggesting its response to fire is species- and context-specific (Hope 2012). The propensity for philopatry in this species (with movements generally of <500 m; Dexter et al. 2011) might slow its rate of recovery. Monitoring rainforest margins will help determine whether fire causes ongoing impacts on bandicoot habitat use.
Traditionally, fire ecology research focused on species distributions and abundance but changes in movement and behaviour in the context of fire regimes are increasingly being documented (Hutchen and Hodges 2019; Nimmo et al. 2019; Sitters and Di Stefano 2020; Blakey et al. 2022; Doherty et al. 2022; Santos et al. 2022). A study of arboreal mammals, for example, found that rates of the movement from an unburnt rainforest gully into surrounding areas decreased sharply following fire (Banks et al. 2015). Fire changes habitat structure and animals appear to modify their behaviour to avoid predators and optimise foraging opportunities (Hutchen and Hodges 2019; Doherty et al. 2022). Animals often perform more directional and rapid movements in unsafe environments, and less directional behaviour in safe environments where fear levels are reduced (Middleton et al. 2013; Hegab et al. 2015; Hutchen and Hodges 2019); however, we found little evidence for that here.
In our study, movement of the generalist R. fuscipes was influenced by an interaction between fire and elevation. In burnt sclerophyll forest, movement probability in R. fuscipes was greatest at higher elevations, but was unaffected by elevation in burnt and unburnt rainforest. This may indicate a shift in normal behaviour patterns owing to fire-related vegetation change, or a differing relationship with elevation depending on latitude (Claridge et al. 2008). In contrast to our study, post-fire movement in R. fuscipes was found to be more tortuous owing to turning at edges of unburnt patches so as to remain in their preferred microhabitat (Fordyce et al. 2016). Fire therefore affects mammal movement behaviour in complex ways depending on the environmental context. Future research is needed to draw generalisations about complex post-fire movement patterns.
Recent increases in wildfires globally have intensified public and scientific interest in fire management for protecting human life and property and sustaining biodiversity (Steffensen 2020; Ward et al. 2020; Wintle et al. 2020; Eliott et al. 2021). These events have sparked a new appreciation that fire management must become more active, with interventions such as post-fire weed and pest management, revegetation or assisted recolonisation now recognised as being critical for biodiversity conservation (Legge et al. 2022; Ward et al. 2022). Our study was small in spatial scale; however, because of limited research on mammal communities in burnt rainforest, we consider these findings a valuable baseline for future research in this area.
Declaration of funding
This project was supported by funds from the School of Agriculture and Food Sciences, University of Queensland.
Author contributions
Rhiannon R. Bird, Annabel L. Smith and Rebeka R. Zsoldos designed the study. Rhiannon R. Bird, Shania J. Watson and Martha V. Jimenez Sandoval collected the data. Rhiannon R. Bird and Annabel L. Smith analysed the data. Rhiannon R. Bird wrote the paper with extensive input from Annabel L. Smith and contributions from all authors.
Acknowledgements
We respectfully acknowledge the Traditional Owners of the lands on which this study took place, the Ugarapul and Yuggera people, and pay our respect to their elders past, present and emerging. Thanks go to Andrew Meiklejohn from the Department of Environment and Science for providing fire-severity data and to Garry Cook for field assistance and useful discussions. Harry Hines provided valuable advice on the study system during project set up. Jennie Bacon and Harry Hines provided assistance with mammal identification.
References
Abdi H, Williams LJ (2010) Principal component analysis. WIREs Computational Statistics 2(4), 433-459.
| Crossref | Google Scholar |
Baker AG, Catterall C, Wiseman M (2022) Rainforest persistence and recruitment after Australia’s 2019–2020 fires in subtropical, temperate, dry and littoral rainforests. Australian Journal of Botany 70(3), 189-203.
| Crossref | Google Scholar |
Banks SC, Lorin T, Shaw RE, McBurney L, Blair D, Blyton MDJ, Smith AL, Pierson JC, Lindenmayer DB (2015) Fine-scale refuges can buffer demographic and genetic processes against short-term climatic variation and disturbance: a 22-year case study of an arboreal marsupial. Molecular Ecology 24(15), 3831-3845.
| Crossref | Google Scholar | PubMed |
Banks SC, McBurney L, Blair D, Davies ID, Lindenmayer DB (2017) Where do animals come from during post-fire population recovery? Implications for ecological and genetic patterns in post-fire landscapes. Ecography 40(11), 1325-1338.
| Crossref | Google Scholar |
Bates D, Mächler M, Bolker B, Walker S (2015) Fitting linear mixed-effects models using lme4. Journal of Statistical Software 67(1), 1-48.
| Crossref | Google Scholar |
Bird RR, Smith AL (2025) Data and code: ‘Wildfire in rainforest margins is associated with variation in mammal diversity and habitat use’. Zenodo 2025, v1.0 [Data files published 30 January 2025].
| Crossref | Google Scholar |
Blakey RV, Sikich JA, Blumstein DT, Riley SPD (2022) Mountain lions avoid burned areas and increase risky behavior after wildfire in a fragmented urban landscape. Current Biology 32(21), 4762-4768.e5.
| Crossref | Google Scholar | PubMed |
Bowman DMJS, Kolden CA, Abatzoglou JT, Johnston FH, van der Werf GR, Flannigan M (2020) Vegetation fires in the Anthropocene. Nature Reviews Earth & Environment 1, 500-515.
| Crossref | Google Scholar |
Brando PM, Balch JK, Nepstad DC, Morton DC, Putz FE, Coe MT, Silvério D, Macedo MN, Davidson EA, Nóbrega CC, Alencar A, Soares-Filho BS (2014) Abrupt increases in Amazonian tree mortality due to drought–fire interactions. Proceedings of the National Academy of Sciences 111(17), 6347-6352.
| Crossref | Google Scholar |
Briani DC, Palma ART, Vieira EM, Henriques RPB (2004) Post-fire succession of small mammals in the cerrado of central Brazil. Biodiversity and Conservation 13, 1023-1037.
| Crossref | Google Scholar |
Byers JE, Cuddington K, Jones CG, Talley TS, Hastings A, Lambrinos JG, Crooks JA, Wilson WG (2006) Using ecosystem engineers to restore ecological systems. Trends in Ecology & Evolution 21(9), 493-500.
| Crossref | Google Scholar | PubMed |
Cazetta TC, Vieira EM (2021) Fire occurrence mediates small-mammal seed removal of native tree species in a neotropical savanna. Frontiers in Ecology and Evolution 9, 793947.
| Crossref | Google Scholar |
Chao A, Gotelli NJ, Hsieh TC, Sander EL, Ma KH, Colwell RK, Ellison AM (2014) Rarefaction and extrapolation with Hill numbers: a framework for sampling and estimation in species diversity studies. Ecological Monographs 84(1), 45-67.
| Crossref | Google Scholar |
Charles FE, Reside AE, Smith AL (2024) The influence of changing fire regimes on specialised plant-animal interactions. Philosophical Transactions of the Royal Society of London B
| Crossref | Google Scholar |
Churchill TB, Laidlaw MJ, McCall AH, Hines HB, Gynther IC, Molyneux J, Teixeira D, Stewart D, Rix MG, Burwell CJ, Lambkin CL, Venz MF, Zadkovich J, Cooper S, Buch W, O’Connell J, Hughes D, Finlayson S, Gurra A, Job C (2023) Bushfire recovery program 2020–2022: priority actions for threatened species in the Gondwana Rainforests of Australia World Heritage Area, South East Queensland. Department of Environment and Science, Queensland Government, Brisbane, Qld, Australia.
Claridge AW, Tennant P, Chick R, Barry SC (2008) Factors influencing the occurrence of small ground-dwelling mammals in southeastern mainland Australia. Journal of Mammalogy 89(4), 916-923.
| Crossref | Google Scholar |
Clarke PJ, Lawes MJ, Murphy BP, Russell-Smith J, Nano CEM, Bradstock R, Enright NJ, Fontaine JB, Gosper CR, Radford I, Midgley JJ, Gunton RM (2015) A synthesis of postfire recovery traits of woody plants in Australian ecosystems. Science of The Total Environment 534, 31-42.
| Crossref | Google Scholar | PubMed |
Cochrane MA (2003) Fire science for rainforests. Nature 421(6926), 913-919.
| Crossref | Google Scholar | PubMed |
Cochrane MA, Laurance WF (2002) Fire as a large-scale edge effect in Amazonian forests. Journal of Tropical Ecology 18(3), 311-325.
| Crossref | Google Scholar |
Cochrane MA, Laurance WF (2008) Synergisms among fire, land use, and climate change in the Amazon. Ambio: a Journal of the Human Environment 37(7-8), 522-527.
| Crossref | Google Scholar |
De Frenne P, Lenoir J, Luoto M, Scheffers BR, Zellweger F, Aalto J, Ashcroft MB, Christiansen DM, Decocq G, De Pauw K, Govaert S, Greiser C, Gril E, Hampe A, Jucker T, Klinges DH, Koelemeijer IA, Lembrechts JJ, Marrec R, Meeussen C, Ogée J, Tyystjärvi V, Vangansbeke P, Hylander K (2021) Forest microclimates and climate change: importance, drivers and future research agenda. Global Change Biology 27(11), 2279-2297.
| Crossref | Google Scholar | PubMed |
Department of Climate Change, Energy, the Environment and Water (2020) Gondwana rainforests of Australia State of conservation update – April 2020. (Australian Government: Canberra, ACT, Australia) Available at https://www.dcceew.gov.au/sites/default/files/documents/gondwana-rainforests-state-conservation-update-april-2020.pdf
Department of Environment (2013) Vegetation assessment guide. (Australian Government: Canberra, ACT, Australia) Available at https://www.dcceew.gov.au/sites/default/files/documents/vegetation-assessment-guide.pdf
Dexter N, Hudson M, Cater T, MacGregor C (2011) Habitat-dependent population regulation in an irrupting population of long-nosed bandicoots (Perameles nasuta). Austral Ecology 36(7), 745-754.
| Crossref | Google Scholar |
Doherty TS, Geary WL, Jolly CJ, Macdonald KJ, Miritis V, Watchorn DJ, Cherry MJ, Conner LM, Gonzalez TM, Legge SM, Ritchie EG, Stawski C, Dickman CR (2022) Fire as a driver and mediator of predator–prey interactions. Biological Reviews 97(4), 1539-1558.
| Crossref | Google Scholar | PubMed |
Dormann CF, McPherson JM, Araújo MB, Bivand R, Bolliger J, Carl G, Davies RG, Hirzel A, Jetz W, Daniel Kissling W, Kühn I, Ohlemüller R, Peres-Neto PR, Reineking B, Schröder B, Schurr FM, Wilson R (2007) Methods to account for spatial autocorrelation in the analysis of species distributional data: a review. Ecography 30(5), 609-628.
| Crossref | Google Scholar |
Driessen MM, Jarman PJ, Troy S, Callander S (2017) Animal detections vary among commonly used camera trap models. Wildlife Research 44(4), 291-297.
| Crossref | Google Scholar |
Eliott MG, Venn TJ, Lewis T, Farrar M, Srivastava SK (2021) A prescribed fire cost model for public lands in south-east Queensland. Forest Policy and Economics 132, 102579.
| Crossref | Google Scholar |
Fick SE, Hijmans RJ (2017) WorldClim 2: new 1-km spatial resolution climate surfaces for global land areas. International Journal of Climatology 37(12), 4302-4315.
| Crossref | Google Scholar |
Fisher D, Jose Lopez M, Davidson E, Butt N (2021) Survival and recovery of threatened animal species in fire-affected Gondwana Rainforest World Heritage Areas. National Environmental Science Program Project 8.3.8, final report. (NESP Threatened Species Recovery Hub: Brisbane, Qld, Australia) Available at https://www.nespthreatenedspecies.edu.au/media/jujnoljq/8-3-8-survival-and-recovery-of-threatened-animal-species-in-fire-affected-gondwana-rainforest-world-heritage-areas-final-report_v3.pdf
Flannigan M, Cantin AS, de Groot WJ, Wotton M, Newbery A, Gowman LM (2013) Global wildland fire season severity in the 21st century. Forest Ecology and Management 294, 54-61.
| Crossref | Google Scholar |
Fletcher M-S, Cadd HR, Mariani M, Hall TL, Wood SW (2020) The role of species composition in the emergence of alternate vegetation states in a temperate rainforest system. Landscape Ecology 35, 2275-2285.
| Crossref | Google Scholar |
Fordyce A, Hradsky BA, Ritchie EG, Di Stefano J (2016) Fire affects microhabitat selection, movement patterns, and body condition of an Australian rodent (Rattus fuscipes). Journal of Mammalogy 97(1), 102-111.
| Crossref | Google Scholar |
Francl KE, Small CJ (2013) Temporal changes and prescribed-fire effects on vegetation and small-mammal communities in central Appalachian forest, creek, and field habitats. Southeastern Naturalist 12(1), 11-26.
| Crossref | Google Scholar |
Frost PGH, Bell MD, Taylor GA (2023) Trail-camera assessment of the fates of northern royal albatross and northern Buller’s mollymawk chicks: 2021 breeding season, Motuhara. (Department of Conservation, Conservation Services Programme: Blenheim, New Zealand) Available at https://www.doc.govt.nz/globalassets/documents/conservation/marine-and-coastal/marine-conservation-services/reports/202223-annual-plan/evaluation-of-motuhara-trail-camera-images-final-report.pdf
Halstead LM, Sutherland DR, Valentine LE, Rendall AR, Coetsee AL, Ritchie EG (2020) Digging up the dirt: quantifying the effects on soil of a translocated ecosystem engineer. Austral Ecology 45(1), 97-108.
| Crossref | Google Scholar |
He T, Lamont BB, Pausas JG (2019) Fire as a key driver of Earth’s biodiversity. Biological Reviews 94(6), 1983-2010.
| Crossref | Google Scholar | PubMed |
Hegab IM, Kong S, Yang S, Mohamaden WI, Wei W (2015) The ethological relevance of predator odors to induce changes in prey species. Acta Ethologica 18(1), 1-9.
| Crossref | Google Scholar |
Hines H, Levy S, Laidlaw M, Midtaune K, Finlayson S, Meiklejohn A (2021) Post-fire assessment report – natural values: 2019 bushfire complex, Main Range National Park and adjacent QPWS estate, South West Queensland Region. Department of Environment and Science, Queensland Government, Brisbane, Qld, Australia.
Hope B (2012) Short-term response of the long-nosed bandicoot, Perameles nasuta, and the southern brown bandicoot, Isoodon obesulus obesulus, to low-intensity prescribed fire in heathland vegetation. Wildlife Research 39(8), 731-744.
| Crossref | Google Scholar |
Hopkins AJM, Tay NE, Bryant GL, Ruthrof KX, Valentine LE, Kobryn H, Burgess TI, Richardson BB, Hardy GESJ, Fleming PA (2021) Urban remnant size alters fungal functional groups dispersed by a digging mammal. Biodiversity and Conservation 30, 3983-4003.
| Crossref | Google Scholar |
Hulton VanTassel HL, Anderson KE (2018) Altered space use and movement distances of Merriam’s kangaroo rat, Dipodomys merriami, in post-fire lands. Journal of Mammalogy 99(3), 684-692.
| Crossref | Google Scholar |
Hutchen J, Hodges KE (2019) Foraging behaviour of snowshoe hares (Lepus americanus) in conifer forests regenerating after fire. Canadian Journal of Zoology 97(5), 446-452.
| Crossref | Google Scholar |
Keeley JE, Pausas JG (2022) Evolutionary ecology of fire. Annual Review of Ecology, Evolution, and Systematics 53, 203-225.
| Crossref | Google Scholar |
Kelly LT, Nimmo DG, Spence-Bailey LM, Taylor RS, Watson SJ, Clarke MF, Bennett AF (2012) Managing fire mosaics for small mammal conservation: a landscape perspective. Journal of Applied Ecology 49(2), 412-421.
| Crossref | Google Scholar |
Kelly LT, Giljohann KM, Duane A, Aquilué N, Archibald S, Batllori E, Bennett AF, Buckland ST, Canelles Q, Clarke MF, Fortin M-J, Hermoso V, Herrando S, Keane RE, Lake FK, McCarthy MA, Morán-Ordóñez A, Parr CL, Pausas JG, Penman TD, Regos A, Rumpff L, Santos JL, Smith AL, Syphard AD, Tingley MW, Brotons L (2020) Fire and biodiversity in the Anthropocene. Science 370(6519), eabb0355.
| Crossref | Google Scholar |
Laidlaw MJ, Hines HB, Melzer RI, Churchill TB (2022) Beyond bushfire severity: mapping the ecological impact of bushfires on the Gondwana Rainforests of Australia World Heritage Area. Australian Zoologist 42(2), 502-513.
| Crossref | Google Scholar |
Lawes MJ, Crisp MD, Clarke PJ, Murphy BP, Midgley JJ, Russell-Smith J, Nano CEM, Bradstock RA, Enright NJ, Fontaine JB, Gosper CR, Woolley L-A (2022) Appraising widespread resprouting but variable levels of postfire seeding in Australian ecosystems: the effect of phylogeny, fire regime and productivity. Australian Journal of Botany 70(2), 114-130.
| Crossref | Google Scholar |
Legge S, Woinarski JCZ, Scheele BC, Garnett ST, Lintermans M, Nimmo DG, Whiterod NS, Southwell DM, Ehmke G, Buchan A, Gray J, Metcalfe DJ, Page M, Rumpff L, van Leeuwen S, Williams D, Ahyong ST, Chapple DG, Cowan M, Hossain MA, Kennard M, Macdonald S, Moore H, Marsh J, McCormack RB, Michael D, Mitchell N, Newell D, Raadik TA, Tingley R (2022) Rapid assessment of the biodiversity impacts of the 2019–2020 Australian megafires to guide urgent management intervention and recovery and lessons for other regions. Diversity and Distributions 28(3), 571-591.
| Crossref | Google Scholar |
Leigh EG, Jr (1975) Structure and climate in tropical rain forest. Annual Review of Ecology and Systematics 6, 67-86.
| Crossref | Google Scholar |
Liedloff AC, Wilson JC, Engeman RM (2018) The effect of wildfire on population dynamics for two native small mammal species in a coastal heathland in Queensland, Australia. Acta Oecologica 88, 58-64.
| Crossref | Google Scholar |
MacGregor CI, Cunningham RB, Lindenmayer DB (2015) Nest-site selection of the long-nosed bandicoot (Perameles nasuta) in a postfire environment. Australian Journal of Zoology 63(5), 324-330.
| Crossref | Google Scholar |
Middleton AD, Kauffman MJ, McWhirter DE, Jimenez MD, Cook RC, Cook JG, Albeke SE, Sawyer H, White PJ (2013) Linking anti-predator behaviour to prey demography reveals limited risk effects of an actively hunting large carnivore. Ecology Letters 16(8), 1023-1030.
| Crossref | Google Scholar | PubMed |
Miles MA, de Souza AA, Póvoa MM (1981) Mammal tracking and nest location in Brazilian forest with an improved spool-and-line device. Journal of Zoology 195(3), 331-347.
| Crossref | Google Scholar |
Mitchell WF, Boulton RL, Ireland L, Hunt TJ, Verdon SJ, Olds LGM, Hedger C, Clarke RH (2022) Using experimental trials to improve translocation protocols for a cryptic, endangered passerine. Pacific Conservation Biology 28(1), 68-79.
| Crossref | Google Scholar |
Neldner V, Butler D, Guymer G, Fensham R, Holman J, Cogger H, Ford H, Johnson C, Holman J, Butler D (2019) Queensland’s regional ecosystems. Building and maintaining a biodiversity inventory, planning framework and information system for Queensland. Department of Environment and Science, Queensland Government, Brisbane, Qld, Australia.
Nimmo DG, Kelly LT, Farnsworth LM, Watson SJ, Bennett AF (2014) Why do some species have geographically varying responses to fire history? Ecography 37(8), 805-813.
| Crossref | Google Scholar |
Nimmo DG, Avitabile S, Banks SC, Bliege Bird R, Callister K, Clarke MF, Dickman CR, Doherty TS, Driscoll DA, Greenville AC, Haslem A, Kelly LT, Kenny SA, Lahoz-Monfort JJ, Lee C, Leonard S, Moore H, Newsome TM, Parr CL, Ritchie EG, Schneider K, Turner JM, Watson S, Westbrooke M, Wouters M, White M, Bennett AF (2019) Animal movements in fire-prone landscapes. Biological Reviews of the Cambridge Philosophical Society 94(3), 981-998.
| Crossref | Google Scholar | PubMed |
Nolan RH, Boer MM, Collins L, Resco de Dios V, Clarke H, Jenkins M, Kenny B, Bradstock RA (2020) Causes and consequences of eastern Australia’s 2019–20 season of mega-fires. Global Change Biology 26(3), 1039-1041.
| Crossref | Google Scholar | PubMed |
Ondei S, Prior LD, McGregor HW, Reid AM, Johnson CN, Vigilante T, Goonack C, Williams D, Bowman DMJS (2021) Small mammal diversity is higher in infrequently compared with frequently burnt rainforest–savanna mosaics in the north Kimberley, Australia. Wildlife Research 48(3), 218-229.
| Crossref | Google Scholar |
Pardon LG, Brook BW, Griffiths AD, Braithwaite RW (2003) Determinants of survival for the Northern Brown Bandicoot under a landscape-scale fire experiment. Journal of Animal Ecology 72(1), 106-115.
| Crossref | Google Scholar |
Queensland Government (2021) Fire extent and severity 2019–2020 – South East Queensland. (State of Queensland) Avaliable at https://www.data.qld.gov.au/dataset/fire-extent-and-severity-2019-2020-south-east-queensland [Verified 11 February 2025]
Robinson NM, Leonard SWJ, Ritchie EG, Bassett M, Chia EK, Buckingham S, Gibb H, Bennett AF, Clarke MF (2013) Review: Refuges for fauna in fire-prone landscapes: their ecological function and importance. Journal of Applied Ecology 50(6), 1321-1329.
| Crossref | Google Scholar |
Ryan CM, Hobbs RJ, Valentine LE (2020) Bioturbation by a reintroduced digging mammal reduces fuel loads in an urban reserve. Ecological Applications 30(2), e02018.
| Crossref | Google Scholar |
Sanford RL, Jr., Saldarriaga J, Clark KE, Uhl C, Herrera R (1985) Amazon rain-forest fires. Science 227(4682), 53-55.
| Crossref | Google Scholar | PubMed |
Santos JL, Hradsky BA, Keith DA, Rowe KC, Senior KL, Sitters H, Kelly LT (2022) Beyond inappropriate fire regimes: a synthesis of fire-driven declines of threatened mammals in Australia. Conservation Letters 15(5), e12905.
| Crossref | Google Scholar |
Santosa Y, Kwatrina RT (2020) Effect of forest fire on mammals: comparisons of species diversity on different time-period and area. IOP Conference Series: Earth and Environmental Science 528, 012023.
| Crossref | Google Scholar |
Sharp Bowman TR, McMillan BR, St. Clair SB (2017) A comparison of the effects of fire on rodent abundance and diversity in the Great Basin and Mojave Deserts. PLoS ONE 12(11), e0187740.
| Crossref | Google Scholar |
Sitters H, Di Stefano J (2020) Integrating functional connectivity and fire management for better conservation outcomes. Conservation Biology 34(3), 550-560.
| Crossref | Google Scholar | PubMed |
Smith AL (2018) Successional changes in trophic interactions support a mechanistic model of post-fire population dynamics. Oecologia 186(1), 129-139.
| Crossref | Google Scholar | PubMed |
Smith AL, Michael Bull C, Driscoll DA (2013) Successional specialization in a reptile community cautions against widespread planned burning and complete fire suppression. Journal of Applied Ecology 50(5), 1178-1186.
| Crossref | Google Scholar |
Smith AL, Landguth EL, Bull CM, Banks SC, Gardner MG, Driscoll DA (2016) Dispersal responses override density effects on genetic diversity during post-disturbance succession. Proceedings of the Royal Society of London – B. Biological Sciences 283(1827), 20152934.
| Crossref | Google Scholar |
Spies TA, White EM, Kline JD, Fischer AP, Ager A, Bailey J, Bolte J, Koch J, Platt E, Olsen CS, Jacobs D, Shindler B, Steen-Adams MM, Hammer R (2014) Examining fire-prone forest landscapes as coupled human and natural systems. Ecology and Society 19(3), 9.
| Crossref | Google Scholar |
Stephens SL, Burrows N, Buyantuyev A, Gray RW, Keane RE, Kubian R, Liu S, Seijo F, Shu L, Tolhurst KG, van Wagtendonk JW (2014) Temperate and boreal forest mega-fires: characteristics and challenges. Frontiers in Ecology and the Environment 12(2), 115-122.
| Crossref | Google Scholar |
Sutherland EF, Dickman CR (1999) Mechanisms of recovery after fire by rodents in the Australian environment: a review. Wildlife Research 26(4), 405-419.
| Crossref | Google Scholar |
Valentine LE, Bretz M, Ruthrof KX, Fisher R, Hardy GESJ, Fleming PA (2017) Scratching beneath the surface: bandicoot bioturbation contributes to ecosystem processes. Austral Ecology 42(3), 265-276.
| Crossref | Google Scholar |
Valentine LE, Ruthrof KX, Fisher R, Hardy GESJ, Hobbs RJ, Fleming PA, Stevens C (2018) Bioturbation by bandicoots facilitates seedling growth by altering soil properties. Functional Ecology 32(9), 2138-2148.
| Crossref | Google Scholar |
van Mantgem EF, Keeley JE, Witter M (2015) Faunal responses to fire in chaparral and sage scrub in California, USA. Fire Ecology 11, 128-148.
| Crossref | Google Scholar |
Vicente-Serrano SM, Quiring SM, Peña-Gallardo M, Yuan S, Dominguez-Castro F (2020) A review of environmental droughts: increased risk under global warming? Earth-Science Reviews 201, 102953.
| Crossref | Google Scholar |
Ward M, Tulloch AIT, Radford JQ, Williams BA, Reside AE, Macdonald SL, Mayfield HJ, Maron M, Possingham HP, Vine SJ, O’Connor JL, Massingham EJ, Greenville AC, Woinarski JCZ, Garnett ST, Lintermans M, Scheele BC, Carwardine J, Nimmo DG, Lindenmayer DB, Kooyman RM, Simmonds JS, Sonter LJ, Watson JEM (2020) Impact of 2019–2020 mega-fires on Australian fauna habitat. Nature Ecology & Evolution 4, 1321-1326.
| Crossref | Google Scholar | PubMed |
Ward M, Tulloch A, Stewart R, Possingham HP, Legge S, Gallagher RV, Graham EM, Southwell D, Keith D, Dixon K, Yong C, Carwardine J, Cronin T, Reside AE, Watson JEM (2022) Restoring habitat for fire-impacted species’ across degraded Australian landscapes. Environmental Research Letters 17, 084036.
| Crossref | Google Scholar |
Watchorn DJ, Dickman CR, Greenville A, Wilson BA, Garkaklis MJ, Driscoll DA, Hodgens P, Hoadley D, Groffen H, Doherty TS (2024) Artificial refuges provide post-fire habitat for small vertebrates. Biological Conservation 291, 110501.
| Crossref | Google Scholar |
Wintle BA, Legge S, Woinarski JCZ (2020) After the megafires: what next for Australian wildlife? Trends in Ecology & Evolution 35(9), 753-757.
| Crossref | Google Scholar | PubMed |
Wood SW, Murphy BP, Bowman DMJS (2011) Firescape ecology: how topography determines the contrasting distribution of fire and rain forest in the south-west of the Tasmanian Wilderness World Heritage Area. Journal of Biogeography 38(9), 1807-1820.
| Crossref | Google Scholar |
Yates DJ, Unwin GL, Doley D (1988) Rainforest environment and physiology. Proceedings of Ecological Society of Australia 15, 31-37.
| Google Scholar |
Zwolak R, Foresman KR (2007) Effects of a stand-replacing fire on small-mammal communities in montane forest. Canadian Journal of Zoology 85(7), 815-822.
| Crossref | Google Scholar |
Zylinski S, Swan M, Sitters H (2022) Contrasting responses of native and introduced mammal communities to fire mosaics in a modified landscape. Ecological Applications 32(6), e2570.
| Crossref | Google Scholar |