The influence of severe wildfire on a threatened arboreal mammal
Mikayla C. Green



A
B
C
Abstract
Fire regimes are changing with ongoing climate change, which is leading to an increase in fire frequency and severity. Australia’s Black Summer wildfires burned >12 million hectares in 2019–2020, affecting numerous threatened animal species. One of the species predicted to be most impacted was the threatened southern greater glider, an arboreal, hollow-dependent folivore, endemic to eastern Australia’s eucalypt forests.
This study aimed to assess how the 2019–2020 wildfires affected greater glider abundance and the resources they depend on in Woomargama National Park, New South Wales, Australia.
We categorised 32 sites into four fire severity treatments with eight sites for each treatment: unburned (continuous unburned vegetation); refuges (unburned patches within the fire’s perimeter); low-moderate severity; and high severity. We carried out two spotlight surveys per site using the double-observer method, beginning 21 months after the fires. We also conducted vegetation assessments on the same transects. To analyse the data, we used Generalised Linear Models to compare habitat differences based on fire severity, and N-mixture models to model greater glider detectability and abundance in relation to habitat and fire severity.
We found that fire severity depleted several habitat variables including canopy cover and the number of potentially hollow-bearing trees, a resource that greater gliders rely on. Greater glider abundance also decreased in all burn categories, with the greatest decline experienced in areas burned at high severity. We also found that greater glider abundance was much lower in fire refuges than unburned habitat outside of the fire zone.
Greater glider declines following severe wildfire can be at least partly attributed to the level of vegetation loss and the associated loss of key habitat resources. The contribution of direct mortality to population declines remains unknown.
Greater glider conservation will rely heavily on protecting expansive unburned areas of suitable habitat and maintaining hollow-bearing trees.
Keywords: Black Summer, fire regime, fire severity, habitat, hollows, marsupial, megafire, threatened species, wildfire.
Introduction
Fire is one of Earth’s most widespread abiotic disturbances and has caused significant ecological change over millions of years (Bowman et al. 2009). Fire consumes plant matter, affecting resources for plants and animals over years, decades, and centuries (Haslem et al. 2011; Bassett et al. 2017). Fire regimes have shifted (Rogers et al. 2020) and are projected to change further due to climate change (Wu et al. 2021). Fire activity in many parts of the world is increasing (Bowman et al. 2020). For example, climate change is prolonging fire seasons, increasing fuel dryness and exacerbating fire risk (Bowman et al. 2020; Duane et al. 2021; Jain et al. 2022). A series of recent extreme wildfire events around the world underscore Earth’s changing fire regimes (Duane et al. 2021), culminating in megafires (>10,000 ha burned) and gigafires (>100,000 ha burned) (Linley et al. 2022).
Fire severity is an important aspect of fire regimes that affects animal survival and population persistence through direct mortality (Jolly et al. 2022) and by causing changes in habitat structure (Chia et al. 2015; Lindenmayer et al. 2021a). Fire severity is the consumption of organic matter during fire: high severity fire, by definition, consumes more organic matter than low severity fire (Keeley 2009; May-Stubbles et al. 2022). In forest ecosystems, low to moderate severity fires burn the understorey and midstorey, whereas high severity fires consume understorey, midstorey, and canopy vegetation (Keeley 2009; Collins et al. 2021; May-Stubbles et al. 2022). High severity fires can cause the depletion of habitat resources, even in landscapes with adaptations to fire, such as eucalypt forests (Possingham et al. 1994; Lindenmayer et al. 2004; Legge et al. 2022). For example, hollow bearing trees – which are used by over 300 vertebrate species in Australia (Lindenmayer et al. 2004) – can be destroyed by high severity fire, and may not form again for decades or longer (Possingham et al. 1994; Lindenmayer et al. 2004; Legge et al. 2022). This loss is detrimental to many species, such as birds and arboreal mammals that are dependent on hollows as refuges for nesting and breeding (Gibbons et al. 2002; McLean et al. 2018). By contrast, low to moderate severity fire can aid the formation of tree hollows by causing limbs to fall off trees, creating areas for hollows to form in the future (Haslem et al. 2016). Therefore, high severity fires may present a particular risk to hollow dependent fauna species (Roberts et al. 2008; Lindenmayer et al. 2014; May-Stubbles et al. 2022).
Australia’s ‘Black Summer’ bushfires were a series of large, severe fires that occurred across southern and eastern Australia during the 2019–2020 spring and summer. The fires followed a prolonged drought (Abram et al. 2021), and displayed unprecedented fire behaviour, including a record number of firestorms (Kablick et al. 2020). Landscape features that usually act as barriers to fire – wet gullies, rocky outcrops, riparian strips, rivers and cliffs – failed to do so under such extreme conditions (Wintle et al. 2020).
The southern greater glider (Petauroides volans, herein ‘greater glider’) is an Australian endemic, arboreal marsupial that inhabits eucalypt forests across eastern Australia (McGregor et al. 2020). Populations of greater gliders are declining due to deforestation, habitat degradation, climate change, and fire (Smith and Smith 2020; Wagner et al. 2020; Ashman et al. 2021). The greater glider is listed as Endangered under the Australian Government’s Environment Protection and Biodiversity Conservation Act 1999 (EPBC Act) (Australian Government 2022b). Greater gliders are Australia’s largest gliding mammals (with a weight range of 900–1700 g) and can glide up to 100 m between trees (Van Dyck and Strahan 2008). They have a specialised folivore diet, consisting primarily of eucalypt leaves (Kavanagh and Lambert 1990). Greater gliders shelter in tree hollows, and when hollow-bearing trees are lost from an area, their abundance declines by up to 40% (McLean et al. 2018). Greater gliders are particularly vulnerable to ecological disturbance and following the 2019–2020 wildfires, experts identified them as one of the most impacted species. Local population losses have been estimated to be between 25 and 85% immediately following high severity fires (Legge et al. 2022) and it is estimated that more than 54% of greater glider location records since 2000 were burned in the 2019–2020 wildfires (Ashman et al. 2021). Isolated populations of greater gliders since these bushfires have concerningly been found to have low genetic diversity (Knipler et al. 2023). Their reliance on tree hollows and their folivorous diet makes them highly vulnerable to the spatial patterns of high severity fires (McLean et al. 2018; Wagner et al. 2021).
This study aimed to investigate the impacts of the 2019–2020 wildfires on greater gliders in a eucalypt forest in Woomargama National Park (NP), in southern New South Wales. Woomargama NP burned as part of a mixed severity fire that started on 29 December 2019 and was not extinguished until close to 2 months later. The western section of the park largely escaped the fire, whereas the eastern section burned at a range of severities, including high severity patches (Fig. 1) (Australian Government 2020). Furthermore, several patches within the perimeter of the fire remained unburned, and therefore could act as fire refuges (Fig. 1). These fire patterns make Woomargama NP an ideal location to study how greater gliders respond to fire, while providing baseline population information for the park. Greater gliders are known to occur in Woomargama NP (NPWS 2009); although records are relatively evenly distributed across the park, they are generally restricted to fire trails (ALA 2022).
Woomargama NP (grey) showing (a) the location of the 32 transects (circles, marking the start and end of each transect), stratified and colour-coded according to fire severity. The 2019–2020 wildfire severity is shown: light (yellow) areas burned at low to moderate fire; darker (orange/red) areas burned at high and very high severity. Photographs show examples of (b) unburned, (c) low-moderately burned, and (d) high severity burned sites. Photos: M. Green.
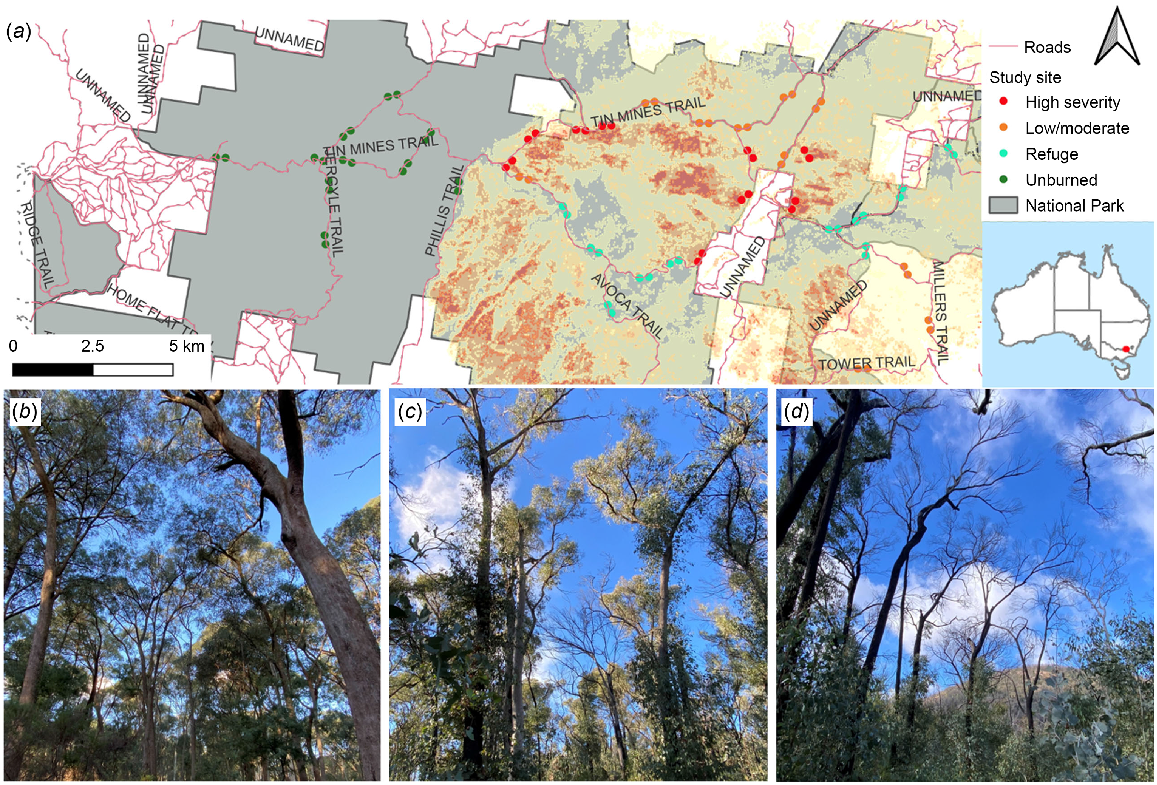
This study specifically aimed to address the following questions:
How did the severity of the 2019–2020 wildfires affect habitat structure within Woomargama NP?
Which habitat structure variables influence greater glider abundance?
Does greater glider abundance differ between fire severity treatments (unburned, low-moderate, high) and does the species occur within fire refuges at similar abundances to continuous unburned areas?
Materials and methods
Study area
This study was conducted in Woomargama NP (Supplementary material 1), located in the South Western Slopes bioregion of New South Wales. The park covers approximately 24,000 ha (NPWS 2009), comprised of wet and dry old growth sclerophyll forest (NPWS 2004; Benson 2008) on hilly and mountainous terrain, ranging from ~200 m to ~1000 m elevation. Dominant tree species include a variety of eucalypts capable of epicormic sprouting, including broad-leaved peppermint (E. dives), narrow-leaved peppermint (E. radiata), long-leaved box (E. goniocalyx), red stringybark (E. macrorhyncha), and brittle gum (E. mannifera) (Kavanagh and Stanton 1998). Wet sclerophyll forests in the park are typically dominated by narrow-leaved and broad-leaved peppermint, and dry sclerophyll forests are dominated by brittle gum and broad-leaved peppermint (Keith and Simpson 2018).
Mean monthly temperatures range between 4.4°C in July to 31.2°C in January and the average annual rainfall is 697.4 mm (Elders Weather 2022). Woomargama NP was severely affected by the Australian 2019–2020 summer wildfires, which burned ~18,000 ha of the park and the adjacent Woomargama State Forest as part of the ~630,000 ha Green Valley/Tunnel Road gigafire (sensuLinley et al. 2022). Greater gliders inhabit these forests with records distributed evenly across the park, although no previous population estimates have been made (Kavanagh and Stanton 1998; ALA 2022; Australian Government 2022b).
Experimental design and fire severity classification
This study used fire severity categories adapted from the Australian Google Earth Engine Burnt Area Map (GEEBAM) classification of the 2019–2020 wildfires developed by the Department of Agriculture, Water and the Environment (Australian Government 2020). GEEBAM has five severity categories ranging from unburned to very high, as well as an added ‘no data’ category. We combined low and medium sites as ‘low-moderate severity’, and high and very high as ‘high severity’. The combination of fire severity classes was undertaken because differences between the combined fire severity classes (i.e. between high and very high) were not always clear in the field, and to increase samples sizes within each treatment. In total, we had four fire severity treatments: unburned (continuous unburned vegetation), refuges (unburned patches within wildfire perimeter), low-moderate severity (burned with some regrowth and intact canopy), and high severity (high canopy scorch).
Eight sites were established in each of the four fire severity classes, totalling 32 sites (Fig. 1). At each site, a 300 m transect was established and ground-truthed to confirm the on-ground fire severity matched the GEEBAM map. Sites were located in the desired fire severity class within a short walking distance of nearby dirt tracks for accessibility. Sites were separated by a minimum of 500 m. Where fire severity did not conform with the map, the transect was moved to a nearby area of desired fire severity. Sites were selected to be within sclerophyll forest (the preferred greater glider habitat) at elevations between 492 m and 786 m, with most sites located in dry forests (25/32) and a smaller number (7/32) occurring in wet sclerophyll forests (based on Keith and Simpson 2018). The number of sites in each vegetation type within each fire severity treatment varied: unburned was comprised of one wet and seven dry sclerophyll transects; refuge had three wet and five dry sclerophyll transects; low-moderate had all dry sclerophyll transects; and high severity had three wet and five dry sclerophyll transects. All transects were within a single fire severity treatment (i.e. not crossing from one into another) and typically ran parallel to dirt four-wheel drive tracks. Refuge transects were within small patches of vegetation that escaped the fire, and were therefore usually close to the boundary of the fires (average distance of transect mid-point to nearest burned edge = 105 m).
Greater glider surveys
Greater glider surveys were conducted between November 2021 and March 2022 on fine/partly cloudy nights. Surveys were not undertaken during strong winds, rain, or fog, which reduce detectability (Laurance 1990; Wayne et al. 2005; Wintle et al. 2005). Thirty-two transects, separated by a minimum of 500 m, were marked with flagging tape prior to surveys. Spotlighting commenced 45 min after dusk when greater gliders were foraging (Lindenmayer et al. 1991a). We employed the double-observer method to increase detectability (Cripps et al. 2021). Two observers (observer 1 = MG, observer 2 = LW) commenced surveys approximately 10–20 min apart, depending on vegetation and visibility of the first observer’s spotlight to the second observer (Cripps et al. 2021). Transect lines were walked for ~30 min, using a handheld spotlight and binoculars to detect greater gliders. The direction, distance from transect, and colouring (light or dark) of all gliders was noted by each observer. Upon completing the transect, observers compared notes, identifying individuals based on those factors. This was done to verify how many greater gliders were recorded by both observers and how many were recorded by one observer. From there, an abundance tally of greater gliders could be formulated for the survey site. Each of the 32 transects were surveyed twice (approximately 2–3 months apart) using the double-observer method. Occupancy detection models verified that two nights of sampling was sufficient to detect greater glider presence with 95% confidence (following Kéry 2002).
Habitat surveys
Habitat surveys were conducted at all sites approximately one month after completion of fauna surveys. Habitat variables were selected based on previous research on greater gliders (see Table 1). A description and justification for each habitat variable is provided in Table 1. All variables were measured at various points along the same 300 m transect line that was used for the greater glider surveys (see Table 1 for details). Elevation was derived from a Geographic Information System (GIS) spatial layer.
Variable | Description | Justification | |
---|---|---|---|
Average midstorey height (mid height) | Height of closest midstorey plant (m) measured with range finder every 10 m along transect (30 times over 300 m) and then averaged. | The midstorey layer creates a distinct second canopy between the overstorey canopy and shrub layers which can facilitate movement but also affect detectability (Incoll et al. 2001). | |
Basal area of midstorey species (basal mid) | Measured three times per transect (0 m, 150 m, 300 m) using the Bitterlich-stick method and then averaged. | Midstorey vegetation can aid in movement and impact detectability of arboreal mammals (Lindenmayer et al. 1991b). | |
Number of vegetation strata (strata) | Number of vegetation strata (1–4) i.e. overstorey, tree midstorey, understorey/shrub layer, ground layer), determined visually three times per transect (0 m, 150 m, 300 m) and averaged. | Old growth forests, which are favoured by greater gliders, tend to have a higher number of vegetation strata (Lindenmayer et al. 1990). | |
Canopy cover (canopy) | Determined visually (present or absent) directly above observer every 10 m along the 300 m transect. Calculated as a proportion of canopy cover for each transect. | Greater gliders forage and spend most of their time within the canopy (Cunningham et al. 2004; Berry et al. 2015). | |
Average tree height (tree height) | Height of closest overstorey tree (m) measured with a range finder every 10 m along the transect (30 times over 300 m) and then averaged. Tree height was measured for both burned and unburned trees from the highest point, regardless of whether or not the canopy was intact. | Greater gliders are typically found in old growth eucalypt forests with tall trees for launching (Vinson et al. 2021). | |
Large potentially hollow-bearing trees (large trees) | The number of potentially hollow-bearing (>80 cm trunk diameter (Lindenmayer et al. 2021b)) trees were counted along a 40 × 300 m belt transect (20 m either side of the transect line). Trunk diameter was determined using a tape measure. | Tree size was used as proxy for hollow potential. Greater gliders are dependent on tree hollows for protection from predators and shelter (Lindenmayer et al. 2021b, 2022; May-Stubbles et al. 2022). | |
Elevation | Determined using a layer on GIS. | Greater gliders attain higher abundance at elevations >500 m (Smith and Smith 2018). Elevation corresponds to changes in temperature, rainfall, soil types, and vegetation composition. |
Data analysis
We used Linear Models (LMs) and Generalised Linear Models (GLMs) to examine differences in habitat variables across the fire severity classes. LMs were developed for continuous response variables including average tree height, average midstorey height, the number of strata at each site, and the basal area for overstorey and midstorey trees. GLMs were developed for modelling canopy cover, which is a proportion and hence modelled assuming a binomial distribution, and the number of large potentially hollow-bearing trees was modelled assuming a quasi-Poisson distribution (to account for overdispersion). Fire severity was a categorical predictor variable with four levels: unburned, refuge, low-moderate severity, and high severity. Unburned sites were specified as the reference category. Differences were regarded as significant if the 95% confidence interval did not overlap zero (for coefficient estimates from LMs) or one (for odds ratios and incidence rate ratios from GLMs).
We created a correlation matrix to assess whether habitat variables exhibited collinearity >0.7 (Dormann et al. 2013). The number of vegetation strata was correlated with the proportion of canopy cover >0.7. Therefore, we included only canopy cover in the N-mixture models.
In order to identify habitat variables related to the abundance of greater gliders – and which therefore might explain differences in greater glider abundance between fire severity classes – we used N-mixture models in R’s unmarked package (Fiske and Chandler 2011; Schmidt et al. 2015). N-mixture models predict abundance at sites while considering factors affecting detection probability (Fiske and Chandler 2011; Schmidt et al. 2015; Kidwai et al. 2019). While our research questions are not focused on detectability, accounting for detectability is important when surveying animals under varying weather conditions, at varying distances from the observer, and in the presence of features that can visually obstruct animals. N-mixture models rely on repeated counts, which was appropriate in our instances because we had counts of greater gliders (i.e. the total number of individual greater gliders observed by the two observers) from two repeat surveys (Keever et al. 2017). We compared N-mixture models fit with Poisson, negative binomial, and zero inflated Poisson distributions using Akaike’s Information Criterion (AIC).
We fit an N-mixture model with habitat variables (Table 1) as predictors of greater glider detection and abundance. We expected that rain (mm within 24 h of the survey) and minimum temperature (within 24 h of the survey) could impact greater glider behaviour. In addition, we expected that basal midstorey, midstorey height, and tree height could influence greater glider detection due to their impact on the level of visual obstruction and distance between the surveyor and greater gliders in the canopy. We also expected that canopy cover, tree height, the number of potentially hollow-bearing trees, midstorey height, midstorey basal area, and elevation would influence greater glider abundance.
We developed a model that included all predictor variables using the dredge function in R package ‘MuMIn’ to fit all combinations of predictors and compared support for each model using Akaike’s Information Criterion (AIC). The Akaike weight (wi) for each model was calculated, and if no model had a weight of <0.9, model averaging was undertaken using the 95% confidence set (Burnham and Anderson 1998). We considered a variable as important to greater glider detectability or abundance when the 85% confidence interval of the coefficient did not include zero (Arnold 2010). All statistical analyses were performed within R ver. 4.3.2 using the unmarked package for N-mixture models (Fiske and Chandler 2011), MuMIn for model selection (Barton and Barton 2015) and ggplot2 for plotting the models (Wickham 2016).
To test how fire severity affects greater glider abundance, the same procedure as above was followed although the predictors were changed. The predicter variables included fire severity (unburned, refuge, low-moderate and high) as well as tree height, elevation, and vegetation type (wet or dry sclerophyll). These latter variables acted as covariates to account for pre-existing differences among sites, and were able to be included alongside the fire severity treatment because they were not colinear with fire severity.
Results
In total, 101 greater glider observations were made at 17 out of 32 (53%) sites over the two survey rounds. Overall, 39 greater gliders were recorded during the first survey round and 62 were recorded during the second survey round (Supplementary material 2). The double observer method proved effective in identifying individuals that single observers missed. In the first round of surveys, this approach resulted in the detection of an additional 7–14 individuals (depending on the individual observer). In the second round, it helped identify between 13 and 15 more individuals. The average number of greater gliders observed during an individual survey was 21.8–55.9% and 26.52–31.95% higher (due to the double observer method) for survey rounds one and two, respectively (Supplementary material 2). More greater gliders were recorded in the second than in the first round, perhaps due to the timing of the second round coinciding with the emergence of independent young. During both surveys, greater gliders were recorded at all unburned sites (8/8), half of the refuge sites (4/8) and low-moderate sites (4/8), and one high severity site (1/8).
How did the 2019–2020 wildfires affect habitat structure?
Several habitat variables differed across the fire severity classes. Compared to unburned sites, burned sites had fewer vegetation strata and large trees, less canopy cover, and a shorter, denser midstorey (Table 2). Often, differences were most pronounced (i.e. larger effect sizes) when comparing unburned and high severity sites (Table 2). Unburned sites and refuges did not differ in the number of large trees, canopy cover, tree height, mid height or midstorey basal area. However, refuge sites tended to have fewer strata than unburned sites (Table 2). There was no significant difference in elevation between unburned sites and any other treatment (Table 2), probably due to the relatively narrow elevation range (~300 m) that the study sites encompassed.
Variable | Predictors | CI | ||
---|---|---|---|---|
Mid height | Estimates | |||
(Intercept) | 8.18 | 7.29–9.06 | ||
Refuge | −1.07 | −2.33 – 0.18 | ||
Low-moderate severity | −1.45 | −2.70 – −0.19 | ||
High severity | −1.09 | −2.35 – 0.17 | ||
R2 | 0.169 | |||
Basal mid | Estimates | |||
(Intercept) | 4.12 | 2.47–5.78 | ||
Refuge | 1.83 | −0.51 – 4.17 | ||
Low-moderate severity | 0.92 | −1.42 – 3.26 | ||
High severity | 2.83 | 0.49–5.17 | ||
R2 | 0.182 | |||
Strata | Estimates | |||
(Intercept) | 3.63 | 3.44–3.81 | ||
Refuge | −0.38 | −0.64 – −0.11 | ||
Low-moderate severity | −0.58 | −0.85 – −0.32 | ||
High severity | −1.54 | −1.81 – −1.27 | ||
R2 | 0.833 | |||
Large trees | Incidence rate ratios | |||
(Intercept) | 52.63 | 41.23–65.94 | ||
Refuge | 0.7 | 0.48–1.00 | ||
Low-moderate severity | 0.51 | 0.34–0.76 | ||
High severity | 0.31 | 0.19–0.50 | ||
R2 | 0.995 | |||
Canopy cover | Odds ratios | |||
(Intercept) | 3.8 | 2.81–5.24 | ||
Refuge | 0.69 | 0.45–1.06 | ||
Low-moderate severity | 0.25 | 0.17–0.38 | ||
High severity | 0 | 0.00–0.01 | ||
Tree height | Estimates | |||
(Intercept) | 18.97 | 16.99–20.95 | ||
Refuge | 1.98 | −0.82 – 4.77 | ||
Low-moderate severity | 0.63 | −2.17 – 3.42 | ||
High severity | −0.14 | −2.94 – 2.65 | ||
R2 | 0.09 | |||
Elevation | Estimates | |||
(Intercept) | 686.21 | 631.92–740.50 | ||
Refuge | −68.47 | −145.25 – 8.31 | ||
Low-moderate severity | −46.93 | −123.71 – 29.85 | ||
High severity | −52.13 | −128.91 – 24.65 | ||
R2 | 0.108 |
Bold = 95% confidence intervals do not overlap zero or one.
How does habitat structure affect greater glider detectability and abundance?
The Poisson model had the lowest AIC value and was therefore used throughout. No model was identified clearly as best during model selection (i.e. wi < 0.9), so model averaging was undertaken. Greater gliders were less detectable at sites with a denser midstorey and were more abundant at sites with greater canopy cover, more large trees, taller midstorey, and with taller trees (Fig. 2). Greater gliders were also more abundant at sites at higher elevation (Table 3).
Effect of (a) number of large potentially hollow-bearing trees, (b) average tree height, (c) average midstorey height and (d) proportion of canopy cover on greater glider abundance (λ).
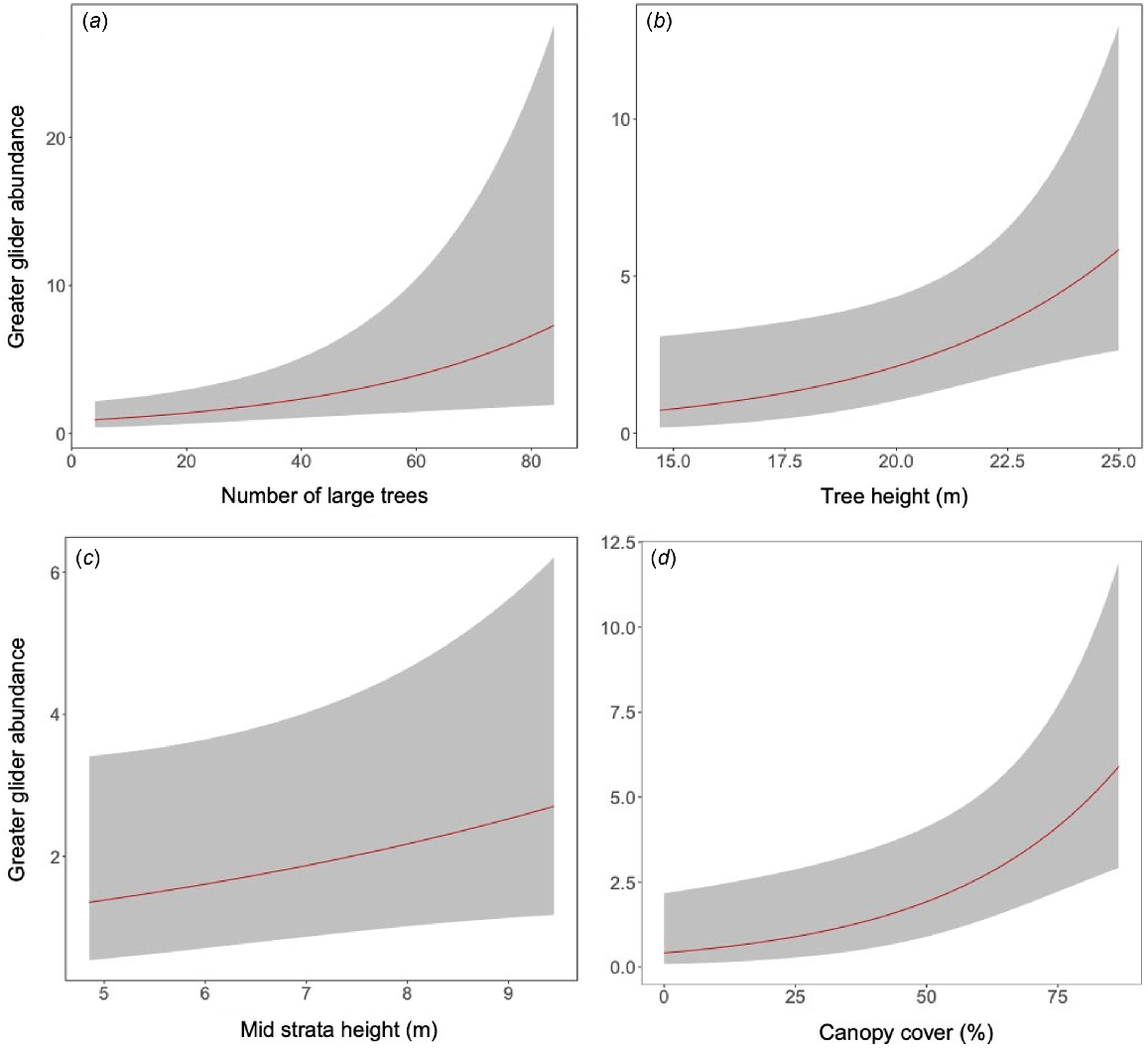
Estimate | s.e. | Z value | Pr(>|z|) | CI | ||
---|---|---|---|---|---|---|
P(Int) | −0.882428 | 0.400946 | 2.201 | 0.0277 | −1.47 – −0.28 | |
P(rainfall) | 0.420568 | 0.565119 | 0.744 | 0.4567 | −0.43 – 1.32 | |
P(basal mid) | −0.471602 | 0.304145 | 1.551 | 0.1210 | −0.92 – −0.02 | |
P(tree height) | 0.130546 | 0.373024 | 0.35 | 0.7263 | −0.36 – 0.68 | |
P(min temp) | 0.088155 | 0.209666 | 0.42 | 0.6741 | −0.22 – 0.39 | |
λ(Int) | 0.713474 | 0.35922 | 1.986 | 0.0470 | 0.23–1.25 | |
λ(canopy prop) | 0.863911 | 0.36604 | 2.36 | 0.0183 | 0.32–1.30 | |
λ(large trees) | 0.656422 | 0.181488 | 3.617 | 0.0003 | 0.50–0.92 | |
λ(mid height) | 0.25284 | 0.125177 | 2.02 | 0.0434 | 0.06–0.41 | |
λ(tree height) | 0.386298 | 0.203274 | 1.9 | 0.0574 | 0.08–0.61 | |
λ(elevation) | 0.300241 | 0.20839 | 1.441 | 0.1497 | 0.00–0.60 | |
λ(basal mid) | 0.005698 | 0.331419 | 0.017 | 0.9863 | −0.33 – 0.54 |
Bold = 85% confidence intervals did not overlap zero.
How does fire severity affect greater glider abundance?
No model was identified clearly as best (i.e. wi < 0.9). Model averaging showed minimum temperature and rainfall within 24 h of the survey had a positive effect on greater glider detectability (Table 4). Unburned sites had a higher abundance of greater gliders than refuge sites, low-moderate, and high fire severity sites (Table 4). Greater gliders were most abundant on unburned sites, with a predicted abundance of 11.24 individuals per site (Fig. 3). Abundance was lower in all other treatments, including refuge sites, ranging between 0.31 and 0.96 gliders (Fig. 3), although the effect size was greatest in high severity sites (indicative of greater reductions in abundance) (Table 4). Tree height and elevation had a positive association with greater glider abundance and greater glider abundance was similar between dry and wet sclerophyll forest types (Table 4).
Estimate | s.e. | Z value | Pr(>|z|) | CI | ||
---|---|---|---|---|---|---|
P(Int) | −1.997815 | 2.027463 | 0.985 | 0.3244 | −4.92 – 0.92 | |
P(min temp) | 0.19147 | 0.091925 | 2.083 | 0.0373 | 5.91–0.32 | |
P(basal midstorey) | 0.045202 | 0.107784 | 0.419 | 0.6749 | −0.1 – 0.20 | |
P(rainfall) | 0.011596 | 0.008042 | 1.442 | 0.1494 | 0.00–0.023 | |
P(tree height) | 0.059877 | 0.160479 | 0.373 | 0.7091 | −0.17 – 0.29 | |
λ(Int) | 2.450027 | 0.260043 | 9.422 | <2e-16 | 2.08–2.82 | |
λ(refuge) | −2.364303 | 0.403566 | 5.859 | <2e-16 | −2.95 – −1.78 | |
λ(low-moderate severity) | −2.484345 | 0.44557 | 5.576 | <2e-16 | −3.13 – −1.84 | |
λ(high severity) | −3.601912 | 0.747643 | 4.818 | 1.50e-06 | −4.68 – −2.53 | |
λ(tree height) | 0.644317 | 0.157756 | 4.084 | 4.42e-05 | 0.41–0.87 | |
λ(elevation) | 0.245798 | 0.170705 | 1.44 | 0.1499 | 0.00–0.49 | |
λ(wet sclerophyll) | 0.558264 | 0.475695 | 1.174 | 0.2406 | −0.12 – 1.24 |
Bold = 85% confidence intervals did not overlap zero.
Discussion
How did the 2019–2020 wildfires affect habitat structure?
The 2019–2020 bushfires significantly affected various vegetation attributes in Woomargama NP, the most noticeable being the impact on overstorey vegetation. Large trees and canopy cover saw considerable reductions, and the number of vegetation layers decreased. These changes were most pronounced at sites that experienced high severity fires. Changes in the midstory were less pronounced, but generally indicated a higher density of shorter plants, most likely rapid regrowth of shrubs and eucalypts stimulated by the fires. Of the five key factors influencing greater glider abundance, three (large trees, canopy cover, and midstorey height) were negatively impacted by the bushfires.
Several studies have documented that fire severity exerts detrimental effects on tree hollow abundance in eucalypt forests (Collins et al. 2012; Lindenmayer et al. 2012; May-Stubbles et al. 2022). High severity fire can damage tree hollows or consume hollow-bearing trees entirely (Collins et al. 2012), and may also trigger the collapse of hollow-bearing trees (Gibbons et al. 2008). During vegetation surveys in Woomargama, large fallen trees were often seen in areas that were subjected to high severity fire. Formation of hollows in eucalypts can take over 150 years (Banks et al. 2011); hence, the impact of the 2019–2020 fires on greater gliders and other hollow-dependent fauna are likely to perist for decades. Canopy vegetation is typically consumed in high severity fires (Smucker et al. 2005), and recurrent severe fires can culminate in the loss of canopy cover, and extensive charring can impede recovery (Haslem et al. 2016).
How does fire severity affect greater gliders?
We found a positive association between greater glider abundance and several habitat factors that are typical of unburned habitat, including the number of large trees, high canopy cover, a taller midstorey, and a more complete canopy. The 2019–2020 bushfires negatively impacted many of these habitat features. Consequently, we observed a marked decrease in the greater glider abundance in all burned areas, with the greatest decline in areas that experienced high severity fires. Similar results have been observed in other studies investigating the impacts of fire on greater glider populations (Berry et al. 2015; McLean et al. 2018; Campbell-Jones et al. 2022), including a recent study that found greater glider occurrence decreased with increasing fire severity in the Southern Tablelands of New South Wales (May-Stubbles et al. 2022).
Fire negatively affects animals through direct mortality (Bradstock et al. 2005; Jolly et al. 2022), and indirect mortality by altering habitat, reducing availability of resources, and causing increased rates of predation in the post-fire landscape (Whelan et al. 2002; Engstrom 2010; Jolly et al. 2022). A systematic review that explored the fate of individual animals before and after fire found that animal mortality during fire is often lower than assumed (1–9%), but that mortality is higher during high severity fires (Jolly et al. 2022). Recent modelling by Zylstra (2023) estimated that the critically endangered arboreal mammal, ngwayir (western ringtail possum, Pseudocheirus occidentalis), suffered 77% mortality during a prescribed burn in a woodland in Western Australia. By contrast, a study that directly tracked the fate of mountain brushtail possums (Trichosurus cunninghami) through Victoria’s Black Saturday fires found no direct mortality (Banks et al. 2011). It has previously been found that greater gliders can often initially survive severe disturbances when their home range has only partially been destroyed, by either remaining in hollows or fleeing. Tyndale-Biscoe and Smith (1969) marked greater gliders at the point of felling during logging, with over 70% of individuals being re-captured within 1 week of logging. This number dropped below 7% of the original capture rate just 1 year after felling (Tyndale-Biscoe and Smith 1969). It is estimated that the intensity of disturbance and spatial distribution of critical habitat and feeding resources drives these population dynamics over time (Wagner et al. 2021). Untangling the relative contributions of direct mortality and fire-induced habitat changes that lead to reductions in greater glider abundance warrants further research.
Fire refuges are areas within the fire zone which could allow animals to persist while burned areas recover (Robinson et al. 2013; Wintle et al. 2020; Mackey et al. 2021). Results from studies on the effectiveness of refugia for population recovery often vary, particularly due to differences in species’ mechanisms for survival, connectivity, dispersal and persistence during and after fire (Meddens et al. 2018). Previous research has shown that small mammals (Griffiths and Brook 2014), birds (Robinson et al. 2014) and some reptile species (Doherty et al. 2015) are able to persist after fire by moving into unburned patches. Our study found that greater glider abundance in fire refuges was significantly lower than in areas of continuously unburned forest outside of the fire zone. A study from the Victorian mountain ash forests found similar results for greater gliders as well as Leadbeater’s possum (Gymnobelideus leadbeateri) and inland sugar glider (Petaurus notatus; previously sugar glider (Petaurus breviceps)) (Lindenmayer et al. 2013). While pre-existing differences between unburned and refuge sites may have plausibly contributed to the observed differences, this is unlikely in our view. Refuge sites were similar to unburned sites in most aspects, including those identified as drivers of greater glider abundance (large trees, canopy cover, mid height, tree height, and elevation). Our refuge sites were mostly unburned strips surrounded by high proportions of burned areas on either side. While our study was not designed to examine the influence of refuge patch size, this may be an important consideration for future studies. With an average distance of ~100 m from refuge transects to the nearest burned edge, it is possible, even likely, that the refuges that we surveyed are too small or narrow to conserve populations of greater gliders after severe fire. The current findings suggest that small unburned refuges within the fire footprint seem to play a minimal role in the conservation of greater gliders in Woomargama NP, and that large expanses of unburned vegetation are vital for their long-term persistence.
While our study offers insight into the effects of severe fire on greater gliders, it is not without limitations. First, our study is a control-impact study, rather than a before-after-control-impact study, and hence our inference assumes that sites were similar prior to the fires. It is possible that differences existed prior to the fires, and that we are interpreting these differences as being driven by fire when they are pre-existing. This underscores the importance of long-term monitoring of threatened species so that before data is available when large disturbances like the 2019–2020 wildfires occur. However, given the consistency between our findings and previous studies of greater glider responses to wildfire, we believe the patterns observed are largely attributable to fire. Second, our study analysed greater glider abundance in relation to broad environmental drivers. Further variation in greater glider abundance would probably be explained by variables that we did not measure, such as the distribution of preferred eucalypt species within transects (Youngentob et al. 2011).
Conclusion
Our study confirms that fire, and particularly high severity fire, transforms vegetation structure and diminishes habitat quality for the greater glider. This likely leads to a reduction in greater glider abundance in burned sites. The conservation of greater gliders in tall eucalypt forests will depend on protecting large expanses of old growth vegetation from severe fire events (Lindenmayer et al. 2013; Andrus et al. 2021; Australian Government 2022a).
Declaration of funding
This project was supported by Murray Local Land Services, Holbrook Landcare and the Gulbali Institute at Charles Sturt University.
Acknowledgements
We acknowledge the Wiradjuri Traditional Owners of the land on which this study occurred. We thank Dave Pearce (NPWS) for access to Woomargama NP and are thankful to the reviewers for providing constructive feedback on the manuscript.
References
Abram NJ, Henley BJ, Sen Gupta A, Lippmann TJR, Clarke H, Dowdy AJ, Sharples JJ, Nolan RH, Zhang T, Wooster MJ, Wurtzel JB, Meissner KJ, Pitman AJ, Ukkola AM, Murphy BP, Tapper NJ, Boer MM (2021) Connections of climate change and variability to large and extreme forest fires in southeast Australia. Communications Earth & Environment 2, 8.
| Crossref | Google Scholar |
Andrus RA, Martinez AJ, Jones GM, Meddens AJH (2021) Assessing the quality of fire refugia for wildlife habitat. Forest Ecology and Management 482, 118868.
| Crossref | Google Scholar |
Arnold TW (2010) Uninformative parameters and model selection using akaike’s information criterion. The Journal of Wildlife Management 74, 1175-1178.
| Crossref | Google Scholar |
Ashman KR, Watchorn DJ, Lindenmayer DB, Taylor MFJ (2021) Is Australia’s environmental legislation protecting threatened species? A case study of the national listing of the greater glider. Pacific Conservation Biology 28, 277-289.
| Crossref | Google Scholar |
Banks SC, Knight EJ, McBurney L, Blair D, Lindenmayer DB (2011) The effects of wildfire on mortality and resources for an arboreal marsupial: resilience to fire events but susceptibility to fire regime change. PLoS ONE 6, e22952.
| Crossref | Google Scholar | PubMed |
Bassett M, Leonard SWJ, Chia EK, Clarke MF, Bennett AF (2017) Interacting effects of fire severity, time since fire and topography on vegetation structure after wildfire. Forest Ecology and Management 396, 26-34.
| Crossref | Google Scholar |
Benson J (2008) New South Wales vegetation classification and assessment: Part 2 plant communities of the NSW south-western slopes bioregion and update of NSW western plains plant communities, version 2 of the NSWVCA database. Cunninghamia 10, 599-673.
| Google Scholar |
Berry LE, Driscoll DA, Banks SC, Lindenmayer DB (2015) The use of topographic fire refuges by the greater glider (Petauroides volans) and the mountain brushtail possum (Trichosurus cunninghami) following a landscape-scale fire. Australian Mammalogy 37, 39-45.
| Crossref | Google Scholar |
Bowman DMJS, Balch JK, Artaxo P, Bond WJ, Carlson JM, Cochrane MA, D’Antonio CM, DeFries RS, Doyle JC, Harrison SP, Johnston FH, Keeley JE, Krawchuk MA, Kull CA, Marston JB, Moritz MA, Prentice IC, Roos CI, Scott AC, Swetnam TW, van der Werf GR, Pyne SJ (2009) Fire in the earth system. Science 324, 481-484.
| Crossref | Google Scholar | PubMed |
Bowman DMJS, Kolden CA, Abatzoglou JT, Johnston FH, van der Werf GR, Flannigan M (2020) Vegetation fires in the anthropocene. Nature Reviews Earth & Environment 1, 500-515.
| Crossref | Google Scholar |
Bradstock RA, Bedward M, Gill AM, Cohn JS (2005) Which mosaic? A landscape ecological approach for evaluating interactions between fire regimes, habitat and animals. Wildlife Research 32, 409-423.
| Crossref | Google Scholar |
Campbell-Jones MM, Bassett M, Bennett AF, Chia EK, Leonard S, Collins L (2022) Fire severity has lasting effects on the distribution of arboreal mammals in a resprouting forest. Austral Ecology 47, 1456-1469.
| Crossref | Google Scholar |
Chia EK, Bassett M, Nimmo DG, Leonard SWJ, Ritchie EG, Clarke MF, Bennett AF (2015) Fire severity and fire-induced landscape heterogeneity affect arboreal mammals in fire-prone forests. Ecosphere 6, 1-14.
| Crossref | Google Scholar |
Collins L, Bradstock RA, Tasker EM, Whelan RJ (2012) Impact of fire regimes, logging and topography on hollows in fallen logs in eucalypt forest of south eastern Australia. Biological Conservation 149, 23-31.
| Crossref | Google Scholar |
Collins L, Hunter A, McColl-Gausden S, Penman TD, Zylstra P (2021) The effect of antecedent fire severity on reburn severity and fuel structure in a resprouting eucalypt forest in Victoria, Australia. Forests 12, 450.
| Crossref | Google Scholar |
Cripps JK, Nelson JL, Scroggie MP, Durkin LK, Ramsey DSL, Lumsden LF (2021) Double-observer distance sampling improves the accuracy of density estimates for a threatened arboreal mammal. Wildlife Research 48, 756-768.
| Crossref | Google Scholar |
Cunningham RB, Pope ML, Lindenmayer DB (2004) Patch use by the greater glider (Petauroides volans) in a fragmented forest ecosystem. III. Night-time use of trees. Wildlife Research 31, 579-585.
| Crossref | Google Scholar |
Doherty TS, Davis RA, van Etten EJB, Collier N, Krawiec J (2015) Response of a shrubland mammal and reptile community to a history of landscape-scale wildfire. International Journal of Wildland Fire 24, 534-543.
| Crossref | Google Scholar |
Dormann CF, Elith J, Bacher S, Buchmann C, Carl G, Carré G, Marquéz JRG, Gruber B, Lafourcade B, Leitão PJ, Münkemüller T, McClean C, Osborne PE, Reineking B, Schröder B, Skidmore AK, Zurell D, Lautenbach S (2013) Collinearity: a review of methods to deal with it and a simulation study evaluating their performance. Ecography 36, 27-46.
| Crossref | Google Scholar |
Duane A, Castellnou M, Brotons L (2021) Towards a comprehensive look at global drivers of novel extreme wildfire events. Climatic Change 165, 43.
| Crossref | Google Scholar |
Engstrom RT (2010) First-order fire effects on animals: review and recommendations. Fire Ecology 6, 115-130.
| Crossref | Google Scholar |
Fiske I, Chandler R (2011) Unmarked: an r package for fitting hierarchical models of wildlife occurrence and abundance. Journal of Statistical Software 43, 1-23.
| Crossref | Google Scholar |
Gibbons P, Lindenmayer DB, Barry SC, Tanton MT (2002) Hollow selection by vertebrate fauna in forests of southeastern Australia and implications for forest management. Biological Conservation 103, 1-12.
| Crossref | Google Scholar |
Gibbons P, Cunningham RB, Lindenmayer DB (2008) What factors influence the collapse of trees retained on logged sites?: a case-control study. Forest Ecology and Management 255, 62-67.
| Crossref | Google Scholar |
Griffiths AD, Brook BW (2014) Effect of fire on small mammals: a systematic review. International Journal of Wildland Fire 23, 1034-1043.
| Crossref | Google Scholar |
Haslem A, Kelly LT, Nimmo DG, Watson SJ, Kenny SA, Taylor RS, Avitabile SC, Callister KE, Spence-Bailey LM, Clarke MF, Bennett AF (2011) Habitat or fuel? Implications of long-term, post-fire dynamics for the development of key resources for fauna and fire. Journal of Applied Ecology 48, 247-256.
| Crossref | Google Scholar |
Haslem A, Leonard SWJ, Bruce MJ, Christie F, Holland GJ, Kelly LT, MacHunter J, Bennett AF, Clarke MF, York A (2016) Do multiple fires interact to affect vegetation structure in temperate eucalypt forests? Ecological Applications 26, 2414-2423.
| Crossref | Google Scholar |
Incoll RD, Loyn RH, Ward SJ, Cunningham RB, Donnelly CF (2001) The occurrence of gliding possums in old-growth forest patches of mountain ash (Eucalyptus regnans) in the Central Highlands of Victoria. Biological Conservation 98, 77-88.
| Crossref | Google Scholar |
Jain P, Castellanos-Acuna D, Coogan SCP, Abatzoglou JT, Flannigan MD (2022) Observed increases in extreme fire weather driven by atmospheric humidity and temperature. Nature Climate Change 12, 63-70.
| Crossref | Google Scholar |
Jolly CJ, Dickman CR, Doherty TS, van Eeden LM, Geary WL, Legge SM, Woinarski JCZ, Nimmo DG (2022) Animal mortality during fire. Global Change Biology 28, 2053-2065.
| Crossref | Google Scholar | PubMed |
Kablick GP, III, Allen DR, Fromm MD, Nedoluha GE (2020) Australian pyrocb smoke generates synoptic-scale stratospheric anticyclones. Geophysical Research Letters 47, e2020GL088101.
| Crossref | Google Scholar |
Kavanagh RP, Lambert MJ (1990) Food selection by the greater glider, petauroides-volans – is foliar nitrogen a determinant of habitat quality. Wildlife Research 17, 285-299.
| Crossref | Google Scholar |
Kavanagh R, Stanton M (1998) Nocturnal forest birds and arboreal marsupials of the southwestern slopes, New South Wales. Australian Zoologist 30, 449-466.
| Crossref | Google Scholar |
Keeley JE (2009) Fire intensity, fire severity and burn severity: a brief review and suggested usage. International Journal of Wildland Fire 18, 116-126.
| Crossref | Google Scholar |
Keever AC, McGowan CP, Ditchkoff SS, Acker PK, Grand JB, Newbolt CH (2017) Efficacy of N-mixture models for surveying and monitoring white-tailed deer populations. Mammal Research 62, 413-422.
| Crossref | Google Scholar |
Kidwai Z, Jimenez J, Louw CJ, Nel HP, Marshal JP (2019) Using n-mixture models to estimate abundance and temporal trends of black rhinoceros (Diceros bicornis L.) populations from aerial counts. Global Ecology and Conservation 19, e00687.
| Crossref | Google Scholar |
Knipler ML, Gracanin A, Mikac KM (2023) Conservation genomics of an endangered arboreal mammal following the 2019–2020 Australian megafire. Scientific Reports 13(1), 480.
| Crossref | Google Scholar | PubMed |
Kéry M (2002) Inferring the absence of a species: a case study of snakes. The Journal of Wildlife Management 66, 330-338.
| Crossref | Google Scholar |
Laurance WF (1990) Effects of weather on marsupial folivore activity in a north Queensland upland tropical rainforest. Australian Mammalogy 13, 41-47.
| Crossref | Google Scholar |
Legge S, Rumpff L, Woinarski JCZ, Whiterod NS, Ward M, Southwell DG, Scheele BC, Nimmo DG, Lintermans M, Geyle HM, Garnett ST, Hayward-Brown B, Ensbey M, Ehmke G, Ahyong ST, Blackmore CJ, Bower DS, Brizuela-Torres D, Burbidge AH, Burns PA, Butler G, Catullo R, Chapple DG, Dickman CR, Doyle KE, Ferris J, Fisher D, Gallagher R, Gillespie GR, Greenlees MJ, Hohnen R, Hoskin CJ, Hunter D, Jolly C, Kennard M, King A, Kuchinke D, Law B, Lawler I, Lawler S, Loyn R, Lunney D, Lyon J, MacHunter J, Mahony M, Mahony S, McCormack RB, Melville J, Menkhorst P, Michael D, Mitchell N, Mulder E, Newell D, Pearce L, Raadik TA, Rowley JJL, Sitters H, Spencer R, Valavi R, West M, Wilkinson DP, Zukowski S (2022) The conservation impacts of ecological disturbance: time-bound estimates of population loss and recovery for fauna affected by the 2019–2020 Australian Megafires. Global Ecology and Biogeography 31, 2085-2104.
| Crossref | Google Scholar |
Lindenmayer DB, Cunningham RB, Tanton MT, Smith AP, Nix HA (1990) The conservation of arboreal marsupials in the Montane ash forests of the central highlands of Victoria, Southeast Australia: I. Factors influencing the occupancy of trees with hollows. Biological Conservation 54, 111-131.
| Crossref | Google Scholar |
Lindenmayer DB, Cunningham RB, Tanton MT, Nix HA (1991a) Aspects of the use of den trees by arboreal and scansorial marsupials inhabiting Montane ash forests in Victoria. Australian Journal of Zoology 39, 57-65.
| Crossref | Google Scholar |
Lindenmayer DB, Cunningham RB, Tanton MT, Nix HA, Smith AP (1991b) The conservation of arboreal marsupials in the montane ash forests of the central highlands of Victoria, South-east Australia: III. The habitat requirements of leadbeater’s possum Gymnobelideus leadbeateri and models of the diversity and abundance of arboreal marsupials. Biological Conservation 56, 295-315.
| Crossref | Google Scholar |
Lindenmayer DB, Pope ML, Cunningham RB (2004) Patch use by the greater glider (Petauroides volans) in a fragmented forest ecosystem. II. Characteristics of den trees and preliminary data on den-use patterns. Wildlife Research 31, 569-577.
| Crossref | Google Scholar |
Lindenmayer DB, Blanchard W, McBurney L, Blair D, Banks S, Likens GE, Franklin JF, Laurance WF, Stein JA, Gibbons P (2012) Interacting factors driving a major loss of large trees with cavities in a forest ecosystem. PLoS ONE 7, e41864.
| Crossref | Google Scholar |
Lindenmayer DB, Blanchard W, McBurney L, Blair D, Banks SC, Driscoll D, Smith AL, Gill AM (2013) Fire severity and landscape context effects on arboreal marsupials. Biological Conservation 167, 137-148.
| Crossref | Google Scholar |
Lindenmayer DB, Blanchard W, McBurney L, Blair D, Banks SC, Driscoll DA, Smith AL, Gill AM (2014) Complex responses of birds to landscape-level fire extent, fire severity and environmental drivers. Diversity and Distributions 20, 467-477.
| Crossref | Google Scholar |
Lindenmayer D, Taylor C, Blanchard W (2021a) Empirical analyses of the factors influencing fire severity in southeastern Australia. Ecosphere 12, e03721.
| Crossref | Google Scholar |
Lindenmayer DB, Blanchard W, Blair D, McBurney L, Taylor C, Scheele BC, Westgate MJ, Robinson N, Foster C (2021b) The response of arboreal marsupials to long-term changes in forest disturbance. Animal Conservation 24, 246-258.
| Crossref | Google Scholar |
Lindenmayer DB, McBurney L, Blanchard W, Marsh K, Bowd E, Watchorn D, Taylor C, Youngentob K (2022) Elevation, disturbance, and forest type drive the occurrence of a specialist arboreal folivore. PLoS ONE 17, e0265963.
| Crossref | Google Scholar | PubMed |
Linley GD, Jolly CJ, Doherty TS, Geary WL, Armenteras D, Belcher CM, Bliege Bird R, Duane A, Fletcher M-S, Giorgis MA, Haslem A, Jones GM, Kelly LT, Lee CKF, Nolan RH, Parr CL, Pausas JG, Price JN, Regos A, Ritchie EG, Ruffault J, Williamson GJ, Wu Q, Nimmo DG (2022) What do you mean, ‘megafire’? Global Ecology and Biogeography 31, 1906-1922.
| Crossref | Google Scholar |
Mackey B, Lindenmayer D, Norman P, Taylor C, Gould S (2021) Are fire refugia less predictable due to climate change? Environmental Research Letters 16, 114028.
| Crossref | Google Scholar |
May-Stubbles JC, Gracanin A, Mikac KM (2022) Increasing fire severity negatively affects greater glider density. Wildlife Research 49, 709-718.
| Crossref | Google Scholar |
McGregor DC, Padovan A, Georges A, Krockenberger A, Yoon H-J, Youngentob KN (2020) Genetic evidence supports three previously described species of greater glider, Petauroides volans, P. minor, and P. armillatus. Scientific Reports 10, 19284.
| Crossref | Google Scholar | PubMed |
McLean CM, Kavanagh RP, Penman T, Bradstock R (2018) The threatened status of the hollow dependent arboreal marsupial, the Greater Glider (Petauroides volans), can be explained by impacts from wildfire and selective logging. Forest Ecology and Management 415-416, 19-25.
| Crossref | Google Scholar |
Meddens AJ, Kolden CA, Lutz JA, Smith AM, Cansler CA, Abatzoglou JT, Meigs GW, Downing WM, Krawchuk MA (2018) Fire refugia: what are they, and why do they matter for global change? BioScience 68, 944-954.
| Crossref | Google Scholar |
Possingham HP, Lindenmayer DB, Norton TW, Davies I (1994) Metapopulation viability analysis of the greater glider Petauroides volans in a wood production area. Biological Conservation 70, 227-236.
| Crossref | Google Scholar |
Roberts SL, van Wagtendonk JW, Miles AK, Kelt DA, Lutz JA (2008) Modeling the effects of fire severity and spatial complexity on small mammals in Yosemite National Park, California. Fire Ecology 4, 83-104.
| Crossref | Google Scholar |
Robinson NM, Leonard SWJ, Ritchie EG, Bassett M, Chia EK, Buckingham S, Gibb H, Bennett AF, Clarke MF (2013) Review: Refuges for fauna in fire-prone landscapes: their ecological function and importance. Journal of Applied Ecology 50, 1321-1329.
| Crossref | Google Scholar |
Robinson NM, Leonard SWJ, Bennett AF, Clarke MF (2014) Refuges for birds in fire-prone landscapes: the influence of fire severity and fire history on the distribution of forest birds. Forest Ecology and Management 318, 110-121.
| Crossref | Google Scholar |
Rogers BM, Balch JK, Goetz SJ, Lehmann CER, Turetsky M (2020) Focus on changing fire regimes: interactions with climate, ecosystems, and society. Environmental Research Letters 15, 030201.
| Crossref | Google Scholar |
Schmidt JH, Johnson DS, Lindberg MS, Adams LG (2015) Estimating demographic parameters using a combination of known-fate and open N-mixture models. Ecology 96, 2583-2589.
| Crossref | Google Scholar | PubMed |
Smith P, Smith J (2018) Decline of the greater glider (Petauroides volans) in the lower Blue Mountains, New South Wales. Australian Journal of Zoology 66, 103-114.
| Crossref | Google Scholar |
Smith P, Smith J (2020) Future of the Greater Glider (Petauroides volans) in the Blue Mountains, New South Wales. Proceedings of the Linnean Society of New South Wales 142, 55-66.
| Google Scholar |
Smucker KM, Hutto RL, Steele BM (2005) Changes in bird abundance after wildfire: importance of fire severity and time since fire. Ecological Applications 15(5), 1535-1549.
| Crossref | Google Scholar |
Tyndale-Biscoe CH, Smith RFC (1969) Studies on the Marsupial Glider, Schoinobates volans (Kerr): III. Response to habitat destruction. The Journal of Animal Ecology 651-659.
| Crossref | Google Scholar |
Vinson SG, Johnson AP, Mikac KM (2021) Current estimates and vegetation preferences of an endangered population of the vulnerable greater glider at Seven Mile Beach National Park. Austral Ecology 46, 303-314.
| Crossref | Google Scholar |
Wagner B, Baker PJ, Stewart SB, Lumsden LF, Nelson JL, Cripps JK, Durkin LK, Scroggie MP, Nitschke CR (2020) Climate change drives habitat contraction of a nocturnal arboreal marsupial at its physiological limits. Ecosphere 11(10), e03262.
| Crossref | Google Scholar |
Wagner B, Baker PJ, Nitschke CR (2021) The influence of spatial patterns in foraging habitat on the abundance and home range size of a vulnerable arboreal marsupial in southeast Australia. Conservation Science and Practice 3(12), e566.
| Crossref | Google Scholar |
Wayne AF, Cowling A, Rooney JF, Ward CG, Wheeler IB, Lindenmayer DB, Donnelly CF (2005) Factors affecting the detection of possums by spotlighting in Western Australia. Wildlife Research 32, 689-700.
| Crossref | Google Scholar |
Wickham H (2016) ‘Ggplot2: elegant graphics for data analysis.’ (Springer-Verlag: New York) Available at https://ggplot2.tidyverse.org
Wintle BA, Kavanagh RP, McCarthy MA, Burgman MA (2005) Estimating and dealing with detectability in occupancy surveys for forest owls and arboreal marsupials. The Journal of Wildlife Management 69, 905-917.
| Google Scholar |
Wintle BA, Legge S, Woinarski JCZ (2020) After the megafires: what next for Australian wildlife? Trends in Ecology & Evolution 35, 753-757.
| Crossref | Google Scholar | PubMed |
Wu C, Venevsky S, Sitch S, Mercado LM, Huntingford C, Staver AC (2021) Historical and future global burned area with changing climate and human demography. One Earth 4, 517-530.
| Crossref | Google Scholar |
Youngentob KN, Wallis IR, Lindenmayer DB, Wood JT, Pope ML, Foley WJ (2011) Foliage chemistry influences tree choice and landscape use of a gliding marsupial folivore. Journal of Chemical Ecology 37, 71-84.
| Crossref | Google Scholar | PubMed |
Zylstra P (2023) Quantifying the direct fire threat to a critically endangered arboreal marsupial using biophysical, mechanistic modelling. Austral Ecology 48, 266-288.
| Crossref | Google Scholar |