Modelling wildfire activity in wildland–urban interface (WUI) areas of Sardinia, Italy
Carla Scarpa


A
B
C
D
Abstract
Wildfire frequency, magnitude and impacts in wildland–urban interface (WUI) areas are increasing in the Mediterranean Basin.
We investigated the role played by socio-economic, vegetation, climatic, and zootechnical drivers on WUI wildfire patterns (area burned and wildfire ignitions) in Sardinia, Italy.
We defined WUI as the 100-m buffer area of the anthropic layers. We created a comprehensive and multi-year dataset of explanatory variables and wildfires, and then trained a set of models and evaluated their performances in predicting WUI fires. We used the best models to assess the single variable’s importance and map wildfire patterns.
Random Forest and Support Vector Machine were the best performing models. In broad terms, wildfire patterns at WUI were influenced by socio-economic factors and herbaceous vegetation types.
Machine learning models can be useful tools to predict wildfire ignitions and area burned at WUI in Mediterranean areas.
Improved knowledge of the main drivers of wildfires at WUI in fire-prone Mediterranean areas can foster the development or optimisation of wildfire risk reduction and prevention strategies.
Keywords: driving factors, fire management, fire regimes, risk, machine learning models, Mediterranean areas, spatial patterns, Wildland–Urban Interface.
Introduction
In the past years, Mediterranean areas in the European Union have experienced an unprecedented increase in wildfire risk and in the occurrence of exceptionally large, extreme, and damaging wildfires (Molina-Terrén et al. 2019; Ribeiro et al. 2020; San-Miguel-Ayanz et al. 2023). The rapid expansion of urban areas towards forests and wildlands, climate change, and a variety of socio-economic changes are favouring wildfire occurrence and spread, especially in the so-called wildland–urban interface (WUI) (Bar-Massada et al. 2023; Schug et al. 2023). WUI are the transitional areas where people and houses are near or intermingled with wildland vegetation (Ribeiro et al. 2020; Ganteaume et al. 2021; Fernández-García et al. 2023). WUI are often characterised by forested zones with a continuous and unmanaged highly flammable fuel layer where human infrastructures are scattered throughout (Viegas et al. 2003; Del Giudice et al. 2021). Fuel availability in the vicinity of WUI has increased because of rural depopulation, reduction of agro-silvo-pastoral activities, and abrupt land use changes (Modugno et al. 2016). In such a dangerous context, the increased awareness of wildfire hazard and risk has led to a need for a deeper knowledge of the factors and mechanisms involved in the occurrence and propagation of fires. Specifically, identifying the drivers of wildfire ignitions and area burned within the WUI as a function of environmental, climatic, land cover, and socio-economic factors became of fundamental importance to foster wildfire prevention and management programs (Rodrigues et al. 2014; Mancini et al. 2018a, 2018b). Identification of the best modelling strategy is crucial to understand and explain the main drivers of wildfire patterns and contribute to wildfire risk assessment and prevention (Elia et al. 2020; Rodrigues et al. 2023).
Machine learning (ML) models have emerged as an innovative tool to improve the knowledge on the relationships between wildfires and their predictor variables (Wang et al. 2021). At the European level, Ganteaume et al. (2013) performed a comprehensive analysis of anthropic and environmental variables identifying the drivers associated with wildfire occurrence in the Mediterranean region. The physical and anthropogenic variables contributing to spatial and temporal patterns of wildfire occurrence at different scales were analysed by several methods, such as the Geographically Weighted Logistic Regression at multiple scales (Oliveira et al. 2013; Rodrigues et al. 2014, 2018), the calibration of Regression models at national and cross-regional scale (Mancini et al. 2018a; D’Este et al. 2021), the application of Linear Models combined with Logistic Regression (Elia et al. 2019), the Multivariate Logistic Regression methodology combined with spatial analysis from Remote Sensing and GIS data (Badia et al. 2011), the Generalised Additive Models at a regional scale, or the Generalised Linear models compared to ML models (Vilar et al. 2016a, 2016b). In recent years, the use of ML models in wildfire science has rapidly increased, and supervised ML applications have been widely and extensively employed for both classification and regression issues (Jain et al. 2020). ML holds the potential to unravel nonlinear responses enhancing the overall accuracy of their predictions (Tonini et al. 2020). In Europe, ML was used to identify the explanatory variables for wildfire occurrence and to elaborate wildfire susceptibility maps considering predisposing factors (Vacchiano et al. 2018; Milanović et al. 2020; Tonini et al. 2020). Gigović et al. (2019) found satisfactory performances of Random Forest, Support Vector Machine and their ensemble method on the creation of wildfire susceptibility maps. Many studies highlighted that ML presented higher prediction accuracy than traditional regression models (Jain et al. 2020; Bot and Borges 2022; Gao et al. 2024), demonstrating their strength for evaluating fire-related phenomena also on a larger scale, and highlighting that ML can ameliorate the prediction of wildfire occurrence in heterogeneous landscapes such as those of the Mediterranean Basin (Elia et al. 2020). For example, Random Forest outperformed Linear Regression for wildfire density mapping (Oliveira et al. 2012) and showed a better predictive ability than Regression models for the assessment of the explanatory variables related to wildfire occurrence (Rodrigues and de la Riva 2014; Milanović et al. 2020).
The high potential of ML in assessing wildfire risk and identifying the most relevant drivers is mostly determined by two factors: (1) ever-increasing availability of comprehensive datasets for both training and testing models; and (2) improvements in computing technologies (Jain et al. 2020). In general, the effectiveness of a model depends on the amount and quality of the training data, even though each single model has its own strengths and weaknesses.
The main aim of this work is to contribute to wildfire prevention and management programs by a better understanding of the drivers of wildfire patterns within the WUI areas in Sardinia, Italy. The specific objective is to investigate the socio-economic, vegetation, climatic, and zootechnical drivers on WUI wildfire patterns in terms of area burned and wildfire ignitions in one of the most wildfire prone areas of Italy. To our knowledge, this study is the first effort to identify the main drivers and predict wildfire patterns at WUI in Sardinia, leveraging an exhaustive, multi-year dataset of wildfire records and burned perimeters.
Materials and methods
Study area
Sardinia (Italy) is located in the western part of the Mediterranean Sea (38°51′N–41°15′N, 8°8′E–9°50′E). With about 24,000 km2 of land, Sardinia is the second largest island in the Mediterranean Basin. The island is composed of 377 municipalities distributed in six provinces. The population, about 1.7 million inhabitants, is concentrated around the metropolitan areas of Cagliari and Sassari; the human presence increases from late spring to early fall due to the touristic flows, which are mostly concentrated in the coastal areas.
The physical geography of the island is complex, with a significant presence of hills and low mountains, and quite heterogeneous conditions in both geological and morphological features. The climate is mostly Mediterranean, with the highest temperatures, the lowest cumulated rainfall and an important water deficit observed during the summer months, particularly in the southern portions. Annual precipitation varies from ~400 mm in the coastal areas to ~1100 mm in the mountains. The mean annual temperature ranges between ~8°C in the mountain areas to ~17°C along the coast; during the summer season maximum temperatures often exceed 30°C (Fick and Hijmans 2017; Salis et al. 2023). According to the Sardinian Land Use Map (2008) (UDS_2008), about 41% of the island is covered by grasslands, pastures, and agricultural lands. Mediterranean maquis and garrigue cover about 27% of land and are mainly represented by Pistacia lentiscus L., Arbutus unedo L., Erica arborea L., Myrtus communis L., Olea europea L. var. sylvestris Brot., Phyllirea spp., Juniperus spp., Cistus spp. and Euphorbia spp. Woodlands and broadleaf forests, mostly characterised by Quercus spp. (Quercus ilex L., Quercus suber L., Quercus pubescens Willd., and Quercus congesta Presl), occupy 21% of the island; Castanea sativa Mill., Taxus bacata L. and Ilex aquifolium L. are the main forest species at higher altitudes. Conifer stands cover 2% of the island, and are primarily represented by Pinus pinea L. and Pinus halepensis Mill.
During the period 2005–2019, Sardinia registered approximately 44,300 wildfire ignitions and about 223,300 ha burned (Fig. 1a), with a large interannual variability. On average, 15,000 ha burned every year, although in years with extreme fire-weather conditions annual area burned doubled or tripled that average value (e.g. in 2009, about 44,800 ha; in 2007, about 34,400 ha). Wildfire impacts were driven by few large wildfires: in the study period, 264 events (about 0.6% of the total wildfires) exhibited an area burned larger than 100 ha, and accounted for 56% of the total area burned. The years that experienced the highest number of wildfires were 2014 and 2017 (about 4,800 and 3,700 fires, respectively). Most wildfires occurred in the May-September timeframe, corresponding to about 90% of the total area burned. Typically, near 95% of wildfires exhibit anthropogenic causes. Previous studies on historical fire regimes in Sardinia indicate that small fires are mainly generated from ignitions near coastal or in flat areas, particularly in the southern Campidano plain, while large wildfires are more often associated with high fuel load, complex topography and hilly conditions (Salis et al. 2021; see Supplementary Figs S1a, S2a).
Identification and delineation of WUI
WUI can be defined and mapped in different ways that usually depend on the scale of analysis. The two main WUI mapping methods are: (1) the point-based approach (Lampin-Maillet et al. 2010; Bar-Massada et al. 2013) and (2) the zonal approach (Radeloff et al. 2005). These methods differ in the geospatial data that are used to determine the locations of buildings throughout the landscape and to calculate the building density (Bar-Massada 2021). Overall, the interface area can be identified as the boundary strip between urban and wildland use or as the intermix region encompassing buildings and residential areas scattered within forest and rural lands. In this work, we defined WUI as the 100-m buffer zone around anthropic values; a 100-m buffer was adopted in previous works carried out in Sardinia and in other Mediterranean areas (Galiana-Martin et al. 2011; Bouillon et al. 2014; Sirca et al. 2017) and falls within the WUI boundary ranges (50–200 m) used in Italy (Modugno et al. 2016). For this purpose, we intersected the 2008 Sardinian Land Use and the Corine Land Cover shapefiles (EEA_2012 - Corine Land Cover 2012) and aggregated all anthropic land covers of ‘class 1’ (urban, industrial and commercial); starting from this layer, we produced a buffer of 100 m. With this analysis, we obtained a WUI area that included the rural, natural and forest lands around anthropic values. Sardinian WUI resulted in about 226,800 ha, and ranged from 22 to 10,700 ha. The biggest towns and the main villages located in the coastal areas presented more than 20% of the municipal territory characterised by WUI (Fig. 1b). The municipalities with the highest percentage of municipal area covered by WUI were in the southern part of Sardinia.
Socio-economic, vegetation, climatic and zootechnical dataset
We collected data on socio-economic, vegetation, climatic, and zootechnical variables, to create a comprehensive dataset at municipal and WUI levels: for each municipality and WUI, we created a dataset of 311 independent variables and two dependent variables (the area burned and the number of ignitions). Within this dataset, as pointed out in next lines, we removed 288 redundant variables and we focused our analysis considering 23 independent variables (Table S1).
Historical climate data for the period 1970–2000 were gathered from the WorldClim global climate database (Fick and Hijmans 2017) and aggregated as mean or total value at the municipal level. Vegetation layers were derived from the Corine Land Cover Map 2012 (EEA_2012 - Corine Land Cover 2012). The socio-economic information was retrieved from the 2011 data from ISTAT census (ISTAT; http://dati-censimentopopolazione.istat.it/Index.aspx#) (Table S1). For the zootechnical variables, we collected information about the total livestock farms and the number of livestock in each municipality from the National Veterinary Registry (Vet_info, Sistema Informativo Veterinario, Statistiche; https://www.vetinfo.it/j6_statistiche/#/new-list).
Due to effect of terrain heterogeneity and topography on wildfire occurrence and size, and the potential inherent effects on wildfires at WUI, we carried out our modelling analysis at the whole regional scale as well as splitting Sardinian municipalities into two groups based on the average altitude and on the elevation range: (1) the ‘Flat’ (F) group, which composed of 181 municipalities with mean altitude <350 m a.s.l. and elevation range <600 m; (2) the ‘Hilly and mountainous’ (H) group, which composed of 196 municipalities, with mean altitude ≥350 m a.s.l. and elevation range ≥600 m (Fig. S3).
Wildfire dataset
We used the 2005–2019 datasets of wildfire ignitions and area burned perimeters provided by the Sardinia Forest Service. The ignition data includes information about the location, date, municipality of origin, and wildfire size of each event. Wildfire perimeters contain spatial information on the area burned by wildfires larger than 0.1 ha.
To analyse wildfire activity inside Sardinian WUI in the study period, we intersected WUI areas with the abovementioned wildfire data. This allowed to create a dataset of wildfire ignitions (IPWUI) and area burned (ABWUI) within each WUI for all municipalities.
Data processing for machine learning modelling
Input data were first normalised to remove the impact of dimensions on models’ predictions, then randomly divided into training set (70%) and testing set (30%). Prediction models were produced by using the training sample, while the accuracy of predictions was evaluated using the testing set.
We performed multicollinearity diagnosis by Pearson’s coefficients, and data cleaning by identifying and removing outliers. Variables with a Pearson’s coefficient higher than 0.90 were excluded from the analysis. The Pearson’s coefficients were calculated at the regional level, and separately for the F and H municipal groups. Within the dataset, 288 redundant variables were removed due to collinearity; a set of 23 independent variables (Table S1) were used to develop the prediction models (correlation matrixes are in Figs S4, S5, and S6).
We used the caret package (Kuhn 2008) in R to build the predictive models. To assess and predict wildfire activity at WUI we used a set of ML models (Table S2). The performances of these models were analysed according to the Pearson’s correlation coefficient (r), the coefficient of determination (R2), and the error indicators Root Mean Squared Error (RMSE) and Mean Absolute Error (MAE).
In the model training phase, control parameters were set, including cross-validation with 10 repetitions to evaluate the model stability. The specific hyperparameters of each model were not objectively set, but a tuneLength parameter was set to five. The tuneLength parameter instructs the algorithm to try different predefined values for the main parameters and finally compares and uses those that attained the highest performances during the cross-validation.
Assessment of the variable importance
We then quantified the variable importance for the best ML models, for both area burned and wildfire ignitions.
The importance of each single variable was assessed through the ‘varImp’ function implemented in the caret package, which calculates and returns the relative importance of each variable with respect to that with the highest raw importance. This is calculated by keeping track of the combinations of variable weights that allow obtaining the highest prediction accuracy through the minimisation of a cost function, obtained from a modification of the Garson’s algorithm to deduce the influences of variables used in algorithms with neural networks (Garson 1991). In this algorithm, the weights associated with each variable are evaluated based on the variance explained by each one.
Mapping modelled wildfires at WUI
The estimated area burned and wildfire occurrence were displayed as maps that show the average annual wildfire patterns at WUIs. Predictive maps were created separately for both ABWUI and IPWUI at the regional scale and for the F and H municipal groups. Finally, we mapped the differences between estimated and observed data to support the spatial evaluation of the model predictions.
Results
Observed wildfire activity at WUI
During the study period, wildfires at WUI represented about 6.7% of the total area burned, and 27% of the total ignitions occurred in Sardinia. On average, about 818 wildfires occurred every year inside WUI, and approximately 995 ha of WUI annually burned. The highest ABWUI values were observed in 2009 and 2007 (about 2,500 and 1,900 ha burned, respectively), whereas the peaks of IPWUI were recorded in 2014 and 2017 (about 1,500 and 1,200, respectively). The annual average ABWUI at the municipal level ranged from 0 to 32.4 ha year−1 observed in north-eastern Sardinia (Fig. 2a). Overall, 214 Sardinian municipalities exhibited an annual ABWUI lower than 1 ha year−1, whereas only 18 municipalities, mainly located in the southern part of the island and in coastal areas, showed values higher than 12 ha year−1. In a few municipalities, particularly in southern Sardinia, more than 40% of the area burned at the municipal level was located inside WUI (Fig. S1b). The peaks of annual ABWUI were in 2009 and in 2007 in central Sardinia (388 and 276 ha burned, respectively). On average, the H group exhibited a slightly bigger annual area burned than the F group (513 vs 482 ha year−1).
(a) Observed annual area burned and (b) observed annual wildfire ignitions at WUI in Sardinia, Italy for the period 2005–2019.
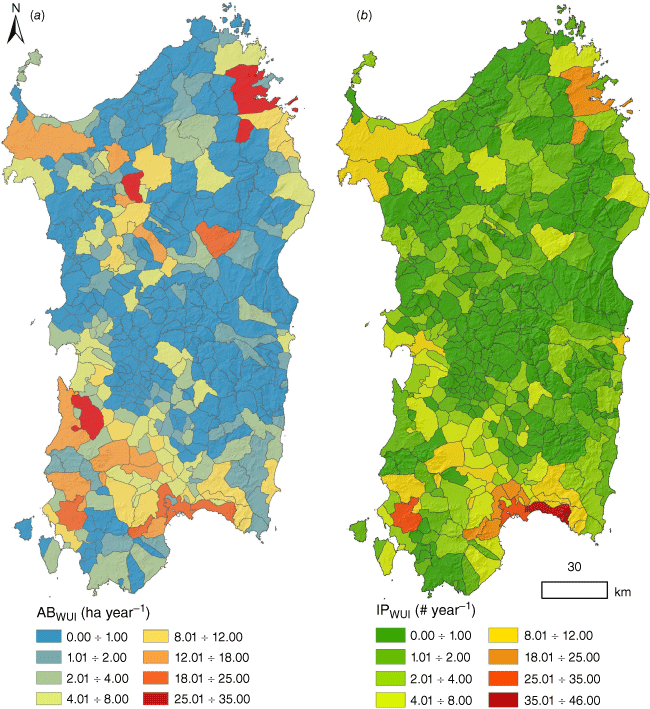
The average annual IPWUI was 2.2 # year−1, and ranged from 0 to 45.3 # year−1 in southern Sardinia (Fig. 2b). Annual IPWUI values higher than 15 were observed in southern Sardinia and in Olbia (north-eastern Sardinia), while most Sardinian municipalities exhibited IPWUI <2 # year−1. In 38 municipalities, mainly in southern and north-eastern Sardinia, more than 40% of wildfire ignitions at the municipal level occurred in WUI areas, with peaks higher than 70% observed in three municipalities of southern Sardinia (Fig. S2b). The F group municipalities showed annual IPWUI slightly higher than the H group (419 vs 399 # year−1). The highest annual IPWUI were observed during the years 2017 and 2014 in the southern municipalities.
Modelled area burned at WUI
Table 1 summarises the performance metrics provided by each ML model on the test dataset. At the regional level, the Random Forest (RF) model showed the best performances among all models. In fact, RF presented the highest Pearson’s correlation coefficient (r) (0.76), the second highest R2 value (0.58), the lowest RMSE (0.10), and the lowest MAE (0.06). The lowest r was showed by SVM-L, LASSO, and ENR models, whereas the highest RMSE values (0.25) were observed in OLS and BRR (for acronyms, see Table S2).
ABWUI | IPWUI | |||||||||
---|---|---|---|---|---|---|---|---|---|---|
r | R2 | RMSE | MAE | r | R2 | RMSE | MAE | |||
Model | Regional scale | |||||||||
OLS | 0.56 | 0.31 | 0.25 | 0.09 | 0.69 | 0.48 | 0.10 | 0.03 | ||
RF | 0.76 | 0.58 | 0.10 | 0.06 | 0.80 | 0.64 | 0.06 | 0.03 | ||
SVM-L | 0.54 | 0.30 | 0.21 | 0.08 | 0.67 | 0.44 | 0.09 | 0.03 | ||
SVM-R | 0.72 | 0.60 | 0.10 | 0.06 | 0.79 | 0.63 | 0.05 | 0.03 | ||
KNN | 0.68 | 0.46 | 0.12 | 0.07 | 0.63 | 0.40 | 0.07 | 0.04 | ||
LASSO | 0.54 | 0.29 | 0.23 | 0.09 | 0.67 | 0.46 | 0.10 | 0.03 | ||
ENR | 0.54 | 0.29 | 0.21 | 0.09 | 0.69 | 0.48 | 0.10 | 0.03 | ||
BRR | 0.55 | 0.30 | 0.25 | 0.09 | 0.70 | 0.49 | 0.09 | 0.03 | ||
Model | F municipal group | |||||||||
OLS | 0.43 | 0.18 | 0.16 | 0.09 | 0.64 | 0.41 | 0.08 | 0.06 | ||
RF | 0.58 | 0.34 | 0.14 | 0.08 | 0.80 | 0.64 | 0.06 | 0.03 | ||
SVM-L | 0.47 | 0.22 | 0.16 | 0.08 | 0.72 | 0.52 | 0.07 | 0.05 | ||
SVM-R | 0.60 | 0.36 | 0.14 | 0.08 | 0.71 | 0.50 | 0.07 | 0.05 | ||
KNN | 0.40 | 0.16 | 0.16 | 0.09 | 0.62 | 0.38 | 0.08 | 0.05 | ||
LASSO | 0.51 | 0.26 | 0.15 | 0.09 | 0.71 | 0.50 | 0.07 | 0.05 | ||
ENR | 0.52 | 0.27 | 0.15 | 0.09 | 0.83 | 0.69 | 0.09 | 0.06 | ||
BRR | 0.51 | 0.26 | 0.15 | 0.09 | 0.65 | 0.43 | 0.08 | 0.05 | ||
Model | H municipal group | |||||||||
OLS | 0.75 | 0.56 | 0.12 | 0.07 | 0.98 | 0.95 | 0.04 | 0.02 | ||
RF | 0.82 | 0.67 | 0.11 | 0.06 | 0.88 | 0.78 | 0.09 | 0.03 | ||
SVM-L | 0.72 | 0.52 | 0.13 | 0.06 | 0.98 | 0.96 | 0.05 | 0.03 | ||
SVM-R | 0.79 | 0.62 | 0.11 | 0.07 | 0.57 | 0.33 | 0.12 | 0.04 | ||
KNN | 0.69 | 0.47 | 0.13 | 0.07 | 0.90 | 0.81 | 0.10 | 0.03 | ||
LASSO | 0.76 | 0.58 | 0.12 | 0.06 | 0.97 | 0.94 | 0.07 | 0.03 | ||
ENR | 0.75 | 0.56 | 0.12 | 0.06 | 0.75 | 0.56 | 0.12 | 0.06 | ||
BRR | 0.74 | 0.55 | 0.12 | 0.06 | 0.97 | 0.95 | 0.05 | 0.03 |
Data refers to the three different analyses conducted at the regional scale and separately for F and H municipal groups. RMSE, Root Mean Squared Error; R2, coefficient of determination; MAE, Mean Absolute Error; r, Pearson’s correlation coefficient. Models are: OLS, Ordinary Least Square regression; RF, Random Forest; SVM-L, Support Vector Machine Kernel-Linear; SVM-R, Support Vector Machine Kernel-Radial; KNN, K-Nearest Neighbours; LASSO, Least Absolute Shrinkage and Selection Operator; ENR, Elastic Net; BRR, Bayesian Ridge Regression.
Focusing on flat municipalities, the best performances were observed for the SVM-R model which exhibited the highest r (0.6) and R2 (0.36) values, a low MAE (0.08) and the lowest RMSE (0.14). The worst performances were observed for the KNN model, which showed the lowest r and R2 (0.40 and 0.16, respectively) and high RMSE and MAE values (0.16 and 0.09, respectively).
As far as hilly WUI are concerned, RF was the best model: in fact, RF presented the highest r (0.82) and R2 (0.67), combined with the lowest RMSE and MAE (0.11 and 0.06, respectively). Again, KNN showed the lowest r (0.69) and R2 (0.47), and was the less performant model (Table 1).
Considering the above points, we selected RF for modelling ABWUI at the regional scale and the H group, and SVM-R for the F group.
According to RF at the regional scale, the most important variable that affected ABWUI was the WUI area covered by herbaceous vegetation (HERB) (100%) (Fig. 3a). On average, HERB cover 44% of the WUI area, varying from 39% in the H group to 50% in the F group; in 54 municipalities, it represents more than 60% of the WUI area, particularly in the western side of the island (Fig. S7a). Other important variables that affected ABWUI at the regional scale included some socio-economic variables, namely the net taxable income in the municipality (POPINC) (43%), the number of commuters (COMM) (35%), and the resident population living inside urban areas (POPURB) (34%). Within climatic variables, the most important one was the annual average wind speed (WS) (12%). The other variables showed values lower than 9% (Fig. 3a).
Variable importance for the best machine learning (ML) models used to assess ABWUI and IPWUI at the regional scale (a, d, respectively), and in F (b, e, respectively) and H (c, f, respectively) municipal groups. The best ML models for ABWUI were RF (a), SVM-R (b), and RF (c), while for IPWUI were RF (d), RF (e), and SVM-L (f). Variable groups: socio-economic (grey); vegetation (green); climatic (blue); zootechnical (red). The variable acronyms are in Table S1.
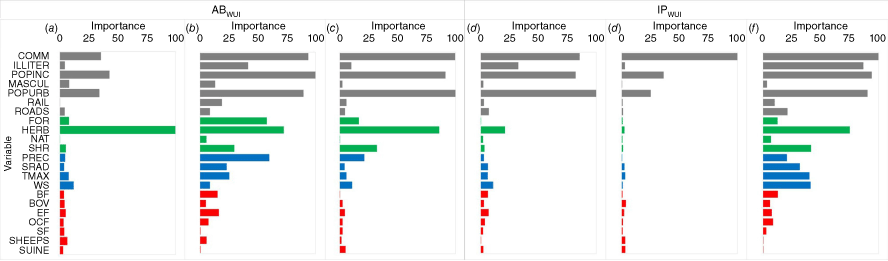
For F and H municipal groups, the most important variables influencing ABWUI were the socio-economic variables, particularly COMM (94% and 100%, respectively), POPINC (100 and 91% respectively), and POPURB (89% and 100%, respectively) (Fig. 3b, c). HERB was also important for both groups (73% and 86%, respectively). The WUI area covered by shrublands (SHR) showed similar importance values for F and H groups (30 and 32%, respectively), whereas the WUI area covered by forests (FOR) presented high value only for the F group (58%). Within climatic variables, the total annual municipal precipitation (PREC) exhibited a high importance value for the F group (60%). The annual average municipal maximum temperature (TMAX), the annual average municipal solar radiation (SRAD) and the number of illiterate people in the municipality (ILLIT) variables exhibited relatively high importance values only for the F group (25, 23, and 42%, respectively).
The observed annual ABWUI maps (Fig. 2a) were compared with the estimated annual ABWUI maps (Fig. 4a, b). The estimated annual values at regional scale ranged between 0.1 and 23.9 ha year−1: the highest ABWUI values were observed in some big municipalities in northern Sardinia and nearby Cagliari. The lowest ABWUI values were concentrated in the central part of Sardinia.
(a) Modelled annual Area Burned at WUI (ABWUI) (ha year−1) considering the analysis at the regional scale and (b) the separate analyses for H and F municipal groups in Sardinia, Italy.
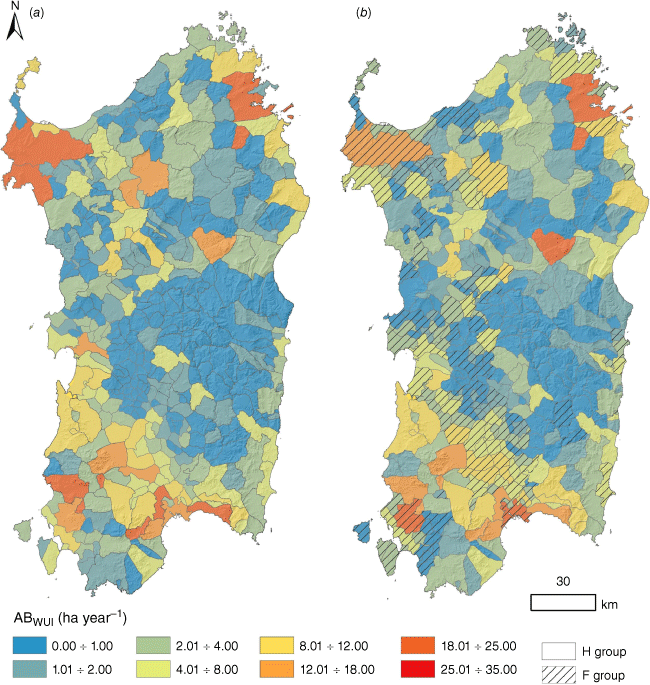
Overall, the map of the differences between modelledand observed data obtained by the analysis at the regional scale (Fig. 5a) exhibited good predictions for the entire island; the main underestimations of ABWUI were observed in a south-western municipality (−15 ha year−1) and in some other municipalities (−7 ÷ −9 ha year−1). The highest overestimations of ABWUI were found in the north-western municipality of Alghero (15 ha year−1).
(a) Differences in annual Area Burned at WUI (ABWUI) (ha year−1) between modelled and observed data considering the analysis at the regional scale, and (b) the separate analyses for the H and F municipal groups in Sardinia, Italy.
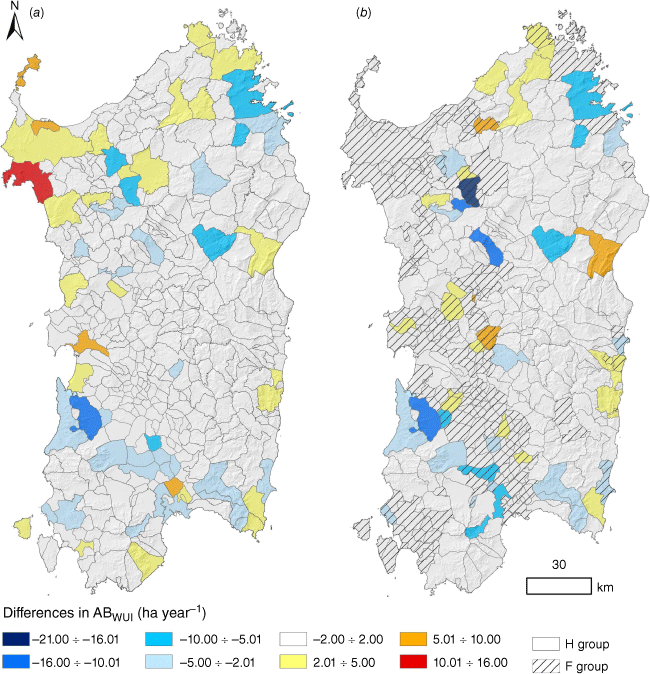
The map of the annual average of the modelled ABWUI conducted separately for the two municipal groups (Fig. 4b) exhibited a range between 0.13 and 23.5 ha year−1. The highest estimated values were in the north-east and the south-west, while the lowest ABWUI values were located in central Sardinia.
Fig. 5b shows the difference values obtained between the model predictions for the F and H groups and the observed annual ABWUI. The map exhibited difference values close to 0 for several municipalities. Overestimations were limited to few municipalities and were lower than 6 ha year−1; the highest underestimations were presented by some municipalities located in central (−20.37 ha year−1) and south-western Sardinia (−15 ha year−1).
Overall, both analyses exhibited good predictions of ABWUI, showing many of the municipalities with limited differences compared to the observed values. The predictions obtained considering the H and F municipal groups exhibited a higher predictive power than that at the regional scale. For both analyses, the highest underestimations of ABWUI for both the analyses were observed in the municipalities affected by the largest wildfires that occurred during the study period (i.e. Mores, which was impacted by the 10,500-ha Bonorva wildfire occurred in July 2009; Nuoro, which was affected by the 9000-ha Orani wildfire in July 2007). In general terms, the models underpredicted ABWUI in those municipalities where the observed ABWUI was high. Conversely, the overestimation errors were mostly slight, apart from the analysis at the regional scale which overestimated ABWUI in Alghero (north-western Sardinia).
Modelled wildfire ignitions at WUI
Performance metrics for the analysis of wildfire ignitions at regional scale showed the RF as the best model (Table 1). RF presented the highest r coefficient (0.80) and the highest R2 value (0.64). The lowest values of R2 and r were observed for the KNN model (0.40 and 0.63, respectively). OLS, LASSO, and ENR models presented the highest RMSE value (0.10), whereas the lowest RMSE values were exhibited by SVM-R and RF models. Although the lowest value of RMSE was observed for the SVM-R model, RF showed both the highest R2 value and the highest r, which overall made RF the model with the best performances (Table 1).
The analysis of the F group confirmed the skills of the RF model compared to the other models. In fact, RF showed the lowest RMSE (0.06) and MAE (0.03) and the second-highest values of r and R2 (0.80 and 0.64, respectively). The ENR model exhibited the highest r and R2 values (0.83 and 0.69), but the highest RMSE and MAE values (0.09 and 0.06, respectively).
For H group, the SVM-L model revealed the highest R2 and r values (0.96 and 0.98, respectively), and RMSE and MAE values (0.05 and 0.03, respectively) among the lowest observed.
According to the analysis of the model performances, we selected RF for modelling IPWUI at the regional scale and for the F group, and SVM-L for the H group.
At the regional scale, the socio-economic variables showed the highest influence on IPWUI (Fig. 3d), particularly POPURB (100%), COMM (86%), POPINC (82%), and ILLITER (33%). HERB was the fifth variable in order of importance (21%).
The separate analysis conducted by RF for the F municipal group and by SVM-L for the H group showed different outcomes (Fig. 3e, f). For both groups, the socio-economic variables represented the most influencing variables, particularly COMM (100% for both), POPINC (36 and 94%, for F and H, respectively), and POPURB (25 and 91%, respectively) (Fig. 3e, f). However, the outcomes between the municipal groups differed when considering other variables, especially for the F group, where some of them exhibited values lower than 5%. Several variables contributed to influencing wildfire occurrence inside WUI of the H group, such as the socio-economic variables represented by ILLITER (87%) and ROADS (21%), vegetation (HERB and SHR (75% and 42%, respectively)), and some climatic variables (WS (41%), TMAX (40%), SRAD (32%), and PREC (21%)) (Fig. 3f).
The map of observed annual IPWUI (Fig. 2b) was compared with the maps of the estimated values (Fig. 6a, b).
(a) Modelled annual wildfire ignitions at WUI (IPWUI) (# year−1) considering the analysis at the regional scale and (b) the separate analyses for the H and F municipal groups in Sardinia, Italy.
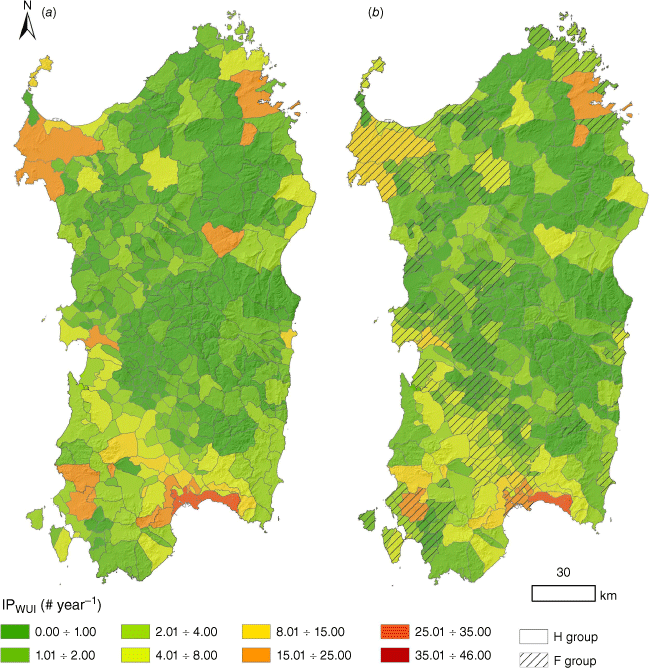
The map of the average annual estimated IPWUI at the regional scale (Fig. 6a) displayed a range between 0.04 and 30.7 # year−1. In general terms, the highest values for both the analyses were predicted for the main towns of the island. The comparison between observed and modelled IPWUI at the regional scale exhibited a high predictive power, with overestimations in a few municipalities located in central (13.6 # year−1) and northern Sardinia (up to 12.9 # year−1) (Fig. 7a). In contrast, the main underestimations were in southern Sardinia (−14.5 # year−1).
(a) Differences in annual wildfire ignitions at WUI (IPWUI) (# year−1) between modelled and observed data considering the analysis at the regional scale, and (b) the separate analyses for the H and F municipal groups in Sardinia, Italy.
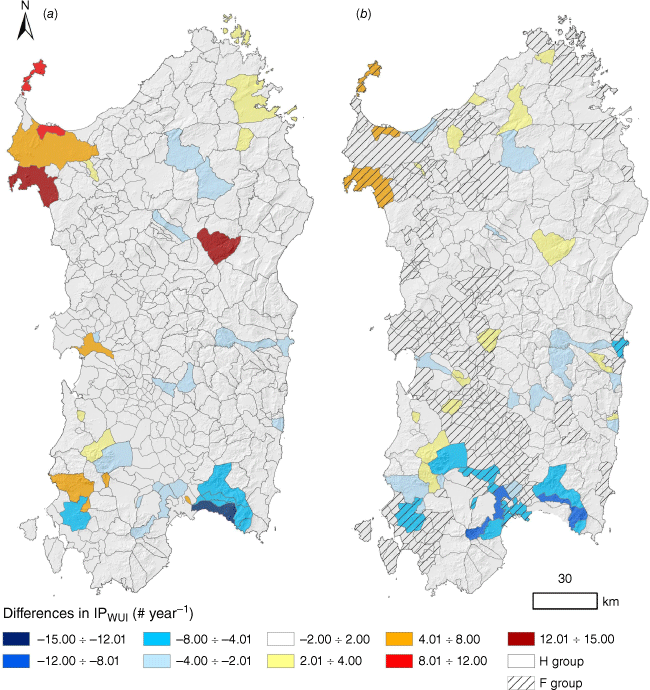
Regarding the separated analysis for H and F municipal groups (Fig. 6b), the map of the annual average estimated IPWUI exhibited a range from 0 to 30.5 # year−1. The highest values were in the south (30.5 # year−1). IPWUI values close to 0 were estimated for several municipalities located in central Sardinia. The differences between observed and modelled data for the two municipal groups F and H showed a better agreement than for the analysis at the regional scale, notably for the municipalities of the F group (Fig. 7b).
For both analyses, the underestimations for IPWUI were concentrated in the municipalities located in southern Sardinia, that during the study period exhibited the highest number of wildfire ignitions inside the WUI, or in the municipalities that presented the highest values of POPINC, POPURB, and COMM (Fig. S7b–d). Conversely, the overpredictions were more significant for the analysis carried out at the regional scale, and were mainly observed in the most populated municipalities of central and northern Sardinia.
Discussion
Modelling approach
Although several wildfire prediction models are available worldwide (Jain et al. 2020), they cannot be effective enough to be used for large scales or in different geographical conditions without local calibrations to improve the accuracy of estimations (Sharma et al. 2022). In this work, different regression models and machine learning models were developed to identify the drivers of area burned and wildfire ignitions inside WUI of Sardinia, Italy, using as input a comprehensive standardised dataset of socio-economic, vegetation, climatic, and zootechnical explanatory variables at the municipal level. The results show that, among the ML models used in this work, RF, SVM-L, and SVM-R outperformed the others, exhibiting better performances according to the Pearson’s correlation coefficient and the error indicators. This finding is consistent with the results from other studies on wildfire regime modelling which highlighted that ML models were more competitive than traditional methods like regression models in terms of prediction accuracy (Rodrigues et al. 2014; Milanović et al. 2020; Gao et al. 2024).
The high predictive potential of SVM models can be explained by their power to manage dynamic relationships and complex data, showing also to be an advantageous algorithm for binary classification problems (Pham et al. 2020; Sharma et al. 2022). Good SVM prediction performances were evidenced for susceptibility mapping of forest fires in western Serbia, India, and Himalaya (Gigović et al. 2019; Sharma et al. 2022; Rihan et al. 2023) and for predicting wildfire occurrence probability in China (Gao et al. 2024). Nevertheless, some authors considered SVM less adequate for wildfire occurrence predictions because its calibration and optimisation are considered more time-consuming with respect to other models such as RF (Bar-Massada et al. 2012; Rodrigues and De la Riva 2014).
The good performance of RF can be explained by its high tolerance for data outliers and the capability to handle categorical variables, considering the interactions between the variables (Pourtaghi et al. 2016; Tonini et al. 2020; Achu et al. 2021). RF showed good predictive performances in several wildfire modelling studies. For example, exhibiting a better overall predictive power than linear regression models for the identification of the main explanatory variables for wildfire occurrence and probability mapping in Serbia (Milanović et al. 2020), or outperforming other models in elaborating susceptibility maps in Liguria region (Tonini et al. 2020) and western Serbia (Gigović et al. 2019). In other works, RF outperformed Multiple Linear Regression for the analysis of the spatial patterns of wildfire occurrence in Mediterranean Europe, displaying its ability in the assessment of fire-related phenomena at broad scale (Oliveira et al. 2012; Achu et al. 2021).
Drivers of wildfire activity at WUI
We analysed the role played by several drivers in determining wildfire activity in Sardinian WUI in the study period. By investigating the spatial relationship among wildfires at WUI and many drivers including socio-economic, vegetation, climatic, and zootechnic factors, we were able to point out that given factors can impact differently local-scale wildfire activity according to specific territorial conditions and heterogeneous characteristics of local communities. In this sense, our study aims at identifying (and ranking the importance of) relevant forces influencing wildfires at WUI in Sardinia, and at evidencing how much given factors play a potential role in wildfire activity at the WUI level.
Regarding the role of socio-economic factors, previous works pointed out that wildfire regime is influenced by the socio-economic context at the local (Mancini et al. 2018a) and broader scales (Jain et al. 2022; Jones et al. 2022; Ochoa et al. 2024).
In our work, the net taxable income in the municipality (POPINC) was identified as one of the main drivers of the area burned and wildfire ignitions at WUI. For ABWUI, this variable exhibited a strong importance for both municipality groups, and a little lower role at the regional level, while for IPWUI was a key driver at the regional level and for the H municipal group. Low financial resources and budgets of landowners and local population can affect their possibility to support fuel management activities within WUI and in neighbouring lands. Furthermore, public investments in wildfire prevention and forest management may vary based on the municipal availability of financial resources, which could be limited or even insufficient in remote and low-income areas.
The number of illiterate people in the municipality (ILLITER) exhibited a strong influence on IPWUI. Many authors reported a correlation between wildfire ignitions and educational level; being better informed, people with high levels of education feel confident on how to perceive and act in wildfire-prone areas or situations (Oliveira et al. 2020a), are more able to comprehend and comply with prevention and safeness regulations (Oliveira et al. 2020b), are likely to adopt wildfire mitigation measures (Gan et al. 2015), and show more awareness of and sensitivity towards wildfires, confirming higher caution and care when dealing with them (e.g. renewal of pastures, burning of stubble, releasing flammable waste) (Mancini et al. 2018a; Elia et al. 2022). Moreover, the educational level might be associated to other factors that indirectly influence IPWUI, such as dwelling locations and conditions, or budget available for fuel management nearby WUI.
Resident population living inside urban areas (POPURB) also played a considerable role in ABWUI and IPWUI in the study area. Over the past decades, the migration from the countryside towards the more populated areas, combined with the increase in the number of workers employed in the tertiary sector rather than in the primary sector, traditionally more involved in the control and management of the territory, has led to a progressive abandonment and changes in the landscape structure (Tonini et al. 2018; Ascoli et al. 2021; Salis et al. 2022). In addition, demographic shifts from rural to urban areas also influenced wildfire occurrence and behaviour (Koutsias et al. 2010; Salis et al. 2019; Spadoni et al. 2023). Furthermore, the presence of residential properties intermingled with forested areas can further increase the probability of wildfire activity nearby WUI with respect to ‘classic interfaces’ (Galiana-Martin et al. 2011; Ganteaume et al. 2013); fragmented distributions of residential properties can also lead to more difficulties in managing fuels and lowering wildfire risk.
Another socio-economic variable that influenced wildfire activity at WUI was the number of commuters (COMM) who travel for work, study, or other purposes. Overall, the more commuters are present, the more area is burned inside WUI and the more frequent are wildfire ignitions at WUI. COMM can therefore likely increase the probability of anthropic ignitions (either accidental or intentional), which in the Mediterranean basin represent most of the total wildfire ignitions. These results support the findings of previous works which selected the ratio of commuting workers in total population as one of the most relevant predictors influencing positively the average fire size in Italy (Mancini et al. 2018a). Our abovementioned findings about the influence of socio-economic variables on ABWUI agree with previous works that found a strong influence of the human presence and anthropic activities on the area burned, particularly at regional and local scale (Aldersley et al. 2011; Curt et al. 2016; Mansuy et al. 2019), and an increasing influence on wildfire occurrence (Chas-Amil et al. 2015; Elia et al. 2019, 2020; Milanović et al. 2020).
Focusing on vegetation drivers, the WUI area covered by herbaceous vegetation (HERB), which includes grasslands, agricultural areas or pastures, was the most influencing driver of ABWUI at the regional level, and played also an important role in the analyses of the separate municipal groups. This result was confirmed by comparing the map of HERB distribution (Fig. S7a) with the map of observed annual ABWUI (ha year−1) for the period 2005–2019 (Fig. 2a): for both variables, the highest values were distributed on the western side of the island, particularly in southern Sardinia. Our results evidence that the relation between the presence of herbaceous vegetation and ABWUI is more evident for the H group. Differently, the role of vegetation on IPWUI is more limited, exhibiting a strong influence of HERB and of the WUI area covered by shrublands (SHR) only for the H group. The above findings are consistent with previous works carried out in Sardinia and other European countries, which highlighted that herbaceous vegetation is often linked to large events and, especially when its distribution is combined with a complex topography and strong winds, is characterised by high wildfire transmission potential (Ager et al. 2014; Alcasena et al. 2017; Elia et al. 2019; Salis et al. 2021). In this regard, agro-pastoral land abandonment and the inherent increases in dead fine fuel load and continuity should be carefully considered in future years, as the probability of large wildfire occurrence able to spread towards WUI or forest areas could be exacerbated (Pais et al. 2020; Rodrigues et al. 2022; Salis et al. 2022).
At the regional level, climatic variables did not exhibit a strong influence on ABWUI and IPWUI. For the separated analyses, the annual average municipal maximum temperature (TMAX), the annual average municipal solar radiation (SRAD) and the total annual municipal precipitation (PREC) played a role in determining ABWUI, more in particular for the F group. Differently, the annual average municipal wind speed (WS), TMAX, SRAD, and PREC influenced IPWUI for the H group. According to this result, the Sardinian hilly areas, which are mostly located in the inner zones of the island, are characterised by limited levels of fuel management, particularly in forest and marginal pastures, and high dryness conditions in the summer season, are therefore more exposed to wildfire ignitions and large wildfire spread; the effect of elevation on wildfire occurrence was also observed in eastern Europe (Milanović et al. 2020). Other authors highlighted climatic factors as the most influencing variables of area burned at regional, continental, and global scales (Aldersley et al. 2011; Curt et al. 2015; Jain et al. 2020, Rodriguez-Jimenez et al. 2023). Grünig et al. (2023) found a strong correlation between the increasing aridity and the largest fires in Europe, while Elia et al. 2020 highlighted the relevant role played by climate variables on wildfire regime in insular regions of Italy.
Lastly, any of the variables of the zootechnical group showed significant effects on ABWUI and IPWUI.
As far as the spatial model predictions of ABWUI and IPWUI are concerned, the results were overall promising for both analyses (regional scale vs municipal groups), with limited differences between observed and estimated values. In general terms, the analysis carried out considering F and H municipal groups performed slightly better than the regional scale analysis. We evidenced underpredictive issues of both models in the municipalities characterised by the highest values of ABWUI. Regarding IPWUI, we noticed an underestimation of both models in southern Sardinia, particularly in those zones characterised by high municipal areas occupied by WUI; on the contrary, overpredictions were mainly concentrated in the most populated municipalities of central and northern Sardinia for the analysis carried out at the regional scale.
The proposed approach in this study has a number of intrinsic limits. For instance, we attributed a set of driving factors and variables for each municipal area in Sardinia, but this did not allow to take into account the spatial complexity of WUI conditions, particularly in some touristic coastal areas or main towns and suburbs. Moreover, when determining WUI, we did not take into account isolated houses or groups of houses that were not captured by the input layers used, and this could have influenced our final results. Furthermore, an obstacle for the adoption of similar ML methods to model wildfire activity is the perceived lack of interpretability of such methods, which are often considered to be black box models (Jain et al. 2020).
Conclusions
In this study, we modelled wildfire activity in WUI areas of Sardinia, Italy by applying a set of predictive models including machine learning and multivariate regression models. We investigated how socio-economic, vegetation, climatic and zootechnical drivers influenced wildfire patterns, ignitions and area burned at WUI, by using a multi-year comprehensive dataset at the municipal and WUI level for the study period 2005–2019. Among the set of candidate models, Random Forest, Support Vector Machine Kernel linear, and Support Vector Machine Kernel Radial presented the best performances for identifying wildfire patterns at WUI in a complex and heterogeneous landscape such as the Sardinia region, and were therefore selected for the analysis and mapping of predicted wildfire ignitions and area burned in the study area. The outcomes of the analysis at the regional scale showed that socio-economic variables and the distribution of herbaceous vegetation strongly affected wildfire ignitions and the area burned inside the Sardinian WUI, both at low and high altitudes.
Our results emphasise the need to consider multiple aspects and factors that can contribute to the origin and propagation of wildfires. The results of this study enrich the knowledge of the role played by the main drivers of wildfires at WUI in a fire-prone Mediterranean area such as Sardinia, underlining the importance of the socio-economic factors that condition wildfire patterns. The methodology presented in this work is replicable and can be applied to other areas. The methods and findings of this work can be used to inform and guide wildfire managers and policymakers in improving, planning, and optimising prevention strategies targeting the reduction of wildfire risk at WUI.
Data availability
The data used in this study are available upon reasonable request from the authors.
Conflicts of interest
The authors declare that they have no known competing financial interests or personal relationships that could have appeared to influence the work reported in this paper.
Declaration of funding
This work was funded by: the FirEUrisk (Grant agreement ID: 101003890) project, funded by the EU under the ‘H2020-EU.3.5. – SOCIETAL CHALLENGES – Climate action, Environment, Resource Efficiency and Raw Materials’ Programme; the MED-Star (grant no. E88H19000120007), Med-Foreste (grant no. B85I1900010007) and Med-Coopfire (grant no. B81I1900010007) projects, supported by the EU under the cross-border ‘Programma Italia–Francia Marittimo’ 2014–2020; the CRITERIA Project (grant no. B53D23029500001), funded by the Italian Ministry of Education, University and Research (MIUR) under the EU Next Generation Program (PNRR – PRIN); the FIRE-ADAPT Project (grant no. 101086416), funded by the EU Marie Skłodowska-Curie Action; the FIREPATHS project (PID2020-116556RA-I00), funded by the Spanish Ministry of Science and Innovation.
Acknowledgements
The authors thank the Sardinia Forest Service for providing wildfire data. The authors also thank the reviewers and the Associate Editor for their helpful comments to improve the quality of the manuscript.
References
Achu A, Thomas J, Aju CD, Gopinath G, Kumar S, Reghunath R (2021) Machine-learning modelling of fire susceptibility in a forest-agriculture mosaic landscape of southern India. Ecological Informatics 64, 101348.
| Crossref | Google Scholar |
Ager AA, Preisler HK, Arca B, Spano D, Salis M (2014) Wildfire risk estimation in the Mediterranean area. Environmetrics 25(6), 384-396.
| Crossref | Google Scholar |
Alcasena FJ, Salis M, Ager AA, Castell R, Vega-García C (2017) Assessing wildland fire risk transmission to communities in northern Spain. Forests 8(2), 30.
| Crossref | Google Scholar |
Aldersley A, Murray SJ, Cornell SE (2011) Global and regional analysis of climate and human drivers of wildfire. Science of The Total Environment 409(18), 3472-3481.
| Crossref | Google Scholar | PubMed |
Ascoli D, Moris JV, Marchetti M, Sallustio L (2021) Land use change towards forests and wooded land correlates with large and frequent wildfires in Italy. Annals of Silvicultural Research 46(2), 177-188.
| Crossref | Google Scholar |
Badia A, Serra P, Modugno S (2011) Identifying dynamics of fire ignition probabilities in two representative mediterranean wildland-urban interface areas. Applied Geography 31, 930-940.
| Crossref | Google Scholar |
Bar-Massada A (2021) A comparative analysis of two major approaches for mapping the wildland-urban interface: a case study in California. Land 10(7), 679.
| Crossref | Google Scholar |
Bar-Massada A, Syphard AD, Stewart S, Radeloff VC (2012) Wildfire ignition- distribution modelling: a comparative study in the Hurone-Manistee National Forest, Michigan, USA. International Journal of Wildland Fire 22(2), 174-183.
| Crossref | Google Scholar |
Bar-Massada A, Stewart SI, Hammer RB, Mockrin MH, Radeloff VC (2013) Using Structure Locations as a Basis for Mapping the Wildland Urban Interface. Journal of Environmental Management 128, 540-547.
| Crossref | Google Scholar | PubMed |
Bar-Massada A, Alcasena F, Schug F, Radeloff VC (2023) The wildland–urban interface in Europe: spatial patterns and associations with socioeconomic and demographic variables. Landscape and Urban Planning 235, 104759.
| Crossref | Google Scholar |
Bot K, Borges JG (2022) A systematic review of applications of machine learning techniques for wildfire management decision support. Inventions 7(1), 15.
| Crossref | Google Scholar |
Bouillon C, Fernandez Ramiro MM, Sirca C, Fierro Garcia B, Casula F, Vila B, Long Fournel M, Pellizzaro G, Arca B, Tedim F, Trebini F, Derudas A, Canè S (2014) A Tool for Mapping Rural-Urban Interfaces on Different Scales. In ‘Advances in Forest Fire Research, Chapter 3 – Fire Management’. (Ed. DX Viegas) pp. 611–625. (University of Coimbra) 10.14195/978-989-26-0884-6_70
Chas-Amil ML, Prestemon JP, McClean CJ, Touza J (2015) Human-ignited wildfire patterns and responses to policy shifts. Applied Geography 56, 164-176.
| Crossref | Google Scholar |
Curt T, Borgniet L, Ibanez T, Moron V, Hély C (2015) Understanding fire patterns and fire drivers for setting a sustainable management policy of the New-Caledonian biodiversity hotspot. Forest Ecology and Management 337, 48-60.
| Crossref | Google Scholar |
Curt T, Fréjaville T, Lahaye S (2016) Modelling the spatial patterns of ignition causes and fire regime features in southern France: implications for fire prevention policy. International Journal of Wildland Fire 25(7), 785-796.
| Crossref | Google Scholar |
Del Giudice L, Arca B, Scarpa C, Pellizzaro G, Duce P, Salis M (2021) The wildland-anthropic interface raster data of the Italy–France maritime cooperation area (Sardinia, Corsica, Tuscany, Liguria, and Provence-Alpes-Côte d’Azur). Data in Brief 38, 107355.
| Crossref | Google Scholar | PubMed |
D’Este M, Giannico V, Lafortezza R, Sanesi G, Elia M (2021) The Wildland-Urban Interface Map of Italy: A Nationwide Dataset for Wildfire Risk Management. Data in Brief 38, 107427.
| Crossref | Google Scholar | PubMed |
EEA_2012 - Corine Land Cover (2012) Available at https://www.eea.europa.eu/data-and-maps/data/clc-2012-raster/link [accessed 25 March 2024]
Elia M, Giannico V, Lafortezza R, Sanesi G (2019) Modeling Fire Ignition Patterns in Mediterranean Urban Interfaces. Stochastic Environmental Research and Risk Assessment 33, 169-181.
| Crossref | Google Scholar |
Elia M, D’Este M, Ascoli D, Giannico V, Spano G, Ganga A, Colangelo G, Lafortezza R, Sanesi G (2020) Estimating the probability of wildfire occurrence in Mediterranean landscapes using artificial neural networks. Environmental Impact Assessment Review 85, 106474.
| Crossref | Google Scholar |
Elia M, Giannico V, Ascoli D, Argañaraz JP, D’Este M, Spano G, Lafortezza R, Sanesi G (2022) Uncovering current pyroregions in Italy using wildfire metrics. Ecological Processes 11, 15.
| Crossref | Google Scholar |
Fernández-García V, Beltrán-Marcos D, Calvo L (2023) Building patterns and fuel features drive wildfire severity in wildland-urban interfaces in southern Europe. Landscape and Urban Planning 3, 231.
| Crossref | Google Scholar |
Fick SE, Hijmans RJ (2017) WorldClim 2: new 1‐km spatial resolution climate surfaces for global land areas. International Journal of Climatology 37(12), 4302-4315.
| Crossref | Google Scholar |
Galiana-Martin L, Herrero G, Solana J (2011) A wildland-urban interface typology for forest fire risk management in Mediterranean areas. Landscape Research 36, 151-171.
| Crossref | Google Scholar |
Gan J, Jarrett A, Gaither CJ (2015) Landowner response to wildfire risk: adaptation, mitigation or doing nothing. Journal of Environmental Management 159, 186-191.
| Crossref | Google Scholar | PubMed |
Ganteaume A, Camia A, Jappiot M, San-Miguel-Ayanz J, Long-Fournel M, Lampin C (2013) A review of the main driving factors of forest fire ignition over Europe. Environmental Management 51, 651-662.
| Crossref | Google Scholar | PubMed |
Ganteaume A, Barbero R, Jappiot M, Maillé E (2021) Understanding future changes to fires in southern Europe and their impacts on the wildland-urban interface. Journal of Safety Science and Resilience 2, 20-29.
| Crossref | Google Scholar |
Gao B, Shan Y, Liu X, Yin S, Yu B, Cui C, Cao L (2024) Prediction and driving factors of forest fire occurrence in Jilin Province, China. Journal of Forestry Research 35(1), 21.
| Crossref | Google Scholar |
Garson GD (1991) Interpreting Neural-Network Connection Weights. AI Expert 6(4), 46-51.
| Google Scholar |
Gigović L, Pourghasemi HR, Drobnjak S, Bai S (2019) Testing a new ensemble model based on SVM and random forest in forest fire susceptibility assessment and its mapping in Serbia’s Tara National Park. Forests 10(5), 408.
| Crossref | Google Scholar |
Grünig M, Seidl R, Senf C (2023) Increasing aridity causes larger and more severe forest fires across Europe. Global Change Biology 29(6), 1648-1659.
| Crossref | Google Scholar | PubMed |
Jain P, Coogan SCP, Subramanian SG, Crowley M, Taylor S, Flannigan MD (2020) A Review of Machine Learning Applications in Wildfire Science and Management. Environmental Reviews 505, 478-505.
| Crossref | Google Scholar |
Jain P, Castellanos-Acuna D, Coogan SC, Abatzoglou JT, Flannigan MD (2022) Observed increases in extreme fire weather driven by atmospheric humidity and temperature. Nature Climate Change 12(1), 63-70.
| Crossref | Google Scholar |
Jones MW, Abatzoglou JT, Veraverbeke S, Andela N, Lasslop G, Forkel M, Smith ALP, Burton C, Betts RA, van der Werf GR, Sitch S, Canadell JG, Santín C, Kolden C, Doerr SH, Le Quéré C (2022) Global and regional trends and drivers of fire under climate change. Reviews of Geophysics 60(3), e2020RG000726.
| Crossref | Google Scholar |
Koutsias N, Martínez-Fernández J, Allgöwer B (2010) Do factors causing wildfires vary in space? Evidence from geographically weighted regression. GIScience & Remote Sensing 47(2), 221-240.
| Crossref | Google Scholar |
Kuhn M (2008) Building predictive models in R using the caret package. Journal of Statistical Software 28, 1-26.
| Crossref | Google Scholar |
Lampin-Maillet C, Jappiot M, Long M, Bouillon C, Morge D, Ferrier JP (2010) Mapping Wildland-Urban Interfaces at Large Scales Integrating Housing Density and Vegetation Aggregation for Fire Prevention in the South of France. Journal of Environmental Management 91, 732-741.
| Crossref | Google Scholar | PubMed |
Mancini LD, Corona P, Salvati L (2018a) Ranking the Importance of Wildfires’ Human Drivers through a Multi-Model Regression Approach. Environmental Impact Assessment Review 72, 177-186.
| Crossref | Google Scholar |
Mancini LD, Elia M, Barbati A, Salvati L, Corona P, Lafortezza R, Sanesi G (2018b) Are wildfires knocking on the built-up areas door. Forests 9, 234.
| Crossref | Google Scholar |
Mansuy N, Miller C, Parisien MA, Parks SA, Batllori E, Moritz MA (2019) Contrasting human influences and macro-environmental factors on fire activity inside and outside protected areas of North America. Environmental Research Letter 14(6), 064007.
| Crossref | Google Scholar |
Milanović S, Marković N, Pamučar D, Gigović L, Kostić P, Milanović SD (2020) Forest fire probability mapping in eastern Serbia: logistic regression versus random forest method. Forests 12(1), 5.
| Crossref | Google Scholar |
Modugno S, Balzter H, Cole B, Borrelli P (2016) Mapping Regional Patterns of Large Forest Fires in Wildland–Urban Interface Areas in Europe. Journal of Environmental Management 172, 112-126.
| Crossref | Google Scholar | PubMed |
Molina-Terrén DM, Xanthopoulos G, Diakakis M, Ribeiro L, Caballero D, Delogu GM, Viegas DX, Silva CA, Cardil A (2019) Analysis of Forest Fire Fatalities in Southern Europe: Spain, Portugal, Greece and Sardinia (Italy). International Journal of Wildland Fire 28(2), 85-98.
| Crossref | Google Scholar |
Ochoa C, Bar-Massada A, Chuvieco E (2024) A European-scale analysis reveals the complex roles of anthropogenic and climatic factors in driving the initiation of large wildfires. Science of The Total Environment 917, 170443.
| Crossref | Google Scholar | PubMed |
Oliveira S, Oehler F, San-Miguel-Ayanz J, Camia A, Pereira JMC (2012) Modeling Spatial Patterns of Fire Occurrence in Mediterranean Europe Using Multiple Regression and Random Forest. Forest Ecology and Management 275, 117-129.
| Crossref | Google Scholar |
Oliveira S, Moreira F, Boca R, San-Miguel-Ayanz J, Pereira JMC (2013) Assessment of Fire Selectivity in Relation to Land Cover and Topography: A Comparison between Southern European Countries. International Journal of Wildland Fire 23, 620-630.
| Crossref | Google Scholar |
Oliveira R, Oliveira S, Zêzere JL, Viegas DX (2020a) Uncovering the perception regarding wildfires of residents with different characteristics. International Journal of Disaster Risk Reduction 43, 101370.
| Crossref | Google Scholar |
Oliveira S, Gonçalves A, Benali A, Sá A, Zêzere JL, Pereira JM (2020b) Assessing risk and prioritizing safety interventions in human settlements affected by large wildfires. Forests 11, 859.
| Crossref | Google Scholar |
Pais S, Aquilué N, Campos J, Sil Â, Marcos B, Martínez-Freiría F, Domínguez J, Brotons L, Honrado JP, Regos A (2020) Mountain farmland protection and fire-smart management jointly reduce fire hazard and enhance biodiversity and carbon sequestration. Ecosystem Services 44, 101143.
| Crossref | Google Scholar |
Pham BT, Jaafari A, Avand M, Al-Ansari N, Dinh Du T, Yen HPH, Phong TV, Nguyen DH, Le HV, Mafi-Gholami D, Prakash I (2020) Performance evaluation of machine learning methods for forest fire modeling and prediction. Symmetry 12(6), 1022.
| Crossref | Google Scholar |
Pourtaghi ZS, Pourghasemi HR, Aretano R, Semeraro T (2016) Investigation of general indicators influencing on forest fire and its susceptibility modeling using different data mining techniques. Ecological Indicators 64, 72-84.
| Crossref | Google Scholar |
Radeloff VC, Hammer RB, Stewart SI, Fried JS, Holcomb SS, McKeefry JF (2005) The Wildland-Urban Interface in the United States. Ecological Applications 15, 799-805.
| Crossref | Google Scholar |
Ribeiro LM, Rodrigues A, Lucas D, Viegas DX (2020) The Impact on Structures of the Pedrógão Grande Fire Complex in June 2017 (Portugal). Fire 3, 57.
| Crossref | Google Scholar |
Rihan M, Bindajam AA, Talukdar S, Naikoo MW, Mallick J, Rahman A (2023) Forest fire susceptibility mapping with sensitivity and uncertainty analysis using machine learning and deep learning algorithms. Advances in Space Research 72(2), 426-443.
| Crossref | Google Scholar |
Rodrigues M, De la Riva J (2014) An insight into machine-learning algorithms to model human-caused wildfire occurrence. Environmental Modelling & Software 57, 192-201.
| Crossref | Google Scholar |
Rodrigues M, De la Riva J, Fotheringham S (2014) Modeling the Spatial Variation of the Explanatory Factors of Human-Caused Wildfires in Spain Using Geographically Weighted Logistic Regression. Applied Geography 48, 52-63.
| Crossref | Google Scholar |
Rodrigues M, Jiménez-Ruano A, Peña-Angulo D, De la Riva J (2018) A Comprehensive Spatial-Temporal Analysis of Driving Factors of Human-Caused Wildfires in Spain Using Geographically Weighted Logistic Regression. Journal of Environmental Management 225, 177-192.
| Crossref | Google Scholar | PubMed |
Rodrigues M, Zúñiga-Antón M, Alcasena F, Gelabert P, Vega-Garcia C (2022) Integrating geospatial wildfire models to delineate landscape management zones and inform decision-making in Mediterranean areas. Safety Science 147, 105616.
| Crossref | Google Scholar |
Rodrigues M, Camprubí ÀC, Balaguer-Romano R, Megía CJC, Castañares F, Ruffault J, Fernandes PM, de Dios VR (2023) Drivers and implications of the extreme 2022 wildfire season in Southwest Europe. Science of The Total Environment 859, 160320.
| Crossref | Google Scholar | PubMed |
Rodriguez-Jimenez F, Fernandes PM, Fernández-Guisuraga JM, Alvarez X, Lorenzo H (2023) Drivers and trends in the size and severity of forest fires endangering WUI areas: a regional case study. Forests 14(12), 2366.
| Crossref | Google Scholar |
Salis M, Arca B, Alcasena-Urdiroz F, Massaiu A, Bacciu V, Bosseur F, Caramelle P, Dettori S, Fernandes de Oliveira AS, Molina-Terren D, Pellizzaro G, Santoni PA, Vega-Garcia C, Duce P (2019) Analyzing the recent dynamics of wildland fires in Quercus suber L. woodlands in Sardinia (Italy), Corsica (France) and Catalonia (Spain). European Journal of Forest Research 138, 415-431.
| Crossref | Google Scholar |
Salis M, Arca B, Del Giudice L, Palaiologou P, Alcasena-Urdiroz F, Ager A, Fiori M, Pellizzaro G, Scarpa C, Schirru M, Ventura A, Casula M, Duce P (2021) Application of Simulation Modeling for Wildfire Exposure and Transmission Assessment in Sardinia, Italy. International Journal of Disaster Risk Reduction 58, 102189.
| Crossref | Google Scholar |
Salis M, Del Giudice L, Jahdi R, Alcasena-Urdiroz F, Scarpa C, Pellizzaro G, Bacciu V, Schirru M, Ventura A, Casula M, Pedes F, Canu A, Duce P, Arca B (2022) Spatial Patterns and Intensity of Land Abandonment Drive Wildfire Hazard and Likelihood in Mediterranean Agropastoral Areas. Land 11(11), 1942.
| Crossref | Google Scholar |
Salis M, Del Giudice L, Alcasena-Urdiroz F, Jahdi R, Arca B, Pellizzaro G, Scarpa C, Duce P (2023) Assessing cross-boundary wildfire hazard, transmission, and exposure to communities in the Italy-France Maritime cooperation area. Frontiers in Forests and Global Change 6, 1241378.
| Crossref | Google Scholar |
San-Miguel-Ayanz J, Durrant T, Boca R, Maianti P, Libertà G, Jacome Felix Oom D, Branco A, De Rigo D, Suarez-Moreno M, Ferrari D, Roglia E, Scionti N, Broglia M, Onida M, Tistan A, Loffler P (2023) ‘Forest Fires in Europe, Middle East and North Africa 2022’. JRC135226. (Publications Office of the European Union: Luxembourg) 10.2760/348120
Schug F, Bar-Massada A, Carlson AR, Cox H, Hawbaker TJ, Helmers D, Hostert P, Kaim S, Kasraee NK, Martinuzzi S, Mockrin MH, Pfoch KA, Radeloff VC (2023) The global wildland–urban interface. Nature 621(7977), 94-99.
| Crossref | Google Scholar | PubMed |
Sharma LK, Gupta R, Fatima N (2022) Assessing the predictive efficacy of six machine learning algorithms for the susceptibility of Indian forests to fire. International Journal of Wildland Fire 31(8), 735-758.
| Crossref | Google Scholar |
Sirca C, Casula F, Bouillon C, García BF, Fernández Ramiro MM, Molina BV, Spano D (2017) A Wildfire Risk Oriented GIS Tool for Mapping Rural-Urban Interfaces. Environmental Modelling & Software 94, 36-47.
| Crossref | Google Scholar |
Spadoni GL, Moris JV, Vacchiano G, Elia M, Garbarino M, Sibona E, Tomao A, Barbati A, Sallustio L, Salvati L, Ferrara C, Francini S, Bonis E, Dalla Vecchia I, Strollo A, Di Leginio M, Munafò M, Chirici G, Romano R, Corona P, Marchetti M, Brunori A, Motta R, Ascoli D (2023) Active governance of agro-pastoral, forest and protected areas mitigates wildfire impacts in Italy. Science of The Total Environment 890, 164281.
| Crossref | Google Scholar | PubMed |
Tonini M, Parente J, Pereira MG (2018) Global assessment of rural–urban interface in Portugal related to land cover changes. Natural Hazards and Earth System Sciences 18(6), 1647-1664.
| Crossref | Google Scholar |
Tonini M, D’Andrea M, Biondi G, Esposti SD, Trucchia A, Fiorucci P (2020) A Machine Learning-Based Approach for Wildfire Susceptibility Mapping. The Case Study of the Liguria Region in Italy. Geosciences 10, 105.
| Crossref | Google Scholar |
UDS_2008 - Sardinia Land Use Map (2008) Carta Uso Suolo 2008. Sardegna Geoportale Available at http://www.sardegnageoportale.it/index.php?xsl=2420&s=40&v=9&c=14480&es=6603&na=1&n=100&esp=1&tb=14401 [accessed 25 March 2024]
Vacchiano G, Foderi C, Berretti R, Marchi E, Motta R (2018) Modeling anthropogenic and natural fire ignitions in an inner-alpine valley. Natural Hazards and Earth System Sciences 18(3), 935-948.
| Crossref | Google Scholar |
Viegas DX, Allgöwer B, Koutsias N, Eftichidis G (2003) Fire Spread and the Wildland Urban Interface Problem. In ‘International Workshop on Forest Fires in the in the WUI, WARM Project’, 15–16 May, Athens, Greece. pp. 93–103. Available at https://www.fria.gr/WARM/chapters/warmCh12Viegas.pdf
Vilar L, Camia A, San-Miguel-Ayanz J, Martín MP (2016a) Modeling Temporal Changes in Human-Caused Wildfires in Mediterranean Europe Based on Land Use-Land Cover Interfaces. Forest Ecology and Management 378, 68-78.
| Crossref | Google Scholar |
Vilar L, Gómez I, Martínez-Vega J, Echavarría P, Riaño D, Martín MP (2016b) Multitemporal modelling of socio-economic wildfire drivers in Central Spain between the 1980s and the 2000s: comparing generalized linear models to machine learning algorithms. PLoS One 11(8), e0161344.
| Crossref | Google Scholar |
Wang SSC, Qian Y, Leung LR, Zhang Y (2021) Identifying key drivers of wildfires in the contiguous US using machine learning and game theory interpretation. Earth’s Future 9(6), e2020EF001910.
| Crossref | Google Scholar | PubMed |