Characterising ignition precursors associated with high levels of deployment of wildland fire personnel
Alison C. Cullen A * , Brian R. Goldgeier A B , Erin Belval C and John T. Abatzoglou DA
B
C
D
Abstract
As fire seasons in the Western US intensify and lengthen, fire managers have been grappling with increases in simultaneous, significant incidents that compete for response resources and strain capacity of the current system.
To address this challenge, we explore a key research question: what precursors are associated with ignitions that evolve into incidents requiring high levels of response personnel?
We develop statistical models linking human, fire weather and fuels related factors with cumulative and peak personnel deployed.
Our analysis generates statistically significant models for personnel deployment based on precursors observable at the time and place of ignition.
We find that significant precursors for fire suppression resource deployment are location, fire weather, canopy cover, Wildland–Urban Interface category, and history of past fire. These results align partially with, but are distinct from, results of earlier research modelling expenditures related to suppression which include precursors such as total burned area which become observable only after an incident.
Understanding factors associated with both the natural system and the human system of decision-making that accompany high deployment fires supports holistic risk management given increasing simultaneity of ignitions and competition for resources for both fuel treatment and wildfire response.
Keywords: Firefighters, Linear regression, Simultaneous wildfire, Suppression personnel competition, Wildfire management, Wildfire response personnel deployment, Wildfire suppression resource.
Introduction
As fire seasons in the Western US intensify and lengthen, fire managers have been grappling with increases in the period during which simultaneous, significant incidents compete for wildfire response resources which in some cases outstrip preparedness and strain the capacity of the current system (Podschwit et al. 2019; Podschwit and Cullen 2020; Abatzoglou et al. 2021; Shuman et al. 2022; Cullen et al. 2023; Thompson et al. 2023). This management challenge leads us to explore a key research question: what precursors on the day of ignition are associated with wildland fires that evolve into incidents requiring high levels of response and suppression personnel? Answering this question is a pivotal step in supporting risk-informed decision making in the response to wildland fire. Our research seeks to establish the relationship between human, fire weather and fuels related factors that influence wildfire danger and their impact on the evolution of ignitions to become significant users of fire suppression response personnel. This analysis supports identification of the specific characteristics and scenarios, which have led ignitions to evolve into resource intensive incidents, and thus provides the agencies with wildland fire responsibilities information about preparedness, as well as proactive and reactive risk mitigation (Cullen et al. 2021). Understanding both the natural system and the systems of decision-making that accompany fires which require substantial deployments of response personnel supports holistic risk management in an era of increasing simultaneity of ignitions and competition for resources for both fuel treatment and wildfire response (Thompson et al. 2023).
Wildland fire danger and incidence have intensified over the last half-century largely as a result of three categories of factors: (1) human presence and actions related to land use (Balch et al. 2017; Radeloff et al. 2018), (2) a changing climate (Abatzoglou and Williams 2016; Abatzoglou et al. 2021), and (3) fuel buildup and composition due to long standing fire suppression policy and due to the influence of climate change on species and stand composition (Haugo et al. 2019). Our research is targeted to identify significant factors associated with elevated resource use and subsequent increases in competition to support decision making about resource planning, sharing and prioritisation.
Background and literature review
The interagency wildland fire response system in the United States is managed at four levels: the incident, the local dispatching area, the geographic area, and the national level (Belval et al. 2022). The initial response to wildland fires is typically managed at the incident and local levels. Local dispatch centres are equipped with a set of standard response protocols used when responding to initial fire reports under different weather conditions. The majority of fires in the US (about 97%) are controlled during the initial response phase, when the fire is smaller than 100 or 300 acres depending on category, by personnel dispatched by the local centre (Calkin et al. 2005). The fires that escape initial attack require extended response, which includes additional personnel, and some grow into large fires (generally defined as over 100 acres in timber fuels, 300 acres in grass and brush fuels) (St. Denis et al. 2023). Fires that continue to grow and require additional resources are managed by Incident Management Teams (IMTs). Historically, the most complex fires requiring the highest levels of personnel have been managed by Type 1 and Type 2 IMTs, with Type 1 teams managing the most complex fires. The management of these fires can require up to thousands of people per day, with IMTs managing the personnel, the heavy ground equipment (fire engines, bulldozers), overhead support (i.e. management personnel) and sometimes aerial equipment (airtankers and helicopters). Depending upon the agency managing the fire, fire management goals and strategies may vary substantially. However, because wildland fire response is an interagency system, large fires typically utilise personnel and equipment supplied by multiple agencies. Because these fires can have a substantial impact on the capacity of the wildfire response system (Thompson et al. 2023), the assignment of resources to these fires is overseen by Geographic Area Coordination Centers. During times of personnel and equipment scarcity, distribution of personnel and equipment between Geographic Areas is managed by the National Interagency Coordination Center.
Previous research related to this topic has been focused largely on characterising suppression costs and expenditures (Gebert et al. 2007; Yoder and Gebert 2012; Hand et al. 2014, 2016; Belval et al. 2019 and others as detailed below). While expenditures are related to personnel counts and deployments, efforts to quantify the number of personnel are needed in order to link suppression costs to the associated workforce capacity (Bayham and Yoder 2020). Workforce capacity is particularly relevant as funds to cover suppression costs are fungible, while the pool of highly skilled personnel needed for wildland fire suppression is both limited and non-fungible in the short term. Fires that have a substantially high demand for personnel and equipment can have a substantial impact on the readiness of the entire wildland fire response system (Thompson et al. 2023). Additionally, because these earlier analyses aimed to examine suppression costs while considering management decisions during fire incidents, they typically take into account both factors that would be observable at the time and place of ignition and also those that are only observable after the fact (e.g. total burned area). Although total burned area is certainly associated with resource demand for an incident, it is not observable until the blaze is extinguished and, additionally, there is debate in the literature about whether including fire size in such models introduces endogeneity issues (Gebert et al. 2007; Hand et al. 2017). For these reasons our analysis of key precursors of ignitions that use a significant amount of personnel focuses on information and characteristics that are observable at the time and place of ignition.
Gebert et al. (2007) developed a model to estimate expenditures on large USFS (US Forest Service) managed fires 1995–2004 (>300 acres, or >121 ha) with a goal of detecting extremely high cost fires, i.e. those responsible for 1–2 standard deviations above the expected expenditure given other characteristics. For these exceptional fires they identify the importance of factors which fall outside of the model, for example the decision to fight fires more or less aggressively as a result of political factors. Yoder and Gebert (2012) built upon this foundation, modelling total and per acre fire suppression costs for large fires (>300 acres, or >121 ha) managed by the USFS and DOI (US Department of the Interior). They conclude that it is possible to develop a predictive model for cost/acre in the absence of specific burned area data, with the inclusion of influential factors including time (year and month), fuel type, location and terrain characteristics (slope, elevation and aspect).
Hand et al. (2014) developed the Stratified Cost Index (SCI) based on data drawn from large fires (not deemed complex and >300 acres, i.e. >121 ha) in the Western US managed by the USFS using a spatial model based on final fire perimeters. The SCI supports forecasting of suppression expenditures, exploration of the role of fire managers in related decisions and allows comparison of realised costs relative to expected costs. These comparisons open an opportunity to delve into the causes for such differences. In further work based on the same dataset, the team considered expenditure projection to support future budget decisions (Hand et al. 2016) by comparing a model which relies solely on ignition point data and a model that incorporates the final spatial and temporal extent of the fire. They found that the latter model performs better due to its ability to adjust based on post hoc information about spread and burned area. This result has the potential to better support national level budgeting. A final iteration of this model added the footprint of previous fires to the variables, finding that previous fires may provide opportunities for suppression that might not otherwise exist, and thus may increase suppression cost due to deployment of additional resources to capitalize on these new opportunities (Belval et al. 2019).
Bayham and Yoder (2020) went a step further in modelling expenditures and resource allocation retrospectively based on a dynamic model (Arellano–Bond systems estimator) and pulling in information about wildfire growth, area burned and home damage. They found that daily expenditures and resource allocation are dynamic, with increases in expenditures associated with extreme fire growth potential of a fire and the presence of threatened homes. Gude et al. (2013) found that the presence of homes within 6 miles of an active fire increases daily suppression cost when looking at fires in California’s Sierra Nevada.
Beyond cost and expenditure as metrics of resource use, Hand et al. (2017) consider instead the impact of IMTs (Incident Management Teams) on suppression personnel deployment numbers relative to the impact of underlying fire conditions. Their work focuses on Type 1 and Type 2 IMTs assigned to fires occurring between 2007 and 2011 in the US. Using ‘days’ as a unit of analysis they find that when checking for fire and landscape characteristics, IMT assignments accounted for 14% of the variation in resource allocation, while teams in California were observed to be associated with higher levels of resource.
Bayham et al. (2020) carried out an exploration of resource deployment in the Western US from 2007 to 2013, delving into the impact of weather over the course of a fire on resource orders on incidents. They found that IMT anticipation of fire growth is influenced by expected weather which in turn affects resource orders, making the weather a primary driver of orders. They also tested other risk metrics such as evacuations but found little associated effect.
Our objective is to model the total personnel time required by individual large wildland fire incidents, as well as the number of personnel allocated on the peak day of usage. We estimate these quantities solely using characteristics which are observable at the time and place of ignition – rather than information gathered in the duration or at the conclusion of the fire event. We aim to support decision makers assessing which ignitions evolve into incidents with significant demand on suppression resources such as personnel, equipment and leadership. The identification of significant precursors, particularly those related to human and fuels factors, may inform and instigate medium term change in policy and management areas such as land use and zoning, fuels reduction and management, or agency interactions and leadership. Finally, while precursors related to fire weather or climate are only alterable on substantially longer time scales than those related to human or landscape processes, knowledge of the relative significance and role of these in fire danger may have an important influence on longer term decision contexts.
Methodology
We develop a framework to integrate the human, fire weather and fuels factors and conditions that might impact resource use, compile a dataset that reflects those factors, and then fit statistical models to estimate total and peak resource use for ignitions in the Western US for fire seasons 2017–2020. As outlined above, our analysis is restricted to precursors known at the time and place of ignition to ensure relevance in support of decision-making during fire response. Our approach is designed to identify the drivers of personnel use to better inform risk management.
Framework
We developed a conceptual framework of the drivers of personnel use that are observable at the time of ignition (Fig. 1). The framework categorises the precursor variables used in our analysis by indicating their relationship to human, fire weather and/or fuel factors. This framework is intended to support the interpretation and application of our results to decision making by reflecting the extent to which human, ecosystem and planetary systemic behaviours influence potential drivers of resource use. The variables in the framework were chosen to support our multivariate regression based on previous research which investigated the drivers of wildland fire suppression costs (see section 2) and thus were hypothesised to have a relationship with personnel deployment.
Conceptual Venn diagram framework capturing the main drivers of personnel use. Each circle presents a thematic category: human (blue), fuels (green), and fire weather (red). Specific drivers which are included in this analysis are placed in the appropriate categories within the Venn diagram. Acronyms: Geographic Area Coordination Center (GACC), Wildland–Urban Interface (WUI).
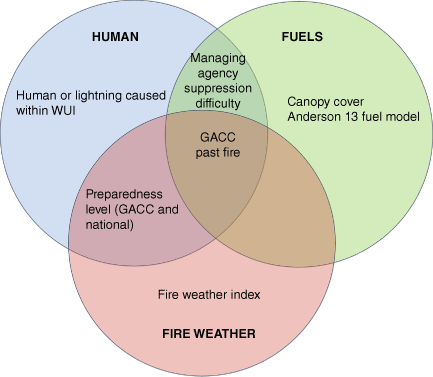
Data
Our outcome variables reflect the level of personnel use and deployment in the Western US for fire seasons from 2017 to 2020 and include (1) total personnel-days on an individual fire incident and (2) peak personnel deployed on an individual incident on the day of peak use. These data are gathered from assignments archived within dispatching software, specifically, the Resource Ordering and Status System (ROSS 2017–2019) and the Interagency Resource Ordering Capability (ROSS/IROC (Resource Ordering and Status System/Interagency Resource Ordering Capability) 2022). Assignments are archived for all crew, equipment, overhead, and aerial resources ordered to a fire. Both total and peak personnel deployment count distributions are characterised by long righthand tails and thus were log-transformed in order to meet the assumptions required to fit a traditional Gaussian regression model (Fig. 2).
Histograms for count of Total and Peak Personnel on individual fire incidents (2017–2020) in the Western US. Lefthand panels display raw counts, righthand panels display log-transformed counts.
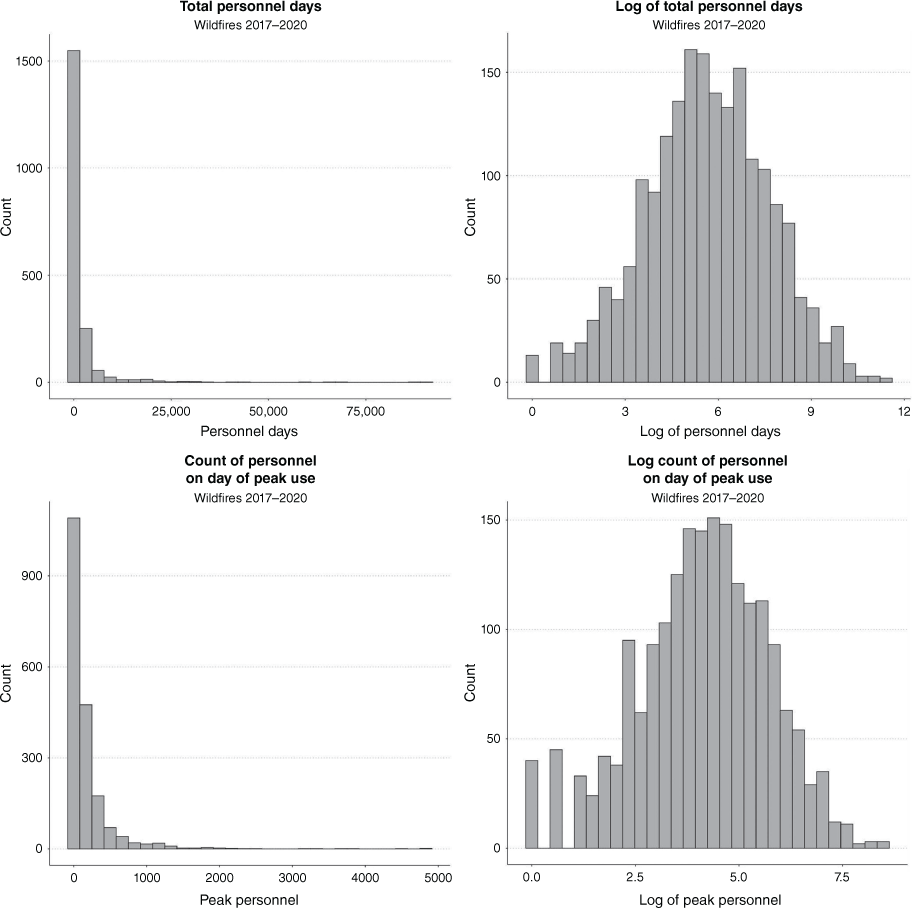
Data are leveraged from multiple sources. Data from the European Centre for Medium-Range Weather Forecasts Reanalysis version 5 (ERA5 2023) support characterisation of fire weather conditions using information drawn from the Canadian Fire Weather Index (FWI) System (Van Wagner 1987) coincident with the ignition date and the three prior days (see Table 1 for details). Data related to the human and fuels themes are pulled from the Fire Occurrence Database Plus (FOD Plus; Pourmohamad et al. 2023), LANDFIRE, IMSR, and datasets from the US Geological Survey (USGS). Table 1 provides an overview of each specific factor name, a short description of the factor, and the source from which we obtained the data, while summary statistics are presented in the Appendix 1. Specifically, the human factors we identified as potential drivers of resource use include the fire cause, i.e. human or lightning (Short 2022), and whether the ignition occurs within an area classified as either interface or intermix WUI (Radeloff et al. 2018). Managing agency for the fire and the suppression difficulty index (SDI) (Pourmohamad et al. 2023) are part of both the human and fuels themes. Similarly, preparedness level (PL) on day of ignition (Pourmohamad et al. 2023) is part of the human and fire weather themes. Fuel model (Landfire 2023a) and canopy cover (Landfire 2023b) are both solely in the fuels category. The Geographic Area Coordination Center (GACC, see Fig. 3) with which the ignition location, and whether there was a fire at the ignition location in the past 10 years, are associated with all three major thematic categories.
Variable | Description | Source | |
---|---|---|---|
Total Personnel | The number of total personnel days assigned, including ground and overhead but excluding aerial personnel on a fire incident. | ROSS/IROC (Resource Ordering and Status System/Interagency Resource Ordering Capability) (2022) | |
Peak Personnel | The number of total personnel assigned, including ground and overhead but excluding aerial personnel, on the day of peak personnel use | ROSS/IROC (Resource Ordering and Status System/Interagency Resource Ordering Capability) (2022) | |
Agency | Managing agency | Pourmohamad et al. (2023) | |
Reference category: | |||
FS = US Forest Service | |||
DOI = US Department of the Interior | |||
Interagency = Multiple agencies | |||
State = State level agency | |||
GACC | Geographic Area Coordination Center encompassing the point of ignition | ROSS/IROC (Resource Ordering and Status System/Interagency Resource Ordering Capability) (2022) | |
Reference category: | |||
NWCC = Pacific Northwest | |||
ONCC = Northern California | |||
OSCC = Southern California | |||
RMCC = Rocky Mountain | |||
NRCC = Northern Rockies | |||
SWCC = Southwest | |||
GBCC = Great Basin | |||
PL | Preparedness Level assigned by NIFC on the day of ignition, related to planning, organisational readiness, burning conditions, fire activity, and resource availability. | Pourmohamad et al. (2023) | |
PLs range from 1 to 5, with PL at level 4 or 5 reflecting a high level of deployment and competition for resources | |||
PL GACC = 1 if the PL for the GACC encompassing the ignition is at 4 or 5, and = 0 otherwise. | |||
National PL = 1 if the PL for the nation is 4 or 5 at the time of ignition, and = 0 otherwise. | |||
Cause | Cause of Ignition | Pourmohamad et al. (2023) | |
Reference category: Natural + Other = Fire started naturally (lightning), or cause is unknown. Human = Fire caused by human activity or behaviour | |||
SDI | Suppression Difficulty Index (SDI) at the point of ignition integrating information about topography, fuels, expected fire behaviour with 15 mph upslope winds and fully cured fuels, firefighter line production rates given fuel conditions, and accessibility (distance from roads/trails). | Pourmohamad et al. (2023) | |
SDI is a static variable taking a continuous value between 1 and 10, with higher values reflecting higher suppression difficulty. | |||
Fire Weather Index (FWI) | Percentile in the historic FWI distribution 2001–2020 corresponding to the average FWI at the ignition point during a 4 day window, including the 3 days prior to ignition and the day of ignition. In this analysis the FWI serves as a proxy for potential fireline intensity dictated by fire weather. Note: the FWI values used in this analysis are drawn from the Fire Weather Index indicator itself from within the Canadian FWI system. | ERA5 (2023), https://www.ecmwf.int/en/forecasts/dataset/ecmwf-reanalysis-v5 | |
Hersbach et al. (2020) | |||
Canadian Fire Weather Index (FWI) System, Van Wagner (1987) | |||
Past Fire | Past fire at the ignition point | Welty and Jeffries (2021) | |
Reference category: No past fire or past fire more than 10 years prior to ignition | https://www.sciencebase.gov/catalog/item/61aa537dd34eb622f699df81 | ||
Past Fire within 0–10 years (Y = 1) | |||
USGS data establish most recent past fire perimeters at point of ignition including wildfire and prescribed burning. | |||
Canopy Cover | Canopy cover is assigned given the average percent cover of the tree canopy in a 1 mile radius around ignition point, to the midpoint of binned values as follows: Bucket raster cells as 10–20%, 20–30%, etc. for percent cover, then assign canopy cover as the value of the midpoint of its corresponding bucket, i.e. 10–20% = 15. For consistency canopy cover data are taken at the point of ignition for the closest year (2014) that preceded our window. | Landfire (2023b) | |
https://www.landfire.gov/cc.php | |||
Anderson 13 | Anderson 13 fuel models for fire behaviour reflect fuel type at ignition point. Values are extracted from LandFire. Fuel models are categorised into 4 main vegetation groups, plus 3 additional groups, according to Anderson (1982). | Anderson (1982) | |
Reference category: Grass | https://www.fs.usda.gov/rm/pubs_int/int_gtr122.pdf | ||
Slash (Y = 1) | Landfire (2023a) | ||
Timber (Y = 1) | https://www.landfire.gov/fbfm13.php | ||
Shrub (Y = 1) | |||
Agriculture (Y = 1) | |||
Barren (Y = 1) | |||
Urban (Y = 1) | |||
Wildland–Urban Interface (WUI) | The WUI variable reflects whether ignition occurs within the WUI, and in particular within an interface or intermix area. | Carlson et al. (2022) | |
0 = not within WUI | https://www.sciencebase.gov/catalog/item/617bfb43d34ea58c3c70038f | ||
1 = within interface WUI | |||
2 = within intermix WUI | |||
Intermix = at least 50% vegetation cover surrounding buildings | |||
Interface = buildings are within 2.4 km of a patch of vegetation at least 5 km2 in size that contains at least 75% vegetation | |||
USGS and GIS calculation of distance from ignition point to nearest WUI polygon, where distance = 0 implies ignition within WUI. |
The spatial boundaries associated with the nine Geographic Area Coordination Centers (GACCs) that cover the continental United States. In this analysis, we did not consider fires that ignited within the Eastern or Southern GACCs.
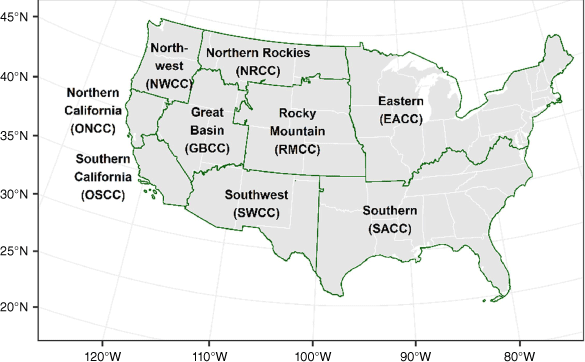
The sample used to fit the statistical model is composed of fire incidents occurring in Western GACCs occurring during the period 2017–2020, for which at least one ICS209 record exists (St. Denis et al. 2023). ICS209 records are created for fires that exceed 100 acres (40 ha) in timber, 300 acres (120 ha) in grass and brush, or has a Type 1 or 2 incident management team assigned; thus, our data only includes fires that were not contained by an initial response effort. Western GACCs include the Pacific Northwest, Northern California, Southern California, Rocky Mountain, Northern Rockies, Southwest, and Great Basin (see Fig. 3 for spatial boundaries.) This time window is narrowed to allow a focus on recent fire seasons, acknowledging that personnel deployment and use has changed substantially over time. Only individual fire incidents were included, while complexes were filtered out. This step was taken because complexes merge multiple ignition points into a single management unit and thus it is not possible to attribute personnel use to each individual ignition point. The sample was restricted to fires for which ground personnel or overhead personnel were deployed, those with only aerial personnel assigned were excluded. The filtering process yielded a sample of 1941 fires available for analysis (see Fig. 4).
Fire incidents in the sample from years 2017 through 2020. Each panel represents 1 year of data, while each marker represents one fire incident. The size of the marker reflects the total personnel used in each incident while the colour of the marker represents the day of ignition recorded in the ROSS/IROC dataset.
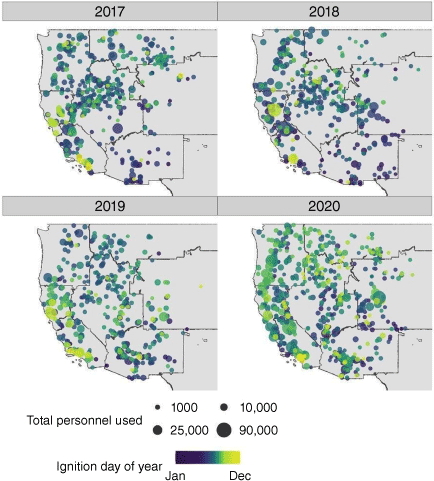
Spatial locations of ignition points are displayed by year between 2017 and 2020 in Fig. 4. Note that we do not use year as a precursor in the regression analysis because the intensity of a particular year/season and its influence on resource demand and deployment is not discernable until the fire season has concluded (and we restrict our analysis to factors that are observable on the day of ignition). We display the data by year to give a sense of the variability of the timing and intensity of fire seasons experienced in the Western US during the temporal scope of our analysis.
Statistical modelling approach
To model the impact of potential drivers on personnel use, we use a multivariate linear regression approach, specifically Ordinary Least Squares (OLS), to explore the association of personnel use with human, fire weather and fuels factors and conditions at the point and time of ignition. This facilitates comparison with existing literature on cost drivers (e.g. Gebert et al. 2007; Yoder and Gebert 2012; Gude et al. 2013; Hand et al. 2014, 2016; Belval et al. 2019; Bayham and Yoder 2020). Like suppression costs, the distribution of resource use on large fires is strictly positive and not normally distributed, while being characterised by a long righthand tail (see Fig. 2). Thus, we transformed the response variables (total personnel and peak personnel) using the log function (Ives 2015; Knief and Forstmeier 2021). There were no zeros or negative values in the response data, so there was no need for any additional modification. We considered the use of a generalised linear mixed model to directly account for the non-normality of the data within the modelling framework, using both a negative binomial and a Poisson response distribution (see O’Hara and Kotze 2010 and St-Pierre et al. 2018). The regression analysis was carried out using the lm function in R core Team with model diagnostics completed using the DHARMa package (Hartig 2022). We found that the OLS model based on log transformed data resulted in a better fit as gauged by diagnostic residual analysis. For additional checks on model robustness, we reviewed AIC (Akaike Information Criterion) for analytic information about model selection and goodness of fit. Separately we carried out a stepwise regression to explore further whether the theoretical basis for inclusion of variables had implications for statistical efficiency. Finally, we partitioned our dataset into a training set and a testing set to check for outliers with undue influence through residual analysis.
Results
We present results of two regression models to assess relationships between total personnel and peak personnel assigned to fire incidents and key precursors related to human, fire weather or fuel factors as outlined below. Table 2 presents the coefficients (β), associated standard errors and P-values relating precursors to the log-transformed outcome variables (i.e. log(total personnel) and log(peak personnel), as well as the interpreted percentage differential in expected personnel assignments (i.e. 100 × (1 − exp(β))). Our models for total personnel (F-value = 27.8) and peak personnel (F-value = 28.2) both explain approximately 26% of the variance in resource use and are statistically significant (P < 0.001). A separate stepwise model excluded almost none of the statistically non-significant variables included in our full model. Specifically, the stepwise regression retained variables representing SDI, managing agency, human versus lightning caused fire, vegetation categories and preparedness level for at least one of our two outcome variables, regardless of P-value. Additionally, applying a stepwise approach did not improve observed explanatory power, i.e. R2 did not increase, and the AIC (Akaike Information Criterion) was approximately the same. For these reasons, and reasons of theoretical defensibility, we present the full models here rather than stepwise models or other models excluding non-significant variables more generally. Additionally, regression residuals were examined and found to be normally distributed, consistent with the assumptions underlying linear regression. Finally, validation by partitioning the dataset into training (80%) and testing (20%) segments found nearly identical results to the full model fit we present in terms of R2, AIC, model coefficients and residuals.
Input Variables | Log(Total Personnel) | Log(Peak Personnel) | |||
---|---|---|---|---|---|
β (s.e.) | % | β (s.e.) | % | ||
DOI (Ref = FS) | 0.299A** (0.152)B | 34.9C | 0.281** (0.122) | 32.5 | |
Interagency | −0.125 (0.147) | −11.8 | −0.295** (0.117) | −25.6 | |
State Agency | 0.069 (0.181) | 7.1 | 0.224 (0.145) | 25.1 | |
GACC ONCC (Ref = NWCC) | 0.867*** (0.191) | 138.0 | 0.895*** (0.152) | 144.7 | |
GACC OSCC | 1.585*** (0.174) | 387.9 | 1.483*** (0.139) | 340.6 | |
GACC RMCC | −0.485*** (0.169) | −38.4 | −0.436*** (0.135) | −35.3 | |
GACC NRCC | −1.071*** (0.160) | −65.7 | −0.999*** (0.128) | −63.2 | |
GACC SWCC | 0.004 (0.154) | 0.4 | −0.193 (0.123) | −17.6 | |
GACC GBCC | −0.372*** (0.129) | −31.1 | −0.228** (0.103) | −20.4 | |
PL GACC = 4/5 (Y = 1) | −0.056 (0.110) | −5.5 | −0.011 (0.088) | −1.1 | |
National PL = 4/5 (Y = 1) | 0.014 (0.098) | 1.4 | −0.104 (0.078) | −9.9 | |
Human Caused (Ref = Natural + Unknown) | 0.007 (0.087) | 0.7 | 0.104 (0.069) | 11.0 | |
Suppression Difficulty Index | 0.145 (0.125) | 15.6 | 0.035 (0.100) | 3.6 | |
Ignition FWI Percentile | 0.011*** (0.002) | 1.1 | 0.009*** (0.002) | 0.9 | |
A13 – Agriculture (Ref = Grass) | 0.116 (0.389) | 12.3 | 0.036 (0.311) | 3.7 | |
A13 – Barren | −0.045 (0.281) | −4.4 | −0.115 (0.225) | −10.9 | |
A13 – Shrub | 0.234** (0.103) | 26.4 | 0.178** (0.082) | 19.5 | |
A13 – Slash | −1.179 (1.019) | −69.2 | −1.936** (0.814) | −85.6 | |
A13 – Timber | 0.239* (0.133) | 27.0 | 0.114 (0.106) | 12.1 | |
A13 – Urban | 0.026 (0.168) | 2.6 | 0.140 (0.135) | 15.0 | |
Past Fire w/in 10 years (Y = 1) | −0.269** (0.130) | −23.6 | −0.208** (0.104) | −18.8 | |
Canopy Cover% w/in 1 Mile | 0.039*** (0.003) | 4.0 | 0.019*** (0.003) | 1.9 | |
WUI-Interface (Ref = Not within WUI) | 0.859** (0.337) | 136.1 | 0.596** (0.269) | 81.5 | |
WUI-Intermix | 0.371*** (0.144) | 44.9 | 0.489*** (0.115) | 63.1 | |
(Intercept) | 4.100*** (0.278) | 3.056*** (0.222) | |||
R2, F-value, model signif. | R2 = 0.26, F(24,1916) = 27.8*** | R2 = 0.26, F(24,1916) = 28.2*** | |||
AIC = 7694.71, BIC = 7839.56 | AIC = 6823.81, BIC = 6968.65 |
The GACC where the ignition occurred and the agency which manages the incident are both found to be significant predictors of personnel counts. Relative to the Pacific Northwest, ignitions in California are on average associated with statistically significantly higher total personnel counts (138% higher for Northern California and 387.9% higher for Southern California) while ignitions in other locations are associated with lower personnel counts (Rocky Mountain 48.5% lower, Northern Rockies 65.7% lower, and Great Basin 31.1% lower). For peak personnel counts we see similar patterns with California ignitions associated with higher peak personnel assignments than in the Northwest (144.7% higher for Northern California and 340.6% higher for Southern California) while other locations are associated with lower counts on average (Rocky Mountain 35.3% lower and Northern Rockies 63.2% lower). Regarding managing agency relative to ignitions managed by the Forest Service, Interagency-managed ignitions are associated with on average 25.6% lower peak personnel counts. In contrast, fires managed by the Department of the Interior (DOI) are associated with 34.9% higher total personnel counts and 32.5% higher peak personnel counts.
We also observe statistically significant relationships between climatological precursors and assigned personnel counts. FWI observed for the 4-day window prior to, and including, the day of ignition as a percentile of the historic distribution at the point of ignition, is observed to be significantly associated with personnel counts. Each additional percentile relative to the historic distribution of FWI (e.g. increase from 93rd percentile to 94th percentile), is associated with on average 1.1% higher counts in total personnel assignments, and 0.9% higher counts for peak personnel, holding all other variables at their means and categorical variables at their reference levels.
With regard to fuels factors, we test both canopy cover and fuel category. Each percent increase in canopy cover averaged over a 1-mile radius around the ignition point is associated with a statistically significant increase of 4% in total personnel and 1.9% in peak personnel. Turning to Anderson 13 categories, compared with grass as the reference category, characterisation of the ignition point as Shrub is associated with 26.4% higher total personnel assignment and 19.5% higher peak personnel assignment, while categorisation as Slash is associated with 85.6% lower peak personnel assignment.
Fire within the past 10 years at the point of ignition is associated with a statistically significant lower count in terms of total personnel assigned (23.6% lower) and peak personnel assigned (18.8% lower). Finally, a WUI designation at the point of ignition is associated with a statistically significant increase in personnel counts relative to non-WUI. WUI Interface categorisation is associated with 136.1% higher total personnel assignment and 81.5% higher peak personnel assignment, while WUI intermix categorisation is associated with 44.9% higher total personnel assignment and 63.1% higher peak personnel assignment.
Discussion
Our analysis yields statistically significant linear regression models for both total personnel use and peak personnel use related to fire response in the Western US explaining 26% of observed variance and relying solely on precursors that are observable at the time and place of ignition. The most significant and impactful precursors are geographic location (represented by GACC), FWI, canopy cover, WUI category and a history of recent fire on the landscape. These results align partially with, but are distinct from, prior studies which (as mentioned above) focus on modelling expenditures related to suppression and include precursors such as total burned area which becomes observable only after an incident.
Fire weather has a pronounced effect on fire behaviour and resource demand in our results. Our model includes two precursors representing the influence of weather. We find that personnel use increased significantly with FWI percentile. Locally, extreme fire weather conditions concurrent with the ignition date have been shown to be linked with a variety of fire impacts including eventual fire size (Riley et al. 2013; Jolly et al. 2019). This result also tracks with several regression models estimating suppression costs that include energy release component or growth potential as the fire weather indices in the model (i.e. Gebert et al. 2007; Yoder and Gebert 2012; Gude et al. 2013; Hand et al. 2016; Belval et al. 2019; Bayham et al. 2020), as well as the Hand et al. (2017) findings indicating that resource use is associated with fire evolution and also with fire weather. While not revealing a statistically significant association with peak personnel use, national and GACC PL do exhibit a negative sign on the fitted coefficient, suggesting a reduction in peak deployment for ignitions which occur during periods in which intense resource competition at the regional and national scale is already being experienced. The importance of fire weather aligns with the impact of simultaneous demand across the whole response system on specific days, a situation which is strongly influenced by larger scale climate/weather anomalies (Abatzoglou et al. 2021).
Further, these results shed light on the potential impacts of increasing competition for resources over time. This competition is driven in part by a relatively small number of large fires that commandeer significant resources (Thompson et al. 2023). Given that future climate projections suggest an increase in the number of simultaneous and significant fires that compete for finite response and management resources (Podschwit and Cullen 2020; McGinnis et al. 2023), this work supports decision making about resource sharing, planning and prioritisation.
Location represented by GACC is observed to be a highly significant precursor in the resource use models presented here. Resource use is significantly higher for wildfire ignitions in California GACCs in this analysis, in alignment with results reported in related research (Wei et al. 2020). This finding may indicate that fire response is approached differently in the California geography given the presence of CAL FIRE (California Department of Forestry and Fire Protection) operations. A distinct signal for California was similarly reported by Hand et al. (2017) and Hand et al. (2016). Interestingly, several other regions are associated with reduced levels of resource assignment on average, including Northern Rockies, Rocky Mountain and Great Basin (for total personnel only), even though our models are controlled for fire weather and the presence or absence of people and property through WUI categories. The presence and influence of different agencies in these regions may be driving some of this difference. For example, CAL FIRE is the second largest wildland firefighter organisation in the world with a mission that aligns with aggressive suppression actions, which often require substantial personnel and equipment. Likewise, approximately half of the population living in the WUI in the Western US resides in the state of California (Radeloff et al. 2018) contributing to California’s overall elevated risk and resource demand.
We find that resource use is positively associated with the extent of canopy cover while resource use is negatively associated with a history of past fire (which serves to reduce fuel load) on the landscape. This result suggests the importance of fuel abundance and availability in contributing to the evolution of an incident from an ignition to a heavy use of personnel. We further include Anderson 13 fuel category as a predictor (e.g. timber, slash, shrub) despite its lack of statistical significance when canopy cover is controlled. The inclusion of both precursors, even though some fuel types are consistent with canopy cover while others are not, represents an acknowledgement that there may be contexts where both fuel type and canopy cover are important precursors for resource use. Earlier work by Gude et al. (2013) also considered the role of fuels in explaining variance in resource use, and found that the percentage of forest in a final fire perimeter is associated with a decrease in daily suppression expenditures. Gebert et al. (2007), Yoder and Gebert (2012), Hand et al. (2016) and Belval et al. (2019) also found fuels to be important. We would note that these previous approaches included different model outcomes (suppression expenditure) and examined precursors such as final fire perimeter known only after the incident had concluded. In addition, some models used a different unit of analysis (day) and a different precursor representing fuel (percentage of forest/wildland rather than canopy cover). All of these represent substantial differences relative to our analysis.
Turning to consideration of people and property we find that ignition within a WUI area is associated with an increase in both total and peak resource use when compared with ignition outside of a WUI area. The presence of people and property in close proximity to ignitions has been established previously as significantly associated with personnel deployment, which is in alignment with our findings. For example, Gebert et al. (2007), Hand et al. (2016), Gude et al. (2013), Belval et al. (2019) and Bayham and Yoder (2020) find that fire proximity to homes increases expenditures and changes resource allocation. Interestingly we do not find SDI and ignition cause (human vs lightning) to be statistically significantly associated with resource use, although this result may be due to the model controlling for location and WUI status, both of which represent the presence or absence of people and property.
We acknowledge that our models leave substantial unexplained variance, a result which is also consistent with previous related work. One possible interpretation of this result is that it is difficult to account for the impact of temporal behaviour as fires evolve. This is true whether one focuses on individual fire incidents as we do, or daily outcomes such as those examined by Hand et al. (2016). We acknowledge that while our model focuses on total personnel which are aggregated across a whole fire incident, and also peak personnel on the day of maximum use, the evolution of fire incidents over time plays an important role in resource demand and deployment.
Finally, the role of the managing agency in resource demand is found to have several significant associations with personnel deployment. Fires managed by the US Department of Interior are found to be associated with higher levels of total and peak personnel use relative to those managed by the US Forest Service, a result which aligns with the impact of agency found in models of suppression costs. By contrast, we find that interagency managed fires are associated with lower peak personnel deployment relative to those managed by the Forest Service. Examining this result a bit further we compared the number of total and peak personnel on each fire in our sample (from archived dispatching records) to the number of personnel recorded in the ICS-209s. In the ICS-209 records we found additional resources recorded for interagency fires beyond those which were included in the archived dispatching records, while US Forest Service fires appear to have relatively complete dispatching records of personnel. This discrepancy aligns with findings in existing literature that interagency fires appear to use resources that are not archived or captured by the interagency wildfire dispatching system (USDA (United States Department of Agriculture) 2023).
The data used in this study open several avenues of future research that might be developed to examine additional questions regarding personnel use and deployment. For example, models might be developed to examine specific personnel types and compared with the presented model to see what precursors are associated with relatively higher use of equipment (i.e. engines and bulldozers) or crews. Future work might also investigate daily use of personnel. While the data compiled did not include daily observations, other datasets do support such analyses. Finally, future research might investigate the use of tools to model nonlinear relationships. We leave these areas as next steps for future studies.
Conclusions
This research identifies the key characteristics of ignitions that are likely to evolve into substantial uses of personnel both across the life of the incident and also on the day of peak deployment. The most significant and impactful precursors are found to be geographic location (with California associated with the most substantially elevated deployments), fire weather, canopy cover, the presence of people and property as indicated by WUI status, and a history of past fire on the landscape. All these precursors are observable at the time and place of ignition and in advance of containment or extinction of the incident. The models were designed using only precursor variables in order to provide historical context and enhanced understanding of personnel use on individual fires to support a wide variety of policies and management decisions such as staffing levels, resource sharing agreements, land use and zoning, and fuels reduction and management. Combining the results of this research with other studies such as projections of climate change or fuels management effects on fire behaviour allows managers to explore the potential impacts of these changes on personnel use. Finally, for precursors for which policy and management levels may exert influence – for example WUI status through zoning or history of fire on the landscape through prescribed burning – these results provide support for taking proactive steps to reduce the long-term risk associated with wildfire and its impacts.
Data availability
The data used in this analysis were accessed through a Data Use Agreement between University of Washington and the US Forest Service (22-JV-11221636-141), from the datasets presented in Pourmohamad et al. (2024), https://doi.org/10.5194/essd-16-3045-2024 and from ERA5 (2023), https://www.ecmwf.int/en/forecasts/dataset/ecmwf-reanalysis-v5.
Declaration of funding
The authors gratefully acknowledge NSF’s Growing Convergence Research Program (Award Number 2019762), for generous support of this work.
Acknowledgements
The authors wish to acknowledge our Data Use Agreement – a Joint Venture agreement between the University of Washington and the US Forest Service Rocky Mountain Research Station 22-JV-11221636-141. In addition, the authors gratefully acknowledge the NSF Growing Convergence Research Program (Award Number 2019762), for generous support of this work. The findings and conclusions in this report are those of the author(s) and should not be construed to represent any official USDA or U.S. Government determination or policy.
Author contributions
A. C. C.: Conceptualisation, Methodology, Investigation, Resources, Writing – Original Draft, Writing – Review and Editing, Supervision, Project Administration, Funding Acquisition. B. R. G.: Methodology, Software, Validation, Analysis, Data Curation, and Review and Editing. E. J. B.: Conceptualisation, Methodology, Investigation, Data curation, Writing – Review & Editing. J. T. A.: Data Curation, and Review and Editing.
References
Abatzoglou JT (2013) Development of gridded surface meteorological data for ecological applications and modelling. International Journal of Climatology 33, 121-131.
| Crossref | Google Scholar |
Abatzoglou JT, Williams AP (2016) Impact of anthropogenic climate change on wildfire across western US Forests. Proceedings of the National Academy of Sciences 113(42), 11770-11775.
| Crossref | Google Scholar | PubMed |
Abatzoglou JT, Juang CS, Williams AP, Kolden CA, Westerling AL (2021) Increasing synchronous fire danger in forests of the western United States. Geophysical Research Letters 48, e2020GL091377.
| Crossref | Google Scholar |
Anderson HE (1982) ‘Aids to Determining Fuel Models For Estimating Fire Behavior.’ (US Department of Agriculture) Available at https://www.fs.usda.gov/rm/pubs_int/int_gtr122.pdf
Balch JK, Bradley BA, Abatzoglou JT, Nagy RC, Fusco EJ, Mahood AL (2017) Human-started wildfires expand the fire niche across the United States. Proceedings of the National Academy of Sciences 114(11), 2946-2951.
| Crossref | Google Scholar | PubMed |
Bayham J, Yoder JK (2020) Resource allocation under fire. Land Economics 96, 92-110.
| Crossref | Google Scholar |
Bayham J, Belval EJ, Thompson MP, Dunn C, Stonesifer CS, Calkin DE (2020) Weather, risk, and resource orders on large wildland fires in the western US. Forests 11, 169.
| Crossref | Google Scholar |
Belval EJ, O’Connor CD, Thompson MP, Hand MS (2019) The role of previous fires in the management and expenditures of subsequent large wildfires. Fire 2(4), 57.
| Crossref | Google Scholar |
Belval EJ, Short KC, Stonesifer CS, Calkin DE (2022) A historical perspective to inform Strategic Planning for 2020 End-of-Year Wildland Fire Response Efforts. Fire 5(2), 35.
| Crossref | Google Scholar |
Carlson AR, Helmers DP, Hawbaker TJ, Mockrin MH, Radeloff VC (2022) Wildland-urban interface maps for the conterminous U.S. based on 125 million building locations: U.S. Geological Survey data release. 10.5066/P94BT6Q7
Calkin DE, Gebert KM, Jones JG, Neilson RP (2005) Forest Service large fire area burned and suppression expenditure trends, 1970–2002. Journal of Forestry 103(4), 179-183.
| Crossref | Google Scholar |
Cullen AC, Axe T, Podschwit H (2021) High-severity wildfire potential – associating meteorology, climate, resource demand and wildfire activity with preparedness levels (PLs). International Journal of Wildland Fire 30, 30-41.
| Crossref | Google Scholar |
Cullen AC, Prichard SJ, Abatzoglou JT, Dolk A, Kessenich L, Bloem S, Bukovsky MS, Humphrey R, McGinnis S, Skinner H, Mearns LO (2023) Growing convergence research: coproducing climate projections to inform proactive decisions for managing simultaneous wildfire risk. Risk Analysis 43, 2262-2279.
| Crossref | Google Scholar | PubMed |
ERA5 (2023) ECMWF Reanalysis v5. Available at https://www.ecmwf.int/en/forecasts/dataset/ecmwf-reanalysis-v5 [accessed January 2023]
Gebert KM, Calkin DE, Yoder J (2007) Estimating suppression expenditures for individual large wildland fires. Western Journal of Applied Forestry 22, 188-196.
| Crossref | Google Scholar |
Gude PH, Jones K, Rasker R, Greenwood MC (2013) Evidence for the effect of homes on wildfire suppression costs. International Journal of Wildland Fire 22, 537-548.
| Crossref | Google Scholar |
Hand MS, Thompson MP, Calkin DE (2016) Examining heterogeneity and wildfire management expenditures using spatially and temporally descriptive data. Journal of Forest Economics 22, 80-102.
| Crossref | Google Scholar |
Hand M, Katuwal H, Calkin DE, Thompson MP (2017) The influence of incident management teams on the deployment of wildfire suppression resources. International Journal of Wildland Fire 26, 615-629.
| Crossref | Google Scholar |
Hartig F (2022) _DHARMa: Residual Diagnostics for Hierarchical (Multi-Level/Mixed) Regression Models_. R package version 0.4.6. Available at https://CRAN.R-project.org/package=DHARMa
Haugo RD, Kellogg BS, Cansler CA, Kolden CA, Kemp KB, Robertson JC, Metlen KL, Vaillant NM, Restaino CM (2019) The missing fire: quantifying human exclusion of wildfire in Pacific Northwest Forests, USA. Ecosphere 10(4), e02702.
| Crossref | Google Scholar |
Hersbach H, Bell B, Berrisford P, Hirahara S, Horanyi A, Munoz-Sabater J, Nicolas J, Peubey C, Radu R, Schepers D, Simmons A (2020) The ERA5 global reanalysis. Quarterly Journal of the Royal Meteorological Society 146(730), 1999-2049.
| Crossref | Google Scholar |
Ives AR (2015) For testing the significance of regression coefficients, go ahead and log‐transform count data. Methods in Ecology and Evolution 6(7), 828-835.
| Crossref | Google Scholar |
Jolly WM, Freeborn PH, Page WG, Butler BW (2019) Severe fire danger index: a forecastable metric to inform firefighter and community wildfire risk management. Fire 2(3), 47.
| Crossref | Google Scholar |
Knief U, Forstmeier W (2021) Violating the normality assumption may be the lesser of two evils. Behavior Research Methods 53(6), 2576-2590.
| Crossref | Google Scholar | PubMed |
Landfire (2023a) 13 Anderson Fire Behavior Fuel Models Layer, LANDFIRE 2.0.0, U.S. Department of the Interior, Geological Survey, and U.S. Department of Agriculture. Available at https://www.landfire.gov/fbfm13.php [accessed 24 January 2023]
Landfire (2023b) Canopy Cover Layer, LANDFIRE 2.0.0, U.S. Department of the Interior, Geological Survey, and U.S. Department of Agriculture. Available at https://www.landfire.gov/cc.php [accessed 22 March 2023]
McGinnis S, Kessenich L, Mearns L, Cullen A, Podschwit H, Bukovsky M (2023) Future regional increases in simultaneous large western USA wildfires. International Journal of Wildland Fire 32(9), 1304-1314.
| Crossref | Google Scholar |
O’Hara RB, Kotze DJ (2010) Do not log-transform count data: do not log-transform count data. Methods in Ecology and Evolution 1(2), 118-122.
| Crossref | Google Scholar |
Podschwit H, Cullen AC (2020) Patterns and trends in simultaneous wildfire activity in the Continental United States from 1984-2015. International Journal of Wildland Fire 29(12), 1057-1071.
| Crossref | Google Scholar |
Podschwit HR, Larkin NK, Steel EA, Cullen A, Alvarado E (2019) Multi-model forecasts of very-large fire occurences during the end of the 21st century. Climate 6(4), 100.
| Crossref | Google Scholar |
Pourmohamad Y, Abatzoglou J, Belval E, Fleishman E, Short K, Reeves MC, Nauslar N, Higuera PE, Henderson E, Ball S, AghaKouchak A, Prestemon JP, Olszewski J, Sadegh M (2024) Physical, social, and biological attributes for improved understanding and prediction of wildfires FPA-FOD-attributes dataset. Earth System Science Data 16(6), 3045-3060.
| Crossref | Google Scholar |
Radeloff VC, Helmers DP, Kramer HA, Mockrin MH, Alexandre PM, Bar-Massada A, Butsic V, Hawbaker TJ, Martinuzzi S, Syphard AD, Stewart SI (2018) Rapid growth of the US Wildland-Urban Interface raises wildfire risk. Proceedings of the National Academy of Sciences 115(13), 3314-3319.
| Crossref | Google Scholar | PubMed |
Riley KL, Abatzoglou JT, Grenfell IC, Klene AE, Heinsch FA (2013) The relationship of large fire occurrence with drought and fire danger indices in the Western USA, 1984–2008: the role of temporal scale. International Journal of Wildland Fire 22(7), 894-909.
| Crossref | Google Scholar |
ROSS/IROC (Resource Ordering and Status System/Interagency Resource Ordering Capability) (2022) Lockheed Martin Enterprise Solutions & Services. Available at https://famit.nwcg.gov/applications/ROSS [accessed 1 July 2022]
Short KC (2022) Spatial Wildfire Occurrence Data for the United States 1992-2020 [FPA_FOD_2022_1014 6th edition, Fort Collins, Colorado Forest Service Research Data Archive]. 10.2737/RDS-2013-0009.6
Shuman JK, Balch JK, Barnes RT, Higuera PE, Roos CI, Schwilk DW, Stavros EN, Banerjee T, Bela MM, Bendix J, Bertolino S, Bililign S, Bladon KD, Brando P, Breidenthal RE, Buma B, Calhoun D, Carvalho LMV, Cattau ME, Cawley KM, Chandra S, Chipman ML, Cobian-Iñiguez J, Conlisk E, Coop JD, Cullen A, Davis KT, Dayalu A, De Sales F, Dolman M, Ellsworth LM, Franklin S, Guiterman CH, Hamilton M, Hanan EJ, Hansen WD, Hantson S, Harvey BJ, Holz A, Huang T, Hurteau MD, Ilangakoon NT, Jennings M, Jones C, Klimaszewski-Patterson A, Kobziar LN, Kominoski J, Kosovic B, Krawchuk MA, Laris P, Leonard J, Loria-Salazar SM, Lucash M, Mahmoud H, Margolis E, Maxwell T, McCarty JL, McWethy DB, Meyer RS, Miesel JR, Moser WK, Nagy RC, Niyogi D, Palmer HM, Pellegrini A, Poulter B, Robertson K, Rocha AV, Sadegh M, Santos F, Scordo F, Sexton JO, Sharma AS, Smith AMS, Soja AJ, Still C, Swetnam T, Syphard AD, Tingley MW, Tohidi A, Trugman AT, Turetsky M, Varner JM, Wang Y, Whitman T, Yelenik S, Zhang X (2022) Reimagine fire science for the Anthropocene. PNAS Nexus 1(3), 115.
| Crossref | Google Scholar | PubMed |
St. Denis LA, Short KC, McConnell K, Cook MC, Mietkiewicz NP, Buckland M, Balch JK (2023) All-hazards dataset mined from the US National Incident Management System 1999–2020. Scientific Data 10, 112.
| Crossref | Google Scholar | PubMed |
St-Pierre AP, Shikon V, Schneider DC (2018) Count data in biology—data transformation or model reformation? Ecology and Evolution 8(6), 3077-3085.
| Crossref | Google Scholar | PubMed |
Thompson MP, Belval EJ, Bayham J, Calkin DE, Stonesifer CS, Flores D (2023) Wildfire response: a system on the brink. Journal of Forestry 121(2), 121-124.
| Crossref | Google Scholar |
USDA (United States Department of Agriculture) (2023) On fire: The report of the Wildland Fire Mitigation and Management Commission. Available at https://www.preventionweb.net/publication/fire-report-wildland-fire-mitigation-and-management-commission
Wei Y, Thompson MP, Belval EJ, Calkin DE, Bayham J (2020) Understand daily fire suppression resource ordering and assignment patterns by unsupervised learning. Machine Learning and Knowledge Extraction 3(1), 14-33.
| Crossref | Google Scholar |
Welty JL, Jeffries MI (2021) Combined wildland fire datasets for the United States and certain territories, 1800s-Present: U.S. Geological Survey data release. 10.5066/P9ZXGFY3
Yoder J, Gebert K (2012) An econometric model for ex ante prediction of wildfire suppression costs. Journal of Forest Economics 18, 76-89.
| Crossref | Google Scholar |
Appendix 1.Summary statistics for precursor input variables
Variable | Mean | s.d. | Median | Min | Max | |
---|---|---|---|---|---|---|
Total Personnel | 1661 | 5284.56 | 277 | 1 | 90598 | |
Peak Personnel | 180.9 | 363.07 | 65 | 1 | 4832 | |
FWI Percentile | 82.54 | 13.27 | 86 | 12 | 99 | |
SDI | 0.30 | 0.36 | 0.16 | 0 | 2.59 | |
Canopy Cover | 12.24 | 16.52 | 3.06 | 0 | 74.88 | |
Anderson 13 | Count | % | ||||
Grass | 808 | 42 | ||||
Agriculture | 21 | 1 | ||||
Barren | 41 | 2 | ||||
Shrub | 504 | 26 | ||||
Slash | 3 | 0 | ||||
Timber | 393 | 20 | ||||
Urban | 171 | 9 | ||||
Total | 1941 | |||||
GACC | Count | % | ||||
OR-NWC | 294 | 15 | ||||
CA-ONCC | 134 | 7 | ||||
CA-OSCC | 193 | 10 | ||||
CO-RMC | 178 | 9 | ||||
MT-NRC | 217 | 11 | ||||
NM-SWC | 307 | 16 | ||||
UT-GBC | 618 | 32 | ||||
Total | 1941 | |||||
Past Fire | Count | % | ||||
No Past Fire | 1729 | 89 | ||||
Fire Within Past 10 Years | 212 | 11 | ||||
Total | 1941 | |||||
Within WUI | Count | % | ||||
Not WUI | 1714 | 88 | ||||
interface | 31 | 2 | ||||
intermix | 196 | 10 | ||||
Total | 1941 | |||||
Agency | Count | % | ||||
FS | 221 | 11 | ||||
DOI | 834 | 43 | ||||
IA | 664 | 34 | ||||
ST | 222 | 11 | ||||
Total | 1941 | |||||
Cause | Count | % | ||||
Natural or Other | 1194 | 62 | ||||
Human | 747 | 38 | ||||
Total | 1941 | |||||
PL GACC High (4/5) | Count | % | ||||
0 | 1506 | 78 | ||||
1 | 435 | 22 | ||||
Total | 1941 | |||||
PL National High (4/5) | Count | % | ||||
0 | 1143 | 59 | ||||
1 | 798 | 41 | ||||
Total | 1941 |