A dynamic and evidence-based approach to mapping burn potential
Richard van Dongen A * , Jaume Ruscalleda-Alvarez A B and Carl R. Gosper AA Biodiversity and Conservation Science, Department of Biodiversity, Conservation and Attractions, 17 Dick Perry Avenue, Kensington, WA 6151, Australia.
B Great Victoria Desert Biodiversity Trust, Perth, WA 6000, Australia.
International Journal of Wildland Fire 32(2) 164-177 https://doi.org/10.1071/WF22077
Submitted: 20 May 2022 Accepted: 19 November 2022 Published: 9 December 2022
© 2023 The Author(s) (or their employer(s)). Published by CSIRO Publishing on behalf of IAWF. This is an open access article distributed under the Creative Commons Attribution-NonCommercial-NoDerivatives 4.0 International License (CC BY-NC-ND)
Abstract
Background: Fire management is a crucial part of managing ecosystems. The years since last burn (YSLB) metric is commonly used in fire planning to predict when an area might be suitable to burn; however, this metric fails to account for variable recovery due to climate variability.
Aim: The aim of this study was to develop a predictor of when an area may be able to ‘carry’ fire based on observed patterns of vegetation recovery and fire occurrence that is responsive to climate variability.
Methods: Fire history maps and Landsat satellite imagery within the Great Victoria Desert of Australia were used to map vegetation recovery following fire. Burn potential models were then created by calculating the distributions of YSLB and vegetation recovery values for areas that subsequently burnt.
Key result: A burn potential model based on vegetation recovery is a better predictor of when an area is likely to burn than a model based on YSLB.
Conclusions: A burn potential model based on vegetation recovery provides an evidence-based and dynamic assessment of whether an area is likely to burn.
Implications: This approach provides a model that is responsive to climate variability that can assist fire managers in burn planning and assessing fire risk.
Keywords: fire management, fire mapping, fire planning, fire recovery, high-resolution imagery, Landsat, LiDAR, vegetation cover.
Introduction
Historical fire regimes across the globe have been altered and are expected to continue to do so owing to a range of factors including climate change (Rodman et al. 2020), changing land-use (Baudena et al. 2020) and depopulation of indigenous people (Burrows et al. 2006). Owing to these factors, dynamic approaches to monitor fire recovery and subsequent burn potential are required to determine risk and guide management actions. In forests environments, the combination of pre- or post-fire drought and fire can impact vegetation recovery rates (Harvey et al. 2016; Werner et al. 2022) and species composition (Connell et al. 2022). Additionally, short fire return intervals can promote early successional species and grasses that may maintain a system in a highly fire-prone state (Baudena et al. 2020).
Fire regimes in Australia’s ecosystems have also undergone significant changes. Across millennia, the Aboriginal nations that inhabited Australian deserts regularly applied small-scale, cool fires on the landscape, creating a fine-grain mosaic of vegetation patches at different stages of post-fire recovery (Gould 1971; Burrows and Christensen 1990; Bliege Bird et al. 2008, 2018). After European colonisation, it is likely that desert de-population resulted in a shift to fire patterns characterised by cycles of hot fires that burn large areas in spring and summer (Burrows and Christensen 1990; Burrows et al. 2006; Burrows and Chapman 2018). However, it is also possible that climatic factors contributed to large fire events also being typical of the period of traditional fire management (Wright et al. 2021).
The Great Victoria Desert (GVD) is Australia’s largest desert, covering an area of 42.2 million ha within Western Australia and South Australia. Traditional Owners in this region have an interest in managing country, and fire management is an integral component of their management. Currently, unmanaged fire is having adverse impacts on economic, environmental, social and cultural values (Burrows et al. 2018). It is therefore one of the critical threats facing the GVD. As in other Triodia-dominated landscapes (spinifex, Triodia spp.), large fires in the GVD follow good rainfall periods, typically after summer storms, and a clear relationship has been established between above-average precedent rainfall and increased fire occurrence (Griffin et al. 1984; Turner et al. 2008).
Contemporary fire management programs are informed from fire occurrence maps and generally have targets involving parameters such as minimum and maximum desirable intervals between fires, fire size and patchiness (Burrows and Butler 2011; Moore et al. 2018; Burrows 2020). The influence of climate can alter management of fire and associated controlled burn planning in various ways. Fire intervals could be shortened, by the increased presence of species that promote regular fire (Baudena et al. 2020) or high post-fire rainfall leading to rapid vegetation recovery. Conversely fire intervals could be lengthened by slow recruitment following severe fire or low rainfall (Harvey et al. 2016). In either case, models that can evaluate current vegetation patterns and respond accordingly will assist managers plan or prepare for future fire scenarios.
In the central deserts of Australia, localised fire history is mapped from field observations. However, across larger scales, imagery captured from satellites is used to interpret fire history and delineate fire boundaries, and to predict and manage wildfire risk in the future. Landsat satellite imagery is used extensively to map the progress of active fires (Schroeder et al. 2016), compile detailed fire histories and ‘years since last burn’ (YSLB) maps (Ruscalleda-Alvarez et al. 2021), and evaluate post-fire vegetation recoveries (Qarallah et al. 2021). The accuracy of fire mapping produced using Landsat imagery in desert environments was demonstrated by Ruscalleda-Alvarez et al. (2021), who reported that, at the landscape scale, Landsat-derived fire maps achieved a low rate of omission and commission errors (3.4 and 8.0%). The observed errors were limited to variability in the fire edge delineation and missed internal unburnt patches.
In the context of fire management, YSLB is commonly used as a predictor of when an area might be able to sustain fire spread or be suitable for fire management burns. The YSLB map is, in many ways, a proxy for vegetation cover. However, Triodia biomass is determined by the accumulated rainfall since the last fire (Allan and Southgate 2002) and YSLB per se. Moseby et al. (2016) stressed the importance of Triodia height as a factor in habitat suitability for the sandhill dunnart (Sminthopsis psammophila). Triodia height was a function of not only time since last fire, but crucially rainfall, which strongly influenced post-fire recovery trajectories.
The amount of biomass available to burn (fuel load) in specific vegetation types can be modelled using satellite indices (Rampant et al. 2019) and Phased Arrayed L-band Synthetic Aperture Radar data (Li et al. 2021). However, these approaches are reliant on sufficiently accurate mapping of vegetation type, which is often lacking particularly in remote regions. In addition to fuel load models specific to vegetation types, more general environmental metrics, such as leaf area index (Macfarlane et al. 2007), fractional cover (Muir et al. 2011) and vegetation cover (van Dongen et al. 2019) can also be mapped from satellite imagery indices. In each of these cases, reference data are required to test and calibrate the indices that are subsequently used to create vegetation cover maps. Reference data can take a variety of forms from destructive sampling and canopy photographs (Macfarlane et al. 2007) to field measures of vegetation cover (Muir et al. 2011), remotely piloted aircraft imagery (Rampant et al. 2019) and archived aerial photography (van Dongen et al. 2019). In each case, the accuracy of the resulting product must be taken into consideration along with the resources available to collect reference data.
Reference data may also be collected with the use of airborne Light Detection and Ranging (LiDAR) data. The use of LiDAR has become increasingly common in ecological studies (Bergen et al. 2009). It provides high-resolution vertical measures of plants and other aboveground objects and can also produce detailed ground elevation models (Polat and Uysal 2018; Zhao et al. 2021). These outputs, when analysed in conjunction with high-resolution optical imagery, have been used to produce accurate vegetation cover maps (Young et al. 2017). Globally, large amounts of high-resolution (~50 cm) satellite imagery are now available, which provides another potential source of reference data. If a strong relationship can be defined between vegetation cover as determined through these high-resolution data sources and data extracted from freely available Landsat images (in the form of, for example, a vegetation index), there is great potential to use Landsat to produce regionally calibrated models of vegetation cover. The models can then be applied to the vast, freely available archive of Landsat satellite imagery to produce current and past vegetation cover datasets over large areas in an efficient and cost-effective way.
The main purpose of the present study was to develop a novel method to quantify, and map, the potential that an area can carry fire. There was also an emphasis on identifying an accessible and cost-effective process to achieve this. Although the focus of this study is the Triodia hummock grassland of the GVD, there is no technical or ecological reason why the approach could not be applied to a broad range of ecosystem types. The objectives of this study are to:
Examine and discuss the comparability and utility of the reference vegetation cover data from the two remote sources: LiDAR and high-resolution airborne imagery (6 cm), and high-resolution satellite imagery (50 cm).
Determine what spectral index from Landsat satellite imagery has the highest correlation with reference vegetation cover data from the GVD; and
Determine if YSLB or vegetation cover change since the last fire (vegetation recovery) is the best predictor of burnt areas.
Methods
Study region
The GVD bioregion extends across Western Australia and South Australia and is divided into six IBRA (Interim Biogeographic Regionalisation for Australia) floristic sub-regions (Thackway and Cresswell 1995; Beard et al. 2013). The region’s dominant vegetation is Triodia hummock grasslands and mallee eucalypts, which are highly flammable. The study area is a 30 by 30 km region in the south-west of the GVD where the Great Victoria Desert Biodiversity Trust (GVDBT) (www.gvdbiodiversitytrust.org.au) is assessing the effects of fire management on conservation outcomes for rare and threatened fauna species. Large fires (>20 000 ha) impacted this area in 2007 and 2014 (Ruscalleda-Alvarez et al. 2021). The location of the management area and Landsat scene used in the study are shown in Fig. 1. The closest rainfall station with a complete rainfall record during the study period (1995–2020) is the Laverton Aero station 260 km northwest of the study area. The average annual rainfall for this station is 281 mm (Fig. 2).
![]() |
![]() |
Spatial datasets
Landsat satellite imagery has become a key dataset for monitoring and modelling environmental change (Wulder et al. 2012). The Landsat series of satellites captures imagery at 30 m resolution across several spectral bands. The satellites began capturing data in 1972 with the Landsat 1 satellite (at 60 m pixel resolution) with regular captures from 1987 (at 30 m pixel resolution). Landsat imagery, processed to surface reflectance (Commonwealth of Australia (Geoscience Australia) 2014), of the study area was downloaded from the Geoscience Australia data cube. Images downloaded included one cloud-free Landsat 5, 7 or 8 image per year captured between 1 January 1995 and 1 December 2021, with the capture month centred on November. This appeared to be the most suitable month, as the majority of rainfall in this region falls between December and March (http://www.bom.gov.au/; 2022). All Fractional Cover (FC) datasets (Lymburner 2021) over the same period were also downloaded. The FC dataset contains three bands: non-photosynthetic vegetation, photosynthetic vegetation and bare ground.
A fire history dataset for the study area created using Landsat imagery was acquired from the Department of Biodiversity, Conservation and Attractions (DBCA) of the Government of Western Australia (DBCA-060; www.data.wa.gov.au). The dataset includes annual fire maps, in shapefile format, for the period 1995–2020. The data were created using a semi-automated approach in eCognition software; they have low rates of omission (3.4%) and commission (8%) error (Ruscalleda-Alvarez et al. 2021). Commission errors were attributed to inaccuracies in fire edge detection as well as internal unburnt patches being mapped as burnt.
LiDAR data were captured from a fixed-wing aircraft across 33 strips (mean dimensions of 0.6 by 54 km) within the GVD. The capture was tasked by the GVDBT and was processed by the data provider (Anditi) and delivered as a point cloud dataset, at 4 points per m2. Digital photography, capturing imagery across the red (630–690 nm), green (510–580 nm) and blue (450–510 nm) (RGB) optical wavelengths at 6 cm resolution, was collected simultaneously with the LiDAR data. The point cloud LiDAR data were converted to a normalised surface model (NSM) at 1 m resolution using LASTools (Isenburg 2019). Owing to the clarity provided by the 6 cm RGB imagery and the binary target classes (bare ground and vegetation cover), this imagery was used as a proxy for field-based reference data. A comparison of vegetation cover measures from high-resolution imagery and field transects was carried out by Rampant et al. (2019). Results showed that the measures of vegetation cover from high-resolution imagery were more suitable as reference data for satellite imagery.
High-resolution satellite imagery was acquired from the ‘Vivid’ satellite mosaic. This mosaic covers Western Australia and was supplied to the West Australian government by Maxar™. The mosaic comprises imagery captured by the WorldView, GeoEye and Quickbird satellites over the period 23 November 2005 to 23 July 2019, with a median date of 8 September 2018. The imagery mosaic has the red (630–690 nm), green (510–580 nm) and blue (450–510 nm) optical wavelengths and is pansharpened to a resolution of 50 cm. The details of the individual scenes that make up the mosaic can be accessed via a reference shapefile (available by request from www.landgate.wa.gov.au). The satellites and acquisition dates that constitute the region of the mosaic used as training data are shown in Table 1.
![]() |
Computing vegetation cover measures
To determine the accuracy of plot-scale percentage vegetation cover calculated from high-resolution satellite imagery against the LiDAR/RGB data, 50 test plots (90 by 90 m) were created. The location of each plot was manually selected to cover the range of vegetation types visible in the imagery. For example, plots were placed in areas of largely bare ground, areas with a mix of grasses and shrubs, and areas of dense tree/shrub cover. Imagery tiles from each plot location clipped from the LiDAR/RGB and high-resolution satellite imagery were then created.
Within each plot/tile, 40 training points were manually added, 20 points in vegetation (photosynthetic and non-photosynthetic) and 20 in bare ground. The RGB imagery was used as a reference to identify vegetation/bare ground. Separate training data were created for the LiDAR/RGB and high-resolution satellite imagery. The LiDAR/RGB training data were buffered by 0.25 m and used to extract the mean pixel values from the RGB and NSM datasets. The training data, which included values from the red, green and blue bands from the high-resolution satellite imagery and the red, green and blue bands plus NSM from the LiDAR/RGB data were then used to create a series of random forest models. The random forest model used was from the ranger package (Wright and Ziegler 2017) in R (R Core Team 2021). The mtry values in the high-resolution satellite imagery model were 1–3 (covering the three bands), and for the LiDAR/RGB model were 1–4 (three bands plus NSM). The splitrule was set to ‘extratrees’ and the number of folds was set to four. Using an iterative process, a separate model was created and applied to the tiles from each of the 50 respective plots (100 individual models in total, 50 plots × 2 datasets). The result of this process was a series of images, in which vegetation and non-vegetation pixels were classified and from which percentage vegetation cover was calculated.
The creation and application of the random forest models was programmed to ensure repeatability and scalability. The application of a separate model per plot per dataset ensures that the classification of each individual plot achieves the highest possible accuracy. Each model is essentially trained on and applied to a very small geographic area (90 by 90 m). The proportions of vegetation cover in each plot, as measured by the LiDAR/RGB and high-resolution satellite imagery, were compared by calculating a correlations coefficient and root-mean-square error (RMSE).
The vegetation cover measures calculated from the high-resolution satellite imagery across the 50 plots were then regressed, using a linear model, against a range of indices from Landsat satellite data (Table 2). The index values were calculated from the mean pixel values within each of the 50 plots.
![]() |
Vegetation cover and post-fire recovery
The Landsat-derived index with the strongest correlation with vegetation cover measures was selected (i35; see Results section). This index, along with the offset and gain from the linear regression (Eqn 1), was applied to the annual Landsat imagery to produce a series of vegetation cover images over time covering the management area.
Eqn 1: Formula to convert Landsat (processed to surface reflectance) dataset to vegetation cover percentage within the study area (R, red band; SWIR, short-wave infrared band):
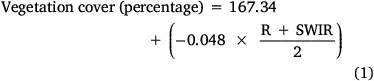
The vegetation cover images were combined with the fire history dataset to produce a vegetation recovery dataset for all years from 1995 to 2020. Using an iterative process in R, a YSLB layer was created for each year in the fire history dataset. For each year in the YSLB layer, the vegetation cover values prior to the burn were calculated, and were then subtracted from the current year’s vegetation cover values (Fig. 3). In the resulting images (one for each year), areas with a negative value have a current vegetation cover level lower than before the last time they burnt and areas with a positive value have cover levels higher than before the last time they burnt.
Burn potential
Burn potential maps were created using YSLB and vegetation recovery raster datasets along with the fire history shapefile as inputs. Annual versions (1995–2020) of the raster datasets were created. Using annual burn polygons from the fire history dataset, an iterative process was then carried out to calculate the distribution of values in each raster for year Y that were burnt in year Y + 1, and the distribution of values that were unburnt in year Y + 1. The distributions from each year were then combined. This resulted in a complete distribution of pixel values that had burnt for each of the raster datasets (YSLB and vegetation recovery).
Quantiles from the distributions were then used to assign burn potential classes (Table 3). For example, with the YSLB distribution, YSLB values that made up less than 5% of the complete distribution of areas that burnt were classified as having low burn potential. This approach provided an evidence-based and standardised approach to determine break points for classes but may need to be modified to suit operational requirements. A schematic diagram of how the YSLB burn potential map was created is shown in Fig. 4. The burn potential maps based on vegetation cover and vegetation recovery were created following a similar process.
![]() |
![]() |
The means of the burnt and unburnt classes from YSLB, vegetation cover and vegetation recovery datasets were compared using an unpaired two-sample Wilcoxon test. The Shapiro–Wilk normality test showed that the data were not normally distributed (P < 0.05). The proportional overlap between burnt and unburnt distributions (shown in the Results) was calculated using the effectsize package (Ben-Shachar et al. 2020) in R. A logistic regression was also applied to each of the burnt and unburnt values from the YSLB, vegetation cover and vegetation recovery measures to test the ability of each measure to predict whether an area had burnt in the following year. The logistic regression was carried out using the glm function in R (R Core Team 2021) with the value of each measure as the independent variable, and whether the area burnt in the following year the dependent variable. The Akaike Information Criterion (AIC) from the three logistic regression models was used to determine the best-performing model. The AIC is commonly used to determine the merit of alternative models (Aho et al. 2014).
To visualise the change in vegetation cover attributable to burn impact and subsequent recovery trajectory, six geographic points were selected within the study area. The location of each point was determined to highlight how different burn and recovery patterns are expressed in the burn potential models. For each point, vegetation cover was calculated from annual Landsat satellite imagery (1 January 1995 and 1 December 2021), using Eqn 1, and graphed. The fire history data were then used to confirm the late burn date.
Results
Vegetation cover model
The accuracies achieved from the classification of vegetation cover from the LiDAR/RGB data (example in Fig. 5) and high-resolution satellite imagery were both very high (Table 4). The mean classification accuracy across the 50 training plots using LiDAR/RGB (99.7%) was only slightly higher than that achieved with the high-resolution satellite imagery (99.1%). The classification of vegetation cover across the 50 training plots from the two datasets was also highly correlated (r2 = 0.881, RMSE = 8.82). At high cover levels (>60%), vegetation cover measures from the high-resolution satellite imagery were, on average, 7.9% higher than those measured from the LiDAR/RGB data (Fig. 6).
![]() |
![]() |
![]() |
Correlations between vegetation cover classifications from the high-resolution satellite imagery and Landsat indices were also high (Table 5). The best-performing index was i35 (Table 2) (r2 = 0.915 and RMSE = 10.54). However, many of the indices tested (i35, SRWI, SATVI, BS, NPV and STVI) (Table 2) all performed well (r2 > 0.85 and RMSE < 13). Although bare soil has a high negative correlation with vegetation cover, it is somewhat offset from vegetation cover values in this study area with areas close to 100% vegetation cover recording a bare soil percentage of ~30% (Fig. 7).
![]() |
![]() |
Vegetation recovery dataset
The vegetation recovery dataset for the study area from 2020 shows the difference between the current vegetation cover level and the vegetation (Fig. 8). Negative values indicate areas that have a vegetation cover value below that at which they previously burnt. These areas generally indicate recent fire where vegetation cover has not yet returned to the level at which it previously burnt. Areas with vegetation recovery values equal to or greater than zero indicate areas where vegetation cover has recovered back to, or beyond, the value at which it previously burnt. The mean vegetation recovery value is −1.5; this indicates that, on average, areas have approximately returned to their previous vegetation cover value. However, the distribution of recovery values is slightly skewed, with the most common value (mode) being −8.
Burn potential models
For the vegetation cover, vegetation recovery (difference from pre-burn cover) and YSLB datasets, the Wilcoxon test showed a significant difference between the burnt and unburnt classes (P < 0.05). The proportional overlap between distributions was lowest for the YSLB data (52.1%), followed by vegetation recovery (62.5%) and then vegetation cover (79.2%) (Fig. 9). The AIC scores from the logistic regression show the vegetation recovery data as the best predictor of when an area will burn (AIC = 256 011); this is closely followed by the YSLB dataset (AIC = 271 578). When using vegetation cover as a predictor, the AIC score is substantially higher (AIC = 926 659).
![]() |
Percentiles, calculated from the burnt class distributions shown in Fig. 9, were used as threshold values to create broad classes of burn potential (Fig. 10). The YSLB and vegetation recovery models are similar, with agreement within one class (i.e. very low/low) of 69% (Table 6). Both models have areas within the management zone that did not burn during the 1995–2020 period. These areas, therefore, cannot be assessed and do not have a percentile value. Unassessed areas account for 15.3% of the management area.
![]() |
![]() |
Areas where the two models predict the same burn potential class are represented in example Points 1 and 2 (Fig. 10 and Table 7). Point 1 last burnt in 2006, equating to a YSLB of 14 years. In the time since this last burn, vegetation cover has increased at a steady rate and is currently close to its pre-burn level. In both models, this puts this location in the ‘below average’ burn potential class, with a YSLB of 14 years being the upper limit of this class. Point 2 has a YSLB of 5 years and a current vegetation cover of 52.4%, which is 38.7% below its last pre-burn level of 91.1%. This puts the point in the ‘very low’ burn potential class for both models.
![]() |
Points 3 and 4 represent locations where the vegetation recovery model predicts a higher burn potential than the YSLB model. A scenario where this occurs is when the current vegetation cover value increases above the pre-burn cover value. This is the case with Point 3, which is currently at 76.0% cover; this is 15.5% higher than the pre-burn vegetation cover value of 60.5%. Faster-than-typical post-fire vegetation recovery can also be associated with higher burn potential in the vegetation recovery than YSLB models. Point 4 has a YSLB of 9 years, burning most recently in 2011. A YSLB of 9 years puts this area at the high end of the ‘very low’ class. However, the vegetation cover percentage returned to close to its pre-burn level, putting it in the ‘below average’ class according to this model. It is notable that it reached this level as early as 2015, 4 years post fire.
Points 5 and 6 demonstrate cases where the vegetation recovery model predicts a lower burn potential than the YSLB model. These appear to be areas where despite long periods since the previous fire, vegetation cover levels have not returned to pre-burn levels. At Point 5, 15 years after the previous fire, vegetation cover is at 59.1, 19.7% lower than the pre-burn value of 78.8%. The 15-year YSLB value puts the point in the ‘above average’ class in the YSLB model, while the large pre-burn to current vegetation cover difference results in it being classified as ‘very low’ burn potential in the vegetation recovery model. A similar response can be seen in Point 6, which, 17 years post fire, is at 65.0% cover, 22.3% lower than the pre-burn value of 87.3%.
Discussion
The classification of vegetation cover from LiDAR/RGB and high-resolution satellite RGB demonstrated that the two remote sources produce reference data that are highly correlated (r2 = 0.881, RMSE = 8.82). This is a significant result as it was expected that, owing to the higher resolution and added height layer, the LiDAR/RGB would outperform the high-resolution satellite data. The similarity in results may be due to the stark differences between the brightness of the soil in comparison with the darker vegetation in the GVD (Fig. 5). Although the LiDAR/RGB data, at 6 cm resolution, can create detailed vegetation cover maps (as evident in Fig. 5), this level of detail is perhaps beyond what is required for a reference dataset for Landsat satellite imagery. Results indicate that high-resolution satellite data, which is a much more cost-effective option, can be used as a reference dataset with a similar result.
Results showed the i35 index as the best predictor of vegetation cover of the Landsat indices (Table 5). However, except for NDVI, the other indices tested recorded correlations (r2) greater than 0.85 and would likely be functionally equivalent to i35. This is consistent with Villarreal et al. (2016) who reported that NDVI was a poor predictor of vegetation cover in a desert grassland ecosystem. The BS fractional cover, which is the inverse of percentage vegetation cover, is one such dataset that recorded a high correlation with percentage vegetation cover. The BS datasets were produced through an extensive network of field sites (~1400) (Gill et al. 2017). However, none of these field sites occurred within the study area or wider GVD. This perhaps explains the offset between percentage vegetation cover measured in this study and those obtainable from the BS dataset. These results are important for land managers who wish to produce locally calibrated vegetation cover datasets.
Consistency between imagery used for fire mapping and imagery used in the annual vegetation cover maps is crucial, and so is a complete and accurate fire mapping dataset. In this study area, the fire mapping dataset spanned 1995 to 2021. The area burnt at least once in this period was 84.7%. This is, therefore, the extent to which the vegetation recovery dataset can be calculated. Extending this back to the start of the regular-capture Landsat Thematic Mapper archive (1988) is likely to push the completeness up closer to 100%. Although occasional captures of Landsat Multispectral Scanner (MSS) data are available back to 1972, it is unlikely that fire maps created from these data could be incorporated in such a system, owing to the limited number of spectral bands available in MSS data.
A mean recovery value close to zero is a sensible result and seems to indicate a somewhat balanced system regarding the spatial and temporal distribution of fires. However, some 6000 ha (6%) of the study area has vegetation cover more than 20% above its previous burn value. This apparent anomaly could be due to varying fire intervals, such as a short fire interval preceding the current fire mapping, followed by a long period without fire, which allows greater vegetative growth. Alternately, high post-fire rainfall events could possibly provide greater growth than has previously been observed. A combination of both factors could be responsible: a longer than ‘usual’ fire interval that includes high post-fire rainfall.
The influence of high post-fire rainfall seems most likely in this study as many of the plots shown in Fig. 11 show a sharp increase in cover between 2011 and 2012 of ~15%. The Laverton Aero weather station recorded 522 mm of rainfall in 2011. This is its highest annual rainfall on record (1995–2021). While drawing direct conclusions from rainfall at this station can be problematic given it is located 260 km from the study area, workers at a nearby gold mine confirmed that rainfall in the study area in 2011 was exceptionally high (Alex Dent, AngloGold Ashanti, pers. comm.). By contrast, the near-complete lack of increases in vegetation cover since 2015 across the plots (Fig. 10) presumably points to little rain since then. However, the Laverton station received >500 mm in 2017 (Fig. 2), well above the long-term average of 281 mm. This discrepancy highlights the difficulty in utilising rainfall data as an explanatory or predicting variable where there is no nearby complete historical record to reference.
![]() |
The distributions of vegetation recovery values show a greater overlap between burnt and unburnt classes in comparison with YSLB (Fig. 4). This may be caused by the nature of these two measures. The YSLB value of unburnt areas increases infinitely with time as long as the area remains unburnt. The vegetation recovery value is constrained, with the mean recovery value in burnt areas being close to zero, meaning most areas reburn once they have returned to their pre-burn value.
The vegetation recovery dataset returned the lowest AIC score, indicating that it was the best predictor of whether an area was likely to burn. However, this was only marginally better than the YSLB model. Although the model performance is similar, the vegetation recovery model provides greater spatial detail of within-fire variability in burn potential. This represents an insight into areas where fire severity has been variable, where there is spatial variability in productivity related to soil fertility, and/or areas within a fire boundary in which rainfall has not been homogeneous.
The main drawback in the vegetation recovery approach is that it relies on the pre-burn cover value from only the previous fire. For each point, this is a single observation that is in turn influenced by the prior fire interval. Along with vegetation cover level, there are random effects like ignition points, time of day and wind, among others (Cary et al. 2009), that play a role in whether a point gets burnt at a particular moment in time. A potential improvement on the method would be to calculate a mean (or minimum) pre-burn cover value from several observations. However, requiring more observations limits the area the model can be applied to, as in the study area only 42% of the area has burnt more than once (van Dongen et al. 2021). A greater number of metrics could be explored in areas with higher fire frequencies.
The greater detail provided by the vegetation recovery model can be critical in fire management planning. Many fire management programs are geared to achieve a diversification of desert landscapes by maximising range and heterogeneity of post-fire recovery stages (also known as seral stages) (Burrows and Butler 2011; MNRAC 2012; KTLA 2014; Burrows 2020). The approach presented here, in which percentage vegetation cover can be mapped with precision at 30 m resolution, means that fire managers can be more aware of the current distribution of recovery stages across the landscape and where diversity could be increased by planned fire or where planned fire may be less important owing to high levels of existing heterogeneity. Vegetation cover maps derived from Landsat imagery can be updated as frequently as every 16 days (depending on cloud-free imagery). This means that fire operations can become more targeted both spatially and temporally, and the results of prescribed burns can also be more accurately assessed. Another potential benefit of such high-resolution vegetation cover maps relates to ecological assessments. It has been well established that different desert fauna show preference for different post-fire recovery stages (Lundie-Jenkins 1993; Pianka 1996; Southgate and Carthew 2007; Bliege Bird et al. 2018). The maps presented in this study can therefore be used to assess habitat suitability for different species as well as to inform targeted biodiversity surveys.
In terms of vegetation recovery in Triodia-dominated deserts, an alternate metric of ‘rain since last burn’ has also been proposed (Burrows and Butler 2011). An issue with this approach is the sparsity of weather stations in arid environments. Although more stations could be installed, the approach is in principle trying to predict vegetation cover from rainfall data, which is not always accessible. In these sparely gauged regions, regional atmospheric reanalysis products are believed to model rainfall more accurately than interpolated data sets (Acharya et al. 2019). However, these datasets currently have coarse resolution products (5–10 km pixel size), and still have an associated error. The approach in the present paper measures vegetation recovery from fire directly, without the need for rainfall data.
Conclusions
In this study, we have shown that highly accurate vegetation cover datasets can be derived from Landsat using widely available high-resolution satellite imagery as a reference. When combined with fire history data, vegetation cover estimates can be used to produce a burn potential model. The burn potential model provides an evidence-based and dynamic assessment of whether an area is likely to burn. Such information can be a very valuable tool for fire managers in desert environments as well as informing development of key ecological landscape attributes.
Data availability
Fire history data were acquired from www.data.wa.gov.au. All Landsat data used were downloaded from the National Computational Infrastructure (https://nci.org.au). The LiDAR dataset is owned under license by the Great Victoria Desert Biodiversity Trust. The Vivid dataset is licensed for use by Western Australian government agencies. All other data that support this study will be shared on reasonable request to the corresponding author.
Conflicts of interest
The authors declare no conflicts of interest.
Declaration of funding
We thank the Great Victoria Desert Biodiversity Trust and the Department of Biodiversity, Conservation and Attractions for financial support to develop the methodologies presented in this investigation.
Author contributions
R. V. D. conceived the initial project concept, which was developed and refined by J. R. A. R. V. D. carried out all image analysis and statistics. R. V. D. led the writing with substantial contributions from J. R. A. and C. R. G. in all sections. All authors contributed critically to the drafts and gave final approval for publication.
Acknowledgements
We thank Paul Rampant for processing LiDAR point cloud data, Bart Huntley for assistance with R and Python codes, Ben Millar and Katherine Zdunic for providing reviews of the final draft versions (all Department of Biodiversity, Conservation and Attractions) and Kathryn Sinclair (Great Victoria Desert Biodiversity Trust) for ongoing support.
References
Acharya SC, Nathan R, Wang QJ, Su CH, Eizenberg N (2019) An Evaluation of Daily Precipitation from a Regional Atmospheric Reanalysis over Australia. Hydrology and Earth System Sciences 23, 3387–3403.| An Evaluation of Daily Precipitation from a Regional Atmospheric Reanalysis over Australia.Crossref | GoogleScholarGoogle Scholar |
Aho K, Derryberry D, Peterson T (2014) Model Selection for Ecologists: The Worldviews of AIC and BIC. Ecology 95, 631–636.
| Model Selection for Ecologists: The Worldviews of AIC and BIC.Crossref | GoogleScholarGoogle Scholar |
Allan G, Southgate R (2002) Fire Regimes in the Spinifex Landscapes of Australia. In ‘Flammable Australia: The Fire Regimes and Biodiversity of a Continent’. (Eds R Bradstock, M Gill, R Williams) pp. 145–176. (Cambridge University Press)
Baudena M, Santana VM, Baeza MJ, Bautista S, Eppinga MB, Hemerik L, et al. (2020) Increased Aridity Drives Post-Fire Recovery of Mediterranean Forests towards Open Shrublands. New Phytologist 225, 1500–1515.
| Increased Aridity Drives Post-Fire Recovery of Mediterranean Forests towards Open Shrublands.Crossref | GoogleScholarGoogle Scholar |
Beard JS, Beeston GR, Harvey JM, Hopkins AJM, Shepherd DP (2013) The Vegetation of Western Australia at the 1:3,000,000 Scale. Explanatory Memoir. Second Edition. Conservation Science Western Australia 9, 1–152. http://www.dpaw.wa.gov.au/images/documents/about/science/cswa/articles/CONSERVATION_SCIENCE_VOL_9.1print_ready_low.pdf
Ben-Shachar MS, Lüdecke D, Makowski D (2020) Effectsize: Estimation of Effect Size Indices and Standardized Parameters. Journal of Open Source Software 5, 2815
| Effectsize: Estimation of Effect Size Indices and Standardized Parameters.Crossref | GoogleScholarGoogle Scholar |
Bergen KM, Goetz SJ, Dubayah RO, Henebry GM, Hunsaker CT, Imhoff ML, Nelson RF, Parker GG, Radeloff VC (2009) Remote Sensing of Vegetation 3-D Structure for Biodiversity and Habitat: Review and Implications for Lidar and Radar Spaceborne Missions. Journal of Geophysical Research: Biogeosciences 114, G00E06
| Remote Sensing of Vegetation 3-D Structure for Biodiversity and Habitat: Review and Implications for Lidar and Radar Spaceborne Missions.Crossref | GoogleScholarGoogle Scholar |
Bliege Bird R, Bird DW, Codding BF, Parker CH, Jones JH (2008) The ‘Fire Stick Farming’ Hypothesis: Australian Aboriginal Foraging Strategies, Biodiversity, and Anthropogenic Fire Mosaics. Proceedings of the National Academy of Sciences 105, 14796–14801.
| The ‘Fire Stick Farming’ Hypothesis: Australian Aboriginal Foraging Strategies, Biodiversity, and Anthropogenic Fire Mosaics.Crossref | GoogleScholarGoogle Scholar |
Bliege Bird R, Bird DW, Fernandez LE, Taylor N, Taylor W, Nimmo D (2018) Aboriginal Burning Promotes Fine-Scale Pyrodiversity and Native Predators in Australia’s Western Desert. Biological Conservation 219, 110–118.
| Aboriginal Burning Promotes Fine-Scale Pyrodiversity and Native Predators in Australia’s Western Desert.Crossref | GoogleScholarGoogle Scholar |
Burrows N (2020) ‘Fire and Introduced Predator Management Plans for the Great Victoria Desert Fauna Management Area.’ (Great Victoria Desert Biodiversity Trust)
Burrows N, Butler R (2011) ‘A fire management plan for Lorna Glen (Matuwa) and Earaheedy (Karara Karara) 2011-2015’. 33 p. (Department of Environment and Conservation: Kensington, WA)
Burrows N, Chapman J (2018) ‘Traditional and Contemporary Fire Patterns in the Great Victoria Desert.’ (Great Victoria Desert Biodiversity Trust: Perth)
Burrows N, Christensen P (1990) A Survey of Aboriginal Fire Patterns in the Western Desert of Australia. In ‘Ecological and Cultural Perspectives’. (Eds S Nodvin, T Waldop) pp.297–305. (Proceedings of an International Symposium USDA Forest Service General: Knoxville)
Burrows N, Burbidge A, Fuller P, Behn G (2006) Evidence of Altered Fire Regimes in the Western Desert Region of Australia. Conservation Science Western Australia 5, 272–284. https://www.dpaw.wa.gov.au/images/documents/about/science/cswa/articles/109.pdf
Burrows N, Gill M, Sharples J (2018) Development and Validation of a Model for Predicting Fire Behaviour in Spinifex Grasslands of Arid Australia. International Journal of Wildland Fire 27, 271–279.
| Development and Validation of a Model for Predicting Fire Behaviour in Spinifex Grasslands of Arid Australia.Crossref | GoogleScholarGoogle Scholar |
Cary GJ, Flannigan MD, Keane RE, Bradstock RA, Davies ID, Lenihan JM, Li C, Logan KA, Parsons RA (2009) Relative importance of fuel management, ignition management and weather for area burned: evidence from five landscape–fire–succession models. International Journal of Wildland Fire 18, 147–156.
| Relative importance of fuel management, ignition management and weather for area burned: evidence from five landscape–fire–succession models.Crossref | GoogleScholarGoogle Scholar |
Commonwealth of Australia (Geoscience Australia) (2014) Surface Reflectance NBAR+T 25 v. 2. Dataset. Available at http://pid.geoscience.gov.au/dataset/ga/102291
Connell J, Hall MA, Nimmo DG, Watson SJ, Clarke MF (2022) Fire, Drought and Flooding Rains: The Effect of Climatic Extremes on Bird Species’ Responses to Time since Fire. Diversity and Distributions 28, 417–438.
| Fire, Drought and Flooding Rains: The Effect of Climatic Extremes on Bird Species’ Responses to Time since Fire.Crossref | GoogleScholarGoogle Scholar |
Curry P, Zdunic K, Wallace J, Law J (2008) Landsat Monitoring of Woodland Regeneration in Degraded Mulga Rangelands: Implications for Arid Landscapes Managed for Carbon Sequestration. In ‘Proceedings of 14th Australasian Remote Sensing & Photogrammetry Conference’, Global Observations of Forest Cover and Land-use Dynamics, Darwin, Australia’.
Gill T, Johansen K, Phinn S, Trevithick R, Scarth P, Armston J (2017) A Method for Mapping Australian Woody Vegetation Cover by Linking Continental-Scale Field Data and Long-Term Landsat Time Series. International Journal of Remote Sensing 38, 679–705.
| A Method for Mapping Australian Woody Vegetation Cover by Linking Continental-Scale Field Data and Long-Term Landsat Time Series.Crossref | GoogleScholarGoogle Scholar |
Gould RA (1971) Uses and Effects of Fire among the Western Desert Aborigines of Australia. Mankind 8, 14–24.
| Uses and Effects of Fire among the Western Desert Aborigines of Australia.Crossref | GoogleScholarGoogle Scholar |
Griffin G, Price N, Portlock H (1984) Wildfires in the Central Australian Rangelands, 1970-1980. Journal of Environmental Management 17, 311–323.
Harvey BJ, Donato DC, Turner MG (2016) High and Dry: Post-Fire Tree Seedling Establishment in Subalpine Forests Decreases with Post-Fire Drought and Large Stand-Replacing Burn Patches. Global Ecology and Biogeography 25, 655–669.
| High and Dry: Post-Fire Tree Seedling Establishment in Subalpine Forests Decreases with Post-Fire Drought and Large Stand-Replacing Burn Patches.Crossref | GoogleScholarGoogle Scholar |
Isenburg G (2019) ‘LAStools – Efficient LiDAR Processing Software.’ (rapidlasso GmbH: Gilching, Germany)
Jafari R, Lewis MM, Ostendorf B (2007) Evaluation of Vegetation Indices for Assessing Vegetation Cover in Southern Arid Lands in South Australia. The Rangeland Journal 29, 39–49.
| Evaluation of Vegetation Indices for Assessing Vegetation Cover in Southern Arid Lands in South Australia.Crossref | GoogleScholarGoogle Scholar |
KTLA (2014) ‘Karajarri Healthy Country Plan 2013 – 2023: Palanapayana Tukjana Ngurra.’ (Karajarri Traditional Lands Association: Bidyadanga, WA)
Li Y, Quan X, Liao Z, He B (2021) Forest Fuel Loads Estimation from Landsat ETM+ and ALOS PALSAR Data. Remote Sensing 13, 1189
| Forest Fuel Loads Estimation from Landsat ETM+ and ALOS PALSAR Data.Crossref | GoogleScholarGoogle Scholar |
Lundie-Jenkins G (1993) Ecology of the Rufous Hare-Wallaby, Lagorchestes hirsutus Gould (Marsupialia : Macropodidae) in the Tanami Desert, Northern Territory. I Patterns of Habitat Use. Wildlife Research 20, 457–475.
| Ecology of the Rufous Hare-Wallaby, Lagorchestes hirsutus Gould (Marsupialia : Macropodidae) in the Tanami Desert, Northern Territory. I Patterns of Habitat Use.Crossref | GoogleScholarGoogle Scholar |
Lymburner L (2021) Geoscience Australia Landsat Fractional Cover Collection 3. [Dataset] Geoscience Australia. Available at http://pid.geoscience.gov.au/dataset/ga/145498
Macfarlane C, Hoffman M, Eamus D, Kerp N, Higginson S, McMurtrie R, Adams M (2007) Estimation of Leaf Area Index in Eucalypt Forest Using Digital Photography. Agricultural and Forest Meteorology 143, 176–188.
| Estimation of Leaf Area Index in Eucalypt Forest Using Digital Photography.Crossref | GoogleScholarGoogle Scholar |
Marsett R, Qi J, Heilman P, Biedenbender S, Watson C, Amer S, Weltz M, Goodrich D, Marsett R (2006) Remote Sensing for Grassland Management in the Arid Southwest. Rangeland Ecology & Management 59, 530–540.
| Remote Sensing for Grassland Management in the Arid Southwest.Crossref | GoogleScholarGoogle Scholar |
MNRAC (2012) ‘Birriliburu Indigenous Protected Area Plan for Country.’ (Mungarlu Ngurrarankatja Rirraunkatja Aboriginal Corporation and Central Desert Services: Perth, Australia)
Moore J, Cooper T, Webb T, Kanowski J (2018) Newhaven Wildlife Sanctuary: 2018 Fire Report. (Australian Wildlife Conservancy)
Moseby K, Read J, McLean A, Ward M, Rogers DJ (2016) How High Is Your Hummock? The Importance of Triodia Height as a Habitat Predictor for an Endangered Marsupial in a Fire-Prone Environment. Austral Ecology 41, 376–389.
| How High Is Your Hummock? The Importance of Triodia Height as a Habitat Predictor for an Endangered Marsupial in a Fire-Prone Environment.Crossref | GoogleScholarGoogle Scholar |
Muir J, Schmidt M, Tindall D, Trevithick R, Scarth P, Stewart J (2011) ‘Field Measurement of Fractional Ground Cover: A Technical Handbook Supporting Ground Cover Monitoring for Australia’. (Eds Australian Collaborative Land Use Mapping Program and Australian Bureau of Agricultural and Resource Economics and Sciences) (ABARES: Canberra)
Pianka E (1996) Long-Term Changes in Lizard Assemblages in the Great Victoria Desert: Dynamic Habitat Mosaics in Response to Wildfires. In ‘Longterm Studies of Vertebrate Communities’. (Eds M Cody, J Smallwood) pp. 191–216. (Academic: San Diego)
| Crossref |
Polat N, Uysal M (2018) An Experimental Analysis of Digital Elevation Models Generated with Lidar Data and UAV Photogrammetry. Journal of the Indian Society of Remote Sensing 46, 1135–1142.
| An Experimental Analysis of Digital Elevation Models Generated with Lidar Data and UAV Photogrammetry.Crossref | GoogleScholarGoogle Scholar |
Qarallah B, Al-Ajlouni M, Al-Awasi A, Alkarmy M, Al-Qudah E, Bani Naser A, Al-Assaf A (2021) Evaluating Post-Fire Recovery of Latroon Dry Forest Using Landsat ETM+, Unmanned Aerial Vehicle and Field Survey Data. Journal of Arid Environments 193, 104587
| Evaluating Post-Fire Recovery of Latroon Dry Forest Using Landsat ETM+, Unmanned Aerial Vehicle and Field Survey Data.Crossref | GoogleScholarGoogle Scholar |
R Core Team (2021) ‘R: A Language and Environment for Statistical Computing.’ (R Foundation for Statistical Computing: Vienna, Austria) Available at https://www.R-project.org/
Rampant P, Zdunic K, Burrows N (2019) UAS and Landsat Imagery to Determine Fuel Condition for Fire Behaviour Prediction on Spinifex Hummock Grasslands of Arid Australia. International Journal of Remote Sensing 40, 9126–9139.
| UAS and Landsat Imagery to Determine Fuel Condition for Fire Behaviour Prediction on Spinifex Hummock Grasslands of Arid Australia.Crossref | GoogleScholarGoogle Scholar |
Rodman KC, Veblen TT, Battaglia MA, Chambers ME, Fornwalt PJ, Holden ZA, Kolb TE, Ouzts JR, Rother MT (2020) A Changing Climate is Snuffing Out Post-Fire Recovery in Montane Forests. Global Ecology and Biogeography 29, 2039–2051.
| A Changing Climate is Snuffing Out Post-Fire Recovery in Montane Forests.Crossref | GoogleScholarGoogle Scholar |
Ruscalleda-Alvarez J, Moro D, van Dongen R (2021) A Multi-Scale Assessment of Fire Scar Mapping in the Great Victoria Desert of Western Australia. International Journal of Wildland Fire 30, 886–898.
| A Multi-Scale Assessment of Fire Scar Mapping in the Great Victoria Desert of Western Australia.Crossref | GoogleScholarGoogle Scholar |
Schroeder W, Oliva P, Giglio L, Quayle B, Lorenz E, Morelli F (2016) Active Fire Detection Using Landsat-8/OLI Data. Remote Sensing of Environment 185, 210–220.
| Active Fire Detection Using Landsat-8/OLI Data.Crossref | GoogleScholarGoogle Scholar |
Southgate R, Carthew S (2007) Post-Fire Ephemerals and Spinifex-Fuelled Fires: A Decision Model for Bilby Habitat Management in the Tanami Desert, Australia. International Journal of Wildland Fire 16, 741–754.
| Post-Fire Ephemerals and Spinifex-Fuelled Fires: A Decision Model for Bilby Habitat Management in the Tanami Desert, Australia.Crossref | GoogleScholarGoogle Scholar |
Thackway R, Cresswell I (1995) ‘An Interim Biogeographic Regionalisation for Australia: A Framework for Setting Priorities in the National Reserves System Cooperative Program.’ (Australian Nature Conservation Agency, Reserve Systems Unit: Canberra)
Tucker C (1979) Red and Photographic Infrared Linear Combinations for Monitoring Vegetation. Remote Sensing of Environment 8, 127–150.
| Red and Photographic Infrared Linear Combinations for Monitoring Vegetation.Crossref | GoogleScholarGoogle Scholar |
Turner D, Ostendorf B, Lewis M (2008) An Introduction to Patterns of Fire in Arid and Semi-Arid Australia, 1998–2004. The Rangeland Journal 30, 95–107.
| An Introduction to Patterns of Fire in Arid and Semi-Arid Australia, 1998–2004.Crossref | GoogleScholarGoogle Scholar |
van Dongen R, Huntley B, Keighery G, Brundrett M (2019) Monitoring Vegetation Recovery in the Early Stages of the Dirk Hartog Island Restoration Programme Using High Temporal Frequency Landsat Imagery. Ecological Management & Restoration 20, 250–261.
| Monitoring Vegetation Recovery in the Early Stages of the Dirk Hartog Island Restoration Programme Using High Temporal Frequency Landsat Imagery.Crossref | GoogleScholarGoogle Scholar |
van Dongen R, Ruscalleda Alvarez J, Sinclair K, Zdunic K (2021) ‘Great Victoria Desert Fire Scar Mapping Report 2020-21.’ (Department of Biodiversity, Conservation and Attractions: Perth, WA)
Villarreal ML, Norman LM, Buckley S, Wallace CSA, Coe MA (2016) Multi-Index Time Series Monitoring of Drought and Fire Effects on Desert Grasslands. Remote Sensing of Environment 183, 186–197.
| Multi-Index Time Series Monitoring of Drought and Fire Effects on Desert Grasslands.Crossref | GoogleScholarGoogle Scholar |
Werner CM, Harrison SP, Safford HD, Bohlman GN, Serata R (2022) Extreme Pre-Fire Drought Decreases Shrub Regeneration on Fertile Soils. Ecological Applications 32, e02464
| Extreme Pre-Fire Drought Decreases Shrub Regeneration on Fertile Soils.Crossref | GoogleScholarGoogle Scholar |
Wilson EH, Sader SA (2002) Detection of Forest Harvest Type Using Multiple Dates of Landsat TM Imagery. Remote Sensing of Environment 80, 385–396.
| Detection of Forest Harvest Type Using Multiple Dates of Landsat TM Imagery.Crossref | GoogleScholarGoogle Scholar |
Wright BR, Laffineur B, Royé D, Armstrong G, Fensham RJ (2021) Rainfall-Linked Megafires as Innate Fire Regime Elements in Arid Australian Spinifex (Triodia spp.) Grasslands. Frontiers in Ecology and Evolution 9, 666241
| Rainfall-Linked Megafires as Innate Fire Regime Elements in Arid Australian Spinifex (Triodia spp.) Grasslands.Crossref | GoogleScholarGoogle Scholar |
Wright M, Ziegler A (2017) Ranger: A Fast Implementation of Random Forests for High Dimensional Data in C++and R. Journal of Statistical Software 77, 1–17.
| Ranger: A Fast Implementation of Random Forests for High Dimensional Data in C++and R.Crossref | GoogleScholarGoogle Scholar |
Wulder MA, Masek JG, Cohen WB, Loveland TR, Woodcock CE (2012) Opening the Archive: How Free Data Has Enabled the Science and Monitoring Promise of Landsat. Remote Sensing of Environment 122, 2–10.
| Opening the Archive: How Free Data Has Enabled the Science and Monitoring Promise of Landsat.Crossref | GoogleScholarGoogle Scholar |
Young M, Andrews J, Caldwell T, Saylam K (2017) Airborne LiDAR and Aerial Imagery to Assess Potential Burrow Locations for the Desert Tortoise (Gopherus agassizii). Remote Sensing 9, 458
| Airborne LiDAR and Aerial Imagery to Assess Potential Burrow Locations for the Desert Tortoise (Gopherus agassizii).Crossref | GoogleScholarGoogle Scholar |
Zhao Y, Liu X, Wang Y, Zheng Z, Zheng S, Zhao D, Bai Y (2021) UAV-Based Individual Shrub Aboveground Biomass Estimation Calibrated against Terrestrial LiDAR in a Shrub-Encroached Grassland. International Journal of Applied Earth Observation and Geoinformation 101, 102358
| UAV-Based Individual Shrub Aboveground Biomass Estimation Calibrated against Terrestrial LiDAR in a Shrub-Encroached Grassland.Crossref | GoogleScholarGoogle Scholar |