Optimising disaster response: opportunities and challenges with Uncrewed Aircraft System (UAS) technology in response to the 2020 Labour Day wildfires in Oregon, USA
Dae Kun Kang A * , Erica Fischer A , Michael J. Olsen A , Julie A. Adams B and Jarlath O’Neil-Dunne CA
B
C
Abstract
The expanding use of Uncrewed Aircraft System (UAS) technology in disaster response shows its immense potential to enhance emergency management. However, there is limited documentation on the challenges and data management procedures related to UAS operation.
This manuscript documents and analyses the operational, technical, political, and social challenges encountered during the deployment of UAS, providing insights into the complexities of using these technologies in disaster situations.
This manuscript documents and analyses the operational, technical, political, and social challenges encountered during the deployment of UAS, providing insights into the complexities of using these technologies in disaster situations.
UAS technology plays a significant role in search and rescue, reconnaissance, mapping, and damage assessment, alongside notable challenges such as extreme flying conditions, data processing difficulties, and airspace authorisation complexities.
The study concludes with the need for updated infrastructure standards, streamlined policies, and better coordination between technological advancements and political processes, emphasising the necessity for reform to enhance disaster response capabilities.
The findings of this study inform future guidelines for the effective and safe use of UAS in disaster situations, advocating for a bridge between state-of-the-art UAS research and its practical application in emergency response.
Keywords: disaster response, drone, hazards, Labour Day fire, Oregon, UAS, UAV, Uncrewed Aircraft System, wildfire.
Introduction
Uncrewed Aircraft System (UAS) technology can be used for several purposes before, during, and after a wide range of disaster situations. In the United States, for example, UAS use in disaster response dates back to 2005, when the University of South Florida Centre for Robot-Assisted Search and Rescue (CRASAR) used small fixed-wing and helicopter-style UAS to search for Hurricane Katrina survivors in Mississippi (Greenwood et al. 2020). At that time, the use of UAS in disaster response was very limited, given concerns that the UAS could disrupt occupied aircraft flights necessary for the response (Murphy 2015). Additionally, a certificate of authorisation (COA) was required to operate a UAS in a disaster situation. A COA was difficult to obtain and required the team operating the UAS to follow strict protocols (Cross 2015).
Many of these barriers have been resolved over the past 15 years (Gupta et al. 2013) as research on the use of UAS in disaster situations progressed and as lightweight sensing technologies, communication relays, and aerial mapping technologies have been developed. The Federal Aviation Administration (FAA) in 2006, in response to these changes, issued the Small UAS Rule (Part 107) as a new regulatory framework for the use of UAS or commercial, non-hobby purposes (Ancel et al. 2017). Part 107 enabled much broader UAS operations for commercial, governmental, and academic purposes. These technological and policy developments created an environment where UAS can be more readily used in disaster situations.
Building on the advancements in UAS technology and policy that have significantly improved their application in disaster scenarios, UAS play a crucial role in addressing the challenges posed by wildfires. Worldwide, wildfires are rapidly amplifying in scale and intensity threatening wildlife, humans, vital infrastructure, and natural resources (Mueller et al. 2020). To help combat these issues, government institutions and firefighters used sophisticated resources and technologies in their attempts to control the unpredictable and rapidly spreading wildfires. Specifically, the use of UAS technology in wildfire response is increasing due to its flexibility, ease of deployment, efficiency, and ability to offer unique vantage points (Gonzalez et al. 2016). UAS can provide data on wildfire-impacted areas that are inaccessible to humans, directly or indirectly help rescue lives, and can help to establish a post-disaster damage assessment and recovery plan.
Although several studies document the use of UAS technology in disaster response, most studies focus on the technological and tactical aspects, such as the sensors for a specific application. Relatively few studies document how actual disaster-related organisations use UAS and the practical challenges they face during operation (Erdelj et al. 2017; Rosser Jr et al. 2018; Jones and Despotou 2019; Cho et al. 2020; Greenwood et al. 2020; Sivasuriyan 2021; Daud et al. 2022; Ojetunde et al. 2022). Thus, the objectives of this study are to: (1) identify current UAS utilisation, applications, infrastructure, and standards; (2) capture and qualitatively analyse information obtained through detailed interviews; (3) document the technical, political, and social challenges that arise when attempting to use UAS technology to aid in wildfire response; and (4) serve as an essential foundational study to create a framework for safe, effective, and systematic UAS utilisation for wildfire mitigation, response, and recovery. These objectives will be met by exploring UAS usage in the 2020 Labour Day fires in Oregon.
Background
Exploring the use of UAS in different disaster scenarios provides a comprehensive perspective to provide context to this study focused on wildfire. Hence, this section presents trends in current UAS use in disaster situations in the broad sense. The advantages UAS offer over traditional remote sensing technologies, such as occupied aircraft and satellite platforms, led to their rapid adoption and implementation for disaster response and recovery (Wartman et al. 2020). This section describes select examples of how UAS technology has been deployed in recent responses to disasters, along with the potential opportunities (Table 1). Amongst all possible disaster events, the intent was to focus on select disasters (e.g. earthquakes, tsunamis, wildfires, and hurricanes) to provide sufficient information presenting a broad view of how UAS technology is used. Detailing all possible natural or anthropogenic hazard that has used UAS technology is beyond the scope of this manuscript.
Disaster type | Application | Example UAS uses | |
---|---|---|---|
Earthquake | RM |
| |
DA |
| ||
MD |
| ||
Other |
| ||
Tsunami | SAR |
| |
RM |
| ||
DA |
| ||
Other |
| ||
Hurricane | RM |
| |
DA |
| ||
MD |
| ||
Wildfire | SAR |
| |
RM |
| ||
DA |
| ||
MD |
| ||
Other |
|
Earthquake
Following significant earthquake events, Rathje and Franke (2016) used UAS to collect 2D orthophotos, video logs, or 3D geometric information to document and quantify damage patterns throughout an impacted city, capture detailed geometric information on failed infrastructure for forensic information, and map and measure ground movements to evaluate stability and further risk. Although often not available, high-quality baseline data from pre-disaster UAS flights can significantly improve quantitative damage analysis after disasters (Restas 2015). In many cases, researchers and disaster responders used lower-spatial resolution satellite data for baseline reference, given its wider availability. These data are essential to capture the initial damage from the actual event, while repeat flights can efficiently monitor changes to the ground surface, slopes, or infrastructure after the earthquake or associated aftershocks. Several recent events demonstrated these applications. For example, following the 2016 Kumamoto, Japan earthquake, Yamazaki et al. (2017) used UAS data to develop 3D models based on the Structure-from-Motion (SfM) reconstruction technique to support identification and quantification of damage throughout the affected area. Montgomery et al. (2021) also used UAS technology for reconnaissance to obtain high resolution imagery of the significant damage observed throughout Palu City and the surrounding Central Sulawesi region of Indonesia following the 2018 Palu-Donggala earthquake. The use of UAS for this application enabled rapid capture of information across a large flowside area efficiently, particularly for areas that are difficult, if not impossible, to access directly by humans to perform conventional surveying and mapping.
Tsunami
UAS have been used to collect data on the inundation extents following tsunamis as well as to generate detailed digital elevation model topographic information to simulate the substantial fluctuations in water levels when a tsunami occurs. For planning purposes, Marfai et al. (2019) used high-resolution UAS imagery and GIS software for developing disaster response and mitigation plans as well as evacuation plans by estimating areas likely to be affected by tsunamis based on scenario events. Similar to earthquakes, UAS technology is also used for damage assessment following a tsunami. For example, De Oliveira et al. (2018) used a UAS equipped with an infrared camera to detect faults in large-scale Photovoltaic plants in tsunami-affected areas. The UAS was very beneficial in terms of cost-efficiency and its ability to detect post-tsunami damage rapidly. UAS also has the potential to be used in direct lifesaving applications. Katayama et al. (2018) explored the usage of UAS technology to provide immediate evacuation guidance to persons in tsunami hazard zones. In this application, multiple UAS coordinate and continuously share information such as their location, evacuation guidance routes, and the number of people each system has guided.
Hurricane
UAS played an important role in supporting reconnaissance of hurricane-impacted areas by enabling quick map generation of heavily impacted areas. Yuan and Liu (2018) devised a framework that combines UAS with social media to quickly deploy UAS to affected areas in order to obtain timely information for response planning. Hurricane affected areas are generally extensive; hence, UAS can prove valuable to determine the extent of damage and plan the recovery efforts compared with deploying humans to the impacted sites. When compared to reliable baseline data (e.g. orthophotos; lidar digital elevation models, DEMs), remotely sensed data from UAS can serve as a low-cost tool to accurately quantify topographical changes resulting from hurricanes. For example, after Hurricane Maria, Schaefer et al. (2020) investigated changes within a damaged area by comparing UAS imagery to aerial photographic data collected before the storm. Notably, given that the UAS is relatively vulnerable to wind and rain, it has primarily been used for post-disaster damage assessment rather than directly during the event (Yeom et al. 2019). UAS usage is limited during hurricane events and in the immediate aftermath for SAR purposes until environmental conditions permit safe UAS operation.
Wildfire
UAS are being widely used and studied as a potential tool to detect wildfires. Early wildfire detection is very important to help prevent large-scale fires, which can grow rapidly. Current fire detection technologies based on satellite imaging or remote cameras tend to be slow to detect fires and have lower accuracy (Bushnaq et al. 2021). Kanand et al. (2020) used UAS equipped with high-resolution RGB and thermal cameras and a modern 5G mobile network infrastructure. They strategically deployed this for early detection of wildfires, enhancing both the speed and accuracy of detection. UAS have demonstrated remarkable capabilities, extending beyond the early detection of wildfires to include identifying and mapping burned areas. Samiappan et al. (2019) compared the UAS-based classification produced from the normalised difference vegetation index (NDVI) and a digital surface model (DSM) with the Landsat-based Burned Area Reflectance Classification (BARC). The authors noted that a UAS platform with a multispectral sensor could provide more timely data for mapping the extent of the burned area than an RGB photogrammetric camera alone.
UAS technology proves effective for supporting a variety of monitoring and detection tasks, most of which are performed manually by human observers watching video feeds from the UAS (Kanand et al. 2020; Tang et al. 2020). Researchers are currently exploring deep learning methods using UAS imagery to rapidly detect and monitor wildfires (Tang et al. 2020, Kang et al. 2023). Although capable of very efficient and promising data analysis, machine learning, more specifically deep learning, require extensive image data sets that are manually annotated (e.g. fire) in order to train a model to identify similar features in other images (Zhao et al. 2018). Hence, a hindrance to this approach will be the robust collection and systematic annotation of the very large dataset necessary to detect wildfires accurately. However, once sufficient datasets are available, deep learning models can automate the detection of fires in imagery, enabling responders to use their time more effectively in responding to priority locations.
During the event, UAS also transport and drop fire suppression materials (e.g. fire retardant, water, or other extinguishing agents) at strategic locations (Saikin et al. 2020). The UAS enables the fire to be extinguished in a safer method than the conventional crewed helicopter method, which puts people directly in harm’s way by flying over the flames and smoke from the fire. By incorporating infra-red camera technology on UAS, wildfire management teams can detect thermal energy and produce images highlighting heat signatures. This capability is critical for identifying hotspots along the perimeter of a fire zone, enhancing the safety and strategic deployment of firefighting crews (Fagen et al. 2021).
Materials and methods
Study area
This study explores UAS usage in preparation for, during, and in response to the 2020 Labour Day Fires in Oregon, USA (Fig. 1), which initiated on 7 September 2020. These fires were some of the most catastrophic on record in the State of Oregon, killing at least 11 people, burning more than 1 million acres of land, and causing more than 40,000 people to flee their homes (Oregon Office of Emergency Management 2020). Additionally, the fires had significant economic impacts, including a USD5.9 billion loss for Oregon’s forest-dependent industries and businesses as well as recovery costs estimated at USD1.15 billion for the state (Oregon Office of Emergency Management 2021; Tillamook Headlight Herald 2021).
Map of the extent of major fires occurring during the 2020 Labour Day Fires in Oregon, USA along with approximate numbers of damaged structures and acreage. (Data sources: NWS Billings, National Institution Fire Centre (NIFC) geodata, and Incident Information system’s (Inciweb) daily fire reports.)
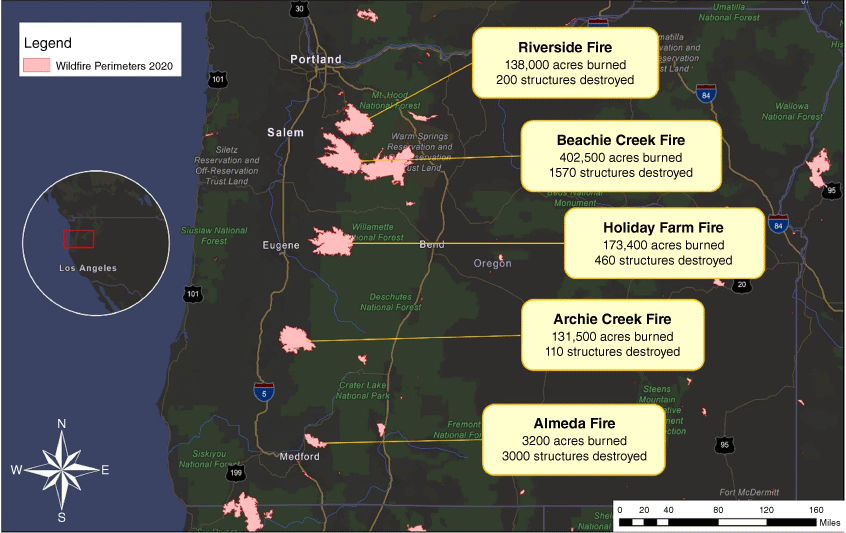
Interview research methodology
Fig. 2 shows an overview of the interview research methodology and how we synthesised the results. We used semi-structured interviews to collect information on UAS usage by institutions in response to the 2020 Labour Day fires. The general goal of a semi-structured interview is to gather systematic information about a set of central topics while also allowing flexibility for exploration when new issues or topics emerge (Longhurst 2009; Wilson 2014). Considering that the purpose of the interviews in this study is to collect information on the use of UAS in wildfire response and associated challenges derived from the situations and experiences of each organisation and expert, the flexibility of the semi-structured interview approach is well-suited for this study given the limited amount of information currently available.
Overview of the research methodology for the application of UAS for disaster response. The topics in the centre column were used to guide the semi- structured interviews.
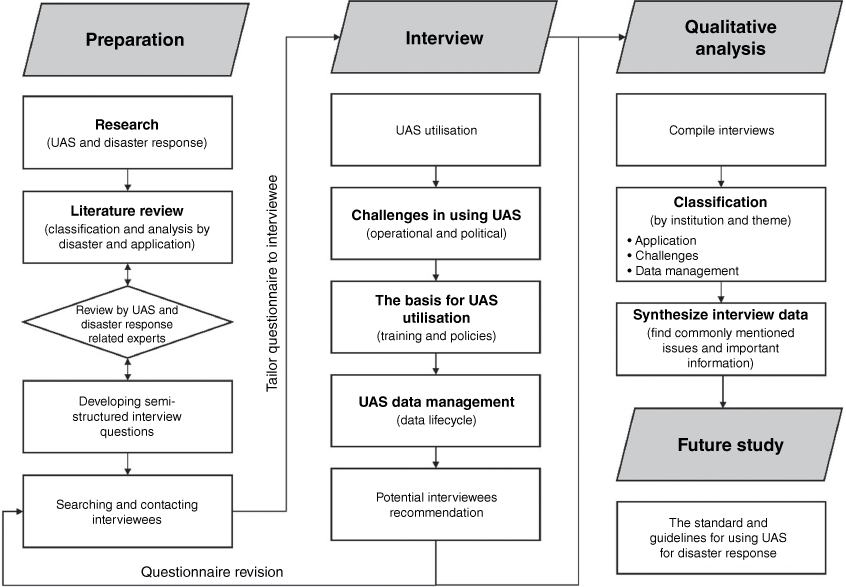
We generated a questionnaire to guide the conversation and address the four aforementioned research objectives. The questionnaire consisted of open-ended questions with prompts for responses when needed. The literature review explored UAS utilisation in disaster questions (Table 1), which enabled the creation of categories for common UAS use methods (Search and Rescue, SAR; Reconnaissance and Mapping, RM; Damage Assessment, DA; Monitoring and Detection, MD). These categories then informed the questionnaire process, enabling the development of more specific questions tailored to the situations in which UAS was used. Synthesising the limitations and further research mentioned in studies, we formulated specific questions to identify the challenges of using UAS in disaster situations, which led to in-depth discussions with respondents. Another important outcome of the literature review was the identification of possible gaps between research and real-world challenges regarding using UAS in disaster situations. Through these discussions with experts, we generated questions about operational and political challenges as well as data management that were not addressed in much of the literature. Given the limited potential number of respondents with UAS experience, we used snowball sampling for participant recruitment (Goodman 1961). After each interview, we revised the questionnaire to tailor questions to the target audience based on input received from the prior interviewee(s). Oregon State University’s Institutional Review Board (IRB) reviewed the questionnaire and associated interview methodology and rendered an exempt decision based on the determination that it was not classified as human subject research.
We employed several methods to identify potential interviewees consisting of government officials and UAS experts involved in wildfire response. First, we identified potential interviewees through the collaboration networks of the authors and their colleagues. The research team then extensively searched media articles to identify key personnel at agencies involved in the response. Second, we explored public agency websites to identify additional potential respondents. If no clear candidate was identified on the webpage, we contacted the heads of relevant divisions to identify suitable personnel to participate in the interviews. Lastly, we asked interviewees to provide the names of other potential respondents.
We conducted each interview as a web-based video conference lasting between 40 and 60 min. The interview questions generally followed the order are in Fig. 2, but were adapted based on the conversation’s flow. We first asked questions about the use of UAS, in general, within the respondent’s institution and their use in wildfire situations. Through this question, the authors tried to understand each institution’s current UAS uses and applications. The authors then asked if there were any problems or challenges that could be improved in the processes for using UAS. This question aimed to identify reasons limiting UAS usage. Finally, we discussed UAS-related policies, training resources, as well as UAS data management and sharing. Some respondents provided follow-up materials, such as presentations and documents, to be reviewed by the research team. Following the interviews, we transcribed key information based on interview notes and recordings. At least two research team members participated in the interviews to reduce potential bias in transcription and interpretation. We also employed a process where multiple team members carefully reviewed the transcriptions to ensure consistency and objectivity in reporting key information.
We applied qualitative analysis methodology to the semi-structured interview results in order to explore how they used UAS before and after wildfire disasters, what were the major barriers to their use, and how they managed the collected UAS data. We integrated each respondent’s response to each question. Lastly, we reclassified the integrated data according to the three pre-determined themes: (1) application; (2) challenges; (3) and data management), with three sub-themes: (1) timeline; (2) operational and political; (3) and data lifecycle. This process identifies common information mentioned by several institutions and information unique to a particular respondent.
Results and discussion
We contacted a total of 25 institutions and 32 people. We were able to interview eight of them from five institutions to determine how they used UAS in response to the wildfire. Most of the institutions declined because they were not aware of any UAS usage for the 2020 Labour Day wildfire by their institution. As much as possible, we conducted the interviews during the ‘off-season’ to improve responder availability. Although the Oregon 2020 Labour Day wildfire danger had receded in October 2020, the potential respondents remained busy throughout the year with continued wildfire response work or preparation for an anticipated substantial 2021 wildfire season. We classified the data obtained through these interviews into three general categories for the qualitative analysis: (1) UAS application; (2) challenges; and (3) data management practices.
UAS applications
Respondents highlighted UAS deployments that occurred before, during, and after the disaster situation. Table 2 shows the utilisation of UAS in wildfires by each institution organised within the context of the event timeline. Agency A was the only institution that indicated the use of UAS before wildfires to identify vulnerable areas and gather image data on these areas. They used these baseline data to prepare for wildfires through strategic mitigation practices.
During wildfire response, the usage of UAS was diverse. Many institutions mentioned that UAS may replace conventional helicopter operations as UAS can perform missions more safely and efficiently. Agency C used UAS for aerial ignition purposes when firefighter safety on the ground was compromised or when large land areas required ignition to slow and help contain the wildfire. Aerial ignition requires flying low to the ground and at a slow speed while igniting the fire. This method of flying is risky and can cause accidents using a helicopter. UAS can substantially reduce the risk. UAS paired with infrared cameras can capture high-resolution photographs during a wildfire. Due to safe flying altitudes, most crewed aircraft will obtain photos with smoke; however, a UAS can fly at significantly lower altitudes above ground and obtain the necessary photos to understand where burning and combustion are occurring. Agencies B and C used UAS for response management through real-time videos and mapping.
After the fires were contained, there was considerable UAS use across the different institutions. All institutions used UAS to collect imagery to evaluate the extent and type of damage resulting from the wildfires. They used vulnerable regions (communities and forested land), the pre-fire imagery for comparison purposes. Agency A used UAS post-wildfire to monitor emissions and community activity to determine which regions are safe for on-the-ground crews to commence the forest restoration processes. Agency D used UAS after a fire to determine where trees threaten the right of way on roadways. UAS imagery showed where trees were significantly compromised and which trees needed to be removed, such that transportation through wildfire-impacted regions is not compromised. Agency E was also conducting an ongoing study using multispectral imagery obtained by UAS to categorise the conditions of trees. Currently, these data are acquired using ground-based lidar; however, a UAS with kinematic lidar is expected to expedite this work and protect crew members. During this evaluation of trees for removal, Agency D also used UAS to monitor and rescue osprey baby birds living in the burn zone. A nest had been constructed on top of a burned, which needed to be removed for safety reasons given its proximity to the highway. The UAS minimised disturbing the osprey by obtaining nest imagery, counting how many baby ospreys were in the nest, and safely removing the nest from the unstable tree.
In addition to government institutions, Agency E, whose primary role is to assist the natural hazards and disaster research communities in collecting perishable data in post-natural disaster reconnaissance. Agency E used UAS to quickly investigate the extent of the wildfire impacted area and produce detailed orthomosaic maps for future disaster monitoring, change detection, debris removal work, and geotechnical assessment of fire-affected structures and landscapes. Note that Agency E is not a first responder performing direct lifesaving or fire suppression tasks. Instead, they used the UAS to obtain research-related data for post-fire damage assessment and disaster recovery.
Challenges in using UAS
As mentioned in the UAS utilisation section, most institutions noted many positive roles for UAS in wildfire situations. However, there were also challenges cited by each institution when using UAS during and after the 2020 Labour Day fires. We classified these challenges into two categories: operational and political.
Table 3 shows the operational challenges in using UAS. Many of the operational challenges are common across institutions. The operational challenges mentioned during the interviews occurred from flying conditions, data processing, and communication. Instability from strong winds was a challenge mentioned by all institutions. In wildfire situations, high temperatures and strong winds pose challenges to a UAS attempting to fly at a constant altitude for data collection. Agency C mentioned maintaining up-to-date software as a particular challenge as outdated UAS-specific software can lead to data acquisition and/or processing errors. On a related note, Agency D had enough personnel to gather the data in the field; however, they had insufficient personnel to support the associated data processing needs. Additionally, Agency D had recently purchased a UAS lidar system, but at the time of the interview, they did not have personnel trained to process the UAS lidar sensor data.
Operational Challenges | Institution | Explanation | |
---|---|---|---|
Strong wind (stability of UAS) | Agency A, B C, D, F | ||
Flight height restrictions (<122 m) and the line of sight | Agency A, B C, D, E | ||
Outdated software (platform) problem | Agency C | ||
Lack of data processing experts and UAS lidar sensor | Agency D | ||
Communication standards | Agency C | ||
Introduction of new technologies and issues of efficiency | Agency A |
Operating under Part 107, Agency D requires the pilot in control to maintain a line of sight with the UAS at all times during a flight, which is also related to a political challenge discussed in the next section. According to FAA Part 107, the maximum allowable altitude is 122 m (400 feet) above the ground, which can significantly hinder obtaining imagery capturing the overall damage experienced within an area, particularly in locales with steep slopes and substantial terrain variation. Such rugged terrain is common in wildfire prone areas in the western US. Regarding this issue, Agency E suggested possible refinement of the definition of 122 m (400 feet) restricted area based on the general terrain height as a potential solution. Currently, flight restrictions are also applied in a circular fashion surrounding an airport area, even if UAS are not within common paths of the crewed aircraft at the airport. Agency E provided suggestions to improve operability near low-volume airports. For example, a more detailed no-fly, or restricted flight zone based on the crewed aircraft’s routes can allow responders to safely work in areas that do not conflict with the crewed aircraft’s flight paths.
Agency C also discussed field operation communication challenges. Communication issues can arise when both UAS and crewed aircraft are flying in the same space at the same time. UAS and crewed aircraft have two different communication standards and extensive standards on how to communicate individually; however, there is a lack of standards or protocols on how to communicate between these two different aircraft. This challenge impedes effective response in wildfire situations and can result in additional safety concerns.
Table 4 shows political challenges mentioned many times by each institution. Experts from Agencies A, B, C, D, and E mentioned that UAS procedures may be a hindrance to using UAS during or after a disaster, particularly during a rapidly changing event such as wildfire, due to the complexity and restrictions imposed by these procedures. All institutions acknowledged the appropriateness of these restrictions around airports. Nevertheless, Agency D mentioned that altitude restrictions hinder data collection in areas with large terrain elevation changes. In special circumstances, the FAA may temporarily restrict access to certain designated airspace for the response, called Temporary Flight Restrictions (TFR). This can be a problem if institutions need to use a type 1 or 2 UAS (UAS over 11.3 kg (25 lbs)). Most type 1 and 2 UAS are not owned by Agency B and are used via contracts with general institutions. Thus, a rapid response will require TFR permissions, and the FAA can require a considerable time to issue these permissions. Many institutions have also mentioned airspace authorisation as a related challenge. Prior to flight, institutions must send a request to obtain airspace authorisation, and most are automatically accepted. However, requests for additional coordination are sometimes rejected or receive no response without further explanation. Considering the nature of disaster response, these delays can increase the likelihood that critical information will not be obtained in time, which can hinder the response.
Political challenges | Institution | Explanation | |
---|---|---|---|
Flight height restrictions (<122 m) | Agency A, B C, D, E | ||
Receiving airspace authorisation | Agency A, B C, E | ||
Temporary flight restriction (TFRs) | Agency B | ||
Exclusive use contracts & Pre training and practice | Agency C | ||
Technological progress outweighed political progress | Agency A, B C, D | ||
Temporal cessation of UAS-related training | Agency C | ||
Privacy issues Security issues for critical areas | Agency A, F |
The second political and social challenge theme is the use of contracts. Institutions, such as Agency C, do not own UAS fleets. Thus, after a wildfire, Agency C will develop an exclusive use contract with a private company. However, because institutions such as Agency C do not consistently work with these companies, they must discuss the scope of work and details each time, ultimately delaying the deployment of UAS and data collection. Agency C is considering remedying this issue by conducting mission reviews and training together with the private contractors in advance of the wildfire season via advance contracting.
The last political and social challenge theme is that technological progress is outpacing political progress. For example, in a mission to classify and remove hazardous trees after the 2020 Labour Day fires, Agency D knew the effectiveness and benefits of data capture using a UAS with lidar sensor payload, but they were unable to use it because there was no official approval from higher authorities. A careful review and verification process is essential when introducing new technology to ensure effective use. However, if this process and technological progress are not balanced, it becomes a hindrance to the use of UAS technology, particularly in the case of wildfires where the response situation changes rapidly. However, these institutions have a rigorous certification process for the aerial fire community, which can certify the reliability of new technology. The COVID-19 pandemic presented many challenges; however, the biggest barrier was the suspension of training. This reduced training was evident when trying to use UAS during and after the 2020 Labour Day fires because, compared to normal operational times, they did not have sufficient institution personnel training.
UAS data management
UAS technology can produce various data types for applications in wildfire response, from basic images to videos to purpose-built maps. The efficiency of a UAS also tends to result in substantial data volumes. Interviewees discussed how they handle UAS data in each institution, particularly from a data-sharing perspective. Notably, most institutions faced similar concerns about data management and sharing, including the difficulty of sharing across institution boundaries and refreshing data with the most up-to-date information. These concerns are summarised in the data lifecycle (Fig. 3), which refers to the sequence of processes from data creation, storage, use, sharing, archival, and destruction.
Most interviewees mentioned the critical need for standards and specific criteria throughout the data life cycle. For example, Agency C noted the need for protocols to distinguish which data are classified as federal data and which are public data. This determination can be ambiguous. Agency C also noted challenges in determining which data to archive as a formal record and which data to dispose of whose purpose was to inform the immediate situation and was soon outdated. Attempting to archive all data are a substantial burden on personnel to provide all necessary metadata to comply with federal data sharing standards. It also creates bottlenecks and confusion in the reuse of the data in the future, given the large data volume users must sift through to locate the data of interest, ultimately causing the most important information to be lost in the vast data repositories.
A consistent data storage format is necessary for effective (and, in some cases, legal) data sharing. However, some institutions, such as Agencies A and C, do not have standards in place. One interviewee noted that this lack of standardisation poses difficulty in sharing data within Agency C. Agency B said that they are working on building an improved data storage system to address these issues.
Conclusion
We applied an exploratory case study methodology to investigate the uses of UAS during the 2020 Labour Day wildfires in Oregon. The primary conclusion is that while the use of UAS in disaster situations continues to increase, research on the infrastructure, standards, as well as technical and political challenges related to UAS use remains insufficient. In this study, we conducted a comprehensive investigation into the current use of UAS through a literature review and interviews with experts (Objective 1). The research commenced with a detailed literature review exploring applications of UAS in disaster response with a focus on wildfire. Building upon those insights and common threads found in the literature review, we carefully crafted a questionnaire centred around the knowledge gaps identified. We then conducted semi-structured interviews with organisations involved in managing wildfires and deploying UAS technology in the vast data repositories. The interview process was refined with each interview and feedback from the experts. These discussions explored the practical use of UAS in wildfire scenarios, seeking to identify and bridge gaps between academic research on UAS applications in disasters and the actual challenges faced in real-world situations. Through a synthesis of the interview results and literature review, we documented and organised important findings into four subject areas: (1) current UAS utilisation; (2) operational challenges; (3) political challenges; and (4) UAS-related data management (Objective 3). Several institutions effectively used the UAS technology to support their response to the Oregon 2020 Labour Day wildfires, including mapping the burn area, monitoring the wildfires, assessing the damage, and evaluating long-term impacts, such as those on wildlife and falling tree hazards. However, we identified several challenges with current FAA Part 107 requirements when operating UAS in steep, narrow canyons with substantial amounts of vegetation. We also found the absence of special disaster-related regulations or procedures. Institutions also acknowledged difficulties in managing and sharing data due to the lack of standards and specific criteria.
Overall, many wildfire responding entities have already used UAS effectively in wildfire response. Nevertheless, improvements are still needed for the application of more efficient UAS. Many institutions mentioned a variety of political restrictions on using UAS. Policy changes through coordination and communication between higher-level organisations and UAS operators based on the understanding of UAS utilisation in wildfires, rather than simple relaxation of restrictions, are needed. It will also be necessary to establish a mutually agreed-upon framework for the operation of UAS in wildfire response. Without such a framework, each institution will devise its unique methods for UAS operation, data collection, management, and sharing. This lack of a framework can reduce the efficiency of collaboration and information sharing between organisations operating UAS during a wildfire. Further studies are needed regarding regulations and organisational structures to provide a framework for safe, effective, and systematic UAS utilisation in broader disaster response situations. Despite these challenges and setbacks, the interviewees all found substantial benefits and great promise from the use of UAS based on their use when responding to the 2020 Labour Day wildfires in Oregon.
This study lays the groundwork for a comprehensive UAS framework by systematically analysing current applications, restrictions, and challenges in wildfire scenarios (Objective 4). It highlights the necessity for enhanced communication and policy reform to facilitate UAS integration into wildfire management. By identifying and documenting these subject areas, this study provides a strategic blueprint for developing a framework that ensures UAS operations are safe, effective, and synergised across different agencies for wildfire mitigation, response, and recovery.
Data availability
Data sharing is not applicable as no new data were generated or analysed during this study.
Declaration of funding
This work was funded, in part, by the Federal Aviation Administration (FAA) through the Alliance for System Safety of UAS through Research Excellence (ASSURE) Centre of Excellence under project A28: Disaster Preparedness and Emergency Response (Phase I). Dr. Jerry Hendrix (University of Alabama) served as the PI of this multi-institutional team. Any opinions or findings presented in this report are those of the authors and do not necessarily reflect the viewpoint of the FAA.
Acknowledgements
The authors appreciate the participation of each of the respondents and the insights, they shared with the research team through the interviews.
References
Aljehani M, Inoue M (2016) Multi-UAV tracking and scanning systems in M2M communication for disaster response. In ‘2016 IEEE 5th global conference on consumer electronics’, Kyoto, Japan. pp. 1–2. (IEEE) 10.1109/GCCE.2016.7800524
Allison RS, Johnston JM, Craig G, Jennings S (2016) Airborne optical and thermal remote sensing for wildfire detection and monitoring. Sensors 16(8), 1310.
| Crossref | Google Scholar | PubMed |
Alvarez LV, Moreno HA, Segales AR, Pham TG, Pillar-Little EA, Chilson PB (2018) Merging unmanned aerial systems (UAS) imagery and echo soundings with an adaptive sampling technique for bathymetric surveys. Remote Sensing 10(9), 1362.
| Crossref | Google Scholar |
Baiocchi V, Dominici D, Mormile M (2013) UAV application in post-seismic environment. International Archives of the Photogrammetry, Remote Sensing and Spatial Information Sciences XL-1/W2, 21-25.
| Crossref | Google Scholar |
Bushnaq OM, Chaaban A, Al-Naffouri TY (2021) The role of UAV-IoT networks in future wildfire detection. IEEE Internet of Things Journal 8(23), 16984-16999.
| Crossref | Google Scholar |
Cho JY, Song JI, Jang CR, Jang MY (2020) A study on the utilization plan of drone videos for disaster management. Journal of the Korea Academia-Industrial Cooperation Society 21(10), 372-378.
| Google Scholar |
Cross AR (2015) Drones for disaster response and relief operations. IssueLab, April, 1, 52. Available at https://www.issuelab.org/resources/21683/21683.pdf
Daud SMSM, Yusof MYPM, Heo CC, Khoo LS, Singh MKC, Mahmood MS, Nawawi H (2022) Applications of drone in disaster management: a scoping review. Science & Justice 62(1), 30-42.
| Crossref | Google Scholar | PubMed |
De Oliveira AKV, Aghaei M, Madukanya UE, Nascimento L, Rüther R (2018) Aerial infrared thermography of a utility-scale PV plant after a meteorological tsunami in Brazil. In ‘2018 IEEE 7th world conference on photovoltaic energy conversion (WCPEC) (A joint conference of 45th IEEE PVSC, 28th PVSEC & 34th EU PVSEC)’, pp. 684–689. (IEEE)
Derricott JC, Willis JB, Peterson CK, Franke KW, Hedengren JD (2019) Disaster reconnaissance using multiple small unmanned aerial vehicles. Mechanical Engineering 141(06), S7-S11.
| Crossref | Google Scholar |
Erdelj M, Natalizio E, Chowdhury KR, Akyildiz IF (2017) Help from the sky: leveraging UAVs for disaster management. IEEE Pervasive Computing 16(1), 24-32.
| Crossref | Google Scholar |
Fagen C, Weir JR and Payne D (2021) Using drones with infrared capabilities to monitor fire behavior | Oklahoma State University. Available at https://extension.okstate.edu/fact-sheets/using-drones-with-infrared-capabilities-to-monitor-fire-behavior.html [verified 05 February 2024]
Freeman M, Vernon C, Berrett B, Hastings N, Derricott J, Pace J, Horne B, Hammond J, Janson J, Chiabrando F, Hedengren J (2019) Sequential earthquake damage assessment incorporating optimized sUAV remote sensing at Pescara del Tronto. Geosciences 9(8), 332.
| Crossref | Google Scholar |
Gonzalez LF, Montes GA, Puig E, Johnson S, Mengersen K, Gaston KJ (2016) Unmanned aerial vehicles (UAVs) and artificial intelligence revolutionizing wildlife monitoring and conservation. Sensors 16(1), 97.
| Crossref | Google Scholar | PubMed |
Goodman LA (1961) Snowball sampling. The Annals of Mathematical Statistics 32, 148-170.
| Crossref | Google Scholar |
Greenwood F, Nelson EL, Greenough PG (2020) Flying into the hurricane: a case study of UAV use in damage assessment during the 2017 hurricanes in Texas and Florida. PLoS One 15(2), e0227808.
| Crossref | Google Scholar | PubMed |
Gupta SG, Ghonge D, Jawandhiya PM (2013) Review of unmanned aircraft system (UAS). International Journal of Advanced Research in Computer Engineering & Technology (IJARCET) 2, 13.
| Crossref | Google Scholar |
Kanand T, Kemper G, König R, Kemper H (2020) Wildfire detection and disaster monitoring system using UAS and sensor fusion technologies. The International Archives of the Photogrammetry, Remote Sensing and Spatial Information Sciences 43, 1671-1675.
| Crossref | Google Scholar |
Karma S, Zorba E, Pallis GC, Statheropoulos G, Balta I, Mikedi K, Vamvakari J, Pappa A, Chalaris M, Xanthopoulos G, Statheropoulos M (2015) Use of unmanned vehicles in search and rescue operations in forest fires: advantages and limitations observed in a field trial. International Journal of Disaster Risk Reduction 13, 307-312.
| Crossref | Google Scholar |
Koukouvelas IΚ, Nikolakopoulos KG, Zygouri V, Kyriou A (2020) Post-seismic monitoring of cliff mass wasting using an unmanned aerial vehicle and field data at Egremni, Lefkada Island, Greece. Geomorphology 367, 107306.
| Crossref | Google Scholar |
Krüll W, Tobera R, Willms I, Essen H, Von Wahl N (2012) Early forest fire detection and verification using optical smoke, gas and microwave sensors. Procedia Engineering 45, 584-594.
| Crossref | Google Scholar |
Lazzeri G, Frodella W, Rossi G, Moretti S (2021) Multitemporal Mapping of Post-Fire Land Cover Using Multiplatform PRISMA Hyperspectral and Sentinel-UAV Multispectral Data: Insights from Case Studies in Portugal and Italy. Sensors 21(12), 3982.
| Crossref | Google Scholar | PubMed |
Longhurst R (2009) Interviews: In-depth, semi-structured. In ‘International Encyclopedia of Human Geography’. (Eds R Kitchin, N Thrift) pp. 580–584. (Elsevier) 10.1016/B978-008044910-4.00458-2.
Mavroulis S, Andreadakis E, Spyrou NI, Antoniou V, Skourtsos E, Papadimitriou P, Kasssaras I, Kaviris G, Tselentis GA, Voulgaris N, Carydis P (2019) UAV and GIS based rapid earthquake-induced building damage assessment and methodology for EMS-98 isoseismal map drawing: The June 12, 2017 Mw 6.3 Lesvos (Northeastern Aegean, Greece) earthquake. International Journal of Disaster Risk Reduction 37, 101169.
| Crossref | Google Scholar |
Montgomery J, Wartman J, Reed AN, Gallant AP, Hutabarat D, Mason HB (2021) Field reconnaissance data from GEER investigation of the 2018 MW 7.5 Palu-Donggala earthquake. Data in Brief 34, 106742.
| Crossref | Google Scholar | PubMed |
Mueller SE, Thode AE, Margolis EQ, Yocom LL, Young JD, Iniguez JM (2020) Climate relationships with increasing wildfire in the southwestern US from 1984 to 2015. Forest Ecology and Management 460, 117861.
| Crossref | Google Scholar |
Murphy R (2015) Drones save lives in disasters, when they’re allowed to fly (op-ed), Space.com. Space. Available at https://www.space.com/30555-beginning-with-katrina-drones-save-lives-in-disasters.html [verified 19 April 2023]
Nedjati A, Vizvari B, Izbirak G (2016) Post-earthquake response by small UAV helicopters. Natural Hazards 80, 1669-1688.
| Crossref | Google Scholar |
Nurulloh UI, Khakhim N, Marfai MA (2020) Tsunami hazard mapping using UAV and geographic information system in Krakal Coastal Area, Gunungkidul, Indonesia. In ‘The 6th Geoinformation Science Symposium 2019’. p. 93. Available at https://www.researchgate.net/profile/Ayi-Priana/publication/368831195_The_Pattern_of_Spatial_Distribution_of_Agriculture_Drought_Using_Landsat_8_OLITIRS_in_Bacukiki_District_City_of_Parepare/links/63fc6a3a5749505945491737/The-Pattern-of-Spatial-Distribution-of-Agriculture-Drought-Using-Landsat-8-OLI-TIRS-in-Bacukiki-District-City-of-Parepare.pdf#page=103
Ojetunde B, Ano S, Sakano T (2022) A practical approach to deploying a drone-based message ferry in a disaster situation. Applied Sciences 12(13), 6547.
| Crossref | Google Scholar |
Oregon Office of Emergency Management (2020) The wildfire Setup. Available at: https://storymaps.arcgis.com/stories/6e1e42989d1b4beb809223d5430a3750 [verified 19 April 2023]
Oregon Office of Emergency Management (2021) 2020 Labor Day Wildfires After Action Report. Oregon Office of Emergency Management. Available at https://www.oregon.gov/oem/Documents/2020-Labor-Day-Wildfires-After-Action-Report.pdf [verified 19 April 2023]
Rahul K, Banyal RK (2020) Data life cycle management in big data analytics. Procedia Computer Science 173, 364-371.
| Crossref | Google Scholar |
Rathje EM, Franke K (2016) Remote sensing for geotechnical earthquake reconnaissance. Soil Dynamics and Earthquake Engineering 91, 304-316.
| Crossref | Google Scholar |
Restas A (2015) Drone applications for supporting disaster management. World Journal of Engineering and Technology 3(3), 316.
| Crossref | Google Scholar |
Rosser Jr JB, Parker BC, Vignesh V (2018) Medical applications of drones for disaster relief: a review of the literature. Surgical Technology International 33, 17-22.
| Google Scholar | PubMed |
Saikin DA, Baca T, Gurtner M, Saska M (2020) Wildfire fighting by unmanned aerial system exploiting its time-varying mass. IEEE Robotics and Automation Letters 5(2), 2674-2681.
| Crossref | Google Scholar |
Samiappan S, Hathcock L, Turnage G, McCraine C, Pitchford J, Moorhead R (2019) Remote sensing of wildfire using a small unmanned aerial system: post-fire mapping, vegetation recovery and damage analysis in Grand Bay, Mississippi/Alabama, USA. Drones 3(2), 43.
| Crossref | Google Scholar |
Schaefer M, Teeuw R, Day S, Zekkos D, Weber P, Meredith T, Van Westen CJ (2020) Low-cost UAV surveys of hurricane damage in Dominica: automated processing with co-registration of pre-hurricane imagery for change analysis. Natural Hazards 101(3), 755-784.
| Crossref | Google Scholar |
Sharma A, Singh PK (2021) UAV‐based framework for effective data analysis of forest fire detection using 5G networks: an effective approach towards smart cities solutions. International Journal of Communication Systems 34, e4826.
| Crossref | Google Scholar |
Shin JI, Seo WW, Kim T, Park J, Woo CS (2019) Using UAV multispectral images for classification of forest burn severity—A case study of the 2019 Gangneung forest fire. Forests 10(11), 1025.
| Crossref | Google Scholar |
Silvagni M, Tonoli A, Zenerino E, Chiaberge M (2017) Multipurpose UAV for search and rescue operations in mountain avalanche events. Geomatics, Natural Hazards and Risk 8(1), 18-33.
| Crossref | Google Scholar |
Sivasuriyan VK (2021) Drone usage and disaster management. Bodhi International Journal of Research in Humanities, Arts and Science 5, 93-97.
| Google Scholar |
Sun J, Li B, Jiang Y, Wen CY (2016) A camera-based target detection and positioning UAV system for search and rescue (SAR) purposes. Sensors 16(11), 1778.
| Crossref | Google Scholar |
Tang Z, Liu X, Chen H, Hupy J, Yang B (2020) Deep learning based wildfire event object detection from 4K aerial images acquired by UAS. AI 1(2), 166-179.
| Crossref | Google Scholar |
Tillamook Headlight Herald (2021) Study finds 2020 Labor Day fires significantly impacted Oregon’s forest sector. Tillamook Headlight Herald, 14 September. Available at https://www.tillamookheadlightherald.com/news/study-finds-2020-labor-day-fires-significantly-impacted-oregon-s-forest-sector/article_3d4d4652-10ca-11ec-96c4-672ef9dd0a98.html [verified 19 April 2023]
Valkaniotis S, Papathanassiou G, Ganas A (2018) Mapping an earthquake-induced landslide based on UAV imagery; case study of the 2015 Okeanos landslide, Lefkada, Greece. Engineering Geology 245, 141-152.
| Crossref | Google Scholar |
Wartman J, Berman JW, Bostrom A, Miles S, Olsen MJ, Gurley KR, Irish J, Lowes L, Tanner T, Dafni J, Grilliot M, Lyda A, Peltier J (2020) Research needs, challenges, and strategic approaches for natural hazards and disaster reconnaissance. Frontiers in Built Environment 6, 182.
| Crossref | Google Scholar |
Wickliffe LC, Riley KL, Morris JA Jr (2019) Spatial technologies for disaster assessment and emergency response for coastal aquaculture in the Gulf of mexico and Caribbean regions. In ‘Guidance on spatial technologies for disaster risk management in aquaculture: Full document’. (Eds J Aguilar-Manjarrez, LC Wickliffe and A Dean) pp 149–226. (FAO: Rome, Italy)
Wilson C (Eds) (2014) Semi-structured interviews. In ‘Interview techniques for UX practitioners’. pp. 23–41. 10.1016/B978-0-12-410393-1.00002-8
Wood RL, Gillins DT, Mohammadi ME, Javadnejad F, Tahami H, Gillins MN, Liao Y (2017) 2015 Gorkha post-earthquake reconnaissance of a historic village with micro unmanned aerial systems. In ‘Proc., 16th World Conference on Earthquake Engineering. Tokyo: International Association for Earthquake Engineering’. Available at https://www.wcee.nicee.org/wcee/article/16WCEE/WCEE2017-2917.pdf
Zhao Y, Ma J, Li X, Zhang J (2018) Saliency detection and deep learning-based wildfire identification in UAV imagery. Sensors 18(3), 712.
| Crossref | Google Scholar | PubMed |