Exploring the influence of the Keetch–Byram Drought Index and McArthur’s Drought Factor on wildfire incidence in Victoria, Australia
M. P. Plucinski




A CSIRO, GPO Box 1700, Canberra, ACT 2601, Australia.
B CSIRO Data61, Private Bag 10, Clayton South, Vic. 3169, Australia.
C Research and Development Team, Fire Risk, Research and Community Preparedness Department, Country Fire Authority, Burwood, Vic. 3151, Australia.
D Department of Energy, Environment and Climate Action, Data Insights, PO Box 500, East Melbourne, Vic. 8002, Australia.
Abstract
Wildfires are thought to become more prevalent during periods of extended dry weather. This issue is examined using two dryness metrics commonly applied in Australian fire management agencies.
This paper investigated links between wildfire incidence and the Keetch–Byram Drought Index (KBDI) and McArthur’s Drought Factor (DF) across the state of Victoria, Australia.
Weather records and data from 41 418 wildfires that occurred across the State over a 17-year period were compiled to examine the distributions of KBDI and DF on days with fires smaller and larger than 5 ha in area and all days, using kernel density plots.
Days with fires, particularly days with fires that escaped initial attack, have higher DFs and KBDIs compared with all days. These differences vary between regions and are greatest in areas with moist climates.
An appreciation of dryness conditions using tools such as KBDI and DF is useful for understanding fire potential, particularly in areas that experience higher and more regular rainfall.
Keywords: drought, Drought Factor, dryness, fire occurrence, fuel availability, ignition timing, Keetch–Byram Drought Index, moisture deficit.
Introduction
Wildfires and weather conditions conducive to their rapid spread are common during the summer period in Victoria, Australia (Long 2006; Harris et al. 2017), with many major fire events occurring in fire seasons experiencing severe rainfall deficit conditions (e.g. Esplin et al. 2003; McCarthy et al. 2012; Victorian Auditor General’s Office 2020). Droughts and dry periods tend to occur at regional scales, influencing the propensity of fires and fire spread across landscapes through the extensive drying of fuels, including coarser fractions such as logs and fallen branches, and areas that usually act as natural barriers to fire spread such as gullies and moist sheltered slopes (Bradstock et al. 2009). They can also lead to increases in fine fuel load in forest areas through the shedding of leaves from trees and shrubs (Ruthrof et al. 2016; Nolan et al. 2020). When dry periods extend over many weeks, they have a higher probability of coinciding with weather conditions that support rapid fire initiation and spread and challenge suppression efforts (Gill 1983). Many previous studies have linked droughts and dry weather with increased fire activity using metrics such as the number of fires and area burned (e.g. Meyn et al. 2010; Dimitrakopoulos et al. 2011; Chen 2022) and the incidence of large fires (e.g. Preisler et al. 2008; Bradstock et al. 2009; Barbero et al. 2015).
Two dryness metrics, the Keetch–Byram Drought Index (KBDI) (Keetch and Byram 1968) and McArthur’s Drought Factor (DF) (McArthur 1967), have been applied in Victoria and many other parts of Australia for over 50 years to track the effects of rainfall and drying conditions to estimate fire potential for fire management.
The KBDI was developed to provide a long-term indication of seasonal drying in the top (0.2 m) layers of soil in south-eastern USA. It is a simple bucket book-keeping model computed through the tracking of daily rainfall amounts and maximum temperatures to estimate the effect of daily evapo-transpiration increases and precipitation decreases on soil dryness. Its values sit on a hypothetical scale between 0 (saturated soil) and 200 (extreme drought, maximum soil moisture deficiency) that represent the amount of rainfall (in mm) required for the soil to become fully saturated (Sullivan 2001). KBDI values tend to increase slowly during dry periods but can drop sharply with rainfall events and can provide a good seasonal view of dryness (e.g. Janis et al. 2002; Finkele et al. 2006b).
The DF provides a daily indication of both the slowly varying long-term influences and the short-term effects of wetting of fine forest fuels to indicate their availability to burn (Sullivan 2001). It is calculated using time since the last rainfall event (up to 20 days), the quantity of rain and KBDI as inputs, and varies between 1 (wet) and 10 (fine fuel is fully available). As with KBDI, DF does not consider the influence of topography or different vegetation and soil types in its native form, although some specialised applications of it for fire spread simulators have adjustments for canopy density and topography (Duff et al. 2018). DF is a direct input into the Forest Fire Danger Index (FFDI) and the associated McArthur (1967) fire spread model, is used for the calculation of the Fire Behaviour Index within the new Australian Fire Danger Rating System for forest and pine fuels (Matthews 2022) and is used to estimate fuel availability in the Vesta Mk 2 forest fire model (Cruz et al. 2022).
There have been no previous investigations of links between these commonly applied dryness metrics and fire occurrence. This paper uses a state-wide dataset to compare the distribution of KBDI and DF on days with small fires and days with fires that burned more than 5 ha with their distributions for all days. Comparisons are made between districts with different climates and ignition rates.
Methods
Records from wildfire incidents occurring between July 2003 and June 2020 in the state of Victoria, Australia, were sourced from the Victorian Country Fire Authority and the Department of Energy, Environment and Climate Action (DEECA; known as the Department of Environment, Land, Water and Planning (DELWP) prior to 1 January 2023). Each fire record contained information on the time the fire was reported and the estimated location of its ignition point. These locations and dates were cross-referenced with spatial and meteorological datasets to provide information on vegetation type and prevailing weather, including dryness.
The compiled agency fire records did not include data on ignition cause or final fire size for most fires; however, a binomial fire size category was available for all fires. This was based on a threshold area burned of 5 ha, which is used as an initial attack success target by Victorian fire agencies (DELWP 2019) and was interpreted through categorical size class and final area burned data. Only a small proportion (4.6%) of fires were ≥5 ha. These were labelled escaped fires (as they had escaped initial attack efforts) and were investigated separately as they were the most likely to impact people and the environment.
Vegetation types for each incident were determined by cross-referencing fire locations with DEECA’s mapped vegetation layers. Fires in urban areas aligned with ‘urban’, ‘non-combustible’ and ‘no fuel’ vegetation types and were removed from the dataset as they were not considered to be representative of potential bushfires and may not have had the potential to become large areas. The remaining fires were categorised into two broad vegetation classes based on the application of McArthur’s Grassland (McArthur 1966) and Forest Fire Danger (McArthur 1967) indices. Fires were assigned to a grassland type (n = 27 093, 65.4%) for vegetation types including open grasslands, croplands, woodlands with grassy understories and similar vegetation, or were assigned to a forest type (n = 14 325, 34.6%) for vegetation types dominated by trees or shrubs such as open and closed forests, forestry plantations, woodlands, mallee, heathlands and other shrublands.
Fire timing and location data were linked to daily KBDI and DF values calculated from half-hourly records from a centrally located Bureau of Meteorology weather station within the same weather district. There are nine weather districts that span Victoria, with each encompassing an area with a fairly similar climatology (Fig. 1 and Table 1). KBDI and DF were calculated using the method of Finkele et al. (2006a, 2006b), using the equations presented in the Supplementary material. The KBDI and DF values assigned to days with fires are those for the day that a fire was first reported. Comparative KBDI and DF conditions for all days at the centrally located weather stations were also used to provide baseline representations of dryness for all days throughout the year within the study period (6210 days from 1 July 2003 to 30 June 2020) to provide an understanding of the distribution of soil dryness and fuel availability conditions experienced within each weather district.
The locations of weather districts across Victoria and the weather stations used within this study.
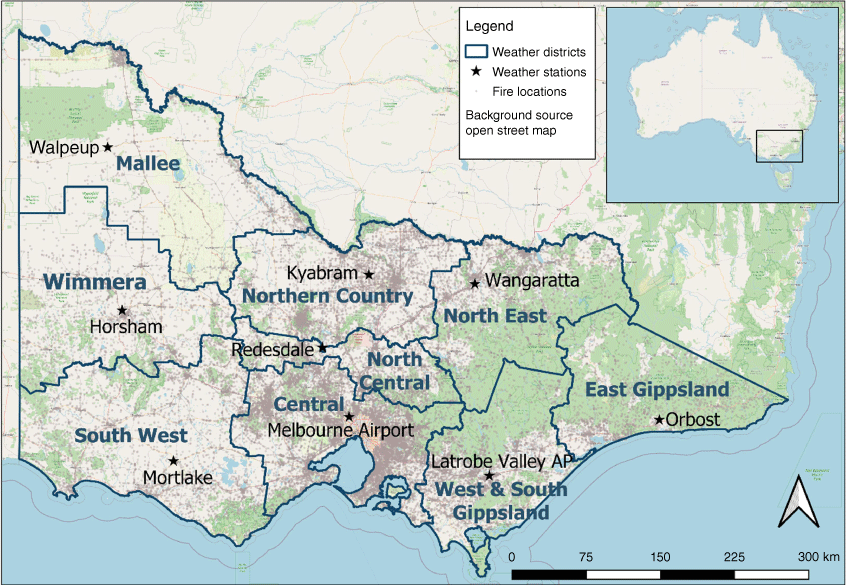
Weather district | Weather station | Mean annual rainfall (mm) | Mean monthly maximum temperature (°C) (min.–max.) | Number of fires (% of all) | Total number of days with fires (% of all days) | |||
---|---|---|---|---|---|---|---|---|
All | Small | Escaped | ||||||
Mallee | Walpeup | 306 | 23.9 (15–32.8) | 2265 | 2125 (93.8%) | 140 (6.2%) | 1615 (26%) | |
Northern Country | Kyabram | 436 | 22.3 (13.6–31.2) | 6857 | 6639 (96.8%) | 218 (3.2%) | 3184 (51.3%) | |
North East | Wangaratta | 587 | 22.3 (13.1–32.2) | 3899 | 3665 (94%) | 234 (6%) | 1988 (32%) | |
Wimmera | Horsham | 378 | 22.1 (13.7–31.3) | 2044 | 1734 (84.8%) | 310 (15.2%) | 1209 (19.5%) | |
North Central | Redesdale | 544 | 20.6 (12.1–29.8) | 2934 | 2818 (96%) | 116 (4%) | 1700 (27.4%) | |
East Gippsland | Orbost | 786 | 20.4 (15.1–26.2) | 2281 | 2034 (89.2%) | 247 (10.8%) | 1195 (19.2%) | |
South West | Mortlake | 590 | 19.3 (13–26.4) | 3696 | 3437 (93%) | 259 (7%) | 1928 (31%) | |
Central | Melbourne Airport | 516 | 20.2 (13.4–27) | 13 454 | 13 230 (98.3%) | 224 (1.7%) | 4130 (66.5%) | |
West and South Gippsland | Latrobe Valley Airport | 712 | 20.2 (13.7–27.1) | 3988 | 3820 (95.8%) | 168 (4.2%) | 2105 (33.9%) |
Distributions of KBDI and DF for all days within the study period and days with fires were explored using density plots. Density plots use a kernel function to provide smoothed distribution profiles of numeric variables. They provide similar information to a histogram but give a clearer indication of the shape of the distribution. These plots were prepared using the R statistical software framework (R Core Team 2023) (v4.2.1) using the ggplot2 package (v3.4.1) (Wickham 2016). The significance of differences in mean KBDI and DF on days with and without fires was assessed using Student’s t-test, with a null hypothesis of no mean difference in KBDI or DF between the days tested. The significance of differences between distributions was assessed using Kolmogorov–Smirnov tests.
Results
State-wide distributions of KBDI and DF on days with small fires and escaped fires and all days are presented in Fig. 2. These plots show that on most days, KDBI conditions are very low while DF values are mostly within the higher part of its range, indicating most days are affected by some short-term drying but are less influenced by longer-term drying. KBDI values were more likely to be low on days where small fires occurred and higher on days with escaped fires, with a maximum of ~60 (Fig. 2a). Days with escaped fires also tended to have higher DFs than those with small fires and all days (Fig. 2b). Very low KBDI was more common on days when grassfires occurred than when forest fires occurred (Fig. 2c), as demonstrated by Kolmogorov–Smirnov tests comparing the grassfire and forest fire distributions, with P values below 10−6. Mean KBDIs were higher for days with fires in forest fuel types than for days with grassfires.
Kernel density plots of the distributions of KBDI (a, c), and Drought Factor (b, d) associated with small (orange) and escaped (red) fires (a, b), and fires in forest (green) and grassland (brown) Fire Danger Index (FDI) fuel types (c, d) in comparison with KBDI and Drought Factor compiled using observations from a central weather station in each of the nine weather districts across Victoria during the 17-year study period.
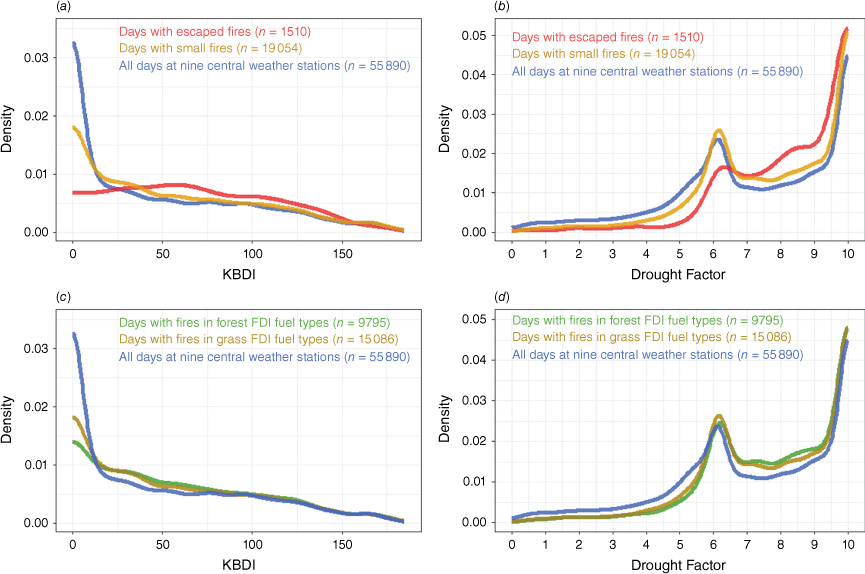
The profiles of DF (Fig. 2b, d) reveal a notable aberration in its distribution for groups with a local peak at ~6.2. This anomaly is an artefact of the DF calculation in which 6.2 is the maximum possible value that can be obtained when the KBDI is less than 20. This trait is the result of the equations used to calculate DF (Finkele et al. 2006a, 2006b), as detailed in the Supplementary material.
Comparisons of DF on days with KBDI less than and greater than 20 (Fig. 3) show that DFs below 5 are uncommon on days with KBDIs below and above 20 (39.3 and 7.7% respectively). Most (75.3%) days with fires have DFs between 5 and 6.2 when the KBDI is below 20. When the KBDI is more than 20, nearly all (92.8%) fires occur on days with DFs more than 6.2, with 39.6% of days with fires occurring when the DF is 9 or above.
Kernel density plots of the distributions of Drought Factor on days when KBDI < 20 (a, c), and ≥20 (b, d) showing abundance of days with small (orange) and escaped (red) fires (a, b), and fires in forest (green) and grassland (brown) Fire Danger Index (FDI) fuel types (c, d) using observations from a central weather station in each of the nine weather districts across Victoria during the 17-year study period. Note the different x-axis scales.
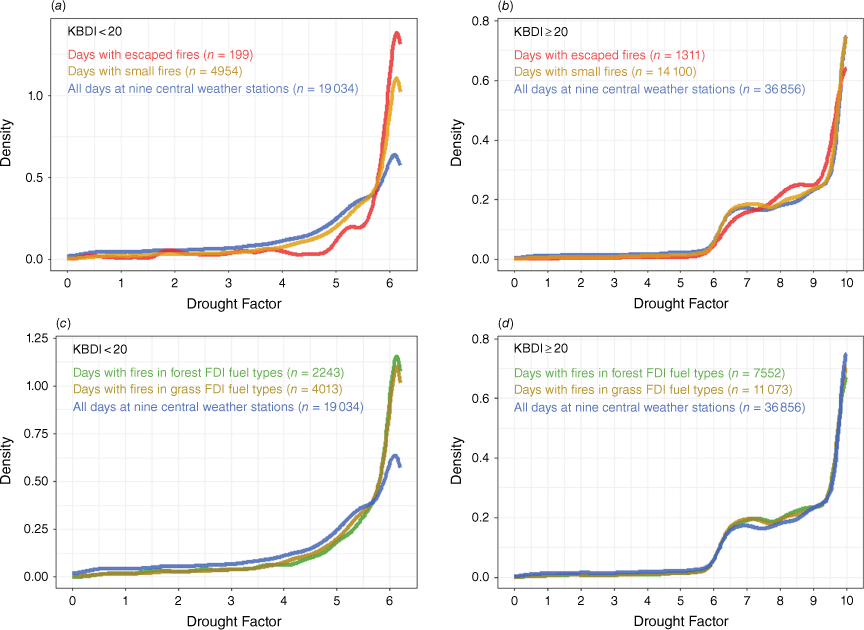
The nine weather districts experienced a range of weather conditions (Table 1) with the driest station (Walpeup, Mallee district) receiving less than half the annual rainfall of the wettest (Orbost, East Gippsland district). Fire loads and the number of days with fires also vary widely, with the weather district with the most fires (Central) having 6.6 times (13 454) the number of fires of the district with the least fires (Wimmera, 2044) (Table 1). These districts also have the smallest and highest proportion of escaped fires. The Central weather district has more than twice the number of fires of any other district. This district contains the most populated parts of the state and the high number of fires is likely to be due to the large population, and the small proportion of escaped fires may be a result of more effective suppression as a result of higher-density road networks and resource bases, as well as more efficient detection and milder weather conditions.
Distributions of KBDI vary considerably across the nine weather districts, with mean KBDI values for all days ranging from 23 in Central district to 115 in the Mallee district (Table 2). Notably, the two driest districts, Mallee and Wimmera, have KBDI distributions that show more frequent higher values than the other districts (Fig. 4). Fires seldom occur during low KBDI conditions in these two dry districts, with only 2.3% (40 of 1729) and 11.4% (138 of 1209) of days with fires having a KBDI of 20 or less. Days with fires had significantly higher KBDIs than those with no fires in all weather districts, with t-test P values below 10−6. The other districts have moister climates and KBDI values are very low on most days. In these districts, days with escaped fires were mostly distributed within higher KBDI ranges than for all days at the centrally located weather stations (Fig. 4). KBDI for days with small fires were mostly distributed between those for all days and days with escaped fires. Interestingly, the mean KBDI was higher on days with small fires than days with escaped fires in the Mallee and Wimmera weather districts (Table 2). Days with small fires were more common during very low KBDI conditions in the Central district than they were for any other district.
Weather district | Mean KBDI for all days (s.d.) [min.–max.] | Mean KBDI (s.d.) on days with: | ||||||
---|---|---|---|---|---|---|---|---|
No fires | Fires | Small fires | Escaped fires | Grass fuel fires | Forest fuel fires | |||
Mallee | 114.5 (42.9) [0–183.7] | 113 (43.1) | 120 (42.3)C | 120 (42.4) | 111 (42.1)A | 118 (43.2) | 125 (40)B | |
Northern Country | 69.5 (45.1) [0–184.7] | 59 (50) | 79 (48.6)C | 79 (48.6) | 86 (45.2)A | 79 (48.7) | 83 (46.6)A | |
North East | 57.8 (42) [0–175.5] | 50 (45.1) | 75 (45)C | 74 (45) | 94 (40.9)C | 75 (45.4) | 82 (43.2)C | |
Wimmera | 77.5 (38.6) [0–167.2] | 75 (45.9) | 86 (43.6)C | 87 (43.7) | 85 (39.5) | 85 (43.3) | 90 (41.5) | |
North Central | 44.5 (36.9) [0–147.3] | 38 (41.1) | 60 (41.3)C | 60 (41.5) | 75 (33.5)C | 61 (40.9) | 64 (41) | |
East Gippsland | 35.7 (31) [0–134.8] | 33 (33.5) | 46 (33.3)C | 46 (33.3) | 48 (31.5) | 43 (32.2) | 50 (32.7)C | |
South West | 36.2 (29) [0–139.8] | 29 (35) | 51 (36.9)C | 50 (36.8) | 61 (35.3)C | 51 (37) | 54 (36) | |
Central | 22.8 (19.3) [0–97.8] | 16 (20.3) | 25 (22.5)C | 25 (22.5) | 36 (23.6)C | 26 (22.6) | 28 (22.8)B | |
West and South Gippsland | 35.5 (31.1) [0–146.4] | 31 (34.9) | 43 (36.7)C | 43 (36.7) | 55 (38.9)C | 43 (36.5) | 48 (36.9)B |
Superscripts indicate significant differences (t-test) between mean KBDIs on days with no fire and fires, small fires and escaped fires, and grass and forest fires at the 0.05A, 0.01B and 0.001C levels.
Kernel density plots showing the distributions of KBDI for small (orange) and escaped (red) fires across the nine weather districts (a–i) in comparison with KBDI observations for all days (n = 6210) calculated from a centrally located weather station (blue lines, station name given in parentheses).
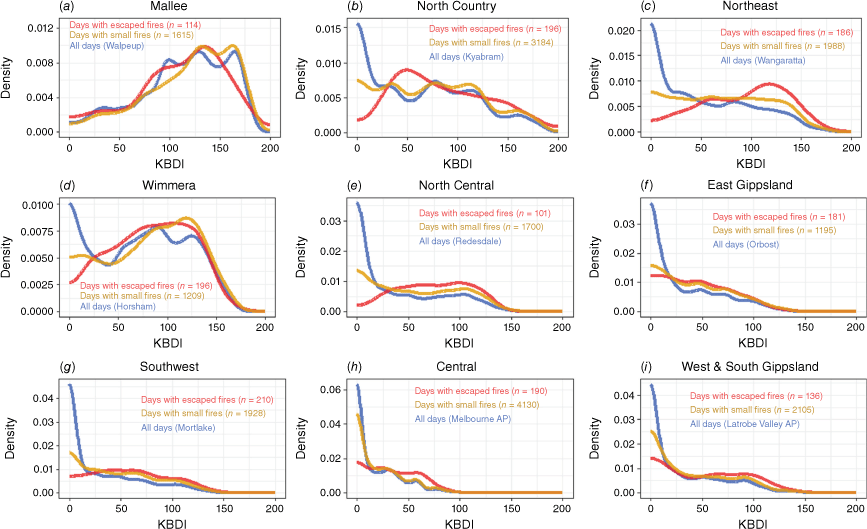
DF distributions were also quite varied between the weather districts with Mallee and Central regions again exhibiting the low and high extremes (Table 3). DF is near its maximum on nearly all days within the Mallee district, whereas this value is rarely reached in the Central district (Fig. 5). Other districts tended to have a significant proportion of days with high DFs, though not as extreme as the Mallee district. Days with escaped and small fires were more common when the DF was above the 6.2 peak than all days, with escaped fires being proportionally more common on days with the highest DFs except in the Mallee, North Country and Wimmera districts. DFs were significantly higher on days with fires than for days without fires, with t-test P values below 10−8 in all weather districts, indicating a link between fire occurrence and short-term rainfall deficits. Mean DFs were higher for days with escaped fires in all weather districts except for Wimmera, while differences in the Mallee weather district were not significant (Table 3).
Weather district | Mean DF for all days (s.d.) [min.–max.] | Mean DF (s.d.) on days with: | ||||||
---|---|---|---|---|---|---|---|---|
No fires | Fires | Small fires | Escaped fires | Grass fuel fires | Forest fuel fires | |||
Mallee | 8.9 (1.83) [0.1–10] | 8.8 (1.92) | 9.2 (1.55)C | 9.1 (1.56) | 9.2 (1.39) | 9.1 (1.58) | 9.3 (1.34)C | |
Northern Country | 7.6 (2.25) [0.1–10] | 7 (2.43) | 8.1 (1.92)C | 8.1 (1.92) | 8.4 (1.7)A | 8.1 (1.9) | 8.3 (1.79)C | |
North East | 6.9 (2.43) [0–10] | 6.4 (2.48) | 7.9 (2)C | 7.9 (2.01) | 8.7 (1.74)C | 7.9 (1.98) | 8.1 (1.97)C | |
Wimmera | 7.9 (2.09) [0.1–10] | 7.8 (2.15) | 8.5 (1.73)C | 8.5 (1.73) | 8.5 (1.58) | 8.5 (1.74) | 8.6 (1.68)C | |
North Central | 6.6 (2.34) [0–10] | 6.2 (2.35) | 7.5 (2.02)C | 7.5 (2.03) | 8.1 (1.88)B | 7.6 (2.01) | 7.7 (1.99)C | |
East Gippsland | 6 (2.35) [0–10] | 5.8 (2.4) | 7 (1.89)C | 7 (1.89) | 7.3 (1.7)A | 6.9 (1.81) | 7.2 (1.88)C | |
South West | 6.2 (2.2) [0.1–10] | 5.8 (2.2) | 7.3 (1.8)C | 7.3 (1.79) | 7.9 (1.55)C | 7.3 (1.78) | 7.5 (1.71)C | |
Central | 5.9 (1.76) [0.1–9.4] | 5.4 (1.87) | 6.2 (1.64)C | 6.2 (1.64) | 6.9 (1.5)C | 6.3 (1.62) | 6.4 (1.55)C | |
West and South Gippsland | 6 (2.29) [0.1–10] | 5.6 (2.32) | 6.8 (2.01)C | 6.8 (2.01) | 7.5 (1.85)C | 6.9 (1.98) | 7.1 (1.96)C |
Superscripts indicate significant differences (t-test) between mean DFs on days with no fire and fires, small fires and escaped fires, and grass and forest fires at the 0.05A, 0.01B and 0.001C levels.
Kernel density plots showing the distributions of Drought Factor for small (orange) and escaped (red) fires across the nine weather districts (a–i) in comparison with Drought Factor observations for all days (n = 6210) calculated from a centrally located weather station (blue lines, station name given in brackets).
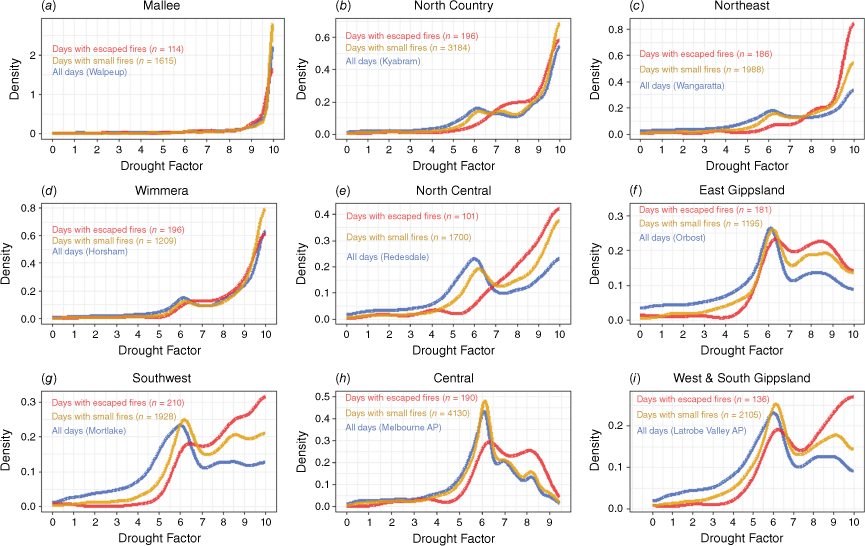
Discussion
The distributions of KBDI and DF show that days with fires, particularly with those with fires that escape initial attack, tend to have higher values of DF and KBDI compared with all days (Figs 4 and 5) and days without fires, with highly significant differences in their means in all weather districts (Tables 2 and 3). Differences in the DF distributions of days with fires and all days are more apparent in weather districts with wetter climates (Fig. 5). Differences in distributions of KBDI are more subtle, but it should be noted that its role as an input for the calculation of DF has a notable impact on how quickly high DF values can be attained (see Fig. 3 and the Supplementary material).
The plots presented here (Figs 2–5) show that there is more difference between the distributions of DF on days with fires and all days than there are of KBDI with these day types. The implications of this are that it need only take a drying event of a few weeks during a fire season to lead to a spike in fire ignitions, including fires that escape initial fire suppression efforts when the KBDI is moderately high (>20).
The use of DF and KBDI for estimating fuel dryness is strongly engrained within Australian fire practices; however, regional differences in their influence indicate the importance of local understanding in the interpretation of their value. Neither KBDI nor DF incorporate latitudinal differences in solar energy and evaporation or the influence of topography or vegetation cover. The plots show that KBDI and DF have less influence on fire occurrence in dry regions, such as the Mallee weather district (Figs 4a, 5a), as they exhibit less variability, and conditions are conducive to the ignition and spread of fires most of the time (Krusel et al. 1993). Krusel et al. (1993) also found there to be limited influence of KBDI on fire activity in this region and suggested that the occurrence of larger fires in this area may be linked to increased fuel continuity resulting from increased fuel growth because of wetter conditions over previous seasons. KBDI and DF have a more noticeable influence on fire occurrence in areas with moister climates as high values provide a strong indication of dryness anomalies. This observation is in agreement with those from previous research that noted greater links between drying events and the occurrence of large fires in humid areas than in areas with dry climates (O et al. 2020).
The influence that weather and rainfall deficits has on wildfire occurrence has important implications for the prediction of fire activity and impacts under current and future climates. Dry periods are frequent in Australia and are likely to become more frequent and severe in future (e.g. Kirono et al. 2020; Ukkola et al. 2020; Richardson et al. 2022). Predicted increases in temperature will result in faster drying between rainfall events, and potentially more fires as a result.
Future work on this topic should also seek a greater understanding of the influence of factors such as ignition cause and vegetation type and investigate the influence that preventative measures, such as fire restrictions, have on limiting ignition numbers. KBDI and DF are only two of many existing metrics that could be used for fire management. Alternative metrics, or locally scaled versions of KBDI and DF, may present more spatially consistent estimates of the effects of drying on fire potential. Understanding the effect that sub-diurnal change in fuel availability, through the moisture content of fine dead fuels, has on fire incidence would also be of value and could potentially also consider the role of ignition cause.
Conclusion
Days with fires have higher KBDI and DFs than all days. Days with fires that escape initial attack mostly have higher KBDI and DF values than days with small fires (Tables 2 and 3). Differences in the distributions of KBDI and DF on days with fires and all days were greatest in districts with moister climates. Predicted increases in the frequency and severity of dry periods are likely to result in greater fire potential (Richardson et al. 2021, 2022). Future research should be directed to gaining a greater understanding of this association with the aim of determining how and what fire prevention measures can mitigrate increased ignitions.
Data availability
The data used were provided by the Country Fire Authority and Department of Environment, Land, Water and Planning and were used under licence.
Acknowledgements
The authors are grateful for the assistance of Rachel Bessell and Tim Wells (Country Fire Authority), Rory McKenzie and Fin Roberts (Department of Energy, Environment and Climate Action). Andrew Sullivan (CSIRO) and the journal reviewers provided very helpful comments that significantly improved draft versions of the manuscript.
References
Barbero R, Abatzoglou JT, Kolden CA, Hegewisch KC, Larkin NK, Podschwit H (2015) Multi‐scalar influence of weather and climate on very large fires in the eastern United States. International Journal of Climatology 35, 2180-2186.
| Crossref | Google Scholar |
Bradstock RA, Cohn JS, Gill AM, Bedward M, Lucas C (2009) Prediction of the probability of large fires in the Sydney region of south-eastern Australia using fire weather. International Journal of Wildland Fire 18, 932-943.
| Crossref | Google Scholar |
Chen A (2022) Evaluating the relationships between wildfires and drought using machine learning. International Journal of Wildland Fire 31, 230-239.
| Crossref | Google Scholar |
Cruz MG, Cheney NP, Gould JS, McCaw WL, Kilinc M, Sullivan AL (2022) An empirical-based model for predicting the forward spread rate of wildfires in eucalypt forests. International Journal of Wildland Fire 31, 81-95.
| Crossref | Google Scholar |
Dimitrakopoulos AP, Vlahou M, Anagnostopoulou CG, Mitsopoulos ID (2011) Impact of drought on wildland fires in Greece: implications of climatic change? Climatic Change 109, 331-347.
| Crossref | Google Scholar |
Duff T, Cawson J, Cirulis B, Nyman P, Sheridan G, Tolhurst K (2018) Conditional performance evaluation: using wildfire observations for systematic fire simulator development. Forests 9, 189.
| Crossref | Google Scholar |
Finkele K, Mills GA, Beard G, Jones DA (2006b) National gridded drought factors and comparison of two soil moisture deficit formulations used in prediction of Forest Fire Danger Index in Australia. Australian Meteorological Magazine 55, 183-197.
| Google Scholar |
Gill AM (1983) Forest fire and drought in eastern Australia. In ‘Proceedings of colloquium on the significance of the Southern Oscillation Index and the need for comprehensive ocean monitoring system in Australia’, 27–28 July 1983, Canberra. pp. 161–185. (Australian Maritime Science Technical Advisory Committee)
Harris S, Mills G, Brown T (2017) Variability and drivers of extreme fire weather in fire-prone areas of south-eastern Australia. International Journal of Wildland Fire 26, 177-190.
| Crossref | Google Scholar |
Janis MJ, Johnson MB, Forthun G (2002) Near-real time mapping of Keetch-Byram drought index in the south-eastern United States. International Journal of Wildland Fire 11, 281-289.
| Crossref | Google Scholar |
Kirono DGC, Round V, Heady C, Chiew FHS, Osbrough S (2020) Drought projections for Australia: updated results and analysis of model simulations. Weather and Climate Extremes 30, 100280.
| Crossref | Google Scholar |
Krusel N, Packham D, Tapper N (1993) Wildfire activity in the mallee shrubland of Victoria, Australia. International Journal of Wildland Fire 3, 217-227.
| Crossref | Google Scholar |
Long M (2006) A climatology of extreme fire weather days in Victoria. Australian Meteorological Magazine 55, 13-18.
| Google Scholar |
Matthews S (2022) ‘Fire behaviour index technical guide.’ (Australasian Fire and Emergency Service Authorities Council (AFAC)) Available at https://www.afac.com.au/initiative/afdrs/article/fire-behaviour-index-technical-guide
McCarthy GJ, Plucinski MP, Gould JS (2012) Analysis of the resourcing and containment of multiple remote fires: the Great Divide Complex of fires, Victoria, December 2006. Australian Forestry 75, 54-63.
| Crossref | Google Scholar |
Meyn A, Taylor SW, Flannigan MD, Thonicke K, Cramer W (2010) Relationship between fire, climate oscillations, and drought in British Columbia, Canada, 1920–2000. Global Change Biology 16, 977-989.
| Crossref | Google Scholar |
Nolan RH, Blackman CJ, de Dios VR, Choat B, Medlyn BE, Li X, Bradstock RA, Boer MM (2020) Linking forest flammability and plant vulnerability to drought. Forests 11, 779.
| Crossref | Google Scholar |
O S, Hou X, Orth R (2020) Observational evidence of wildfire-promoting soil moisture anomalies. Scientific Reports 10, 11008.
| Crossref | Google Scholar |
Preisler HK, Chen SC, Fujioka F, Benoit JW, Westerling AL (2008) Wildland fire probabilities estimated from weather model-deduced monthly mean fire danger indices. International Journal of Wildland Fire 17, 305-316.
| Crossref | Google Scholar |
Richardson D, Black AS, Monselesan DP, Risbey JS, Squire DT, Tozer CR, Canadell JG (2021) Increased extreme fire weather occurrence in southeast Australia and related atmospheric drivers. Weather and Climate Extremes 34, 100397.
| Crossref | Google Scholar |
Richardson D, Black AS, Irving D, Matear RJ, Monselesan DP, Risbey JS, Squire DT, Tozer CR (2022) Global increase in wildfire potential from compound fire weather and drought. npj Climate and Atmospheric Science 5, 23.
| Crossref | Google Scholar |
Ruthrof KX, Fontaine JB, Matusick G, Breshears DD, Law DJ, Powell S, Hardy G (2016) How drought-induced forest die-off alters microclimate and increases fuel loadings and fire potentials. International Journal of Wildland Fire 25, 819-830.
| Crossref | Google Scholar |
Ukkola AM, De Kauwe MG, Roderick ML, Abramowitz G, Pitman AJ (2020) Robust future changes in meteorological drought in CMIP6 projections despite uncertainty in precipitation. Geophysical Research Letters 47, e2020GL087820.
| Crossref | Google Scholar |